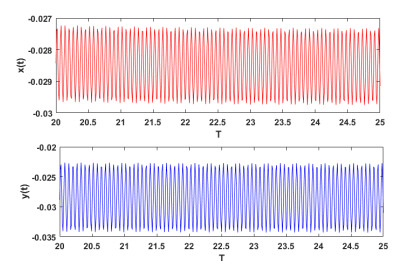
In this paper, a recurrent intermittent control (RIC) for the synchronization of fractional-order chaotic neural networks (FOCNNs) is proposed in view of the extended dissipativity-based approach. Successively, standard linear matrix inequalites (LMIs)-based extended dissipative criteria are derived through differential inclusions and inequality mechanisms. Several sufficient conditions are obtained to ensure the synchronization of FOCNNs. Furthermore, RIC is generated to solve the synchronization problem for the considered FOCNNs. Based on the piecewise Lyapunov functional, this paper derives a exponentially stable criterion in connection with linear matrix inequalities using the Matlab toolbox. Extended dissipativity can be employed to precisely define L2–L∞, H∞, passivity, and (Q,S,R)-ϑ dissipative performance. This is achieved by modifying the weighting matrices to achieve the desired performance level. The successful application of the stability criterion that was planned is demonstrated by the outcomes of the simulation.
Citation: Saravanan Shanmugam, R. Vadivel, S. Sabarathinam, P. Hammachukiattikul, Nallappan Gunasekaran. Enhancing synchronization criteria for fractional-order chaotic neural networks via intermittent control: an extended dissipativity approach[J]. Mathematical Modelling and Control, 2025, 5(1): 31-47. doi: 10.3934/mmc.2025003
[1] | M. Haripriya, A. Manivannan, S. Dhanasekar, S. Lakshmanan . Finite-time synchronization of delayed complex dynamical networks via sampled-data controller. Mathematical Modelling and Control, 2025, 5(1): 73-84. doi: 10.3934/mmc.2025006 |
[2] | Weisong Zhou, Kaihe Wang, Wei Zhu . Synchronization for discrete coupled fuzzy neural networks with uncertain information via observer-based impulsive control. Mathematical Modelling and Control, 2024, 4(1): 17-31. doi: 10.3934/mmc.2024003 |
[3] | Yao Chu, Xiuping Han, R. Rakkiyappan . Finite-time lag synchronization for two-layer complex networks with impulsive effects. Mathematical Modelling and Control, 2024, 4(1): 71-85. doi: 10.3934/mmc.2024007 |
[4] | Lusong Ding, Weiwei Sun . Neuro-adaptive finite-time control of fractional-order nonlinear systems with multiple objective constraints. Mathematical Modelling and Control, 2023, 3(4): 355-369. doi: 10.3934/mmc.2023029 |
[5] | Guoyi Li, Jun Wang, Kaibo Shi, Yiqian Tang . Some novel results for DNNs via relaxed Lyapunov functionals. Mathematical Modelling and Control, 2024, 4(1): 110-118. doi: 10.3934/mmc.2024010 |
[6] | Xianhao Zheng, Jun Wang, Kaibo Shi, Yiqian Tang, Jinde Cao . Novel stability criterion for DNNs via improved asymmetric LKF. Mathematical Modelling and Control, 2024, 4(3): 307-315. doi: 10.3934/mmc.2024025 |
[7] | Yuyang Zhao, Yang Liu . Output controllability and observability of mix-valued logic control networks. Mathematical Modelling and Control, 2021, 1(3): 145-156. doi: 10.3934/mmc.2021013 |
[8] | Gani Stamov, Ekaterina Gospodinova, Ivanka Stamova . Practical exponential stability with respect to $ h- $manifolds of discontinuous delayed Cohen–Grossberg neural networks with variable impulsive perturbations. Mathematical Modelling and Control, 2021, 1(1): 26-34. doi: 10.3934/mmc.2021003 |
[9] | Iman Malmir . Novel closed-loop controllers for fractional nonlinear quadratic systems. Mathematical Modelling and Control, 2023, 3(4): 345-354. doi: 10.3934/mmc.2023028 |
[10] | Biresh Kumar Dakua, Bibhuti Bhusan Pati . A frequency domain-based loop shaping procedure for the parameter estimation of the fractional-order tilt integral derivative controller. Mathematical Modelling and Control, 2024, 4(4): 374-389. doi: 10.3934/mmc.2024030 |
In this paper, a recurrent intermittent control (RIC) for the synchronization of fractional-order chaotic neural networks (FOCNNs) is proposed in view of the extended dissipativity-based approach. Successively, standard linear matrix inequalites (LMIs)-based extended dissipative criteria are derived through differential inclusions and inequality mechanisms. Several sufficient conditions are obtained to ensure the synchronization of FOCNNs. Furthermore, RIC is generated to solve the synchronization problem for the considered FOCNNs. Based on the piecewise Lyapunov functional, this paper derives a exponentially stable criterion in connection with linear matrix inequalities using the Matlab toolbox. Extended dissipativity can be employed to precisely define L2–L∞, H∞, passivity, and (Q,S,R)-ϑ dissipative performance. This is achieved by modifying the weighting matrices to achieve the desired performance level. The successful application of the stability criterion that was planned is demonstrated by the outcomes of the simulation.
In the past decades, neural networks (NNs) have been attracting significant interest from academics in recent decades due to their numerous uses in signal processing, pattern recognition, secure communication, and other related domains [1,2,3,4]. Unlike integer-order calculus, fractional-order (FO) calculus offers an invaluable resource for better characterizing memories and inheritance in a variety of materials and processes. Consequently, fractional calculus offers the important benefit of more accurately understanding a broad spectrum of instances in numerous fields and it also broadens the concept of mathematical differentiation and integration from an integer order to an arbitrary order [5,6,7]. Because they possess memory and non locality, fractional calculus algorithms have been used extensively in artificial NNs over the past few years [6,7,8,9]. The researchers of [10,11] used feedback control methodologies to tackle the matter of stability and stabilization problems for fractional-order neural networks (FOCNNs). The usage of FOCNNs has been emphasized in [12], which relies on discontinuous systems with indefinite Lyapunov-Krasovskii functionals.
Synchronization, a group of dynamic behaviors, was initially introduced to chaotic systems by Pecora and Carroll in 1990 [13]. Since then, it has become significant in numerous fields such as information processing, engineering, pattern recognition, and communication security [14,15,16,17]. The synchronization of nonlinear dynamic systems has been highly regarded by researchers across various disciplines. The examination of synchronization in FOCNNs has recently acquired significant attention and evolved into a prominent area of research. This is primarily the result of the wide range of potential uses in information science [17,18]. The fundamental impression of synchronization control is that one system employs a viable controller to achieve synchronization with another autonomous system. The advancement of studies has led to the utilization of multiple control methodologies in synchronization analysis, including adaptive control [19], quantized control [20], intermittent control (IC) [21], and pinning control [22]. The synchronization dynamics of FOCNNs have become a popular area of research. In this paper, we utilize a recurrent intermittent controller (RIC) in order to stabilize the FOCNNs under consideration. The time domain of interest is split into two distinct types of time intervals: the control interval and the rest interval. The exorbitant expenses linked to the complete monitoring of state measurements over time render continuous feedback methods of control, including control systems, impractical. Discontinuous methods of control, such as impulsive control (where the control inputs are activated at discrete times) and IC (where the control inputs are triggered at specific intervals), have earned the admiration of scholars by virtue of their broad investigations and many potential applications [23,24,25,26]. In the study conducted by [27], the authors investigated the synchronization of FOCNNs with reaction-diffusion terms using IC as a method. The researchers in [26] examined the synchronization of FO memristive recurrent NNs using RIC. Recently, the study of feedback and periodic IC-based finite-time synchronization of FOCNNs has been explored in [24].
