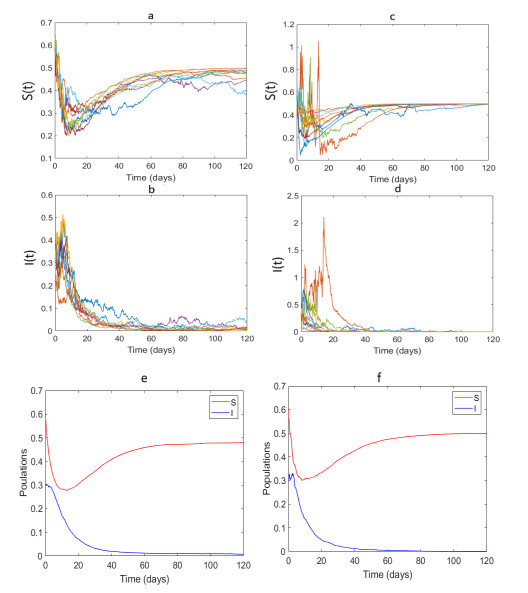
Epidemic models are used to understand the dynamics of disease transmission and explore the possible measures for preventing the spread of infection in the population. Disease transmission is intrinsically random and severely affected by environmental factors. We investigated a stochastic population model of the susceptible-infected-susceptible (SIS) type, in which infection spreads via both vertical and horizontal transmission routes. To incorporate stochasticity to the system, white multiplicative noise was taken into account in the horizontal disease transmission term. We proved that noise intensity, disease transmission, and recovery rates are potential routes for eradicating the disease. Furthermore, the parasite population reduces its fitness for some fixed noise if the relative fecundity of infected hosts and the disease transmission are low. However, if either of these is increased, it observes enhanced fitness. A simulation study illustrated the system's analytically dynamic properties and provided different insights. A case study for the imperfect vertical and horizontal infection transmission is also presented, supporting some of our observed theoretical results.
Citation: Abhijit Majumder, Debadatta Adak, Adeline Samson, Nandadulal Bairagi. Persistence and extinction of infection in stochastic population model with horizontal and imperfect vertical disease transmissions[J]. Mathematical Biosciences and Engineering, 2025, 22(4): 846-875. doi: 10.3934/mbe.2025030
[1] | Minna Shao, Hongyong Zhao . Dynamics and optimal control of a stochastic Zika virus model with spatial diffusion. Mathematical Biosciences and Engineering, 2023, 20(9): 17520-17553. doi: 10.3934/mbe.2023778 |
[2] | Xueqing He, Ming Liu, Xiaofeng Xu . Analysis of stochastic disease including predator-prey model with fear factor and Lévy jump. Mathematical Biosciences and Engineering, 2023, 20(2): 1750-1773. doi: 10.3934/mbe.2023080 |
[3] | Kangbo Bao, Qimin Zhang, Xining Li . A model of HBV infection with intervention strategies: dynamics analysis and numerical simulations. Mathematical Biosciences and Engineering, 2019, 16(4): 2562-2586. doi: 10.3934/mbe.2019129 |
[4] | Yang Chen, Wencai Zhao . Dynamical analysis of a stochastic SIRS epidemic model with saturating contact rate. Mathematical Biosciences and Engineering, 2020, 17(5): 5925-5943. doi: 10.3934/mbe.2020316 |
[5] | Chun Lu, Bing Li, Limei Zhou, Liwei Zhang . Survival analysis of an impulsive stochastic delay logistic model with Lévy jumps. Mathematical Biosciences and Engineering, 2019, 16(5): 3251-3271. doi: 10.3934/mbe.2019162 |
[6] | Tianfang Hou, Guijie Lan, Sanling Yuan, Tonghua Zhang . Threshold dynamics of a stochastic SIHR epidemic model of COVID-19 with general population-size dependent contact rate. Mathematical Biosciences and Engineering, 2022, 19(4): 4217-4236. doi: 10.3934/mbe.2022195 |
[7] | Ming-Zhen Xin, Bin-Guo Wang, Yashi Wang . Stationary distribution and extinction of a stochastic influenza virus model with disease resistance. Mathematical Biosciences and Engineering, 2022, 19(9): 9125-9146. doi: 10.3934/mbe.2022424 |
[8] | Cristina Anton, Alan Yong . Stochastic dynamics and survival analysis of a cell population model with random perturbations. Mathematical Biosciences and Engineering, 2018, 15(5): 1077-1098. doi: 10.3934/mbe.2018048 |
[9] | Zhongwei Cao, Jian Zhang, Huishuang Su, Li Zu . Threshold dynamics of a stochastic general SIRS epidemic model with migration. Mathematical Biosciences and Engineering, 2023, 20(6): 11212-11237. doi: 10.3934/mbe.2023497 |
[10] | Linda J. S. Allen, P. van den Driessche . Stochastic epidemic models with a backward bifurcation. Mathematical Biosciences and Engineering, 2006, 3(3): 445-458. doi: 10.3934/mbe.2006.3.445 |
Epidemic models are used to understand the dynamics of disease transmission and explore the possible measures for preventing the spread of infection in the population. Disease transmission is intrinsically random and severely affected by environmental factors. We investigated a stochastic population model of the susceptible-infected-susceptible (SIS) type, in which infection spreads via both vertical and horizontal transmission routes. To incorporate stochasticity to the system, white multiplicative noise was taken into account in the horizontal disease transmission term. We proved that noise intensity, disease transmission, and recovery rates are potential routes for eradicating the disease. Furthermore, the parasite population reduces its fitness for some fixed noise if the relative fecundity of infected hosts and the disease transmission are low. However, if either of these is increased, it observes enhanced fitness. A simulation study illustrated the system's analytically dynamic properties and provided different insights. A case study for the imperfect vertical and horizontal infection transmission is also presented, supporting some of our observed theoretical results.
Epidemic models deal with the transmission of infection in a population. They are frequently used to gain insights into disease dynamics and control mechanisms. Susceptible-infected (SI) type deterministic compartmental epidemic models with constant rate parameters have been extensively used to explore infection-related issues in different populations [1,2,3]. However, disease transmission is a random process that varies randomly due to exogenous factors. For example, disease transmission might be significantly affected by environmental factors like temperature, humidity, rainfall, etc. [4,5]. We cannot fully explain all the unknown factors in disease transmission through deterministic rules alone. Therefore, it is natural to use epidemic models incorporating random variations to account for environmental unpredictability. Incorporating such random variation in mathematical models leads to stochastic differential equations. Stochastic models can be derived by perturbing the model parameters randomly. An alternative approach consists of perturbing the state variables' equations, but the interpretability of the effect of the noise is more complicated here [6,7,8].
Disease transmission from one infected individual to another susceptible individual of a population is a significant issue, and the persistence of parasites and their virulence largely depend on this transmission mechanism [9,10,11]. Horizontal and vertical transmissions are two utterly distinct disease transmission modes usually followed by different pathogens [12]. Here, we consider a homogeneous mixture of susceptible and infected populations, where a disease spreads following both horizontal and vertical transmissions. In horizontal transmission, the infection spreads through contact. On the contrary, vertical transmission occurs through birth only. Though many parasites spread disease through multiple pathways, horizontal transmission is the predominant infection-spreading mode [13]. Infected hosts can give birth to susceptible and infected individuals, so the vertical transmission may be imperfect [9]. Stochastic population models with only horizontal disease transmission have been proposed in [14,15]. These researchers proved the existence of a stationary distribution condition for eradicating the disease from the population. A simple stochastic susceptible-infected-recovered (SIR) model with horizontal and vertical transmissions was considered in [16]. This model, however, entailed the linear birth rate of all populations, contrary to the realistic density-dependent birth. It is shown that considerable noise is conducive to controlling the infection. Li et al. [17] analyzed an SIRS epidemic model with a nonlinear incidence rate and environmental stochasticity and only horizontal transmission of infection with density-independent growth of susceptible populations. A set of sufficient conditions for the disease extinction and persistence is provided, and simulation results are presented.
Our main objective is to study a susceptible-infected-susceptible type compartmental population model with both disease transmission modes and uncertainty/stochasticity in the horizontal disease transmission term. The deterministic version of this model has similarities to the model studied by [2,18,19]. These researchers obtained the local stability results of the deterministic models and studied the trade-offs between modes of transmission and virulence. We determine the local and global stabilities of the corresponding deterministic system to compare its results with the stochastic model. A set of conditions is provided under which the deterministic solution becomes a limiting case of the stochastic solution. We also study the law of the disease extinction time. It is experimentally demonstrated that the relative fecundity of parasites plays a significant role in the persistence of infection and survival of both the host and parasite populations [19,20,21]. One way to measure the parasites' fitness is to determine the extinction time of the infected host population. We show how the relative fecundity and the disease transmissibility jointly affect the parasite fitness.
The rest of the paper is organized as follows. The SIS epidemic model is presented in Section 2. A brief study of the deterministic model is presented in Section 3. The stochastic system analysis is given in Section 4. The analytical results are illustrated in Section 5 including local and global sensitivity analyses. A case study for the imperfect vertical and horizontal infection transmission is provided in Section 6 to fortify our observed theoretical findings, and the paper ends with a discussion in Section 7.
The host population is divided into susceptible and infected classes in the presence of some parasite infection. Let S(t) and I(t) denote the densities of the susceptible and infected host populations at any time t, respectively. Infected hosts give birth to susceptible and infected individuals at rates bI and e, respectively. Parameter e thus represents the vertical transmission. The birth rate of the susceptible host is denoted by bS. Parasites may affect the fecundity and morbidity rates of the host population [22,23]. It is therefore assumed that the death rate of infected hosts is never less than that of susceptible hosts, i.e., uI≥uS, and the birth rate of susceptible hosts (bS) is higher than the total birth rate of infected hosts, i.e., bS≥bI+e. An infected individual may recover from the infection and join the susceptible class to be reinfected. Let β be the horizontal disease transmission coefficient, K be the environment's carrying capacity, and μ be the recovery rate of infected individuals. The following coupled nonlinear differential equations can represent the SIS epidemic model:
dSdt=bSS[1−(S+I)K]−uSS−βSI+eI[1−(S+I)K]+μI,dIdt=bII[1−(S+I)K]−uII+βSI−μI. | (2.1) |
This deterministic model has similarities to the model studied by Lipsitch et al. [2], where they considered μ=0 so that the underlying epidemic system is of SI type. They obtained the local stability results of the SI epidemic model and observed the implications of different transmission modes for the evolution of virulence. Mangin et al. [18], and Ebert et al. [19] considered a similar deterministic SI epidemic model with horizontal transmission only (where e=0 and μ=0) to understand the trade-offs between modes of transmission and virulence. A discrete version of the model (2.1) with μ=0 was also analyzed in [24]. Thus, the SIS model with both transmission modes is a modification of earlier models. However, our main interest is in the stochastic version of the above model. In the following, we proved the global stability of the equilibrium points of the system (2.1), which has not been studied.
