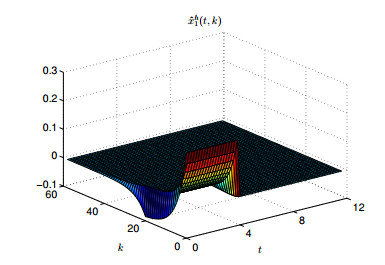
This paper was concerned with the problem of filter design for the continuous-discrete system in the Takagi-Sugeno (T-S) fuzzy model. In a known finite frequency (FF) domain, an FF H∞ performance was defined for the nonlinear continuous-discrete system. With the designed filter, sufficient conditions were then established for the filtering error system to be asymptotically stable and having a prescribed FF H∞ performance. After that, a systematic method for the filter design was proposed. Finally, an example was provided to check effectiveness of the derived results.
Citation: Zhaoxia Duan, Jinling Liang, Zhengrong Xiang. Filter design for continuous-discrete Takagi-Sugeno fuzzy system with finite frequency specifications[J]. Mathematical Modelling and Control, 2023, 3(4): 387-399. doi: 10.3934/mmc.2023031
[1] | Ruxin Zhang, Zhe Yin, Ailing Zhu . Numerical simulations of a mixed finite element method for damped plate vibration problems. Mathematical Modelling and Control, 2023, 3(1): 7-22. doi: 10.3934/mmc.2023002 |
[2] | Xiaoyu Ren, Ting Hou . Pareto optimal filter design with hybrid H2/H∞ optimization. Mathematical Modelling and Control, 2023, 3(2): 80-87. doi: 10.3934/mmc.2023008 |
[3] | Hongli Lyu, Yanan Lyu, Yongchao Gao, Heng Qian, Shan Du . MIMO fuzzy adaptive control systems based on fuzzy semi-tensor product. Mathematical Modelling and Control, 2023, 3(4): 316-330. doi: 10.3934/mmc.2023026 |
[4] | Lusong Ding, Weiwei Sun . Neuro-adaptive finite-time control of fractional-order nonlinear systems with multiple objective constraints. Mathematical Modelling and Control, 2023, 3(4): 355-369. doi: 10.3934/mmc.2023029 |
[5] | Hongwei Zheng, Yujuan Tian . Exponential stability of time-delay systems with highly nonlinear impulses involving delays. Mathematical Modelling and Control, 2025, 5(1): 103-120. doi: 10.3934/mmc.2025008 |
[6] | Weisong Zhou, Kaihe Wang, Wei Zhu . Synchronization for discrete coupled fuzzy neural networks with uncertain information via observer-based impulsive control. Mathematical Modelling and Control, 2024, 4(1): 17-31. doi: 10.3934/mmc.2024003 |
[7] | M. Haripriya, A. Manivannan, S. Dhanasekar, S. Lakshmanan . Finite-time synchronization of delayed complex dynamical networks via sampled-data controller. Mathematical Modelling and Control, 2025, 5(1): 73-84. doi: 10.3934/mmc.2025006 |
[8] | Shaosan Xia, Xianyi Li . Complicate dynamics of a discrete predator-prey model with double Allee effect. Mathematical Modelling and Control, 2022, 2(4): 282-295. doi: 10.3934/mmc.2022027 |
[9] | Biresh Kumar Dakua, Bibhuti Bhusan Pati . A frequency domain-based loop shaping procedure for the parameter estimation of the fractional-order tilt integral derivative controller. Mathematical Modelling and Control, 2024, 4(4): 374-389. doi: 10.3934/mmc.2024030 |
[10] | Jiaquan Huang, Zhen Jia, Peng Zuo . Improved collaborative filtering personalized recommendation algorithm based on k-means clustering and weighted similarity on the reduced item space. Mathematical Modelling and Control, 2023, 3(1): 39-49. doi: 10.3934/mmc.2023004 |
This paper was concerned with the problem of filter design for the continuous-discrete system in the Takagi-Sugeno (T-S) fuzzy model. In a known finite frequency (FF) domain, an FF H∞ performance was defined for the nonlinear continuous-discrete system. With the designed filter, sufficient conditions were then established for the filtering error system to be asymptotically stable and having a prescribed FF H∞ performance. After that, a systematic method for the filter design was proposed. Finally, an example was provided to check effectiveness of the derived results.
In the last few decades, many researchers have investigated two-dimensional (2-D) systems including continuous-continuous, discrete-discrete and continuous-discrete settings, as these systems have great applications in engineering fields such as process control, multi-dimensional digital filtering and image processing; see, for instance, [1] and reference therein. Recently, due to the continuous-discrete systems having an advantage of describing vehicle platoon model [2], linear repetitive processes [3], iterative learning control systems [4] and others, the study of continuous-discrete systems has been a major topic. Now, the main research is about stability problem [5] and feedback control [6].
In practice, many systems have complex characteristics and nonlinearities, which can not be fully described by the linear system models [7,8,9]. Utilizing the Takagi-Sugeno (T-S) fuzzy model [10] to approximate the nonlinear systems via fuzzy sets and fuzzy reasoning is an efficient approach. It is well known that the T-S fuzzy model has the favourable ability to approximate any nonlinear system [11], which provides sufficient tools and techniques for researchers to design complex nonlinear systems [12]. To this day, some designers have tried to investigate the continuous-discrete nonlinear systems via using fuzzy-model-based control methods [13]. Now, the T-S fuzzy model has been an efficient tool to solve nonlinear problems by adopting the mature linear system theories.
On the other hand, the practical systems are often disturbed by the noise signals; therefore, the filtering issues for nonlinear systems have been studied extensively by the T-S fuzzy model approach [14]. In order to describe systems more accurately, filtering problem is considered to estimate the system state by using the known information under the noisy disturbance. One commonly studied scheme for 2-D systems is H∞ filtering [15,16].
Among most of the existing research results on the filtering problem, frequency of the disturbances is usually assumed to occupy the entire frequency domain, which actually brings over-design compared to the finite frequency (FF) case since the useful information of the FF range of disturbances are not fully utilized. In some practical engineering applications, the disturbance signals are intrinsically dominated within FF ranges, which could be known in advance [17]. It is more reasonable and useful to design controllers or filters according to the frequency characteristics of disturbances. The generalized Kalman-Yakubovich-Popov lemma proposed in [18] presented an efficient way in inspecting the FF specifications of the controlled system, which can be directly utilized to derive certain linear matrix inequality (LMI) conditions so as to design appropriate filters. The 2-D generalized Kalman-Yakubovich-Popov lemmas proposed in [19,20] are useful tools when dealing with the problems of H∞ control, H∞ filtering and fault detection observer/filter design for linear 2-D systems in FF domains, which have been studied in [21,22].
It should be noted that the system properties formalized as frequency domain inequalities are inapplicable to nonlinear systems. Due to this reason, it cannot describe the system representations accurately through the method of frequency-domain analysis. Stimulated by the concept of time domain interpretations of the frequency domain inequalities [23], the authors of [24] dealt with the problem of filtering design for nonlinear systems in the T-S fuzzy model by introducing the FF H∞ index. Based on the above theories, the filter design method and FF static output feedback H∞ controller design method have been proposed for Roesser-type 2-D discrete systems in the T-S fuzzy model in [25,26], respectively. The most striking characteristic of continuous-discrete systems lies in that there are a differential equation and a difference equation with respect to the continuous and discrete variables, respectively [27]. Therefore, the results proposed for discrete and continuous 2-D systems are unable to be used for the addressed systems directly in this paper. To the authors' best knowledge, there has been no results on FF H∞ filtering for nonlinear continuous-discrete systems. Thus, it is necessary to develop the FF filtering theory specially for continuous-discrete T-S fuzzy systems. Inspired by these works, the authors aim to design the H∞ filter for continuous-discrete T-S fuzzy systems in this paper.
