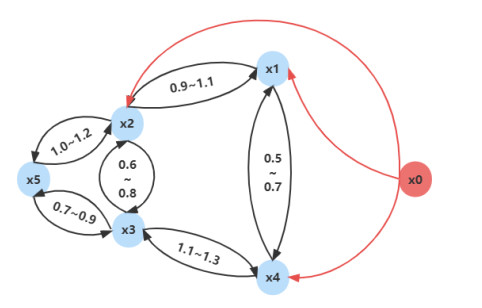
This paper discussed the synchronization of impulsive fuzzy neural networks (FNNs) with uncertainty of information exchange. Since the data of neural networks (NNs) cannot be completely measured in reality, we designed an observer-based impulsive controller on the basis of the partial measurement results and achieved the purpose of reducing the communication load and the controller load of FNNs. In terms of the Lyapunov stability theory, an impulsive augmented error system (IAES) was established and two sufficient criteria to guarantee the synchronization of our FNNs system were obtained. Finally, we demonstrated the validity of the results by a numerical example.
Citation: Weisong Zhou, Kaihe Wang, Wei Zhu. Synchronization for discrete coupled fuzzy neural networks with uncertain information via observer-based impulsive control[J]. Mathematical Modelling and Control, 2024, 4(1): 17-31. doi: 10.3934/mmc.2024003
[1] | Yao Chu, Xiuping Han, R. Rakkiyappan . Finite-time lag synchronization for two-layer complex networks with impulsive effects. Mathematical Modelling and Control, 2024, 4(1): 71-85. doi: 10.3934/mmc.2024007 |
[2] | Gani Stamov, Ekaterina Gospodinova, Ivanka Stamova . Practical exponential stability with respect to $ h- $manifolds of discontinuous delayed Cohen–Grossberg neural networks with variable impulsive perturbations. Mathematical Modelling and Control, 2021, 1(1): 26-34. doi: 10.3934/mmc.2021003 |
[3] | M. Haripriya, A. Manivannan, S. Dhanasekar, S. Lakshmanan . Finite-time synchronization of delayed complex dynamical networks via sampled-data controller. Mathematical Modelling and Control, 2025, 5(1): 73-84. doi: 10.3934/mmc.2025006 |
[4] | Lusong Ding, Weiwei Sun . Neuro-adaptive finite-time control of fractional-order nonlinear systems with multiple objective constraints. Mathematical Modelling and Control, 2023, 3(4): 355-369. doi: 10.3934/mmc.2023029 |
[5] | Saravanan Shanmugam, R. Vadivel, S. Sabarathinam, P. Hammachukiattikul, Nallappan Gunasekaran . Enhancing synchronization criteria for fractional-order chaotic neural networks via intermittent control: an extended dissipativity approach. Mathematical Modelling and Control, 2025, 5(1): 31-47. doi: 10.3934/mmc.2025003 |
[6] | Hongwei Zheng, Yujuan Tian . Exponential stability of time-delay systems with highly nonlinear impulses involving delays. Mathematical Modelling and Control, 2025, 5(1): 103-120. doi: 10.3934/mmc.2025008 |
[7] | Yanchao He, Yuzhen Bai . Finite-time stability and applications of positive switched linear delayed impulsive systems. Mathematical Modelling and Control, 2024, 4(2): 178-194. doi: 10.3934/mmc.2024016 |
[8] | Yunsi Yang, Jun-e Feng, Lei Jia . Recent advances of finite-field networks. Mathematical Modelling and Control, 2023, 3(3): 244-255. doi: 10.3934/mmc.2023021 |
[9] | Yanfei Wang, Changxi Li, Jun-e Feng . Distributed pinning controllers design for set stabilization of $ k $-valued logical control networks. Mathematical Modelling and Control, 2023, 3(1): 61-72. doi: 10.3934/mmc.2023006 |
[10] | Hongli Lyu, Yanan Lyu, Yongchao Gao, Heng Qian, Shan Du . MIMO fuzzy adaptive control systems based on fuzzy semi-tensor product. Mathematical Modelling and Control, 2023, 3(4): 316-330. doi: 10.3934/mmc.2023026 |
This paper discussed the synchronization of impulsive fuzzy neural networks (FNNs) with uncertainty of information exchange. Since the data of neural networks (NNs) cannot be completely measured in reality, we designed an observer-based impulsive controller on the basis of the partial measurement results and achieved the purpose of reducing the communication load and the controller load of FNNs. In terms of the Lyapunov stability theory, an impulsive augmented error system (IAES) was established and two sufficient criteria to guarantee the synchronization of our FNNs system were obtained. Finally, we demonstrated the validity of the results by a numerical example.
Fuzzy neural networks (FNNs) [1], which allow the activity of a neuron to be a "fuzzy" rather than an "all-or-one" process, have attracted popularity as a powerful tool for modeling complex systems due to their ability to handle uncertain and nonlienar dynamics. Recently, such neural networks (NNs) have drawn considerable attention. For example, a novel Takagi-Sugeno (T-S) fuzzy coupling NN with adjustable coupling intensity was designed [2]. In the work [3], a fuzzy pulse controller that does not need to share fuzzy parameters with FNNs has been presented. In the work [4], a topology-based fuzzy impulsive mechanism is proposed to schedule information transmission on the network for the first time, and the exponential synchronization of discrete and continuous systems are derived. Moreover, FNNs arise naturally in a number of applications, such as data compression [5], pattern recognition [6], image processing [7], adaptive signal processing [8], associative memory [9], optimization problems [10], and so on [11,12,13,14]. The advantage of FNNs is the ability to handle uncertainty and adapt to specific problems, allowing for greater flexibility than traditional NNs. Unfortunately, many existing excellent studies are about continuous-time FNNs, but there are few studies on discrete-time conditions.
The uncertainty in the information interaction NNs is unavoidable and may lead to packet dropouts, communication delay, communication error, or other problems [15,16,17,18]. In recent years, many research results have emerged around the uncertainty model of NNs. In [19], based on the event-triggered mechanism, the quasi-consensus tracking problems of uncertain multi-agent systems were studied. In [20], the synchronization conditions for NNs with bounded delay are achieved. As time goes on, robust synchronization attracted lots of attention since it became a powerful tool to study the uncertainty between coupled neural nodes [21]. In [22], using the impulsive control and the stability theory, several criteria for local and global robust synchronization in complex dynamical networks with unknown network coupling functions are developed. In [23], global robust synchronization for the multiple memory NNs with uncertain parameters was studied by an adaptive coupling method. Therefore, it is worthful and meaningful to devise a method to make NN models with uncertain information.
