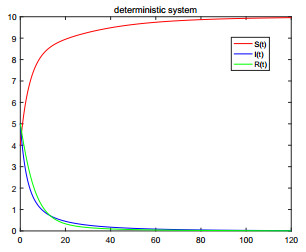
Citation: Yang Chen, Wencai Zhao. Dynamical analysis of a stochastic SIRS epidemic model with saturating contact rate[J]. Mathematical Biosciences and Engineering, 2020, 17(5): 5925-5943. doi: 10.3934/mbe.2020316
[1] | Zhongwei Cao, Jian Zhang, Huishuang Su, Li Zu . Threshold dynamics of a stochastic general SIRS epidemic model with migration. Mathematical Biosciences and Engineering, 2023, 20(6): 11212-11237. doi: 10.3934/mbe.2023497 |
[2] | Yanmei Wang, Guirong Liu . Dynamics analysis of a stochastic SIRS epidemic model with nonlinear incidence rate and transfer from infectious to susceptible. Mathematical Biosciences and Engineering, 2019, 16(5): 6047-6070. doi: 10.3934/mbe.2019303 |
[3] | Yoichi Enatsu, Yukihiko Nakata . Stability and bifurcation analysis of epidemic models with saturated incidence rates: An application to a nonmonotone incidence rate. Mathematical Biosciences and Engineering, 2014, 11(4): 785-805. doi: 10.3934/mbe.2014.11.785 |
[4] | Tingting Xue, Xiaolin Fan, Zhiguo Chang . Dynamics of a stochastic SIRS epidemic model with standard incidence and vaccination. Mathematical Biosciences and Engineering, 2022, 19(10): 10618-10636. doi: 10.3934/mbe.2022496 |
[5] | Yu Zhu, Liang Wang, Zhipeng Qiu . Threshold behaviour of a stochastic SIRS $ \mathrm {L\acute{e}vy} $ jump model with saturated incidence and vaccination. Mathematical Biosciences and Engineering, 2023, 20(1): 1402-1419. doi: 10.3934/mbe.2023063 |
[6] | Qingshan Yang, Xuerong Mao . Stochastic dynamics of SIRS epidemic models withrandom perturbation. Mathematical Biosciences and Engineering, 2014, 11(4): 1003-1025. doi: 10.3934/mbe.2014.11.1003 |
[7] | Fang Zhang, Wenzhe Cui, Yanfei Dai, Yulin Zhao . Bifurcations of an SIRS epidemic model with a general saturated incidence rate. Mathematical Biosciences and Engineering, 2022, 19(11): 10710-10730. doi: 10.3934/mbe.2022501 |
[8] | Andrea Franceschetti, Andrea Pugliese, Dimitri Breda . Multiple endemic states in age-structured $SIR$ epidemic models. Mathematical Biosciences and Engineering, 2012, 9(3): 577-599. doi: 10.3934/mbe.2012.9.577 |
[9] | Luis F. Gordillo, Stephen A. Marion, Priscilla E. Greenwood . The effect of patterns of infectiousness on epidemic size. Mathematical Biosciences and Engineering, 2008, 5(3): 429-435. doi: 10.3934/mbe.2008.5.429 |
[10] | Tingting Ding, Tongqian Zhang . Asymptotic behavior of the solutions for a stochastic SIRS model with information intervention. Mathematical Biosciences and Engineering, 2022, 19(7): 6940-6961. doi: 10.3934/mbe.2022327 |
Recently, a new type of pneumonia caused by the coronavirus, named COVID-19, is spreading around the world. The issue of infectious diseases has once again aroused people's great concern. How to prevent and control infectious diseases has been an important subject facing human beings[1,2,3,4,5,6,7,8]. The SIR model assumes that the infected person can obtain permanent immunity after recovery. However, for smallpox, cholera, malaria and other diseases, individuals recovered from treatment can return to the susceptible category after temporary immunization, which can be described by SIRS model. Moreover, for some bacterial infectious diseases, such as meningitis and sexually transmitted diseases, some individuals can not produce effective antibodies after treatment and may be infected again. Others may gain temporary immunity, but then lose immunity and become susceptible [9,10,11,12]. Literature [10] established an SIRS model with a general population-size dependent contact rate λ(N) and proportional transfer rate from the infective class to susceptible class. The authors studied the threshold conditions of disease extinction and discussed the stability of disease-free equilibrium and endemic equilibrium.
