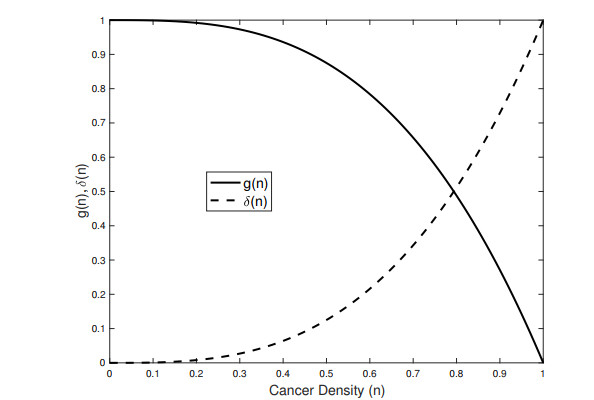
In this paper, we propose a stochastic SIHR epidemic model of COVID-19. A basic reproduction number Rs0 is defined to determine the extinction or persistence of the disease. If Rs0<1, the disease will be extinct. If Rs0>1, the disease will be strongly stochastically permanent. Based on realistic parameters of COVID-19, we numerically analyze the effect of key parameters such as transmission rate, confirmation rate and noise intensity on the dynamics of disease transmission and obtain sensitivity indices of some parameters on Rs0 by sensitivity analysis. It is found that: 1) The threshold level of deterministic model is overestimated in case of neglecting the effect of environmental noise; 2) The decrease of transmission rate and the increase of confirmed rate are beneficial to control the spread of COVID-19. Moreover, our sensitivity analysis indicates that the parameters β, σ and δ have significantly effects on Rs0.
Citation: Tianfang Hou, Guijie Lan, Sanling Yuan, Tonghua Zhang. Threshold dynamics of a stochastic SIHR epidemic model of COVID-19 with general population-size dependent contact rate[J]. Mathematical Biosciences and Engineering, 2022, 19(4): 4217-4236. doi: 10.3934/mbe.2022195
[1] | Tiberiu Harko, Man Kwong Mak . Travelling wave solutions of the reaction-diffusion mathematical model of glioblastoma growth: An Abel equation based approach. Mathematical Biosciences and Engineering, 2015, 12(1): 41-69. doi: 10.3934/mbe.2015.12.41 |
[2] | Ardak Kashkynbayev, Yerlan Amanbek, Bibinur Shupeyeva, Yang Kuang . Existence of traveling wave solutions to data-driven glioblastoma multiforme growth models with density-dependent diffusion. Mathematical Biosciences and Engineering, 2020, 17(6): 7234-7247. doi: 10.3934/mbe.2020371 |
[3] | Lifeng Han, Steffen Eikenberry, Changhan He, Lauren Johnson, Mark C. Preul, Eric J. Kostelich, Yang Kuang . Patient-specific parameter estimates of glioblastoma multiforme growth dynamics from a model with explicit birth and death rates. Mathematical Biosciences and Engineering, 2019, 16(5): 5307-5323. doi: 10.3934/mbe.2019265 |
[4] | Tracy L. Stepien, Erica M. Rutter, Yang Kuang . A data-motivated density-dependent diffusion model of in vitro glioblastoma growth. Mathematical Biosciences and Engineering, 2015, 12(6): 1157-1172. doi: 10.3934/mbe.2015.12.1157 |
[5] | Zijuan Wen, Meng Fan, Asim M. Asiri, Ebraheem O. Alzahrani, Mohamed M. El-Dessoky, Yang Kuang . Global existence and uniqueness of classical solutions for a generalized quasilinear parabolic equation with application to a glioblastoma growth model. Mathematical Biosciences and Engineering, 2017, 14(2): 407-420. doi: 10.3934/mbe.2017025 |
[6] | Leibo Wang, Xuebin Zhang, Jun Liu, Qingjun Liu . RETRACTED ARTICLE: Kinesin family member 15 can promote the proliferation of glioblastoma. Mathematical Biosciences and Engineering, 2022, 19(8): 8259-8272. doi: 10.3934/mbe.2022384 |
[7] | Benxin Zhang, Xiaolong Wang, Yi Li, Zhibin Zhu . A new difference of anisotropic and isotropic total variation regularization method for image restoration. Mathematical Biosciences and Engineering, 2023, 20(8): 14777-14792. doi: 10.3934/mbe.2023661 |
[8] | Margherita Carletti, Matteo Montani, Valentina Meschini, Marzia Bianchi, Lucia Radici . Stochastic modelling of PTEN regulation in brain tumors: A model for glioblastoma multiforme. Mathematical Biosciences and Engineering, 2015, 12(5): 965-981. doi: 10.3934/mbe.2015.12.965 |
[9] | Chichia Chiu, Jui-Ling Yu . An optimal adaptive time-stepping scheme for solving reaction-diffusion-chemotaxis systems. Mathematical Biosciences and Engineering, 2007, 4(2): 187-203. doi: 10.3934/mbe.2007.4.187 |
[10] | Christopher E. Elmer . The stability of stationary fronts for a discrete nerve axon model. Mathematical Biosciences and Engineering, 2007, 4(1): 113-129. doi: 10.3934/mbe.2007.4.113 |
In this paper, we propose a stochastic SIHR epidemic model of COVID-19. A basic reproduction number Rs0 is defined to determine the extinction or persistence of the disease. If Rs0<1, the disease will be extinct. If Rs0>1, the disease will be strongly stochastically permanent. Based on realistic parameters of COVID-19, we numerically analyze the effect of key parameters such as transmission rate, confirmation rate and noise intensity on the dynamics of disease transmission and obtain sensitivity indices of some parameters on Rs0 by sensitivity analysis. It is found that: 1) The threshold level of deterministic model is overestimated in case of neglecting the effect of environmental noise; 2) The decrease of transmission rate and the increase of confirmed rate are beneficial to control the spread of COVID-19. Moreover, our sensitivity analysis indicates that the parameters β, σ and δ have significantly effects on Rs0.
Glioblastoma multiforme (GBM) is the most common and aggressive form of primary brain cancer, with roughly 12{, }000 cases diagnosed yearly in the United States [1]. Median survival in the U.S. hovers around one year [1] and is not likely to exceed 15 months even with aggressive therapy in clinical trials [2]. The standard of care for treatment at diagnosis was largely established by the seminal 2005 trial by Stupp et al. [2] and consists of maximal safe surgical resection followed by a course of daily oral temozolomide with radiotherapy (chemoradiation), followed by maintenance adjuvant temozolomide. While population-based studies indicate small increases in median survival over recent decades with the advent of more aggressive therapies, including adjuvant chemoradiation and larger tumor resections [1,3], long-term survival is rare.
GBM inevitably recurs following initial therapy, after which there is no standard of care [4,5,6]. Although recent advances in the understanding of GBM biology offer the prospect of new and improved treatments [7,8], patients with recurrent GBM are managed on an individualized basis, according to response to therapy and functional performance status [7,9]. Treatment options include additional surgical resection; chemotherapy (e.g., temozolomide, lomustine, fotemustine, and irinotecan); and re-irradiation [10]. The use of tumor treating fields, consisting of alternating low-intensity electric fields delivered by transducers applied to the scalp, are supported by several clinical trials [11,12].
At diagnosis, GBMs typically appear on standard T1-weighted magnetic resonance imaging (MRI) as a bright gadolinium contrast-enhancing tumor rim that correlates with leaky tumor-associated vasculature and proliferating cancer cells. Generally, the rim surrounds a necrotic core with a potentially large edematous T2-hyperintense region extending through otherwise apparently normal brain [13]. It is well established that glioblastomas diffusely invade beyond the contrast-enhancing rim [14].
There have been many efforts over the past three decades to devise mathematical models of the growth of GBM tumors in realistic brain domains. Most models of the gross evolution of GBM tumor growth use a reaction-diffusion equation that is the sum of a diffusion term plus a growth term, which may be exponential or logistic. Murray [15] suggested the Fisher-Kolmogorov (FK) equation, originally proposed in the 1930s to model the spread of genetic traits in a population [16]. The details of this equation are given in Section 2.1.1. The FK equation and variants have been used extensively to perform numerical simulations that capture the growth of gliomas at macroscopic scales; examples include [17,18,19,20,21].
A natural question is the extent to which reaction-diffusion models can predict the progression of GBM tumors. In the simplest case, the solution of the FK model evolves as a propagating front whose velocity is proportional to √Dρ, where D is the diffusion rate and ρ is the cellular growth rate. Insofar as the expansion rate of a given GBM tumor potentially can be estimated from a time series of MRI scans, several studies have attempted to determine "patient-specific" model parameters using a variety of methods [19,21,22,23,24,25]. Murray [25] applied the FK equation with a purely exponential growth term to estimate patient survival times from initial diagnosis. His analysis suggests that tumors with similar overall front velocities but different diffusion-to-growth ratios may have considerably different distributions of tumor cells, which in turn may affect responses to surgical resection and other treatments.
Some recent work has focused on the potential for dynamical PDE models to be applied to the treatment of GBM patients. Hormuth et al. [20] incorporated tumor surviving fraction into their calibrated reaction-diffusion model, achieving low error in predictions of tumor response to chemoradiation in a cohort of 9 high-grade glioma patients. Martens et al. [21] applied a deep convolutional neural network (DCNN) to multimodal MRI images to reconstruct whole brain cell density distribution and estimate diffusion and proliferation parameters for the reaction-diffusion model. Adequate performance of this approach was demonstrated on synthetic tumors grown over MR brain domains of healthy volunteers and MR data of a real GBM patient. Lipková et al. [26] attempted to combine Bayesian inference and the FK model adapted for structural (MRI) and metabolic (FET-PET) scans to generate personalized radiotherapy plans in 8 cases of GBM that spare more healthy tissue than existing radiotherapy plans.
Efforts to apply a deterministic GBM growth model to clinical data must cope with many uncertainties. Besides the parameters that appear explicitly in the model, the patient brain geometries at the imaging time points must be co-registered (mapped) to each other or to a reference domain. Geometric interpolations may be complicated by the mass effect of the growing tumor [27].