This paper aims to design a filter for the continuous-discrete system in the T-S fuzzy model with FF disturbances. The main contributions of this work are summarized as follows:
1) Motivated by the time-domain interpretations of the frequency domain inequalities [23], definition of the FF H∞ performance for continuous-discrete systems is proposed, which contains the frequency information of the disturbance input and generalizes the standard H∞ performance.
2) Based on the Parseval's theorem for continuous-discrete systems, the FF H∞ performance analysis results have been obtained, which generalizes the FF bounded real lemma for linear systems [20].
3) Sufficient conditions for the existence of the desired filter and a systematic method for the filter design are developed to ensure the asymptotic stability and FF H∞ performance of the filtering error system. By setting the matrix and decision variables dependent of the membership function, the proposed results are applicable to linear systems, which means that the prosed results are very general.
The paper proceeds as follows. The preliminaries and problem formulation are presented in Section 2. Main results, including FF H∞ performance analysis and filter design are given in Section 3. An illustrative example demonstrates the theoretical results potency in Section 4. Finally, some conclusions are summarized in Section 5.
Notations: R, C and Hn stand for the sets of all real numbers, complex numbers and Hermitian n×n dimensional matrices, respectively. A−1, AT and A∗ denote the inverse, the transpose and the complex conjugate transpose of matrix A, respectively.
He(A):=(A+A∗)/2 |
and tr(A) stand for the Hermitian and the trace of square matrix A, respectively. The notation A>0 (A≥0) means that A is positive definite (positive semi-definite). NA denotes a matrix whose columns form a basis of the null-space of matrix A. The symbol "⋆" represents the term originated by conjugate symmetry in a matrix. The L2 norm of a 2-D signal w(t,k) is given by
‖w‖22=∞∑k=0∫∞t=0wT(t,k)w(t,k)dt. |
w(t,k) is said to belong to L2{[0,∞),⌊0,∞)} if ‖w‖2<∞, where ⌊0,∞):={0,1,2,⋯}.
Consider the continuous-discrete T-S fuzzy system with its i-th rule as follows:
Rule i: IF θ1(t,k) is Mi1, ⋯, and θL(t,k) is MiL, THEN
˜x(t,k)=Aix(t,k)+Biw(t,k),y(t,k)=Cix(t,k)+Diw(t,k),z(t,k)=Lix(t,k)+Eiw(t,k) | (2.1) |
with
˜x(t,k)=[∂xh(t,k)∂txv(t,k+1)],x(t,k)=[xh(t,k)xv(t,k)], |
where i∈N_={1,2,⋯,N}, t∈[0,∞) and k∈⌊0,∞); xh(⋅,⋅)∈Rnh and xv(⋅,⋅)∈Rnv are state vectors that vary in the horizontal and the vertical directions, respectively; x(⋅,⋅) denotes the whole state in Rn with n=nh+nv; w(⋅,⋅)∈Rnw, y(⋅,⋅)∈Rny and z(⋅,⋅)∈Rnz are the disturbance input, the measured output and the signal to be estimated, respectively. θp(t,k), p=1,2,..,L, are the premise variables; Mip is the fuzzy set; L is the number of premise variables; N is the number of IF-THEN rules; Ai, Bi, Ci, Di, Li and Ei are known matrices of appropriate dimensions. The energy of the disturbance w(t,k) is assumed to be dominated in a known rectangular FF region introduced later.
Via using the inference product, the singleton fuzzifer and the center-average defuzzifer, (2.1) can be described by
˜x(t,k)=A(μ)x(t,k)+B(μ)w(t,k),y(t,k)=C(μ)x(t,k)+D(μ)w(t,k),z(t,k)=L(μ)x(t,k)+E(μ)w(t,k) | (2.2) |
with
[A(μ)B(μ)C(μ)D(μ)L(μ)E(μ)]=N∑i=1μi(t,k)[AiBiCiDiLiEi],μi(t,k)=βi(t,k)N∑i=1βi(t,k),βi(t,k)=LΠp=1Mip(θp(t,k)), | (2.3) |
where Mip(θp(t,k)) is the degree of the membership function of θp(t,k) in Mip. Assume that Mip(θp(t,k))≥0 for all i∈N_ and p∈L_={1,2,⋯,L}, then for all t⩾0 and k∈⌊0,∞), the normalized membership function μi(t,k) satisfies
μi(t,k)≥0andN∑i=1μi(t,k)=1. |
The boundary conditions (BCs) associated with (2.1) are
xh(t,k)={h0,t=0,0≤k≤z2,0,t=0,∀k>z2,xv(t,k)={v0,k=0,0≤t≤z1,0,k=0,∀t>z1, |
where h0∈Rnh and v0∈Rnv are given vectors, z1 is a positive scalar and z2 is a positive integer.
In order to estimate the signal z(t,k), a fuzzy Roesser-type filter is designed as follows:
Filter rule i: IF θ1(t,k) is Mi1, ⋯, and θL(t,k) is MiL, THEN
˜xF(t,k)=AF,ixF(t,k)+BF,iy(t,k),zF(t,k)=CF,ixF(t,k)+DF,iy(t,k) | (2.4) |
with xhF(0,k)=0, xvF(t,0)=0 for t≥0 and k∈⌊0,∞),
˜xF(t,k)=[∂xhF(t,k)∂txvF(t,k+1)],xF(t,k)=[xhF(t,k)xvF(t,k)], |
where xhF(⋅,⋅)∈Rnh and xvF(⋅,⋅)∈Rnv are the horizontal and the vertical filter states, respectively; zF(⋅,⋅)∈Rnz is an estimation of z(⋅,⋅); AF,i, BF,i, CF,i and DF,i (i∈N_) are appropriately dimensioned filter matrices to be determined.
The defuzzified output of system (2.4) is obtained as
˜xF(t,k)=AF(μ)xF(t,k)+BF(μ)y(t,k),zF(t,k)=CF(μ)xF(t,k)+DF(μ)y(t,k), | (2.5) |
where
[AF(μ)BF(μ)CF(μ)DF(μ)]=N∑i=1μi(t,k)[AF,iBF,iCF,iDF,i]. | (2.6) |
Defining
ˆz(t,k)=z(t,k)−zF(t,k), |
ˆxh(t,k)=[xh(t,k)xhF(t,k)],ˆxv(t,k)=[xv(t,k)xvF(t,k)] |
and augmenting the filter states with system (2.2), the following filtering error system (FES) can then be obtained
˜ˆx(t,k)=ˆA(μ)ˆx(t,k)+ˆB(μ)w(t,k),ˆz(t,k)=ˆC(μ)ˆx(t,k)+ˆD(μ)w(t,k), | (2.7) |
where
ˆD(μ)=E(μ)−DF(μ)D(μ),˜ˆx=[∂ˆxh(t,k)∂tˆxv(t,k+1)],ˆx(t,k)=[ˆxh(t,k)ˆxv(t,k)],ˆA(μ)=Π[A(μ)0BF(μ)C(μ)AF(μ)]ΠT,ˆC(μ)=[L(μ)−DF(μ)C(μ)−CF(μ)]ΠT,ˆB(μ)=Π[B(μ)BF(μ)D(μ)],Π=[Inh00000Inh00Inv00000Inv]. |
In practice, effects of the noises are usually dominated in FF ranges. Based on the practical situation, denoting the Fourier transform of w(t,k) as W(jwh,ejwv) with j being the imaginary unit, a rectangular FF domain R for the noise signal w(t,k) is given as follows:
R={(wh,wv):wh1≤wh≤wh2,wv1≤wv≤wv2}, | (2.8) |
where wh1,wh2∈R and wv1,wv2∈(−π,π] are the lower and upper bounds of frequency variables wh and wv respectively.