Horizontal disease transmission may occur directly through the effective contact between a susceptible and an infective or indirectly through the environment and intermediate host populations [25]. Such transmissions are primarily unknown and random. Assuming that the disease transmission process is random and directly affects the horizontal disease transmission parameter β, we replace the parameter β by β+σdξt, where σ is a real constant that measures the intensity of the noise and ξ(t)t≥0 is a standard Wiener process defined on a complete probability space (Ω,F,P) with a filtration {Ft}t∈ℜ+ and satisfies <dξ(t)>=0, <dξ(t),dξ(t′)>=δ(t−t′), where δ is the Dirac delta function. It induces a multiplicative noise and thus avoids the negativity of the solution due to initial negative fluctuations. Zero becomes a lower bound in this case, even if the initial population size is small and there is a negative fluctuation of noise [26]. Under this assumption, the stochastic SIS epidemic model (2.1) with horizontal transmission and imperfect vertical transmission becomes
dS=[bSS{1−S+IK}−uSS−βSI+eI{1−S+IK}+μI]dt−σSIdξt,dI=[bII{1−S+IK}−uII+βSI−μI]dt+σSIdξt. | (2.2) |
Here, we analyze stochastic system (2.2), which considers density-dependent growth of host populations and horizontal as well as imperfect vertical transmissions of infection. We provide the local and global dynamics of the corresponding deterministic system with respect to the deterministic basic reproduction number. The persistence and extinction conditions of disease for the stochastic system are derived. The stochastic process' ergodicity and the stationary distribution criteria are proved. We present different simulation results to illustrate the theoretical results and provide insights into parasites' fitness.
Deterministic system (2.1) includes an additional recovery term of the infected host with respect to the model studied in [2]. Local stability of different equilibrium points of the system (2.1) with no recovery (i.e., μ=0) has been proved [2,24]. Here, we start by recalling (without proof) the local dynamics of system (2.1), where recovery is considered, and then provide a new global stability result.
The basic reproductive number is an essential measure in epidemics and is critical in eradicating infection. It is defined as the number of new cases arising from a single infected individual when introduced into a susceptible population group [27]. The disease cannot be established in the host population if the basic reproduction number is less than unity. Otherwise, the disease can invade the population. We measure the basic reproduction number of deterministic system (2.1) through the next-generation matrix [28].
Proposition 1. The basic reproduction number, RD0, of deterministic system (2.1) is
RD0=bIuS+βˆSbSbS(uI+μ). | (3.1) |
Proof. In the absence of infection, the susceptible population has equilibrium density ˆS=K(1−usbs). The Jacobian matrix of the epidemic model (2.1) evaluated at (ˆS,0) is
J11=(bS−2bSˆSK−uSe+μ−bSˆSK−eˆSK−βˆS0bI(1−ˆSK)+βˆS−(uI+μ)). | (3.2) |
The sub-matrix of J11 associated with the infectious compartment is a one-by-one matrix
J12=bI(1−ˆSK)+βˆS−(uI+μ)=F−V, |
where F=bI(1−ˆSK)+βˆS and V=(uI+μ). The next-generation matrix is then given by
FV−1=1uI+μ[bI(1−ˆSK)+βˆS]=bIuSbS(uI+μ)+βˆSuI+μ=bIuS+βˆSbSbS(uI+μ). |
The basic reproduction number of system (2.1) is the spectral radius of the scalar matrix FV−1 [28] and is thus
RD0=ρ(FV−1)=bIuS+βˆSbSbS(uI+μ). | (3.3) |
As mentioned earlier, infection will die out from the population if the basic reproduction number is less than 1. A straightforward way to reduce the basic reproduction number is to decrease the horizontal transmission coefficient β. Increasing parameter μ can also reduce the value of the basic reproduction number and may be a parameter of interest.
From a biological point of view and for the model's applicability, solutions should be non-negative at any time, and the deterministic model solutions should stay bounded. For this, one can easily prove the following result in the line of [29].
Proposition 2. Solutions of system (2.1) are positively invariant and uniformly bounded in the domain G⊂R2+, where G={(S,I)∈R2+|0≤S(t),I(t)≤K}.
The local stability results (without proof) may be summarized as follows.
Theorem 1. Model (2.1) has three non-negative equilibrium points.
(i) The trivial equilibrium point E(1)0 = (0,0) is locally asymptotically stable if bS<uS and bI<uI+μ.
(ii) The disease-free equilibrium point E(1)1=(ˆS,0), where ˆS=K(1−uSbS), uS<bS, is locally asymptotically stable if RD0<1.
(iii) The infected (interior) equilibrium point E(1)∗=(S∗,I∗), having equilibrium densities
S∗=−B2A+12A√B2−4ACandI∗=1bI[K(bI−uI−μ)+(βK−bI)S∗], |
exists and becomes locally asymptotically stable under conditions
bS≥bI+e,μ+uI<bI<βKandRD0>1, |
where A=βKb2I[βK(bI+e)+bI(bS−bI−e)], B=−K(bS−uS)+KbI[(bI−uI−μ)(Kβ+bS+e)−(e+μ)(βK−bI)]+2Ke(βK−bI)bI2(bI−uI−μ), C=−K2bI2[bI−uI−μ][μ(bI+e)+euI].
Biologically, all populations go extinct if the birth rate of susceptible hosts is less than its death rate and the ensemble clearance rate of infected hosts exceeds its birth rate. Noticeably, the stability of the trivial equilibrium ceases the existence of the other two equilibrium points.
The disease-free equilibrium point exists if the birth rate of susceptible hosts is larger than its death rate and becomes stable if the basic reproduction number is less than unity. It is worth mentioning that local stability criteria of E(1)1 makes E(1)∗ and E(1)0 unstable.
The infected equilibrium E(1)∗ exists, and both populations persist in a stable state if the birth rate of susceptible hosts is higher than the total birth rate of infected hosts, i.e., bS≥bI+e. In addition, the vertical birth rate is higher than its ensemble clearance rate of infected hosts but lower than the maximum disease transmission rate through horizontal transmission, i.e., μ+uI<bI<βK, and the basic reproduction number is greater than unity. Thus, the basic reproduction number R(D)0 must be less than unity to make the system disease-free. This is achievable through parameter μ, which transforms an SI system into an SIS one.
Local asymptotic stability guarantees that the solutions will reach equilibrium if they start close to the equilibrium value. However, if the considered initial values are far from equilibrium, they may not arrive at the equilibrium point. To assure that the equilibrium point's stability does not depend on the initial values, it is necessary to show its global stability. We prove that the local stability results of different equilibrium points are sufficient for their global stability.
Theorem 2. Each equilibrium of the model (2.1) is locally and globally asymptotically stable throughout domain G⊂R2+, unless the solution starts from the other two equilibrium points.
Proof. We first show that there is no periodic orbit in D⊂G, where D = {(S,I): 0<S<K, 0<I<K}. For this, we define a continuously differentiable function B(S,I) in D, where B(S,I) = 1/(SI). Defining F=(F1,F2), where F1=bS{(1−S+IK)−uS−βI}S+eI[1−S+IK]+μI, F2=bII[1−S+IK]−uII+βSI−μI, and noting that I≤Kby Proposition2, one then has
div(BF)=∂(BF1)∂S+∂(BF2)∂I=−bSKI−bIKS−μS2+eS2(I−K)K<0. | (3.4) |
Hence, by Bendixson-Dulac criteria [30], system (2.1) has no periodic orbit in the interior of D. Since E(1)0 is the only stable equilibrium, there is no periodic orbit in the domain of definition. Therefore, E(1)0 is globally asymptotically stable whenever it is locally asymptotically stable. Similar arguments prove that local stability criteria of E(1)1 and E(1)∗ also assure their global stability if the solutions are not started from the equilibrium point E(1)∗ for the first case and E(1)1 for the second case.
In this section, we present our major results of the SIS epidemic model with both modes of disease transmission. We use the Lyapunov analysis method, Ito's formula, Chebyshev's inequality, the law of large numbers, and the other standard techniques to obtain new results for system (2.2).
A population explosion may occur in the case of a multiplicative noise [31]. It is, therefore, necessary to show that such an explosion does not happen here. Also, biological populations should always be nonnegative. For this, we prove the stochastic solutions' global existence, positivity, and boundedness.
Proposition 3. For any initial value (S(0),I(0)) ∈ R2+, there exists a unique solution (S(t),I(t)) of the system (2.2) for t≥0, and the solution remains in R2+ with probability 1.