In the past decades, many effective control strategies have been proposed to synchronize NNs, such as state-feedback control [24,25,26], adaptive control, coupling control [27], pinning control [28], and so on. Among these control methods, impulse control is one of the most practical and economical strategies since it is discontinuous. Therefore, the implementation of the controller is easier and the control cost is effective [29,30,31]. In recent years, many scholars have studied the synchronization of NNs based on impulse control strategies. The synchronization of nonlinear delay systems is investigated by means of event-triggered impulsive control [32], where impulsive instants are determined by a Lyapunov-based event-triggered mechanism. In [33], the Lyapunov stability of impulsive systems via event-triggered impulsive control is explored, where dynamical systems evolve depending on continuous time equations most of the time, but occasionally exhibit instantaneous jumps when impulsive events are triggered. Two sufficient criterion for the hybrid delay stochastic reaction diffusion NNs to achieve exponential synchronization are designed by an impulsive controller in [34]. Almost all the existing synchronization strategies are designed on the condition that the state of the master system is available [35]. Due to the constraints of physical systems and sensors, part states of high-order systems cannot be measured directly [36]. Therefore, it is worthy and necessary to design a new type of synchronization control strategy for FNNs by making full use of the available measurement information. In [37], asynchronous observer and fault detection algorithms are designed to tackle a hidden Markov model. Although the continuous time impulsive systems have been studied comprehensively, there are few studies on the synchronization of discrete time FNNs by using impulse control strategies; thus, it is valuable to strengthen the research in discrete-time FNNs.
Motivated by the above discussions, a discrete-time FNN (2.2) with uncertain information exchange is established, and a distributed impulsive observer-based controller (DIOBC) and impulsive augmented error system (IAES) are designed. Based on the Lyapunov method, two sufficient conditions for the model to achieve synchronization are obtained, and the validity of our results is illustrated by numerical examples. The main contributions of our article are summarized as follows:
(1) The traditional general control strategy will lead to heavy communication load and waste of controller resources due to too many control times. The impulsive controller we designed works only at the impulsive time tm, and the FNN also interacts only at tm time. By designing such an impulsive controller, the controller benefit is greatly improved.
(2) An impulsive controller with unknown weight information between nodes has been designed, which is the main challenge to the research of master-slave FNNs. The uncertain weights are transformed into a Laplacian matrix with bounded norm to deal with the uncertain information interaction caused by the uncertain weights of the nodes in the FNNs.
(3) The case where we only measure the state information of FNNs is considered, and through these measurements, the observer-based impulsive controller model is established. In addition, the controller with an impulsive observer also makes our synchronization analysis more complicated.
We introduce the FNNs, DIOBC and IAES in Section 2, and in Section 3, the synchronization of FNNS is proved. In Section 4, the gains about controller and observer are designed. In Section 5, a numerical example is presented to clarify the validity of the results. Our conclusion is depicted in Section 6.
Notations: Z, Z+ stand for nonnegative integers and positive integers, respectively. Rn and Rm×n denote the n-dimensional Euclidean space and the set of all m×n matrices. The 2-norm for a vector is expressed as ‖⋅‖2. For a matrix A, its transpose, largest eigenvalue, and smallest eigenvalue are denoted by AT, ˉλ(A), and λ_(A), respectively. The notation ∗ inside the matrix denotes the term induced by symmetry. The positive definite matrix A is represented by A>0, and the notation A1−A2>0 (A1−A2≥0) means A1>A2(A1≥A2), where A1 and A2 are symmetric matrices. The matrix diagn{⋅} stands for a diagonal matrix belonging to Rn×n. The symbol ⊗ stands for the Kronecker product. aij(k)>0,∀i≠j means that the nodes vi and vj can exchange information mutually. The Laplacian matrix of the connection condition is represented by L(k), where Lii(k)=∑i≠jaij(k) and Lij(k)=−aij(k).
Each node in the FNNs model needs to satisfy the following rules:
Plant Rule l: If ξ1(k) is ml1 and … and ξp(k) is mlp, then
{xi(k+1)=Dlxi(k)+Blg(xi(k))+ui(k),yi(k+1)=Clxi(k), | (2.1) |
where
i∈ΦN≜{1,2,…,N},xi(k)∈Rn, |
and ui(k)∈ Rn are the state and the control input of the FNNs node i, respectively. ξ1(k),…,ξp(k) are premise variables that are functions of time, and ml1,…,mlp are the fuzzy sets for each premise variable. The index l belongs to the set
Φl≜{1,2,…,r}, |
where r denotes the number of fuzzy rules.
g(xi(k))=[g1(xi1(k)),g2(xi2(k)),…,gn(xin(k))]T |
represents the nonlinear activation functions, and the matrix
Dl=diagn{dl1,…,dln}∈Rn×n,Bl∈Rn×n,Cl∈Rn×m |
of FNNs is the matrix of coefficients, that we know.
Integrate all the nodes together, and the FNNs (2.1) can be given as
{xi(k+1)=r∑l=1θl(k){Dlxi(k)+Blg(xi(k))}+ui(k),yi(k+1)=r∑l=1θl(k)Clxi(k), | (2.2) |
where θl(k) satisfies
θl(k)=∏pι=1mlι(ξι(k))∑rj=1∏pι=1mjι(ξι(k)) |
with
r∑l=1θl(k)=1, |
and mlι(ξι(k)) is the grade of membership of ξι(k) in
mlι,ι∈Φp≜{1,2,…,p}. |
Define s(k) as the trajectory, which the FNNs (2.2) aim to follow:
{s(k+1)=r∑l=1θl(k){Dls(k)+Blg(s(k))},ys(k+1)=r∑l=1θl(k)Cls(k), | (2.3) |
where g(s(k)) is an activation function and satisfies the following assumption.
Assumption 1. The functions
gj(⋅),j∈Φn≜{1,2,…,n} |
satisfy gj(0)=0, and there exist the constants ˉϑj and ϑ_j such that
ϑ_j≤gj(χ1)−gj(χ2)χ1−χ2≤ˉϑj, | (2.4) |
where the constants χ1,χ2∈R satisfy χ1≠χ2.
Remark 2.1. Each state s(k) is a target signal of FNNs (2.2) to achieve synchronization by using the leader-follower method [38]. In [39], the T-S fuzzy logic is used to study the synchronization of master-slave NNs in terms of fuzzy rules to tackle local linear representation of nonlinear systems. Founded on the above, we investigate the synchronization of a group of master-slave NNs with n nodes. It is worth noting that most of the existing works assume that the states of FNNs (2.2) and unforced FNNs (2.3) are available, whereas in reality, due to the constraints of physical systems and sensors, only partial states of the FNNs (2.2) and (2.3) can be measured.
The continuous-time control causes problems such as high communication loads and low controller efficiency. In this section, an observer-based impulsive controller is designed to optimize these problems. The impulsive instants are denoted as
{tm},m∈Z. | (2.5) |
Define t0=−1. The impulsive intervals τm,m∈Z+ satisfy
τm≜tm−tm−1. |
Before arguing our main conclusions, we need the following assumption:
Assumption 2.2. The interval τm,m∈Z+ satisfies
0<τm≤τ. | (2.6) |
Based on the partial information obtained from FNNs measurements, the following observers were designed. For the non-impulsive instants k, the observers are given as follows:
{ˆxi(k+1)=r∑l=1θl(k){Dlˆxi(k)+Blg(ˆxi(k))},ˆs(k+1)=r∑l=1θl(k){Dlˆs(k)+Alg(ˆs(k))}, | (2.7) |
where ˆxi(k) and ˆs(k) are observer states of FNNs (2.2) and (2.3), respectively.