Definition 2.1. For the FF domain R and a given positive scalar γ, the FES (2.7) is said to have an FF H∞ index γ if the following two conditions are satisfied:
(i) When w(t,k)≡0, the FES (2.7) is AS;
(ii) Under the zero BCs, the inequality
‖ˆz‖22<γ2‖w‖22 | (2.9) |
holds for all solutions of (2.7) with
w(⋅,⋅)∈L2{[0,∞),⌊0,∞)}∖{0} |
such that
∞∑k=0∫∞0(wh1ˆxh(t,k)+j∂ˆxh(t,k)∂t)×(wh2ˆxh(t,k)+j∂ˆxh(t,k)∂t)∗dt<0, | (2.10) |
ej(wav)∫∞0[∞∑k=0(ˆxv(t,k+1)−ejwv1ˆxv(t,k))×(ˆxv(t,k+1)−ejwv2ˆxv(t,k))∗]dt<0, | (2.11) |
where
wav=(wv2−wv1)/2. |
The FF filtering problem to be addressed in this paper is to design a fuzzy filter in the form of (2.4), such that the FES (2.7) is AS and has an FF H∞ performance level γ. To get the main results, we still need the following lemma.
Lemma 2.1. ([28]) If the 2-D complex vector functions x(t,k) and y(t,k) belong to L2{[0,∞),⌊0,∞)}, then the corresponding Laplace-Z transforms X(s,z) and Y(s,z) exist and the following relation holds, provided that x(t,k) and y(t,k) have the same dimensions
∞∑k=0∫∞0tr(x(t,k)y∗(t,k))dt=1(2π)2∫π−π∫∞−∞tr(X(jwh,ejwv)Y∗(jwh,ejwv))dwhdwv. |
In the following, one sufficient condition is presented for the FES (2.7) having an FF H∞ performance.
Theorem 3.1. Assume that the FES (2.7) is asymptotically stable (AS). For the FF domain R and the scalar γ>0, the FES (2.7) has an FF H∞ performance γ if there exist symmetric matrices
ˆP=diag{ˆPh,ˆPv}∈R2n×2n |
and
ˆQ=diag{ˆQh,ˆQv}∈R2n×2n, |
such that ˆPh>0, ˆQv>0 and
[ˆA(μ)ˆB(μ)I0]T[−ˆP−ˆΛ∗ˆPˆW+ˆΛ∗ˆQ−ˆW∗ˆPˆΛ+ˆQˆΛˆLhˆP−ˆW∗ˆPˆW−ˆLvˆQ]×[ˆA(μ)ˆB(μ)I0]+[ˆCT(μ)ˆC(μ)ˆCT(μ)ˆD(μ)ˆDT(μ)ˆC(μ)−γ2I+ˆDT(μ)ˆD(μ)]<0 | (3.1) |
with
ˆΛ=diag{I2nh,e−j(wcv)I2nv}, ˆW=diag{−j(wch)I2nh,02nv}, |
ˆLv=diag{0,2cos(wav)I2nv}, ˆLh=diag{(wah)2I2nh,I2nv}, |
wch=(wh1+wh2)/2, wah=(wh2−wh1)/2, wcv=(wv1+wv2)/2. |
Proof. Multiplying the inequality (3.1) by [ˆxT(t,k)wT(t,k)] from the left and by its transpose from the right with
w(⋅,⋅)∈L2{[0,∞),⌊0,∞)}∖{0}, |
we have
[˜ˆx(t,k)ˆx(t,k)]T[−ˆP−ˆΛ∗ˆPˆW+ˆΛ∗ˆQ−ˆW∗ˆPˆΛ+ˆQˆΛˆLhˆP−ˆW∗ˆPˆW−ˆLvˆQ]×[˜ˆx(t,k)ˆx(t,k)]+Φ(t,k)=(∂ˆxh(t,k)∂t)TˆQhˆxh(t,k)+(ˆxh(t,k))TˆQh∂ˆxh(t,k)∂t−(ˆxv(t,k+1))TˆPvˆxv(t,k+1)+(ˆxv(t,k))TˆPvˆxv(t,k)+Φ(t,k)−tr(ˆQv[−e−j(wcv)ˆxv(t,k+1)(ˆxv(t,k))T−ej(wcv)ˆxv(t,k)(ˆxv(t,k+1))T+2ˆxv(t,k)cos(wav)×(ˆxv(t,k))T])−tr(ˆPhHe((wh1ˆxh(t,k)+j∂ˆxh(t,k)∂t)×(wh2ˆxh(t,k)+j∂ˆxh(t,k)∂t)∗))<0 | (3.2) |
with
Φ(t,k)=[ˆx(t,k)w(t,k)]T[ˆCT(μ)ˆC(μ)ˆCT(μ)ˆD(μ)ˆDT(μ)ˆC(μ)−γ2I+ˆDT(μ)ˆD(μ)]×[ˆx(t,k)w(t,k)]. |
Taking integration for t from zero to ∞ and summation for integer k from zero to ∞, in view of the zero BCs, we have
∞∑k=0∫∞0Φ(t,k)dt−tr(He(ˆPhSh))−tr(ˆQvSv)<0, | (3.3) |
where
Sh:=∞∑k=0∫∞0(wh1ˆxh(t,k)+j∂ˆxh(t,k)∂t)×(wh2ˆxh(t,k)+j∂ˆxh(t,k)∂t)∗dt,Sv:=∫∞0∞∑k=0{−e−j(wcv)ˆxv(t,k+1)(ˆxv(t,k))T−ej(wcv)ˆxv(t,k)×(ˆxv(t,k+1))T+ˆxv(t,k)2cos(wav)(ˆxv(t,k))T}dt. |
Clearly, Sv is Hermitian, and it follows from condition (2.10) that Sh<0. Due to the zero BCs, it is true that
Sv=ej(wav)∫∞0∞∑k=0[(ˆxv(t,k+1)−ejwv1ˆxv(t,k))×(ˆxv(t,k+1)−ejwv2ˆxv(t,k))∗]dt, |
and combining with constraint (2.11) infers that Sv<0. Applying Lemma 2.1 to Sh, we have
Sh=1(2π)2∫π−π∫∞−∞(wh1−wh)(wh2−wh)ˆXh(jwh,ejwv)×(ˆXh(jwh,ejwv))∗dwhdwv, |
which directly guarantees that Sh is also Hermitian, where ˆXh(jwh,ejwv) denotes the 2-D Laplace-Z transform of ˆxh(t,k). Additionally, since ˆPh>0 and ˆQv>0, −tr(He(ˆPhSh))−tr(ˆQvSv) is also positive, then it follows from (3.3) that
∞∑k=0∫∞0Φ(t,k)dt<0, |
which means that FES (2.7) has an FF H∞ performance γ according to Definition 2.1. This completes the proof.
To further achieve the main result, the following lemma is needed to guarantee the asymptotic stability of FES (2.7).
Lemma 3.1. [28] The FES (2.7) with zero input is AS if positive definite matrices ˉPh∈R2nh×2nh and ˉPv∈R2nv×2nv exist such that
[ˆA(μ)I]T[ˉQ11ˉQ12ˉQ12ˉQ22][ˆA(μ)I]<0 | (3.4) |
holds, where
ˉQ11=diag{0,ˉPv}, ˉQ12=diag{ˉPh,0} |
and
ˉQ22=diag{0,−ˉPv}. |
Lemma 3.1 gives a sufficient condition ensuring the considered system to be AS. To facilitate the filter design, it is necessary to decouple the product terms between the matrix variables ˆP, ˆQ and the system matrices ˆA(μ), ˆB(μ) in (3.1). Thus, we need to present an alternative of Theorem 3.1.