MRI sequences for patients with suspected GBM include T1-weighted sequences before and after gadolinium administration (T1Gd) and T2-weighted fluid-attenuated inversion recovery (T2-FLAIR). Such sequences do not visualize the cancer cells per se but rather identify regions of abnormal, leaky vasculature that are associated with the rapid proliferation of tumor cells. In retrospective studies (including this one), often the only readily available data are time series of MRI scans of individual patients and associated records of clinical visits and treatment timelines. Without extensive biopsy data, one must attempt somehow to initialize the model by inferring tumor cell densities from imaging data; such efforts require "segmentations" of patient images into regions corresponding to T1-weighted enhancement and possibly others (such as necrotic core and tumor-associated edema). If one assumes that the diffusivity of GBM cells differs between white and gray matter, then segmentations of those areas must also be defined as part of the computational domain. (Our experience, described in more detail below, suggests that the outputs from automated segmentation tools often vary considerably between patients and within imaging sequences of the same patient.) Treatment artifacts, such as radiation necrosis, may cause regions of MR signal enhancement that resemble those of rapidly growing tumor, a phenomenon called pseudoprogression [28]; anti-angiogenic agents can reduce the MR signal and produce a pseudoresponse [29].
Finally, even if model parameters could be estimated at a given time point, it seems unlikely that they will stay fixed, as the growth of the tumor waxes and wanes as a consequence of continuing treatment. In summary, uncertainties abound: in the choice of model parameters; the computational domain; the interpretation of MR images; the initial conditions; in quantitative comparisons of model outputs with subsequent patient images; and in the assessment of model errors, as no tractable mathematical model can capture all the relevant details of GBM tumor biology.
The goal of this paper is twofold. First, we introduce a model of GBM growth—which we call the ASU-Barrow (ASUB) model—that may be more biologically realistic than the FK model, which assumes that all GBM cells have the same growth capacity. The ASUB model divides the tumor cell population into two groups: proliferating cells and quiescent (or necrotic) cells. The model is designed to be analytically tractable, efficient to integrate numerically, and to introduce a minimum number of additional parameters. Second, using a retrospective series of 10 patients who all exhibit tumor progression following initial treatment, we investigate whether it is possible to find combinations of parameterizations and initializations that are representative of the range of observed clinical responses to subsequent therapy; both the FK and ASUB models are investigated. Our study is based only on data from MRI sequences that are a routine part of clinical surveillance. All the patients exhibit obvious pathology and have undergone prior surgical resection and continuing and variable treatment protocols. One potential long-term goal of such an effort is to devise a computational tool that could be used as part of efforts to counsel patients about the range of possible outcomes from continued treatment.
In this study, we consider two cellular growth models. The first is the Fisher-Kolmogorov (FK) equation, which to our knowledge was first proposed as a way to describe the gross expansion of GBM tumors by Murray [15]. It assumes that tumor cells diffuse linearly (i.e., Fickian diffusion) throughout brain tissue, and that their proliferation at a given location follows a logistic growth trajectory:
∂n(x,t)∂t=∇⋅(D(x)∇n(x,t))+ρ(x)n(x,t)(1−n(x,t)K). | (2.1) |
Here n(x,t) represents the cancer cell density at time t and location x. The diffusion coefficient is D(x), which we assume is piecewise constant (possibly greater in white matter than in gray matter). The carrying capacity, K, of the tumor is normalized to 1 for simplicity.
One potential limitation of the Fisher-Kolmogorov model (2.1) is that the model does not consider intratumoral heterogeneity; all cancer cells are assumed to have the same growth and diffusion capability. However, GBM tumors typically outgrow their available blood supply, leaving a rapidly proliferating rim that surrounds a "core" of necrotic or hypoxic cells [14]. Several models have been proposed that divide tumor cells into distinct subpopulations [22,23,30,31,32,33,34]. The two-population model used in this paper is described next.
Han et al. [34] proposed a two-population reaction-diffusion model, with proliferating and quiescent (or necrotic) cells that incorporates density-dependent (cross) diffusion. The model can capture the intratumoral heterogeneity and is simple enough that parameter estimation techniques can be applied. However, by introducing a singularity at tumor-free locations, the cross-diffusion terms in this equation complicate the mathematical analysis and make numerical integration computationally expensive (see (2.2)–(2.3) of section 2.1.2). One goal of this work is to present a simplified version of the Han model, which we call the ASUB model, that is computationally inexpensive, amenable to mathematical analysis and parameter estimation techniques, and retains the ability to describe the growth and structure of gliomas.
In the initial model, the tumor is divided into actively proliferating cells, p(x,t), and quiescent cells, q(x,t), with x and t representing location and time, respectively. The quiescent cells are a lumped compartment representing cells that have ceased active division due to competition for space or nutrients, as well as the cells that have undergone necrosis. The model in [34] is given by
∂p(x,t)∂t=∇⋅[(Dp(x)p(x,t)n(x,t))∇n(x,t)]+˜g(n)p(x,t)−˜δ(n)p(x,t), | (2.2) |
∂q(x,t)∂t=∇⋅[(Dq(x)q(x,t)n(x,t))∇n(x,t)]+˜δ(n)p(x,t), | (2.3) |
where
n(x,t)=p(x,t)+q(x,t)K, | (2.4) |
and Dp(x) and Dq(x) are the diffusion coefficients for proliferating and quiescent cells respectively. Proliferating cells grow at the per capita rate ˜g(n) and transition to the quiescent compartment at rate ˜δ(n). Both rates are monotonic functions of n(x,t), which represents the total cancer cell density, as a fraction of K, at a given location and time. As in the FK model, the carrying capacity, K, of the tumor is normalized to 1 for simplicity.
The functions ˜g and ˜δ satisfy the requirement that for all n∈[0,1],
d˜g(n)dn≤0andd˜δ(n)dn≥0, | (2.5) |
with
˜g(0)≥˜δ(0)=0and˜δ(1)>˜g(1)=0. | (2.6) |
In other words, tumor cell growth (death) must be a decreasing (increasing) function of the total cell density. No proliferation occurs at the maximum cell density, and no necrosis occurs at minimal cell density. Similarly to Gyllenberg and Webb (cf. system (2.64) and (2.71) on page 58 of [35]), we implicitly assume that: (1) the necrotic population consists of quiescent and dead cells; (2) the rate at which quiescent cells die is inversely correlated with the nutrient density; and (3) the nutrient density is a decreasing function of the total population n. As a result, the recruitment rate of the necrotic population is a decreasing function of the nutrient density and hence is an increasing function of the total population.
The cross-diffusion terms in Eqs. (2.2)–(2.3) allow the concentration of one cancer cell population to cause a flux in the other. This formulation may be more realistic than simple linear diffusion, where cell populations diffuse independently, and has been employed in previous cancer models [30,31]. However, the cross-diffusion terms also complicate the mathematical analysis and make the integration of this system computationally expensive. For this reason, we consider the simpler model
∂p(x,t)∂t=∇⋅(D(x)∇p(x,t))+˜g(n)p(x,t)−˜δ(n)p(x,t), | (2.7) |
∂q(x,t)∂t=˜δ(n)p(x,t), | (2.8) |
where n(x,t) is given by Eq. (2.4) and D(x) is the diffusion rate of the proliferating cells. In contrast to the original model (2.2)–(2.3), Eqs. (2.7)–(2.8), which we will call the ASU-Barrow (ASUB) model, we assume that proliferating cells diffuse linearly, while quiescent cells do not move (but do take up space). Similar to (2.2)–(2.3), this simpler model emits a traveling wave that can also produce gross growth dynamics that are representative of glioma progression. Although the linear diffusion term ∇(D∇p) implies that only the proliferating cell gradient ∇p drives the invasion process, it does little to recolonize the areas saturated by quiescent cells (q≫p, n≈1). The wave solution p component takes the form of a single forward-moving pulsation, and near the tail (where q≫p, n≈1) of this formation, the recolonization force pales in comparison to the high death rate at this location.
The model used here defines
˜g(n)=ρ(x)g(n)and˜δ(n)=k(x)δ(n), | (2.9) |
where ρ(x) is the maximum per capita growth rate, k(x) is the maximum death (or quiescence) rate, g(n)=1−B(n;α,β), and δ(n)=B(n;α,β), where B(n;α,β) is the beta cumulative distribution function (CDF) with shape parameters α and β. To be more precise, we define B(n;α,β) such that
B(n;α,β)=b(n;α,β)b(1;α,β), | (2.10) |
where
b(n;α,β)=∫n0τα−1(1−τ)β−1dτ. | (2.11) |
The beta CDF is chosen because it is a convenient family of functions whose values are between 0 and 1 with extreme values at the endpoints of the unit interval. In this case, 1−B yields a growth coefficient g(n) that is maximal when the total cell density is small (n≈0) and is zero when n≈1. By varying the shape parameters, the beta CDF can represent linear, concave up, concave down, or sigmoid proliferation and quiescence responses to changes in the total tumor cell density n. We have chosen α=3 and β=1 for the simulations in this paper, as they yield a monotonically decreasing (increasing) and concave down (up) growth (death) term as a function of n, as shown in Figure 1. This choice of g and δ also allows for analytical estimation of D, ρ, and k via the method described in Han et al. [34], but other choices may still allow for identifiable parameters [34].
As discussed in the introduction, there are many uncertainties in the initialization of the model as well as in the parameters that appear explicitly within it. In the remainder of this paper, we distinguish between model parameters, such as the diffusion and proliferation rates that appear explicitly in the PDE, and initialization parameters, which affect the computational domain and choice of initial conditions from patient MRIs. The model parameters for both the FK and ASUB models include the diffusion coefficient D(x) and the maximum growth rate ρ(x). The ASUB model has one additional parameter, the maximum quiescence rate k(x). The computational implementation allows the values of D, ρ, and k to vary according to the type of brain and tumor tissue: in principle, different values may be chosen accordingly as x represents a voxel corresponding to white matter, gray matter, edematous tissue, necrotic tumor core, or enhancing tumor rim. Some prior studies (e.g., [18,19]) suggest that diffusion rates of GBM cells are faster in white matter than in gray matter. The patients in this study have undergone additional treatment for their recurrences, which in some cases includes the implantation of chemotherapy wafers at the boundary of the resection cavity and re-irradiation of unresected tissue along and near regions that exhibit enhancement on T1Gd MRI scans. For these reasons, we allow for the possibility of differential model parameter values in tumorous regions.
Initialization parameters include choices of initial GBM cell population densities in edematous tissue, along the T1Gd-enhancing rim, and the necrotic tumor core, if present. The classification of tumorous regions can change at every imaging time point, because the tumor is re-segmented and the model is re-initialized from the subsequent MR scans and tissue classification.