Furthermore, at the impulsive instants tm, the observer model is
{ˆs(tm+1)=ˆs(tm)+r∑n=1θn(tm){Rsn(ys(tm)−ˆys(tm))},ˆxi(tm+1)=ˆxi(tm)+r∑n=1θn(tm){Rin(yi(tm)−ˆyi(tm))}+ui(tm), | (2.8) |
where Rsn∈ Rn×m and Rin∈ Rn×m are observer gains.
Remark 2.2. Using available measurement values to estimate the system state is an important technique in the field of control. In [40], Cheng et al. designed an asynchronous state observer by using a hidden Markov model and obtained sufficient conditions for the existence of a fuzzy asynchronous fault detection filter for a class of nonlinear Markov jump systems. However, the system state may not be fully accessible. In this case, we need to construct an observer and establish a corresponding tracking error system between the observer and the target. It should be noted that the system (2.2) under the control input ui(t) is a type of pulse system. Therefore, in order to build a suitable tracking error system, it is natural to consider the observer with impulse effect.
Based on the observer model given above, we design the following impulsive controller. With u(k)=0 at the non-impulsive instants k and at the instants tm, our FNNs of the node i are given as
ui(tm)=r∑l=1θl(tm){KilN∑j=1aij(tm)ˆxj(tm)+ςiHil(ˆxi(tm)−ˆs(tm))+MilN∑j=1aij(tm)yj(tm)+ςiFil(yi(tm)−ys(tm))}, | (2.9) |
where matrices Kil∈Rn×n,Hil∈Rn×n,Mil∈Rn×m, Fil∈Rn×m, and ςi≥0 are the controller gain and pinning gain respectively.
Remark 2.3. As far as we know, most of the synchronization controllers used in NNs depend on the state information of the master-slave systems. However, the synchronization of FNNs are derived based on the the coupling effect between nodes and an uncertain information impulsive controller [41]. Motivated by this method, we employ the fuzzy theory to describe the nonlinear model and design a fuzzy uncertain information impulsive controller.
The uncertainty of the connection weight aij(k), i,j∈ΦN between the nodes of FNNs will lead to uncertain information interaction. In order to deal with this problem, we assume that these connection weights will vary over an interval, that is,
0≤a_ij≤aij(k)≤ˉaij,i≠j. | (2.10) |
To solve the problem of uncertain node weights, we introduce the following notations:
ˇaij={12∑Nj=1,i≠j(a_ij+ˉaij),i=j,−12(a_ij+ˉaij),i≠j,ˆaij={12∑Nj=1,i≠j(a_ij−ˉaij),i=j,−12(a_ij−ˉaij),i≠j. | (2.11) |
Through the above discussion, the uncertain connection weight (2.10) can be rewritten as
aij(k)=ˇaij+ˆaijΛl(k), | (2.12) |
where the index is
l=N(i−1)+j, |
and the time-varying scalar Λl(k) satisfies
−I≤Λl(k)≤I. |
The Laplacian matrix of the distributed protocol is expressed as
L(k)=L+ΔL(k)=L+MΛ(k)N, | (2.13) |
where
L=[ˇa11ˇa12…ˇa1Nˇa21ˇa22…ˇa2N⋮⋮⋱⋮ˇaN1ˇaN2…ˇaNN],M=[√ˆa11e1,√ˆa12e1,…,√ˆa1Ne1,…,√ˆaN1eN,…,√ˆaNNeN]∈RN×N2,N=[√ˆa11e1,√ˆa12e2,…,√ˆa1NeN,…,√ˆaN1e1,…,√ˆaNNeN]T∈RN2×N,ei=[0,…,0⏟i−1,1,0,…,0⏟N−i]T,Λ(k)=diagN2{Λ1(k),Λ2(k),…,ΛN2(k)}. |
We denote the estimation error of the leader FNNs, the state error, and the estimation error between the leader FNNs (2.3) and the FNNs node i as
es(k)≜s(k)−ˆs(k),ei(k)≜xi(k)−s(k) |
and
ˆei(k)≜ˆxi(k)−ˆs(k), |
respectively. Furthermore, the augmented state
ηi(k)=[eTs(k) eTi(k) ˆeTi(k)]T |
is defined. By integrating the formulae (2.2), (2.3), (2.8), (2.9), and (2.12), we have
ηi(k+1)={∑rl=1θl(k){(I3⊗Dl)ηi(k)+(I3⊗Bl)¯g(ηi(k))},k≠tm,∑rl=1θl(tm)∑rn=1θn(tm)×{ˉDηi(tm)+(I3⊗Bln)¯g(ηi(tm))+ˉK∑Nj=1Lijηj(tm)}, k=tm, | (2.14) |
where
ˉK=[0000MinClKin0MinClKin],¯g(ηi(k))=[g(s(k))−g(ˆs(k))g(xi(k))−g(s(k))g(ˆxi(k))−g(ˆs(k))],D11=[Dl−RsnCl00Dl+ςiFinCl],D12=[0ςiHin]T,D21=[RinCl−RsnClRinCl+ςiFinCl],D22=Dl−RinCl+ςiHin,ˉD=[D11D12D21D22]. |
Considering the system (2.14) and defining the augmented error state
η(k)=[eTs(k) eT1(k) ˆeT1(k) … eTN(k) ˆeTN(k)]T, |
we have the following IAES:
η(k+1)={∑rl=1θl(k){(I2N+1⊗Dl)η(k)+(I2N+1⊗Bl)g(η(k))},k≠tm,∑rl=1θl(k)∑rn=1θn(k){Dlnη(k)+(I2N+1⊗Bl)g(η(k))},k=tm, | (2.15) |
where
Dln=(D1+M((L+ΔL)⊗I2m)C+K((L+ΔL)⊗I2n)I),D1=[Dl−RsnCl0RD22],ˉRi=[0RinCl−RsnCl],D22=diagN{D221,D222,…,D22N},D22i=[Dl+ςiFinClςiHinRinCl+ςiFinClDl−RinCl+ςiHin],R=[ˉRT1nˉRT2n…ˉRTNn]T,K=[0KT12]T,K12=diagN{K121,K122,…,K12N},M=[0MT12]T,I=[0IN⊗ˉI],M12=diagN{M121,M122,…,M12N},K12i=[0Kin0Kin],ˉI=[000In],M12i=[Min0Min0],C=[0C12],C12=diagN{C121,C122,…,C12N},C12i=diag2{Cl,0},˜g(es(k))=g(s(k))−g(ˆs(k)),g(η(k))=[˜gT(es(k)) ˜gT(η1(k)) … ˜gT(ηN(k))]T,˜g(ηi(k))=[g(xi(k))−g(s(k))g(ˆxi(k))−g(ˆs(k))]. |
Remark 2.4. Since the information exchange between the large-scale biological system and the artificial system is not fixed, the robustness of the coupling system connection weight is very important. In order to describe the uncertainty of connection weights between FNN nodes in more detail, the interval uncertainty model (2.12) is introduced here.
For the third part of the proof, we give the following definition and lemma.
