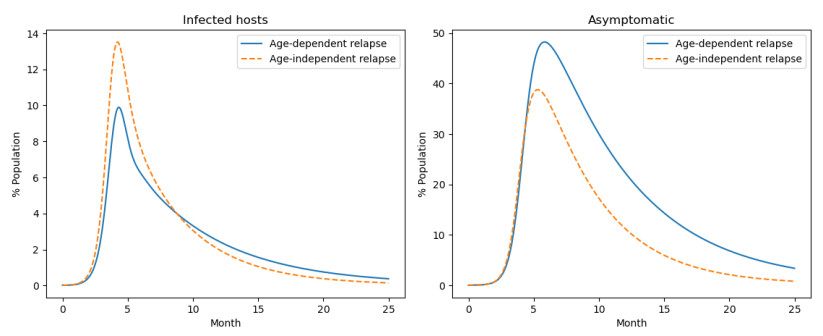
Mosquito-borne infectious diseases represent a significant public health issue. Age has been identified as a key risk factor for these diseases, and another phenomenon reported is relapse, which involves the reappearance of symptoms after a symptom-free period. Recent research indicates that susceptibility to and relapse of mosquito-borne diseases are frequently age-dependent. This paper proposes a new model to better capture the dynamics of mosquito-borne diseases by integrating two age-dependent factors: chronological age and asymptomatic-infection age. Chronological age refers to the time elapsed from the date of birth of the host to the present time. On the other hand, asymptomatic infection age denotes the time elapsed since the host became asymptomatic after the primary infection. The system of integro-differential equations uses flexible, unspecified functions to represent these dependencies, assuming they are integrable. We analyzed the global stability of both the disease-free and endemic equilibrium states using the direct Lyapunov method with Volterra-type Lyapunov functionals. Additionally, the paper explores several special cases involving well-known host-vector models.
Citation: Maria Guadalupe Vazquez-Peña, Cruz Vargas-De-León, Jorge Velázquez-Castro. Global stability for a mosquito-borne disease model with continuous-time age structure in the susceptible and relapsed host classes[J]. Mathematical Biosciences and Engineering, 2024, 21(11): 7582-7600. doi: 10.3934/mbe.2024333
[1] | Yan-Xia Dang, Zhi-Peng Qiu, Xue-Zhi Li, Maia Martcheva . Global dynamics of a vector-host epidemic model with age of infection. Mathematical Biosciences and Engineering, 2017, 14(5&6): 1159-1186. doi: 10.3934/mbe.2017060 |
[2] | Alian Li-Martín, Ramón Reyes-Carreto, Cruz Vargas-De-León . Dynamics of a dengue disease transmission model with two-stage structure in the human population. Mathematical Biosciences and Engineering, 2023, 20(1): 955-974. doi: 10.3934/mbe.2023044 |
[3] | Joan Ponce, Horst R. Thieme . Can infectious diseases eradicate host species? The effect of infection-age structure. Mathematical Biosciences and Engineering, 2023, 20(10): 18717-18760. doi: 10.3934/mbe.2023830 |
[4] | C. Connell McCluskey . Global stability for an $SEI$ model of infectious disease with age structure and immigration of infecteds. Mathematical Biosciences and Engineering, 2016, 13(2): 381-400. doi: 10.3934/mbe.2015008 |
[5] | María Guadalupe Vázquez-Peña, Cruz Vargas-De-León, Jorge Fernando Camacho-Pérez, Jorge Velázquez-Castro . Analysis and Bayesian estimation of a model for Chikungunya dynamics with relapse: An outbreak in Acapulco, Mexico. Mathematical Biosciences and Engineering, 2023, 20(10): 18123-18145. doi: 10.3934/mbe.2023805 |
[6] | Shanjing Ren . Global stability in a tuberculosis model of imperfect treatment with age-dependent latency and relapse. Mathematical Biosciences and Engineering, 2017, 14(5&6): 1337-1360. doi: 10.3934/mbe.2017069 |
[7] | Yang Li, Jia Li . Stage-structured discrete-time models for interacting wild and sterile mosquitoes with beverton-holt survivability. Mathematical Biosciences and Engineering, 2019, 16(2): 572-602. doi: 10.3934/mbe.2019028 |
[8] | Chen Liang, Hai-Feng Huo, Hong Xiang . Modelling mosquito population suppression based on competition system with strong and weak Allee effect. Mathematical Biosciences and Engineering, 2024, 21(4): 5227-5249. doi: 10.3934/mbe.2024231 |
[9] | C. Connell McCluskey . Global stability for an SEI epidemiological model with continuous age-structure in the exposed and infectious classes. Mathematical Biosciences and Engineering, 2012, 9(4): 819-841. doi: 10.3934/mbe.2012.9.819 |
[10] | Xia Wang, Yuming Chen . An age-structured vector-borne disease model with horizontal transmission in the host. Mathematical Biosciences and Engineering, 2018, 15(5): 1099-1116. doi: 10.3934/mbe.2018049 |
Mosquito-borne infectious diseases represent a significant public health issue. Age has been identified as a key risk factor for these diseases, and another phenomenon reported is relapse, which involves the reappearance of symptoms after a symptom-free period. Recent research indicates that susceptibility to and relapse of mosquito-borne diseases are frequently age-dependent. This paper proposes a new model to better capture the dynamics of mosquito-borne diseases by integrating two age-dependent factors: chronological age and asymptomatic-infection age. Chronological age refers to the time elapsed from the date of birth of the host to the present time. On the other hand, asymptomatic infection age denotes the time elapsed since the host became asymptomatic after the primary infection. The system of integro-differential equations uses flexible, unspecified functions to represent these dependencies, assuming they are integrable. We analyzed the global stability of both the disease-free and endemic equilibrium states using the direct Lyapunov method with Volterra-type Lyapunov functionals. Additionally, the paper explores several special cases involving well-known host-vector models.
Mosquito-borne infectious diseases are of global importance. In the last two decades, vector-borne diseases have emerged at a growing rate, and several vector-borne pathogens have colonized new regions [1]. More than 400,000 people die each year from malaria, while dengue, yellow fever, chikungunya, and Zika have caused serious disease outbreaks in many urban areas [2].
Age has been identified as a risk factor for mosquito-borne diseases. In the study by Hasyim et al. [3], age was identified as a risk factor for malaria, with participants older than 35 years being 7.98 times more likely to contract the disease compared to those younger than 35. In a case-control study [4], older patients were found to have a lower risk of contracting dengue compared to younger adults aged 16 to 30 years. In a review [5], they affirmed that the vast majority of dengue cases affect children under 15 years of age. A study from coastal Ecuador [6] reported that dengue seroprevalence was strongly age-dependent, increasing rapidly with age and reaching 97% by age 60. In contrast, chikungunya infection peaked in childhood, with a maximum prevalence of 42% around age 9.
Another phenomenon that has been documented in mosquito-borne diseases is the relapse phenomenon, understood as the reappearance of symptoms after a symptom-free period. In malaria infection, some types of the parasite (such as Plasmodium vivax and Plasmodium ovale) can cause relapses because they form hypnozoites in the liver that can reactivate and trigger a new phase of the disease [7]. In chikungunya infection, a study of 509 people in France found that 72% of patients experienced relapses, averaging four episodes, and the mean delay between two relapses was 8 weeks [9]. Another study conducted in 2015 on 5870 inhabitants of Acapulco, México, showed that 31% of infected individuals had at least one relapse after being symptom-free for a month [10].