Altogether, up to 3 model parameters (diffusion rate D, proliferation rate ρ, and quiescence rate k) must be selected for each of 5 tissue classifications. There are also 3 choices of initialization parameters corresponding to the estimated density of each tumor cell population within each tumorous region (T1-enhancing rim, edema, and necrotic core). Thus, there are potentially 3×5+3 parameters in each simulation of the FK model and 3×5+3×2 parameters for the ASUB model (since initial population thresholds must be selected for two subpopulations). Furthermore, to explore the variability of the simulation results, each parameter must be selected from one of several values (or levels) within some reasonable a priori range.
Clearly, even for a small number of levels, it is impossible to run simulations over all potential parameters to determine whether some combination produces a result that approximates the evolution of a given patient's tumor. To make the problem tractable, we treat the simulation process as though it were an experimental design and use a so-called Taguchi method [36] to identify sets of parameters that yield a representative range of outcomes using a relatively small number of model runs.
In the Taguchi approach, one first identifies the parameters to which the results are the most sensitive. The values of these parameters are selected independently from a set of a priori levels. For example, if the range of the parameters p1 and p2 is the interval [1,2], and there are 5 levels, then p1 and p2 may assume any of the values 1.0,1.25,1.50,1.75,2.0 in the numerical experiments, and their values are selected independently.
The remaining parameters, to which the results are less sensitive, are grouped into blocks, and one chooses from among one of the vectorial combinations; that is, they are not selected independently. For example, if q and r also lie in the interval [1,2], then one chooses from one of the pairs (qi,ri), i=1,…,5. One can let qi=ri or define the pairs in some other way, e.g., (q1,r1)=(1,2), (q2,r2)=(1.25,1.75), \ldots, (q5,r5)=(2,1).
Once the number of levels is fixed, the blocked and independently-chosen parameters are identified, and their values are selected according to an orthogonal array. The approach is a kind of Latin hypercube design. We omit further details, as there is a large literature on the topic; see [36] for one overview.
Imaging data of patients who have undergone surgical resection and subsequent treatment at the Barrow Neurological Institute (BNI) were retrospectively analyzed from June 2010 to March 2019. The study's inclusion criteria were the following: age 18 years or older, surgical resection at the BNI, eligibility for standard therapy after surgery, available postoperative MRI consisting of T1-weighted without contrast, T1-weighted with contrast, T2-weighted FLAIR, and sequential progression seen between two MRI scans in either the T1-weighted with contrast or T2-weighted FLAIR sequences. Patients were excluded if excessive motion artifact was present on imaging, at least one MRI sequence was unavailable, the imaging did not show a perceptible progression of disease, or postoperative change and/or radiation necrosis were suspected based on serial imaging. The scans consist of 24 to 30 axial images, extending from the brain stem to the top of the skull, for each patient.
A cohort of 70 patients with histopathologically confirmed glioblastoma multiforme (World Health Organization Grade IV Astrocytoma, GBM) were evaluated. Ten patients met the criteria and were included in the analysis. All patients underwent the standard GBM treatment protocol (i.e., Stupp Protocol) at the BNI and received dexamethasone therapy on the same day of surgical resection. In addition, all patients started radiation therapy with concurrent temozolomide within six weeks of surgical resection. The use of other adjuvant medications (e.g., Afinitor®, Avastin®, Gliadel® wafers, lomustine, Optune®, and procarbazine) were at the discretion of the treating oncologist. Postoperative images were taken at a fixed schedule according to BNI treatment protocol. Images that were taken out of schedule were due either to increase in symptoms or the need for another operation. The study was approved by the St. Joseph's Hospital and Medical Center Institutional Review Board (PHX-19-500-182-20-08).
All patient MRIs are preprocessed using a combination of the MATLAB® software package Statistical Parameter Mapping 12 (SPM-12) [37] and the open-source software package 3D Slicer [38]. SPM-12 is used to convert the T1-weighted (with and without contrast) and T2-weighted FLAIR sequences from a DICOM file format into a NIFTI file format. Once converted, 3D Slicer's N4ITK MRI bias-correction tool is used on all sequences to remove any signal inhomogeneity from the MRI data. Then, for each patient, the SPM-12 software is used to co-register all sequences into the native brain space associated with the T1-weighted (without contrast) sequence of the patient's first scan. The resolution of the axial cross-sections was reduced to approximately 256×256, corresponding to a horizontal resolution of 0.75 to 1.0 mm, depending on the patient. (The coarse graining is done by dividing the voxels of each cross-section into 2×2 squares and taking their average as the value for the new grid point.) To keep the method general, default settings were used with all processes. The result in each case is a fully 3-dimensional computational volume consisting of approximately 256×256×25 voxels. (The number of vertical levels depends on the number of axial images in the first MRI dataset for each patient.) No-flux boundary conditions are imposed wherever brain tissue meets the skull or cerebro-spinal fluid (CSF).
After preprocessing, each tumor voxel is categorized into one of three idealized tissue classifications: necrotic core (hypointense areas on T1-weighted MRI with gadolinium contrast), contrast-enhancing rim (hyperintense on T1-weighted MRI with gadolinium contrast), and tumor-associated edema (hyperintense on T2-FLAIR). For convenience, the names "core, " "rim, " and "edema" will be used to identify these respective segmentations. To create the segmentations, the NIFTI data is loaded into the 3D Slicer software and the areas that correspond to core, rim, and edema are manually marked using the segmentation tool. These segmentations are performed by experts in neuroanatomy, neuroimaging, and neurosurgery.
Finally, the patient's brain is segmented into tissue types, with each voxel assigned to exactly one of three classes: white matter, gray matter, or CSF. Segmentations are created for each scan via the automatic segmentation tool (with the default settings) provided by SPM-12, using the original (unprocessed) NIFTI file corresponding to the T1-weighted sequence without contrast. Resection cavities are categorized as CSF during this process. The results then undergo the coregistration and resolution reductions described above. All patients of this study have undergone an initial resection of their tumor, and the MRI images are postoperative scans. Consequently, the anatomy of the brain is distorted, and postoperative cavities sometimes are present.
Figure 2 shows a representative 2D cross-section of the 3D computational domain for patient 1. Each panel represents the same slice of a different scan. The number of days since the first scan, t, is written at the upper left of each panel, while the time since the previous scan, Δt, is written at the upper right. The left side of each panel shows the preprocessed T2-weighted sequence with contrast for patient 1, and the right side is the associated brain segmentations. Similar figures can be found for the other patients in the supplementary material (Figures S1–S9).
The simulation is initialized using the tumor segmentations described in Section 2.2.2. Each simulation is prescribed density thresholds N1, N2, and N3 (with 0<N3<N2<N1<1) that determine the tumor tissue classifications (i.e., core, rim, and edema) and determine the cell densities for the initial conditions. Voxels that belong to the enhancing rim segmentation are assigned densities between N2 and N1, while voxels belonging to the edema segmentation receive densities between N3 and N2. Recent studies support the notion that GBM cell density is approximately linearly related to the signal of the T2-FLAIR and T1 weighted (with contrast) sequences [39,40]. Within a given segmented region, tumor cell densities are vary linearly with respect to MR intensity within the prescribed ranges. (For example, voxels at the maximum intensity within the enhancing rim are assigned an initial cell density of N1, those of minimum intensity N2, and linearly in between.) The initialization parameters N1, N2, and N3 are selected according to the Taguchi sampling design outlined in Sections 2.1.4 and 2.3.2.
The initial data for each patient consists of a post-resection MRI. Because most patients in our dataset have had an initial gross total resection, no necrotic tumor core is visible on the initial MRI. The resected tumor tissue has been filled with CSF, and the initial segmentation is labeled accordingly.
For the ASUB model, the tumor is further divided into proliferating and quiescent cells based on the simulation parameters R1 and R2, which ascribe an initial percentage of quiescent cells in the rim and edema, respectively. We assume that the rim consists mostly of proliferating cells that are slowly infiltrating the edematous regions. We fix R1=0.0625 and R2=0.01.
Both models are integrated using a Runge-Kutta-Chebyshev (RKC) solver by Shampine et al. [41]. RKC methods have been developed especially for reaction-diffusion equations, which tend to yield stiff systems of ordinary differential equations after finite-difference discretization. The diffusion term is integrated explicitly, and the reaction term implicitly. The approach is particularly efficient when the reaction term depends only on the state at a single grid point, which is the case for the models considered here. Given the complex geometry of the brain and the no-flux boundary conditions, the RKC approach is considerably simpler to implement than a fully implicit solver. A multipoint stencil is used to calculate the diffusion term; it avoids the discretization artifacts of a 7-point stencil and allows for larger time steps in an explicit numerical scheme [42]. A 60-day simulation on a typical 256×256×25 computational domain takes about 30 seconds using one core of an Intel Xeon processor.
Simulations are performed on a three-dimensional brain geometry based on the tissue segmentations described in Section 2.2.2. The spatial resolution varies for each patient but is approximately 0.94×0.94×6.25 mm with slice thickness having the most variability, ranging between 5 mm and 7.5 mm. We assume that a voxel is part of the tumor core whenever the tumor cell density n(x,t) satisfies N1≤n(x,t), the rim whenever N2≤n(x,t)<N1, and part of the edema whenever N3≤n(x,t)<N2. All other voxels within the brain interior (i.e., where n(x,t)≤N3) are labeled as white matter, gray matter, or CSF based on the assignment rendered by SPM-12. Cells proliferate and diffuse through the brain at varying rates as described in Section 2.1.3, while a no-flux condition is imposed at the boundary between brain tissue and CSF.
As noted in the introduction, one objective of this preliminary study is to determine whether we can find sets of model parameters within biologically plausible ranges that can simulate clinically realistic tumors. Of course, neither of the models considered here attempts to incorporate details of GBM biology beyond the gross expansion of the tumor cell population. Our goal is to find model and initialization parameters for which the simulated tumors approximately resemble the clinically observed ones in terms of overall volume (e.g., of T1Gd-enhancing tissue) and approximate location.