Theorem 3.2. For the FF domain R and the scalar γ>0, the FES (2.7) has an FF H∞ performance γ if there exist matrices ˆG(μ), ˆF(μ), ˉG(μ), ˉF(μ)∈R2n×2n and ˆH(μ)∈Rnw×2n, symmetric matrices
ˆP=diag{ˆPh,ˆPv}∈R2n×2n, ˉPh∈R2nh×2nh, ˉPv∈R2nv×2nv |
and
ˆQ=diag{ˆQh,ˆQv}∈R2n×2n, |
such that ˆPh>0, ˆQv>0, ˉPh>0, ˉPv>0 and
[Φ11(μ)Φ12(μ)Φ13(μ)0⋆Φ22(μ)Φ23(μ)ˆCT(μ)⋆⋆Φ33(μ)ˆDT(μ)⋆⋆⋆−Inz]<0, | (3.5) |
[ˉQ11−2He(ˉGT(μ))ˉQ12+ˉG(μ)ˆA(μ)−ˉFT(μ)⋆ˉQ22+2He(ˆAT(μ)ˉFT(μ))]<0, | (3.6) |
where
Φ11(μ)=−ˆP−ˆG(μ)−ˆGT(μ),Φ12(μ)=−ˆΛ∗ˆPˆW+ˆΛ∗ˆQ+ˆG(μ)ˆA(μ)−ˆFT(μ),Φ13(μ)=ˆG(μ)ˆB(μ)−ˆHT(μ),Φ22(μ)=ˆLhˆP−ˆLvˆQ−ˆW∗ˆPˆW+2He(ˆF(μ)ˆA(μ)),Φ23(μ)=ˆF(μ)ˆB(μ)+ˆAT(μ)ˆHT(μ)Φ33(μ)=−γ2I+2He(ˆH(μ)ˆB(μ)), |
and the other notations are the same as defined in Theorem 3.1 and Lemma 3.1.
Proof. Define
Γ(μ)=[−ˆP−ˆΛ∗ˆPˆW+ˆΛ∗ˆQ0−ˆW∗ˆPˆΛ+ˆQˆΛΓ22(μ)ˆCT(μ)ˆD(μ)0ˆDT(μ)ˆC(μ)Γ33(μ)],U(μ)=[−I2nˆA(μ)ˆB(μ)],Y(μ)=[ˆGT(μ)ˆFT(μ)ˆHT(μ)]T, |
where
Γ22(μ)=ˆLhˆP−ˆLvˆQ−ˆW∗ˆPˆW+ˆCT(μ)ˆC(μ) |
and
Γ33(μ)=−γ2I+ˆDT(μ)ˆD(μ). |
Then, we have
Ψ(μ):=Y(μ)U(μ)+UT(μ)YT(μ)+Γ(μ)=[Ψ11(μ)Ψ12(μ)Ψ13(μ)⋆Ψ22(μ)Ψ23(μ)⋆⋆Ψ33(μ)] | (3.7) |
with
Ψ11(μ)=−ˆP−ˆG(μ)−ˆGT(μ),Ψ12(μ)=−ˆΛ∗ˆPˆW+ˆΛ∗ˆQ+ˆG(μ)ˆA(μ)−ˆFT(μ),Ψ22(μ)=ˆLhˆP−ˆLvˆQ−ˆW∗ˆPˆW+ˆCT(μ)ˆC(μ)+2He(ˆF(μ)ˆA(μ)),Ψ13(μ)=ˆG(μ)ˆB(μ)−ˆHT(μ),Ψ23(μ)=ˆCT(μ)ˆD(μ)+ˆF(μ)ˆB(μ)+ˆAT(μ)ˆHT(μ)Ψ33(μ)=−γ2I+ˆDT(μ)ˆD(μ)+2He(ˆH(μ)ˆB(μ)). |
By Schur complement lemma, Ψ(μ)<0 is equivalent to inequality (3.5), then by taking
NU(μ)=[ˆA(μ)ˆB(μ)I2n00Inw], |
pre- and post-multiplying both sides of Ψ(μ)<0 by NTU(μ) and NU(μ), respectively, and noting that NU(μ) is column full rank, it is known that inequality (3.1) does hold.
In addition, we set
ˉΓ(μ)=[ˉQ11ˉQ12ˉQ12ˉQ22],ˉY(μ)=[ˉG(μ)ˉF(μ)],ˉU(μ)=[−I2nˆA(μ)], |
where ˉQ11, ˉQ12 and ˉQ22 are defined in Lemma 3.1. The constraint condition (3.6) is exactly
ˉΨ(μ):=ˉY(μ)ˉU(μ)+ˉUT(μ)ˉYT(μ)+ˉΓ(μ)<0. |
Similarly, taking
ˉNU(μ)=[ˆAT(μ)I2n]T, |
pre- and post-multiplying both sides of ˉΨ(μ)<0 by NTˉU(μ) and NˉU(μ), respectively, and noting that NˉU(μ) is column full rank, it follows that
[ˆA(μ)I2n]T[ˉQ11ˉQ12ˉQ12ˉQ22][ˆA(μ)I2n]<0. |
From Lemma 3.1, we know that the FES (2.7) with w(⋅,⋅)≡0 is AS. The proof is completed by resorting to Theorem 3.1 and Definition 2.1.
Remark 3.1. The slack matrices ˆG(μ), ˆF(μ), ˉG(μ), ˉF(μ) and ˆH(μ) are introduced to decouple the products between the Lyapunov matrices ˆP, ˉPh, ˉPv, ˆQ and the system matrices, which aids in the filter design while also bringing certain conservatism.
The following result can be derived via specifying the structure of the slack matrices in Theorem 3.2 and coverting the filter design problem to a group of LMI constraints, which are numerically tractable.
