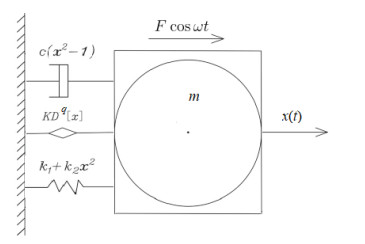
In this study, a fractional-order model for COVID-19 disease transmission is proposed and studied. First, the disease-free equilibrium and the basic reproduction number, R0 of the model has been communicated. The local and global stability of the disease-free equilibrium have been proved using well-constructed Lyapunov functions. Moreover, a normalized sensitivity analysis for the model parameters has been performed to identify their influence on R0. Real data on COVID-19 disease from Wuhan in China has been used to validate the proposed model. Finally, a simulation of the model has been performed to determine the effects of memory and control strategies. Overall, one can note that vaccination and quarantine have the potential to minimize the spread of COVID-19 in the population.
Citation: Mlyashimbi Helikumi, Paride O. Lolika. Dynamics and analysis of COVID-19 disease transmission: The effect of vaccination and quarantine[J]. Mathematical Modelling and Control, 2023, 3(3): 192-209. doi: 10.3934/mmc.2023017
[1] | Zhoujin Cui . Primary resonance and feedback control of the fractional Duffing-van der Pol oscillator with quintic nonlinear-restoring force. AIMS Mathematics, 2023, 8(10): 24929-24946. doi: 10.3934/math.20231271 |
[2] | Nasser. A. Saeed, Amal Ashour, Lei Hou, Jan Awrejcewicz, Faisal Z. Duraihem . Time-delayed control of a nonlinear self-excited structure driven by simultaneous primary and 1:1 internal resonance: analytical and numerical investigation. AIMS Mathematics, 2024, 9(10): 27627-27663. doi: 10.3934/math.20241342 |
[3] | Xiping Liu, Mei Jia, Zhanbing Bai . Nonlocal problems of fractional systems involving left and right fractional derivatives at resonance. AIMS Mathematics, 2020, 5(4): 3331-3345. doi: 10.3934/math.2020214 |
[4] | Zhiwei Lv, Jiafa Xu, Donal O'Regan . Solvability for a fractional p-Laplacian equation in a bounded domain. AIMS Mathematics, 2022, 7(7): 13258-13270. doi: 10.3934/math.2022731 |
[5] | Lei Hu, Cheng Wang, Shuqin Zhang . New results for nonlinear fractional jerk equations with resonant boundary value conditions. AIMS Mathematics, 2020, 5(6): 5801-5812. doi: 10.3934/math.2020372 |
[6] | Hany Bauomy . Control and optimization mechanism of an electromagnetic transducer model with nonlinear magnetic coupling. AIMS Mathematics, 2025, 10(2): 2891-2929. doi: 10.3934/math.2025135 |
[7] | Guijun Xing, Huatao Chen, Zahra S. Aghayan, Jingfei Jiang, Juan L. G. Guirao . Tracking control for a class of fractional order uncertain systems with time-delay based on composite nonlinear feedback control. AIMS Mathematics, 2024, 9(5): 13058-13076. doi: 10.3934/math.2024637 |
[8] | Hany Bauomy, A. T. EL-Sayed, A. M. Salem, F. T. El-Bahrawy . The improved giant magnetostrictive actuator oscillations via positive position feedback damper. AIMS Mathematics, 2023, 8(7): 16864-16886. doi: 10.3934/math.2023862 |
[9] | Abdukhafiz Bobodzhanov, Burkhan Kalimbetov, Valeriy Safonov . Asymptotic solutions of singularly perturbed integro-differential systems with rapidly oscillating coefficients in the case of a simple spectrum. AIMS Mathematics, 2021, 6(8): 8835-8853. doi: 10.3934/math.2021512 |
[10] | Shuqi Tang, Chunhua Li . Decay estimates for Schrödinger systems with time-dependent potentials in 2D. AIMS Mathematics, 2023, 8(8): 19656-19676. doi: 10.3934/math.20231002 |
In this study, a fractional-order model for COVID-19 disease transmission is proposed and studied. First, the disease-free equilibrium and the basic reproduction number, R0 of the model has been communicated. The local and global stability of the disease-free equilibrium have been proved using well-constructed Lyapunov functions. Moreover, a normalized sensitivity analysis for the model parameters has been performed to identify their influence on R0. Real data on COVID-19 disease from Wuhan in China has been used to validate the proposed model. Finally, a simulation of the model has been performed to determine the effects of memory and control strategies. Overall, one can note that vaccination and quarantine have the potential to minimize the spread of COVID-19 in the population.
Rolling mill is a key equipment in the steel industry and an important equipment in the modern heavy machinery field. It is a complex working system, and its safe and stable operation is crucial to ensure efficient production of rolled products. With the development of society, higher requirements have been put forward for the surface quality of strip mills, so the requirements of high precision and high dynamic performance have been promoted for rolling mills [1]. However, the high rolling speed and strength of modern rolling mills often result in unstable rolls during the rolling process. For example, due to the presence of many nonlinear factors within the system, the rolling mill roll system exhibits complex nonlinear vibration characteristics. When rolling high strength and thin strip steel, the mill frequently appears "ghost" vibration, which mostly includes vertical vibration, horizontal vibration, axial oscillation, transverse and longitudinal vibration of the strip steel, torsional vibration, and axial vibration of the main drive system [2]. These vibrations seriously affect the working performance and reliability of the rolling mill system and restrict the stability of the rolling production process [3,4]. Therefore, it is necessary to strengthen the analysis and study of the vibration causes of rolling mill and deal with the problems in time to ensure the stable operation of the equipment.
The study of nonlinear dynamics of vibration in rolling systems has attracted widespread attention and has been ongoing for decades. Many experts and scholars have conducted many beneficial exploratory studies from various angles. These researchers mainly focus on why rolling mills vibrate, how they vibrate, and how to suppress vibration (see [5,6,7,8,9,10,11,12,13] and the reference therein). The authors in [5] assumed that the workpiece is an elastic part with linear stiffness and established a linear vertical vibration model for the rolling mill frame based on linear vibration theory. In order to study the vibration characteristics of the rolling mill, the authors in [6] studied the effect of tension on nonlinear vibration of rolling mills. By changing the external excitation frequency to analyze the stability of the rolling mill vibration system, it was concluded that rolling speed and strip thickness have a significant impact on system stability. A horizontal friction vibration model of the rolling mill rolls was established in [7], and simulation analysis was conducted under the conditions of eliminating the bearing clearance of the rolling mill frame and adding a floating support for the coupling. By analyzing the effects of changes in workpiece thickness and motor speed on the connection angle and roll gap friction, a nonlinear torsional vibration model of the rolling mill was established in [9], indicating that reducing damping coefficient and nonlinear stiffness helps to reduce vibration intensity. In terms of research on vibration control, the authors in [11] designed a global sliding mode controller for the rolling mill drive system to suppress the uncertainty of rolling parameters and achieved good tracking performance. In [12], a displacement time-delay feedback link was introduced to control the vibration of the roller system, and different time-delay parameters were selected to test the control effect. The research results indicated that appropriate time-delay feedback parameters can suppress the unstable vibration of the roller system. The authors in [13] studied the vibration characteristics of the corrugated roller system and designed a time-delay feedback controller to control the parameter excitation vibration of the system.