The model and initialization parameters form a very large space. Insofar as ours is an exploratory study, we believe that a Taguchi design, as outlined in Section 2.1.4, is appropriate for assessing simulation variability. To simplify the parameter search, we choose D(x) to be a piecewise constant function such that D(x)=D1 whenever x corresponds to a region of white matter or enhancing tumor rim, and and D(x)=D2 elsewhere within the brain. The growth rate is taken to be ρ(x)=ρ1 and the quiescence rate k(x)=k1 when x is in the enhancing rim region, and ρ(x)=ρ2 and k(x)=k2 elsewhere.
One important caveat, and a potential source of simulation error, is that SPM's automated classification of gray and white matter varies considerably between patients and even between scans of the same patient, perhaps because of tumor mass effect and the presence of resection cavities. Manual segmentation of gray- and white-matter tracts has not been possible with the time available to the authors.
Based on analytical and numerical investigations, we expect that the model parameters D1, D2, ρ1, and k1 and the initialization parameters N1 and N2 are the most sensitive; thus, they are allowed to vary independently. Twelve different choices (levels) for each parameter are possible. The possible levels for the white-matter diffusion rate D1 are twice as large as those for D2 (cf. Table 1). The levels of proliferation and quiescence rates, ρ1 and k1, are chosen independently from the same interval. The cell densities corresponding to enhancing rim are taken from a relatively large interval, given the relatively large variability in the associated MR intensities [39,40]. The simulation results are less sensitive to choices of the other parameters, which are selected as a block (cf. Table 2). The simulation results include every possible pair of diffusion rates D1 and D2 in Table 1.
Levels | D1 | D2 | ρ1 | k1 | N1 | N2 |
1 | 0.2000 | 0.1000 | 0.0038 | 0.0038 | 0.3000 | 0.0600 |
2 | 0.2182 | 0.1091 | 0.0047 | 0.0047 | 0.3500 | 0.0700 |
3 | 0.2364 | 0.1182 | 0.0056 | 0.0056 | 0.4000 | 0.0800 |
4 | 0.2545 | 0.1273 | 0.0068 | 0.0068 | 0.4500 | 0.0900 |
5 | 0.2727 | 0.1364 | 0.0083 | 0.0083 | 0.5000 | 0.1000 |
6 | 0.2909 | 0.1455 | 0.0101 | 0.0101 | 0.5500 | 0.1100 |
7 | 0.3091 | 0.1545 | 0.0122 | 0.0122 | 0.6000 | 0.1200 |
8 | 0.3273 | 0.1636 | 0.0148 | 0.0148 | 0.6500 | 0.1300 |
9 | 0.3455 | 0.1727 | 0.0178 | 0.0178 | 0.7000 | 0.1400 |
10 | 0.3636 | 0.1818 | 0.0217 | 0.0217 | 0.7500 | 0.1600 |
11 | 0.3818 | 0.1909 | 0.0257 | 0.0257 | 0.8000 | 0.1800 |
12 | 0.4000 | 0.2000 | 0.0315 | 0.0315 | 0.8500 | 0.2000 |
Levels | ρ2 | k2 | N3 |
1 | 0.0032 | 0.0032 | 0.0100 |
2 | 0.0038 | 0.0038 | 0.0120 |
3 | 0.0047 | 0.0047 | 0.0140 |
4 | 0.0056 | 0.0056 | 0.0160 |
5 | 0.0068 | 0.0068 | 0.0180 |
6 | 0.0083 | 0.0083 | 0.0200 |
7 | 0.0101 | 0.0101 | 0.0220 |
8 | 0.0122 | 0.0122 | 0.0240 |
9 | 0.0148 | 0.0148 | 0.0260 |
10 | 0.0178 | 0.0178 | 0.0280 |
11 | 0.0217 | 0.0217 | 0.0300 |
12 | 0.0257 | 0.0257 | 0.0320 |
Model performance, in terms of predicting tumor evolution from an initial patient MRI to some future scan, is assessed by comparing the simulation results to the actual tumor segmentations. Let S and R denote the set of voxels containing the simulated and real (segmented) tumors respectively, with |S| and |R| being the number of voxels in each. Let |S∩R| denote the number of voxels occupied by both the simulated and real tumors and |S∪R|, by one or the other. We define the containment, C, as the fraction of the simulated tumor that overlaps the real one:
C=|S∩R||S|. | (2.12) |
The agreement, A, is defined as
A=|S∩R||S∪R|. | (2.13) |
Suppose for example that the simulated tumor (voxel set S) occupies twice the volume of the actual tumor (voxel set R) as segmented at the comparison time. If the simulated tumor completely contains the actual one, then the containment is 100 percent, but the agreement is only 50 percent. Agreement is a stricter criterion insofar as it measures how well the model predicts the future location of the tumor as well as its volume. The agreement is 100 percent if and only if the simulated and actual tumors occupy the same voxels and is 0 if there is no overlap between them. The definitions imply that A≤C for every nonempty tumor.
The results presented here focus on the time intervals when each patient shows the greatest clinical expansion of tumor. The spatial plots are axial cross-sections and represent slices that typically contain the greatest tumor extent, based on the MRI study. (There are 24 to 30 axial cross-sections for each patient in each MRI series.)
Figures 3–5 show representative 2D axial cross-sections, taken from the 3D computational domains created in Section 2.2.2, for Patients 1–3 respectively. (Graphs for the other patients are located in the supplementary material in Figures S10–S16.) For each patient-scan combination in the study, the ensemble simulations, generated by the method outlined in 2.3.2, are overlaid on top of both the T1-weighted sequence (with contrast) as well as the brain segmentation (cf. Section 2.2.2). To improve the visualization, the edema segmentation has been removed from the brain segmentation and the core segmentation has been combined with the rim segmentation (the core and rim are both green) in these images. The panels on the left-hand side display results generated from the Fisher-Kolmogorov model, Eq. (2.1), and those on the right, the ASUB model, Eqs. (2.6)–(2.7). Each panel shows an axial slice of the T1-weighted, post-contrast MRI on the left and the same cross-section of the computational domain on the right. Panels on the same row correspond to the same scan but different models. For each scan, the time t from the first post-operative scan is shown in the title of the left panel, while the simulation time Δt (the time since the previous scan) is indicated in the title of the right panel.
In each plot, the green curves represent the outer boundary of the rim segmentation at the initial simulation time; the red curves, at the time of the next scan. Each blue curve is a contour line where the total cancer cell density n, as generated by the simulation, is equal to half the maximum value. This is done so that a common metric, which does not depend on specific choices of the free parameters N1, N2, and N3, can be used to compare the experiments. The "50-percent" contour represents a point that is halfway to the wave front, which we believe provides a good representation of tumor densities found in the enhancing rim. Other reasonable choices of the contour lines also are possible (cf. Section 3.2). There are 144 blue curves on each plot, one for each choice of the parameters as described in Section 2.3.2.
The spacing between the contour lines in a region illustrates the sensitivity of the simulation results to the choices of the parameters. Closely spaced contours suggest that many choices of the model and initialization parameters yield comparable results. On the other hand, if only a single contour exists in a location, then the results are sensitive to the choice of one or more model or initialization parameters.
We measure both the containment and agreement, Eqs. (2.11)–(2.12), for each set of simulations by comparing the outputs with the tumor segmentations described in Section 2.2.2. In this case, we define R to be the set of voxels that belong to either the rim or core segmentation, and S as the set of voxels such that n is greater than or equal to half the maximum density of the experimental result. In the supplementary material, the containment and agreement results for each patient-scan combination in the study are summarized in Table S1 and Table S2, respectively. For each patient case, the minimum, maximum, and average value and its standard deviation, are reported.
Table 3 shows the average containment and agreement measurements among all simulations. The entries are computed by taking the mean of the appropriate column in Table S1 or Table S2. The same combinations of model and initialization parameters are used for each patient. In some cases, the parameters yield solutions that agree poorly with the observed tumors and correspond to entries in Table 3 that have low containment and agreement scores. The results shown in the "average" columns in Table 3 suggest that the FK model has slightly better containment, while the ASUB model has slightly better agreement with the clinically observed tumors at the next patient-scan time.
Containment | Agreement | |||||||
Model | Min | Max | Average | Std Dev | Min | Max | Average | Std Dev |
Fisher | 0.19 | 0.45 | 0.29 | 0.06 | 0.10 | 0.29 | 0.20 | 0.04 |
ASUB | 0.13 | 0.46 | 0.27 | 0.07 | 0.09 | 0.31 | 0.21 | 0.05 |
Containment | Agreement | |||||||
Model | Min | Max | Average | Std Dev | Min | Max | Average | Std Dev |
Fisher | 0.51 | 0.64 | 0.59 | 0.03 | 0.14 | 0.25 | 0.18 | 0.02 |
ASUB | 0.39 | 0.64 | 0.57 | 0.05 | 0.15 | 0.33 | 0.22 | 0.04 |
Finally, we remark that the results in Table 3 are highly dependent on the choice of contour lines. As noted above, we have chosen the contour lines where the tumor cell density is 50 percent of the carrying capacity. Other reasonable choices are possible. For example, we can draw the contours corresponding to the maximum tumor cell density in the enhancing rim of the tumor (i.e., N2, which is an initialization parameter that varies between simulations). Table 4 shows the containment and agreement measures averaged over all patients and over all simulations. The containment values are significantly better and the agreement values are slightly better across the board. However, these contours suggest simulated tumors that are larger in general than the observed ones (see Figures S17–S19 in the supplementary material).
In this preliminary study, we have adapted a single-population model based on the Fisher-Kolmogorov equation and a simple two-population reaction-diffusion model (ASUB), Eqs. (2.6)–(2.7), to simulate the gross behavior of proliferating and quiescent (necrotic) tumor cells using clinical MRI scans from patients undergoing treatment for glioblastoma multiforme (GBM). All simulations have been performed using 3D computational domains constructed from individual patients' brain geometries. The simulations attempt to follow the clinical trajectories of 10 individual patients who have undergone either gross total or partial resection of tumor followed by radiation and chemotherapy according to the Stupp protocol [2]. As there is no standard of care for patients who have experienced tumor recurrence, any mathematical model that can predict, with reasonable accuracy, the range of likely progressions would be clinically useful. The results would be useful for patient counseling or for evaluating the therapeutic effect of further treatment, whose benefits must be balanced against potential toxicity and quality of life measures.
For each patient-scan combination, we have generated an ensemble of 144 simulations using a Taguchi design to explore the large parameter space associated with the models and their initializations. Altogether, tumor simulations over 17 different time intervals have been performed, and the results are compared against manual segmentations of tumor imaging performed by neurosurgical experts. All patients who had both imaging evidence of recurrence and biopsy proven recurrence on reoperation were included in the study.