Real dynamical systems frequently have unexpected behaviors due to a variety of factors, including linear approximation, modeling errors, and external disturbances, which further degrade the system. The problem of analyzing the effectiveness of a system with disturbance attenuation has been resolved through various methods [28,29,30]. Here, we discuss a few techniques, including H∞, L2–L∞, mixed H∞, passivity, and (Q,S,R)-ϑ dissipative performances. Extended dissipativity is a brand-new performance metric that in [31] established. All of the aforementioned performances are included in the expanded dissipativity notion by appropriately altering the weighting matrices. An extensive amount of research has been carried out on extended dissipativity analysis and control problems for a variety of different kinds of integer-order NNs and has been studied in recent works in the literature [32,33,34,35,36]. With regard to FOCNNS with uncertainty, [37] addressed the issue of extended dissipativity analysis. As far as the author knows, there have been no studies that have examined the synchronization criteria for FOCNNs via RIC in view of the extended dissipative approach.
Inspired by the preceding discussion, this article centers its attention on synchronization analysis and extended dissipativity approach for FOCNNs with RIC. The key contributions are outlined as follows:
(ⅰ) The investigation of FOCNNs has demonstrated synchronization for extended dissipativity, contributing to their dynamical characteristics and potential applications in various domains.
(ⅱ) We formulate the RIC specifically for the periodic aspect of the slave system. This controller ensures the desired performance of the error system and offers a novel methodology for examining various categories of extended dissipative conditions.
(ⅲ) By utilizing fractional-order inequalities, a suitable Lyapunov function, and various analytical techniques, sufficient criteria have been obtained in the form of linear matrix inequalities (LMIs) to ensure the exponentially stablity of the considered FOCNNs. Additionally, an extended dissipativity criterion has been derived. This approach provides a novel perspective for examining performance levels such as L2–L∞, H∞, passivity, and (Q,S,R)-ϑ dissipativity.
(ⅳ) The formulation of LMIs, which is a method that may be effectively executed through the use of the Matlab LMI toolbox, is ultimately what establishes the required conditions for FOCNNs. In order to validate the theoretical outputs, an illustrative case is utilized. Finally, the examples serve to substantiate the accessibility and practicability of the solutions that were derived.
Remark 1.1. In this work, we have studied a detailed characterization and visualization of chaotic behavior through phase portraits and time series analysis. In contrast, [21,25] concentrated on synchronization in fractional-order complex and memristive neural networks using intermittent and periodic intermittent control strategies, without exploring the chaotic dynamics of these systems. Similarly, [38] employed piecewise Lyapunov functions for intermittent control to synchronize fractional-order neural networks, primarily addressing synchronization rather than chaotic behavior. Meanwhile, the authors of [6] addressed the mean square asymptotic stability of discrete-time fractional-order stochastic neural networks with multiple time-varying delays, focusing on stability under stochastic influences rather than chaos. This paper uniquely contributes to the field by providing a comprehensive understanding and visualization of chaos in FOCNNs with an extended dissipative approach, which is not the primary focus of the other works, and outlines a path for future bifurcation analysis to further understand the complex dynamics of such systems.
Notation
For the purpose of this work, the following notations will be utilized:
(ⅰ) In the n-dimensional Euclidean space, the symbol Rn represents the collection of real numbers, encompassing the entire space.
(ⅱ) The notation λmax(A) denotes the largest eigenvalue of a real matrix A, while λmin(A) indicates the smallest eigenvalue. These values offer a thorough understanding of the spectral properties of the matrix A.
(ⅲ) A matrix P is considered to be positive definite, written as P>0, if and only if the quadratic form xTPx produces a positive value for all non zero vectors x. This feature is a fundamental concept in linear algebra and has important applications.
(ⅳ) In a matrix, the symmetric term is represented by notation ∗.
(ⅴ) Integers are represented by the Z.
(ⅵ) Natural numbers are represented by N.
(ⅶ) The notation C1[a,b] represents the collection of functions that have continuous first derivatives on the closed interval [a,b]. On the other hand, C[a,b] encompasses functions that are continuous on the same interval. These notations provide a mathematical framework for studying various degrees of differentiability and continuity in function spaces.
In this section, we present some definitions, and lemmas, and recall the well-known results of fractional differential equations.
Definition 2.1. [39] Let the fractional integral of order α describe the integral of a function x(t) concerning time t, which is referred to
Ct0D−αtx(t)=1Γ(α)∫tt0(t−s)α−1x(s)ds, |
where t≥t0 and α>0, and Γ(⋅) is the Gamma function.
Definition 2.2. [39] Let the Caputo fractional derivative be defined as the fractional derivative of order α for a function x(t) as follows:
Ct0Dαtx(t)=t0D−(n−α)t(dndtnx(t))=1Γ(n−α)∫tt0(t−s)n−α−1x(n)(τ)dτ, |
where n−1<α<n and n∈Z+. Specifically, when n=1 and 0<α<1, the Caputo fractional derivative is defined as:
Ct0Dαt=t0D−(1−α)t(ddtx(t))=1Γ(1−α)∫tt0x′(τ)(t−τ)αdτ. |
According to the definitions above, the integral derivative focuses on the nearby points of a function to calculate its derivative, while fractional derivatives, especially when using the Caputo approach, incorporate information from the entire course of a function, making them memory-dependent. The Caputo approach has the advantage of retaining the same format of the initial conditions as integer-order differential equations. It also gives a derivative of zero for constants. Therefore, it is a powerful tool for modeling complex systems with memory, such as FOCNNs. The notation Dα is chosen as the Caputo operator for the fractional derivative CaDαt.
Consider the drive NNs, which incorporate FOCNNs utilizing the Caputo fractional-order derivative
CaDαtx(t)=−Ax(t)+Bg(x(t)),ˆz(t)=Cx(t), | (2.1) |
with a range of 0<α<1; the notation C0Dαt represents the Caputo fractional derivative of order α. The state vector is represented by
x(t)=[x1(t),x2(t),…,xn(t)]T∈Rn. |
The resulting output vector is represented by ˆz∈Rn. Additional characterization of the influence of the system's dynamics is provided by the positive diagonal matrix A, which is written as
A=diag{a1,a2,…,an}. |
The connection weight matrix is B. In addition, the neuron activation function is expressed as
g(x(t))=[g1(x1(t)),g2(x2(t)),…,gn(xn(t))]T∈Rn. |
For FOCNNs (2.1), the corresponding response NNs are considered to be
CaDαty(t)=−Ay(t)+Bg(y(t))+Dω(t)+u(t),˜z(t)=Cy(t), | (2.2) |
where
y(t)=[y1(t),y2(t),…,yn(t)]T∈Rn |
is the state vector, ˜z in Rn is the output vector, ω(t)∈Rq is the disturbance associated with L2[0,∞), D is the known matrix, and u(t)∈Rn is the control input.
Assumption 2.1. For all x,y∈R, x≠y, the neuron activation functions gi(⋅), i=1,2,…,n, satisfy the following conditions:
l−i≤gi(x)−gi(y)x−y≤l+i, |
where l+i,l−i, and i=1,2,…,n are constants.
Assumption 2.2. The known real symmetric matrices ˜Ψ1–˜Ψ4 satisfy the following conditions:
1) ˜Ψ1=˜ΨT1≤0, ˜Ψ3=˜ΨT3>0, ˜Ψ4=˜ΨT4≥0,
2) (||˜Ψ1||+||˜Ψ2||)⋅||˜Ψ4||=0.