In recent years, fractional calculus and its application in different fields have attracted widespread attention, providing a very useful mathematical tool for describing the memory and genetics of various materials and processes, such as fractional modeling of robotic manipulator [14], bibliographic analysis on artificial neural networks based on fractional calculus [15], fractional model of cerebral aneurysm [16], fractional model of ENSO phenomenon [17], fractional mechanics [18], fractional memristor circuit [19], fractional infectious disease model [20], and so on. Even if all individuals in the system have integer order dynamic characteristics, the overall dynamic characteristics of the system may still be fractional order. It can better describe the viscoelasticity of materials, such as suspension [21], air spring [22], magneto rheological damper [23] and hydraulic bushing [24]. In the research of rolling systems, fractional calculus has also begun to be involved [25,26,27,28]. Among them, the authors in [26,27] introduced a fractional derivative term when establishing a horizontal nonlinear vibration model for rolling mills. In [28], the resonance characteristics of the fractional roller system under high-frequency and low-frequency excitation signals were studied.
With the continuous development of active control technology, there is an increasing amount of research on actively utilizing time-delay feedback to achieve various control objectives. Time-delayed feedback control, as an effective control method, has been widely applied in the field of vibration control [29,30,31]. Adding fractional order factors to the simulation of rolling mill systems is more reasonable, but there are many problems that need to be solved urgently in the current research on fractional order nonlinear systems. For example, complex dynamic characteristics such as the influence of system parameters on periodic solutions under time-delay feedback, as well as many problems such as bifurcation control, require further research. Therefore, based on the superiority of fractional calculus and time-delayed feedback control, it is necessary to study the dynamic characteristics of fractional order nonlinear rolling systems and the bifurcation control problem under time-delayed feedback, which has important theoretical significance and application value. Motivated by [13,26,27,28], we focus on the vibration characteristics of the system and time-delay feedback controllability of the horizontal nonlinear roller system, and corrects the expression errors in existing literature. The innovation lies in systematically studying the resonance of fractional roller systems and conducting corresponding vibration control, further validating the effectiveness of the theoretical research through numerical simulation, providing new ideas for the research of roller system vibration theory.
The paper is organized as follows: In the second section, the fractional derivative term is considered in the horizontal nonlinear roller system, and the nonlinear vibration model is established. In the third section, the amplitude-frequency response equations of the primary resonance and time-delay feedback control are obtained using the multiple scale method, and numerical analysis is conducted. In the fourth section, we mainly present the amplitude-frequency response equations of the secondary resonance and time-delay feedback control and analyze the numerical simulation results to verify the effectiveness of theoretical research. The comparison of approximate analytical solution and numerical solution is fulfilled in the fifth section. In the last section, we conclude this paper.
Referring to the model of the horizontal roller system in [26,27,28], in order to study the nonlinear vibration characteristics of the strip rolling mill in the horizontal direction, the Duffing and the Van der Pol oscillators were introduced, and the nonlinear damping and stiffness within interface of the rolling mill were considered to establish a fractional horizontal nonlinear parametric vibration model for the rolling mill work roll, as shown in Figure 1.
The vibration model can be given by a second-order non-autonomous differential equation as follows
m¨x+c(x2−1)˙x+KDqtx+(k1+k2x2)x=Fcosωt, | (2.1) |
where x is the horizontal displacement of the roller system and is a function of time t, m is equivalent mass of the roll, c(x2−1) represents nonlinear damping coefficient term between roller system and rolling piece, c is the nonlinear damping coefficient. k1+k2x2 represents nonlinear stiffness coefficient term between roller system and frame, and k1 is the linear stiffness coefficient, k2 is the nonlinear stiffness coefficient. Dqtx is the q-order derivative of x with respect to time represents fractional derivative term, K is a positive coefficient. Due to the presence of friction, clearance, and additional bending moments, the roll system is subjected to a horizontal resultant force, which is defined as the horizontal excitation force Fcosωt, with the amplitude and frequency parameters F and ω, respectively. There are many definitions available for the fractional-order derivative, in this study, Dqtx with 0≤q≤1 is the Caputo's fractional derivative of x(t) described by
Dqtx(t)=1Γ(1−q)∫t0˙x(s)(t−s)−qds, |
in which Γ(z) is Gamma function satisfying Γ(z+1)=zΓ(z).
The roll system of the rolling mill is a highly nonlinear hysteresis system. In the rolling process of composite plates, the elastic-plastic deformation process of the rolled piece is a nonlinear deformation process with time delay characteristics. The time-delay feedback control method is one of the effective methods for studying bifurcation control of nonlinear systems. We adopt time-delay feedback control to suppress the nonlinear vibration. The block diagram is shown in Figure 2.
The horizontal vibration of the roller system is a weak vibration, and the multiple scale method can be used to conduct an approximate resonant solution of Eq (2.1), for which a small time scale parameter ε is required. This method begins by introducing new time variables Tn=εnt,(n=0,1), then an approximate solution of Eq (2.1) with small amplitudes can be represented by
x=x0(T0,T1)+εx1(T0,T1)+⋯. | (2.2) |
For such a small parameter ε, the following variable substitution is introduced for the system,
εμ=Km,ω0=√k1m,εα=cm,εβ=k2m,f=Fm, | (2.3) |
where ω0 is the undamped natural frequency of the system, α, β, μ are equivalent nonlinear damping coefficient, equivalent fractional derivative coefficient and equivalent cubic stiffness coefficient, respectively.
Then, Eq (2.1) becomes
¨x+ω20x+εμDqtx+εα(x2−1)˙x+εβx3=fcosωt. | (2.4) |
The derivatives with respect to t can be expressed in terms of the new scaled times Tn as a series of partial derivatives (see [16]),
ddt=∂∂T0dT0dt+∂∂T1dT1dt+⋯=D0+εD1+⋯, | (2.5a) |
d2dt2=D20+2εD0D1+ε2D21+⋯, | (2.5b) |
Dqt=Dq0+qεDq−10D1+⋯, | (2.5c) |
in which Dq0=∂q∂Tq0, Dn=∂∂Tn, D2n=∂2∂T2n,(n=0,1).
The nonlinear vibration characteristics of the horizontal roller system may lead to various resonance phenomena during the rolling process, such as internal resonance, primary resonance, and secondary resonance. First, we analyze the primary resonance when the excitation frequency is close to the natural frequency.
Regarding the primary resonance, soft excitation is applied implying that the amplitude of excitation is small, thus the external force is given by
f→εf, | (3.1) |
and the resonance relation is considered to be ω=ω0 or ω≈ω0, a detuning parameter σ describing the nearness of ω to ω0 is introduced by
ω=ω0+εσ, | (3.2) |
then ωt=ω0T0+σT1. Substituting (2.2), (2.5a)–(2.5c) into (2.4) leads to the following equation
(D20+2εD0D1+ε2D21)(x0+εx1)+ω20(x0+εx1)+εμ⋅(Dq0+qεDq−10D1)(x0+εx1)+εα[(x0+εx1)2−1]⋅(D0+εD1)(x0+εx1)+εβ(x0+εx1)3=εfcos(ω0T0+σT1). |
Equating the coefficients of the same power of ε, a set of linear differential equations are obtained:
O(ε0):D20x0+ω20x0=0, | (3.3) |
O(ε1):D20x1+ω20x1=−2D0D1x0−μDq0x0−βx30−α(x20−1)D0x0+fcos(ω0T0+σT1), | (3.4) |
from which x0 and x1 can be solved one-by-one respectively. In this way, the resonant solution x is dominated by x0, collected by εx1.
The general solution of Eq (3.3) is of the form,
x0=A(T1)eiω0T0+¯A(T1)e−iω0T0, | (3.5) |
where A(T1) and ¯A(T1) are unknown functions, ¯A(T1) denotes the complex conjugate of A(T1).