Our results suggest that simple models may be able to provide predictions, with modest skill, of the clinical evolution of recurrent tumors in individual patients over periods of several weeks to months as they undergo a variety of treatments. The performance of the FK and ASUB models is comparable, according to measures of tumor containment and agreement. Of the 17 time intervals studied, parameter sets can be identified in 9 of them such that tumors simulated with the FK and ASUB models contain at least 40 percent of the observed clinical tumor, as defined by Eq. (2.11), and whose overall agreement, Eq. (2.12), is at least 20 percent for the FK model and 29 percent for the ASUB model (cf. Tables S1 and S2 in the supplementary material).
In several cases, the simulation approach outlined in Section 2.3 yields results in reasonable agreement with the observed subsequent state of the GBM tumor. The results suggest that the it may be possible to determine parameter ranges that yield reasonable simulation results for a variety of patient cases. However, the parameter ranges that produce reasonably good results for Patients 1–3 do not work well for Patients 7 and 10. More work is needed to refine the intervals from which the parameters are selected, and other experimental designs should be considered to assess the sensitivity of the results to a wider range of parameter choices.
One difficulty is the initialization of the models from MRI data, particularly in edematous regions. Although the models often produce a tumor that contains the edematous region, they overestimate the tumor cell density there. Edema occurs as the result of fluid buildup within brain tissue due to a complex combination of leaky tumor-related vasculature, invasion of normal brain tissue by tumor cells, treatment-related effects, and other factors [44]. Edema is associated with tumor recurrence [45] and is treated clinically with dexamethasone [46]. Our models do not attempt to simulate hydrodynamic effects in the brain tissue. In the present simulations, edematous regions are treated simply as areas where tumor cells are present at low levels. Better models of the cellular dynamics in these regions are needed.
Other challenges in the clinical assessment of GBM using MRI include pseudoresponse and pseudoprogession, wherein tumors may appear to shrink or grow, respectively, on surveillance scans, when in fact the results simply are artifacts of treatment [13]. Based on available medical records, all patients included in this study were believed to have experienced actual recurrence at the time of each scan and were treated accordingly, but imaging artifacts due to ongoing treatment cannot be ruled out.
One possible way to make our study more robust would be the use of positron emission tomography (PET) or diffusion-weighted imaging (DWI) to infer tumor cell density. Such imaging techniques have been used in other modeling studies [20,21,26], but they are expensive and are not part of standard clinical care. In addition, these studies either did not use pathological subjects, surgical resection was not performed, or areas of hypointensity (i.e., necrosis or resection cavity) were not segmented independently. We believe our study is more clinically relevant and generalizable because it incorporates standard imaging studies and treatment protocols, while also considering the effects of surgery (e.g., resection cavity).
The numerical implementations in this study do not attempt to account for the mass effect of the tumors (i.e., the deformation of the brain anatomy due to the malignant tissue). While finite-element methods can produce realistic depictions of mass effect [17], they complicate the modeling effort; stiffness coefficients for the various brain tissues must be estimated for each patient, among other initialization parameters.
The scans from each patient must be co-registered with one another so that the results of one simulation can be compared with the segmentations at the next scan. In a few cases, the co-registration errors in the vicinity of the segmented tumor are nontrivial (e.g., the agreement between the resection cavities between two successive scans is as low as 75 percent). Such errors decrease the containment and agreement measures even in cases where the model otherwise generates a clinically realistic tumor.
Our simulations do not account for the variability in patient treatment timelines. For example, many of the patients received additional therapeutic treatment that was at the discretion of the oncologist. Depending on the dose and duration, treatment may affect the clinical progression of the tumor within a given simulation interval. Future work should involve more detailed analyses of treatment timelines with more patients to try to determine, for instance, whether growth and quiescence parameters are significantly affected temporarily by adjuvant therapeutics, and appropriate optimization algorithms should be explored. In addition, future work should include imaging modalities that can estimate the tumor cell density within the tumor (i.e., intratumoral heterogeneity). Inclusion of parameters for cell density will lead to more accurate simulations that agree in directionality.
Finally, we have investigated the potential of a reaction-diffusion equation (the ASUB model) that includes a component for quiescent (or necrotic) cells. The ASUB model appears to perform comparably to the usual FK model, but it introduces another parameter (the cellular quiescence rate), and it takes about twice as long to simulate. On the other hand, the simulations are initialized on patients who have undergone surgical resections that have removed the necrotic core of each tumor, so the ASUB model may not offer an advantage over the simpler FK model for this data set
The analytical approach of Han et al. [34] can be adapted to the ASUB model to determine a priori parameter estimates in such cases, but we defer such an exploration to future work. Kalman filtering and related data assimilation methods also may be applicable [47,48], but the analysis of MRI data needs more refinement to account for potential treatment effects. Future work will explore methods to construct observation operators from MRI data and to provide alternative patient-specific estimates of model parameters using Bayesian approaches.
The authors thank the Barrow Neurological Institute for providing MR images and the anonymous referees for many helpful comments.
The authors declare no conflicts of interest.
This work was funded by the Arizona Biomedical Research Commission under grant number ADH516-162514. Additional support was provided from the Newsome Chair in Neurosurgery Research held by Mark C. Preul; the National Institutes of Health under award number 5R01GM131405-02 and by the National Science Foundation under grant DMS-1757663 to Yang Kuang; and by funds from the School of Mathematical and Statistical Sciences at Arizona State University.
Tissue/Tumor segmentations
Ensemble results
Containment and agreement results
Fisher | ASUB | |||||||
Patient/Time | Min | Max | Avg | StD | Min | Max | Avg | StD |
1 / 56-114 | 0.18 | 0.41 | 0.28 | 0.05 | 0.08 | 0.38 | 0.25 | 0.06 |
1 / 114-142 | 0.16 | 0.45 | 0.23 | 0.04 | 0.14 | 0.53 | 0.23 | 0.05 |
1 / 142-168 | 0.31 | 0.85 | 0.39 | 0.09 | 0.27 | 0.86 | 0.38 | 0.07 |