Systems of ordinary differential equations are commonly used to model the propagation of mosquito-borne infectious diseases. We identified some mosquito-borne disease models that consider children and adults as different subpopulations. In dengue and malaria, two age classes have been considered [8,11,12,13]. Chamnan et al. [14] developed a three-age-class model for dengue, dividing the population into under 10, 10–44, and over 44 years old. We identified a few mosquito-borne disease models that consider relapses. Huo and Qiu [15] and Ghosh et al. [16] developed malaria models with relapses. More recently, Vázquez-Peña et al. developed a model for chikungunya dynamics with relapse [17].
In mosquito-borne diseases, variability exists in the susceptibility and relapse periods for each infection. Although mathematical models for these diseases address these issues, they often focus on specific scenarios, either using models with two or three age classes [8,11,12,13,14] or assuming constant relapse rates [15,16,17]. It is well established that compartments without age structure imply that the time distribution of transitions from one stage to another is assumed to be exponential when utilized. For many analyses, this serves as an adequate approximation; however, in other cases, particularly in relapse scenarios, a more specific timing is necessary to model complex behaviors. Recent empirical research indicates that susceptibility and relapse rates are frequently age-dependent. Vargas-De-León et al. [18] introduced and analyzed a host-vector model where the host population is structured by chronological age, with host susceptibility assumed to be age-dependent. Additionally, Vargas-De-León [19] developed a model for non-vector-borne diseases that accounts for variability in relapse periods by incorporating the concept of asymptomatic-infection age. Recent evidence in the literature shows the need for more comprehensive models that integrate both susceptibility and variability in relapse rates to better reflect the complex dynamics of these diseases.
In this paper, we present a model to describe the spread of mosquito-borne diseases, incorporating two different age dependencies: chronological age and asymptomatic-infection age. Chronological age refers to the time elapsed from the date of birth of the host to the present time. Asymptomatic infection age denotes the time elapsed since the host became asymptomatic after the primary infection. From a biological perspective, the introduction of age dependency implies the possibility of analyzing more complex relationships between the probability of infection and age. For example, age dependence on risk could manifest as a smooth gradient or even a bimodal effect for more susceptible children and older adults. From a biological standpoint, it is crucial to model the effect of a well-defined distribution period during which relapse can occur, rather than relying solely on an information-poor distribution where only the mean is considered, as implied in compartmental models without age structure. Furthermore, the age dependencies in the epidemiological model directly relate to the physiological evolution of the disease within the body, allowing the microscopic and individual-scale dynamics of the disease to be associated with the epidemiological-scale description of a disease.
We use unspecified functions for both dependencies, provided they are integrable. We will analyze the global stability of both the disease-free and endemic equilibrium states using the direct Lyapunov method with the following Volterra-type functional:
f(x)=x−1−lnx. | (1.1) |
This analysis enables the characterization of a family of vector-borne disease models as epidemiologically significant, either with or without age structure. The introduction of unspecified functions for the time dependencies of chronological age and symptomatic-infection age facilitates the analysis and identification of emergent and non-trivial complex dynamical behavior of the diseases due to those age structure dependencies. A particularly salient aspect of the model is that it establishes the foundation for analyzing periodic or time-distributed control strategies.
We assume that both host and mosquito populations are stratified by their epidemiological stages. The total host population, denoted by Nh, is divided into four classes: susceptible Sh(t), infected Ih(t), asymptomatic Ah(t), and recovered Rh(t). The total mosquito population, denoted by Nm, is divided into two classes: susceptible Sm(t) and infected Im(t).
The chronological age is denoted by the variable τ. Thus, sh(t,τ) represents the number of susceptible hosts aged τ at time t. Therefore, ∫τ2τ1sh(t,τ)dτ gives the number of susceptible hosts whose ages fall between τ1 and τ2 at time t. The total number of susceptible individuals is given by Sh(t)=∫∞0sh(t,τ)dτ. The chronological age of the infected hosts was not explicitly described because, in contrast to age-dependent susceptibility, age-dependent transmissibility is not readily observable through medical records. This complication makes including this dependence less beneficial, as there is limited data to infer this relationship. Conversely, the importance of explicitly describing age-dependent susceptibility lies in its potential to analyze age-dependent risk and focus control strategies based on age. In contrast, infected individuals are presumed to be readily identifiable, and measures can be implemented irrespective of age.
Similarly, we consider the age of asymptomatic infection, denoted by ω. Here, ah(t,ω) denotes the number of individuals in the asymptomatic hosts aged ω at time t. The expression ∫ω2ω1ah(t,ω)dω represents the number of hosts in the asymptomatic state for a duration between ω1 and ω2. Ah(t)=∫∞0ah(t,ω)dω represents the total number of asymptomatic individuals.
We assume that all hosts are born susceptible at a constant rate Λ, which leads to the boundary condition sh(t,0)=Λ. When a host is bitten by an infected mosquito, there is a probability of contracting the pathogen that depends on the age of the host, denoted by βh(τ). Therefore, the number of infections is proportional to this parameter and the number of bites. If b is the average number of bites per mosquito per unit time, each individual receives b/Nh bites, resulting in a transmission rate of βh(τ)b/Nh. Thus, the term bβh(τ)sh(t,τ)Im(t)/Nh stands for the rate of infections of susceptible hosts of age τ by the infected mosquitoes Im. Because there is no distinction between infected individuals due to their age, the infections should be integrated over all ages in the corresponding equation, resulting in the term bNh∫∞0bβh(τ)sh(t,τ)Im(t)dτ.
We also assume that the host population decreases due to natural mortality at a constant rate μh. Additionally, we introduce the parameter ρ(τ) to represent an infection prevention strategy, such as vaccination, that is applied only to specific age groups. Consequently, the rate of removing individuals from the susceptible class is given by εh(τ)=μh+ρ(τ). Thus, the term ε(τ)sh(t,τ) stands for the total rate of removal from the group of the susceptible hosts aged τ.
The parameter γ denotes the rate at which symptomatology disappears from infected hosts, leading to the term (μh+γ)Ih(t) that represents the removal rate from the infected compartment due to natural per-capita mortality μh plus symptom disappearance. The parameter p represents the fraction of infected individuals leaving the infected class γIh, who do not fully recover but instead enter an asymptomatic phase for a period of time ω. Thus, this leads to the boundary condition ah(t,0)=pγIh(t). When symptoms reappear, they return to the infected stage according to a relapse rate dependent on the asymptomatic infection age, δh(ω). The integral in the infected class equation ∫∞0δh(ω)ah(t,ω)dω stands for the rate of transition of all asymptomatic hosts. A fraction (1−p) of infected hosts is assumed to undergo full recovery with permanent immunity, and (1−p)γ represents the recovery rate of the infected hosts.
On the other hand, mosquitoes are assumed to be born and die at a constant rate μm, as is commonly proposed to describe a stationary population. The constant vector population assumption implies that the vectors are continuously reproducing, and the system has reached a state where the population death rate equates to its population birth rate.