Proof. Since the coefficients of system (2.2) satisfy local Lipschitz conditions, there is a unique positive local solution on [0,νe), where νe is the explosion time [32]. We show that this solution is global, meaning νe=∞. One gets from (2.2)
ddt(S+I)=bSS(1−S+IK)+eI(1−S+IK)+bII(1−S+IK)−uSS−uII≤{bSS+eI+bII}(1−S+IK)−uSS−uII≤{bSS+(bI+e)I}(1−S+IK)≤bS(S+I)−bSK(S+I)2[∵bS≥bI+e], |
giving
0≤lim |
Let m_0 > 0 be sufficiently large so that S(0) , I(0) lie within the interval [\frac{1}{m_0}, m_0] . For any integer m \geq m_0 , define a sequence of stopping times by
\nu_m = \mbox{inf}\; \; \bigg\{t \in [0, \nu_e]: S(t) \notin \left(\frac{1}{m}, m\right) \; \; \mbox{or}\; \; I(t) \notin \left(\frac{1}{m}, m\right)\bigg\}, |
where we set inf \Phi = \infty ( \Phi represents the empty set). Since \nu_m is non-decreasing as m \to \infty , one gets \nu_\infty = \lim_{n \to \infty}\nu_m. Then, \nu_\infty \leq \nu_e almost surely (a.s.). Now, we prove that \nu_\infty = \infty a.s. If this statement is violated, then there exists M > 0 and \delta \in (0, 1) such that P\{\nu_\infty \leq M\} > \delta. Thus, there is an integer m_1 \geq m_0 such that
\begin{equation} P\{\nu_m\leq M\} \geq \delta \; \; \; \forall m \geq m_1. \end{equation} | (4.1) |
Define a C^2 function V: \mathbb{R}^2_+ \to \mathbb{R}_+ by
\begin{equation} V(S, I) = (S-1-\mbox{log}\; S)+ (I-1-\mbox{log}\; I).\nonumber \end{equation} |
Using Ito's formula, we have
\begin{equation} \label{unique_solution} \begin{split} dV(S, I) & = \left(1-\frac{1}{S}\right)\left[b_S S \left(1-\frac{S+I}{K}\right)-u_S S-\beta S I+ e I \left(1-\frac{S+I}{K}\right)+\mu I \right] dt \nonumber \\ &+ \frac{1}{2} \sigma^2I^2 dt+\frac{1}{2}\sigma^2S^2 dt +\left(1-\frac{1}{I}\right)\left[b_I I \left(1-\frac{S+I}{K}\right)-u_I I+\beta S I-\mu I \right] dt \nonumber \\ &- \sigma \left(1-\frac{1}{S}\right)SI\; d\xi(t)+ \sigma \left(1-\frac{1}{I}\right) SI\; d\xi(t) \nonumber \\ &\leq \left[(u_S+u_I+\mu)+(b_S+b_I+e+\beta)K+\sigma^2 K^2\right] dt +\sigma (I-S)\; d\xi(t). \end{split} \end{equation} |
Let U = (u_S+u_I+\mu)+(b_S+b_I+e+\beta)K+\sigma^2 K^2 . Integrating both sides of (4.2) from 0 to \nu_m \wedge M , one gets
\begin{equation} \int^{\nu_m \wedge M}_0 dV(S(u), I(u)) \leq \int^{\nu_m \wedge M}_0 U du + \int^{\nu_m \wedge M}_0\left(\sigma S\; d\xi_1(u)+ \sigma I\; d\xi_2(u)\right). \end{equation} | (4.2) |
Expectation in both sides yields
\mathbb{E}\left(V(S(\nu_m \wedge M), I(\nu_m \wedge M))\right) \leq V(S(0), I(0)) +U M. |
Set G_m = \{\nu_m \leq M\} for m\geq m_1 and from (4.1), we have \mathbb{P}(G_m) \geq \delta. For every \tau \in G_m , S(\nu_m, \tau) , I(\nu_m, \tau) are equal to m or \frac{1}{m} , implying that V(S(\nu_m, \tau), I(\nu_m, \tau)) is no less than min \{m-1-\; \mbox{ln} (m), 1/m-1- \; \mbox{ln} (1/m)\} . Therefore, we have
\begin{equation} \begin{split} & V(S(0), I(0))+UM \geq \mathbb{E}\left(1_{G_m(\tau)} V(S(\nu_m), I(\nu_m))\right) \geq \nonumber \\ &\delta\; \mbox{min}\; \left\{m-1-\; \mbox{ln}\; m, \frac{1}{m}-1-\; \mbox{ln}\; \frac{1}{m}\right\}, \nonumber \end{split} \end{equation} |
where 1_{G_m(\tau)} is the indicator function of G_m . Then, m \to \infty leads to the contradiction
\infty = V(S(0), I(0))+UM < \infty. |
Therefore, \nu_\infty = \infty a.s. This completes the proof.
Proposition 4. Solutions of system (2.2) are stochastically bounded on any time interval for any initial value (S(0), I(0)) \in \mathbb{R}^2_+ .
Proof. From (2.2), we have
\begin{equation} \begin{split} &d(S+I) = b_S S \left(1-\frac{S+I}{K}\right)+(b_I+e)I\left(1-\frac{S+I}{K}\right)-u_S S-u_II \nonumber\\ &\geq M (S+I)\left(1-\frac{S+I}{K}\right)-N (S+I), \nonumber \\ &\; \; \mbox{where}\; M = \; \mbox{min}\; \{b_S, b_I+e\}, \; N = \; \mbox{min}\; \{u_S, u_I\} \nonumber \\ & = (M-N) (S+I)-\frac{M}{K}(S+I)^2. \nonumber \end{split} \end{equation} |
Therefore, \lim_{t \to \infty} (S+I) \geq \frac{K}{M}(M-N) = \bar{L} (say).
Define the function
W(S, I) = e^t (S^\theta+I^\theta) = e^t U(S, I), |
for (S, I) \in \mathbb{R}^2_+ , \theta > 1 and U(S, I) = S^\theta+I^\theta . By Ito's formula, we have
\begin{equation} \label{expectation} \begin{split} dW(S, I) & = e^t \bigg[\theta S^{\theta-1}\left(b_S S\left(1-\frac{S+I}{K}\right)-u_S S-\beta SI +e I\left(1-\frac{S+I}{K}\right)+\mu I\right) \nonumber\\ & + \theta I^{\theta-1}\left(b_I I\left(1-\frac{S+I}{K}\right)-u_I I+\beta SI -\mu I\right) \nonumber \\ & +\frac{\theta (\theta-1)}{2}\bigg(\sigma^2 S^\theta I^2+ \sigma^2 I^\theta S^2\bigg)\bigg]dt+ e^t \theta \left[-\sigma S^\theta I\; d\xi(t) + \sigma I^\theta S d\xi(t)\right] \nonumber \\ & \leq e^t \bigg[\theta K^{\theta-1}(b_S+e+\mu) K+\theta K^{\theta-1}(b_I+ \beta K) K+\theta (\theta-1)\sigma^2 K^{\theta+2}\bigg] dt \nonumber \\ & + e^t \theta \left[-\sigma S^\theta I\; d\xi(t) + \sigma I^\theta S d\xi(t)\right] \nonumber\\ & = A e^t dt+ \theta \sigma e^t \bigg[- S^\theta I\; d\xi(t) + I^\theta S d\xi(t)\bigg], \end{split} \end{equation} |
where A = \theta K^{\theta-1}(b_S+e+\mu) K+\theta K^{\theta-1}(b_I+ \beta K) K+\theta (\theta-1)\sigma^2 K^{\theta+2} .
Using Proposition 3 and from (4.3), one gets
\mathbb{E}\left(e^{t\wedge \nu_m}U(S(t \wedge \nu_m), I(t \wedge \nu_m))\right)\leq W(S(0), I(0))+ A \mathbb{E} \left( \int^{t\wedge \nu_m}_{0} e^u du\right). |
Making m \to \infty , we have
\mathbb{E}\left( e^t U(S(t), I(t)) \right) \leq W(S(0), I(0))+A(e^t-1), |
implying
\mathbb{E}\left(U(S(t), I(t)) \right) \leq e^{-t}W(S(0), I(0))+A-A e^{-t}. |
Defining |Y(t)| = \left(S^2(t)+I^2(t)\right)^{\frac{1}{2}} and noting that |Y(t)|^\theta = (S^2(t)+I^2(t))^\frac{\theta}{2} \leq 2^{\frac{\theta}{2}} \; \mbox{max}\; \left\{S^\theta (t), I^\theta(t)\right\} \leq 2^{\frac{\theta}{2}}\left(S^\theta+I^\theta\right), we obtain \mathbb{E}\left(|Y(t)|^\theta\right) \leq 2^{\frac{\theta}{2}}\left(e^{-t}W(S(0), I(0))+A-Ae^{-t}\right). Thus, \limsup_{t \to \infty} \mathbb{E}\left(|Y(t)|^\theta\right)\leq 2^\frac{\theta}{2} A < \infty. Therefore, there exists a positive constant \eta such that \limsup_{t \to \infty} \mathbb{E}\left(\sqrt{Y(t)}\right) < \eta. For any \nu > 0 , \omega = \frac{\eta^2}{\nu^2} > 0 , and using the Chebyshev's inequality, \mathbb{P}(|Y(t)| > \omega)\leq \frac{\mathbb{E}\left(\sqrt{Y(t)}\right)}{\sqrt\omega}, one gets
\limsup\limits_{t \to \infty}\mathbb{P}\left(|Y(t)| > \omega\right)\leq \frac{\eta}{\sqrt\omega} = \nu. |
Hence, the result is proven.
One important concern in epidemiology is the eradication of infection from the population. The average infected population in the time interval [0, t] is \frac{1}{t} \int_{0}^{t}I(\kappa)\; d\kappa . The infected population is said to be strongly non-persistent or extinct if the supremum of its limiting average population is zero. In this case, the infection is said to be removed from the system. On the other hand, if the infimum of its limiting average population is always positive, then the I population is said to be strictly persistent [33]. In this case, the eventual average I will always stay away from zero, and the infection consistently remains in the system. In the following two theorems, we provide sufficient conditions to eliminate the disease or persist almost surely in stochastic system (2.2).
Before proving these results, we state the following well-known lemma.
Lemma 1. [34] Suppose w(t)\in C(\Omega\times[0, \infty), \mathbb{R}^0_+) , where \mathbb{R}^0_+ = \{p\; \rvert\; p > 0, p\in\mathbb{R}\} .
(i) If there exist two positive constants T and \kappa_0 such that
\begin{equation} \nonumber \ln(w(t))\leq\kappa t-\kappa_0\int^t_0 w(\theta)d\theta+\Sigma^n_{i = 1}\alpha_iB_i(t), \; \; \forall t\geq T, \kappa \in \mathbb{R}, \end{equation} |
where \alpha_i\; (1\leq i\leq n) are constants, then
\begin{equation} \nonumber \left\{ \begin{array}{ll} \limsup\nolimits_{t\rightarrow \infty}\frac{1}{t}\int^t_0 w(\theta)d\theta \leq \frac{\kappa}{\kappa_0}\; \; almost\; surely\; (a.s.)\; if \; \kappa\geq 0;& \\ \lim\nolimits_{t\rightarrow \infty} w(t) = 0\; \; a.s. \; if\; \kappa < 0.& \end{array} \right. \end{equation} |
(ⅱ) If there exist three positive constants T, \; \kappa, \; \kappa_0 such that
\begin{equation} \nonumber \ln(w(t))\geq\kappa t-\kappa_0\int^t_0 w(\theta)d\theta+\Sigma^n_{i = 1}\alpha_iB_i(t), \; \; \forall t\geq T, \end{equation} |
then
\begin{equation} \nonumber \liminf\limits_{t\rightarrow \infty}\frac{1}{t}\int^t_0 w(\theta)d\theta \geq \frac{\kappa}{\kappa_0}\; \; a.s. \end{equation} |
Using the above definition of extinction and the Lemma 1(ⅰ), we present a vital result that guarantees disease eradication in the stochastic system.
Theorem 3. If R^S_0 = \frac{\frac{\beta^2}{2 \sigma^2}+b_I}{u_I+\mu} < 1 , then the infected population of model (2.2) goes to extinction almost surely.