2 / 56-158 | 0.06 | 0.64 | 0.43 | 0.15 | 0.02 | 0.57 | 0.26 | 0.17 |
3 / 182-238 | 0.22 | 0.48 | 0.35 | 0.06 | 0.12 | 0.44 | 0.27 | 0.08 |
3 / 238-266 | 0.11 | 0.33 | 0.18 | 0.05 | 0.11 | 0.41 | 0.22 | 0.06 |
4 / 107-168 | 0.56 | 0.76 | 0.66 | 0.05 | 0.29 | 0.76 | 0.58 | 0.10 |
5 / 118-180 | 0.09 | 0.21 | 0.17 | 0.03 | 0.06 | 0.20 | 0.13 | 0.03 |
5 / 266-329 | 0.31 | 0.52 | 0.38 | 0.05 | 0.22 | 0.48 | 0.33 | 0.04 |
6 / 0- 61 | 0.21 | 0.33 | 0.26 | 0.03 | 0.13 | 0.34 | 0.24 | 0.05 |
6 / 61-112 | 0.20 | 0.31 | 0.25 | 0.02 | 0.12 | 0.32 | 0.24 | 0.05 |
7 / 0- 58 | 0.12 | 0.40 | 0.26 | 0.07 | 0.05 | 0.37 | 0.18 | 0.07 |
7 / 58- 83 | 0.16 | 0.56 | 0.24 | 0.09 | 0.15 | 0.55 | 0.22 | 0.07 |
8 / 93-144 | 0.02 | 0.19 | 0.13 | 0.02 | 0.09 | 0.23 | 0.15 | 0.03 |
9 / 80-182 | 0.00 | 0.03 | 0.02 | 0.01 | 0.00 | 0.06 | 0.03 | 0.01 |
9 / 182-245 | 0.03 | 0.12 | 0.07 | 0.02 | 0.02 | 0.11 | 0.07 | 0.02 |
10 / 136-215 | 0.44 | 0.68 | 0.61 | 0.05 | 0.32 | 0.69 | 0.56 | 0.08 |
Fisher | ASUB | |||||||
Patient/Time | Min | Max | Avg | StD | Min | Max | Avg | StD |
1 / 56-114 | 0.18 | 0.33 | 0.25 | 0.03 | 0.08 | 0.36 | 0.24 | 0.06 |
1 / 114-142 | 0.16 | 0.43 | 0.23 | 0.04 | 0.14 | 0.48 | 0.23 | 0.05 |
1 / 142-168 | 0.31 | 0.55 | 0.37 | 0.05 | 0.27 | 0.51 | 0.37 | 0.05 |
2 / 56-158 | 0.06 | 0.36 | 0.29 | 0.08 | 0.02 | 0.38 | 0.21 | 0.11 |
3 / 182-238 | 0.16 | 0.32 | 0.26 | 0.03 | 0.11 | 0.31 | 0.23 | 0.05 |
3 / 238-266 | 0.11 | 0.28 | 0.18 | 0.04 | 0.11 | 0.34 | 0.21 | 0.06 |
4 / 107-168 | 0.14 | 0.62 | 0.46 | 0.13 | 0.22 | 0.62 | 0.50 | 0.09 |
5 / 118-180 | 0.08 | 0.13 | 0.11 | 0.01 | 0.06 | 0.14 | 0.11 | 0.02 |
5 / 266-329 | 0.05 | 0.20 | 0.16 | 0.04 | 0.08 | 0.21 | 0.19 | 0.02 |
6 / 0- 61 | 0.09 | 0.26 | 0.18 | 0.04 | 0.12 | 0.29 | 0.20 | 0.03 |
6 / 61-112 | 0.11 | 0.26 | 0.21 | 0.03 | 0.12 | 0.27 | 0.20 | 0.04 |
7 / 0- 58 | 0.12 | 0.23 | 0.17 | 0.02 | 0.05 | 0.22 | 0.15 | 0.04 |
7 / 58- 83 | 0.10 | 0.25 | 0.18 | 0.03 | 0.10 | 0.28 | 0.19 | 0.03 |
8 / 93-144 | 0.01 | 0.18 | 0.11 | 0.03 | 0.08 | 0.21 | 0.13 | 0.02 |
9 / 80-182 | 0.00 | 0.02 | 0.01 | 0.01 | 0.00 | 0.05 | 0.03 | 0.01 |
9 / 182-245 | 0.03 | 0.11 | 0.06 | 0.02 | 0.02 | 0.10 | 0.07 | 0.02 |
10 / 136-215 | 0.01 | 0.24 | 0.07 | 0.07 | 0.01 | 0.29 | 0.17 | 0.08 |
Alternative ensemble results
[1] | World Health Organization. Available from: https://covid19.who.int. |
[2] |
A. Din, Y. Li, T. Khan, G. Zaman, Mathematical analysis of spread and control of the novel corona virus (COVID-19) in China, Chaos Solitons Fractals, 141 (2020), 110286. https://doi.org/10.1016/j.chaos.2020.110286 doi: 10.1016/j.chaos.2020.110286
![]() |
[3] |
S. Allegretti, I. Bulai, R. Marino, M. Menandro, K. Parisi, Vaccination effect conjoint to fraction of avoided contacts for a Sars-Cov-2 mathematical model, Math. Model. Num. Sim. Appl., 2 (2021), 56–66. https://doi.org/10.53391/mmnsa.2021.01.006 doi: 10.53391/mmnsa.2021.01.006
![]() |
[4] |
P. Naik, M. Yavuz, S. Qureshi, J. Zu, S. Townley, Modeling and analysis of COVID-19 epidemics with treatment in fractional derivatives using real data from Pakistan, Eur. Phys. J. Plus., 135 (2020), 795. https://doi.org/10.1140/epjp/s13360-020-00819-5 doi: 10.1140/epjp/s13360-020-00819-5
![]() |
[5] |
D. Okuonghae, A. Omame, Analysis of a mathematical model for COVID-19 population dynamics in Lagos, Nigeria, Chaos Solitons Fractals, 139 (2020), 110032. https://doi.org/10.1016/j.chaos.2020.110032 doi: 10.1016/j.chaos.2020.110032
![]() |
[6] |
F. ¨Ozk¨ose, M. Yavuz, Investigation of interactions between COVID-19 and diabetes with hereditary traits using real data: A case study in Turkey, Comput. Bio. Med., 141 (2021), 105044. https://doi.org/10.1016/j.compbiomed.2021.105044 doi: 10.1016/j.compbiomed.2021.105044
![]() |
[7] |
J. Deng, S. Tang, H. Shu, Joint impacts of media, vaccination and treatment on an epidemic Filippov model with application to COVID-19, J. Theor. Biol., 523 (2021), 110698. https://doi.org/10.1016/j.jtbi.2021.110698 doi: 10.1016/j.jtbi.2021.110698
![]() |
[8] |
L. Humphrey, E. Thommes, R. Fields, L. Coudeville, N. Hakim, A. Chit, et al., Large-scale frequent testing and tracing to supplement control of COVID-19 and vaccination rollout constrained by supply, Infect. Dis. Modell., 6 (2021) 955–974. https://doi.org/10.1016/j.idm.2021.06.008 doi: 10.1016/j.idm.2021.06.008
![]() |
[9] |
J. Asamoah, Z. Jin, G. Sun, B. Seidu, E. Yankson, A. Abidemi, et al., Sensitivity assessment and optimal economic evaluation of a new COVID-19 compartmental epidemic model with control interventions, Chaos Solitons Fractals, 146 (2021), 110885. https://doi.org/10.1016/j.chaos.2021.110885 doi: 10.1016/j.chaos.2021.110885
![]() |
[10] |
X. Duan, X. Li, M. Martcheva, S. Yuan, Using an age-structured COVID-19 epidemic model and data to model virulence evolution in Wuhan, China, J. Biol. Dynam., 16 (2022), 14–28. https://doi.org/10.1080/17513758.2021.2020916 doi: 10.1080/17513758.2021.2020916
![]() |
[11] |
S. Jiao, M. Huang, An SIHR epidemic model of the COVID-19 with general population-size dependent contact rate, AIMS Math., 5 (2020), 6714–6725. https://doi.org/10.3934/math.2020431 doi: 10.3934/math.2020431
![]() |
[12] |
J. Zhang, Z. Ma, Global dynamics of an SEIR epidemic model with saturating contact rate, Math. Biosci., 185 (2003), 15–32. https://doi.org/10.1016/S0025-5564(03)00087-7 doi: 10.1016/S0025-5564(03)00087-7
![]() |
[13] |
Q. Liu, D. Jiang, N. Shi, T. Hayat, B. Ahmad, Stationary distribution and extinction of a stochastic SEIR epidemic model with standard incidence, Physica A, 476 (2017), 58–69. https://doi.org/10.1016/j.physa.2017.02.028 doi: 10.1016/j.physa.2017.02.028
![]() |
[14] |
S. Jamshidi, M. Baniasad, D. Niyogi, Global to USA county scale analysis of weather, urban density, mobility, homestay, and mask use on COVID-19, Int. J. Environ. Res. Public Health, 17 (2020), 7847. https://doi.org/10.3390/ijerph17217847 doi: 10.3390/ijerph17217847
![]() |
[15] |
M. S. Hossain, S. Ahmed, M. J. Uddin, Impact of weather on COVID-19 transmission in south Asian countries: An application of the ARIMAX model, Sci. Total Environ., 761 (2021), 143315. https://doi.org/10.1016/j.scitotenv.2020.143315 doi: 10.1016/j.scitotenv.2020.143315
![]() |
[16] |
M. Habeebullah, H. A. Abd El-Rahim, A. Morsy, Impact of outdoor and indoor meteorological conditions on the COVID-19 transmission in the western region of Saudi Arabia, J. Environ. Manage., 288 (2021), 112392. https://doi.org/10.1016/j.jenvman.2021.112392 doi: 10.1016/j.jenvman.2021.112392
![]() |
[17] |
M. Baniasad, G. Mofrad, B. Bahmanabadi, S. Jamshidi, COVID-19 in Asia: Transmission factors, re-opening policies, and vaccination simulation, Environ. Res., 202 (2021), 111657. https://doi.org/10.1016/j.envres.2021.111657 doi: 10.1016/j.envres.2021.111657
![]() |
[18] | O. Damette, S. Goutte, Weather, pollution and COVID-19 spread: A time series and wavelet reassessment, HAL, 2020. Available from: https://halshs.archives-ouvertes.fr/halshs-02629139. |
[19] | M. Keeling, P. Rohani, Modeling infectious diseases in human and animals, Princeton University Press, New Jersey, 2008. https://doi.org/10.2307/j.ctvcm4gk0 |
[20] |
A. Gray, D. Greenhalgh, L. Hu, X. Mao, J. Pan, A stochastic differential equation SIS epidemic model, SIAM J. Appl. Math., 71 (2014), 876–902. https://doi.org/10.2307/23073365 doi: 10.2307/23073365