A susceptible mosquito becomes infected only if it bites an infected host and the bite effectively transmits the pathogen. Similar to host transmission, the probability that a mosquito will contract the pathogen after biting an infected host is denoted by βm, and the transmission rate in mosquitoes depends on the bite rate b. We introduce the parameter θ to account for any potential differences in transmission probability between infected and asymptomatic hosts, leading to the term θβmbNhSm(t)∫∞0ah(t,ω)dω representing the mosquito infections due to asymptomatic hosts.
The dynamics described above translate into the following system of integro–differential equations:
∂sh(t,τ)∂t+∂sh(t,τ)∂τ=−bNhβh(τ)sh(t,τ)Im(t)−εh(τ)sh(t,τ),sh(t,0)=Λ,dIh(t)dt=bNh∫∞0βh(τ)sh(t,τ)Im(t)dτ−(μh+γ)Ih(t)+∫∞0δh(ω)ah(t,ω)dω,∂ah(t,ω)∂t+∂ah(t,ω)∂ω=−(μh+δh(ω))ah(t,ω),ah(t,0)=pγIh(t),dRh(t)dt=(1−p)γIh(t)+∫∞0ρ(τ)sh(t,τ)dτ−μhRh(t),dSm(t)dt=μmNm−βmbNhSm(t)Ih(t)−θβmbNhSm(t)∫∞0ah(t,ω)dω−μmSm(t),dIm(t)dt=βmbNhSm(t)Ih(t)+θβmbNhSm(t)∫∞0ah(t,ω)dω−μmIm(t). | (2.1) |
Considering that the mosquito population is Nm=Sm(t)+Im(t), and the equation corresponding to the recovery class is decoupled from the rest of the equations of the system, it could be reduce to:
∂sh(t,τ)∂t+∂sh(t,τ)∂τ=−bNhβh(τ)sh(t,τ)Im(t)−εh(τ)sh(t,τ),sh(t,0)=Λ,dIh(t)dt=bNh∫∞0βh(τ)sh(t,τ)Im(t)dτ−(μh+γ)Ih(t)+∫∞0δh(ω)ah(t,ω)dω,∂ah(t,ω)∂t+∂ah(t,ω)∂ω=−α(ω)ah(t,ω), with α(ω)=μh+δh(ω),ah(t,0)=pγIh(t),dIm(t)dt=βmbNh(Nm−Im(t))(Ih(t)+θ∫∞0ah(t,ω)dω)−μmIm(t), | (2.2) |
with the initial conditions given by
sh(0,τ)=sh0(τ), Ih(0)=Ih0, ah(0,ω)=ah0(ω), Iv(0)=Iv0. |
sh0(τ) and ah0(ω) are the initial distribution of the susceptible host and asymptomatic host with chronological age and asymptomatic-infection age, respectively. Ih0 and Iv0 are the initial infected hosts and infected mosquitoes, respectively. In what follows, we also have assumed that the initial conditions are in the invariant subspace where Nh=Λ/μ is constant. By adding the host equations, it can be verified that dNhdt=Λ−μNh and thus Nh=Λ/μ is also a stable subspace.
The basic reproductive number of the system (2.2) is given by:
R0=ηpγNh+DβmbNmNh(μh+γ), |
where D and η are defined by
D=bμmNh∫∞0s0h(τ)βh(τ)dτ, | (3.1) |
η=∫∞0[δh(v)+k]exp(−∫v0α(ϕ)dϕ)dv, | (3.2) |
with
k=βmb2NmθN2hμm∫∞0s0h(τ)βh(τ)dτ. | (3.3) |
The parameter R0 has significant epidemiological meaning; it represents the average number of secondary cases generated by a primary case introduced into a fully susceptible host population. This includes disease transmission from asymptomatic and infected hosts to susceptible mosquitoes, as well as from infected mosquitoes to susceptible hosts.
We define a positive auxiliary function [18,19],
g(ω)=∫∞ω[δh(v)+c]exp(−∫vωα(ϕ)dϕ)dv,c>0, | (3.4) |
that we will use for the construction of Lyapunov functionals in the proof of global stability of the equilibrium state for the system (2.2) by the direct Lyapunov method. Observe that g(ω)>0 for any w≥0 and it verifies g(0)=η when we consider c=k, where k is given in (3.3).
Through direct calculations, the disease-free equilibrium state E0 is obtained taking Ih(t)=0, ah(t,ω)=0, and Im(t)=0, and then dsh(τ)dτ=−εh(τ)sh(τ). Solving this differential equation, we have that the disease-free equilibrium state is E0=(s0h(τ),0,0,0) where
s0h(τ)=Λexp(−∫τ0εh(ϕ)dϕ). | (3.5) |
To prove its global stability, we consider a Lyapunov functional, which is the linear combination of Volterra-type and linear functions:
W(t)=∫∞0s0h(τ)f(sh(t,τ)s0h(τ))dτ+Ih(t)+∫∞0g(ω)a(t,ω)dω+bμmNhIm(t)∫∞0s0h(τ)βh(τ)dτ, |
where f(x) is given by (1.1) and g(ω) by (3.4) with the constant c equal to k defined in (3.3). W(t) satisfies
dWdt=∫∞0(1−s0h(τ)sh(t,τ))∂sh(t,τ)∂tdτ+dIh(t)dt+∫∞0g(ω)∂a(t,ω)∂tdω+bμmNh(∫∞0s0h(τ)βh(τ)dτ)dIm(t)dt. |
Using the equations of the system (2.2),
dWdt=−∫∞0(1−s0h(τ)sh(t,τ))(∂sh(t,τ)∂τ+bNhβh(τ)sh(t,τ)Im(t)+εh(τ)sh(t,τ))dτ+bNh∫∞0βh(τ)sh(t,τ)Im(t)dτ−(μh+γ)Ih(t)+∫∞0δh(ω)ah(t,ω)dω−∫∞0g(ω)[∂ah(t,ω)∂ω+α(ω)ah(t,ω)]dω+DβmbNh(Nm−Im(t))(Ih(t)+θ∫∞0ah(t,ω)dω)−DμmIm(t).=−∫∞0s0h(τ)(sh(t,τ)s0h(τ)−1)(∂sh(t,τ)∂τsh(t,τ)+εh(τ))dτ+bNh∫∞0s0h(τ)βh(τ)Im(t)dτ−(μh+γ)Ih(t)−∫∞0[g(ω)α(ω)−δh(ω)−βmbNhDNmθ]ah(t,ω)dω−∫∞0g(ω)∂ah(t,ω)∂ωdω−DμmIm(t)+βmbNmNhDIh(t)−βmbNhDIm(t)Ih(t)−θβmbNhDIm(t)∫∞0ah(t,ω)dω, |
with D given by (3.1).