Proof. Let (S(t), I(t)) be a solution of the system (2.2) with initial value (S(0), I(0)) . Applying Ito's formula in the second equation of system (2.2), we have
\begin{equation} \label{extinction_eqn} \begin{split} d(\ln I(t)) & = \left[b_I\left(1-\frac{S+I}{K}\right)-u_I+\beta S-\mu-\frac{1}{2} \sigma^2 S^2\right] dt+\sigma S d\xi(t) \nonumber \\ & = \left[b_I-\frac{\sigma^2}{2}\left(S-\frac{\beta}{\sigma^2}\right)^2+\frac{\beta^2}{2 \sigma^2}-(u_I+\mu)-\frac{b_I}{K}(S+I)\right]dt+\sigma S d\xi(t) \nonumber \\ &\leq \left[\frac{\beta^2}{2 \sigma^2}+b_I-(u_I+\mu)\right] dt +\sigma S d\xi(t). \end{split} \end{equation} |
Integration on the both sides of (4.3) from 0 to t , and division by t yields
\begin{equation} \frac{\ln I(t)}{t} \leq \left[\frac{\beta^2}{2 \sigma^2}+b_I-(u_I+\mu)\right] + \frac{\ln I(0)}{t}+\frac{M(t)}{t}, \end{equation} | (4.3) |
where, M(t) = \int_0^t \sigma S(\tau)d\xi(\tau) is the local martingale with M(0) = 0 . Moreover, < M, M > _t = \left(\int_0^t \sigma S(\tau) d\xi(\tau)\right)^2 = \int_0^t \sigma^2 S^2(\tau) d\tau \leq \sigma^2 K^2 t . One then has \limsup_{t \to \infty} \frac{ < M, M > _t}{t} \leq\sigma^2 K^2 < \infty a.s. Therefore, by the law of a large number [35], \lim_{t \to \infty} \frac{M(t)}{t} = 0 a.s. Taking limit superior on both sides of (4.3) and using the Lemma 1, we obtain
\begin{equation} \limsup\limits_{t \to \infty} \frac{\ln I(t)}{t} \leq \frac{\beta^2}{2 \sigma^2}+b_I-(u_I+\mu) < 0, \nonumber \end{equation} |
whenever R^S_0 = \frac{\frac{\beta^2}{2 \sigma^2}+b_I}{u_I+\mu} < 1 . Thus, we have \lim_{t \to \infty} I(t) = 0 for R^S_0 < 1. This completes the proof.
The condition R^S_0 < 1 may be considered the equivalent basic reproduction number for the stochastic system. The stochastic basic reproduction number ( R^S_0 ) prescribes some restrictions on the system parameters and noise. If the condition is satisfied, the infected population will go extinct, and the system will be disease-free. One can easily observe that R^S_0 is a decreasing function of the recovery rate, \mu , and the noise intensity, \sigma . The recovery rate may be increased by taking suitable external measures; consequently, R^S_0 can be made less than unity to make the eradication process possible. Similarly, additional noise may also be helpful in disease elimination. However, the disease transmission parameter \beta and the birth rate b_I of the infected host are positively correlated with R^S_0 .
The following theorem is an ergodic behavior of I population of stochastic system (2.2).
Theorem 4. If \frac{b_I-u_I-\mu}{\frac{b_I}{K}+\frac{A(b_I+e)}{b_S}-\frac{A u_I}{b_S}} > 0 , the infected population I persists in mean a.s., and
\liminf\limits_{t\rightarrow \infty} < I(t) > = \frac{b_I-u_I-\mu}{\frac{b_I}{K}+\frac{A(b_I+e)}{b_S}-\frac{A u_I}{b_S}}, \; \; \mathit{\mbox{where}}\; \; A = \; \mathit{\mbox{min}}\; \left\{\beta-\frac{b_I}{K}, \frac{K \sigma^2}{2}\right\}. |
Proof. Integrating both the Equations of (2.2) from 0 to t and dividing by t , one obtains
\begin{equation} \label{persistence_cal} \begin{split} \frac{S(t)-S(0)}{t}+\frac{I(t)-I(0)}{t} & = b_S \bigg < S\left(1-\frac{S+I}{K}\right)\bigg > -u_S < S > + e \bigg < I\left(1-\frac{S+I}{K}\right)\bigg > \nonumber \\ &+ b_I \bigg < I\left(1-\frac{S+I}{K}\right)\bigg > -u_I < I > , \end{split} \end{equation} |
where < x(t) > = \frac{1}{t}\int_0^t x(s)\; ds. Since S and I are stochastically ultimately bounded, \lim_{t \to \infty}\frac{S(t)-S(0)}{t} = 0 and \lim_{t \to \infty}\frac{I(t)-I(0)}{t} = 0 . Eq (4.4) then becomes
\begin{equation} \begin{split} & b_S \bigg < \left(S-\frac{S^2}{K}\right)\bigg > + e < I > +b_I < I > -u_I < I > \; \; \geq b_S \bigg < S\left(1-\frac{S+I}{K}\right)\bigg > \nonumber \\ &- u_S < S > + e \bigg < I\left(1-\frac{S+I}{K}\right)\bigg > +b_I \bigg < I\left(1-\frac{S+I}{K}\right)\bigg > -u_I < I > . \end{split} \end{equation} |
Taking limit as t goes to \infty , we have
\begin{equation} \lim\limits_{t \to \infty} \bigg < \left(S-\frac{S^2}{K}\right)\bigg > \geq \frac{u_I-(b_I+e)}{b_S} < I > . \end{equation} | (4.4) |
Using Ito's formula in the second equation of system (2.2), one gets
\begin{equation} \label{persistence_eqn} \begin{split} d(\mbox{ln} I(t)) & = \left[(b_I-u_I-\mu)+\left(\beta S-\frac{b_I}{K}S-\frac{1}{2} \sigma^2 S^2\right)-\frac{b_I}{K}I\right] dt+\sigma S d\xi(t) \nonumber \\ & \geq \left[(b_I-u_I-\mu)+A\left(S-\frac{S^2}{K}\right)-\frac{b_I}{K}I\right] dt+\sigma S d\xi(t), \end{split} \end{equation} |
where A = \; \mbox{min}\; \left\{\beta-\frac{b_I}{K}, \frac{K \sigma^2}{2}\right\} . Integrating both sides of (4.5) from 0 to t , dividing by t , and using (4.4), we get
\begin{equation} \frac{\mbox{ln}\; I(t)}{t} \geq (b_I-u_I-\mu)- \left\{\frac{b_I}{K}+\frac{A(b_I+e)}{b_S}-\frac{A u_I}{b_S}\right\} < I > +\frac{\mbox{ln}\; I(0)}{t}+\frac{\sigma}{t}\int_0^t S(\tau) d\xi(\tau). \end{equation} | (4.5) |
Since I is bounded, \lim_{t \to \infty}\frac{\mbox{ln}\; I(0)}{t} = 0 and by the argument given previously, \lim_{t \to \infty}\frac{\sigma}{t}\int_0^t S(\tau) d\xi(\tau) = 0 . Therefore, using Lemma 1, we have
\begin{equation} \liminf\limits_{t\rightarrow \infty} < I(t) > \; \geq\; \frac{b_I-u_I-\mu}{\frac{b_I}{K}+\frac{A(b_I+e)}{b_S}-\frac{A u_I}{b_S}}. \end{equation} | (4.6) |
Thus, if the condition stated in the theorem holds then the infected population persists almost surely for all future time.
It is worth mentioning that stochastic system (2.2) has no explicit equilibrium density, unlike the deterministic system (2.1). Instead, stochastic solutions fluctuate around the deterministic equilibrium or a fixed value. We provide below the conditions under which the behavior of the stochastic system is similar to that of the deterministic equilibrium solution.
Theorem 5. Let (S(t), I(t)) be the solution of (2.2) with initial value (S(0), I(0)) \in \mathbb{R}_+^2 and the stability criteria of E^* hold. If \frac{b_S}{K}(S^*+1)+g_1 \bar{L}+\frac{b_I I^*}{K}+u_S-\left(\frac{3}{2}b_s+e+b_I\right) > 0 , \frac{e}{K}(S^*+I^*)+\frac{(b_S+b_I) S^*}{K}+\frac{b_I}{K}I^*+g_2 \bar{L}+ \frac{c_1 b_I}{K}+u_I-\left(\frac{b_S}{2}+2(e+b_I)\right) > 0 and \frac{2S^* b_S }{K}+\frac{e(S^*+I^*)}{K}+\frac{b_I(S^*+I^*)}{K}-b_S-b_I-e > 0 , then \limsup_{t\rightarrow \infty}\frac{1}{t} \int_0^t [(S(\tau)-S^*)^2+(I(\tau)-I^*)^2] d(\tau) \leq G_1 \sigma^2 \; \; \mathit{\mbox{a.s.}}, where E^* = (S^*, I^*) is the endemic equilibrium of the deterministic system and G_1 = \frac{K^2\left(K^2+\frac{c_1}{2}I^*\right)}{H} , H = \mathit{\mbox{min}}\; \bigg\{\frac{b_S}{K}(S^*+1)+g_1 \bar{L}+\frac{b_I I^*}{K}+u_S-\left(\frac{3}{2}b_s+e+b_I\right), \frac{e}{K}(S^*+I^*)+\frac{(b_S+b_I) S^*}{K}+\frac{b_I}{K}I^*+g_2 \bar{L}+ \frac{c_1 b_I}{K}+u_I-\left(\frac{b_S}{2}+2(e+b_I)\right) \bigg\} , g_1 = \mathit{\mbox{min}}\left\{\frac{e}{K}, \frac{b_S}{K}\right\} , g_2 = \mathit{\mbox{min}}\left\{\frac{e}{K}, \frac{b_I}{K}\right\} and \bar{L} = \frac{K}{M}(M-N) .