Infection rate is an important index to measure the intensity of disease transmission. Employing an appropriate infection rate based on a specific disease for the mathematical model plays a vital role in the disease prevention and control. In the literature [13], Thieme and Castillo-Chavez proposed the incidence βϱ(N(t))S(t)I(t)N(t), where N(t) represents the total population. On that basis, Heesterbeek et al. [14] gave the following saturating contact rate
ϱ(N(t))=bN(t)1+bN(t)+√1+2bN(t). |
Obviously, ϱ(N(t)) is a non-decreasing function of N(t). ϱ(N(t))N(t) is a non-increasing function of N(t). If N(t) is sufficiently small, ϱ(N(t))∼bN. Conversly, if N(t) is fully large, ϱ(N(t))∼1. Compared with the bilinear incidence βS(t)I(t) and the standard incidence βS(t)I(t)N(t), the saturating contact rate is more closer to the transmission of many diseases. The saturated contact rate is widely used in the study of infectious disease modeling. For example, Zhang et al.[15] constructed an SEIS model with general saturated incidence rate, and proved the global asymptotic stability of the endemic equilibrium by using the autonomous convergence theorem. Lan et al.[16] considered an SIS epidemic model with saturating contact rate, by using Itô's formula, the conditions for disease extinction and the existence of stationary solutions were obtained. In reference [11], Li et al. established an SIRS epidemic model with a general incidence, which considered both the transfer from the infected to the susceptible and the transfer from the recovered to the susceptible. Motivated by the above literature, we formulate a deterministic SIRS epidemic model with saturating contact rate and transfer from infectious to susceptible:
{dS(t)dt=Λ−uS(t)−βbS(t)I(t)g(N(t))+γ1I(t)+δR(t),dI(t)dt=βbS(t)I(t)g(N(t))−(u+γ1+γ2+α)I(t),dR(t)dt=γ2I(t)−(u+δ)R(t), | (1.1) |
where g(N(t))=1+bN(t)+√1+2bN(t), N(t)=S(t)+I(t)+R(t) is the total population. S(t), I(t), R(t) represent the number of susceptible individuals, infected individuals and recovered individuals, respectively. Λ is the recruitment rate of susceptible individuals. u denotes the natural mortality rate. α represents the mortality rate caused by diseases. γ1 is the transfer rate from the infected individuals to the susceptible individuals. γ2 is the transfer rate from the infected individuals to the recovered individuals, and δ denotes the immunity loss rate.
Due to the influence of environmental noise, the prevalence and transmission of diseases is often random. For example, the change of temperature and the influence of climate will lead to the fluctuation of mortality, morbidity and so on. In recent years, mathematical models of infectious diseases described by stochastic differential equations have been widely concerned [17,18,19,20,21,22]. There are many ways to construct a stochastic differential equation model, such as adding random perturbations to the parameters of deterministic system [23,24,25,26], or introducing proportional perturbations to state variables [27,28,29,30,31]. Recently, considering the effect of two different white noises on the model parameters, reference [32] established a stochastic SIS model with two correlated Brownian motions, in which the threshold of disease extinction as well as the variance and mean of the stationary distribution were investigated. In this paper, we consider that the incidence coefficient βb is disturbed by white noise, that is βb→βb+σdB(t), where σ2 is the intensity of white noise, B(t) is defined as the standard Brownian motion in the complete probability space (Ω,F,{F}t≥0,P). Thus, the above model (1.1) is transformed into the following SIRS stochastic epidemic model:
{dS(t)=[Λ−uS(t)−βbS(t)I(t)g(N(t))+γ1I(t)+δR(t)]dt−σS(t)I(t)g(N(t))dB(t),dI(t)=[βbS(t)I(t)g(N(t))−(u+γ1+γ2+α)I(t)]dt+σS(t)I(t)g(N(t))dB(t),dR(t)=[γ2I(t)−(u+δ)R(t)]dt. | (1.2) |
As far as we know, there have been a lot of studies on the epidemic model disturbed by environmental noise, but there are few stochastic models considering saturating contact rate and transfer from infectious to susceptible, especially the existence of stationary solution. The paper is organized as follows: In section 2, we give some notations and related lemmas. The thresholds of deterministic system and stochastic system are established in sections 3 and 4, respectively. Sufficient condition for the existence of stationary solution in the stochastic system (1.2) is provided in section 5. In section 6, we verify the results of theoretical derivation by numerical simulations.
Throughout this paper, we let R3+={xi>0,i=1,2,3}. For an integrable function h on [0,+∞), we define ⟨h(t)⟩=1t∫t0h(π)dπ. By using the methods from Liu et al. [33], we can prove that the region
Γ={(S(t),I(t),R(t))∈R3+,Λu+α≤N(t)≤Λu} |
is a positively invariant set of system (1.2).
Lemma 2.1. For any given initial value (S(0),I(0),R(0))∈R3+, then the model (1.2) has a unique positive solution (S(t),I(t),R(t)) on t≥0, and the solution will remain in R3+ with probability 1.
Next, we will introduce some contents of stationary Markov process. The n-dimensional stochastic differential equation can be expressed by the following formula
dx(t)=f(x(t),t)dt+l∑i=1gi(x(t),t)dBi(t),∀t≥t0, | (2.1) |
with the initial value x(t0)=x0∈Rn. Integrating from 0 to t for both sides of the Eq (2.1), one can obtain that
x(t)=x0+∫tt0f(x(θ),θ)dθ+l∑i=1∫tt0gi(x(θ),θ)dBi(θ),∀t≥t0. | (2.2) |
Assume that the vectors f(x,t), g1(x,t), ..., gl(x,t)(t≥t0,x∈Rn) are continuous functions of (x,t), satisfying the following conditions for some constant D,
(A1)|f(x,t)−f(y,t)|+l∑i=1|gi(x,t)−gi(y,t)|≤D|x−y|,(A2)|f(x,t)|+l∑i=1|gi(x,t)|≤D(1+|x|). | (2.3) |
According to the literature [34] Theorem 3.7, we have the following Lemma.
Lemma 2.2. Suppose that the coefficients of (2.2) are independent of t, and the conditions (2.3) hold in UG(∀G>0). There exists a function V(x)∈C2 with the following properties in Rn
V(x)≥0andsup|x|>GLV(x)=−MG→−∞(G→∞), | (2.4) |
where C2 represents a class of functions that are twice continuously differentiable relative to x in Rn. Further, we assume that there is at least one x∈Rn, such that the process Xx(t) is regular. Then there exists a solution of system (2.2) which is a stationary Markov process.