By direct calculations can be shown as
∂∂τf(sh(t,τ)s0h(τ))=(sh(t,τ)s0h(τ)−1)(∂sh(t,τ)∂τsh(t,τ)+εh(τ)), |
which makes it possible to simplify the first term of dW/dt integrating by parts:
∫∞0s0h(τ)∂∂τf(sh(t,τ)s0h(τ))dτ=[s0h(τ)f(sh(t,τ)s0h(τ))]∞+∫∞0εh(τ)s0h(τ)f(sh(t,τ)s0h(τ))dτ. | (3.6) |
Applying integration by parts over another term of dW/dt with the boundary condition ah(t,0)=pγIh(t) results in
∫∞0g(ω)∂ah(t,ω)∂ωdω=[g(ω)ah(t,ω)]∞−ηpγIh(t)−∫∞0[g(ω)α(ω)−δh(ω)−k]ah(t,ω)dω. | (3.7) |
Using Eqs (3.6) and (3.7) results in:
dWdt=−[s0h(τ)f(sh(t,τ)s0h(τ))+g(ω)ah(t,ω)]∞−∫∞0εh(τ)s0h(τ)f(sh(t,τ)s0h(τ))dτ−DβmbNhIm(t)Ih(t)−DθβmbNhIm(t)∫∞0ah(t,ω)dω−Ih(t)(μh+γ)[1−R0]. |
Therefore, W(t) is a positive definite function whose derivative dW/dt is negative provided that R0<1. Moreover, the only positively invariant set where dW/dt=0 is {E0}. By Theorem 2.53 of Smith and Thieme [20], the disease-free equilibrium state is globally asymptotically stable when R0≤1. We arrived at the following results:
Theorem 3.1. The disease-free equilibrium state E0 of the system (2.2) is globally asymptotically stable if R0≤1.
The equilibrium state E∗=(s∗h(τ),I∗h,a∗h(ω),I∗m) must satisfy the system of equations:
ds∗h(τ)dτ=−bNhβh(τ)s∗h(τ)I∗m−εh(τ)s∗h(τ), | (3.8) |
s∗h(0)=Λ, | (3.9) |
0=bNh∫∞0βh(τ)s∗h(τ)I∗mdτ−(μh+γ)I∗h+∫∞0δh(ω)a∗h(ω)dω, | (3.10) |
da∗h(ω)dω=−α(ω)a∗h(ω), | (3.11) |
a∗h(0)=pγI∗h, | (3.12) |
0=βmbNh(Nm−I∗m)(I∗h+θ∫∞0a∗h(ω)dω)−μmI∗m. | (3.13) |
Although the system of Eqs (3.8)–(3.13) will not be solved explicitly, but we can prove the existence of the endemic equilibrium state.
Solving the differential equation (3.11) with initial condition (3.12), we have that:
a∗h(ω)=pγI∗hexp(−∫ω0α(ϕ)dϕ), | (3.14) |
and replacing it in Eq (3.13), we obtain the following expression:
I∗h=μmNhI∗mβmb(Nm−I∗m)[1+θpγ∫∞0exp(−∫ω0α(ϕ)dϕ)dω]. | (3.15) |
In addition,
s∗h(τ)=s0h(τ)exp(−bNhI∗m∫τ0β(ϕ)dϕ) | (3.16) |
is the particular solution of (3.8) under the initial condition (3.9) and s0h(τ) has been defined in (3.5).
Replacing the Eqs (3.15), (3.14), and (3.16) in (3.10) we get:
0= I∗m((μh+γ)μmNh−pγμmNh∫∞0δh(ω)exp(−∫ω0α(ϕ)dϕ)dωβmb(Nm−I∗m)[1+θpγ∫∞0exp(−∫ω0α(ϕ)dϕ)dω])⋅(βmb2(Nm−I∗m)[1+θpγ∫∞0exp(−∫ω0α(ϕ)dϕ)dω]Nh[(μh+γ)μmNh−pγμmNh∫∞0δh(ω)exp(−∫ω0α(ϕ)dϕ)dω]∫∞0βh(τ)s0h(τ)exp(−bNhI∗m∫τ0β(ϕ)dϕ)dτ−1). | (3.17) |
The case I∗m=0 reduces to the disease-free equilibrium state. The second term in (3.17) is always positive because
∫∞0δh(ω)exp(−∫ω0α(ϕ)dϕ)dω≤∫∞0δh(ω)exp(−∫ω0δh(ϕ)dϕ)dω=1−exp(−∫∞0δh(ϕ)dϕ), | (3.18) |
so the equality in (3.17) only can be satisfied when the last term in the product of the right member of (3.17) is equal to 0. To prove that, we define the function h(I∗m) given by:
h(I∗m)=βmb2[1+θpγ∫∞0exp(−∫ω0α(ϕ)dϕ)dω](Nm−I∗m)Nh[(μh+γ)μmNh−pγμmNh∫∞0δh(ω)exp(−∫ω0α(ϕ)dϕ)dω]∫∞0βh(τ)s0h(τ)exp(−bNhI∗m∫τ0β(ϕ)dϕ)dτ, |
which satisfies that h(Nm)=0 and h(0)=R0N2hμm(μh+γ)−pγ∫∞0N2hμmδh(v)exp(−∫v0α(ϕ)dϕ)N2hμm(μh+γ)−pγ∫∞0N2hμmδh(v)exp(−∫v0α(ϕ)dϕ). It is clear that h(0)>1 when R0>1.
In order to analyse the behavior of the function h(I∗m) in the interval (0,Nm), the first derivative test is applied. By simple calculations, we obtain
h′(I∗m)=−βmb2[1+θpγ∫∞0exp(−∫ω0α(ϕ)dϕ)dω]ΔN2hμm[μh+γ(1−p∫∞0δh(ω)exp(−∫ω0α(ϕ)dϕ)dω)], | (3.19) |
with
Δ=∫∞0βh(τ)s0h(τ)(1+bNh(Nm−I∗m)∫τ0β(ϕ)dϕ)exp(−bNhI∗m∫τ0β(ϕ)dϕ)dτ. |
Using (3.18), we can note that the denominator of (3.19) is positive, so that h′(I∗m)<0. Hence, h(I∗m) is a decreasing monotonic function that satisfies h(Nm)=0 and h(0)>1 when R0>1, so there exists one and only I∗m∈(0,Nm) such that h(I∗m)=1, being only determined by sh(τ), I∗h and a∗h(ω) in Eqs (3.16), (3.15), and (3.14), respectively. We arrived at the following results:
Theorem 3.2. The system (2.2) exhibits an endemic equilibrium state when R0>1.
Now, we proove the global stability of the endemic equilibrium state using the direct Lyapunov method. We consider the Volterra-type Lyapunov functional:
L(t)=μmNhI∗mb∫∞0s∗h(τ)f(sh(t,τ)s∗h(τ))dτ+I∗hμmNhI∗mbf(Ih(t)I∗h)+μmNhI∗mb∫∞0a∗h(ω)g(ω)f(ah(t,ω)a∗h(ω))dω+f(Im(t)I∗m)∫∞0βh(τ)s∗h(τ)dτ, |
where g(ω) is defined in (3.4) with the constant c=(bNh)2βmμm(Nm−I∗m)θ∫∞0βh(τ)s∗h(τ)dτ.