Proof. Define a positive function G = \frac{1}{2}(S-S^*+I-I^*)^2+ c_1\left(I-I^*-I^*\mbox{log}\; \frac{I}{I^*}\right) , where c_1 is a positive constant to be determined later. At (S^*, I^*) , we have
\begin{equation} \label{equilibrium_condition} \begin{split} b_S S^* \left(1-\frac{S^*+I^*}{K}\right) +e I^* \left(1-\frac{S^*+I^*}{K}\right) & = u_S S^*+\beta S^* I^* -\mu I^* \nonumber \\ \beta S^* I^*+b_I I^* \left(1-\frac{S^*+I^*}{K}\right) & = u_II^*+\mu I^*. \end{split} \end{equation} |
Using (4.7) and Ito's formula, one obtains
\begin{equation} \label{stability_stochastic} \begin{split} dG & = (S-S^*+I-I^*)\bigg[b_S S\left(1-\frac{S+I}{K}\right)-u_sS+eI \left(1-\frac{S+I}{K}\right)+ b_II \left(1-\frac{S+I}{K}\right)-u_I I\bigg]dt +\sigma^2 S^2I^2 dt\nonumber \\ & +c_1 \left(1-\frac{I^*}{I}\right) \bigg[\bigg\{b_I I \left(1-\frac{S+I}{K}\right) - u_I I+\beta SI-\mu I\bigg\}dt+\sigma SI d\xi(t)\bigg] +\frac{c_1}{2} \sigma^2 S^2 I^* dt \nonumber\\ & = \left[b_s-\frac{b_S}{K}(S+S^*+1)-\frac{eI}{K}-\frac{b_I I^*}{K}-u_S\right](S-S^*)^2 +\bigg[e+b_I -\frac{e}{K}(S^*+I^*+I)- \frac{(b_S+b_I) S^*}{K} \nonumber\\ & -\frac{b_I}{K}(I+I^*) -\frac{c_1 b_I}{K}-u_I\bigg] (I-I^*)^2 + \bigg[b_S-\frac{b_S }{K}(2S^*+S+I)+e-\frac{e}{K}(S^*+I^*+2I)+b_I \nonumber\\ & -\frac{b_I}{K}(s^*+I^*+2I) - u_I-u_S+ c_1\left(\beta -\frac{b_I}{K}\right) \bigg](S-S^*)(I-I^*) +\sigma^2 S^2I^2 dt+\frac{c_1}{2} \sigma^2 S^2 I^* dt \nonumber\\ & + c_1\sigma(I-I^*)S d\xi(t)\nonumber \\ & \leq \left[b_s-\frac{b_S}{K}(S^*+1)-g_1\bar{L}-\frac{b_I I^*}{K}-u_S\right](S-S^*)^2 +\bigg[e+b_I-\frac{e}{K}(S^*+I^*) -\frac{(b_S+b_I) S^*}{K}-\frac{b_I}{K}I^* \nonumber \\ &-g_2 \bar{L} -\frac{c_1 b_I}{K}-u_I\bigg] (I-I^*)^2 +\bigg[b_S-\frac{2S^* b_S }{K}+e -\frac{e(S^*+I^*)}{K}+b_I-\frac{b_I(S^*+I^*)}{K} \nonumber \\ & + c_1\left(\beta -\frac{b_I}{K}\right)\bigg] (S-S^*)(I-I^*) + \bigg[\frac{b_S}{K}(S+I) + \frac{2(e+b_I)I}{K}\bigg] |(S-S^*)(I-I^*)| +\sigma^2 S^2I^2 dt \nonumber \\ & +\frac{c_1}{2} \sigma^2 S^2 I^* dt + c_1\sigma(I-I^*)S d\xi(t). \end{split} \end{equation} |
Choosing c_1 = \frac{1}{\beta-\frac{b_I}{K}} \bigg[\frac{2S^* b_S }{K}+\frac{e(S^*+I^*)}{K}+\frac{b_I(S^*+I^*)}{K}-b_S-b_I-e\bigg] > 0 and using |(S-S^*)(I-I^*)| \leq \frac{1}{2}\left((S-S^*)^2+(I-I^*)^2\right) , (4.7) becomes
\begin{equation} \label{stability_eqn} \begin{split} dG &\leq -\bigg\{\left[\frac{b_S}{K}(S^*+1)+g_1 \bar{L}+\frac{b_I I^*}{K}+u_S-\left(\frac{3}{2}b_s+e+b_I\right)\right](S-S^*)^2 +\bigg[\frac{e}{K}(S^*+I^*)+\frac{(b_S+b_I) S^*}{K} \nonumber \\ & +\frac{b_I}{K}I^*+g_2 \bar{L} + \frac{c_1 b_I}{K}+u_I-\left(\frac{b_S}{2}+2(e+b_I)\right)\bigg] (I-I^*)^2\bigg\} +\sigma^2 S^2I^2 dt+\frac{c_1}{2} \sigma^2 S^2 I^* dt \nonumber \\ &+ c_1\sigma(I-I^*)S d\xi(t), \end{split} \end{equation} |
where \bar{L} = \frac{K}{M}(M-N) .
Define H = \mbox{min}\; \bigg\{\frac{b_S}{K}(S^*+1)+g_1 \bar{L}+\frac{b_I I^*}{K}+u_S-\left(\frac{3}{2}b_s+e+b_I\right), \frac{e}{K}(S^*+I^*)+\frac{(b_S+b_I) S^*}{K}+\frac{b_I}{K}I^*+g_2 \bar{L}+ \frac{c_1 b_I}{K}+u_I-\left(\frac{b_S}{2}+2(e+b_I)\right) \bigg\} . Integrating (4.7) from 0 to t , one gets
\begin{equation} \begin{split} G(t)-G(0) &\leq -H \int_0^t [(S(\tau)-S^*)^2+(I(\tau)-I^*)^2] d(\tau)+\sigma^2K^2\left(K^2+\frac{c_1}{2}I^*\right) t \nonumber\\ &+ c_1\sigma \int_0^t (I(\tau)-I^*)S(\tau) d\xi(\tau) \nonumber \end{split} \end{equation} |
\begin{equation} \label{final_stochastic_stability} \begin{split} \therefore \int_0^t [(S(\tau)-S^*)^2+(I(\tau)-I^*)^2] d(\tau) &\leq \frac{G(0)}{H}+ \frac{\sigma^2K^2\left(K^2+\frac{c_1}{2}I^*\right) t}{H} + \frac{c_1\sigma}{H} \int_0^t (I(\tau)-I^*)S(\tau) d\xi(\tau). \nonumber \end{split} \end{equation} |
Let N_1(t) = \int_0^t (I(\tau)-I^*)S(\tau) d\xi(\tau) , which is a continuous martingale and N_1(0) = 0 . Also, < N_1, N_1 > _t = \left(\int_0^t (I(\tau)-I^*)S(\tau) d\xi(\tau)\right)^2 = \int_0^t (I(\tau)-I^*)^2S^2(\tau) d(\tau) \leq 4 K^4 t and \limsup_{t \to \infty} \frac{ < N_1, N_1 > _t}{t}\leq 4K^4 < \infty \; \mbox{a.s} . Therefore, by the law of large numbers [35], \lim_{t \to \infty} \frac{N_1(t)}{t} = 0 \; \; \mbox{a.s} . Combining these results and then dividing (4.7) by t and taking limit superior, we have
\begin{equation} \begin{split} &\limsup\limits_{t\rightarrow \infty}\frac{1}{t} \int_0^t [(S(\tau)-S^*)^2+(I(\tau)-I^*)^2] d(\tau) \leq G_1 \sigma^2 \; \; \mbox{a.s.}, \; \mbox{where}\; \; G_1 = \frac{K^2\left(K^2+\frac{c_1}{2}I^*\right)}{H}. \end{split} \end{equation} | (4.7) |
Hence, the theorem is proven.
Furthermore, if \sigma \rightarrow 0 , then
\begin{equation} \limsup\limits_{t\rightarrow \infty}\frac{1}{t} \int_0^t [(S(\tau)-S^*)^2+(I(\tau)-I^*)^2] d(\tau) \rightarrow 0. \nonumber \end{equation} |
Therefore, \lim_{t\rightarrow \infty}S(t) \rightarrow S^*, \; \lim_{t\rightarrow \infty}I(t) \rightarrow I^* , and the stochastic solution tends to the deterministic equilibrium solution. This implies that if the noise intensity is low, the stochastic system behaves similarly to the asymptotic solution of the deterministic system, provided the restrictions in the above theorem hold.
In this part, we compute the exact expression of the density function of the stationary distribution. The S -compartment of deterministic model (2.1), under usual assumption of constant population S(t) + I(t) = N , reads
\begin{equation} \frac{dS}{dt} = b_S S \left(1-\frac{N}{K}\right) -u_S S - \beta S (N-S) + e(N-S)\left(1- \frac{N}{K}\right) + \mu(N - S). \end{equation} | (4.8) |
Under the stochastic perturbation of \beta to \beta + \sigma \xi(t) , the above equation becomes
\begin{equation} \frac{dS}{dt} = b_S S \left(1-\frac{N}{K}\right) -u_S S - \beta S (N-S) + e(N-S)\left(1- \frac{N}{K}\right) + \mu(N - S) - \sigma S (N - S) \xi(t). \end{equation} | (4.9) |
In the case of white noise perturbation with \xi(t) , the probability density function p(S, t) of S(t) is governed by the classic Fokker-Planck equation
\begin{equation} \frac{\partial p(S, t)}{dt} + \frac{\partial}{\partial S}[h(S) p(S, t)] = \frac{1}{2}\frac{\partial ^2}{\partial S^2}[\sigma^2(S) p(S, t)], \end{equation} | (4.10) |
where, h(S) = b_S S \left(1-\frac{N}{K}\right) -u_S S - \beta S (N-S) + e(N-S)\left(1- \frac{N}{K}\right) + \mu(N - S) and \sigma(S) = - \sigma S (N - S) . The closed-form stationary solution p_0(S) = \lim_{t \to \infty} p(S, t) of the Fokker-Planck equation is given by p_0(S) = \frac{\mathcal{N}}{\sigma ^2(S)} \mbox{exp}\left(2 \int_{}^{S}\frac{h(\omega)}{\sigma^2(\omega)}d\omega \right), where \mathcal{N} is the normalizing factor. Upon simplification, the closed-form expression is given by
\begin{equation} \begin{split} p_0(S) & = \frac{\mathcal{N}}{\sigma^2}\; e^{2\left(\frac{b_S \left(1 - \frac{N}{K}\right) - u_S}{\sigma^2 N(N- S)} - \frac{e \left(1 - \frac{N}{K}\right) + \mu}{\sigma^2 N S}\right)} \times S^ {\frac{2}{\sigma^2 N^2} \left\{(b_S- e) \left(1 - \frac{N}{K}\right) + (\mu - u_S - \beta N) - \sigma^2 N^2\right\}}\\ & \times (N- S)^{- \frac{2}{\sigma^2 N^2}\left\{\left(1 - \frac{N}{K}\right)(b_S + e) - (u_S - \mu + \beta N) + \sigma^2 N^2\right\}}. \end{split} \end{equation} | (4.11) |
For the I compartment, we similarly assume that q(I, t) is the pdf that is governed by the Fokker-Planck equation
\begin{equation} \frac{\partial q(I, t)}{dt} + \frac{\partial}{\partial I}[h'(I) q(I, t)] = \frac{1}{2}\frac{\partial ^2}{\partial I^2}[\sigma'^2(I) q(I, t)], \end{equation} | (4.12) |
where h'(I) = b_I I \left(1 - \frac{N}{K}\right) - u_I I + \beta (N - I) I - \mu I and \sigma'(I) = \sigma (N-I)I . The closed form stationary solution is given by
q_0(I) = \lim\limits_{t \to \infty} q(I, t), |
where
q_0(I) = \frac{\mathcal{N'}}{\sigma'^2(I)} \mbox{exp}\left(2 \int_{}^{I}\frac{h'(\omega)}{\sigma'^2(\omega)}d\omega \right), |
and \mathcal{N'} is the normalizing factor. Simplifying the above expression, we obtain
\begin{equation} \begin{split} q_0(I) & = \frac{\mathcal{N'}}{\sigma^2} e^{\frac{2}{\sigma^2 N (N - I)}\left\{b_I\left(1 - \frac{N}{K}\right) - u_I - \mu\right\}} \times I^{\frac{2}{\sigma^2N^2}\left\{b_I \left(1 - \frac{N}{K}\right) - u_I - \mu + \beta N - \sigma^2 N^2\right\}} \times \\ & (N - I)^{\frac{2}{\sigma^2N^2}\left\{u_I + \mu + \sigma^2 N^2 - \beta N - b_I\left(1 - \frac{N}{K}\right)\right\}}. \end{split} \end{equation} | (4.13) |
We present simulation results of stochastic system (2.2). The following system parameters are considered fixed unless it is stated: b_S = 0.2, \; b_I = 0.03, u_S = 0.1, \; u_I = 0.15, \; e = 0.02, \; {K = 1}, \; \beta = 0.5, \; \mbox{and}\; \mu = 0.1 \; [9]. Without loss of generality, we set K = 1 , effectively selecting an appropriate spatial unit for measuring population densities, as outlined in Lipsitch et al. [9]. The initial value is assumed to be (S(0), I(0)) = (0.6, 0.3).