For Lemma 2.2, we need to note the following two points.
Remark 2.1 (ⅰ) Condition (2.3) can be replaced by the global existence of solution of system (2.2) (see [35] Remark 5);
(ⅱ) Condition (2.4) can be replaced by the weaker condition LV(x)≤−1 (see [34] Chapter 4).
In this section, we concentrate on the stability of the equilibria. Firstly, using N(t) as a variable instead of the variable S(t), we convert system (1.1) into the following form
{dN(t)dt=Λ−uN(t)−αI(t),dI(t)dt=βbI(t)g(N(t))(N(t)−I(t)−R(t))−(u+γ1+γ2+α)I(t),dR(t)dt=γ2I(t)−(u+δ)R(t). | (3.1) |
Obviously, the system (3.1) exists a boundary equilibrium P0=(Λu,0,0). Define
R0=Λβbu(u+γ1+γ2+α)g(Λu). |
By direct calculation, if R0>1, we get system (3.1) has a positive equilibrium P∗=(N∗,I∗,R∗) with
I∗=Λ−uN∗α,R∗=γ2(Λ−uN∗)α(u+δ), |
where N∗ is the unique positive root of the following function
ϕ(N)=βb[N−Λ−uNα−γ2(Λ−uN)α(u+δ)]−(u+γ1+γ2+α)g(N). |
In fact, we have
ϕ(Λu)=(u+γ1+γ2+α)g(Λu)(R0−1)>0 |
and
ϕ(Λu+α)=−βbγ2Λ(u+α)(u+δ)−(u+γ1+γ2+α)g(Λu+α)<0. |
Let dϕ(N)dN=0, it can be got
N∗∗={α(u+δ)(u+γ1+γ2+α)β[(α+u)(u+δ)+uγ2]−α(u+δ)(u+γ1+γ2+α)}2−12b, |
which implies that ϕ(N) is increasing if N≥N∗∗, and ϕ(N) is decreasing if N<N∗∗. Therefore, function ϕ(N) has a unique positive root, then the system (3.1) exists a unique positive equilibrium P∗=(N∗,I∗,R∗).
Theorem 3.1. For system (3.1), we have
(i) If R0<1, then P0=(Λu,0,0) is a unique stable equilibrium, which implies the disease of system (3.1) goes extinct.
(ii) If R0>1, then P∗=(N∗,I∗,R∗) is a stable positive equilibrium, which implies the disease of system (3.1) is permanent.
Proof. (ⅰ) The Jacobian matrix of system (3.1) evaluated at P0=(Λu,0,0) is
J0=[−u−α00Λβbug(Λu)−(u+γ1+γ2+α)00γ2−(u+δ)], |
which has three eigenvalues:
λ1=−u<0,λ2=−(u+δ)<0,λ3=(u+γ1+γ2+α)(R0−1)<0. |
Therefore, according to stability theory, P0 is stable if R0<1.
(ⅱ) The Jacobian matrix at P∗=(N∗,I∗,R∗) is
J∗=[−u−α0a21a22a230γ2−(u+δ)], |
where
a21=βbI∗[g(N∗)−(N∗−I∗−R∗)(b+b√1+2bN∗)]g2(N∗)=βbI∗(1+√1+2bN∗−bN∗√1+2bN∗)g2(N∗)+βbI∗(I∗+R∗)g2(N∗)(b+b√1+2bN∗)=βbI∗g(N∗)√1+2bN∗+βbI∗(I∗+R∗)g2(N∗)(b+b√1+2bN∗)>0,a22=βb(N∗−R∗−2I∗)g(N∗)−(u+γ1+γ2+α)=−βbI∗g(N∗)<0,a23=−βbI∗g(N∗)=a22<0. |
Hence, the characteristic equation of J∗ is
λ3+a1λ2+a2λ+a3=0, |
where
a1=u+βbI∗g(N∗)+u+δ>0,a2=α[βbI∗g(N∗)√1+2bN∗+βbI∗(I∗+R∗)g2(N∗)(b+b√1+2bN∗)]+(2u+δ+γ2)βbI∗g(N∗)+u(u+δ)>0 |
and
a3=α(u+δ)[βbI∗g(N∗)√1+2bN∗+βbI∗(I∗+R∗)g2(N∗)(b+b√1+2bN∗)]+u(u+δ+γ2)βbI∗g(N∗)>0. |
Then,
a1a2−a3=αua21−[(2u+δ)(2u+δ+γ2)+uγ2]a22−αa21a22+(2u+δ+γ2)a222+u(u+δ)(2u+δ)>0. |
Therefore, the equilibrium P∗ is stable when it exists. This completes the proof of Theorem 3.1.
In the previous section, we have obtained the threshold for ordinary differential equation (ODE) system (1.1). Similarly, the threshold of stochastic differential equation (SDE) system (1.2) is also crucial, which determines the extinction and persistence of the disease. {Define the following parameter
Rs0=Λβbu(u+γ1+γ2+α+σ2Λ22u2g2(Λu))g(Λu), |
where g(x)=1+bx+√1+2bx. In this section, we will prove that Rs0 is the threshold of system (1.2). Now, we give the following definition.
Definition 4.1.
(i) The disease I(t) is said to be extinct if limt→+∞I(t)=0;
(ii) The disease I(t) is said to be permanent in mean if there is a positive constant ϕ such that limt→∞inf⟨I(t)⟩≥ϕ.
Theorem 4.1. Set (S(t),I(t),R(t)) be a solution of system (1.2) with any given initial value (S(0),I(0),R(0))∈Γ.