Theorem 3.3. For the FF domain R and the scalar γ>0, the FES (2.7) has an FF H∞ performance γ if there exist matrices
VAF,i∈Rn×n, VBF,i∈Rn×ny, CF,i∈Rnz×n, DF,i∈Rnz×ny,H1,i∈Rnw×n, P2=diag{Ph2,Pv2}∈Rn×n,Q2=diag{Qh2,Qv2}∈Rn×n,Gl,i,Fl,i,ˉGl,i,ˉFl,i∈Rn×n, (l=1,2),V∈Rn×n |
and symmetric matrices
Pf=diag{Phf,Pvf}∈Rn×n,Qf=diag{Qhf,Qvf}∈Rn×n, (f=1,3),Phs∈Rnh×nh, ˉPvs∈Rnv×nv, (s=1,2,3), |
such that
[diag{ˉPh1,ˉPv1}diag{ˉPh2,ˉPv2}⋆diag{ˉPh3,ˉPv3}]>0, | (3.8) |
[Ph1Ph2⋆Ph3]>0,[Qv1Qv2⋆Qv3]>0, | (3.9) |
[T11,iiT12,ijT13,ij0⋆T22,ijT23,ij˜CTi,j⋆⋆T33,ijˆDTi,j⋆⋆⋆−Inz]<0, | (3.10) |
[L11,iiL12,ij⋆L22,ij]<0 | (3.11) |
hold for all i,j∈N_, where
T11,ii=[−P1−G1,i−GT1,i−P2−V−GT2,i⋆−P3−V−VT],T13,ij=[G1,iBj+VBF,iDj−HT1,iG2,iBj+VBF,iDj],T12,ij=[−Λ∗P1W+Λ∗Q1+G1,iAj+VBF,iCj−FT1,i−Λ∗PT2W+Λ∗QT2+G2,iAj+VBF,iCj−Λ∗P2W+Λ∗Q2+VAF,i−FT2,i−Λ∗P3W+Λ∗Q3+VAF,i],T22,ij=[LhP1−LvQ1−W∗P1W+2He(F1,iAj)⋆LhP2−LvQ2−W∗P2W+ATjFT2,iLhP3−W∗P3W−LvQ3],T23,ij=[F1,iBj+ATjHT1,iF2,iBj],T33,ij=−γ2Inw+2He(H1,iBj),˜Cij=[Lj−DF,iCj−CF,i],ˆDij=Ej−DF,iDj,L11,ii=[diag{0,ˉPv1}−ˉG1,i−ˉGT1,i⋆diag{0,ˉPv2}−V−ˉGT2,idiag{0,ˉPv3}−V−VT],L12,ij=[diag{ˉPh1,0}+ˉG1,iAj+VBF,iCj−ˉFT1,idiag{ˉPh2,0}+ˉG2,iAj+VBF,iCj−VTdiag{ˉPh2,0}+VAF,i−ˉFT2,idiag{ˉPh3,0}+VAF,i−VT],L22,ij=[−diag{0,ˉPv1}+2He(ˉF1,iAj+VBF,iCj)⋆−diag{0,ˉPv2}+VAF,i+ATjˉFT2,i+CTjVTBF,i−diag{0,ˉPv3}+2He(VAF,i)]. |
Moreover, the coefficients of filter (2.4) are designed as
AF,i=V−1VAF,i,BF,i=V−1VBF,i. | (3.12) |
Proof. Parameterize the slack matrices ˆG(μ), ˆF(μ), ˉG(μ), ˉF(μ) and ˆH(μ) as
ˆG(μ)=Π[G1(μ)VG2(μ)V]ΠT,ˆF(μ)=Π[F1(μ)0F2(μ)0]ΠT,ˉG(μ)=Π[ˉG1(μ)VˉG2(μ)V]ΠT,ˉF(μ)=Π[ˉF1(μ)VˉF2(μ)V]ΠT,ˆH(μ)=[H1(μ)0]ΠT | (3.13) |
with \Pi given in (2.7). Define matrix variables \hat P , \bar P^h , \bar P^v and \hat Q as follows:
\begin{align} \hat P & = \Pi \left[ {\begin{array}{*{20}{c}} {{P_1}}&{{P_2}}\\ \star &{{P_3}} \end{array}} \right]{\Pi ^ T },\quad\;\; \hat Q = \Pi \left[ {\begin{array}{*{20}{c}} {{Q_1}}&{{Q_2}}\\ \star &{{Q_3}} \end{array}} \right]{\Pi ^ T }, \\ {\bar{Q}_{12}} & = \Pi \left[ {\begin{array}{*{20}{c}} {{\rm{diag}} \{ {\bar P_1^h,0} \}}&{{\rm{diag}} \{ {\bar P_2^h,0} \}}\\ \star &{{\rm{diag}} \{ {\bar P_3^h,0} \}} \end{array}} \right]{\Pi ^ T },\\ {\bar{Q}_{11}} & = - {\bar{Q}_{22}} = \Pi \left[ {\begin{array}{*{20}{c}} {{\rm{diag}} \{ {0,\bar P_1^v} \}}&{{\rm{diag}} \{ {0,\bar P_2^v} \}}\\ \star &{{\rm{diag}} \{ {0,\bar P_3^v} \}} \end{array}} \right]{\Pi ^ T }, \end{align} | (3.14) |
i.e.,
\begin{align*} \bar{P}^v = \left[ \begin{array}{cc} \bar{P}_1^v&\bar{P}_2^v\\ \star&\bar{P}_3^v \end{array} \right],\qquad \bar{P}^h = \left[ \begin{array}{cc} \bar{P}_1^h&\bar{P}_2^h\\ \star&\bar{P}_3^h \end{array} \right]. \end{align*} |
In addition, we can obtain the following equalities
\begin{align} \hat \Lambda & = \Pi \left[ {\begin{array}{*{20}{c}} \Lambda &0\\ 0&\Lambda \end{array}} \right]{\Pi ^ T },\;\;\ \ \ {\hat L_v} = \Pi \left[ {\begin{array}{*{20}{c}} {{L_v}}&0\\ 0&{{L_v}} \end{array}} \right]{\Pi ^ T },\\ {\hat L_h} & = \Pi \left[ {\begin{array}{*{20}{c}} {{L_h}}&0\\ 0&{{L_h}} \end{array}} \right]{\Pi ^ T },\;\;\; \hat W = \Pi \left[ {\begin{array}{*{20}{c}} W &0\\ 0&W \end{array}} \right]{\Pi ^ T }. \end{align} | (3.15) |
Due to {\Pi}^T\Pi = I , taking
{V_{AF}}(\mu ) = V{A_F}(\mu ),\ \ \ {V_{BF}}(\mu ) = V{B_F}(\mu ) |
and putting (3.8), (3.9), (3.13)–(3.15) into (3.5) and (3.6), respectively, we have
\begin{align} & \left[ {\begin{array}{*{20}{c}} {{\Phi _{11}}(\mu )}&{{\Phi _{12}}(\mu )}&{{\Phi _{13}}(\mu )}&0\\ \star &{{\Phi _{22}}(\mu )}&{{\Phi _{23}}(\mu )}&{{{\hat C}^ T }(\mu )}\\ \star & \star &{{\Phi _{33}}(\mu )}&{{{\hat D}^ T }(\mu )}\\ \star & \star & \star &{ - {I_{{n_z}}}} \end{array}} \right] < 0, \end{align} | (3.16) |
\begin{align} & \left[ {\begin{array}{*{20}{c}} {{\Delta _{11}}(\mu )}&{{\Delta _{12}}(\mu )}\\ \star&{{\Delta _{22}}(\mu )} \end{array}} \right] < 0 \end{align} | (3.17) |
with
\begin{align*} {\Phi _{11}}(\mu ) & = \Pi {\Xi _{11}}(\mu ){\Pi ^ T },\ \ {\Phi _{12}}(\mu ) = \Pi {\Xi _{12}}(\mu ){\Pi ^ T },\\ {\Phi _{13}}(\mu ) & = \Pi {\Xi _{13}}(\mu ), \quad {\Phi _{22}}(\mu ) = \Pi {\Xi _{22}}(\mu ){\Pi ^ T },\\ {\Phi _{23}}(\mu ) & = \Pi {\Xi _{23}}(\mu ), \quad {\Phi _{33}}(\mu ) = {\Xi _{33}}(\mu ),\\ \hat C(\mu )& = \tilde C(\mu ){\Pi ^ T }, \quad {\Delta _{11}}(\mu ) = \Pi {L_{11}}(\mu ){\Pi ^ T }\\ {\Delta _{12}}(\mu )& = \Pi {L_{12}}(\mu ){\Pi ^ T }, \quad {\Delta _{22}}(\mu) = \Pi {L_{{\rm{22}}}}(\mu ){\Pi ^ T }. \end{align*} |
Define
\begin{align*} {V_{AF}}(\mu ) = & \sum\limits_{i = 1}^N {{\mu _i}(t,k)} {V_{AF,i}},\;\;\;{V_{BF}}(\mu ) = \sum\limits_{i = 1}^N {{\mu _i}(t,k)} {V_{BF,i}},\\ {H_1}(\mu ) = & \sum\limits_{i = 1}^N {{\mu _i}(t,k)} {H_{1,i}},\;\;\;\;\;\;\ {G_l}(\mu ) = \sum\limits_{i = 1}^N {{\mu _i}(t,k)} {G_{l,i}},\\ {F_l}(\mu ) = & \sum\limits_{i = 1}^N {{\mu _i}(t,k)} {F_{l,i}},\;\;\;\;\;\;\;\;{{\bar G}_l}(\mu ) = \sum\limits_{i = 1}^N {{\mu _i}(t,k)} {{\bar G}_{l,i}},\\ {{\bar F}_l}(\mu ) = &\sum\limits_{i = 1}^N {{\mu _i}(t,k)} {{\bar F}_{l,i}} \end{align*} |
with l = 1, \; 2 . Pre- and post-multiplying both sides of (3.16) by {\rm{diag}} \{ {{\Pi ^ T }, {\Pi ^ T }, I, I} \} and {\rm{diag}} \{ {{\Pi }, {\Pi }, I, I} \} , respectively, (3.16) is equivalent to condition (3.10). Similarly, pre- and post-multiplying both sides of (3.17) by {\rm{diag}} \{ {{\Pi ^ T }, {\Pi ^ T }} \} and {\rm{diag}}\{{{\Pi}, {\Pi}}\} , respectively, (3.17) is equivalent to condition (3.11). It is noted that (3.11) guarantees -V-V^T < 0 , which means that V is nonsingular and the filter coefficients could be calculated by (3.12). This concludes the proof.