Definition 2.1. [42] The synchronization of FNNs (2.2) and (2.3) will be arrived if the following inequality holds
limk→∞‖η(k)‖2=0. | (2.16) |
Lemma 2.1. [43] For a real matrix Ξ=ΞT, the following assertions are equivalent
Ξ:=(Ξ11Ξ12∗Ξ22)<0,Ξ11<0,Ξ22−ΞT12Ξ−111Ξ12<0,Ξ22<0,Ξ11−Ξ12Ξ−122ΞT12<0. |
In this section, sufficient conditions for the synchronization of FNNs (2.2) with the trajectory (2.3) are presented.
Theorem 3.1. Suppose that the scalars α1 and α2 satisfy 0<α2<1≤α1. FNNs (2.2) are said to be synchronized with (2.3) if there exist matrices Pl>0,ˆϵ1>0, Fin,Hin,Kin,Min,Rsn, and Rin and Conditions (3.1)–(3.3) hold for ∀σ∈Φl:
[−α1Pl−¯T1ˆϵ1¯T2ˆϵ1(I2N+1⊗Dl)TPl∗−ˆϵ1(I2N+1⊗Bl)TPl∗∗−Pσ]<0, | (3.1) |
[−α2Pl−¯I1ˆϵ1¯T2ˆϵ1DT∗−ˆϵ1(I2N+1⊗Bl)T∗∗−P−1σ]<0, | (3.2) |
(τ−1)lnα1+lnα2<0, | (3.3) |
where
¯T1=diag2N+1{T1,T1,…,T1},¯T2=diag2N+1{T2,T2,…,T2},T1=diagn{ϑ_1ˉϑ1,ϑ_2ˉϑ2,…,ϑ_nˉϑn},T2=diagn{ϑ1+ˉϑ12,ϑ_2+ˉϑ22,…,ϑ_n+ˉϑn2},ˆϵ1=diag2N+1{ϵ1,ϵ1,…,ϵ1},ϵ1=diagn{ϵ11,ϵ12,…,ϵ1n}. |
Proof. Based on DIOBC and IAES, we establish the following Lyapunov functional
V(η(k),k)=ηT(k)(r∑l=1θσ(k)Pσ)η(k), | (3.4) |
where Pσ>0, ∀σ∈Φl, and for the sake of simplicity, we use V(k) to represent V(η(k),k).
Step 1. When k≠tm, we define the Lyapunov functional (3.4) as
ΔV(k)≜V(k+1)−α1V(k). | (3.5) |
By substituting the IAES (2.15) into (3.5), we obtain
ΔV(k)=r∑σ=1θσ(k+1)r∑l=1θl(k)×{ηT(k+1)TPση(k+1)−α1ηT(k)Plη(k)}=r∑σ=1θσ(k+1)r∑l=1θl(k)r∑n=1θj(k)×{(ηT(k)(I2N+1⊗Dl)T+gT(η(k))(I2N+1⊗Bl)T)×Pσ((I2N+1⊗Dn)η(k)+(I2N+1⊗Bn)g(η(k)))−α1ηT(k)Plη(k)}≤r∑σ=1θσ(k+1)r∑l=1θl(k)×{(ηT(k)(I2N+1⊗Dl)T+gT(η(k))(I2N+1⊗Bl)T)×Pσ((I2N+1⊗Dl)η(k)+(I2N+1⊗Bl)g(η(k)))−α1ηT(k)Plη(k)}=r∑σ=1θσ(k+1)r∑l=1θl(k)×{ηT(k)(I2N+1⊗Dl)TPσ(I2N+1⊗Dl)η(k)+ηT(k)(I2N+1⊗Dl)TPσ(I2N+1⊗Bl)g(η(k))+η(k)(I2N+1⊗Dl)PσgT(η(k))(I2N+1⊗Bl)T+gT(η(k))(I2N+1⊗Bl)TPσ(I2N+1⊗Bl)g(η(k))−α1ηT(k)Plη(k)}=r∑σ=1θσ(k+1)r∑l=1θl(k){ζT(k)Ωσlζ(k)}, | (3.6) |
where
Ωσl=[Ω111−α1PσΩ112∗Ω122],Ω111=(I2N+1⊗Dl)TPσ(I2N+1⊗Dl),Ω112=(I2N+1⊗Dl)TPσ(I2N+1⊗Bl),Ω122=(I2N+1⊗Bl)TPσ(I2N+1⊗Bl),ζ(k)=[ηT(k)gT(η(k))]T. |
Combining with Assumption 2.1, we obtain the inequalities (3.7) and (3.8) about the functions gj(⋅), j∈ΦN for any scalar ϵ1j>0
ϵ1j(gj(xij(k))−ϑ_jxij(k))(gj(xij(k))−ˉϑjxij(k))≤0, | (3.7) |
ϵ1j(gj(ˆxij(k))−ϑ_jˆxij(k))(gj(ˆxij(k))−ˉϑjˆxij(k))≤0. | (3.8) |
By combining with inequalities (3.7) and (3.8), we get that the following (3.9) holds for any ϵ1>0
ˆϵ1(g(η(k))−ˇϑ_η(k))T(g(η(k))−ˆˉϑη(k))≤0, | (3.9) |
where
ˇϑ_=diag2N+1{ˆϑ_,ˆϑ_,…,ˆϑ_},ˆϑ_=diagn{ϑ_1,ϑ_2,…,ϑ_n},ˇˉϑ=diag2N+1{ˆˉϑ,ˆˉϑ,…,ˆˉϑ},ˆˉϑ=diagn{ˉϑ1,ˉϑ2,…,ˉϑn}. |
According to the inequality of the activation function (3.9), it follows that
ζT(k)[¯T1ˆϵ1−¯T2ˆϵ1∗ˆϵ1]ζ(k)≤0. | (3.10) |
The difference is that (3.5) satisfies the following inequality (3.11) by taking (3.6) into (3.10):
ΔV(k)≤r∑σ=1θσ(k+1)r∑l=1θl(k){ζT(k)Ωσl2ζ(k)}, | (3.11) |
where
Ωσl2=[Ω111−α1Pl−¯T1ˆϵ1Ω112+¯T2ˆϵ1∗Ω122−ˆϵ1]. |
Pre-multiplying and post-multiplying the inequality condition (3.1) with diag3{I,I,P} and applying Lemma 2.1, we have Ωσl2<0, that is,
V(k+1)<α1V(k). | (3.12) |
Step 2. When the time is k=tm, we define the Lyapunov functional (3.4) as:
ΔV(tm)=V(tm+1)−α2V(tm). | (3.13) |
Putting the IAES (2.15) into (3.13), we obtain
ΔV(tm)=r∑σ=1θσ(tm+1)r∑l=1θl(tm)×{ηT(tm+1)Pση(tm+1)−α2ηT(tm)Plη(tm)}=r∑σ=1θσ(tm+1)r∑l=1θl(tm)r∑n=1θn(tm)r∑a=1θa(tm)r∑b=1θb(tm)⋅{gT(η(tm))(Dlnη(tm)+(I2N+1⊗Bl))T×pσ(Dabη(tm)+(I2N+1⊗Ba)g(η(tm)))−α2ηT(tm)Plη(tm)}≤r∑σ=1θσ(tm+1)r∑l=1θl(tm)r∑n=1θn(tm)×{gT(η(tm))(Dlnη(tm)+(I2N+1⊗Bl))T×pσ(Dlnη(tm)+(I2N+1⊗Bl)g(η(tm)))−α2ηT(tm)Plη(tm)}=r∑σ=1θσ(tm+1)r∑l=1θl(tm)r∑n=1θn(tm)⋅{ηT(tm)⋅DTlnPσDlnη(tm)+2ηT(tm)DlnPσ(I2N+1⊗Bl)⋅g(η(tm))+gT(η(tm))(I2N+1⊗Bl)TPσ(I2N+1⊗Bl)⋅g(η(tm))−α2ηT(tm)Plη(tm)}. | (3.14) |
Combining inequalities of the activation function (3.10) and the difference functional (3.14), we have
ΔV(k)≤r∑σ=1θσ(tm+1)r∑l=1θl(tm)r∑n=1θn(tm)×{ζT(k)Ωσlnζ(k)}, | (3.15) |
where
Ωσln=[Ω311Ω312∗(I2N+1⊗Bl)TPl(I2N+1⊗Bl)−ˆϵ1],Ω311=DTlnPlDln−α2Pσ−¯T1ˆϵ1,Ω312=DTlnPl(I2N+1⊗Bl)+¯T2ˆϵ1. |
Applying Lemma 2.1 to (3.2), the inequality (3.16) holds with (3.15)
V(tm+1)<α2V(tm). | (3.16) |
Step 3. Based on the above two steps, we discuss the synchronization for the FNNs (2.2).