(i) If σ2>max{β2b22(u+γ1+γ2+α),βbug(Λu)Λ} holds, then
limt→∞suplnI(t)t≤β2b22σ2−(u+γ1+γ2+α)<0a.s., |
(ii) If Rs0<1 and σ2<βbug(Λu)Λ holds, then
limt→∞suplnI(t)t≤(u+γ1+γ2+α+σ2Λ22u2g2(Λu))(Rs0−1)<0a.s., |
which implies that the disease dies out with probability 1. In addition, we have
limt→∞⟨S(t)⟩=Λua.s., |
limt→∞⟨R(t)⟩=0a.s.. |
Proof. Set ∼V=lnI(t), by the Itô's formula, one can get
d(lnI(t))=[βbS(t)g(N(t))−(u+γ1+γ2+α)−σ2S2(t)2g2(N(t))]dt+σS(t)g(N(t))dB(t)=Φ(S(t)g(N(t)))dt+σS(t)g(N(t))dB(t), | (4.1) |
where Φ(x)=−σ22x2+βbx−(u+γ1+γ2+α).
Case (ⅰ): If condition (ⅰ) is satisfied, then
Φ(S(t)g(N(t)))=−σ22(S(t)g(N(t))−βbσ2)2+β2b22σ2−(u+γ1+γ2+α)≤β2b22σ2−(u+γ1+γ2+α). | (4.2) |
Substituting (4.2) into (4.1), one can obtain that
d(lnI(t))≤[β2b22σ2−(u+γ1+γ2+α)]dt+σS(t)g(N(t))dB(t). | (4.3) |
By using the strong law of large numbers for martingales, we obtain
limt→∞∫t0σS(ξ)g(N(ξ))dξt=0. |
Integrating from 0 to t, dividing by t, and taking superior limit on both sides of Eq (4.3) yields
limt→∞suplnI(t)t≤β2b22σ2−(u+γ1+γ2+α)<0.a.s. | (4.4) |
Case (ⅱ): If condition (ⅱ) Rs0<1 and σ2<βbug(Λu)Λ are satisfied, then
Φ(S(t)g(N(t)))=−σ22(S(t)g(N(t))−βbσ2)2+β2b22σ2−(u+γ1+γ2+α)≤−σ22(Λug(Λu)−βbσ2)2+β2b22σ2−(u+γ1+γ2+α)=(u+γ1+γ2+α+σ2Λ22u2g2(Λu))(Rs0−1). | (4.5) |
From Eq (4.1), one can get
limt→∞suplnI(t)t≤(u+γ1+γ2+α+σ2Λ22u2g2(Λu))(Rs0−1)<0a.s.. | (4.6) |
The inequalities (4.4) and (4.6) imply limt→∞I(t)=0 and the disease goes to extinction.
Next, adding up the three equations of system (1.2), integrating both sides from 0 to t and dividing by t, one can see that
S(t)−S(0)t+I(t)−I(0)t+δu+δR(t)−R(0)t=Λ−u⟨S(t)⟩−(u+α+uγ2u+δ)⟨I(t)⟩. | (4.7) |
So, from Eq (4.7) we have
⟨S(t)⟩=Λu−(u+αu+γ2u+δ)⟨I(t)⟩−Θ(t), | (4.8) |
where Θ(t)=1u[S(t)−S(0)t+I(t)−I(0)t+δu+δR(t)−R(0)t]. Obviously, limt→∞Θ(t)=0 and we have
limt→∞⟨S(t)⟩=Λua.s.. |
Similarly, from the third equation of the system (1.2) yields
⟨R(t)⟩=γ2u+δ⟨I(t)⟩−R(t)−R(0)(u+δ)t. | (4.9) |
Let t→∞, we get that
limt→∞⟨R(t)⟩=0a.s. |
This finishes the proof.
Theorem 4.2. If Rs0>1, the disease is persistence in mean. Moreover, we have
limt→∞inf⟨I(t)⟩≥I∗∗>0,limt→∞inf⟨Λu−S(t)⟩≥(u+αu+γ2u+δ)I∗∗>0,limt→∞inf⟨R(t)⟩≥γ2u+δI∗∗>0, |
where
I∗∗=u(u+δ)[u+γ1+γ2+α+Λ2σ22u2g2(Λu)]g(Λu)(Rs0−1)βb[(u+α)(u+δ)+γ2u]. |
Proof. In view of Eq (4.1), we have
d(lnI(t))≥[βbS(t)g(Λu)−(u+γ1+γ2+α)−σ2Λ22u2g2(Λu)]dt+σS(t)g(N(t))dB(t). | (4.10) |
Integrating from 0 to t and dividing by t on both sides of Eq (4.10), one can get that
lnI(t)−lnI(0)t≥βbg(Λu)⟨S(t)⟩−(u+γ1+γ2+α)−σ2Λ22u2g2(Λu)+∫t0σS(ξ)g(N(ξ))dξt. | (4.11) |
Substituting Eq (4.8) into Eq (4.11), we can get
lnI(t)−lnI(0)t≥[u+γ1+γ2+α+σ2Λ22u2g2(Λu)](Rs0−1)−βbΘ(t)ug(Λu)+∫t0σS(ξ)g(N(ξ))dξt−βb[(u+α)(u+δ)+γ2u]u(u+δ)g(Λu)⟨I(t)⟩. | (4.12) |
Hence,
lnI(t)≥[u+γ1+γ2+α+σ2Λ22u2g2(Λu)](Rs0−1)t+F(t)−βb[(u+α)(u+δ)+γ2u]u(u+δ)g(Λu)∫t0I(ξ)dξ. | (4.13) |
where F(t)=lnI(0)+∫t0σS(ξ)g(N(ξ))dξ−βbtΘ(t)ug(Λu). Obviously, limt→∞F(t)t=0a.s.. From Lemma 1 in [12], we obtain
limt→∞inf⟨I(t)⟩≥u(u+δ)[u+γ1+γ2+α+Λ2σ22u2g2(Λu)]g(Λu)(Rs0−1)βb[(u+α)(u+δ)+γ2u]=I∗∗. |
According to Eqs (4.8) and (4.9), we can see that
limt→∞inf⟨Λu−S(t)⟩=(u+αu+γ2u+δ)limt→∞inf⟨I(t)⟩≥(u+αu+γ2u+δ)I∗∗ |
and
limt→∞inf⟨R(t)⟩=γ2u+δlimt→∞inf⟨I(t)⟩≥γ2u+δI∗∗. |
The proof of Theorem 4.2 is completed.