To solve Eq (3.4), the qth-order (0≤q≤1) derivative of eiωt is approximated written as following (see [32]),
Dqteiωt≈(iω)qeiωt. | (3.6) |
Substituting (3.5) and (3.6) into Eq (3.4) and using
cos(ω0T0+σT1)=ei(ω0T0+σT1)+e−i(ω0T0+σT1)2, |
the right-hand of Eq (3.4) becomes
[−2iω0D1A−μA(iω0)q−(3β+iω0α)A2ˉA+iω0αA+f2eiσT1]eiω0T0+NST+cc, | (3.7) |
where NST stands for the terms that do not produce secular terms, cc denotes the complex conjugate of the preceding terms.
In order that x1 is periodic, the secular terms with eiω0T0 must be zero, namely
2iω0D1A+μA(iω0)q+(3β+iω0α)A2ˉA−iω0αA−f2eiσT1=0. | (3.8) |
To solve Eq (3.8), we write A(T1) in the polar form as following
A(T1)=a(T1)2eiθ(T1), | (3.9) |
in which a(T1) and θ(T1) are real functions of T1.
With the help of the Euler formula
iq=(eiπ/2)q=eiqπ/2=cosqπ2+isinqπ2, | (3.10) |
let φdef=σT1−θ, by separating the real and imaginary parts of Eq (3.8), the differential equations governing amplitude a(T1) and φ(T1) of A(T1) are expressed as follows respectively
D1a=−μa2ωq−10sinqπ2+αa2−αa38+f2ω0sinφ, | (3.11a) |
aD1φ=σa−μa2ωq−10cosqπ2−3β8ω0a3+f2ω0cosφ. | (3.11b) |
The steady state motions for the primary resonance response correspond to the fixed points of (3.11a) and (3.11b), that is, D1a=0 and D1φ=0, namely
−μa2ωq−10sinqπ2+αa2−αa38=−f2ω0sinφ, | (3.12a) |
σa−μa2ωq−10cosqπ2−3β8ω0a3=−f2ω0cosφ. | (3.12b) |
By performing square operations and eliminating φ from Eqs (3.12a) and (3.12b), the following amplitude-frequency response equation is determined,
[(μ2ωq−10sinqπ2−α2+α8a2)2+(σ−μ2ωq−10cosqπ2−3β8ω0a2)2]a2=(f2ω0)2. | (3.13) |
The amplitude of the response is a function of external detuning parameter and the amplitude of excitation. It should be pointed out that in [26], the multiple scale method was used to obtain the amplitude-frequency response equation of Eq (2.1) for the primary resonance, but the coefficients in the expression were incorrect. In addition, when μ=0, Eq (2.4) is transformed into an integer-order model, and the corresponding amplitude-frequency response equation for the primary resonance has been studied in [13], but the expression has certain problems.
To determine the stability of steady state motion through the nature of singular points in Eqs (3.11a) and (3.11b), the following method can be used. Assume that (a,φ)=(a∗,φ∗) is a steady solution of Eqs (3.11a) and (3.11b), let Δa=a−a∗ and Δφ=φ−φ∗. According to Eqs (3.12a) and (3.12b), the linearized differential equations governing Δa and Δφ are
D1Δa=−[μ2ωq−10sinqπ2−α2+3α(a∗)28]Δa+f2ω0cosφ∗Δφ, | (3.14a) |
D1Δφ=[σa∗−μ2a∗ωq−10cosqπ2−9β8ω0a∗]Δa−f2ω0a∗sinφ∗Δφ. | (3.14b) |
Let P=μ2ωq−10sinqπ2−α2+3α(a∗)28,Q=μ2ωq−10sinqπ2−α2+α(a∗)28, M=σ−μ2ωq−10cosqπ2−3β8ω0(a∗)2,N=σ−μ2ωq−10cosqπ2−9β8ω0(a∗)2, then the characteristic equation can be rewritten as
|−P−λ−a∗M1a∗N−Q−λ|=0. | (3.15) |
By expanding the determinant, one has
λ2+(P+Q)λ+(PQ+MN)=0. | (3.16) |
Consider when P+Q>0, then the steady solution (a,φ)=(a∗,φ∗) is asymptotically stable if and only if Λ>0, where
Λdef=PQ+MN. | (3.17) |
The influence of different parameters on the amplitude of resonance solution is investigated numerically, as shown in the Figures 3 and 4. With fixed parameter values, all the figures exhibit typical characteristics of hardening spring.
First, the influence of fractional order q on the resonant solutions is shown in Figure 3, where the parameters are selected to be, α=0.04, β=0.1, ω0=1, μ=0.02 and f=0.1. It can be seen that the smaller the order q is, the larger the maximum amplitude is. In addition, compared with the integer order case when q=1, the bending degree, resonance peak, and resonance region of the amplitude-frequency curve of the fractional system change accordingly with the decrease of the fractional order q. The reason for this is that the fractional differential term has both stiffness and damping characteristics, which have a significant impact on the amplitude frequency response curve of the system. When the fractional-order q approaches 0, the fractional differential term is almost equivalent to the effect of linear stiffness; When q tends towards 1, the fractional differential term is almost equivalent to the effect of linear damping, and the larger the damping, the smaller the peak value.
Figure 4 presents parameter effect on resonant amplitudes with respect to μ, f, α and β. Here, the fractional-order q=0.9 and the natural frequency ω0=1. In Figure 4(a), with the increase of μ, the nonlinear jump of the system weakens and the resonance amplitude of the system decreases. In other words, as μ increases, the unstable portions decrease. In Figure 4(b), with the increase of pulse pressure f, the nonlinear jump of the system is more obvious, and the resonance range and resonance amplitude of the system increase. In Figure 4(c), when the value of α increases, the amplitude of the system decreases. Since α is the nonlinear damping coefficient, increasing α means that the damping term increases, and the amplitude of resonance correspondingly decreases. In Figure 4(d), when the nonlinear stiffness coefficient β increases, the curve shifts to the right and and the degree of curvature increases. It can also be observed that the jumping phenomenon occur in the system, leading to system oscillations. Another phenomenon is that the amplitude does not change with the stiffness coefficient and remains consistent. According to the above analysis results, a controller should be designed to reduce the influence of primary resonance.
In order to eliminate the jumping and hysteresis phenomena of the primary resonance in the horizontal roller system, the time-delay displacement feedback strategy is adopted here, and the fractional equation with time-delay feedback control can be established as follows:
¨x+ω20x+εμDqtx+εα(x2−1)˙x+εβx3=εfcosωt+εg1x(t−τ1)+εg2x3(t−τ2), | (3.18) |
where g1 is the linear control gain, g2 is the nonlinear control gain, τ1 and τ2 are time-delay parameters.
Substituting (2.2), (2.5a)–(2.5c) into (3.18) leads to the following equation
(D20+2εD0D1+ε2D21)(x0+εx1)+ω20(x0+εx1)+εμ⋅(Dq0+qεDq−10D1)(x0+εx1)+εα[(x0+εx1)2−1]⋅(D0+εD1)(x0+εx1)+εβ(x0+εx1)3=εfcos(ω0T0+σT1)+εg1x0(t−τ1)+εg2x30(t−τ2). |
Thus, a set of linear differential equations can be obtained:
O(ε0):D20x0+ω20x0=0, | (3.19) |
O(ε1):D20x1+ω20x1=−2D0D1x0−μDq0x0−βx30−α(x20−1)D0x0+fcos(ω0T0+σT1)+g1x0(T0−τ1)+g2x30(T0−τ2). | (3.20) |
Assume that the general solution of Eq (3.19) is (3.5), substituting (3.5) into Eq (3.20), the right-hand of Eq (3.20) becomes
[−2iω0D1A−μA(iω0)q−(3β+iω0α)A2ˉA+iω0αA+f2eiσT1+g1Ae−iω0τ1+3g2A2ˉAe−iω0τ2]eiω0T0+NST+cc, | (3.21) |
where NST stands for the terms that do not produce secular terms, cc denotes the complex conjugate of the preceding terms.