From the above result, it is known that by solving the convex optimization problem,
\begin{equation} \min \; {\gamma ^2}\;\; s.t. \;\; {\rm LMIs} \;\; (3.8)-(3.11), \end{equation} | (3.18) |
a sub-optimal filter with filter gains designed in (3.12) could be achieved with FF H_\infty performance \gamma^{**} , which is the minimum value of \gamma .
Remark 3.2. The conditions {\bar P}^h > 0 , {\bar P}^v > 0 , {\hat P}^h > 0 and {\hat Q}^v > 0 in Theorem 3.2 are implied by (3.8) and (3.9) in Theorem 3.3, utilizing the equivalent variable transformation.
The filter design method will be applied to a practical process of gas absorption, which is represented by the following nonlinear differential equation
\begin{align} \frac{{{\partial ^{\rm{2}}}\varphi (p,q)}}{{\partial p\partial q}} = & {a_1}\frac{{\partial \varphi (p,q)}}{{\partial q}} + {a_2}\frac{{\partial \varphi (p,q)}}{{\partial p}} + bw(p,q) \\ & + {a_0}( 1-0.25 {\sin ^2}(\varphi (p,q)))\varphi (p,q), \end{align} | (4.1) |
where \varphi (p, q) is the variable function; a_0 , a_1 , a_2 and b are real coefficients; w(p, q) is the disturbance input; the BCs are
\varphi (p,0) = {\varphi _1}(p)\; \; \text{and}\; \; \varphi (0,q) = {\varphi _2}(q) . |
Define
\begin{align*} {\varsigma}(p,q)& = \frac{{\partial \varphi (p,q)}}{{\partial q}} - {a_2}\varphi (p,q),\ {x^h}(t,k) = {\varsigma}(t,k): = {\varsigma}(t,kT), \\ {x^v}(t,k) & = \varphi (t,k): = \varphi (t,kT),\ \frac{{\partial {x^v}(t,k)}}{{\partial {k}}}\cong \frac{{ {x^v}(t,k+1)-x^v(t,k)}}{T}, \end{align*} |
where T is the difference step. Next, the following continuous-discrete model can be obtained
\begin{align} \left[ {\begin{array}{*{20}{c}} {\frac{{\partial {x^h}(t,k)}}{{\partial t}}}\\ {x^v}(t,k+1) \end{array}} \right] = & \left[ {\begin{array}{*{20}{c}} {{a_1}}&{{a_1}{a_2} + {a_0} - 0.25a_0{{\sin }^2}({x^v}(t,k)))}\\ T&{{1+Ta_2}} \end{array}} \right] \\ & \times \left[ {\begin{array}{*{20}{c}} {{x^h}(t,k)}\\ {{x^v}(t,k)} \end{array}} \right] + \left[ {\begin{array}{*{20}{c}} b\\ 0 \end{array}} \right]w(t,k) \end{align} | (4.2) |
with BCs
{x^h}(0,k) = \varphi (0,k+1)/T-(1/T+a_2)\varphi (0,k) |
and
x^v(t,0) = \varphi (t,0). |
Take the membership functions
{\mu _1}(t,k) = 1 - {\sin ^2}({x^v}(t,k))\; \; \text{and}\; \; {\mu _2}(t,k) = {\sin ^2}({x^v}(t,k)) |
in consideration of two IF-THEN rules. System (4.2) could be further approximated by the following continuous-discrete T-S fuzzy system
IF {\sin ^2}({x^v}(t, k)) is about zero, THEN
\begin{align} \left[ {\begin{array}{*{20}{c}} {\frac{{\partial {x^h}(t,k)}}{{\partial t}}}\\ {x^v(t,k+1)} \end{array}} \right] = {A_1} \left[ {\begin{array}{*{20}{c}} {{x^h}(t,k)}\\ {{x^v}(t,k)} \end{array}} \right] + {B_1}w(t,k). \end{align} | (4.3) |
IF {\sin ^2}({x^v}(t, k)) is about one, THEN
\begin{align} \left[ {\begin{array}{*{20}{c}} {\frac{{\partial {x^h}(t,k)}}{{\partial t}}}\\ {x^v(t,k+1)} \end{array}} \right] = {A_2} \left[ {\begin{array}{*{20}{c}} {{x^h}(t,k)}\\ {{x^v}(t,k)} \end{array}} \right] + {B_2}w(t,k), \end{align} | (4.4) |
where
\begin{align*} &{A_1} = \left[ {\begin{array}{*{20}{c}} {{a_1}}&{{a_1}{a_2}+a_0}\\ {T}&{{1+a_2T}} \end{array}} \right],\;\; {B_1} = {B_2} = \left[ {\begin{array}{*{20}{c}} b\\ 0 \end{array}} \right],\\ & {A_2} = \left[ {\begin{array}{*{20}{c}} {{a_1}}&{{a_1}{a_2} +0.75 {a_0} }\\ {T}&{{1+a_2T}} \end{array}} \right]. \end{align*} |
Letting {a_0} = -2.4 , {a_1} = -0.4 , {a_2} = -5.5 , b = - 0.1 and T = 0.2 , the other matrix parameters are also taken as follows for the purpose of discussion: {E_1} = 1.5 , {E_2} = 1 ,
\begin{align*} {A_1}& = \Big[ {\begin{array}{*{20}{c}} { - 0.4}&{-0.2}\\ {0.2}&{ - 0.1} \end{array}} \Big],\;\; {B_1} = {B_2} = \Big[ {\begin{array}{*{20}{c}} { - 0.{\rm{1}}}\\ 0 \end{array}} \Big],\\ {D_1}& = 0.1,\;\; {C_1} = { \Big[ {\begin{array}{*{20}{c}} { - {\rm{1}}}\\ 1 \end{array}} \Big]^T},\\ {A_2}& = \Big[ {\begin{array}{*{20}{c}} { - 0.4}&{ 0.4}\\ {0.2}&{ - 0.1} \end{array}} \Big],\;\; {C_2} = { \Big[ {\begin{array}{*{20}{c}} {0.3}\\ {0.3} \end{array}} \Big]^T},\\ {L_1}& = [ {\begin{array}{*{20}{c}} 1&{ - 1} \end{array}} ], \;\; {D_2} = 0.7,\;\; {L_2} = [ {\begin{array}{*{20}{c}} { - 1.2}&1 \end{array}} ]. \end{align*} |
The aim is to design a fuzzy continuous-discrete filter in the form of (2.5) to guarantee the asymptotic stability and an FF H_\infty performance of the FES (2.7). The FF domain of the disturbance input signal is taken from interval [0.5, 13.06] \times [0, 1.57] . Via solving the sub-optimal problem (3.18), the sub-optimal FF H_\infty performance level \gamma^{**} = 1.9112 is obtained and the corresponding solution to the LMIs is available, which is partially presented for space consideration