We assume that ϖ(t) and z(t) are the synchronization error between the drive system (2.1) and the response system (2.2) by specifying that
ϖ(t)=y(t)−x(t) |
and
z(t)=˜z(t)−ˆz(t).CaDαtϖ(t)=−Aϖ(t)+Bf(ϖ(t))+Dω(t)+u(t),z(t)=Cϖ(t), | (2.3) |
where
f(ϖ(t))=g(y(t))−g(x(t)). |
Assumption 2.1 states that
f(0)=0 |
and fi(ϖi(t)) fulfills the condition
l−i≤fi(ϖi(t))ϖi(t)≤l+i,∀ϖi(t)=0,i=1,2,…,n, | (2.4) |
where l−i and l+i (for i=1,2,…,n) are constants.
To facilitate synchronization between the drive system (2.1) and the response system (2.2), consider the following RIC, denoted by u(t)
u(t)={Kϖ(t),kT≤t<kT+ε,0,kT+ε≤t<(k+1)T. | (2.5) |
This control mechanism is designed to play an important role in the two systems (2.1) and (2.2). A compact form for the error system (2.3) can be utilized as follows:
CaDαtϖ(t)=−Aiϖ(t)+Bf(ϖ(t))+Dω(t), (i=1,2),z(t)=Cϖ(t), | (2.6) |
where,
A1=A+K, A2=A, a=kTanda=kT+ε. |
Definition 2.3. [31] The FOCNNs (2.3) are described as extended dissipative NNs if Assumption 2.2 is fulfilled for the input matrices ˜Ψ1–˜Ψ4. The following inequality applies if there are any Tf≥0 and any ω(t):
∫Tf0J(t)dt≥sup0≤t≤TfzT(t)˜Ψ4z(t), 0≤t≤Tf, | (2.7) |
where,
J(t)=zT(t)˜Ψ1z(t)+2zT(t)˜Ψ2ω(t)+ωT(t)˜Ψ3ω(t). |
Definition 2.4. [40] The system (2.6) is said to be exponentially stable with a convergence rate b>0 if a positive constant a exists such that
‖x(t)‖≤ae−b(t−t0)‖x(t0)‖,∀t≥t0. |
Lemma 2.1. [41] Assume that ϖ(t)∈Rn represents a vector of continuous and differentiable functions. For all time instances t≥t0, with t0 denoting a predetermined initial time, and for any 0<α≤1, the following inequality is satisfied:
12 Ct0Dαt(ϖT(t)Pϖ(t))≤ϖT(t)PCt0Dαtϖ(t). |
Lemma 2.2. [38] Given a function V(t) in C1[b,c), if
CbDαtV(t)≤λV(t), |
where 0<α<1, b≥0, c<+∞, and λ is a constant, then the inequality
V(t)≤V(b)exp(λΓ(α+1)(t−b)α) |
holds.
Remark 2.1. The definition of extended dissipativity for FOCNNs is obtained by transforming Definition 2.3, as shown in (2.7). This conceptual framework offers a generalization of well-established performance indices through the strategic specification of weighting matrices ˜Ψi (i=1,2,3,4). To further explain this idea, different performance index instances can be created by properly configuring the weighting matrices. Some examples include the following:
(1) To achieve H∞ performance, the weighting matrices are set as follows: ˜Ψ1=−I, ˜Ψ2=0, ˜Ψ3=ϑ2I, and ˜Ψ4=0. Consequently, (2.7) transforms into the representation of H∞ performance.
(2) For L2–L∞ performance, the weighting matrices are configured as: ˜Ψ1=0, ˜Ψ2=0, ˜Ψ3=ϑ2I, and ˜Ψ4=I. This configuration of matrices transforms (2.7) into the expression of L2–L∞ performance.
(3) Setting the weighting matrices as follows achieves passivity performance: ˜Ψ1=0, ˜Ψ2=I, ˜Ψ3=ϑI, and ˜Ψ4=0. In this case, (2.7) simplifies to represent passivity performance.
(4) To express (Q,S,R)-ϑ dissipativity performance, the weighting matrices are defined as ˜Ψ1=Q, ˜Ψ2=S, ˜Ψ3=R−ϑI, and ˜Ψ4=0. Subsequently, (2.7) characterizes (Q,S,R)-ϑ dissipativity performance.
These configurations show how the extended dissipativity framework can be customized to define performance metrics that meet different systems' requirements.
In this section, we establish a synchronization problem for a class of FOCNNs explored by designing RIC with an extended dissipativity criterion. To facilitate a more comprehensive discussion, we introduce the following notation:
L1=diag{l+1,l+2,…,l+n},L2=diag{l−1,l−2,…,l−n},L=diag{max{|l+1|,|l−1|},max{|l+2|,|l−2|},…,max{|l+n|,|l−n|}}=diag{l1,l2,…,ln}. |
Theorem 3.1. Assume that Assumption 2.1 holds. For the given scalars α>0, δ1>0, δ2>0, β>0, and ϑ>0 and the gain K>0, the matrices are ˜Ψ1, ˜Ψ2, ˜Ψ3, and ˜Ψ4, satisfying Assumption 2.2. If there are the positive definite matrices
P1=PT1>0, P2=PT20, |
and the diagonal matrices
M1≥0, M2≥0, W1≥0, W2≥0, |
the FOCNNs of (2.1) will exponentially synchronize with the FOCNNs of (2.2) with an extended dissipative approach with RIC (2.5) such that the following inequality holds:
Σ1=[ϕ11ϕ12ϕ13∗ϕ220∗∗ϕ33]<0, | (3.1) |
Σ2=[¯ϕ11¯ϕ12¯ϕ13∗¯ϕ220∗∗¯ϕ33]<0, | (3.2) |
[−PiCT˜Ψ4∗−˜Ψ4]≤0, i=1,2, | (3.3) |
Δ1εα−Δ2(T−ε)α−2lnβ>0, | (3.4) |
and