Through setting the coefficient of eiω0T0 equal to zero to eliminate the secular terms, the following equation can be obtained as:
2iω0D1A+μA(iω0)q+(3β+iω0α)A2ˉA−iω0αA−f2eiσT1−g1Ae−iω0τ−3g2A2ˉAe−iω0τ2=0. | (3.22) |
Similar to the previous discussion, the following differential equations about amplitude and phase can be obtained,
D1a=−μa2ωq−10sinqπ2+αa2−αa38−g1a2ω0sin(ω0τ1)−3g2a38ω0sin(ω0τ2)+f2ω0sinφ, | (3.23a) |
aD1φ=σa−μa2ωq−10cosqπ2−3βa38ω0+g1a2ω0cos(ω0τ1)+3g2a38ω0cos(ω0τ2)+f2ω0cosφ. | (3.23b) |
The steady state motions for the primary resonance response correspond to the fixed points of (3.23a) and (3.23b), that is, D1a=0 and D1φ=0, namely
−μa2ωq−10sinqπ2+αa2−αa38−g1a2ω0sin(ω0τ1)−3g2a38ω0sin(ω0τ2)=−f2ω0sinφ, | (3.24a) |
σa−μa2ωq−10cosqπ2−3βa38ω0+g1a2ω0cos(ω0τ1)+3g2a38ω0cos(ω0τ2)=−f2ω0cosφ. | (3.24b) |
The amplitude-frequency response equation of primary resonance with time-delay control can be obtained as:
[(12μe+αe8a2)2+(σe−3βe8ω0a2)2]a2=(f2ω0)2, | (3.25) |
in which
μe=μωq−10sinqπ2+g1ω0sin(ω0τ1)−α, αe=α+3g2ω0sin(ω0τ2), |
σe=σ−μ2ωq−10cosqπ2+g12ω0cos(ω0τ1), βe=β−g2cos(ω0τ2). |
From Eq (3.25), it can be seen that the amplitude of the response is a function of external detuning parameter, feedback gain, time delay and the amplitude of excitation.
The impact of adding time-delay feedback control on the amplitude-frequency response curve of the primary resonance is presented through numerical simulation, as shown in Figure 5. In Figure 5, the primary resonance amplitude and resonance region can be controlled, the primary resonance bifurcations can be reduced by properly adjusting the delay parameters (τ1,τ2) and feedback gains (g1,g2). After adding time-delay feedback control, the resonance peak value decreases, the curvature of the curve also decreases and the jumping phenomenon has also weakened. It can also be seen from Figure 5(a) that the control effect of simultaneously adjusting delay parameters (τ1,τ2) and feedback gains (g1,g2) is better than that of separately adjusting linear feedback gain g1 or nonlinear feedback gain g2.
In Figure 5(b), as the linear gain g1 and nonlinear gain g2 gradually increase, the amplitude of the primary resonance of the system gradually decreases, and the jumping phenomenon of the curve is eliminated. Therefore, it can be seen that when using only feedback control gain as the control parameter, if both linear and nonlinear feedback control gains increase simultaneously, the primary resonance phenomenon of the system can be reasonably controlled. In Figure 5(c), as the delay parameters (τ1,τ2) gradually increase, the amplitude and the resonance domain of the system gradually decreases. However, the adjustment of delay parameters has little effect on the degree of curve curvature. On the contrary, by adjusting the feedback control gains g1 and g2, it is relatively easy to eliminate the jumping phenomenon of the primary resonance. This indicates that using feedback control gain as the control object has a better control effect than using delay parameters as the control object.
In this section, we will discuss secondary resonance, namely superharmonic and subharmonic resonance. Only superharmonic resonance is considered here, and the issue of subharmonic resonance will be studied in subsequent papers.
During the inspection process of third-order superharmonic resonance, when the excitation frequency is far away from the natural frequency, unless its amplitude is sufficiently large, the impact of excitation is minimal. Therefore, in superharmonic resonance, the excitation amplitude is of order ε0. Third-order superharmonic resonance with limited amplitude occurs in the Eq (2.4) when 3ω=ω0 or 3ω≈ω0, the resonance relation is represented as
3ω=ω0+εσ, | (4.1) |
in which σ is again the detuning parameter, then 3ωT0=ω0T0+σT1. The following derivations in this section are similar to those in the previous section, only the main steps will be retained for ease of reading.
Substituting (4.1), (2.5a)–(2.5c) into (2.4) leads to the following equation
(D20+2εD0D1+ε2D21)(x0+εx1)+ω20(x0+εx1)+εμ⋅(Dq0+qεDq−10D1)(x0+εx1)+εα[(x0+εx1)2−1]⋅(D0+εD1)(x0+εx1)+εβ(x0+εx1)3=fcos(ωT0). |
Equating the coefficients of the same power of ε, a set of linear differential equations are obtained:
O(ε0):D20x0+ω20x0=fcos(ωT0), | (4.2) |
O(ε1):D20x1+ω20x1=−2D0D1x0−μDq0x0−βx30−α(x20−1)D0x0. | (4.3) |
The general solution of Eq (4.2) is of the following form,
x0=A(T1)eiω0T0+¯A(T1)e−iω0T0+B(eiωT0+e−iωT0), | (4.4) |
where A(T1) and ¯A(T1) are complex functions in terms of slow time scale, ¯A(T1) denotes the complex conjugate of A(T1) and B=f2(ω20−ω2).
Substituting (4.4) into Eq (4.3), the right-hand of Eq (4.3) becomes
[−2iω0D1A−μA(iω0)q−(3A2ˉA+6AB2+B3eiσT1)β−(A2ˉA+2AB2−A)iω0α−αiωB3eiσT1]eiω0T0+NST+cc, | (4.5) |
where NST stands for the terms that do not produce secular terms, cc denotes the complex conjugate of the preceding terms.
Thus, the solvability condition takes the form
2iω0D1A+μA(iω0)q+(3A2ˉA+6AB2)β+(A2ˉA+2AB2−A)iω0α=−(β+αiω)B3eiσT1. | (4.6) |
Separating the real and imaginary parts, and letting φdef=σT1−θ to transform this into an autonomous system. Seeking the steady state, we let D1a=0 and D1φ=0. Eliminating φ leads to the nonlinear the amplitude-frequency equation
[(μ2ωq−10sinqπ2−4−a2−8B28α)2+(σ−μ2ωq−10cosqπ2−3(a2+8B2)8ω0β)2]a2=(β2+α2ω2)B6ω20. | (4.7) |
From Eq (4.7), it can be concluded that there is an interaction between the nonlinear term and the external force term to the third-order superharmonic resonance of the first-order perturbation analysis. According to Eq (4.7), different superharmonic resonance amplitude-frequency characteristic curves can be obtained by different μ, α, β, and f.
First, we present an image of the amplitude-frequency response curve of third-order superharmonic resonance, as shown in Figure 6. Here, the parameters are selected to be, q=0.9, α=0.08, β=0.2, ω0=1, μ=0.08, and f=0.24. It can be clearly seen from Figure 6 that the fractional roller system generates superharmonic resonance under the above parameter conditions.