![]() |
[21] |
D. Li, J. Cui, M. Liu, S. Liu, The evolutionary dynamics of stochastic epidemic model with nonlinear incidence rate, Bull. Math. Biol., 77 (2015), 1705–1743. https://doi.org/10.1007/s11538-015-0101-9 doi: 10.1007/s11538-015-0101-9
![]() |
[22] |
Y. Cai, J. Li, Y. Kang, K. Wang, W. Wang, The fluctuation impact of human mobility on the influenza transmission, J. Franklin Inst., 357 (2020), 8899–8924. https://doi.org/10.1016/j.jfranklin.2020.07.002 doi: 10.1016/j.jfranklin.2020.07.002
![]() |
[23] |
J. Asamoah, M. Owusu, Z. Jin, F. Oduro, A. Abidemi, E. Gyasi, Global stability and cost-effectiveness analysis of COVID-19 considering the impact of the environment: using data from Ghana, Chaos Solitions Fractals, 140 (2020), 110103. https://doi.org/10.1016/j.chaos.2020.110103 doi: 10.1016/j.chaos.2020.110103
![]() |
[24] |
C. Manski, F. Molinari, Estimating the COVID-19 infection rate: anatomy of an inference problem, J. Econom., 220 (2020), 181–192. https://doi.org/10.1016/j.jeconom.2020.04.041 doi: 10.1016/j.jeconom.2020.04.041
![]() |
[25] |
Z. Cao, W. Feng, X. Wen, L. Zu, M. Cheng, Dynamics of a stochastic SIQR epidemic model with standard incidence, Physica A, 527 (2019), 121180. https://doi.org/10.1016/j.physa.2019.121180 doi: 10.1016/j.physa.2019.121180
![]() |
[26] |
D. Adak, A. Majumder, N. Bairagi, Mathematical perspective of COVID-19 pandemic: Disease extinction criteria in deterministic and stochastic models, Chaos Solitions Fractals, 27 (2021), 104472. https://doi.org/10.1016/j.chaos.2020.110381 doi: 10.1016/j.chaos.2020.110381
![]() |
[27] |
M. Fatini, M. Khalifi, R. Gerlach, A. Laaribi, R. Taki, Stationary distribution and threshold dynamics of a stochastic SIRS model with a general incidence, Physica A, 534 (2019), 120696. https://doi.org/10.1016/j.physa.2019.03.061 doi: 10.1016/j.physa.2019.03.061
![]() |
[28] |
C. Ji, D. Jiang, Threshold behaviour of a stochastic SIR model, Appl. Math. Model., 38 (2014), 5067–5079. https://doi.org/10.1016/j.apm.2014.03.037 doi: 10.1016/j.apm.2014.03.037
![]() |
[29] |
Y. Zhou, W. Zhang, S. Yuan, Survival and stationary distribution of a SIR epidemic model with stochastic perturbations, Appl. Math. Comput., 244 (2014), 118–131. https://doi.org/10.1016/j.amc.2014.06.100 doi: 10.1016/j.amc.2014.06.100
![]() |
[30] |
Q. Liu, D. Jiang, Dynamics of a stochastic multigroup SIQR epidemic model with standard incidence rates, J. Franklin Inst., 356 (2019), 2960–2993. https://doi.org/10.1016/j.jfranklin.2019.01.038 doi: 10.1016/j.jfranklin.2019.01.038
![]() |
[31] |
Q. Liu, Stability of SIRS system with random perturbations, Physica A, 388 (2009), 3677–3686. https://doi.org/10.1016/j.physa.2009.05.036 doi: 10.1016/j.physa.2009.05.036
![]() |
[32] |
G. Lan, S. Yuan, B. Song, The impact of hospital resources and environmental perturbations to the dynamics of SIRS model, J. Franklin Inst., 358 (2021), 2405–2433. https://doi.org/https://doi.org/10.1016/j.jfranklin.2021.01.015 doi: 10.1016/j.jfranklin.2021.01.015
![]() |
[33] |
T. Tuong, H. Dang, N. Dieu, K. Tran, Extinction and permanence in a stochastic SIRS model in regime-switching with general incidence rate, Nonlinear Anal. Hybri., 34 (2019), 121–130. https://doi.org/10.1016/j.nahs.2019.05.008 doi: 10.1016/j.nahs.2019.05.008
![]() |
[34] |
M. Benaïm, C. Lobry, Lotka-volterra with randomly fluctuating environments or how switching between beneficial environments can make survival harder, Ann. Appl. Probab., 26 (2016), 3754–3785. https://doi.org/10.1214/16-AAP1192 doi: 10.1214/16-AAP1192
![]() |
[35] | M. Benaïm, Stochastic persistence, arXiv: 1806.08450. |
[36] |
A. Hening, H. D. Nguyen, Coexistence and extinction for stochastic kolmogorov systems, Ann. Appl. Probab., 28 (2018), 1893–1942. https://doi.org/10.1214/17-AAP1347 doi: 10.1214/17-AAP1347
![]() |
[37] | X. Mao, Stochastic Differential Equations and Applications, Horwood Publishing, Chichester, 2007. |
[38] |
N. Dang, G. Yin, Stability of regime-switching diffusion systems with discrete states belonging to a countable set, SIAM J. Control Optim., 56 (2018), 3893–3917. https://doi.org/10.1137/17M1118476 doi: 10.1137/17M1118476
![]() |
[39] | F. Klebaner, Introduction to Stochastic Calculus with Applications, Imperial College Press, 2005. |
[40] |
R. Ikram, A. Khan, M. Zahri, A. Saeed, M. Yavuz, P. Kumam, Extinction and stationary distribution of a stochastic COVID-19 epidemic model with time-delay, Comput. Biol. Medi., 141 (2022), 105115. https://doi.org/10.1016/j.compbiomed.2021.105115 doi: 10.1016/j.compbiomed.2021.105115
![]() |
[41] |
D. Higham, An algorithmic introduction to numerical simulation of stochastic differential equations, SIAM Rev., 43 (2001), 525–546. https://doi.org/10.1137/S0036144500378302 doi: 10.1137/S0036144500378302
![]() |
[42] |
A. Din, Y. Li, Stationary distribution extinction and optimal control for the stochastic hepatitis B epidemic model with partial immunity, Phys. Scripta, 7 (2021), 074005. https://doi.org/10.1088/1402-4896/abfacc doi: 10.1088/1402-4896/abfacc
![]() |
[43] |
A. Din, Y. Li, Stochastic optimal control for norovirus transmission dynamics by contaminated food and water, Chinese Phys. B, 2021. https://doi.org/10.1088/1674-1056/ac2f32 doi: 10.1088/1674-1056/ac2f32
![]() |
[44] |
A. Din, The stochastic bifurcation analysis and stochastic delayed optimal control for epidemic model with general incidence function, Chaos, 31 (2021), 104649. https://doi.org/10.1063/5.0063050 doi: 10.1063/5.0063050
![]() |
[45] |
A. Yang, B. Song, S. Yuan, Noise-induced transitions in a non-smooth SIS epidemic model with media alert, Math. Biosci. Eng., 18 (2021), 745–763. https://doi.org/10.3934/mbe.2021040 doi: 10.3934/mbe.2021040
![]() |
[46] |
J. Jaramillo, J. Ma, P. Driessche, S. Yuan, Host contact structure is important for the recurrence of Influenza A, J. Math. Biol., 77 (2018), 1563–1588. https://doi.org/10.1007/s00285-018-1263-5 doi: 10.1007/s00285-018-1263-5
![]() |
[47] |
Y. Zhao, L. Zhang, S. Yuan, The effect of media coverage on threshold dynamics for a stochastic SIS epidemic model, Physica A, 512 (2018), 248–260. https://doi.org/10.1016/j.physa.2018.08.113 doi: 10.1016/j.physa.2018.08.113
![]() |
1. | Duane C. Harris, Changhan He, Mark C. Preul, Eric J. Kostelich, Yang Kuang, Critical Patch Size of a Two-Population Reaction Diffusion Model Describing Brain Tumor Growth, 2024, 84, 0036-1399, S249, 10.1137/22M1509631 |
Levels | D1 | D2 | ρ1 | k1 | N1 | N2 |
1 | 0.2000 | 0.1000 | 0.0038 | 0.0038 | 0.3000 | 0.0600 |
2 | 0.2182 | 0.1091 | 0.0047 | 0.0047 | 0.3500 | 0.0700 |
3 | 0.2364 | 0.1182 | 0.0056 | 0.0056 | 0.4000 | 0.0800 |
4 | 0.2545 | 0.1273 | 0.0068 | 0.0068 | 0.4500 | 0.0900 |
5 | 0.2727 | 0.1364 | 0.0083 | 0.0083 | 0.5000 | 0.1000 |
6 | 0.2909 | 0.1455 | 0.0101 | 0.0101 | 0.5500 | 0.1100 |
7 | 0.3091 | 0.1545 | 0.0122 | 0.0122 | 0.6000 | 0.1200 |
8 | 0.3273 | 0.1636 | 0.0148 | 0.0148 | 0.6500 | 0.1300 |
9 | 0.3455 | 0.1727 | 0.0178 | 0.0178 | 0.7000 | 0.1400 |
10 | 0.3636 | 0.1818 | 0.0217 | 0.0217 | 0.7500 | 0.1600 |
11 | 0.3818 | 0.1909 | 0.0257 | 0.0257 | 0.8000 | 0.1800 |
12 | 0.4000 | 0.2000 | 0.0315 | 0.0315 | 0.8500 | 0.2000 |
Levels | ρ2 | k2 | N3 |
1 | 0.0032 | 0.0032 | 0.0100 |
2 | 0.0038 | 0.0038 | 0.0120 |
3 | 0.0047 | 0.0047 | 0.0140 |
4 | 0.0056 | 0.0056 | 0.0160 |
5 | 0.0068 | 0.0068 | 0.0180 |