We obtain that the following inequalities hold for k∈(0,t1] by the inequality (3.12) and Assumption 2.2
V(k)<α1V(k−1)<⋯<αk1V(0)≤ατ−11V(0). | (3.17) |
According to the inequalities (3.16) and (3.17), the inequality (3.18) is derived at the instant k=t1+1.
V(t1+1)<α2ατ−11V(0). | (3.18) |
For instants k∈(t1+1,t2], it follows from (3.12) and (3.17) that
V(k)<α1V(k−1)<⋯<αk−(t1+1)1V(t1+1)<ατ−11V(t1+1)<α2α2τ−21V(0). | (3.19) |
In view of (3.16) and (3.19), we have the following inequality at instant k=t2+1
V(t2+1)<α22α2τ−21V(0). | (3.20) |
By combining the formulas (3.17) to (3.20), the inequality (3.21) will hold for ∀m∈Z
V(tm+1)<(α2ατ−11)mV(0), | (3.21) |
and the inequality (3.21) implies that
λ_(P)‖η(tm+1)‖22<(α2ατ−11)mˉλ(P)‖η(0)‖22. |
Furthermore, we have
‖η(tm+1)‖22<ˉλ(P)λ_(P)(α2ατ−11)m‖η(0)‖22. | (3.22) |
Due to 1≤α1 and 0<α2<1, it follows that
0<α2ατ−11. | (3.23) |
By the inequality (3.3), one can derive that
α2ατ−11=elnα2ατ−11=e(τ−1)lnα1+lnα2<1. | (3.24) |
On the basis of Definition 2.1 and inequalities (3.22)–(3.24), we get that the state is exponentially stable at instants tm+1,m∈Z+, which implies
limm→∞‖η(tm+1)‖2=0. | (3.25) |
Through comparing with the inequalities (3.17) and (3.19) and combining with the inequality (3.22), we can derive the following inequality ∀k∈(tm+1,tm+1],m∈Z
‖η(k)‖2<√ˉλ(P)λ_(P)ατ−11‖η(tm+1)‖2. | (3.26) |
Due to the term (ˉλ(P)/λ_(P))ατ−11 being bounded, the following conditions exist ∀k∈(tm+1,tm+1],m∈Z
limk→∞‖η(k)‖2<√ˉλ(P)λ_(P)ατ−11limm→∞‖η(tm+1)‖2=0. |
Taking account of (3.25) and (3.26), we have
limk→∞‖η(k)‖=0, |
which implies that the synchronization of FNNs (2.2) are achieved.
In this section, we design the gains of an impulsive observer and controller on the conclusions of Part III.
Theorem 4.1. Given scalars 0<α2<1≤α1, the group of FNNs (2.2) is said to be synchronization if there exist matrices Pl>0,ˆϵ1>0,ˆFil, Gl,ˆHil,ˆKil,ˆMil,ˆRs, and ˆRil and scalars ϵ2>0 and ϵ3>0 such that Conditions (3.1), (3.3), and (4.1) hold for ∀σ,l,j∈Φl.
[ˆΨ11¯T2ˆϵ1ˆΨ13ˆΨ140ˆΨ160∗−ˆϵ1ˆΨ230000∗∗ˆΨ330ˆΨ350ˆΨ37∗∗∗−ϵ2I000∗∗∗∗−ϵ2I00∗∗∗∗∗−ϵ3I0∗∗∗∗∗∗−ϵ3I]<0, | (4.1) |
where
ˆΨ11=−α2Pl−¯T1ˆϵ1,ˆΨ23=(I2N+1⊗Bl)TGTj,ˆD222=diagN{ˆD1,ˆD2,…,ˆDN},ˆΨ13=ˆDlj1T+(ˆM(L⊗I2m)C+ˆK(L⊗I2n)I)T,ˆDlj1=[GjDl−ˆRSC0ˆRˆD222],ˆRil=[0ˆRilCl−ˆRSCl],ˆDilj=[GjDl+ςiˆFijClςiˆHijˆRijCl+ςiˆFijClGDl−ˆRiCl+ςiˆHij],ˆR=[ˆR1lˆR2l…ˆRNl],ˆM=[0ˆMT12]T,ˆM12=diagN{ˆM121,ˆM122,…,ˆM12N},ˆK12=diagN{ˆK121,ˆK122,…,ˆK12N},ˆM12i=[ˆMij0ˆMij0],ˆK12i=[0ˆKij0ˆKij],ˆK=[0ˆKT12]T,ˆΨ14=ϵ2CT(N⊗I2m)T,ˆΨ35=ˆM(M⊗I2m),ˆΨ16=ϵ3IT(N⊗I2n)T,ˆΨ37=ˆK(M⊗I2n),ˆΨ33=Pσ−Gj−GTj,Gj=diag2N+1{Gj,Gj,…,Gj}. |
Thus, the observer and controller gains are listed as follows:
Fil=G−1lˆFil,Hil=G−1lˆHil,Kil=G−1lˆKil,Mil=G−1lˆMil,Ril=G−1lˆRil,Rs=G−1lˆRs. |
Proof. In terms of the Schur complement theorem, the following inequality holds based on the condition (4.1):
Σ1+ϵ2ΣT2Σ2+ϵ−12Σ3ΣT3+ϵ3ΣT4Σ4+ϵ−13Σ5ΣT5<0, | (4.2) |
where
Σ1=[−α2Pl−¯T1ˆϵ1¯T2ˆϵ1ˆΨ13∗−ˆϵ1(I2N+1⊗Bl)TGTj∗∗Pσ−Gj−GTj],Σ2=[(N⊗I2m)C00],Σ3=[00(M⊗I2m)TˆMT]T,Σ4U=[(N⊗I2n)I00],Σ5=[00(M⊗I2n)TˆKT]T. |
For every matrix Pσ>0, we can get P−1σ>0, and notice that Pσ−Gj is not a zero matrix. By the properties of positive definite matrices, we have
(Pσ−Gj)P−1σ(Pσ−Gj)T≥0, |
which implies
Pσ−Gj−GTj≥GjP−1σGTj. | (4.3) |
Define
ˆFil≜GlFil,ˆHil≜GlHil,ˆKil≜GlKil,ˆMil≜GlMil,ˆRil≜GlRil,ˆRs≜GlRs. |
According to Lemma 2.1, (4.2), and (4.3), it follows that
[−α2Pl−¯T1ˆϵ1¯T2ˆϵ1DTGTj∗−ˆϵ1(I2N+1⊗Bl)TGTj∗∗−GjPσGTj]<0. | (4.4) |
Pre-multiplying and post-multiplying the obtained inequality (4.4) with diag3{I,I,G−1j} and its transpose, the condition (3.2) holds, and the group of FNNs (2.2) will achieve synchronization by combining conditions (3.1) and (3.3).