By changing the nonlinear damping coefficient α, nonlinear stiffness coefficient β, rolling force amplitude f, and fractional damping coefficient μ of the roller system, the superharmonic resonance curves with different amplitude-frequency characteristics can be obtained, as shown in Figure 7. Here, the fractional order q=0.9 and the natural frequency ω0=1. In Figure 7(a), when the nonlinear damping coefficient α increases, the amplitude decreases and the resonance domain decreases. In Figure 7(b), with the nonlinear stiffness coefficient β increases, the curve shifts to the right and the bending degree increases. In Figure 7(c), with the increase of rolling force, the amplitude and resonance region of the system increase obviously. In Figure 7(d), when μ increases, the amplitude and the resonance domain decrease, and the nonlinear jump of the system weakens.
In order to study the control problem of superharmonic resonance, the fractional equation with time-delay feedback control can be established as follows:
¨x+ω20x+εμDqtx+εα(x2−1)˙x+εβx3=fcosωt+εg1x(t−τ1)+εg2x3(t−τ2). | (4.8) |
Substituting (4.1), (2.5a)–(2.5c) into (4.8), we can obtain
(D20+2εD0D1+ε2D21)(x0+εx1)+ω20(x0+εx1)+εμ⋅(Dq0+qεDq−10D1)(x0+εx1)+εα[(x0+εx1)2−1]⋅(D0+εD1)(x0+εx1)+εβ(x0+εx1)3=fcos(ωT0)+g1x0(T0−τ1)+g2x30(T0−τ2). |
Equating the coefficients of the same power of ε, a set of linear differential equations are obtained:
O(ε0):D20x0+ω20x0=fcos(ωT0), | (4.9) |
O(ε1):D20x1+ω20x1=−2D0D1x0−μDq0x0−βx30−α(x20−1)D0x0+g1x0(t−τ1)+g2x30(t−τ2). | (4.10) |
Assume that the solution of the zeroth approximation equation (4.9) is (4.4), substituting (4.4) into Eq (4.10), the right-hand of Eq (4.10) becomes
[−2iω0D1A−μA(iω0)q−(3A2ˉA+6AB2+B3eiσT1)β−(A2ˉA+2AB2−A)iω0α−αiωB3eiσT1+g1Ae−iω0τ1+3g2A2ˉAe−iω0τ2]eiω0T0+NST+cc, | (4.11) |
where NST stands for the terms that do not produce secular terms, cc denotes the complex conjugate of the preceding terms.
The solvability condition takes the form
2iω0D1A+μA(iω0)q+(3A2ˉA+6AB2+B3eiσT1)β+(A2ˉA+2AB2−A)iω0α+αiωB3eiσT1−g1Ae−iω0τ1−3g2A2ˉAe−iω0τ2=0. | (4.12) |
Based on the condition of steady solution, the nonlinear amplitude-frequency equation of superharmonic vibration with time-delay control can be obtained as follows
[(12μs+αs8a2)2+(σs−3βs8ω0a2)2]a2=(β2+α2ω2)B6ω20, | (4.13) |
in which
μs=μωq−10sinqπ2+g1ω0sin(ω0τ1)−α+2B2α, αs=α+3g2ω0sin(ω0τ2),σs=σ−μ2ωq−10cosqπ2+g12ω0cos(ω0τ1)−3B2βω0, βs=β−g2ω0cos(ω0τ2). |
From Eq (4.13), it can be concluded that there is an interaction between the feedback gain, time delay, nonlinear term and the external force term to the third-order superharmonic resonance of the first-order perturbation analysis.
The impact of adding time-delay feedback control on the amplitude-frequency response curve of the third-order superharmonic resonance is presented through numerical simulation, as shown in Figure 8. Figure 8 shows that the amplitude and resonance region can be controlled and the superharmonic resonance bifurcations can be reduced by properly adjusting the delay parameters (τ1,τ2) and feedback gains (g1,g2). After adding time-delay feedback control, the resonance peak value decreases, the curvature of the curve decreases, and the jumping phenomenon has weakened.
In Figure 8(a), as the feedback gains (g1,g2) gradually increase, the amplitude of the system gradually decreases, the resonance domain gradually decreases, and the curve bifurcation is eliminated. The resonance domain also shows significant movement. It can be concluded that using feedback control gain as the control parameter and increasing both linear and nonlinear feedback control gains can effectively control the phenomenon of superharmonic vibration in the system. In Figure 8(b), when the delay parameters (τ1,τ2) gradually increases, the amplitude of the system gradually decreases and the resonance domain gradually decreases. Through comparison, it can be found that whether using feedback control gains or time-delay parameters as the control object, the control effect is significant during the control of superharmonic vibration.
At the end of this section, a time history diagram is used to briefly illustrate the impact of feedback control on the model. Figure 9 shows the time history of the superharmonic resonance under feedback control. The various parameters of the system in Figure 9 are q=0.9, ω0=1, α=0.08, β=0.2, μ=0.08, and f=0.24. It can be observed that the amplitude of the resonance decreases obviously after the delay control is added, and the larger the delay is, the greater the amplitude reduction is.
According to Eq (3.13), the primary resonance amplitude-frequency response curve of the system can be drawn. For comparison, we adopt the power series method introduced in reference [33,34], and its calculation formula is
Dqtn[y(tn)]≈h−qn∑j=0Cqjy(tn−j), | (5.1) |
where tn=nh is the sample points, h is the sample step, and Cqj is the fractional binomial coefficient with the iterative relationship as
Cq0=1,Cqj=(1−1+qj)Cqj−1. | (5.2) |
According to Eqs (5.1) and (5.2), the numerical scheme for Eq (2.4) can be expressed as
x(tn)=y(tn−1)h−n∑j=1C1jx(tn−j), | (5.3a) |
y(tn)={fcos(ωtn)−λx(tn)−βx3(tn)+α[1−x2(tn)]x(tn−1)−μz(tn−1)}h−n∑j=1C1jy(tn−j), | (5.3b) |
z(tn)=y(tn)h1−q−n∑j=1C1−qjz(tn−j). | (5.3c) |
The numerical amplitude-frequency curve marked with circle in Figure 10, where the stepsize of time is h=0.005, and the total computation time is 100s with the first 25s neglected. It shows that the resonant amplitude calculated from Eq (3.13) is in good agreement with the numerical results, especially when the ω≈ω0.
In the present research, we study the nonlinear vibration characteristics and time-delay feedback controllability of a fractional horizontal roll systems for rolling mill, described by a damped fractional Duffing-van der Pol oscillator under external harmonic excitation. In response to the inaccurate conclusions in existing literature, we conducted rigorous derivation. The accurate amplitude-frequency response equations were obtained by the multiple scale method. The influence of parameters on system characteristics was studied using amplitude frequency response equation. Furthermore, the time-delay feedback controller is designed to control the parameter excitation vibration. The numerical simulation results verified the effectiveness of the time-delay controller in eliminating the jumping and hysteresis phenomena of the rolling system. It can also be concluded that fractional order and the damping coefficient are very important in fractional horizontal roll systems. For example, a larger fractional order and larger damping coefficient can reduce the effective amplitude of resonance and change the resonance frequency.
Through the study of the roll model, it is inspired that in the design process of strip rolling mills, the influence of rolling force amplitude on primary resonance and superharmonic resonance should be avoided and reduced. Further research is needed to combine active vibration control techniques such as PID control, adaptive control, fuzzy control, and other control methods to analyze the vibration control effect of the rolling mill.
Zhoujin Cui performed the conceptualization, methodology, writing-original draft and writing-review & editing; Xiaorong Zhang performed the investigation and validation; Tao Lu performed the validation and supervision. All authors have read and approved the final version of the manuscript for publication.