6 | 0.0083 | 0.0083 | 0.0200 |
7 | 0.0101 | 0.0101 | 0.0220 |
8 | 0.0122 | 0.0122 | 0.0240 |
9 | 0.0148 | 0.0148 | 0.0260 |
10 | 0.0178 | 0.0178 | 0.0280 |
11 | 0.0217 | 0.0217 | 0.0300 |
12 | 0.0257 | 0.0257 | 0.0320 |
Containment | Agreement | |||||||
Model | Min | Max | Average | Std Dev | Min | Max | Average | Std Dev |
Fisher | 0.19 | 0.45 | 0.29 | 0.06 | 0.10 | 0.29 | 0.20 | 0.04 |
ASUB | 0.13 | 0.46 | 0.27 | 0.07 | 0.09 | 0.31 | 0.21 | 0.05 |
Containment | Agreement | |||||||
Model | Min | Max | Average | Std Dev | Min | Max | Average | Std Dev |
Fisher | 0.51 | 0.64 | 0.59 | 0.03 | 0.14 | 0.25 | 0.18 | 0.02 |
ASUB | 0.39 | 0.64 | 0.57 | 0.05 | 0.15 | 0.33 | 0.22 | 0.04 |
Fisher | ASUB | |||||||
Patient/Time | Min | Max | Avg | StD | Min | Max | Avg | StD |
1 / 56-114 | 0.18 | 0.41 | 0.28 | 0.05 | 0.08 | 0.38 | 0.25 | 0.06 |
1 / 114-142 | 0.16 | 0.45 | 0.23 | 0.04 | 0.14 | 0.53 | 0.23 | 0.05 |
1 / 142-168 | 0.31 | 0.85 | 0.39 | 0.09 | 0.27 | 0.86 | 0.38 | 0.07 |
2 / 56-158 | 0.06 | 0.64 | 0.43 | 0.15 | 0.02 | 0.57 | 0.26 | 0.17 |
3 / 182-238 | 0.22 | 0.48 | 0.35 | 0.06 | 0.12 | 0.44 | 0.27 | 0.08 |
3 / 238-266 | 0.11 | 0.33 | 0.18 | 0.05 | 0.11 | 0.41 | 0.22 | 0.06 |
4 / 107-168 | 0.56 | 0.76 | 0.66 | 0.05 | 0.29 | 0.76 | 0.58 | 0.10 |
5 / 118-180 | 0.09 | 0.21 | 0.17 | 0.03 | 0.06 | 0.20 | 0.13 | 0.03 |
5 / 266-329 | 0.31 | 0.52 | 0.38 | 0.05 | 0.22 | 0.48 | 0.33 | 0.04 |
6 / 0- 61 | 0.21 | 0.33 | 0.26 | 0.03 | 0.13 | 0.34 | 0.24 | 0.05 |
6 / 61-112 | 0.20 | 0.31 | 0.25 | 0.02 | 0.12 | 0.32 | 0.24 | 0.05 |
7 / 0- 58 | 0.12 | 0.40 | 0.26 | 0.07 | 0.05 | 0.37 | 0.18 | 0.07 |
7 / 58- 83 | 0.16 | 0.56 | 0.24 | 0.09 | 0.15 | 0.55 | 0.22 | 0.07 |
8 / 93-144 | 0.02 | 0.19 | 0.13 | 0.02 | 0.09 | 0.23 | 0.15 | 0.03 |
9 / 80-182 | 0.00 | 0.03 | 0.02 | 0.01 | 0.00 | 0.06 | 0.03 | 0.01 |
9 / 182-245 | 0.03 | 0.12 | 0.07 | 0.02 | 0.02 | 0.11 | 0.07 | 0.02 |
10 / 136-215 | 0.44 | 0.68 | 0.61 | 0.05 | 0.32 | 0.69 | 0.56 | 0.08 |
Fisher | ASUB | |||||||
Patient/Time | Min | Max | Avg | StD | Min | Max | Avg | StD |
1 / 56-114 | 0.18 | 0.33 | 0.25 | 0.03 | 0.08 | 0.36 | 0.24 | 0.06 |
1 / 114-142 | 0.16 | 0.43 | 0.23 | 0.04 | 0.14 | 0.48 | 0.23 | 0.05 |
1 / 142-168 | 0.31 | 0.55 | 0.37 | 0.05 | 0.27 | 0.51 | 0.37 | 0.05 |
2 / 56-158 | 0.06 | 0.36 | 0.29 | 0.08 | 0.02 | 0.38 | 0.21 | 0.11 |
3 / 182-238 | 0.16 | 0.32 | 0.26 | 0.03 | 0.11 | 0.31 | 0.23 | 0.05 |
3 / 238-266 | 0.11 | 0.28 | 0.18 | 0.04 | 0.11 | 0.34 | 0.21 | 0.06 |
4 / 107-168 | 0.14 | 0.62 | 0.46 | 0.13 | 0.22 | 0.62 | 0.50 | 0.09 |
5 / 118-180 | 0.08 | 0.13 | 0.11 | 0.01 | 0.06 | 0.14 | 0.11 | 0.02 |
5 / 266-329 | 0.05 | 0.20 | 0.16 | 0.04 | 0.08 | 0.21 | 0.19 | 0.02 |
6 / 0- 61 | 0.09 | 0.26 | 0.18 | 0.04 | 0.12 | 0.29 | 0.20 | 0.03 |
6 / 61-112 | 0.11 | 0.26 | 0.21 | 0.03 | 0.12 | 0.27 | 0.20 | 0.04 |
7 / 0- 58 | 0.12 | 0.23 | 0.17 | 0.02 | 0.05 | 0.22 | 0.15 | 0.04 |
7 / 58- 83 | 0.10 | 0.25 | 0.18 | 0.03 | 0.10 | 0.28 | 0.19 | 0.03 |
8 / 93-144 | 0.01 | 0.18 | 0.11 | 0.03 | 0.08 | 0.21 | 0.13 | 0.02 |
9 / 80-182 | 0.00 | 0.02 | 0.01 | 0.01 | 0.00 | 0.05 | 0.03 | 0.01 |
9 / 182-245 | 0.03 | 0.11 | 0.06 | 0.02 | 0.02 | 0.10 | 0.07 | 0.02 |
10 / 136-215 | 0.01 | 0.24 | 0.07 | 0.07 | 0.01 | 0.29 | 0.17 | 0.08 |
Levels | D1 | D2 | ρ1 | k1 | N1 | N2 |
1 | 0.2000 | 0.1000 | 0.0038 | 0.0038 | 0.3000 | 0.0600 |
2 | 0.2182 | 0.1091 | 0.0047 | 0.0047 | 0.3500 | 0.0700 |
3 | 0.2364 | 0.1182 | 0.0056 | 0.0056 | 0.4000 | 0.0800 |
4 | 0.2545 | 0.1273 | 0.0068 | 0.0068 | 0.4500 | 0.0900 |
5 | 0.2727 | 0.1364 | 0.0083 | 0.0083 | 0.5000 | 0.1000 |
6 | 0.2909 | 0.1455 | 0.0101 | 0.0101 | 0.5500 | 0.1100 |
7 | 0.3091 | 0.1545 | 0.0122 | 0.0122 | 0.6000 | 0.1200 |
8 | 0.3273 | 0.1636 | 0.0148 | 0.0148 | 0.6500 | 0.1300 |
9 | 0.3455 | 0.1727 | 0.0178 | 0.0178 | 0.7000 | 0.1400 |
10 | 0.3636 | 0.1818 | 0.0217 | 0.0217 | 0.7500 | 0.1600 |
11 | 0.3818 | 0.1909 | 0.0257 | 0.0257 | 0.8000 | 0.1800 |
12 | 0.4000 | 0.2000 | 0.0315 | 0.0315 | 0.8500 | 0.2000 |
Levels | ρ2 | k2 | N3 |
1 | 0.0032 | 0.0032 | 0.0100 |
2 | 0.0038 | 0.0038 | 0.0120 |
3 | 0.0047 | 0.0047 | 0.0140 |
4 | 0.0056 | 0.0056 | 0.0160 |
5 | 0.0068 | 0.0068 | 0.0180 |
6 | 0.0083 | 0.0083 | 0.0200 |
7 | 0.0101 | 0.0101 | 0.0220 |
8 | 0.0122 | 0.0122 | 0.0240 |
9 | 0.0148 | 0.0148 | 0.0260 |
10 | 0.0178 | 0.0178 | 0.0280 |
11 | 0.0217 | 0.0217 | 0.0300 |
12 | 0.0257 | 0.0257 | 0.0320 |
Containment | Agreement | |||||||
Model | Min | Max | Average | Std Dev | Min | Max | Average | Std Dev |
Fisher | 0.19 | 0.45 | 0.29 | 0.06 | 0.10 | 0.29 | 0.20 | 0.04 |
ASUB | 0.13 | 0.46 | 0.27 | 0.07 | 0.09 | 0.31 | 0.21 | 0.05 |
Containment | Agreement | |||||||
Model | Min | Max | Average | Std Dev | Min | Max | Average | Std Dev |
Fisher | 0.51 | 0.64 | 0.59 | 0.03 | 0.14 | 0.25 | 0.18 | 0.02 |
ASUB | 0.39 | 0.64 | 0.57 | 0.05 | 0.15 | 0.33 | 0.22 | 0.04 |
Fisher | ASUB | |||||||
Patient/Time | Min | Max | Avg | StD | Min | Max | Avg | StD |
1 / 56-114 | 0.18 | 0.41 | 0.28 | 0.05 | 0.08 | 0.38 | 0.25 | 0.06 |
1 / 114-142 | 0.16 | 0.45 | 0.23 | 0.04 | 0.14 | 0.53 | 0.23 | 0.05 |
1 / 142-168 | 0.31 | 0.85 | 0.39 | 0.09 | 0.27 | 0.86 | 0.38 | 0.07 |
2 / 56-158 | 0.06 | 0.64 | 0.43 | 0.15 | 0.02 | 0.57 | 0.26 | 0.17 |
3 / 182-238 | 0.22 | 0.48 | 0.35 | 0.06 | 0.12 | 0.44 | 0.27 | 0.08 |
3 / 238-266 | 0.11 | 0.33 | 0.18 | 0.05 | 0.11 | 0.41 | 0.22 | 0.06 |
4 / 107-168 | 0.56 | 0.76 | 0.66 | 0.05 | 0.29 | 0.76 | 0.58 | 0.10 |
5 / 118-180 | 0.09 | 0.21 | 0.17 | 0.03 | 0.06 | 0.20 | 0.13 | 0.03 |
5 / 266-329 | 0.31 | 0.52 | 0.38 | 0.05 | 0.22 | 0.48 | 0.33 | 0.04 |
6 / 0- 61 | 0.21 | 0.33 | 0.26 | 0.03 | 0.13 | 0.34 | 0.24 | 0.05 |
6 / 61-112 | 0.20 | 0.31 | 0.25 | 0.02 | 0.12 | 0.32 | 0.24 | 0.05 |
7 / 0- 58 | 0.12 | 0.40 | 0.26 | 0.07 | 0.05 | 0.37 | 0.18 | 0.07 |
7 / 58- 83 | 0.16 | 0.56 | 0.24 | 0.09 | 0.15 | 0.55 | 0.22 | 0.07 |
8 / 93-144 | 0.02 | 0.19 | 0.13 | 0.02 | 0.09 | 0.23 | 0.15 | 0.03 |
9 / 80-182 | 0.00 | 0.03 | 0.02 | 0.01 | 0.00 | 0.06 | 0.03 | 0.01 |
9 / 182-245 | 0.03 | 0.12 | 0.07 | 0.02 | 0.02 | 0.11 | 0.07 | 0.02 |
10 / 136-215 | 0.44 | 0.68 | 0.61 | 0.05 | 0.32 | 0.69 | 0.56 | 0.08 |
Fisher | ASUB | |||||||
Patient/Time | Min | Max | Avg | StD | Min | Max | Avg | StD |
1 / 56-114 | 0.18 | 0.33 | 0.25 | 0.03 | 0.08 | 0.36 | 0.24 | 0.06 |
1 / 114-142 | 0.16 | 0.43 | 0.23 | 0.04 | 0.14 | 0.48 | 0.23 | 0.05 |
1 / 142-168 | 0.31 | 0.55 | 0.37 | 0.05 | 0.27 | 0.51 | 0.37 | 0.05 |
2 / 56-158 | 0.06 | 0.36 | 0.29 | 0.08 | 0.02 | 0.38 | 0.21 | 0.11 |
3 / 182-238 | 0.16 | 0.32 | 0.26 | 0.03 | 0.11 | 0.31 | 0.23 | 0.05 |
3 / 238-266 | 0.11 | 0.28 | 0.18 | 0.04 | 0.11 | 0.34 | 0.21 | 0.06 |
4 / 107-168 | 0.14 | 0.62 | 0.46 | 0.13 | 0.22 | 0.62 | 0.50 | 0.09 |
5 / 118-180 | 0.08 | 0.13 | 0.11 | 0.01 | 0.06 | 0.14 | 0.11 | 0.02 |
5 / 266-329 | 0.05 | 0.20 | 0.16 | 0.04 | 0.08 | 0.21 | 0.19 | 0.02 |
6 / 0- 61 | 0.09 | 0.26 | 0.18 | 0.04 | 0.12 | 0.29 | 0.20 | 0.03 |
6 / 61-112 | 0.11 | 0.26 | 0.21 | 0.03 | 0.12 | 0.27 | 0.20 | 0.04 |
7 / 0- 58 | 0.12 | 0.23 | 0.17 | 0.02 | 0.05 | 0.22 | 0.15 | 0.04 |
7 / 58- 83 | 0.10 | 0.25 | 0.18 | 0.03 | 0.10 | 0.28 | 0.19 | 0.03 |
8 / 93-144 | 0.01 | 0.18 | 0.11 | 0.03 | 0.08 | 0.21 | 0.13 | 0.02 |
9 / 80-182 | 0.00 | 0.02 | 0.01 | 0.01 | 0.00 | 0.05 | 0.03 | 0.01 |
9 / 182-245 | 0.03 | 0.11 | 0.06 | 0.02 | 0.02 | 0.10 | 0.07 | 0.02 |
10 / 136-215 | 0.01 | 0.24 | 0.07 | 0.07 | 0.01 | 0.29 | 0.17 | 0.08 |