β=sup1≤i≠j≤2λmin(Pi)λmax(Pj),ϕ11=−P1A−ATPT1+P1K+KTPT1+δ1P1−2L1M1L2+LW1L−˜Ψ1,ϕ12=P1B+L1W1+L2W1, ϕ13=P1D−˜Ψ2,ϕ22=−2W2−M2, ϕ33=−˜Ψ3,¯ϕ11=−P2A−AP2+δ2P2−2L1M2L2+LW2L,¯ϕ12=P2B+L1W2+L2W2, ¯ϕ13=P2D−˜Ψ2,¯ϕ22=−2W2−M2, ¯ϕ33=−˜Ψ3. |
Proof. Choose the Lyapunov candidate as follows:
V(ϖ(t))={V1(ϖ(t)),kT≤t<kT+ε,V2(ϖ(t)),kT+ε≤t<(k+1)T, | (3.5) |
where
V1(ϖ(t))=ϖT(t)P1ϖ(t) |
and
V2(ϖ(t))=ϖT(t)P2ϖ(t),Vi(ϖ(t))≤βVj(ϖ(t)), |
where i,j∈{1,2} and
β=sup1≤i≠j≤2λmin(Pi)λmax(Pj). |
Thus, for i=1,2, we have
infi={1,2}λmin(Pi)||ϖ(t)||2≤V(ϖ(t))≤supi={1,2}λmax(Pi)||ϖ(t)||2. | (3.6) |
Considering Assumption 2.1 and (2.6), we can derive
0≤−2n∑j=1mij(fj(ϖj(t))−l+jϖj(t))(fj(ϖj(t))−l−jϖj(t))=−2(f(ϖ(t))−L1ϖ(t))TMi(f(ϖ(t))−L2ϖ(t)) | (3.7) |
and
0≤−n∑j=1wij(fj(ϖj(t))+ljϖj(t))(fj(ϖj(t))−ljϖj(t))=−[fT(ϖ(t))Wif(ϖ(t))−ϖT(t)LWiLϖ(t)], | (3.8) |
where
Wi=diag{wi1,wi2,...,win}≥0,Mi=diag{mi1,mi2,...,min}≥0, i=1,2. |
According to Lemma 2.1, when
kT≤t≤kT+ε, k=0,1,2,… |
and i=1, we take the derivative on V(ϖ(t)) and get the following:
CkTDαtV(ϖ(t))=CkTDαt(ϖT(t)P1ϖ(t))≤2ϖT(t)P1 CkTDαtϖ(t)=2ϖT(t)P1[(K−A)ϖ(t)+Bf(ϖ(t))+Dω(t)]. | (3.9) |
By applying (3.7) and (3.8) with i=1, we obtain the following:
CkTDαtV(ϖ(t))≤ϖT(t)[−P1A−ATPT1+P1K+KTPT1]ϖ(t)+2ϖT(t)P1Bf(ϖ(t))+2ϖT(t)P1Dω(t)−2(f(ϖ(t))−L1ϖ(t))TM1(f(ϖ(t))−L2ϖ(t))−(fT(ϖ(t))W1f(ϖ(t))−ϖT(t)LW1ϖ(t)=−δ1V(ϖ(t))+δ1ϖT(t)Pϖ(t)+ϖT(t)[−P1A−AP1+P1K+KTP1]ϖ+2ϖT(t)P1Bf(ϖ(t))+2ϖT(t)P1Dω(t)−2(f(ϖ(t))−L1ϖ(t))TM1(f(ϖ(t))−L2ϖ(t))−(fT(ϖ(t))W1f(ϖ(t))−ϖT(t)LW1Lϖ(t), |
From the above, the following inequality holds:
CkTDαtV(ϖ(t))−J(t)≤−δ1V(ϖ(t))+ξT(t)Σ1ξ(t)≤−δ1V(ϖ(t)), | (3.10) |
where
ξ(t)=[ϖ(t)f(ϖ(t))ω(t)]. |
Consequently
CkTDαtV(ϖ(t))≤−δ1V(ϖ(t)), kT≤t≤kT+ε. | (3.11) |
According to Lemma 2.2, within the time interval
kT≤t≤kT+ε |
for any k∈N, it can be inferred that
V(ϖ(t))≤V(ϖ(kT))exp(Δ1.(t−kT)α). | (3.12) |
Similary, when
kT+ε≤t≤(k+1)T, |
k=0,1,2,… and i=2.
By Lemma 2.1, for any ϖ(t)∈Rn, we have
CkT+εDαtV(ϖ(t))=CkT+εDαt(ϖT(t)P2ϖ(t))≤2ϖT(t)P2 CkT+εDαtϖ(t)=2ϖT(t)P2[−Aϖ(t)+Bf(ϖ(t))+Dω(t)]. | (3.13) |
According to (3.2), by using (3.7) and (3.8) with i=2, we can get
CkT+εDαtV(ϖ(t))≤ϖT(t)[−P2A−ATPT2]ϖ(t)+2ϖT(t)P2B×f(ϖ(t))+2ϖT(t)P2Dω(t)−2(f(ϖ(t))−L1ϖ(t))TM2(f(ϖ(t))−L2ϖ(t))−(fT(ϖ(t))W2f(ϖ(t))−ϖT(t)LW2ϖ(t)=δ2V(ϖ(t))−δ2ϖT(t)P2ϖ(t)+ϖT(t)(−P2A−AP2)ϖ(t)+2ϖT(t)P2Bf(ϖ(t))+2ϖT(t)P2Dω(t)−2(f(ϖ(t))−L1ϖ(t))TM2(f(ϖ(t))−L2ϖ(t))−(fT(ϖ(t))W2f(ϖ(t))−ϖT(t)LW2Lϖ(t). |
From the above results, the following inequality holds:
CkT+εDαtV(ϖ(t))−J(t)≤δ2V(ϖ(t))+ξT(t)Σ2ξ(t)≤δ2V(ϖ(t)). | (3.14) |
Thus, we have
CkT+εDαtV(ϖ(t))≤δ2V(ϖ(t)),kT+ε≤t<(k+1)T. | (3.15) |
By Lemma 2.2, it is implied that within the interval
KT+ε≤t<(k+1)T |
for any k∈N, the following holds:
V(ϖ(t))≤V(ϖ(kT+ϵ))exp(Δ2.(t−kT−ε)α). | (3.16) |
Thus, by inequalities (3.12) and (3.16), we can get V(ϖ(kT)+) and V(ϖ(kT)−) from
Vi(ϖ(kT))≤βVjϖ(kT) |
for k∈N, near the instant t=kT. Thus, we obtain
V(ϖ(kT)+)=ϖT((kT)+)P1ϖ(kT)+=ϖT(kT)P1ϖ(kT)≤βϖT(kT)P2ϖ(kT)=βϖT((kT)−)P2ϖ((kT)−)),V(ϖ(kT)+)≤βV(ϖ(kT)−). | (3.17) |
Similarly, we can consider
t=kT+ε, |
and we can then get
V(ϖ(kT+ε)+)≤βV(ϖ(kT+ε)−). | (3.18) |
Therefore, according to the equations above, for any k≥1, for
kT≤t<kT+ε, |
we obtain
V(ϖ(t))≤V(ϖ(t))exp(Δ1(t−kT)α)=V(ϖ(kT)+))exp(Δ1(t−kT)α)≤βV(ϖ(kT)−))exp(Δ1(t−kT)α)≤V(ϖ(0))exp(−kΔ1εα+kΔ2(T−ε)α+2klnβ)×exp(Δ1(t−kT)α), | (3.19) |
where
Δ1=−δ1Γ(α+1 |
and
Δ2=δ2Γ(α+1 |
and for
kT+ε≤t<(k+1)T, |
and
V(ϖ(t))≤V(ϖ(kT+ε))exp(Δ2(t−kT−ε)α)=V(ϖ(kT+ε)+))exp(Δ2(t−kT−ε)α)≤βV(ϖ(kT+ε)−))exp(Δ2(t−kT−ε)α)≤V(ϖ(0))exp(−(k+1)Δ1εα+kΔ2(T−ε)α+(2k+1)lnβ)exp(Δ2(t−kT−ε)α), | (3.20) |
Thus, from (3.4) and (3.19), one can see that for k≥1, when kT≤t<kT+ε,
V(ϖ(t))≤V(ϖ(0))exp(−kΔ1εα+kΔ2(T−ε)α+2klnβ)×exp(Δ1(t−kT)α)≤V(ϖ(0))exp(k(Δ1εα+Δ2(T−ε)α+2lnβ))≤V(ϖ(0))exp(Δ1εα+Δ2(T−ε)α+2lnβT(t−ε))≤V(ϖ(0))exp(−γ(t−ε)), | (3.21) |
where
γ=Δ1εα−Δ2(T−ε)α−2lnβT. |
By (3.4) and (3.20), when
kT+ε≤t<(k+1)T, |
we can get
V(ϖ(t))≤V(ϖ(0))exp(−(k+1)Δ1εα+kΔ2(T−ε)α+(2k+1)lnβ)exp(Δ2(t−kT−ε)α)≤V(ϖ(0))exp((k+1)Δ1εα+kΔ2(T−ε)α+2(k+1)lnβ)exp(Δ2(T−ε)α)=V(ϖ(0))exp((k+1)(Δ1εα+Δ2(T−ε)α+2lnβ))≤V(ϖ(0))exp(Δ1εα+Δ2(T−ε)α+2lnβTt)≤V(ϖ(0))exp(Δ1εα+Δ2(T−ε)α+2lnβT(t−ε))≤V(ϖ(0))exp(−γ(t−ε)). | (3.22) |
Examining (3.15)–(3.18), it becomes apparent that in the case of k=0, the conditions hold for 0≤t<ε,
V(ϖ(t))≤V(ϖ(0))exp(Δ1tα)≤V(ϖ(0)), | (3.23) |
and for ε≤t<T,
V(ϖ(t))≤βV(ϖ(0))exp(Δ2(t−ε)α)≤V(ϖ(0))exp(γε). | (3.24) |
Therefore, it can be deduced that the stated inequalities are applicable for any k∈N.