To prove the validity of our results, we present a numerical example of FNNs (2.2) with five nodes here, whose parameters are as follows:
B1=[−0.2−0.4−0.4−0.1],B2=[0.2−0.4−0.3−0.1],C1=[11],C2=[10],D1=diag2{−0.85,−0.85},D2=diag2{−0.85,−0.95}. |
According to the connection topology of DIOBC, as shown in Figure 1, its uncertain connection weights are expressed as
a12(k)=a21(k)=0.8+0.1sin(k),a14(k)=a41(k)=0.9+0.1sin(k),a23(k)=a32(k)=1.1+0.1sin(k),a25(k)=a52(k)=1+0.1sin(k),a34(k)=a43(k)=1.2+0.1sin(k),a35(k)=a53(k)=1+0.1sin(k). |
The pinning gains of the DIOBC are ς1=1,ς2=1,ς3=0,ς4=1,ς5=0. The nonlinear activation functions gi(⋅),i∈Φn of our discrete-time FNNs (2.2) are defined as
g1(xi1(k))=0.2(|xi1(k)+1|−|xi1(k)−1|),g2(xi2(k))=0.1(|xi2(k)+1|−|xi2(k)−1|), |
which imply ϑ_1=0,ϑ_2=0,ˉϑ1=0.4 and ˉϑ2=0.2.
Assuming that the iterative step side is ε=0.02, according to the algorithm in [44], we can get that α1=1.04,α2=0.76, and the maximal allowed impulsive interval is τ=8. The observer and controller gains are listed below
R0=[−0.1667−0.2082],R11=[−0.1699−0.2081],R21=[−0.4254−0.5601],R31=[−0.1411−0.1591],R41=[−0.2185−0.2761],R51=[−0.1205−0.1325],H11=[0.13180.09540.13600.1696],F11=[0.30100.3676],H21=[0.0258−0.0589−0.0106−0.0628],F21=[0.48540.6181],H41=[0.15700.11240.17690.1872],F41=[0.37440.4716],H31=0,H51=0,F31=0,F51=0,K11=[−0.0002−0.0005−0.0007−0.0009],M11=[0.00070.0003],K21=[0.01520.02560.02030.0376],M21=[−0.0321−0.0399],K31=[0.04200.02840.04340.0453],M31=[0.09710.1133],K41=[−0.0150−0.0193−0.0212−0.0241],M41=[−0.0188−0.0250],K51=[0.05140.03480.05490.0531],M51=[0.11560.1297],R22=[−0.4254−0.6301],R32=[−0.1351−0.1291],R42=[0.2325−0.1224],R52=[−0.1205−0.1435],H12=[0.14230.14260.13560.1246],F12=[0.24300.5626],H22=[0.0258−0.0589−0.2346−0.7428],F22=[0.43540.5381],H42=[0.15700.11240.14190.4272],F42=[0.34540.1216],H32=0,H52=0,F32=0,F52=0,K12=[−0.0012−0.0004−0.0002−0.0010],M12=[0.00050.0007],K22=[0.01520.02560.03520.0264],M22=[−0.0523−0.0743],K32=[0.04200.02840.04120.0653],M32=[0.04510.1112],K42=[−0.0150−0.0423−0.0412−0.0621],M42=[−0.0588−0.0450],K52=[0.05140.03480.03190.0521],M52=[0.34160.3256],R12=[−0.1252−0.3451]. |
The fuzzy rules membership functions are
θ1(k)=sin2(3k),θ2(k)=cos2(3k). |
The FNNs initial states are as follows:
s(0)=[1−2],x1(0)=[25],x2(0)=[−31],x3(0)=[−1−2],x4(0)=[−4−3],x5(0)=[−24], |
Under the above conditions, the states of our three fuzzy neural nodes with impulsive control, general control, and without control are shown in Figures 2–4, respectively. We can see that in these three kinds, both impulsive control and general control will achieve synchronization along the expected trajectory over time, and the synchronization time is almost the same.
However, as shown in Table 1, the control times of impulsive control to achieve synchronization are less than those of general control; that is, although the time required for impulsive control and general control to achieve synchronization is the same, the efficiency of impulsive control is higher than that of general control.
Synchronization error | Impulsive control times | General control times |
|e1|≤0.01 | 16 | 27 |
|e2|≤0.01 | 15 | 26 |
|e3|≤0.01 | 19 | 27 |
In this paper, we investigate the synchronization property of a set of discrete-time FNNs, considering the case of uncertain information exchange caused by uncertain weights of nodes between FNNs nodes. Based on the measured partial states of FNNs, the controller based on the impulse observer is designed, the IAES is derived, and by proving the stability of IAES, the synchronization sufficient conditions of FNNs and the corresponding gain matrices of the observer and controller are obtained. Finally, a numerical example is given to illustrate the validity of our results.
During the preparation of this work, we used the AI tool "QuillBot" in order to revise the grammar. After using this tool, we reviewed and edited the content as needed and take full responsibility for the content of the publication.
This work is supported by the National Natural Science Foundation of China Grant No. 12171065, the Science and Technology Research Program of Chongqing Municipal Education Commission Grant No. KJQN202000601, the Natrual Science Foundation Project of Chongqing under Grant cstc2020jcyj-msxmX0593, the Open Project of Key Laboratory No. CSSXKFKTM202007, Mathematical College, Chongqing Normal University.
All authors declare that they have no conflicts of interest.