\begin{align*} & { V_{AF,1}} = \left[ {\begin{array}{*{20}{c}} { -1.5152 }&{0.0243} \\ { 0.0213 }&{-0.2846} \end{array}} \right],\; {V_{BF,1}} = \left[ {\begin{array}{*{20}{c}} {0.0694}\\ {0.1927} \end{array}} \right],\\ & { V_{AF,2}} = \left[ {\begin{array}{*{20}{c}} { -1.5209 }&{0.0243} \\ { 0.0235 }&{-0.2846} \end{array}} \right],\; {V_{BF,2}} = \left[ {\begin{array}{*{20}{c}} {0.0458}\\ {0.2574} \end{array}} \right]. \end{align*} |
By (3.12), the obtained matrix parameters of the FF H_\infty filter are designed as follows: D_{F, 1} = -0.1157 , D_{F, 2} = 1.0978 ,
\begin{align*} &{ A_{F,1}} = \left[ {\begin{array}{*{20}{c}} { -0.5950 }&{0.0078} \\ { 0.0203 }&{-0.1779} \end{array}} \right],\quad {B_{F,1}} = \left[ {\begin{array}{*{20}{c}} {0.0249}\\ {0.1201} \end{array}} \right],\\ &{ A_{F,2}} = \left[ {\begin{array}{*{20}{c}} { -0.0214 }&{0.0003} \\ { 0.0003 }&{-0.0040} \end{array}} \right],\quad {B_{F,2}} = \left[ {\begin{array}{*{20}{c}} {0.0006}\\ {0.0036} \end{array}} \right],\\ &{C_{F,1}} = \left[ {\begin{array}{*{20}{c}} { 0.1303 }&{0.0021} \end{array}} \right],\ \ {C_{F,2}} = \left[ {\begin{array}{*{20}{c}} { 0.0620 }&{0.0258} \end{array}} \right]. \end{align*} |
In the following, simulation results are provided. First, Figures 1–5 show the trajectories of \hat x_1^h(t, k) , \hat x_2^h(t, k) , \hat x_1^v(t, k) , \hat x_2^v(t, k) and \hat z(t, k) of the FES without disturbance input, respectively, where the BCs are
\begin{align*} {\hat{x}^h}(t,k) & = \left\{ {\begin{array}{*{20}{l}} {{ [ {\begin{array}{*{20}{c}} {0.2}&0 \end{array}} ]^T},\;\;\; \;\; t = 0, \; 0 \le k \le 20},\\ {{ [ {\begin{array}{*{20}{c}} {0\;\;\,}&0 \end{array}} ]^T}, \;\;\;\;\; t = 0,\;k > 20}, \end{array}}\right. \\ {\hat{x}^v}(t,k) & = \left\{ {\begin{array}{*{20}{l}} {{ [ {\begin{array}{*{20}{c}} {0.2}&0 \end{array}} ]^T},\;\;\;\;\; 0 \le t \le 4, \;k = 0},\\ {{ [ {\begin{array}{*{20}{c}} {0\;\;\;}&0 \end{array}}]^T},\;\;\;\;\; t > 4,\; k = 0.} \end{array}}\right. \end{align*} |
Figures 1–4 further show that the FES is AS. Next, take the disturbance input as follows:
\begin{align} w( {t,k} ) = & 0.8\cos \left( {{6.78}(t - 0.5)} \right) \\ &\times [u(t) - u(t - 1)]\frac{{\sin \left( {1.57k} \right)}}{\pi k}, \end{align} | (4.5) |
where u(t) is the unit step function. Applying the Fourier transformation, the FF domain of the disturbance input signal (4.5) is [0.5, 13.06] \times [0, 1.57] . Under the zero BCs, it can be calculated that {\| \hat z\|}_2/{{\| w\|}_2}\doteq 1.5338 with the disturbance input (4.5). Thus, the constraint (2.9) is satisfied, which means that the FES satisfies a prescribed FF H_\infty performance \gamma^{**} = 1.9112 .
Finally, to investigate the relationship between the size of the FF domain and the conservativeness of the proposed result, the obtained sub-optimal values of \gamma based on Theorem 3.3 for different FF sets of disturbances are displayed in Table 1. From Table 1, we find that, for a fixed continuous component interval, \gamma^{**} becomes larger as the discrete one becomes wider; while for a fixed discrete component interval, if the continuous part is wider, then the obtained \gamma^{**} is larger. That is, a larger FF domain leads to a more conservative filtering performance.
Frequency domain | \gamma ^{**} | Feasibility |
[5.2, 8.7]\times[0, 1.57] | 1.4013 | \checkmark |
[0.5, 13.06]\times[0, 1.57] | 1.9112 | \checkmark |
[0.5, 13.06]\times[0.5, 1.2] | 1.3135 | \checkmark |
[0,130.6]\times[-2, 3] | Null | \times |
[0, 1.3 \times 10^8]\times[-\pi, \pi] | Null | \times |
The filter design problem has been concerned for the continuous-discrete T-S fuzzy systems in the Roesser model with FF disturbances. The systematic method has been proposed for the filter design, with which the FES was AS and had an FF H_\infty performance. A simulation case further showed validity of the results discussed above. Applying the similar techniques to FF fault detection problems and extending the proposed technique to the 2-D T-S fuzzy systems with delays are important and challenging research topics that deserve more future efforts.
The authors declare they have not used Artificial Intelligence (AI) tools in the creation of this article.
This work was supported in part by the National Natural Science Foundation of China under Grant (No. 62373191), in part by the China Postdoctoral Science Foundation funded project under Grant (No. 2020M671293), in part by the Jiangsu Planned Projects for Postdoctoral Research Fund under Grant (No. 2020Z125), and in part by the Fundamental Research Funds for the Central Universities under Grant (No. 2242023K40010).
All authors declare that there are no conflicts of interest in this paper.