The authors declare they have not used Artificial Intelligence (AI) tools in the creation of this article.
The authors express gratitude to the reviewers and editors for their helpful comments and suggestions, as well as to the financial support from High Level Talent Research Launch Fund of Jiangsu Second Normal University (No. 928201/058).
All authors declare no conflicts of interest in this paper.
[1] |
M. Higazy, Novel fractional order SIDARTHE mathematical model of COVID-19 pandemic, Chaos, Solitons & Fractals, 139 (2020), 110007. http://doi.org/10.1016/j.chaos.2020.110007 doi: 10.1016/j.chaos.2020.110007
![]() |
[2] |
C. H. Jeong, K. J. Wan, R. S. Ki, K. Y. Kyung, S. J. Soo, M. J. Ho, et al., COVID-19 transmission and blood transfusion: A case report, J. INFECT. PUBLIC HEAL., 13 (2020), 1678–1679. http://doi.org/10.1016/j.jiph.2020.05.001 doi: 10.1016/j.jiph.2020.05.001
![]() |
[3] |
R. J. Larsen, R. M. Margaret, D. J. Martin, K. Peter, B. J. Hicks, Modeling the onset of symptoms of COVID-19, Front. Public Health, 8 (2020), 473. http://doi.org/10.3389/fpubh.2020.00473 doi: 10.3389/fpubh.2020.00473
![]() |
[4] |
H. Abdul, A. Shmmon, S. S. Ali, A. Mumtaz, M. Shruti, A review of COVID-19 (Coronavirus Disease-2019) diagnosis, treatments and prevention, Ejmo, 4 (2020), 116–125. http://doi.org/10.14744/ejmo.2020.90853 doi: 10.14744/ejmo.2020.90853
![]() |
[5] |
R. Yelena, B. Jennifer, M. Haley, H. Whitney, B. Karen, C. J. Rhonda, A model of disparities: risk factors associated with COVID-19 infection, International journal for equity in health, 19 (2020), 1–10. http://doi.org/10.1186/s12939-020-01242-z doi: 10.1186/s12939-020-01242-z
![]() |
[6] |
B. A. William, G. O. Ouslander, B. A. William, G. O. Joseph, COVID-19 Presents High Risk to Older Persons, J. AM. GERIATR. SOC., 68 (2020), 681. https://doi.org/10.1111/jgs.16426 doi: 10.1111/jgs.16426
![]() |
[7] |
C. Shao-Chung, C. Yuan-Chia, C. Y-L. Fan, C. Yu-Chan, C. Mingte, Y. Chin-Hua, et al., First case of Coronavirus Disease 2019 (COVID-19) pneumonia in Taiwan, J. FORMOS. MED. ASSOC., 119 (2020), 747–751. http://doi.org/10.1016/j.jfma.2020.02.007 doi: 10.1016/j.jfma.2020.02.007
![]() |
[8] |
A. Khadijah, B. Y. Abdul, Y. Maryam, T. Javaid, S. I. Abira. Progress of COVID-19 Epidemic in Pakistan, Asia Pacific Journal of Public Health, 32 (2020), 154–156 http://doi.org/10.1177/1010539520927259 doi: 10.1177/1010539520927259
![]() |
[9] |
J. M. Abdul-Rahman, K. H. Alfred, Mathematical modelling on COVID-19 transmission impacts with preventive measures: a case study of Tanzania, J. Biol. Dyn., 14 (2020), 748–766. http://doi.org/10.1080/17513758.2020.1823494 doi: 10.1080/17513758.2020.1823494
![]() |
[10] |
A. Imran, ML. A. Omar, COVID-19: Disease, management, treatment, and social impact, Sci. Total Environ., 728 (2020), 138861 http://doi.org/10.1016/j.scitotenv.2020.138861 doi: 10.1016/j.scitotenv.2020.138861
![]() |
[11] |
M. I. Baltazar, N. J. Samwel, M. Melina, S. L. Philip, P. J. Jackson, A. Caroline, et al., Community engagement in COVID-19 prevention: experiences from Kilimanjaro region, Northern Tanzania, The Pan African Medical Journal, 35 (2020), 146. http://doi.org/10.11604/pamj.supp.2020.35.2.24473 doi: 10.11604/pamj.supp.2020.35.2.24473
![]() |
[12] |
L. Marc, E. D. Natalie, Understanding COVID-19 vaccine efficacy, Science, 370 (2020), 763–765. http://doi.org/10.1126/science.abe5938 doi: 10.1126/science.abe5938
![]() |
[13] | S. Adekunle, O. Chuku, H. Zaheeda, P. Risha, D. Priyank, P. Stephanie, et al., Global pandemicity of COVID-19: situation report, Infectious Diseases: Research and Treatment, 14 (2020). |
[14] |
R. R. Kumar, K. Subhas, T. P. Kumar, V. Ezio, M. A. Kumar, Impact of social media advertisements on the transmission dynamics of COVID-19 pandemic in India, J. Appl. Math. Comput., 68 (2020), 19–44. http://doi.org/10.1007/s12190-021-01507-y doi: 10.1007/s12190-021-01507-y
![]() |
[15] |
G. Said, B. Yassir, A. Abdelghafour, B. Mostafa, K. Fahd, M. Driss, An adaptive social distancing SIR model for COVID-19 disease spreading and forecasting, Epidemiologic Methods, 10 (2021), 20200044. http://doi.org/10.1515/em-2020-0044 doi: 10.1515/em-2020-0044
![]() |
[16] |
K. W. Ogilvy, G. M. Anderson, Contributions to the mathematical theory of epidemics. Ⅱ.—The problem of endemicity, The Royal Society London, 138 (1932), 55–83. http://doi.org/10.1007/BF02464424 doi: 10.1007/BF02464424
![]() |
[17] |
G. Major, G. Y. Udny, An inquiry into the nature of frequency distributions representative of multiple happenings with particular reference to the occurrence of multiple attacks of disease or of repeated accidents, Journal of the Royal statistical society, 83 (1920), 255–279. http://doi.org/10.2307/2341080 doi: 10.2307/2341080
![]() |
[18] |
R. Ronald, An application of the theory of probabilities to the study of a priori pathometry.—Part I, The Royal Society London, 92 (1916), 204–230. http://doi.org/10.1098/rspa.1917.0014 doi: 10.1098/rspa.1917.0014
![]() |
[19] |
B. Daniel, Essai d'une nouvelle analyse de la mortalité causée par la petite vérole, et des avantages de l'inoculation pour la prévenir, Des Math. And Phis., Mem., (1960), 1–45. doi: 10.1177/003591571801101305
![]() |
[20] |
B. John, Certain Aspects of the Theory of Epidemiology in Special Relation to Plague, Proceedings of the Royal Society of medicine, 11 (1918), 85–132. http://doi.org/10.1177/003591571801101305 doi: 10.1177/003591571801101305
![]() |
[21] |
E. S. Herbert, The interpretation of periodicity in disease prevalence, JSTOR, 92 (1929), 34–73. http://doi.org/10.2307/2341437 doi: 10.2307/2341437
![]() |
[22] |
G. Major, The statistical study of infectious diseases, JSTOR, 109 (1946), 85–110. http://doi.org/10.2307/2981176 doi: 10.2307/2981176
![]() |
[23] | TJ. B. Norman, The mathematical theory of infectious diseases and its applications, Charles Griffin & Company Ltd, 5a Crendon Street, High Wycombe, Bucks HP13 6LE., 1975. http://doi.org/10.2307/3009004 |