The time evolution of a stochastic system is different for each run. However, that of the deterministic system is unique for a given parameter set. Thus, due to inherent randomness, a considerable difference may exist between the solutions of the stochastic system for different runs. To illustrate this, we have presented 10 simulation results for two distinct noises in the first two rows of Figure 1. The first column shows that the disease persists for low values of the noise ( \sigma = 0.4 ) when the parameter set satisfies the conditions of Theorem 4 with \frac{b_I-u_I-\mu}{\frac{b_I}{K}+\frac{A(b_I+e)}{b_S}-\frac{A u_I}{b_S}} = 22 > 0 . The second column illustrates that the disease dies out for higher values of the noise ( \sigma = 0.8 ) when the parameter set satisfies the conditions provided by Theorem 3 with R_0^S = 0.9012 < 1 . The solution trajectories here differ in each simulation, though the initial condition and parameter values remain the same. It is, therefore, more justified to present the average behavior of the stochastic solutions. In the last row, we have given the mean value of 100 solutions of stochastic system (2.2) for \sigma = 0.4 ((a), (b), (e)) and \sigma = 0.8 ((c), (d), (f)), which show similar behaviors.
It is worth mentioning that the noise-induced stochastic system (2.2) has no steady-state value. In Theorem 5, we have proven that the stochastic and deterministic systems behave similarly if the noise intensity is low. However, if the noise intensity is high, the stochastic solution largely deviates from its deterministic counterpart. We illustrate such behavior of the system in Figure 2 for two noises: \sigma = 0.05 and \sigma = 0.2 . The conditions of the stationary distribution (Theorem 5) are satisfied here.
We further examine the probabilistic smoke cloud around the deterministic steady state. We repeat the simulation 10,000 times and plot the values of S and I populations at t = 120 in Figure 3. This figure shows that species densities are primarily concentrated in the neighbourhood of deterministic concentrated in the neighborhood of deterministic equilibrium value (S^*, I^*) = (0.47, 0.014) (blue point) if the noise intensity is low (\sigma = 0.05) . Observe that the frequency distribution of the susceptible population ( S ) is distributed in the range 0.45-0.48 around its equilibrium value S^* = 0.47 (Figure 3(b)), and the same for the infected population ( I ) is distributed in the range 0.005-0.025 around its equilibrium value I^* = 0.014 (Figure 3(c)). This shows the strength of the stabilizing factors of the population interaction compared to the diffusive effects of the random environmental fluctuations [36].
Indeed, the probability cloud is compact if the interaction strength can overcome the noise. However, if the interaction strength is weak compared to the intensity of the environmental variance, the cloud covers a larger area (Figure 3(d)) if the noise intensity is high (\sigma = 0.15) . The corresponding frequency distributions (Figures 3(e), (f)) show that S and I are distributed over a more extensive range of 0.41-0.5 and 0.002-0.045 , respectively, around their deterministic equilibrium values. This reveals that the stochastic system does not deviate too much from its deterministic system if the noise is low, but it does if it is high.
We further examined the evolution of histograms of S and I populations, as an approximation of their probability density functions. We repeat the simulation 50,000 times to plot the histograms. As time progresses, one can observe a shift in the mean and spread of both S(t) and I(t) . At time t = 20 , the distribution of S(t) is centered around 0.31 and I(t) around 0.07, indicating that a smaller proportion of the population is infected (Figure 4(a)). At t = 40 , the distribution is shifted to around 0.40, and I(t) has a peak around 0.02. This may indicate an increase in the susceptible individuals as the infected population temporarily decreases, possibly due to recovery or a reduction in transmission (Figure 4(b)). At t = 80 , S(t) has a mean near 0.46, and I(t) near 0.01, with both distributions showing a greater variability (Figure 4(d). As time progresses, S(t) tends to fluctuate near 0.48 and I(t) near 0.01, which indicates that the system is approaching a stationary distribution, even though individual realizations of S(t) and I(t) still experience random fluctuations. This is useful for analyzing long-term behavior in stochastic models, as it suggests that the system has an average state around that it oscillates due to random environmental noise.
It is observed that the noise intensity ( \sigma ) and the disease transmission rate ( \beta ) play a pivotal role in the extinction and persistence of the disease. We present, in Figure 5(a), the mean extinction time of the infected population of the stochastic system (2.2) with the variations in \beta and \sigma . To find the extinction time numerically, we repeat the simulation 100 times for each pair of ( \beta , \sigma ) and plot the mean of the extinction time of 100 simulations. The infected population is considered extinct if it goes below 0.0001 . Moreover, R^S_0 < 1 is satisfied throughout the considered range of \beta and \sigma . Figure 5(a) shows that the extinction time is longer if \beta is high and \sigma is low. However, the extinction time decreases if \beta is low or \sigma is high. We also investigate how disease extinction time is influenced by the parameter variations in \beta and b_I . We select four values of \sigma , namely, 0.2, \; 0.4, \; 0.6, \; 0.8 . For each of these \sigma values, we jointly vary \beta and b_I and record the mean extinction time for 100 simulations (see Figure 5(b)–(e)). It has been observed that disease extinction takes a shorter time if both \beta and b_I are low. However, disease extinction time is prolonged for higher values of \beta and b_I . We further observe that when \sigma is low, disease extinction time in the whole parametric space of \beta and b_I is high compared to the higher value of \sigma . This reveals that stronger environmental noise helps eradicate the disease from the system early, implying that it may act as a regulatory mechanism to control the disease.
To unveil the underlying law of extinction time, we plot the mean of extinction time of 100 simulations with the variation of \sigma for three particular values of \beta : 0.1, 0.2 , and 0.3 (Figure 6(a)). Mean extinction time gradually decreases as \sigma increases. An exponential curve fits it. A similar figure was drawn with three fixed values of \sigma , 0.2, 0.5 , and 0.8 , for the variation of \beta (Figure 6(b)). In the latter case, the disease extinction time gradually increases with the increase of the disease transmission coefficient. The mean extinction time fits the positive exponential curve.
It is shown that R^S_0 is a decreasing function of the recovery rate, \mu . Here, we explore the disease extinction scenario for \mu for different values of \sigma and \beta . Figure 7 shows that the extinction time curves have two distinct natures for the variations in \mu . If \sigma is much higher than \beta (cyan curve, bottom), the extinction time follows the negative exponential law. As the difference between \sigma and \beta decreases while \sigma remains higher (purple and green curves), the extinction time increases. On the other hand, if \sigma and \beta are both elevated and nearly equal in magnitude, the extinction time becomes substantially larger, exhibiting a slower rate of decline with respect to \mu . All these have a long tail, implying that a shorter extinction time of the disease is possible for an extended range of \mu .
As mentioned earlier, parasite infectivity and the relative fecundity of infected hosts are critical for parasite fitness. The survival fitness of parasites depends on how long the infected hosts persist in the system. For this, we plot (Figure 8) the extinction time of infected hosts from the system for the simultaneous variations in \beta and \frac{b_I+e}{b_S} . Recall the ensemble fecundity of the infected population as b_{I}+e , and that of the susceptible as b_S . The ratio \frac{b_I+e}{b_S} denotes the relative fecundity of the infected host population. By our assumption, b_S\geq b_I+e and therefore, the ratio \frac{b_I+e}{b_S} is always less than or equal to 1 . If the force of infection is not too high ( \beta < 0.6 ), then the infection eradication time increases with the increasing relative fecundity of the infected host for some fixed value of noise. However, for the higher force of infection ( \beta > 0.6 ), the relative fecundity of infected hosts has a negligible effect on extinction time. The trend remains the same if the noise intensity is altered. Thus, the parasite fitness is low if the relative fecundity and disease transmissibility are low but gradually increase if any of them increase.
To assess how variations in parameters control disease transmission and progression with pseudo-recovery, a sensitivity analysis of model (2.1) is conducted numerically, following the approach outlined in [37,38]. The normalized forward-sensitivity index of a variable, v , that depends differentiably on a parameter, p , is defined as
\Upsilon_p^v = \frac{\partial v}{\partial p} \times \frac{p}{v}. |
We numerically evaluate the local time-dependent normalized sensitivity index for the variables S and I . The positive sensitivity of S with respect to \mu and negative sensitivity of I is expected, as the higher recovery rate would naturally shift more individuals from the infected state back to the susceptible state. The oscillatory behavior here might be due to the model's feedback between S and I , where changes in recovery (through \mu ) indirectly affect S and I by influencing the rate at which individuals transition between susceptible and infected states.
In a sensitivity analysis based on variance, such as the Sobol method, the total variance in the model output is decomposed to attribute portions of this variance to different input parameters [39]. Let Y represent the model output (either S or I ) and \theta be a vector of model parameters ( \beta, b_i, b_s, e, K, \mu, u_i, u_s ). The variance of Y , \mathrm{Var}(Y) , can be decomposed as:
\mathrm{Var}(Y) = \sum\limits_i \mathrm{Var}_{\theta_i}(Y) + \sum\limits_{i < j} \mathrm{Var}_{\theta_i, \theta_j}(Y) + \cdots + \mathrm{Var}_{\theta_1, \ldots, \theta_n}(Y), |
where \mathrm{Var}_{\theta_i}(Y) is the partial variance due to parameter \theta_i alone, while \mathrm{Var}_{\theta_i, \theta_j}(Y) represents the variance due to the interaction between \theta_i and \theta_j , and so on. The first-order Sobol index S_i for each parameter \theta_i is defined as
S_i = \frac{\mathrm{Var}_{\theta_i}(Y)}{\mathrm{Var}(Y)}. |
This index quantifies the direct effect of parameter \theta_i on Y .
In the above pie chart, each segment represents the proportion of first-order variance in S or I . A larger segment implies a higher influence of that parameter on the respective model output. In the first-order Sobol index of S , we observe that the parameters \beta and u_S strongly influence the variance of S , indicating that variation in these parameters significantly affects the susceptible population. In the right panel, u_S and u_I have the highest impact on the infected population.