[1] |
M. S. Branicky, V. S. Borkar, S. K. Mitter, A unified framework for hybrid control: model and optimal control theory, IEEE Trans. Autom. Control, 43 (1998), 31–45. https://doi.org/10.1109/9.654885 doi: 10.1109/9.654885
![]() |
[2] | S. Knorn, A two-dimensional systems stability analysis of vehicle platoons, Ph. D. thesis, National University of Ireland Maynooth, 2013. |
[3] |
L. Wu, H. Gao, C. Wang, Quasi sliding mode control of differential linear repetitive processes with unknown input disturbance, IEEE Trans. Ind. Electron., 58 (2011), 3059–3068. https://doi.org/10.1109/TIE.2010.2072891 doi: 10.1109/TIE.2010.2072891
![]() |
[4] |
D. A. Bristow, M. Tharayil, A. G. Alleyne, A survey of iterative learning control, IEEE Control Syst. Mag., 26 (2006), 96–114. https://doi.org/10.1109/MCS.2006.1636313 doi: 10.1109/MCS.2006.1636313
![]() |
[5] |
L. Wang, W. Wang, J. Gao, W. Chen, Stability and robust stabilization of 2-D continuous-discrete systems in Roesser model based on KYP lemma, Multidimens. Syst. Signal Process., 28 (2017), 251–264. https://doi.org/10.1007/s11045-015-0355-2 doi: 10.1007/s11045-015-0355-2
![]() |
[6] |
G. Chesi, R. H. Middleton, LMI-based fixed order output feedback synthesis for two-dimensional mixed continuous-discrete-time systems, IEEE Trans. Autom. Control, 63 (2018), 960–972. https://doi.org/10.1109/TAC.2017.2727685 doi: 10.1109/TAC.2017.2727685
![]() |
[7] |
X. Wang, Y. Sun, D. Ding, Adaptive dynamic programming for networked control systems under communication constraints: a survey of trends and techniques, Int. J. Network Dyn. Intell., 1 (2022), 85–98. https://doi.org/10.53941/ijndi0101008 doi: 10.53941/ijndi0101008
![]() |
[8] |
X. Liu, S. Zhong, Stability analysis of delayed switched cascade nonlinear systems with uniform switching signals, Math. Modell. Control, 1 (2021), 90–101. https://doi.org/10.3934/mmc.2021007 doi: 10.3934/mmc.2021007
![]() |
[9] |
Y. Su, H. Cai, J. Huang, The cooperative output regulation by the distributed observer approach, Int. J. Network Dyn. Intell., 1 (2022), 20–35. https://doi.org/10.53941/ijndi0101003 doi: 10.53941/ijndi0101003
![]() |
[10] |
T. Takagi, M. Sugeno, Fuzzy identification of systems and its applications to modelling and control, IEEE Trans. Syst. Man Cybern., SMC-15 (1985), 116–132. https://doi.org/10.1109/TSMC.1985.6313399 doi: 10.1109/TSMC.1985.6313399
![]() |
[11] | G. Feng, Analysis and synthesis of fuzzy control systems: a model-based approach, Boca Raton: CRC Press, Inc., 2010. |
[12] |
J. Wang, J. Liang, C. T. Zhang, Dissipativity analysis and synthesis for positive Roesser systems under the switched mechanism and Takagi-Sugeno fuzzy rules, Inf. Sci., 546 (2021), 234–252. https://doi.org/10.1016/j.ins.2020.08.034 doi: 10.1016/j.ins.2020.08.034
![]() |
[13] |
J. Liang, J. Wang, T. Huang, l_1 filtering for continuous-discrete T-S fuzzy positive Roesser model, J. Franklin Inst., 355 (2018), 7281–7305. https://doi.org/10.1016/j.jfranklin.2018.07.017 doi: 10.1016/j.jfranklin.2018.07.017
![]() |
[14] |
Y. Luo, Z. Wang, J. Liang, G. Wei, F. E. Alsaadi, H_{\infty} control for 2-D fuzzy systems with interval time-varying delays and missing measurements, IEEE Trans. Cybern., 47 (2017), 365–377. https://doi.org/10.1109/TCYB.2016.2514846 doi: 10.1109/TCYB.2016.2514846
![]() |
[15] |
L. Li, W. Wang, X. Li, New approach to H_{\infty} filtering of two-dimensional T-S fuzzy systems, Int. J. Robust Nonlinear Control, 23 (2013), 1900–2012. https://doi.org/10.1002/rnc.2866 doi: 10.1002/rnc.2866
![]() |
[16] |
X. Ren, T. Hou, Pareto optimal filter design with hybrid H_2 / H_{\infty} optimization, Math. Modell. Control, 3 (2023), 80–87. https://doi.org/10.3934/mmc.2023008 doi: 10.3934/mmc.2023008
![]() |
[17] |
Y. Chen, W. Zhang, H. Gao, Finite frequency H_{\infty} control for building under earthquake excitation, Mechatronics, 20 (2010), 128–142. https://doi.org/10.1016/j.mechatronics.2009.11.001 doi: 10.1016/j.mechatronics.2009.11.001
![]() |
[18] |
T. Iwasaki, S. Hara, Generalized KYP lemma: unified frequency domain inequalities with design applications, IEEE Trans. Autom. Control, 50 (2005), 41–59. https://doi.org/10.1109/TAC.2004.840475(410) doi: 10.1109/TAC.2004.840475(410)
![]() |
[19] |
X. Li, H. Gao, C. Wang, Generalized Kalman-Yakubovich-Popov lemma for 2-D FM LSS model, IEEE Trans. Autom. Control, 57 (2012), 3090–3103. https://doi.org/10.1109/TAC.2012.2200370 doi: 10.1109/TAC.2012.2200370
![]() |
[20] |
L. Wang, W. Wang, G. Zhang, W. Chen, Generalised Kalman-Yakubovich-Popov lemma with its application in finite frequency positive realness control for two-dimensional continuous-discrete systems in the Roesser model form, IET Control Theory Appl., 9 (2015), 1676–1682. https://doi.org/10.1049/iet-cta.2014.0875 doi: 10.1049/iet-cta.2014.0875
![]() |
[21] |
H. Gao, X. Li, H_{\infty} filtering for discrete-time state-delayed systems with finite frequency specifications, IEEE Trans. Autom. Control, 56 (2011), 2935–2941. https://doi.org/10.1109/TAC.2011.2159909 doi: 10.1109/TAC.2011.2159909
![]() |
[22] |
G. Wang, H. Xu, L. Wang, J. Yao, Robust H_{\infty} filtering for uncertain two-dimensional continuous-discrete state-delay systems in finite frequency domains, IET Control Theory Appl., 12 (2018), 2316–2327. https://doi.org/10.1049/iet-cta.2018.5671 doi: 10.1049/iet-cta.2018.5671
![]() |
[23] |
T. Iwasaki, S. Hara, A. L. Fradkov, Time domain interpretations of frequency domain inequalities on (semi) finite ranges, Syst. Control Lett., 54 (2005), 681–691. https://doi.org/10.1016/j.sysconle.2004.11.007 doi: 10.1016/j.sysconle.2004.11.007
![]() |
[24] |
D. W. Ding, G. H. Yang, Fuzzy filter design for nonlinear systems in finite-frequency domain, IEEE Trans. Fuzzy Syst., 18 (2010), 935–945. https://doi.org/10.1109/TFUZZ.2010.2058807 doi: 10.1109/TFUZZ.2010.2058807
![]() |
[25] |
Z. Duan, J. Shen, I. Ghous, J. Fu, H_{\infty} filtering for discrete-time 2D T-S fuzzy systems with finite frequency disturbances, IET Control Theory Appl., 13 (2019), 1983–1994. https://doi.org/10.1049/iet-cta.2018.5918 doi: 10.1049/iet-cta.2018.5918
![]() |
[26] |
M. Wang, G. Feng, J. Qiu, Finite-frequency fuzzy output feedback controller design for Roesser-type two-dimensional nonlinear systems, IEEE Trans. Fuzzy Syst., 29 (2021), 861–873. https://doi.org/10.1109/TFUZZ.2020.2966155 doi: 10.1109/TFUZZ.2020.2966155
![]() |
[27] | D. Franke, 2D-analysis of Hybrid systems, In: P. M. Frank, Advances in control, Springer, 1999, 293–299. |
[28] |
Z. Duan, J. Liang, Z. Xiang, H_\infty control for continuous-discrete systems in T-S fuzzy model with finite frequency specifications two-dimensional nonlinear systems, Discrete Contin. Dyn. Syst., 15 (2022), 3155–3172. https://doi.org/10.3934/dcdss.2022064 doi: 10.3934/dcdss.2022064
![]() |
Frequency domain | \gamma ^{**} | Feasibility |
[5.2, 8.7]\times[0, 1.57] | 1.4013 | \checkmark |
[0.5, 13.06]\times[0, 1.57] | 1.9112 | \checkmark |
[0.5, 13.06]\times[0.5, 1.2] | 1.3135 | \checkmark |
[0,130.6]\times[-2, 3] | Null | \times |
[0, 1.3 \times 10^8]\times[-\pi, \pi] | Null | \times |