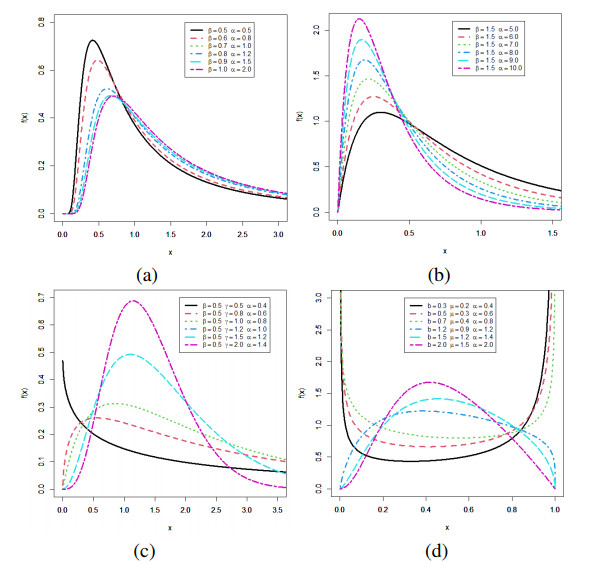
Recent innovations have focused on the creation of new families that extend well-known distributions while providing a huge amount of practical flexibility for data modeling. Weighted distributions offer an effective approach for addressing model building and data interpretation problems. The main objective of this work is to provide a novel family based on a weighted generator called the length-biased truncated Lomax-generated (LBTLo-G) family. Discussions are held about the characteristics of the LBTLo-G family, including expressions for the probability density function, moments, and incomplete moments. In addition, different measures of uncertainty are determined. We provide four new sub-distributions and investigated their functionalities. Subsequently, a statistical analysis is given. The LBTLo-G family's parameter estimation is carried out using the maximum likelihood technique on the basis of full and censored samples. Simulation research is conducted to determine the parameters of the LBTLo Weibull (LBTLoW) distribution. Four genuine data sets are considered to illustrate the fitting behavior of the LBTLoW distribution. In each case, the application outcomes demonstrate that the LBTLoW distribution can, in fact, fit the data more accurately than other rival distributions.
Citation: Amal S. Hassan, Najwan Alsadat, Christophe Chesneau, Ahmed W. Shawki. A novel weighted family of probability distributions with applications to world natural gas, oil, and gold reserves[J]. Mathematical Biosciences and Engineering, 2023, 20(11): 19871-19911. doi: 10.3934/mbe.2023880
[1] | Said G. Nassr, Amal S. Hassan, Rehab Alsultan, Ahmed R. El-Saeed . Acceptance sampling plans for the three-parameter inverted Topp–Leone model. Mathematical Biosciences and Engineering, 2022, 19(12): 13628-13659. doi: 10.3934/mbe.2022636 |
[2] | Walid Emam, Ghadah Alomani . Predictive modeling of reliability engineering data using a new version of the flexible Weibull model. Mathematical Biosciences and Engineering, 2023, 20(6): 9948-9964. doi: 10.3934/mbe.2023436 |
[3] | Manal M. Yousef, Rehab Alsultan, Said G. Nassr . Parametric inference on partially accelerated life testing for the inverted Kumaraswamy distribution based on Type-II progressive censoring data. Mathematical Biosciences and Engineering, 2023, 20(2): 1674-1694. doi: 10.3934/mbe.2023076 |
[4] | M. Nagy, Adel Fahad Alrasheedi . The lifetime analysis of the Weibull model based on Generalized Type-I progressive hybrid censoring schemes. Mathematical Biosciences and Engineering, 2022, 19(3): 2330-2354. doi: 10.3934/mbe.2022108 |
[5] | Walid Emam, Khalaf S. Sultan . Bayesian and maximum likelihood estimations of the Dagum parameters under combined-unified hybrid censoring. Mathematical Biosciences and Engineering, 2021, 18(3): 2930-2951. doi: 10.3934/mbe.2021148 |
[6] | Lernik Asserian, Susan E. Luczak, I. G. Rosen . Computation of nonparametric, mixed effects, maximum likelihood, biosensor data based-estimators for the distributions of random parameters in an abstract parabolic model for the transdermal transport of alcohol. Mathematical Biosciences and Engineering, 2023, 20(11): 20345-20377. doi: 10.3934/mbe.2023900 |
[7] | Wael S. Abu El Azm, Ramy Aldallal, Hassan M. Aljohani, Said G. Nassr . Estimations of competing lifetime data from inverse Weibull distribution under adaptive progressively hybrid censored. Mathematical Biosciences and Engineering, 2022, 19(6): 6252-6275. doi: 10.3934/mbe.2022292 |
[8] | M. G. M. Ghazal, H. M. M. Radwan . A reduced distribution of the modified Weibull distribution and its applications to medical and engineering data. Mathematical Biosciences and Engineering, 2022, 19(12): 13193-13213. doi: 10.3934/mbe.2022617 |