Therefore from the inequality (3.21) holds, t≥0 for the interval kT≤t≤kT+ε:
V(ϖ(t)≤V(ϖ(0))exp(−γ(t−ε)). | (3.25) |
This exponential decay property holds for any natural number k. Now consider a non-negative integer k∗ such that k∗T≤t<(k∗T+ε), equivalent to k in the established inequality. The conclusion is reached by generalizing this exponential decay property for any t within the interval k∗T≤t<(k∗T+ε)
V(ϖ(t)≤V(ϖ(0))exp(−γ(t−ε)). | (3.26) |
Similarly, from the inequality from (3.22) for the interval k∗T≤t<(k∗+1)T+ε
V(ϖ(t))≤V(ϖ(0))exp(−γ(t−ε)). | (3.27) |
From the (3.26) and (3.27) with the help of (3.5), we have
||ϖ(t)||≤√supi={1,2}λmax(Pi)infi={1,2}λmin(Pi)||ϖ(0)||exp(−ˆγ(t−ε)), | (3.28) |
where,
ˆγ=Δ1εα−Δ2(T−ε)α−2lnβ2T. |
On the basis of Definition 2.4, it can be concluded that the error system exhibits exponential stability. Next, we have to prove the extended dissipation.
From the error system (2.6), the intermittent control over the intervals
kT≤t≤kT+ε, |
and
kT+ε≤t≤(k+1)T |
is exponentially stable with
Σi<0, i=1,2. |
It is evident that under the conditions (3.1)–(3.4) holds, according to the Lyapunov stability theory. Then from the inequalities (3.10) and (3.14), we can conclude that
CaDαtV(ϖ(t))−J(t)≤0 |
for the intervals
kT≤t≤kT+ε |
and
kT+ε≤t≤(k+1)T. |
This implies the existence of a sufficiently small scalar ν>0,
CaDαtV(ϖ(t))−J(t)≤−ν||ϖ(t)||2. | (3.29) |
The inequalities (3.10) and (3.14) collectively establish the following evident relations:
V(ϖ(t))=ϖT(t)Piϖ(t) |
and
J(t)≥ CaDαtV(ϖ(t)). | (3.30) |
Now, by performing the integration of both sides of (3.30) over the interval from 0 to Tf, we arrive at the following inequalities based on the definitions provided:
∫Tf0J(t)dt≥ CaD−1Tf( CaDαTfV(ϖ(t)))= CaD−(1−α)TfV(ϖ(t))− CaD−(1−α)TfV(ϖ(0)). | (3.31) |
Since
V(ϖ(0))=0, |
we have
CaD−(1−α)TfV(ϖ(0))=1Γ(1−α)∫Tf0(Tf−s)−αV(ϖ(0))ds=V(ϖ(0)T1−αfΓ(2−α)=0. |
By fractional integration, we get
CaD−(1−α)TfV(ϖ(t))≥0. |
Thus, we have
∫Tf0J(t)dt≥0. | (3.32) |
According to Assumption 2.2, the Definition 2.3 and the matrices ˜Ψ1–˜Ψ4, the inequality that follows is valid:
∫Tf0J(t)dt−sup0≤t≤Tf{zT(t)˜Ψ4z(t)}≥0. | (3.33) |
Assumption 2.2 specifies the criteria for extended dissipativity as follows:
(ⅰ) When the H∞ performance, passivity, and strictly (Q,S,R)-ϑ dissipativity conditions are met, ˜Ψ4 equals zero
∫Tf0J(t)dt=sup0≤t≤TfzT(s)˜Ψ4z(s) |
for any Tf≥0.
(ⅱ) When ˜Ψ4>0, we obtain ˜Ψ1=0, ˜Ψ2=0, and ˜Ψ3>0 from Assumption 2.2. Then it can be shown that
∫t0J(s)ds≥V(ϖ(t))>0, | (3.34) |
and for any t≥0, Tf≥0, Tf≥t, 0≤t≤Tf, for all t∈[0,Tf], we have
∫Tf0J(s)ds>∫t0J(s)ds≥ϖT(t)Piϖ(t)>0. |
From (3.3), we get
zT(t)˜Ψ4z(t)=ϖT(t)CT˜Ψ4Cϖ(t). | (3.35) |
Using these inequalities, we get
∫Tf0J(s)ds≥ϖT(t)Piϖ(t)≥ϖT(t)CT˜Ψ4Cϖ(t). | (3.36) |
This ultimately leads to inequality
∫Tf0J(t)dt−sup0≤t≤TfzT(t)˜Ψ4z(t)≥0, | (3.37) |
the conditions in Definition 2.3 are satisfied, it is reasonable to conclude that the FOCNNs (2.3) are extended dissipative.
This completes the proof.
Theorem 3.2. Assuming that Assumption 2.1 is valid, and considering the scalars α>0, δ1>0, and δ2>0, with the matrices ˜Ψ1–˜Ψ4 satisfying Assumption 2.2, the synchronization of the drive system (2.1) and response system (2.2) under RIC (2.5) is established with
K=P−11Q. |
This synchronization holds true if we have the matrices
P1=PT1>0, P2=PT2>0, |
diagonal matrices
M1≥0, M2≥0, W1≥0andW2≥0, |
along with any matrix Q and a scalar β>0, satisfying the following inequalities:
¯Σ1=[ˆϕ11ϕ12ϕ13∗ϕ220∗∗ϕ33]<0, | (3.38) |
¯Σ2=[¯ϕ11¯ϕ12¯ϕ13∗¯ϕ220∗∗¯ϕ33]<0, | (3.39) |
[−PiCT˜Ψ4∗−˜Ψ4]≤0, i=1,2, | (3.40) |
Δ1εα−Δ2(T−ε)α−2lnβ>0, | (3.41) |
where,
β=sup1≤i≠j≤2λmin(Pi)λmax(Pj),ˆϕ11=−P1A−ATPT1+Q+QT+δ1P1−2L1M1L2+LW1L−˜Ψ1,ϕ12=P1B+L1W1+L2W1, ϕ13=P1D−˜Ψ2,ϕ22=−2W2−M2, ϕ33=−˜Ψ3,¯ϕ11=−P2A−AP2+δ2P2−2L1M2L2+LW2L,¯ϕ12=P2B+L1W2+L2W2, ¯ϕ13=P2D−˜Ψ2,¯ϕ22=−2W2−M2, ¯ϕ33=−˜Ψ3. |
Proof. Applying a similar proof technique as employed in Theorem 3.1, we can derive the desired LMIs given by Eqs (3.38)–(3.41).