Remark 4.1. According to Theorem 4.1 (i), if the intensity of white noise is large enough that the condition σ2>max{β2b22(u+γ1+γ2+α),βbug(Λu)Λ} holds, then the disease goes to extinction. Therefore, a large environmental nose intensity can suppress the spread of disease. In addition, by comparing the thresholds of systems (1.2) and (1.1), it can be found that if the intensity of environmental noise σ2=0, then Rs0=R0; if σ2≠0, then Rs0<R0. When Rs0<1<R0, the deterministic system (1.1) has a stable positive equilibrium, while the disease of the stochastic system (1.2) dies out with probability 1. This means that the presence of environmental noise is conducive to disease control.
Theorem 5.1. If Rs0>1, there exists a solution of system (1.2) which is a stationary Markov process.
Proof. Let (S(t),I(t),R(t)) be a solution of system (1.2) with any given initial value (S(0),I(0),R(0))∈Γ. Then we construct a C2-function G as following:
G(S(t),I(t),R(t))=M(−lnI(t)−c1lnS(t)−c2g2(N(t))+4bc2g(Λu)u+δR(t))−lnS(t)−lnR(t)−ln(N(t)−Λu+α)−ln(Λu−N(t))=MV1+V2+V3+V4+V5, |
where V1=−lnI(t)−c1lnS(t)−c2g2(N(t))+4bc2g(Λu)u+δR(t), V2=−lnS(t), V3=−lnR(t), V4=−ln(N(t)−Λu+α), V5=−ln(Λu−N(t)), c1=u+γ1+γ2+α+Λ2σ22u2g2(Λu)u and c2=u+γ1+γ2+α+Λ2σ22u2g2(Λu)2bΛg(Λu). The M is a positive constant and satisfies the following condition
−3M[u+γ1+γ2+α+Λ2σ22u2g2(Λu)](3√Rs0−1)+βbΛug(Λu)+σ2Λ22u2g2(Λu)+4u+δ+α≤−2. | (5.1) |
Furthermore, G(S(t),I(t),R(t)) is a continuous function, which exists a minimum point (−S0,−I0,−R0). Next, we define a nonnegative C2-function V
V(S(t),I(t),R(t))=G(S(t),I(t),R(t))−G(−S0,−I0,−R0). |
Applying Itô's formula for V1, we can see
LV1=−1I(t)[βbS(t)I(t)g(N(t))−(u+γ1+γ2+α)I(t)]+σ2S2(t)g2(N(t))−c1S(t)[Λ−uS(t)−βbS(t)I(t)g(N(t))+γ1I(t)+δR(t)]+c1σ2I2(t)2g2(N(t))−2bc2g(N(t))(1+1√1+2bN(t))(Λ−uN(t)−αI(t))+4bc2g(Λu)u+δ[γ2I(t)−(u+δ)R(t)]≤−βbS(t)g(N(t))−c1ΛS(t)−2bΛc2g(N(t))+(u+γ1+γ2+α)+Λ2σ22u2g2(Λu)+c1u+c1βbI(t)g(Λu+α)+c1σ2I2(t)2g2(Λu+α)+2bΛc2g(Λu)−4bc2g(Λu)R(t)+2bαc2g(N(t))(1+1√1+2bN(t))I(t)+4bc2γ2g(Λu)u+δI(t)≤−33√2Λ2βb2c1c2+3[u+γ1+γ2+α+Λ2σ22u2g2(Λu)]+c1σ2I2(t)2g2(Λu+α)+[c1βbg(Λu+α)+4bαc2g(Λu)+4bc2γ2g(Λu)u+δ]I(t)=−3[u+γ1+γ2+α+Λ2σ22u2g2(Λu)](3√Rs0−1)+c1σ2I2(t)2g2(Λu+α)+[c1βbg(Λu+α)+4bc2g(Λu)(α+γ2u+δ)]I(t). | (5.2) |
Similarly, we have
LV2=−ΛS(t)+u+βbI(t)g(N(t))−γ1I(t)S(t)−δR(t)S(t)+σ2I2(t)2g2(N(t))≤−ΛS(t)+u+βbΛug(Λu)+σ2Λ22u2g2(Λu), | (5.3) |
LV3=−γ2I(t)R(t)+u+δ, | (5.4) |
LV4=−Λ−uN(t)−αI(t)N(t)−Λu+α=u+α−α(S(t)+R(t))N(t)−Λu+α, | (5.5) |
LV5=Λ−uN(t)−αI(t)Λu−N(t)=u−αI(t)Λu−N(t). | (5.6) |
Therefore, in view of Eqs (5.2)–(5.6), we get that
LV≤−3M[u+γ1+γ2+α+Λ2σ22u2g2(Λu)](3√Rs0−1)+Mc1σ2I2(t)2g2(Λu+α)−ΛS(t)+M[c1βbg(Λu+α)+4bc2g(Λu)(α+γ2u+δ)]I(t)+u+βbΛug(Λu)+σ2Λ22u2g2(Λu)−γ2I(t)R(t)+u+δ+u+α−α(S(t)+R(t))N(t)−Λu+α+u−αI(t)Λu−N(t)≤−2+M[c1βbg(Λu+α)+4bc2g(Λu)(α+γ2u+δ)]I(t)+Mc1σ2I2(t)2g2(Λu+α)−ΛS(t)−γ2I(t)R(t)−α(S(t)+R(t))N(t)−Λu+α−αI(t)Λu−N(t):=−2+MλI(t)+Mc1σ2I2(t)2g2(Λu+α)−ΛS(t)−γ2I(t)R(t)−α(S(t)+R(t))N(t)−Λu+α−αI(t)Λu−N(t), |
where λ=c1βbg(Λu+α)+4bc2g(Λu)(α+γ2u+δ).