After calculating the derivative with respect to time and using the equations of the model (2.2), it results in:
dLdt=−μmNhI∗mb∫∞0(1−s∗h(τ)sh(t,τ))(∂sh(t,τ)∂τ+bNhβh(τ)sh(t,τ)Im(t)+εh(τ)sh(t,τ))dτ+μmNhI∗mb(1−I∗hIh(t))(Im(t)bNh∫∞0βh(τ)sh(t,τ)dτ−(μh+γ)Ih(t)+∫∞0δh(ω)ah(t,ω)dω)−μmNhI∗mb∫∞0g(ω)(1−a∗h(ω)ah(t,ω))(∂ah(t,ω)∂ω+α(ω)ah(t,ω))dω+1I∗m∫∞0βh(τ)s∗h(τ)dτ(1−I∗mIm(t))(βmbNh(Nm−Im(t))(Ih(t)+θ∫∞0ah(t,ω)dω)−μmIm(t)). | (3.20) |
Using Eq (3.11), it can be directly shown that
∂∂ωf(ah(t,ω)a∗h(ω))=1a∗h(ω)(1−a∗h(ω)ah(t,ω))(∂ah(t,ω)∂ω+α(ω)ah(t,ω)). |
After integrating by parts with the boundary condition ah(t,0)=pγIh(t) and Eq (3.12), we have
∫∞0g(ω)a∗h(ω)∂∂ωf(ah(t,ω)a∗h(ω))dω=[g(ω)a∗h(ω)f(ah(t,ω)a∗h(ω))]ω=∞−ηpγI∗hf(Ih(t)I∗h)+∫∞0(δh(ω)+k)a∗h(ω)f(ah(t,ω)a∗h(ω))dω. | (3.21) |
Similarly, by Eq (3.8), it is proved that
∂∂τf(sh(t,τ)s∗h(τ))=(sh(t,τ)s∗h(τ)−1)(∂sh(t,τ)∂τsh(t,τ)+bNhI∗mβh(τ)+εh(τ)), |
and integrating by parts again, we have
∫∞0s∗h(τ)∂∂τf(sh(t,τ)s∗h(τ))dτ=[s∗h(τ)f(sh(t,τ)s∗h(τ))]τ=∞−∫∞0∂s∗h(τ)∂τf(sh(t,τ)s∗h(τ))dτ. | (3.22) |
After several calculations making use of (3.8) and the result in (3.22) to simplify the first term of (3.20), rewriting the identity (3.10) as μh+γ=(NhI∗h)−1bI∗v∫∞0βh(τ)s∗h(τ)dτ+(I∗h)−1∫∞0δh(ω)a∗h(ω)dω for the second term, using the result of the integral (3.21) for the third term, and rephrasing Eq (3.13) as μmI∗v=βmbN−1h(Nv−I∗v)(I∗h+θ∫∞0a∗h(ω)dω) for the fourth term of the derivative of L, this reduces to
dLdt=−μmNhI∗mb∫∞0s∗h(τ)(Sh(t,τ)s∗h(τ)−1)(∂Sh(t,τ)∂τSh(t,τ)+bNhI∗mβh(τ)+κh(τ))dτ+μm∫∞0s∗h(τ)βh(τ)(Sh(t,τ)s∗h(τ)−Sh(t,τ)Im(t)s∗h(τ)I∗m+Im(t)I∗m−1)dτ+μm∫∞0βh(τ)s∗h(τ)(Sh(t,τ)Im(t)s∗h(τ)I∗m−Ih(t)I∗h−Sh(t,τ)I∗hIm(t)s∗h(τ)Ih(t)I∗m+1)dτ+μmNhI∗mb∫∞0δh(ω)a∗h(ω)(ah(t,ω)a∗h(ω)−Ih(t)I∗h−I∗hah(t,ω)Ih(t)a∗h(ω)+1)dω−μmNhI∗mb[g(ω)a∗h(ω)f(ah(t,ω)a∗h(ω))]ω=∞+μmNhI∗mb∫∞0δh(ω)a∗h(ω)(f(Ih(t)I∗h)−f(ah(t,ω)a∗h(ω)))dω+βmbNmθI∗mNh∫∞0s∗h(τ)βh(τ)dτ∫∞0a∗h(ω)(f(Ih(t)I∗h)−f(ah(t,ω)a∗h(ω)))dω+βmb2(Nm−I∗m)θI∗mN2hμm∫∞0s∗h(τ)βh(τ)dτ∫∞0a∗h(ω)(ah(t,ω)a∗h(ω)−Im(t)I∗m−ah(t,ω)I∗ma∗h(ω)Im(t)+1)dω+βmb2(Nm−I∗m)I∗hI∗mN2hμm∫∞0s∗h(τ)βh(τ)dτ(Ih(t)I∗h−Im(t)I∗m−Ih(t)I∗mI∗hI(mt)+1)+βmb2N2hμm∫∞0s∗h(τ)βh(τ)dτ(2−I∗mIm(t)−Im(t)I∗m)(Ih(t)+θ∫∞0ah(t,ω)dω). |
As a result of various calculations, we have
dLdt=−μmNhI∗mb[s∗h(τ)f(sh(t,τ)s∗h(τ))]τ=∞−μmNhI∗mb∫∞0εh(τ)s∗h(τ)f(sh(t,τ)s∗h(τ))dτ+βmbNh∫∞0βh(τ)s∗h(τ)dτ(2−I∗mIm(t)−Im(t)I∗m)(Ih(t)+θ∫∞0ah(t,ω)dω)−[μmNhI∗mbg(ω)a∗h(ω)f(ah(t,ω)a∗h(ω))]ω=∞+βmbI∗hNhI∗m(Nm−I∗m)∫∞0βh(τ)s∗h(τ)(ln(sh(t,τ)I∗hIm(t)s∗h(τ)Ih(t)I∗m)+1−sh(t,τ)I∗hIm(t)s∗h(τ)Ih(t)I∗m+ln(Ih(t)I∗mI∗hIm(t))+1−Ih(t)I∗mI∗hIm(t))dτ+βmbθNhI∗m(Nm−I∗m)∫∞0∫∞0a∗h(ω)βh(τ)s∗h(τ)(ln(sh(t,τ)s∗h(τ))−sh(t,τ)I∗hIm(t)s∗h(τ)Ih(t)I∗m−ah(t,ω)I∗ma∗h(ω)Im(t))dτdω+βmbθNhI∗m(Nm−I∗m)∫∞0∫∞0a∗h(ω)βh(τ)s∗h(τ)(2−ln(Ih(t)I∗h)+ln(ah(t,ω)a∗h(ω)))dτdω+μmNhI∗mb∫∞0δh(ω)a∗h(ω)(−I∗hah(t,ω)Ih(t)a∗h(ω)+1−ln(Ih(t)I∗h)+ln(ah(t,ω)a∗h(ω)))dω=−μmNhI∗mb[s∗h(τ)f(sh(t,τ)s∗h(τ))]τ=∞−μmNhI∗mb∫∞0εh(τ)s∗h(τ)f(sh(t,τ)s∗h(τ))dτ−[μmNhI∗mbg(ω)a∗h(ω)f(ah(t,ω)a∗h(ω))]ω=∞−βmbNh∫∞oβh(τ)s∗h(τ)dτ(Ih(t)+θ∫∞0ah(t,ω)dω)(f(I∗mIm(t))+f(Im(t)I∗m))−βmbNh(Nm−I∗m)I∗hI∗m∫∞0βh(τ)s∗h(τ)(f(sh(t,τ)I∗hIm(t)s∗h(τ)Ih(t)I∗m)+f(Ih(t)I∗mI∗hIm(t)))dτ−βmbNhI∗m(Nm−I∗m)θ∫∞0∫∞0a∗h(ω)βh(τ)s∗h(τ)(f(sh(t,τ)I∗hIm(t)s∗h(τ)Ih(t)I∗m)+f(ah(t,ω)I∗ma∗h(ω)Im(t)))dτdω−μmNhI∗mb∫∞0δh(ω)a∗h(ω)(f(I∗hah(t,ω)Ih(t)a∗h(ω)))dω. |
Hence, R0>1 guarantees that the endemic equilibrium state exists and L(t) is a positive definite functional such that dL/dt≥0 and {E∗} is the maximum invariant set where dL/dt=0. If R0>1, by Theorem 2.53 of Smith and Thieme [20], the endemic equilibrium E∗ is globally asymptotically stable. We arrived at the following results:
Theorem 3.3. The endemic equilibrium state E∗ of the system (2.2) is globally asymptotically stable if R0>1.