Remark 3.1. Within most existing literature on the stability analysis of FOCNNs, this paper takes a unique approach to addressing exponentially stable and extended dissipativity. Instead of computing the integer-order derivative of the Lyapunov functional, we directly compute the fractional derivative of the proposed functional. This approach allows us to utilize the system's trajectory information more effectively. Note that for the Caputo derivative, the initial point is at 0. While some values of V(ϖ(t)) are negative, these values are restricted to the initial value range of the fractional differential equation. Therefore, these negative values do not impact the theoretical results.
Remark 3.2. In the error system (2.3), only the first node is actively controlled, and the control scheme implemented by the controller (2.5) follows a periodic pattern. Within each period, the timeline is divided into two segments: the operational phase
kT≤t<kT+ϵ |
and the resting phase
kT+ϵ≤t<(k+1)T. |
During the operational phase, the controller exerts control over the dynamic network described in (2.5), while remaining inactive during the resting phase. As a result of this periodic, intermittent control strategy, it becomes evident that the overall cost of control is effective.
In this section, we present numerical examples to demonstrate the effectiveness and practical implications of our results. These examples illustrate the performance and robustness of the proposed methods in different scenarios, complement the analytical aspects, and provide a comprehensive understanding of the synchronization approach.
Example 4.1. Consider the FOCNNS (2.3), which have the following fractional-order α=0.9, and take the parameters below:
A=[4.5004.5],B=[1.20−0.051],C=[−0.4−0.1[0.2cm]0.2−0.03],D=[0.1000.5]. |
Define the activation functions as
f(ϖ(t))=tanh(2ϖ(t)), |
where L1 is a diagonal matrix with 0 elements and L2 is a diagonal matrix with entries of 2. The disturbance input vector is ω(t)=sint. Additionally, two scalar values are introduced: δ1=5 and δ2=6. To assess the extended dissipative requirements for FOCNNs, we systematically explore the criteria of L2 – L∞ performance, passivity, H∞ performance, and (Q,S,R)–ϑ dissipativity in successive sections. Using these defined values, we compute the LMIs with a standard MATLAB LMI toolbox. The extended dissipative analysis of the system (2.3) incorporates the effectiveness of the weighting matrices ˜Ψ1–˜Ψ4, leading to the determination of ϑ=0.5. This exploration demonstrates the practical application of the proposed synchronization approach, linking theory with numerical demonstrations. Using specific values and standard tools makes the results clear and easy to replicate.
˜Ψ1=0, ˜Ψ2=0, ˜Ψ3=ϑI,and˜Ψ4=I. |
By applying the specified parameters, we can obtain the resulting gain by solving the LMIs of Theorem 3.2, utilizing the conventional MATLAB LMI toolbox.
K=[0.05640.00050.00600.0447]. |
˜Ψ1=−I, ˜Ψ2=0, ˜Ψ3=ϑI,and˜Ψ4=0. |
Estimating the LMIs in Theorem 3.2 is straightforward, and the resulting gain matrix is as follows:
K=[0.023000−0.3750]. |
Let us establish the following matrices:
˜Ψ1=0, ˜Ψ2=I, ˜Ψ3=ϑI,and˜Ψ4=0. |
With these matrices, the analysis of system (2.3) focuses on evaluating its passivity performance. In order to assess the feasibility of Theorem 3.2, we calculate the resulting benefit using the MATLAB LMI toolbox in the following manner,
K=[0.05830.00180.00770.1078]. |
Consider the matrices
˜Ψ1=Q, ˜Ψ2=S, ˜Ψ3=R−ϑI,and˜Ψ4=0, |
where
Q=[−100−1],S=[0.300.40.25],R=[0.3000.3]. |
Similarly, by solving the LMIs of Theorem 3.2 and applying the aforementioned parameters, the resultant gain matrices are as follows:
K=[0.0518−0.0000−0.00030.0422]. |
Figure 1 shows the oscillation of the state variables x(t) and y(t) in the error system of (2.3). The system evolves with time without controlling input.
Further, the existence of the limitation of oscillation with respect to the fractional order α is demonstrated. Figure 2 shows the fractional order in the range of
α∈[0.75,1.0]. |
The calculation obtains the maximum of the time series x(t) is observed (Poincaré cross-section; for periodic oscillation, we will get only one point). The fractional order varied in the x-axis and the maximum of the oscillations in the y-axis. The red colour shows the maxima of the oscillations.
From this figure, until
α=0.78 |
oscillation exists. If we tune the fractional order, further the system goes to a fixed point. No oscillation will exist after that. This is clearly visualized by the red line approaching zero.
Figure 3, illustrates the synchronization dynamics among the variables x1(t), x2(t), y1(t), and y2(t). Additionally, it displays the error dynamics between the drive and response systems, denoted as ϖ1(t) and ϖ2(t). The error systems are calculated in the L2–L∞ performance matrix. Similarly, other performances, such as H∞, passivity performance and (Q,S,R)-ϑ dissipativity, are calculated and plotted individually in Figure 4.
Example 4.2. On the basis of (2.1), the drive FOCNNs are represented as follows:
CaDαtx(t)=−Ax(t)+Bg(x(t)), | (4.1) |
where
A=[100010001],B=[2−1.201.81.711.15−4.7501.1], |
with
α=0.96, δ1=8andδ2=12. |
The activation functions are defined as:
g(x(t))=tanh(1.5x(t)). |
The function g(x(t)) satisfies Assumption 2.1 with
L=diag{1.5,1.5,1.5}. |
The initial value for the drive system is
x0=[−1.5,2,−0.8]T. |
On the basis of Eq (4.1), the response system can be described as follows:
CaDαty(t)=−Ay(t)+Bg(y(t))+Dω(t)+u(t), | (4.2) |
where
D=[−0.10−0.10.20.5−0.10.7−0.2−0.5]. |
Moreover, the noise disturbance is modeled as
ω(t)=e−0.1tsin(t). |
The system (4.1) is computed using a fractional algorithm with fixed initial conditions as (−1.5,2.0,−0.8). The system exhibits a chaotic attractor, as visually represented in Figure 5 in various projections. In Figure 5, the phase portraits and time evolution distinctly demonstrate the existence of chaotic oscillations. Notice that chaotic oscillation obtained here without control input.
In Figure 6, we present (a) three-dimensional phase diagrams of chaotic oscillation and Poincaré detection of the x1(max) variable, accompanied by its power spectrum. The Poincaré red circled dot is not periodic, and it confirms the presence of chaotic oscillations. Similarly, the power spectrum shows a broad band, which confirms the presence of chaotic oscillations. The power spectrum was computed using MATLAB with a sampling frequency of
Fs=1/T. |
The controller u(t) is specified as an RIC, as indicated in (2.5). Upon employing a suitable LMI solver to obtain a feasible numerical solution, the calculated value for β is 2.940. Following this, the derivation of the control gain matrix K is accomplished as outlined below:
K=[3.88730.0175−0.48340.04471.82740.1295−0.38610.13261.6198]. |
Similarly, for Example 2, the synchronization between the master and slave variables of x(t),y(t) and z(t) is shown in Figure 7; in the bottom panel, the error between the variables is calculated and plotted in a different color.
Remark 4.1. If we analyze the expressions for the convergence times T in (3.41) in Theorem 3.2, a significant difference emerges in the role of the control parameter β. This parameter exhibits variation among distinct control strategies, impacting the associated convergence times T as described in (3.41). A consistent observation across all intermittent control methods is that an augmentation in the value of β corresponds to a reduction in the inequality presented in (3.41), leading to expedited convergence rates.