Define the following bounded closed set
Dε={(S(t),I(t),R(t))∈Γ:ε≤S(t)≤Λu,ε≤I(t)≤Λu,ε2≤R(t)≤Λu, |
Λu+α+ε3≤N(t)≤Λu−ε3}, |
where ε is a sufficiently small constant satisfying the following inequalities (5.7)–(5.11)
−2+MλΛu+Mc1σ2Λ22u2g2(Λu+α)−Λε≤−1, | (5.7) |
−2+Mλε+Mc1σ2ε22g2(Λu+α)≤−1, | (5.8) |
−2+MλΛu+Mc1σ2Λ22u2g2(Λu+α)−γ2ε≤−1, | (5.9) |
−2+MλΛu+Mc1σ2Λ22u2g2(Λu+α)−α(1+ε)ε2≤−1, | (5.10) |
−2+MλΛu+Mc1σ2Λ22u2g2(Λu+α)−αε2≤−1. | (5.11) |
For convenience, we divide Γ∖Dε into five domains
D1={(S(t),I(t),R(t))∈Γ,0<S(t)<ε},D2={(S(t),I(t),R(t))∈Γ,0<I(t)<ε},D3={(S(t),I(t),R(t))∈Γ,I(t)≥ε,0<R(t)<ε2},D4={(S(t),I(t),R(t))∈Γ,S(t)≥ε,I(t)≥ε,R(t)≥ε2,Λu+α<N(t)<Λu+α+ε2},D5={(S(t),I(t),R(t))∈Γ,S(t)≥ε,I(t)≥ε,R(t)≥ε2,Λu−ε2<N(t)<Λu}. |
So, we only need to prove LV≤−1 on the above five domains.
Case ⅰ: If (S(t),I(t),R(t))∈D1, from Eq (5.7), one can obtain that
LV≤−2+MλI(t)+Mc1σ2I2(t)2g2(Λu+α)−ΛS(t)≤−2+MλΛu+Mc1σ2Λ22u2g2(Λu+α)−Λε≤−1. | (5.12) |
Case ⅱ: If (S(t),I(t),R(t))∈D2, in view of Eq (5.8), we have
LV≤−2+MλI(t)+Mc1σ2I2(t)2g2(Λu+α)≤−2+Mλε+Mc1σ2ε22g2(Λu+α)≤−1. | (5.13) |
Case ⅲ: If (S(t),I(t),R(t))∈D3, according to Eq (5.9), we obtain
LV≤−2+MλI(t)+Mc1σ2I2(t)2g2(Λu+α)−γ2I(t)R(t)≤−2+MλΛu+Mc1σ2Λ22u2g2(Λu+α)−γ2ε≤−1. | (5.14) |
Case ⅳ: If (S(t),I(t),R(t))∈D4, by Eq (5.10), one can see that
LV≤−2+MλI(t)+Mc1σ2I2(t)2g2(Λu+α)−α(S(t)+R(t))N(t)−Λu+α≤−2+MλΛu+Mc1σ2Λ22u2g2(Λu+α)−α(1+ε)ε2≤−1. | (5.15) |
Case ⅴ: If (S(t),I(t),R(t))∈D5, in view Eq (5.11), we derive
LV≤−2+MλI(t)+Mc1σ2I2(t)2g2(Λu+α)−αI(t)Λu−N(t)≤−2+MλΛu+Mc1σ2Λ22u2g2(Λu+α)−αε2≤−1. | (5.16) |
Consequently, for a sufficiently small ε, we have
LV(S(t),I(t),R(t))≤−1,for∀(S(t),I(t),R(t))∈Γ∖Dε. |
In view of Lemma 2.2, there exists a solution of system (1.2) which is a stationary Markov process. This completes the proof.
Remark 5.1. Theorem 5.1 shows that if the intensity of white noise is small enough to make Rs0>1, then the system (1.2) has a stationary solution. That is to say, disease will exist for a long time and form endemic disease.