We consider the change of variable Sh(t)=∫∞0sh(t,τ)dτ, which represents the total number of susceptible hosts. By defining the functions βh(τ)=βh and εh(τ)=εh, we integrate the first equation of the system (2.2) using the boundary condition given in the second expression. This yields:
dShdt=Λ−βhbNhSh(t)Im(t)−ρhSh(t),dIh(t)dt=βhbNhSh(t)Im(t)dτ−(μh+γ)Ih(t)+∫∞0δh(ω)ah(t,ω)dω,∂ah(t,ω)∂t+∂ah(t,ω)∂ω=−(μh+δh(ω))ah(t,ω),ah(t,0)=pγIh(t),dIm(t)dt=βmbNh(Nm−Im(t))(Ih(t)+θ∫∞0ah(t,ω)dω)−μmIm(t). |
The model is now independent of the age of susceptible hosts and depends only on the asymptomatic-infection age.
To reduce the original model (2.2) to one that depends only on the age of susceptible hosts, we define A(t)=∫∞0a(t,ω)dω as the total number of asymptomatic hosts and consider the function to be a constant δh(ω)=δh. By integrating the fourth equation of (2.2) with the boundary condition given in the fifth expression, we obtain:
∂sh(t,τ)∂t+∂sh(t,τ)∂τ=−βh(τ)bNhsh(t,τ)Im(t)−ρh(τ)sh(t,τ), | (4.1) |
sh(t,0)=Λ, | (4.2) |
dIh(t)dt=∫∞0βh(τ)bNhsh(t,τ)Im(t)dτ−(μh+γ)Ih(t)+δhAh(t), | (4.3) |
dAh(t)dt=pγIh(t)−(μh+δh)Ah(t), | (4.4) |
dIm(t)dt=βmbNh(Nm−Im(t))(Ih(t)+θAh(t))−μmIm(t). | (4.5) |
We have an age-independent model of relapse that depends only on the chronological age. In the special case where δh=θ=p=0, the model reduces to an SIR-SI host-vector model with chronological age of susceptible hosts [18].
Now, applying both transformations Sh(t)=∫∞0sh(t,τ)dτ and A(t)=∫∞0a(t,ω)dω simultaneously, and defining the constant functions βh(τ)=βh, ρh(τ)=μh, δh(ω)=δh, and Λ=μhNh, we integrate the first equation in (2.2) with respect to τ and the fourth equation with respect to ω, applying their respective boundary conditions. This reduction yields:
dShdt=μhNh−βhbNhSh(t)Im(t)−μhSh(t),dIhdt=βhbNhSh(t)Im(t)−(μh+γ)Ih(t)+δhAh(t),dAhdt=pγIh(t)−(μh+δh)Ah(t),dImdt=βmbNh(Nm−Im(t))(Ih(t)+θAh(t))−μmIm(t). |
This model is independent of both the age of susceptible hosts and the relapse age. It is isomorphic to the malaria model without temporary immunity [15] and the same for the chikungunya model [17]. In the special case where δh=θ=p=0, the age-independent model reduces to a classical dengue model [21]. In [17], this model was fitted to a chikungunya outbreak in Mexico. It was shown that relapse has important effects on the dynamics of the disease; however, the age-dependent model can describe a more detailed and complex dynamic as the reduced model, as we will see in the next section of numerical simulations.
Numerical simulations were performed to test the effect of chronological age and asymptomatic-infection age. We used the method of lines with a first-order upwind scheme for derivatives concerning the chronological age τ and the age of asymptomatic infection ω [22]. For integration in time t, the odeint Python function that employs the lsoda algorithm was used. We used the following function: βh(τ)
βh(τ)={β1, if 0<τ≤τβminβ2, if τβmin<τ≤τβmedβ3, if τβmed<τ, |
where τβmin, τβmed, and τβmax are chronological ages, and β1, β2, and β3, are positive constants. This function represents three age groups: young people, adults, and older adults, where the probability of contracting the pathogen of an infected mosquito in young people is greater than in adults (β1>β2), and the probability of contagion in adults is greater than in older adults (β2>β3). First we made a simulation of the model without asymptomatic age-dependence relapse. Then we compared with the case of age of asymptomatic infection dependence. We employed the following relapse rate δ(ω) reported in [19]:
δ(ω)={δ1, if 0<ω≤ωδminδ2, if ωδmin<ω≤ωδmedδ3, if ωδmed<ω, |
where ωδmin, ωδmed, and ωδmax are asymptomatic-infection ages, and δ1, δ2, and δ3, are positive constants.
We observe that asymptomatic age dependence leads to a prolonged period of infection following the primary outbreak and is caused by the relapse. Consequently, the duration of the infection is extended, and the asymptomatic cases are amplified. The unconventional behavior observed in Figure 1 (right) can only be achieved by considering the relapse rate dependence on the asymptomatic age, in contrast to the common behavior shown by traditional compartmental models, as shown in Figure 1 (left).
We also compare a simulation of an age-independent vaccination strategy with a more realistic age-dependence vaccination strategy. We used a specific form of the age-dependent vaccination rate ρ(τ):
ρ(τ)={0, if 0<τ≤τρminρ0, if τρmin<τ≤τρmed0, if τρmed<τ, |
where ρ0 is a positive constant. The age group of those under τρmin and over τmed represents those children and older adults for whom the vaccine is not approved. The vaccination is applied for the age group τρmin<τ≤τρmed with the highest risk of mosquito-borne infection.
Figure 2 shows the importance of considering age dependence in control strategies. If not, the effectiveness of a strategy can be overestimated, as shown in Figure 2 (left). This overestimation also occurs in the effect of the control in asymptomatic cases. In realistic scenarios, vaccination is focused on an age group, as shown in Figure 2 (right) (vaccination between 12 and 22 years), because of vaccine specifications or economic constraints. The values for the simulations were taken from [17], and are shown in Tables 1 and 2.
Parameter | Value |
βh | 0.7379 |
βv | 0.7570 |
b | 3.2158 |
μv | 3.3510 |
γ | 4.5821 |
p | 0.6536 |
δh | 0.7506 |
σ | 0.2558 |
Nv | 19,440.1813 |
parameter | β1 | β2 | β3 | τβmin | τβmed |
value | 1.5β2 | 0.7379 | 0.5β2 | 12 years | 60 years |
parameter | δ1 | δ2 | δ3 | ωδmin | ωδmed |
value | 0 | 0.7506 | 0.5δ2 | 1 week | 2 months |
parameter | ρ0 | τρmin | τρmed | ||
values | 100/(τρmed−τρmin) | 12 years | 22 years |
In this study, we have developed a mathematical model to describe the dynamics of mosquito-borne diseases by incorporating both chronological age and asymptomatic-infection age. Chronological age accounts for the time since birth, affecting susceptibility and exposure, while asymptomatic-infection age reflects the duration of the asymptomatic phase before relapse. By allowing both dependencies to be functions of ages τ and ω, our model provides a more complete view of mosquito-borne disease dynamics, addressing variability in susceptibility and relapse rates, which have been ignored in other models.