[24] | M. A. Roy, The population dynamics of infectious diseases: theory and applications, Springer, 2013. http://doi.org/10.2307/4361 |
[25] |
H. R. Thieme, Convergence results and a Poincare-Bendison trichotomy for asymptotical autonomous differential equations, J. Math. Biol., 30 (1992), 755–763. http://doi.org/10.1007/bf00173267 doi: 10.1007/bf00173267
![]() |
[26] | S. Lenhart, JT. Workman, Optimal Control Applied to Biological Models, Chapman and Hall/CRC, London, 2007. http://doi.org/10.1201/9781420011418 |
[27] |
W. Wang, X-Q. Zha, Threshold dynamics for compartment epidemic models in periodic environments, J. Dyn. Differ. Equ., 20 (2008), 699–717. http://doi.org/10.1007/s10884-008-9111-8 doi: 10.1007/s10884-008-9111-8
![]() |
[28] |
SF. Dowel, Seasonal Variation in Host Susceptibility and Cycles of Certain Infectious Diseases, EMERG. INFECT. DIS., 7 (2001), 369–374. http://doi.org/10.3201/eid0703.010301 doi: 10.3201/eid0703.010301
![]() |
[29] |
Z. Shuai, J. A. P. Heesterbeek, P. van den Driessche, Extending the type reproduction number to infectious disease control targeting contacts between types, J. Math. Biol., 67 (2013), 1067–1082. http://doi.org/10.1007/s00285-012-0579-9 doi: 10.1007/s00285-012-0579-9
![]() |
[30] | LS. Pontryagin, VT. Boltyanskii, RV. Gamkrelidze, EF. Mishcheuko, The mathematical theory of optimal processes, Wiley, New Jersey, 1962. http://doi.org/10.1057/jors.1965.92 |
[31] |
P. Van den Driessche, J. Watmough, Reproduction number and subthreshold endemic equilibria for compartment models of disease transmission, Math. Biosci., 180, (2002), 29–48. https://doi.org/10.1016/S0025-5564(02)00108-6 doi: 10.1016/S0025-5564(02)00108-6
![]() |
[32] |
M. Saeedian, M. Khalighi, N. A.-Tafreshi, GR. Jafari, A. Marcel, Memory effects on epidemic evolution: The susceptible-infected-recovered epidemic model, Phys. Rev. E, 95 (2017), 022409. http://doi.org/10.1103/physreve.95.022409 doi: 10.1103/physreve.95.022409
![]() |
[33] |
FA. Rihan, QM. A-Mdallal, HJ. AlSakaji, A. H. Adel, A fractional-order epidemic model with time-delay and nonlinear incidence rate, Chaos, Solitons & Fractals, 126 (2019), 97–105. http://doi.org/10.1016/j.chaos.2019.05.039 doi: 10.1016/j.chaos.2019.05.039
![]() |
[34] |
H. Nur'Izzati, K. Adem, Analysis of the fractional order dengue transmission model: a case study in Malaysia, Adv. Differ. Equations, 1 (2019), 1–13. http://doi.org/10.1186/s13662-019-1981-z doi: 10.1186/s13662-019-1981-z
![]() |
[35] | M. Abderrahim, B. Adnane, H. Khalid, Y. Noura, A fractional order SIR epidemic model with nonlinear incidence rate, Springer, 2018. http://doi.org/10.1186/s13662-018-1613-z |
[36] |
M. Awais, F. S. Alshammari, S. Ullah, M. A. Khan, S. Islam, Modeling and simulation of the novel coronavirus in Caputo derivative, Results Phys., 19 (2020), 103588. http://doi.org/10.1016/j.rinp.2020.103588 doi: 10.1016/j.rinp.2020.103588
![]() |
[37] |
B. Samia, S. Tareq, FM. T. Delfim, Z. Anwar, Control of COVID-19 dynamics through a fractional-order model, Alex. Eng. J., 60 (2020), 3587–3592. http://doi.org/10.1016/j.aej.2021.02.022 doi: 10.1016/j.aej.2021.02.022
![]() |
[38] |
O.-M. Isaac, A. Lanre, O.Bismark, S. I. Olaniyi, A fractional order approach to modeling and simulations of the novel COVID-19, Adv. Differ. Equations, 1 (2020), 1–21. http://doi.org/10.1186/s13662-020-03141-7 doi: 10.1186/s13662-020-03141-7
![]() |
[39] |
A. Idris, B. I. Abdullahi, Y. Abdullahi, K. Poom, K. Wiyada, Analysis of Caputo fractional-order model for COVID-19 with lockdown, Adv. Differ. Equations, 2020 (2020), 394. http://doi.org/10.1186/s13662-020-02853-0 doi: 10.1186/s13662-020-02853-0
![]() |
[40] |
B. B. Abdullahi, B. Bulent, Optimal control of a fractional order model for the COVID–19 pandemic, Chaos, Solitons & Fractals, 144 (2021), 110678. http://doi.org/10.1016/j.chaos.2021.110678 doi: 10.1016/j.chaos.2021.110678
![]() |
[41] |
A. Omame, M. Abbas, CP. Onyenegecha, A fractional-order model for COVID-19 and tuberculosis co-infection using Atangana–Baleanu derivative, Chaos, Solitons & Fractals, 153 (2021), 111486 http://doi.org/10.1016/j.chaos.2021.111486 doi: 10.1016/j.chaos.2021.111486
![]() |
[42] |
A. Muhammad, F. Muhammad, A. Ali, S. Meng, Modeling and simulation of fractional order COVID-19 model with quarantined-isolated people, Math. Methods Appl. Sci., 44 (2021), 6389–6405. http://doi.org/10.1002/mma.7191 doi: 10.1002/mma.7191
![]() |
[43] |
A. Ul Rehman, R. Singh, Ram, P. Agarwal, Modeling, analysis and prediction of new variants of covid-19 and dengue co-infection on complex network, Chaos, Solitons & Fractals, 150 (2021), 111008. http://doi.org/10.1016/j.chaos.2021.111008 doi: 10.1016/j.chaos.2021.111008
![]() |
[44] |
R. Singh, P. Tiwari, S. S. Band, A. Ul Rehman, S. Mahajan, Y. Ding, et al., Impact of quarantine on fractional order dynamical model of Covid-19, Comput. Biol. Med., 151 (2022), 106266. http://doi.org/10.1016/j.compbiomed.2022.106266 doi: 10.1016/j.compbiomed.2022.106266
![]() |
[45] |
P. Agarwal, R. Singh, A. Ul Rehman, Numerical solution of hybrid mathematical model of dengue transmission with relapse and memory via Adam–Bashforth–Moulton predictor-corrector scheme, Chaos, Solitons & Fractals, 143 (2021), 110564. http://doi.org/10.1016/j.chaos.2020.110564 doi: 10.1016/j.chaos.2020.110564
![]() |
[46] |
N. Sharma, R. Singh, J. Singh, O. Castillo, Modeling assumptions, optimal control strategies and mitigation through vaccination to zika virus, Chaos, Solitons & Fractals, 150 (2021), 111137. http://doi.org/10.1016/j.chaos.2021.111137 doi: 10.1016/j.chaos.2021.111137
![]() |
[47] |
A. Nursanti, B. L. Kalvein, Modeling of COVID-19 spread with self-isolation at home and hospitalized classes, Results Phys., 36 (2022), 105378. http://doi.org/10.1016/j.rinp.2022.105378 doi: 10.1016/j.rinp.2022.105378
![]() |
[48] |