Here, we present a case study for the imperfect vertical and horizontal infection transmission that supports some of our observed theoretical results of the deterministic and stochastic systems. The parasite Holospora Undulata frequently infects the protozoa Paramecium caudatum and uses both the horizontal and vertical transmission pathways [40]. P. caudatum are filter feeders and ingest infectious forms of Holospora Undulata while taking food from the water. Lunn et al. [41] performed a microcosm study, where the 34 days time series data of P. caudatum and its specialist bacterial parasite Holospora Undulata under different nutrient concentrations (high and low) have been investigated. We fitted our SIS deterministic and stochastic models with these 34 days of experimental data corresponding to high nutrient concentrations following the optimization technique presented in Appendix Ⅰ. During the estimation of the parameter set, we maintain the model assumptions u_I\geq u_S and b_S\geq b_I+e . The experimental values are plotted on a logarithmic scale for ease of fitting. The best-fit parameter set thus obtained by fitting the stochastic system (2.2) is as follows:
b_S = 1.5, \; K = 35.9, \; u_S = 0.16, \; \beta = 0.18, \; e = 0.0026, \; \mu = 0.7, \; b_I = 0.11, \; u_I = 0.77, \; \mbox{and}\; \sigma = 0.035. |
The deterministic basic reproduction number R_0^{(1)} for this parameter set is 4.1801 , implying the disease is persistent. The average value (solid line) of 1000 solutions of the stochastic system (2.2) with the above parameter set has been plotted in Figure 11 with respect to time (day) with 95\% confidence interval (CI). This shows that the stochastic solution matches the experimental data except at some initial data points.
Mathematical models of epidemics have helped understand the disease spread in a population. After the benchmark work of Kermack and McKendrick [42], many mathematical models have been proposed and analyzed, considering the epidemiological demands. Most of these models are deterministic types represented by ordinary differential equations. Deterministic models have been criticized for viewing all the rate parameters as constant, even though these constants constantly fluctuate due to the various unknown environmental noises. Uncertainty is an integral part of modeling biological phenomena due to the complexity and lack of knowledge of microscopic events involved in the system. In particular, the disease transmission phenomena are random, and the transmission rate depends on various factors. Incorporating such randomness in a model leads to stochastic differential equation models.
In this paper, we consider an SIS-type stochastic epidemic model, where the disease is transmitted through horizontal and vertical transmission modes. To incorporate stochasticity in the model, we introduce a white noise in the horizontal disease transmission term, the predominant disease transmission mechanism. The fluctuation in the transmission rate usually occurs around some mean value. Therefore, the error term follows a normal distribution, enabling us to approximate it by a white noise [43].
Eradication or control of various emerging and reemerging diseases is a global challenge. Environmental factors like temperature, humidity, rainfall, and pollution significantly affect disease persistence. Knowing the different routes for theoretically eradicating an infection from the population is essential. Our mathematical analysis revealed that the population can be disease-free by modulating some parameters and the noise intensity. We have shown that if the stochastic basic reproduction number, R^S_0 , can be made less than unity, the disease can be eradicated from the system. It is observed that noise intensity plays a pivotal role in eliminating and has an inverse relationship with R^S_0 . The environmental noise can make a system disease-free if it significantly affects the transmission mechanism and makes R^S_0 < 1 . On the contrary, R^S_0 is directly proportional to the disease transmission coefficient. Thus, R^S_0 may exceed the threshold value if the disease transmissibility increases. Therefore, the management strategy should be to reduce horizontal disease transmissibility. Our numerical computations revealed that the disease eradication time follows a negative exponential law with increasing noise intensity. In contrast, the disease transmission coefficient follows the positive exponential law.
Furthermore, disease eradication is also possible with respect to controllable parameter \mu . This parameter measures the recovery rate of the infected population and can be used in the disease eradication process. Moreover, an infected host may recover from the infection due to its immune mechanism. Recovery of infected hosts is also possible with the help of some external measures. Extinction time follows either a negative exponential or Gaussian law for some given noise and disease transmissibility values. In the former case, the noise must be high, or the transmission coefficient must be low. In the latter case, the effects of noise and disease transmissibility should be reversed. The eradication time will be significantly quicker if the recovery rate is high. On the other hand, disease management would be more challenging if the noise intensity is low and the disease transmissibility is high.
Several researchers have demonstrated that parasites have a detrimental effect on the host's fecundity. The survival of parasites depends on the infected host density and the extinction time of the infected host population. Thus, parasites are expected to evolve towards higher relative fecundity of the infected host to enhance fitness. Our simulation result for simultaneous variation of the disease transmission coefficient and relative fecundity rate shows that parasite fitness increases with increasing relative fecundity of parasites when the transmissibility is low. The relative fecundity of infected hosts, however, has a negligible effect on the extinction time of the infected host population and, consequently, on the parasite survival fitness if the disease transmissibility of parasites is high. A similar trend is also maintained if the noise intensity is altered.
We utilizie a susceptible-infected-susceptible framework, which is appropriate for diseases where recovered individuals do not gain permanent immunity and can reenter the susceptible population. However, many infectious diseases exhibit immunity following infection, aligning more closely with the SIR or SIRS model frameworks, where recovered individuals gain immunity before potentially returning to the susceptible class. Integrating stochastic effects and vertical transmission in these models could reveal important nuances in epidemic behavior under varying immunity dynamics with the increasing complexity of mathematical treatment [16,44,45]. One of the central limitations of the white noise perturbation is that increasing the white noise leads to quicker disease extinction, which is typically not observed in real-world epidemics. Therefore, the white noise model can underestimate the severity of disease transmissions [46,47]. This is also counterintuitive because the increase of noise in the transmission parameter can be a relaxation of the restrictions of contact among species. Thus, the increased noise that could lead to rapid disease eradication might be mathematically correct, but the real-world implications of such a conclusion must be carefully examined. However, temporally correlated noise (modeled via the Ornstein-Uhlenbeck process) introduces more realistic fluctuations in transmission rate and does not necessarily foster disease extinction due to increased stochastic noise [48,49].
The authors declare they have not used Artificial Intelligence (AI) tools in the creation of this article.
This work is founded by the Indo-French Centre For Applied Mathematics (IFCAM), Ref No. MA/IFCAM/18/49.
The authors declare there is no conflicts of interest.
Deterministic model parameters were estimated from the experimental data set through the nonlinear least square technique. To begin, we considered an arbitrary parameter set {\Lambda} = (\Lambda_1, \Lambda_2, ...., \Lambda_n) and then minimized the sum of squared differences between the experimental data Y = (y_1, y_2, ..., y_n) and the corresponding model solution data. We used lsqcurvefit, an optimization toolbox from Matlab, to solve the nonlinear least square problem. Lsqcurvefit fits a function to a set of data points using the Levenberg-Marquardt (LM) algorithm, which is an iterative process used to find the minimum of the sum of squares of the nonlinear function [50]. It can be understood as an amalgamation of steepest descent and Gauss-Newton methods [51]. The assumed objective function is defined as
J = \mbox{argmin} \sum\limits_{j = 1}^{n}\left(g(t_j, \Lambda)-y_j\right)^2, |
where g(t_j, \Lambda) is the model solution at time step t_j at which the solution is to be estimated, and n is the total number of data points. The optimization process will provide the best fit solution g(t_j, \tilde{\Lambda}) to the experimental data corresponding to the optimal parameter set \tilde{\Lambda} = (\tilde {\Lambda}_1, \tilde{\Lambda}_2, ...., \tilde {\Lambda}_n) , which should satisfy the model's basic assumptions. After estimating parameters for the deterministic system, we estimated the noise intensity for the stochastic system to obtain a good agreement between the output and the experimental data. We computed the corresponding r -squared value for stochastic simulation data and experimental data to find the suitable noise strength. Here, we considered 10,000 different random values of the noise intensity \sigma between 0 and 1 through Latin hypercube sampling. Then, for each of these 10,000 values of \sigma , the stochastic system was simulated 100 times. Taking mean of the 100 simulations, the r- squared was computed between the average stochastic simulation output and the experimental data. Noise intensity was selected for which the r -squared was maximum.