This research investigates the synchronization challenges of FOCNNs with extended dissipativity performance. The integration of IC principles is employed to achieve synchronization by exerting control over the involved nodes. The essential conditions for achieving exponential stability in the error system, when utilizing a designed controller, are derived. These conditions are expressed in terms of LMIs through the construction of an appropriate Lyapunov functional. By incorporating the proposed stability criterion, a new set of conditions is derived, which prove to be sufficient for addressing issues related to L2–L∞, H∞, passivity, and (Q,S,R)-ϑ dissipative performance analyses. Finally, the effectiveness of the proposed strategy is validated through numerical illustrations. In the future work, the proposed method will be extended to more general multi-agent systems with actuator saturation, switching topologies, or network attack.
The authors declare they have not used Artificial Intelligence (AI) tools in the creation of this article.
The authors declare that there are no conflicts of interest in this paper.
[1] |
Z. Zhang, K. Yang, J. Qian, L. Zhang, Real-time surface emg pattern recognition for hand gestures based on an artificial neural network, Sensors, 19 (2019), 3170. https://doi.org/10.3390/s19143170 doi: 10.3390/s19143170
![]() |
[2] |
S. Shanmugam, R. Vadivel, N. Gunasekaran, Finite-time synchronization of quantized markovian-jump time-varying delayed neural networks via an event-triggered control scheme under actuator saturation, Mathematics, 11 (2023), 2257. https://doi.org/10.3390/math11102257 doi: 10.3390/math11102257
![]() |
[3] |
Y. Liu, Y. Zheng, J. Lu, J. Cao, L. Rutkowski, Constrained quaternion-variable convex optimization: a quaternion-valued recurrent neural network approach, IEEE Trans. Neural Networks Learn. Syst., 31 (2019), 1022–1035. https://doi.org/10.1109/TNNLS.2019.2916597 doi: 10.1109/TNNLS.2019.2916597
![]() |
[4] |
M. S. Ali, S. Saravanan, Finite-time stability for memristor based switched neural networks with time-varying delays via average dwell time approach, Neurocomputing, 275 (2018), 1637–1649. https://doi.org/10.1016/j.neucom.2017.10.003 doi: 10.1016/j.neucom.2017.10.003
![]() |
[5] |
C. Aouiti, J. Cao, H. Jallouli, C. Huang, Finite-time stabilization for fractional-order inertial neural networks with time-varying delays, Nonlinear Anal. Model., 27 (2022), 1–18. https://doi.org/10.15388/namc.2022.27.25184 doi: 10.15388/namc.2022.27.25184
![]() |
[6] |
D. Yang, Y. Yu, W. Hu, X. Yuan, G. Ren, Mean square asymptotic stability of discrete-time fractional order stochastic neural networks with multiple time-varying delays, Neural Process. Lett., 55 (2023), 9247–9268. https://doi.org/10.1007/s11063-023-11200-9 doi: 10.1007/s11063-023-11200-9
![]() |
[7] |
C. A. Popa, Mittag-leffler stability and synchronization of neutral-type fractional-order neural networks with leakage delay and mixed delays, J. Franklin Inst., 360 (2023), 327–355. https://doi.org/10.1016/j.jfranklin.2022.11.011 doi: 10.1016/j.jfranklin.2022.11.011
![]() |
[8] |
J. Cao, G. Stamov, I. Stamova, S. Simeonov, Almost periodicity in impulsive fractional-order reaction–diffusion neural networks with time-varying delays, IEEE Trans. Cybern., 51 (2020), 151–161. https://doi.org/10.1109/TCYB.2020.2967625 doi: 10.1109/TCYB.2020.2967625
![]() |
[9] |
N. H. Sau, M. V. Thuan, N. T. T. Huyen, Passivity analysis of fractional-order neural networks with time-varying delay based on lmi approach, Circuits Syst. Signal Process., 39 (2020), 5906–5925. https://doi.org/10.1007/s00034-020-01450-6 doi: 10.1007/s00034-020-01450-6
![]() |
[10] |
H. Wu, X. Zhang, S. Xue, L. Wang, Y. Wang, Lmi conditions to global mittag-leffler stability of fractional-order neural networks with impulses, Neurocomputing, 193 (2016), 148–154. https://doi.org/10.1016/j.neucom.2016.02.002 doi: 10.1016/j.neucom.2016.02.002
![]() |
[11] |
L. Chen, Y. Chai, R. Wu, T. Ma, H. Zhai, Dynamic analysis of a class of fractional-order neural networks with delay, Neurocomputing, 111 (2013), 190–194. https://doi.org/10.1016/j.neucom.2012.11.034 doi: 10.1016/j.neucom.2012.11.034
![]() |
[12] |
K. Udhayakumar, F. A. Rihan, R. Rakkiyappan, J. Cao, Fractional-order discontinuous systems with indefinite LKFs: an application to fractional-order neural networks with time delays, Neural Networks, 145 (2022), 319–330. https://doi.org/10.1016/j.neunet.2021.10.027 doi: 10.1016/j.neunet.2021.10.027
![]() |
[13] |
L. M. Pecora, T. L. Carroll, Synchronization in chaotic systems, Phys. Rev. Lett., 64 (1990), 821. https://doi.org/10.1103/PhysRevLett.64.821 doi: 10.1103/PhysRevLett.64.821
![]() |
[14] | C. Ge, X. Liu, Y. Liu, C. Hua, Event-triggered exponential synchronization of the switched neural networks with frequent asynchronism, IEEE Trans. Neural Networks Learn. Syst., 2022. https://doi.org/10.1109/TNNLS.2022.3185098 |
[15] |
E. Vassilieva, G. Pinto, J. de Barros, P. Suppes, Learning pattern recognition through quasi-synchronization of phase oscillators, IEEE Trans. Neural Networks, 22 (2010), 84–95. https://doi.org/10.1109/TNN.2010.2086476 doi: 10.1109/TNN.2010.2086476
![]() |
[16] |
A. A. Koronovskii, O. I. Moskalenko, A. E. Hramov, On the use of chaotic synchronization for secure communication, Phys. Usp., 52 (2009), 1213–1238. https://doi.org/10.3367/UFNe.0179.200912c.1281 doi: 10.3367/UFNe.0179.200912c.1281
![]() |
[17] |
X. Yang, X. Wan, C. Zunshui, J. Cao, Y. Liu, L. Rutkowski, Synchronization of switched discrete-time neural networks via quantized output control with actuator fault, IEEE Trans. Neural Networks Learn. Syst., 32 (2020), 4191–4201. https://doi.org/10.1109/TNNLS.2020.3017171 doi: 10.1109/TNNLS.2020.3017171
![]() |
[18] |
J. Xiao, J. Cao, J. Cheng, S. Zhong, S. Wen, Novel methods to finite-time mittag-leffler synchronization problem of fractional-order quaternion-valued neural networks, Inf. Sci., 526 (2020), 221–244. https://doi.org/10.1016/j.ins.2020.03.101 doi: 10.1016/j.ins.2020.03.101
![]() |
[19] |
S. Kumar, A. E. Matouk, H. Chaudhary, S. Kant, Control and synchronization of fractional-order chaotic satellite systems using feedback and adaptive control techniques, Int. J. Adapt Control Signal Process., 35 (2021), 484–497. https://doi.org/10.1002/acs.3207 doi: 10.1002/acs.3207