[9] | Raquel Caballero-Águila, María J. García-Ligero, Aurora Hermoso-Carazo, Josefa Linares-Pérez . Unreliable networks with random parameter matrices and time-correlated noises: distributed estimation under deception attacks. Mathematical Biosciences and Engineering, 2023, 20(8): 14550-14577. doi: 10.3934/mbe.2023651 |
[10] | Ibrahim Alkhairy . Classical and Bayesian inference for the discrete Poisson Ramos-Louzada distribution with application to COVID-19 data. Mathematical Biosciences and Engineering, 2023, 20(8): 14061-14080. doi: 10.3934/mbe.2023628 |
Recent innovations have focused on the creation of new families that extend well-known distributions while providing a huge amount of practical flexibility for data modeling. Weighted distributions offer an effective approach for addressing model building and data interpretation problems. The main objective of this work is to provide a novel family based on a weighted generator called the length-biased truncated Lomax-generated (LBTLo-G) family. Discussions are held about the characteristics of the LBTLo-G family, including expressions for the probability density function, moments, and incomplete moments. In addition, different measures of uncertainty are determined. We provide four new sub-distributions and investigated their functionalities. Subsequently, a statistical analysis is given. The LBTLo-G family's parameter estimation is carried out using the maximum likelihood technique on the basis of full and censored samples. Simulation research is conducted to determine the parameters of the LBTLo Weibull (LBTLoW) distribution. Four genuine data sets are considered to illustrate the fitting behavior of the LBTLoW distribution. In each case, the application outcomes demonstrate that the LBTLoW distribution can, in fact, fit the data more accurately than other rival distributions.
Weighted distributions (WDs) provide an approach to deal with model specification and data interpretations problems. They adjust the probabilities of the actual occurrence of events to arrive at a specification of the probabilities when those events are recorded. Reference [1] extended the basic ideas of the methods of ascertainment upon the estimation of frequencies in [2]. The author defined a unifying concept of the WDs and described several sample conditions that the WDs can model. The usefulness and applications of the WDs in various areas, including medicine, ecology, reliability, and branching processes, can also be seen in [3,4,5]. Important findings on the WDs have been reported by several research. For examples, reference [6] suggested a weighted x-gamma distribution, reference [7] derived a new generalized weighted Weibull distribution, reference [8] introduced the weighted exponential-Gompertz distribution, reference [9] studied the new weighted inverse Rayleigh distribution, reference [10] introduced a weighted version of the generalized inverse Weibull distribution, reference [11] proposed a bounded weighted exponential distribution, reference [12] derived a new weighted exponential distribution, reference [13] proposed a weighted power Lomax distribution, reference [14] derived a new generalized weighted exponential distribution, reference [15] introduced a new version of the weighted Weibull distribution, reference [16] proposed the modified weighted exponential distribution, and reference [17] proposed a weighted Nwikpe distribution, reference [18] introduced a new version of the double weighted quasi Lindley distribution and reference [19] proposed the modified length-biased weighted Lomax distribution.