[1] |
R. M. Anderson, R. M. May, The population dynamics of microparasites and their invertebrate hosts, Philos. Trans. R. Soc. B Biol. Sci., 291 (1981), 451–524. https://doi.org/10.1098/rstb.1981.0005 doi: 10.1098/rstb.1981.0005
![]() |
[2] |
M. Lipsitch, M. A. Nowak, D. Ebert, R. M. May, The population dynamics of vertically and horizontally transmitted parasites, Proc. R. Soc. B, 260 (1995), 321–327. https://doi.org/10.1098/rspb.1995.0099 doi: 10.1098/rspb.1995.0099
![]() |
[3] |
A. M. Dunn, J. E. Smith, Microsporidian life cycles and diversity: The relationship between virulence and transmission, Microbes Infect., 3 (2001), 381–388. https://doi.org/10.1016/S1286-4579(01)01394-6 doi: 10.1016/S1286-4579(01)01394-6
![]() |
[4] |
R. Fayer, Effect of high temperature on infectivity of Cryptosporidium parvum oocysts in water, Appl. Environ. Microbiol., 60 (1994), 2732–2735. https://doi.org/10.1128/aem.60.8.2732-2735.1994 doi: 10.1128/aem.60.8.2732-2735.1994
![]() |
[5] | F. Memarzadeh, Literature review of the effect of temperature and humidity on viruses, ASHRAE Trans., 118 (2012), 1049–1060. |
[6] |
A. Gray, D. Greenhalgh, L. Hu, X. Mao, J. Pan, A stochastic differential equation SIS epidemic model, SIAM J. Appl. Math., 71 (2011), 876–902. https://doi.org/10.1137/10081856X doi: 10.1137/10081856X
![]() |
[7] |
Y. Zhao, D. Jiang, The threshold of a stochastic SIRS epidemic model with saturated incidence, Appl. Math. Lett., 34 (2014), 90–93. https://doi.org/10.1016/j.aml.2013.11.002 doi: 10.1016/j.aml.2013.11.002
![]() |
[8] |
A. Majumder, D. Adak, N. Bairagi, Phytoplankton-zooplankton interaction under environmental stochasticity: Survival, extinction and stability, Appl. Math. Model., 89 (2021), 1382–1404. https://doi.org/10.1016/j.apm.2020.06.076 doi: 10.1016/j.apm.2020.06.076
![]() |
[9] |
M. Lipsitch, S. Siller, M. A. Nowak, The evolution of virulence in pathogens with vertical and horizontal transmission, Evolution, 50 (1996), 1729–1741. https://doi.org/10.1111/j.1558-5646.1996.tb03560.x doi: 10.1111/j.1558-5646.1996.tb03560.x
![]() |
[10] |
Y. Chen, J. Evans, M. Feldlaufer, Horizontal and vertical transmission of viruses in the honey bee, Apis mellifera, J. Invertebr. Pathol., 92 (2006), 152–159. https://doi.org/10.1016/j.jip.2006.03.010 doi: 10.1016/j.jip.2006.03.010
![]() |
[11] |
D. H. Clayton, D. M. Tompkins, Ectoparasite virulence is linked to mode of transmission, Proc. R. Soc. B, 256 (1994), 211–217. https://doi.org/10.1098/rspb.1994.0072 doi: 10.1098/rspb.1994.0072
![]() |
[12] | P. W. Ewald, Evolution of Infectious Disease, Oxford University Press on Demand, 1994. |
[13] |
J. Antonovics, A. J. Wilson, M. R. Forbes, H. C. Hauffe, E. R. Kallio, H. C. Leggett, et al., The evolution of transmission mode, Philos. Trans. R. Soc. B Biol. Sci., 372 (2017), 20160083. https://doi.org/10.1098/rstb.2016.0083 doi: 10.1098/rstb.2016.0083
![]() |
[14] |
N. Gao, Y. Song, X. Wang, J. Liu, Dynamics of a stochastic SIS epidemic model with nonlinear incidence rates, Adv. Contin. Discrete Models, 41 (2019), 1–19. https://doi.org/10.1186/s13662-019-1980-0 doi: 10.1186/s13662-019-1980-0
![]() |
[15] |
G. Lan, Y. Huang, C. Wei, S. Zhang, A stochastic SIS epidemic model with saturating contact rate, Phys. A Stat. Mech. Appl., 529 (2019), 121504. https://doi.org/10.1016/j.physa.2019.121504 doi: 10.1016/j.physa.2019.121504
![]() |
[16] |
A. Miao, T. Zhang, J. Zhang, C. Wang, Dynamics of a stochastic SIR model with both horizontal and vertical transmission, J. Appl. Anal. Comput., 8 (2018), 1108–1121. https://doi.org/10.11948/2018.1108 doi: 10.11948/2018.1108
![]() |
[17] |
D. Li, J. Cui, M. Liu, S. Liu, The evolutionary dynamics of stochastic epidemic model with nonlinear incidence rate, Bull. Math. Biol., 77 (2015), 1705–1743. https://doi.org/article/10.1007/s11538-015-0101-9 doi: 10.1007/s11538-015-0101-9
![]() |
[18] |
K. Mangin, M. Lipsitch, D. Ebert, Virulence and transmission modes of two microsporidia in Daphnia magna, Parasitology, 111 (2009), 133–142. https://doi.org/10.1017/S0031182000064878 doi: 10.1017/S0031182000064878
![]() |
[19] |
D. Ebert, M. Lipsitch, K. L. Mangin, The effect of parasites on host population density and extinction: Experimental epidemiology with Daphnia and six microparasites, Am. Nat., 156 (2000), 459–477. https://doi.org/10.1086/303404 doi: 10.1086/303404
![]() |
[20] |
R. E. Sorensen, D. J. Minchella, Parasite influences on host life history Echinostoma revolutum parasitism of Lymnaea elodes snails, Oecologia, 115 (1998), 188–195. https://doi.org/10.1007/s004420050507 doi: 10.1007/s004420050507
![]() |
[21] |
D. Tompkins, M. Begon, Parasites can regulate wildlife populations, Trends Parasitol., 15 (1999), 311–313. https://doi.org/10.1016/s0169-4758(99)01484-2 doi: 10.1016/s0169-4758(99)01484-2
![]() |
[22] |
J. C. HOLMES, Modification of intermediate host behaviour by parasites, Behav. Asp. Parasite Transm., (1972), 123–149. https://doi.org/10.5555/19730804260 doi: 10.5555/19730804260
![]() |
[23] |
K. D. Lafferty, A. K. Morris, Altered behavior of parasitized killifish increases susceptibility to predation by bird final hosts, Ecology, 77 (1996), 1390–1397. https://doi.org/10.2307/2265536 doi: 10.2307/2265536
![]() |
[24] | P. Saha, N. Bairagi, Dynamics of vertically and horizontally transmitted parasites: Continuous vs discrete models, preprint, arXiv: 1906.03026. https://doi.org/10.48550/arXiv.1906.03026 |
[25] |
N. C. Grassly, C. Fraser, Mathematical models of infectious disease transmission, Nat. Rev. Microbiol., 6 (2008), 477–487. https://doi.org/10.1038/nrmicro1845 doi: 10.1038/nrmicro1845
![]() |
[26] | A. S. Mikhailov, A. Y. Loskutov, Foundations of Synergetics II: Complex Patterns, Springer Nature, 2012. https://link.springer.com/book/10.1007/978-3-642-80196-9 |
[27] | R. M. Anderson, B. Anderson, R. M. May, Infectious Diseases of Humans: Dynamics and Control, Oxford Academic, 1991. https://doi.org/10.1093/oso/9780198545996.001.0001 |
[28] |
P. Van den Driessche, J. Watmough, Reproduction numbers and sub-threshold endemic equilibria for compartmental models of disease transmission, Math. Biosci., 180 (2002), 29–48. https://doi.org/10.1016/s0025-5564(02)00108-6 doi: 10.1016/s0025-5564(02)00108-6
![]() |
[29] |
N. Bairagi, D. Adak, Complex dynamics of a predator–prey–parasite system: An interplay among infection rate, predator's reproductive gain and preference, Ecol. Complex., 22 (2015), 1–12. https://doi.org/10.1016/j.ecocom.2015.01.002 doi: 10.1016/j.ecocom.2015.01.002
![]() |
[30] | M. Kot, Elements of Mathematical Ecology, Cambridge University Press, 2001. https://doi.org/10.1017/CBO9780511608520 |
[31] |
D. Valenti, A. Fiasconaro, B. Spagnolo, Stochastic resonance and noise delayed extinction in a model of two competing species, Phys. A Stat. Mech. Appl., 331 (2004), 477–486. https://doi.org/10.1016/j.physa.2003.09.036 doi: 10.1016/j.physa.2003.09.036
![]() |
[32] | X. Mao, Stochastic Differential Equations and Applications, Elsevier, 2007. |
[33] |
M. Liu, K. Wang, Persistence and extinction in stochastic non-autonomous logistic systems, J. Math. Anal. Appl., 375 (2011), 443–457. https://doi.org/10.1016/j.jmaa.2010.09.058 doi: 10.1016/j.jmaa.2010.09.058
![]() |
[34] |
C. Ji, D. Jiang, Threshold behaviour of a stochastic SIR model, Appl. Math. Model., 38 (2014), 5067–5079. https://doi.org/10.1016/j.apm.2014.03.037 doi: 10.1016/j.apm.2014.03.037
![]() |
[35] |
V. V. Petrov, On the strong law of large numbers, Theory Probab. Appl., 14 (1969), 183–192. https://doi.org/10.1137/1114027 doi: 10.1137/1114027
![]() |
[36] | R. M. May, Stability and Complexity in Model Ecosystems, Princeton University Press, 2019. https://doi.org/10.2307/j.ctvs32rq4 |
[37] |
S. Olaniyi, O. S. Obabiyi, Qualitative analysis of malaria dynamics with nonlinear incidence function, Appl. Math. Sci., 8 (2014), 3889–3904. http://dx.doi.org/10.12988/ams.2014.45326 doi: 10.12988/ams.2014.45326
![]() |
[38] | S. Mushayabasa, C. Bhunu, Modeling HIV transmission dynamics among male prisoners in sub-saharan africa, Int. J. Appl. Math., 41 (2011). |
[39] |
I. M. Sobol, Global sensitivity indices for nonlinear mathematical models and their monte carlo estimates, Math. Comput. Simul., 55 (2001), 271–280. https://doi.org/10.1016/S0378-4754(00)00270-6 doi: 10.1016/S0378-4754(00)00270-6
![]() |
[40] |
O. Kaltz, J. C. Koella, Host growth conditions regulate the plasticity of horizontal and vertical transmission in Holospora undulata, a bacterial parasite of the protozoan paramecium caudatum, Evolution, 57 (2003), 1535–1542. https://doi.org/10.1111/j.0014-3820.2003.tb00361.x doi: 10.1111/j.0014-3820.2003.tb00361.x
![]() |
[41] |
D. Lunn, R. J. Goudie, C. Wei, O. Kaltz, O. Restif, Modelling the dynamics of an experimental host-pathogen microcosm within a hierarchical Bayesian framework, PLOS One, 8 (2013), e69775. https://doi.org/10.1371/journal.pone.0069775 doi: 10.1371/journal.pone.0069775
![]() |
[42] |
W. O. Kermack, A. G. McKendrick, A contribution to the mathematical theory of epidemics, Proc. R. Soc. Lond. A, 115 (1927), 700–721. https://doi.org/10.1098/rspa.1927.0118 doi: 10.1098/rspa.1927.0118
![]() |
[43] |
M. Liu, K. Wang, Global stability of a nonlinear stochastic predator-prey system with Beddington-DeAngelis functional response, Commun. Nonlinear Sci. Numer. Simul., 16 (2011), 1114–1121. https://doi.org/10.1016/j.cnsns.2010.06.015 doi: 10.1016/j.cnsns.2010.06.015
![]() |
[44] |
S. Liu, Y. Pei, C. Li, L. Chen, Three kinds of TVS in a SIR epidemic model with saturated infectious force and vertical transmission, Appl. Math. Model., 33 (2009), 1923–1932. https://doi.org/10.1016/j.apm.2008.05.001 doi: 10.1016/j.apm.2008.05.001
![]() |
[45] | J. Tanimoto, Sociophysics Approach to Epidemics, Springer, 23 (2021). https://link.springer.com/book/10.1007/978-981-33-6481-3 |
[46] |
B. Zhou, D. Jiang, B. Han, T. Hayat, Threshold dynamics and density function of a stochastic epidemic model with media coverage and mean-reverting Ornstein-Uhlenbeck process, Math. Comput. Simul., 196 (2022), 15–44. https://doi.org/10.1016/j.matcom.2022.01.014 doi: 10.1016/j.matcom.2022.01.014
![]() |
[47] | E. Allen, Environmental variability and mean-reverting processes, Discrete Contin. Dyn. Syst. Ser. B, 21 (2016), 2073–2089. https://www.aimsciences.org/article/doi/10.3934/dcdsb.2016037 |
[48] |
K. Mamis, M. Farazmand, Stochastic compartmental models of the Covid-19 pandemic must have temporally correlated uncertainties, Proc. R. Soc. A, 479 (2023), 20220568. https://doi.org/10.1098/rspa.2022.0568 doi: 10.1098/rspa.2022.0568
![]() |
[49] |
K. Mamis, M. Farazmand, Modeling correlated uncertainties in stochastic compartmental models, Math. Biosci., 374 (2024), 109226. https://doi.org/10.1016/j.mbs.2024.109226 doi: 10.1016/j.mbs.2024.109226
![]() |
[50] | J. Nocedal, S. J. Wright, Numerical optimization, 2nd edition, Springer, New York, 2006. |
[51] | M. I. Lourakis, A brief description of the Levenberg-Marquardt algorithm implemented by levmar, Foundation Res. Technol., 4 (2005), 1–6. http://www.ics.forth.gr/lourakis/levmar |