[1] |
J. Buckley, Y. Hayashi, Fuzzy neural networks: a survey, Fuzzy Sets Syst., 66 (1994), 1–13. https://doi.org/10.1016/0165-0114(94)90297-6 doi: 10.1016/0165-0114(94)90297-6
![]() |
[2] |
X. Wang, Y. Yu, S. Zhong, K. Shi, N. Yang, D. Zhang, et al., Novel heterogeneous mode-dependent impulsive synchronization for piecewise T-S fuzzy probabilistic coupled delayed neural networks, IEEE Trans. Fuzzy Syst., 30 (2021), 2142–2156. https://doi.org/10.1109/TFUZZ.2021.3076525 doi: 10.1109/TFUZZ.2021.3076525
![]() |
[3] |
X. Wang, Y. Yu, K. Shi, H. Chen, S. Zhong, X. Yang, et al., Membership-mismatched impulsive exponential stabilization for fuzzy unconstrained multilayer neural networks with node-dependent delays, IEEE Trans. Fuzzy Syst., 31 (2022), 1214–1228. https://doi.org/10.1109/TFUZZ.2022.3197925 doi: 10.1109/TFUZZ.2022.3197925
![]() |
[4] |
X. Wang, Y. Yu, J. Cai, N. Yang, K. Shi, S. Zhong, et al., Multiple mismatched synchronization for coupled memristive neural networks with topology-based probability impulsive mechanism on time scales, IEEE Trans. Cybern., 53 (2021), 1485–1498. https://doi.org/10.1109/TCYB.2021.3104345 doi: 10.1109/TCYB.2021.3104345
![]() |
[5] |
A. Iwata, Y. Nagasaka, N. Suzumura, Data compression of the ECG using neural network for digital Holter monitor, IEEE Eng. Med. Biol. Mag., 9 (1990), 53–57. https://doi.org/10.1109/51.59214 doi: 10.1109/51.59214
![]() |
[6] |
M. Patricia, D. Sánchez, Multi-objective optimization for modular granular neural networks applied to pattern recognition, Inf. Sci., 460-461 (2018), 594–610. https://doi.org/10.1016/j.ins.2017.09.031 doi: 10.1016/j.ins.2017.09.031
![]() |
[7] |
Y. Zhao, X. He, T. Huang, J. Huang, P. Li, A smoothing neural network for minimization l1-lp in sparse signal reconstruction with measurement noises, Neural Networks, 122 (2020), 40–53. https://doi.org/10.1016/j.neunet.2019.10.006 doi: 10.1016/j.neunet.2019.10.006
![]() |
[8] |
M. R. G. Meireles, P. E. Almeida, M. G. Simes, A comprehensive review for industrial applicability of artificial neural networks, IEEE Trans. Ind. Electron., 50 (2003), 585–601. https://doi.org/10.1109/TIE.2003.812470 doi: 10.1109/TIE.2003.812470
![]() |
[9] |
G. A. Carpenter, Neural network models for pattern recognition and associative memory, Neural Networks, 2 (1989), 243–257. https://doi.org/10.1016/0893-6080(89)90035-X doi: 10.1016/0893-6080(89)90035-X
![]() |
[10] |
W. He, Y. Dong, Adaptive fuzzy neural network control for a constrained robot using impedance learning, IEEE Trans. Neural Networks Learn. Syst., 29 (2017), 1174–1186. https://doi.org/10.1109/TNNLS.2017.2665581 doi: 10.1109/TNNLS.2017.2665581
![]() |
[11] |
Q. Song, W. Yu, J. Cao, F. Liu, Reaching synchronization in networked harmonic oscillators with outdated position data, IEEE Trans. Cybern., 46 (2016), 1566–1578. https://doi.org/10.1109/TCYB.2015.2451651 doi: 10.1109/TCYB.2015.2451651
![]() |
[12] |
T. Takagi, M. Sugeno, Fuzzy identification of systems and its applications to modeling and control, IEEE Trans. Syst. Man Cybern., 15 (1993), 116–132. https://doi.org/10.1109/TSMC.1985.6313399 doi: 10.1109/TSMC.1985.6313399
![]() |
[13] |
S. Selcuk, New stability results for Takagi-Sugeno fuzzy Cohen-Grossberg neural networks with multiple delays, Neural Networks, 114 (2019), 60–66. https://doi.org/10.1016/j.neunet.2019.02.010 doi: 10.1016/j.neunet.2019.02.010
![]() |
[14] |
A. Wu, Z. Zeng, Boundedness, Mittag-Leffler stability and asymptotical periodicity of fractional order fuzzy neural networks, Neural Networks, 74 (2016), 73–84. https://doi.org/10.1016/j.neunet.2015.11.003 doi: 10.1016/j.neunet.2015.11.003
![]() |
[15] |
Y. Xu, J. Li, R. Lu, C. Liu, Y. Wu, Finite-horizon l2-l∞ synchronization for time-varying Markovian jump neural networks under mixed-type attacks: observer-based case, IEEE Trans. Neural Networks Learn. Syst., 30 (2018), 1695–1704. https://doi.org/10.1109/TNNLS.2018.2873163 doi: 10.1109/TNNLS.2018.2873163
![]() |
[16] |
X. Yang, G. Feng, C. He, J. Cao, Event-triggered dynamic output quantization control of switched T-S fuzzy systems with unstable modes, IEEE Trans. Fuzzy Syst., 30 (2022), 4201–4210. https://doi.org/10.1109/TFUZZ.2022.3145808 doi: 10.1109/TFUZZ.2022.3145808
![]() |
[17] |
J. Li, B. Zhang, R. Lu, Y. Xu, T. Huang, Distributed H∞ state estimator design for time-delay periodic systems over scheduling sensor networks, IEEE Trans. Cybern., 51 (2019), 462–472. https://doi.org/10.1109/TCYB.2019.2894392 doi: 10.1109/TCYB.2019.2894392
![]() |
[18] |
Y. Xu, J. Dong, R. Lu, L. Xie, Stability of continuous-time positive switched linear systems: a weak common copositive Lyapunov functions approach, Automatica, 97 (2018), 278–285. https://doi.org/10.1016/j.automatica.2018.04.037 doi: 10.1016/j.automatica.2018.04.037
![]() |
[19] |
X. Xie, T. Wei, X. Li, Hybrid event-triggered approach for quasi-consensus of uncertain multi-agent systems with impulsive protocols, IEEE Trans. Circuits Syst., 69 (2022), 872–883. https://doi.org/10.1109/TCSI.2021.3119065 doi: 10.1109/TCSI.2021.3119065
![]() |
[20] |
H. Trentelman, K. Takaba, N. Monshizadeh, Robust synchronization of uncertain linear multi-agent systems, IEEE Trans. Autom. Control, 58 (2013), 1511–1523. https://doi.org/10.1109/TAC.2013.2239011 doi: 10.1109/TAC.2013.2239011
![]() |
[21] |
T. Li, M. Fu, L. Xie, J. Zhang, Distributed consensus with limited communication data rate, IEEE Trans. Autom. Control, 56 (2010), 279–292. https://doi.org/10.1109/TAC.2010.2052384 doi: 10.1109/TAC.2010.2052384
![]() |
[22] |
T. Li, F. Wu, J. Zhang, Multi-agent consensus with relative-state-dependent measurement noises, IEEE Trans. Autom. Control, 59 (2014), 2463–2468. https://doi.org/10.1109/TAC.2014.2304368 doi: 10.1109/TAC.2014.2304368
![]() |
[23] |