In this paper, we study the dynamics of a stochastic SIRS epidemic model with saturating contact rate. Firstly, the threshold of disease extinction for deterministic system (1.1) is obtained by using the Jacobian matrix. If R0<1, system (1.1) has a unique stable equilibrium and the disease goes to extinct. If R0>1, system (1.1) forms endemic disease after a sufficiently long time. Secondly, the threshold parameter Rs0 of stochastic system (1.2) is established. If the intensity of the white noise is small enough to satisfy the condition Rs0>1, the disease is persistent. Otherwise, the disease will die out. Finally, we prove that there exists a stationary solution under condition Rs0>1 in system (1.2).
In order to demonstrate the above theoretical derivation, we use MATLAB software to carry out some numerical simulations. Next, we choose the relevant parameters of system (1.1) as follows:
Λ=1, u=0.1, b=0.6, δ=0.13, γ1=0.1, γ2=0.08, α=0.12. |
Firstly, we simulate the deterministic system and set β=0.66. By an ordinary computation, R0=0.9335<1. From the first condition of the Theorem 3.1, one can obtain that the system (1.1) has a unique stable equilibrium point E0=(10,0,0) (Figure 1). Furthermore, if we increase β to 0.8, in this case, we have R0=1.1315>1. The condition (ⅱ) in Theorem 3.1 is established, then by Theorem 3.1, the disease of system (1.1) is persistent (Figure 2).
Next, we perform numerical simulations on stochastic system. We keep the parameters of deterministic system (1.1) unchanged, and only select different intensities of white noise σ in system (1.2).
Case ⅰ: In order to verify the conclusion (ⅰ) of the Theorem 4.1, we let σ=0.8. By computing, we can obtain σ2=0.64, g(Λu)=10.6056 and max{β2b22(u+γ1+γ2+α),βbug(Λu)Λ}=0.5091, which implies the parameters satisfy the condition (ⅰ) σ2>max{β2b22(u+γ1+γ2+α),βbug(Λu)Λ}. This shows that the disease in system (1.2) dies out with probability 1 (Figure 3).
Case ⅱ: In Figure 4, we assume that σ=0.45. It is not difficult to obtain that σ satisfies the second condition of Theorem 4.1. {Then, we have Rs0=0.9236<1 and 0.2025=σ2<βbug(Λu)Λ=0.5091.} As can be seen from Figure 4, the disease is going extinct.
Case ⅲ: If σ=0.1, by calculation, we get Rs0=1.1190>1. According to Theorem 4.2, the disease in system (1.2) is permanent in the time mean (Figure 5). Furthermore, by Theorem 5.1, the system (1.2) has a stationary solution (Figure 6).
This work is supported by Shandong Provincial Natural Science Foundation of China (No. ZR2019MA003).
The authors declare that there is no conflict of interests regarding the publication of this paper.
[1] | S. Ullah, M. A. Khan, M. Farooq, T. Gul, Modeling and analysis of Tuberculosis (TB) in Khyber Pakhtunkhwa, Pakistan, Math. Comput. Simulation, 165 (2019), 181-199. |
[2] | G. Sun, J. Xie, S. Huang, Z. Jin, M. Li, L. Liu, Transmission dynamics of cholera: Mathematical modeling and control strategies, Commun. Nonlinear Sci. Numer. Simul., 45 (2017), 235-244. |
[3] | Y. Bai, X. Mu, Global asymptotic stability of a generalized SIRS epidemic model with transfer from infectious to susceptible, J. Appl. Anal. Comput., 8 (2018), 402-412. |
[4] | J. Lai, S. Gao, Y. Liu, X. Meng, Impulsive switching epidemic model with benign worm defense and quarantine strategy, Complexity, 540 (2020), 1-12. |
[5] | Q. Liu, D. Jiang, Threshold behavior in a stochastic SIR epidemic model with Logistic birth, Phys. A, 2020 (2020), 123488. |
[6] | D. Zhao, S. Yuan, Threshold dynamics of the stochastic epidemic model with jump-diffusion infection force, J. Appl. Anal. Comput., 9 (2019), 440-451. |
[7] | B. Zhang, Y. Cai, B. Wang, W. Wang, Dynamics and asymptotic profiles of steady states of an SIRS epidemic model in spatially heterogenous environment, Math. Biosci. Eng., 17 (2019), 893-909. |
[8] | Y. Tu, S. Gao, Y. Liu, D. Chen, Y. Xu, Transmission dynamics and optimal control of stagestructured HLB model, Math. Biosci. Eng., 16 (2019), 5180. |
[9] | H. W. Hethcote, Qualitative analyses of communicable disease models, Math. Biosci., 28 (1976), 335-356. |
[10] | J. Chen, An SIRS epidemic model, Appl. Math. J. Chinese Univ. Ser. B, 19 (2004), 101-108. |
[11] | T. Li, F. Zhang, H. Liu, Y. Chen, Threshold dynamics of an SIRS model with nonlinear incidence rate and transfer from infectious to susceptible, Appl. Math. Lett., 70 (2017), 52-57. |
[12] | Y. Wang, G. Liu, Dynamics analysis of a stochastic SIRS epidemic model with nonlinear incidence rate and transfer from infectious to susceptible, Math. Biosci. Eng., 16 (2019), 6047-6070. |