We have analytically demonstrated the global stability of the system using the direct Lyapunov method with a Volterra-type functional. Our results confirm that the system (2.2) behaves as follows: If R0≤1, the disease-free equilibrium state E0 is globally asymptotically stable. Conversely, if R0>1, the endemic equilibrium state E∗ exists and is also globally asymptotically stable.
It is noteworthy that we only impose integrability conditions on the age-specific probability of becoming an infected host through mosquito bites βh(ω), age-dependent vaccination rate ρ(τ), and age-dependent relapse rate δh(ω) in the model, and hence, the global stability results derived are robust and do not depend on specific forms of this function.
Some forms of the functions ρ(τ), βh(τ), and δ(ω) have been proposed in [19,23]. The mosquito-host transmission function βh(τ)=β0τexp[−β1τ] is reported in [23], with β0>0, β1>0, and τ>0 as the chronological age. We proposed the following function βh(τ):
βh(τ)={β1, if 0<τ≤τβminβ2, if τβmin<τ≤τβmedβ3, if τβmed<τ<τβmax, |
where τβmin, τβmed, and τβmax are chronological ages, and β1, β2, and β3, are positive constants. This function represents three age groups: young people, adults, and older adults, where the probability of contracting the pathogen of an infected mosquito in young people is greater than in adults (β1>β2), and the probability of contagion in adults is greater than in older adults (β2>β3).
We proposed a specific form of the age-dependent vaccination rate ρ(τ):
ρ(τ)={0, if 0<τ≤τρminρ0τexp[−ρ1τ], if τρmin<τ≤τρmed0, if τρmed<τ<τρmax, |
where ρ0 and ρ1 are positive constants. The age group of those under τρmin and over τmed represents those children and older adults for whom the vaccine is not approved. ρ0τexp[−ρ1τ] is the vaccination strategy for the age group τρmin<τ≤τρmed with the highest risk of mosquito-borne infection.
The following relapse rate δ(ω) is reported in [19]:
δ(ω)={δ1, if 0<ω≤ωδminδ2, if ωδmin<ω≤ωδmedδ3, if ωδmed<ω<ωδmax, |
where ωδmin, ωδmed, and ωδmax are asymptomatic-infection ages, and δ1, δ2, and δ3 are positive constants. The relapse rate and the duration of an asymptomatic infection are monitored in this function using the variable asymptomatic-infection age. Considering the fact that relapses decrease in frequency over time, 1/δ2<1/δ3.
We further explored specific cases of the model to understand the impact of different assumptions on its dynamics. By focusing on asymptomatic-infection age and simplifying the model to be independent of the chronological age of susceptible hosts, we derived a model that emphasizes the influence of the variability of relapse periods. Additionally, by concentrating on chronological age and simplifying the model to be independent of the asymptomatic-infection age, the model reduces to the SIR-SI model with the chronological age of susceptible hosts [18]. By applying transformations to eliminate dependencies on both the chronological age of susceptible hosts and the asymptomatic-infection age, we obtained a model that simplifies to forms isomorphic to existing malaria, chikungunya, and dengue models [15,17,21]. These examples demonstrate the versatility of the model and its applicability to a range of mosquito-borne diseases. In [17], a simplified version of the model presented in this manuscript without age-dependent factors is fitted to an actual chikungunya outbreak in Acapulco, Mexico. The model gave a good general description of the outbreak, although the outbreak data showed a fat tail in the infected class. On the other hand, the numerical simulations in this manuscript showed that the age-dependent factors in the proposed model led to a fat tail epidemic curve, making this model a plausible explanation of this observed phenomenon. Models with age structure have significant potential to describe complex dynamics resulting from non-trivial relationships between age-related transitions between compartments. For instance, it has been observed that Zika exhibits vertical transmission in addition to the presence of asymptomatic Zika [24,25]. The effect of these phenomena should be analyzed using an age-structured model in future research.
Finally, we suggest that the age-dependent functions ρ(τ), βh(τ), and δ(ω) for the basic reproductive number can offer useful guidance for control and prevention strategies. These models with continuous-time age structure can be applied to the dynamics of mosquito-borne diseases.
The authors declare they have not used Artificial Intelligence (AI) tools in the creation of this article.
This work has been partially supported by the CONAHCYT-Ciencia de Frontera "Ecuaciones de evolución, sus estados estacionarios y su comportamiento asintótico con aplicaciones en Física y Biología" (project N. 684340). Vázquez-Peña is indebted to CONAHCYT for a fellowship that enabled him to pursue graduate studies for the degree of Bachelor in Matemáticas Aplicadas.
The authors declare there is no conflict of interest.