P. O. Lolika, M. Helikumi, Global stability analysis of a COVID-19 epidemic model with incubation delay, Math. Model. Control, 3 (2023), 23–38. http://doi.org/10.3934/mmc.2023003 doi: 10.3934/mmc.2023003
![]() |
[49] |
J. Huo, H. Zhao, L. Zhu, The effect of vaccines on backward bifurcation in a fractional order HIV model, NONLINEAR ANAL-REAL., 26 (2015), 289–305. http://doi.org/10.1016/j.nonrwa.2015.05.014 doi: 10.1016/j.nonrwa.2015.05.014
![]() |
[50] |
P. A. Naik, J. Zu, K. M. Owolabi, Global dynamics of a fractional order model for the transmission of HIV epidemic with optimal control, Chaos, Solitons & Fractals, 138 (2020), 109826. http://doi.org/10.1016/j.chaos.2020.109826 doi: 10.1016/j.chaos.2020.109826
![]() |
[51] |
K. M. Owolabi, Behavioural study of symbiosis dynamics via the Caputo and Atangana–Baleanu fractional derivatives, Chaos, Solitons & Fractals, 122 (2019), 89–101. http://doi.org/10.1016/j.chaos.2019.03.014 doi: 10.1016/j.chaos.2019.03.014
![]() |
[52] |
K. M. Owolabi, A. Atangana, Mathematical analysis and computational experiments for an epidemic system with nonlocal and nonsingular derivative, Chaos, Solitons & Fractals, 126 (2019), 41–49. http://doi.org/10.1016/j.chaos.2019.06.001 doi: 10.1016/j.chaos.2019.06.001
![]() |
[53] |
Z. U. A. Zafar, K. Rehan, M. Mushtaq, HIV/AIDS epidemic fractional-order model, J. Differ. Equations Appl., 23 (2017), 1298–1315. http://doi.org/10.1080/10236198.2017.1321640 doi: 10.1080/10236198.2017.1321640
![]() |
[54] |
H. L. Li, L. Zhang, C. Hu, Y. L. Jiang, Z. Teng, Dynamical analysis of a fractional-order predator-prey model incorporating a prey refuge, J. Appl. Math. Comput., 54 (2017), 435–449. http://doi.org/10.1007/s12190-016-1017-8 doi: 10.1007/s12190-016-1017-8
![]() |
[55] |
D. Barros, L. C. Lopes, M. M. S. Pedro, F. Esmi, E. Santos, D. E. Sánchez, The memory effect on fractional calculus: an application in the spread of COVID-19, Comput. Appl. Math., 40 (2021), 1–21. http://doi.org/10.1007/s40314-021-01456-z doi: 10.1007/s40314-021-01456-z
![]() |
[56] |
V.-De-Leó n, Volterra-type Lyapunov functions for fractional-order epidemic systems, Commun. Nonlinear Sci. Numer. Simul., 24 (2015), 75–85. http://doi.org/10.1016/j.cnsns.2014.12.013 doi: 10.1016/j.cnsns.2014.12.013
![]() |
[57] |
M. Caputo, Linear models of dissipation whose Q is almost frequency independent-Ⅱ, Geophys. J. Int., 13 (1967), 529–539. http://doi.org/10.1111/j.1365-246x.1967.tb02303.x doi: 10.1111/j.1365-246x.1967.tb02303.x
![]() |
[58] |
K. Diethelm, The Analysis of Fractional Differential Equations: An Application-Oriented Exposition using Differential Operators of Caputon type, Lecture Notes in Mathematics, 247 (2010). https://doi.org/10.1007/978-3-642-14574-2 doi: 10.1007/978-3-642-14574-2
![]() |
[59] | I. Podlubny, Fractional Differential Equations, San Diego: Academic Pres, 1999. |
[60] |
H. Delavari, D. Baleanu, J. Sadati, Stability analysis of Caputo fractional-order nonlinear systems revisited, Nonlinear Dyn., 67 (2012), 2433–2439. http://doi.org/10.1007/s11071-011-0157-5 doi: 10.1007/s11071-011-0157-5
![]() |
[61] |
O. Diekmann, J. A. P. Heesterbeek, J. A. J. Metz, On the definition and the computation of the basic reproduction ratioR0 in models for infectious diseases in heterogeneous populations, J. Math. Biol., 28 (1990), 365–382. https://doi.org/10.1007/BF00178324 doi: 10.1007/BF00178324
![]() |
[62] | J. P. LaSalle, The Stability of Dynamical Systems, Society for Industrial and Applied Mathematics, 1976. |
[63] |
H. Mlyashimbi, E. Gideon, M. Steady, Dynamics of a Fractional-Order Chikungunya Model with Asymptomatic Infectious Class, Computational and Mathematical Methods in Medicine, (2022). http://doi.org/10.1155/2022/5118382 doi: 10.1155/2022/5118382
![]() |
[64] |
A. Dénes, B. A. Gumel, Modeling the impact of quarantine during an outbreak of Ebola virus disease, Infectious Disease Modelling, 4 (2019), 12–27. http://doi.org/10.1016/j.idm.2019.01.003 doi: 10.1016/j.idm.2019.01.003
![]() |
[65] |
A. Aatif, U. Saif, M. A. Khan, The impact of vaccination on the modeling of COVID-19 dynamics: a fractional order model, Nonlinear Dynam., (2022), 1–20. http://doi.org/10.1007/s11071-022-07798-5 doi: 10.1007/s11071-022-07798-5
![]() |
[66] | A. Leon, J. Hyman, Forward and adjoint sensitivity analysis with applications in dynamical systems, Lecture Notes in Linear Algebra and Optimization, (2005). |
[67] |
N. Faïçal, A. Iván, J. N. Juan, FM. T. Delfim, Mathematical modeling of COVID-19 transmission dynamics with a case study of Wuhan, Chaos, Solitons & Fractals, 135 (2020), 109846. http://doi.org/10.1016/j.chaos.2020.109846 doi: 10.1016/j.chaos.2020.109846
![]() |
[68] |
F. Wang, L. Cao, X. Song, Mathematical modeling of mutated COVID-19 transmission with quarantine, isolation and vaccination, Math. Biosci. Eng., 19 (2022), 8035–8056. https://doi.org/10.3934/mbe.2022376 doi: 10.3934/mbe.2022376
![]() |
[69] |
Y. B. Ruhomally, M. Mungur, A. A. H. Khoodaruth, V. Oree, M. A. Dauhoo, Assessing the Impact of Contact Tracing, Quarantine and Red Zone on the Dynamical Evolution of the Covid-19 Pandemic using the Cellular Automata Approach and the Resulting Mean Field System: A Case study in Mauritius, Appl. Math. Model., 111 (2022), 567–589. http://doi.org/10.1016/j.apm.2022.07.008 doi: 10.1016/j.apm.2022.07.008
![]() |
[70] |
C. Hou, J. Chen, Y. Zhou, L. Hua, J. Yuan, S. He, et al., The effectiveness of quarantine of Wuhan city against the Corona Virus Disease 2019 (COVID-19): A well-mixed SEIR model analysis, J. Med. Virol., 92 (2020), 841–848. http://doi.org/10.1002/jmv.25827 doi: 10.1002/jmv.25827
![]() |
[71] |
J. Nelder, R. Mead, A simplex method for function minimization, The computer Journal, 7 (1964), 308–313. http://doi.org/10.1093/comjnl/7.4.308 doi: 10.1093/comjnl/7.4.308
![]() |
1. | Zhoujin Cui, Tao Lu, Bo Chen, Ammar Alsinai, Traveling Wave Solutions of the Conformable Fractional Klein–Gordon Equation With Power Law Nonlinearity, 2024, 2024, 2314-4629, 10.1155/2024/8367957 |