[13] | H. R. Thieme, C. Castillo-Chavez, On the role of variable infectivity in the dynamics of the human immunodeficiency virus epidemic, Mathematical and Statistical Approaches to AIDS Epidemiology, Springer, Berlin, 1989. |
[14] | J. Heesterbeek, J. A. Metz, The saturating contact rate in marriage and epidemic models, J. Math. Biol., 31 (1993), 529-539. |
[15] | H. Zhang, Y. Li, W. Xu, Global stability of an SEIS epidemic model with general saturation incidence, Appl. Math., 2013 (2013), 1-11. |
[16] | G. Lan, Y. Huang, C. Wei, S. Zhang, A stochastic SIS epidemic model with saturating contact rate, Phys. A, 529 (2019), 121504. |
[17] | Y. Cai, J. Jiao, Z. Gui, Y. Liu, W. Wang, Environmental variability in a stochastic epidemic model, Appl. Math. Comput., 329 (2018), 210-226. |
[18] | D. Kiouach, L. Boulaasair, Stationary distribution and dynamic behaviour of a stochastic SIVR epidemic model with imperfect vaccine, J. Appl. Math., 2018 (2018), 1-11. |
[19] | X. Zhang, H. Peng,, Stationary distribution of a stochastic cholera epidemic model with vaccination under regime switching, Appl. Math. Lett., 102 (2020), 106095. |
[20] | W. Wang, C. Ji, Y. Bi, S.Liu, Stability and asymptoticity of stochastic epidemic model with interim immune class and independent perturbations, Appl. Math. Lett., 104 (2020), 106245. |
[21] | R. Lu, F. Wei, Persistence and extinction for an age-structured stochastic SVIR epidemic model with generalized nonlinear incidence rate, Phys. A, 513 (2019), 572-587. |
[22] | T. Feng, Z. Qiu, X. Meng, Dynamics of a stochastic hepatitis-c virus system with host immunity, Discrete Contin. Dyn. Syst. Ser. B, 24 (2019), 6367. |
[23] | A. Gray, D. Greenhalgh, L. Hu, X. Mao, J. Pan, A stochastic differential equation SIS epidemic model, SIAM J. Appl. Math., 71 (2011), 876-902. |
[24] | W. Guo, Q. Zhang, X. Li, W. Wang, Dynamic behavior of a stochastic SIRS epidemic model with media coverage, Math. Methods Appl. Sci., 41 (2018), 5506-5525. |
[25] | Y. Zhang, K. Fan, S. Gao, Y. Liu, S. Chen., Ergodic stationary distribution of a stochastic SIRS epidemic model incorporating media coverage and saturated incidence rate, Phys. A, 514 (2019), 671-685. |
[26] | Y. Cai, Y. Kang, M. Banerjee, W. Wang, A stochastic SIRS epidemic model with infectious force under intervention strategies, J. Differ. Equations, 259 (2015), 7463-7502. |
[27] | D. Jiang, Q. Liu, N. Shi, T. Hayat, A. Alsaedi, P. Xia, Dynamics of a stochastic HIV-1 infection model with logistic growth, Phys. A, 469 (2017), 706-717. |
[28] | T. Feng, Z. Qiu, Global analysis of a stochastic TB model with vaccination and treatment, Discrete Contin. Dyn. Syst. Ser. B, 24 (2018), 2923. |
[29] | S. Zhao, S. Yuan, H. Wang, Threshold behavior in a stochastic algal growth model with stoichiometric constraints and seasonal variation, J. Differ. Equations, 268 (2020), 5113-5139. |
[30] | X. Ji, S. Yuan, T. Zhang, H. Zhu, Stochastic modeling of algal bloom dynamics with delayed nutrient recycling, Math. Biosci. Eng., 16 (2019), 1-24. |
[31] | Q. Liu, D. Jiang, T. Hayat, A. Alsaedi, B. Ahmad, A stochastic SIRS epidemic model with logistic growth and general nonlinear incidence rate, Phys. A, 551 (2020), 124152. |
[32] | S. Cai, Y. Cai, X. Mao, A stochastic differential equation SIS epidemic model with two correlated Brownian motions, Nonlinear Dynam., 97 (2019), 2175-2187. |
[33] | Q. Liu, D. Jiang, N. Shi, T. Hayat, A. Alsaedi, The threshold of a stochastic SIS epidemic model with imperfect vaccination, Math. Comput. Simulation, 144 (2018), 78-90. |
[34] | R. Khasminskii, Stochastic Stability of Differential Equations, Springer, Heidelberg, 2011. |
[35] | D. Xu, Y. Huang, Z. Yang, Existence theorems for periodic Markov process and stochastic functional differential equations, Discrete Contin. Dyn. Syst., 24 (2009), 1005. |
1. | Moustafa El-Shahed, Asma Al-Nujiban, Nagdy F. Abdel-Baky, Stochastic Modelling of Red Palm Weevil Using Chemical Injection and Pheromone Traps, 2022, 11, 2075-1680, 334, 10.3390/axioms11070334 | |
2. | Yousef Alnafisah, Moustafa El-Shahed, Stochastic Analysis of a Hantavirus Infection Model, 2022, 10, 2227-7390, 3756, 10.3390/math10203756 | |
3. | Guihua Li, Yuanhang Liu, Carmen Coll, The Dynamics of a Stochastic SIR Epidemic Model with Nonlinear Incidence and Vertical Transmission, 2021, 2021, 1607-887X, 1, 10.1155/2021/4645203 | |
4. | Nursanti Anggriani, Lazarus Kalvein Beay, Meksianis Z. Ndii, Fatuh Inayaturohmat, Sanubari Tansah Tresna, A mathematical model for a disease outbreak considering waning-immunity class with nonlinear incidence and recovery rates, 2024, 6, 25889338, 170, 10.1016/j.jobb.2024.05.005 |