[1] |
B. Chala, F. Hamde, Emerging and re-emerging vector-borne infectious diseases and the challenges for control: a review, Front. Public Health, 9 (2021), 715759. https://doi.org/10.3389/fpubh.2021.715759 doi: 10.3389/fpubh.2021.715759
![]() |
[2] |
R. T. Jones, T. H. Ant, M. M. Cameron, J. G. Logan, Novel control strategies for mosquito-borne diseases, Phil. Trans. R. Soc. B, 376 (2021), 20190802. https://doi.org/10.1098/rstb.2019.0802 doi: 10.1098/rstb.2019.0802
![]() |
[3] |
H. Hasyim, W. C. Dewi, W. C. Lestari, R. Flora, N. Novrikasari, I. A. Liberty, et al., Risk factors of malaria transmission in mining workers in Muara Enim, South Sumatra, Indonesia, Sci. Rep., 13 (2023), 14755. https://doi.org/10.1038/s41598-023-40418-9 doi: 10.1038/s41598-023-40418-9
![]() |
[4] |
N. Thang, C. Duy, C. Xuan, H. Thi, H. Mats, N. Hung, et al., Risk factors of dengue fever in an urban area in Vietnam: a case-control study, BMC Public Health, 21 (2021), 664. https://doi.org/10.1186/s12889-021-10687-y doi: 10.1186/s12889-021-10687-y
![]() |
[5] |
L. M. Verhagen, R. de Groot, Dengue in children, J. Infect., 69 (2014), S77–S86. https://doi.org/10.1016/j.jinf.2014.07.020 doi: 10.1016/j.jinf.2014.07.020
![]() |
[6] |
I. C. Ster, A. Rodriguez, N. C. Romero, N. C. Lopez, M. Chico, J. Montgomery, et al., Age-dependent seroprevalence of dengue and chikungunya: inference from a cross-sectional analysis in Esmeraldas Province in coastal Ecuador, BMJ Open, 10 (2020), e040735. https://doi.org/10.1136/bmjopen-2020-040735 doi: 10.1136/bmjopen-2020-040735
![]() |
[7] |
B. Nadjm, R. H. Behrens, Malaria: An update for physicians, Infect. Dis. Clin., 26 (2012), 243–259. https://doi.org/10.1016/j.idc.2012.03.010 doi: 10.1016/j.idc.2012.03.010
![]() |
[8] |
A. K. Supriatna, E. Soewono, S. A. van Gils, A two-age-classes dengue transmission model, Math. Biosci., 216 (2008), 114–121. https://doi.org/10.1016/j.mbs.2008.08.011 doi: 10.1016/j.mbs.2008.08.011
![]() |
[9] |
E. Couturier, F. Guillemin, M. Mura, L. León, J. M. Virion, J. M. Letort, et al., Impaired quality of life after Chikungunya virus infection: a 2-year follow-up study, Rheumatology, 51 (2012), 1315–1322. https://doi.org/10.1093/rheumatology/kes015 doi: 10.1093/rheumatology/kes015
![]() |
[10] |
I. X. De La Cruz-Castro, E. Nava-Aguilera, A. Morales-Pérez, Á. F. Betanzos-Reyes, M. Flores-Moreno, L. Morales-Nava, et al., Factors associated with Chikungunya relapse in Acapulco, México, Vector-Borne Zoonotic Dis, 20 (2020), 782–787. https://doi.org/10.1089/vbz.2020.2615 doi: 10.1089/vbz.2020.2615
![]() |
[11] |
D. Aldila, T. Götz, T. Soewono, An optimal control problem arising from a dengue disease transmission model, Math. Biosci., 242 (2013), 9–16. https://doi.org/10.1016/j.mbs.2012.11.014 doi: 10.1016/j.mbs.2012.11.014
![]() |
[12] |
E. Beretta, V. Capasso, D. G. Garao, A mathematical model for malaria transmission with asymptomatic carriers and two age groups in the human population, Math. Biosci., 300 (2018), 87–101. https://doi.org/10.1016/j.mbs.2018.03.024 doi: 10.1016/j.mbs.2018.03.024
![]() |
[13] |
A. Li-Martín, R. Reyes-Carreto, C. Vargas-De-León, Dynamics of a dengue disease transmission model with two-stage structure in the human population, Math. Biosci. Eng., 20 (2023), 955–974. https://doi.org/10.3934/mbe.2023044 doi: 10.3934/mbe.2023044
![]() |
[14] |
A. Chamnan, P. Pongsumpun, I. M. Tang, N. Wongvanich, Effect of a vaccination against the dengue fever epidemic in an age structure population: From the perspective of the local and global stability analysis, Mathematics, 10 (2022), 904. https://doi.org/10.3390/math10060904 doi: 10.3390/math10060904
![]() |
[15] |
H. F. Huo, G. M. Qiu, Stability of a mathematical model of malaria transmission with relapse, Abstr. Appl. Anal., 1 (2014), 289349. https://doi.org/10.1155/2014/289349 doi: 10.1155/2014/289349
![]() |
[16] |
G. M. Ghosh, S. Olaniyi, O. S. Obabiyi, Mathematical analysis of reinfection and relapse in malaria dynamics, Appl. Math. Comput., 373 (2020), 125044. https://doi.org/10.1016/j.amc.2020.125044 doi: 10.1016/j.amc.2020.125044
![]() |
[17] |
O. S. Vázquez-Peña, C. Vargas-De-León, J. F. Camacho-Pérez, J. Velázquez-Castro, Analysis and Bayesian estimation of a model for Chikungunya dynamics with relapse: An outbreak in Acapulco, Mexico, Math. Biosci. Eng., 20 (2023), 18123–18145. https://doi.org/10.3934/mbe.2023805 doi: 10.3934/mbe.2023805
![]() |
[18] |
C. Vargas-De-León, L. Esteva, A. Korobeinikov, Age-dependency in host-vector models: The global analysis, Appl. Math. Comput., 243 (2014), 969–981. http://dx.doi.org/10.1016/j.amc.2014.06.042 doi: 10.1016/j.amc.2014.06.042
![]() |
[19] |
C. Vargas-De-León, Global stability properties of age‐dependent epidemic models with varying rates of recurrence, Math. Methods Appl. Sci., 39 (2016), 2057–2064. https://doi.org/10.1002/mma.3621 doi: 10.1002/mma.3621
![]() |
[20] | H. L. Smith, H. L. Thieme, Dynamical Systems and Population Persistence, American Mathematical Society, 2011. |
[21] |
L. Esteva, C. Vargas, Analysis of a dengue disease transmission model, Math. Biosci., 150 (1998), 131–151. https://doi.org/10.1016/S0025-5564(98)10003-2 doi: 10.1016/S0025-5564(98)10003-2
![]() |
[22] | C. Hirsch, Numerical Computation of Internal and External Flows: Fundamentals of Computational Fluid Dynamics, Elsevier/Butterworth-Heinemann, 2007. |
[23] |
G. Cruz-Pacheco, L. Esteva, C. Vargas, Vaccination strategies for SIR vector-transmitted diseases, Bull. Math. Biol., 76 (2014), 2073–2090. https://doi.org/10.1007/s11538-014-9999-6 doi: 10.1007/s11538-014-9999-6
![]() |
[24] |
M. M. Haby, M. Pinart, V. Elias, L. Reveiz, Prevalence of asymptomatic Zika virus infection: a systematic review, Bull. World Health Organ., 96 (2018), 402–413D. https://doi.org/10.2471/BLT.17.201541 doi: 10.2471/BLT.17.201541
![]() |
[25] |
A. E. Ades, A. Soriano-Arandes, A. Alarcon, F. Bonfante, C. Thorne, C. S. Peckham, et al., Vertical transmission of Zika virus and its outcomes: a Bayesian synthesis of prospective studies, Lancet Infect. Dis., 21 (2021), 537–545. https://doi.org/10.1016/s1473-3099(20)30432-1 doi: 10.1016/s1473-3099(20)30432-1
![]() |
Parameter | Value |
βh | 0.7379 |
βv | 0.7570 |
b | 3.2158 |
μv | 3.3510 |
γ | 4.5821 |
p | 0.6536 |
δh | 0.7506 |
σ | 0.2558 |
Nv | 19,440.1813 |
parameter | β1 | β2 | β3 | τβmin | τβmed |
value | 1.5β2 | 0.7379 | 0.5β2 | 12 years | 60 years |
parameter | δ1 | δ2 | δ3 | ωδmin | ωδmed |
value | 0 | 0.7506 | 0.5δ2 | 1 week | 2 months |
parameter | ρ0 | τρmin | τρmed | ||
values | 100/(τρmed−τρmin) | 12 years | 22 years |
Parameter | Value |
βh | 0.7379 |
βv | 0.7570 |
b | 3.2158 |
μv | 3.3510 |
γ | 4.5821 |
p | 0.6536 |
δh | 0.7506 |
σ | 0.2558 |
Nv | 19,440.1813 |
parameter | β1 | β2 | β3 | τβmin | τβmed |
value | 1.5β2 | 0.7379 | 0.5β2 | 12 years | 60 years |
parameter | δ1 | δ2 | δ3 | ωδmin | ωδmed |
value | 0 | 0.7506 | 0.5δ2 | 1 week | 2 months |
parameter | ρ0 | τρmin | τρmed | ||
values | 100/(τρmed−τρmin) | 12 years | 22 years |