![]() |
[20] |
R. Tang, H. Su, Y. Zou, X. Yang, Finite-time synchronization of markovian coupled neural networks with delays via intermittent quantized control: linear programming approach, IEEE Trans. Neural Networks Learn. Syst., 33 (2021), 5268–5278. https://doi.org/10.1109/TNNLS.2021.3069926 doi: 10.1109/TNNLS.2021.3069926
![]() |
[21] |
L. Zhang, J. Zhong, J. Lu, Intermittent control for finite-time synchronization of fractional-order complex networks, Neural Networks, 144 (2021), 11–20. https://doi.org/10.1016/j.neunet.2021.08.004 doi: 10.1016/j.neunet.2021.08.004
![]() |
[22] |
S. Wang, Z. Zhang, C. Lin, J. Chen, Fixed-time synchronization for complex-valued bam neural networks with time-varying delays via pinning control and adaptive pinning control, Chaos Solitons Fract., 153 (2021), 111583. https://doi.org/10.1016/j.chaos.2021.111583 doi: 10.1016/j.chaos.2021.111583
![]() |
[23] |
X. Han, F. Cheng, S. Tang, Y. Zhang, Y. Fu, W. Cheng, et al., Synchronization analysis of fractional-order neural networks with adaptive intermittent-active control, IEEE Access, 10 (2022), 75097–75104. https://doi.org/10.1109/ACCESS.2022.3191801 doi: 10.1109/ACCESS.2022.3191801
![]() |
[24] |
M. Hui, C. Wei, J. Zhang, H. H. C. Iu, R. Yao, L. Bai, Finite-time synchronization of fractional-order memristive neural networks via feedback and periodically intermittent control, Commun. Nonlinear Sci. Numer. Simul., 116 (2023), 106822. https://doi.org/10.1016/j.cnsns.2022.106822 doi: 10.1016/j.cnsns.2022.106822
![]() |
[25] |
L. Zhang, Y. Yang, F. Wang, Lag synchronization for fractional-order memristive neural networks via period intermittent control, Nonlinear Dyn., 89 (2017), 367–381. https://doi.org/10.1007/s11071-017-3459-4 doi: 10.1007/s11071-017-3459-4
![]() |
[26] |
S. Zhang, Y. Yang, X. Sui, Y. Zhang, Synchronization of fractional-order memristive recurrent neural networks via aperiodically intermittent control, Math. Biosci. Eng, 19 (2022), 11717–11734. https://doi.org/10.3934/mbe.2022545 doi: 10.3934/mbe.2022545
![]() |
[27] |
Y. Xu, F. Sun, W. Li, Exponential synchronization of fractional-order multilayer coupled neural networks with reaction-diffusion terms via intermittent control, Neural Comput. Appl., 33 (2021), 16019–16032. https://doi.org/10.1007/s00521-021-06214-0 doi: 10.1007/s00521-021-06214-0
![]() |
[28] |
H. Shen, Y. Zhu, L. Zhang, J. H. Park, Extended dissipative state estimation for markov jump neural networks with unreliable links, IEEE Trans. Neural Networks Learn. Syst., 28 (2016), 346–358. https://doi.org/10.1109/TNNLS.2015.2511196 doi: 10.1109/TNNLS.2015.2511196
![]() |
[29] |
S. Shanmugam, R. Vadivel, M. Rhaima, H. Ghoudi, Improved results on an extended dissipative analysis of neural networks with additive time-varying delays using auxiliary function-based integral inequalities, AIMS Math., 8 (2023), 21221–21245. https://doi.org/10.3934/math.20231082 doi: 10.3934/math.20231082
![]() |
[30] |
R. Anbuvithya, S. D. Sri, R. Vadivel, N. Gunasekaran, P. Hammachukiattikul, Extended dissipativity and non-fragile synchronization for recurrent neural networks with multiple time-varying delays via sampled-data control, IEEE Access, 9 (2021), 31454–31466. https://doi.org/10.1109/ACCESS.2021.3060044 doi: 10.1109/ACCESS.2021.3060044
![]() |
[31] |
B. Zhang, W. X. Zheng, S. Xu, Filtering of markovian jump delay systems based on a new performance index, IEEE Trans. Circuits Syst. I, 60 (2013), 1250–1263. https://doi.org/10.1109/TCSI.2013.2246213 doi: 10.1109/TCSI.2013.2246213
![]() |
[32] |
R. Vadivel, P. Hammachukiattikul, S. Vinoth, K. Chaisena, N. Gunasekaran, An extended dissipative analysis of fractional-order fuzzy networked control systems, Fractal Fract., 6 (2022), 591. https://doi.org/10.3390/fractalfract6100591 doi: 10.3390/fractalfract6100591
![]() |
[33] |
T. N. Tuan, N. T. Thanh, M. V. Thuan, New results on robust finite-time extended dissipativity for uncertain fractional-order neural networks, Neural Process. Lett., 55 (2023), 9635–9650. https://doi.org/10.1007/s11063-023-11218-z doi: 10.1007/s11063-023-11218-z
![]() |
[34] |
X. Sun, X. Song, Dissipative analysis for fractional-order complex-valued reaction–diffusion neural networks, 2022 13th Asian Control Conference (ASCC), 2022,269–273. https://doi.org/10.23919/ASCC56756.2022.9828311 doi: 10.23919/ASCC56756.2022.9828311
![]() |
[35] |
D. T. Hong, N. H. Sau, M. V. Thuan, New criteria for dissipativity analysis of fractional-order static neural networks, Circuits Syst. Signal Process., 41 (2022), 2221–2243. https://doi.org/10.1007/s00034-021-01888-2 doi: 10.1007/s00034-021-01888-2
![]() |
[36] |
N. T. Phuong, N. T. T. Huyen, N. T. H. Thu, N. H. Sau, M. V. Thuan, New criteria for dissipativity analysis of caputo fractional-order neural networks with non-differentiable time-varying delays, Int. J. Nonlinear Sci. Numer. Simul., 2022. https://doi.org/10.1515/ijnsns-2021-0203 doi: 10.1515/ijnsns-2021-0203
![]() |
[37] |
M. Shafiya, G. Nagamani, Extended dissipativity criterion for fractional-order neural networks with time-varying parameter and interval uncertainties, Comput. Appl. Math., 41 (2022), 95. https://doi.org/10.1007/s40314-022-01799-1 doi: 10.1007/s40314-022-01799-1
![]() |
[38] |
Y. Yang, Y. He, M. Wu, Intermittent control strategy for synchronization of fractional-order neural networks via piecewise lyapunov function method, J. Franklin Inst., 356 (2019), 4648–4676. https://doi.org/10.1016/j.jfranklin.2018.12.020 doi: 10.1016/j.jfranklin.2018.12.020
![]() |
[39] | I. Podlubny, Fractional differential equations: an introduction to fractional derivatives, fractional differential equations, to methods of their solution and some of their applications, Elsevier, 1999. https://doi.org/10.1016/s0076-5392(99)x8001-5 |
[40] |
N. Gunasekaran, G. Zhai, Q. Yu, Exponential sampled-data fuzzy stabilization of nonlinear systems and its application to basic buck converters, IET Control Theory Appl., 15 (2021), 1157–1168. https://doi.org/10.1049/cth2.12113 doi: 10.1049/cth2.12113
![]() |
[41] |
N. Aguila-Camacho, M. A. Duarte-Mermoud, J. A. Gallegos, Lyapunov functions for fractional order systems, Commun. Nonlinear Sci. Numer. Simul., 19 (2014), 2951–2957. https://doi.org/10.1016/j.cnsns.2014.01.022 doi: 10.1016/j.cnsns.2014.01.022
![]() |