B. Liu, X. Liu, G. Chen, H. Wang, Robust impulsive synchronization of uncertain dynamical networks, IEEE Trans. Circuits Syst. I, 52 (2005), 1431–1441. https://doi.org/10.1109/TCSI.2005.851708 doi: 10.1109/TCSI.2005.851708
![]() |
[24] |
S. Yang, Z. Guo, J. Wang, Robust synchronization of multiple memristive neural networks with uncertain parameters via nonlinear coupling, IEEE Trans. Syst. Man Cybern., 45 (2015), 1077–1086. https://doi.org/10.1109/TSMC.2014.2388199 doi: 10.1109/TSMC.2014.2388199
![]() |
[25] |
T. Chen, X. Liu, W. Lu, Pinning complex networks by a single controller, IEEE Trans. Circuits Syst. I, 54 (2007), 1317–1326. https://doi.org/10.1109/TCSI.2007.895383 doi: 10.1109/TCSI.2007.895383
![]() |
[26] |
W. Yu, G. Chen, J. Lü, On pinning synchronization of complex dynamical networks, Automatica, 45 (2009), 429–435. https://doi.org/10.1016/j.automatica.2008.07.016 doi: 10.1016/j.automatica.2008.07.016
![]() |
[27] |
X. Li, X. Yang, S. Song, Lyapunov conditions for finite-time stability of time-varying time-delay systems, Automatica, 103 (2019), 135–140. https://doi.org/10.1016/j.automatica.2019.01.031 doi: 10.1016/j.automatica.2019.01.031
![]() |
[28] |
X. Yang, Y. Liu, J. Cao, L. Rutkowski, Synchronization of coupled time-delay neural networks with mode-dependent average dwell time switching, IEEE Trans. Neural Networks Learn. Syst., 31 (2020), 5483–5496. https://doi.org/10.1109/TNNLS.2020.2968342 doi: 10.1109/TNNLS.2020.2968342
![]() |
[29] |
J. Lu, C. Ding, J. Lou, J. Cao, Outer synchronization of partially coupled dynamical networks via pinning impulsive controllers, J. Franklin Inst., 352 (2015), 5024–5041. https://doi.org/10.1016/j.jfranklin.2015.08.016 doi: 10.1016/j.jfranklin.2015.08.016
![]() |
[30] |
X. Li, S. Song, J. Wu, Exponential stability of nonlinear systems with delayed impulses and applications, IEEE Trans. Automa. Control, 64 (2019), 4024–4034. https://doi.org/10.1109/TAC.2019.2905271 doi: 10.1109/TAC.2019.2905271
![]() |
[31] |
X. Li, D. Ho, J. Cao, Finite-time stability and settling-time estimation of nonlinear impulsive systems, Automatica, 99 (2019), 361–368. https://doi.org/10.1016/j.automatica.2018.10.024 doi: 10.1016/j.automatica.2018.10.024
![]() |
[32] |
X. Li, X. Yang, J. Cao, Event-triggered impulsive control for nonlinear delay systems, Automatica, 117 (2020), 108981. https://doi.org/10.1016/j.automatica.2020.108981 doi: 10.1016/j.automatica.2020.108981
![]() |
[33] |
X. Li, D. Peng, J. Cao, Lyapunov stability for impulsive systems via event-triggered impulsive control, IEEE Trans. Autom. Control, 65 (2020), 4908–4913. https://doi.org/10.1109/TAC.2020.2964558 doi: 10.1109/TAC.2020.2964558
![]() |
[34] |
W. Zhu, D. Wang, L. Liu, G. Feng, Event-based impulsive control of continuous-time dynamic systems and its application to synchronization of Memristive neural networks, IEEE Trans. Neural Networks Learn. Syst., 29 (2018), 3599–3609. https://doi.org/10.1109/TNNLS.2017.2731865 doi: 10.1109/TNNLS.2017.2731865
![]() |
[35] |
S. Ding, Z. Wang, Event-triggered synchronization of discrete-time neural networks: a switching approach, Neural Networks, 125 (2020), 31–40. https://doi.org/10.1016/j.neunet.2020.01.024 doi: 10.1016/j.neunet.2020.01.024
![]() |
[36] |
H. Rao, F. Liu, H. Peng, Y. Xu, R. Lu, Observer-based impulsive synchronization for neural networks with uncertain exchanging information, IEEE Trans. Neural Networks Learn. Syst., 31 (2020), 3777–3787. https://doi.org/10.1109/TNNLS.2019.2946151 doi: 10.1109/TNNLS.2019.2946151
![]() |
[37] |
P. Chen, J. Wang, S. He, X. Luan, F. Liu, Observer-based asynchronous fault detection for conic-type nonlinear jumping systems and its application to separately excited DC motor, IEEE Trans. Circuits Syst. I, 67 (2019), 951–962. https://doi.org/10.1109/TCSI.2019.2949368 doi: 10.1109/TCSI.2019.2949368
![]() |
[38] |
M. de Magistris, M. di Bernardo, E. di Tucci, S. Manfredi, Synchronization of networks of non-identical Chua's circuits: analysis and experiments, IEEE Trans. Circuits Syst. I, 59 (2012), 1029–1041. https://doi.org/10.1109/TCSI.2012.2185279 doi: 10.1109/TCSI.2012.2185279
![]() |
[39] |
F. Liu, C. Liu, R. Rao, Y. Xu, T. Wang, Reliable impulsive synchronization for fuzzy neural networks with mixed controllers, Neural Networks, 143 (2021), 759–766. https://doi.org/10.1016/j.neunet.2021.08.013 doi: 10.1016/j.neunet.2021.08.013
![]() |
[40] |
P. Chen, S. He, V. Stojanovic, X. Luan, F. Liu, Fuzzy fault detection for Markov jump systems with partly accessible hidden information: an event-triggered approach, IEEE Trans. Cybern., 52 (2021), 7352–7361. https://doi.org/10.1109/TCYB.2021.3050209 doi: 10.1109/TCYB.2021.3050209
![]() |
[41] |
H. Rao, F. Liu, H. Peng, Y. Xu, R. Lu, Observer-based impulsive synchronization for neural networks with uncertain exchanging information, IEEE Trans. Neural Networks Learn. Syst., 31 (2020), 3777–3787. https://doi.org/10.1109/TNNLS.2019.2946151 doi: 10.1109/TNNLS.2019.2946151
![]() |
[42] |
L. Wang, Z. Wang, Q. Han, G. Wei, Synchronization control for a class of discrete-time dynamical networks with packet dropouts: a coding-decoding-based approach, IEEE Trans. Cybern., 48 (2018), 2437–2448. https://doi.org/10.1109/TCYB.2017.2740309 doi: 10.1109/TCYB.2017.2740309
![]() |
[43] |
L. Xie, Output feedback H∞ control of systems with parameter uncertainty, Int. J. Control, 63 (1996), 741–750. https://doi.org/10.1080/00207179608921866 doi: 10.1080/00207179608921866
![]() |
[44] |
H. Rao, F. Liu, H. Peng, Y. Xu, R. Lu, Observer-based impulsive synchronization for neural networks with uncertain exchanging information, IEEE Trans. Neural Networks Learn. Syst., 31 (2020), 3777–3787. https://doi.org/10.1109/TNNLS.2019.2946151 doi: 10.1109/TNNLS.2019.2946151
![]() |
Synchronization error | Impulsive control times | General control times |
|e1|≤0.01 | 16 | 27 |
|e2|≤0.01 | 15 | 26 |
|e3|≤0.01 | 19 | 27 |