In contrast, statistical models have the capacity to depict and predict real-world phenomena. Over the past few decades, numerous extended distributions have been extensively utilized in data modeling. Recent progress has been centered on the development of novel distribution families that not only enhance existing distributions but also offer significant versatility in practical data modeling. Engineering, economics, biology, and environmental science are particular examples of this. Regarding this, a number of writers suggested some of the created families of continuous distributions, (see for example [20,21,22]). Our interest here is in the same scheme used for the beta-G (B-G) family prepared in [23]. The following is the cumulative distribution function (cdf) for the B-G family:
F(x)=∫G(x)0r(t)dt, | (1.1) |
where G(x) is a cdf of a continuous distribution and r(t) is the probability density function (pdf) of the beta distribution. Naturally, any new family can be created by taking another pdf for r(t) with support [0,1] (see reference [23]).
As a matter of fact, few works about the weighted-G family have been proposed in the literature. For example, reference [24] studied the weighted exponential-G family, reference [25] introduced the weighted exponentiated family, reference [26] proposed a weighted general family, and reference [27] developed a weighted Topp-Leone-G family.
The primary purpose of this study is to introduce the length-biased truncated Lomax-G (LBTLo-G) family. The following arguments give enough motivation to study it:
1) The LBTLo-G family is very flexible and simple.
2) The LBTLo-G family contains some new distributions.
3) The shapes of the pdfs of the generated distributions can be unimodal, decreasing, bathtub, right-skewed, and symmetric. Also, the hazard rate function (hrf) shapes for these distributions can be increasing, decreasing, U-shaped, upside-down-shaped, or J- shaped.
After emphasizing these important aspects, some statistical and mathematical properties of the newly suggested family are discussed. The maximum likelihood (ML) method of estimation is used to estimate the LBTLo Weibull (LBTLoW) model parameters based on complete and type Ⅱ censoring (T2C).
The variability of the LBTLoW distribution is demonstrated through four authentic data sets. The first data set describes age data on rest times (in minutes) for analgesic patients. The second data set shows the percentage of natural gas reserves in 44 countries in 2020. The third authentic data set listed the top 20 countries by oil reserves. Proven reserves refer to the quantities of petroleum that can be predicted as commercially recoverable from known reservoirs, based on the analysis of geological and engineering data. These estimates are made considering existing economic conditions and are projected from a specific period onwards. The fourth data set displays the top 100 central banks in terms of gold reserves. This gold reserve data, collected from IMF IFS figures, tracks central banks' reported gold purchases and sales as a percentage of their international reserves. The application results show that the LBTLoW distribution can indeed match the data better than other competing distributions.
The following is the structure for this article: Section 2 defines the crucial functions of the LBTLo-G family and provides four special distributions of the family. In Section 3, some statistical properties of the LBTLo-G family are provided. Section 4 deals with the ML estimates (MLEs) of the unknown parameters. A simulation study to examine the theoretical performance of MLEs for the LBTLoW distribution is studied in Section 5. Section 6 presents the applicability and goodness of fit of the proposed models using four real data sets. The paper ends with a few last observations, as may be seen in Section 7.
Here, we suggest a new weighted family based on the weighted version of the truncated Lomax distribution, which is called the LBTLo distribution [28]. The cdf and pdf of the LBTLo distribution are, respectively, given by
G(t;α)=Λ(α)[(1+t)−α(1+αt)−1],0<t<1,α>0, | (2.1) |
g(t;α)=α(1−α)Λ(α)t(1+t)−(α+1), | (2.2) |
where Λ(α)=[2−α(1+α)−1]−1. For these functions, it is assumed the standard complementary values for t≤0 and t≥1.
As mentioned in [28], the following advantages of the LBTLo distribution are outlined: (ⅰ) It depends on only one parameter; (ⅱ) the pdf has only one maximum point with a relatively sharp peak and a heavy tail; (ⅲ) the hrf has increasing behavior or is N-shaped; and (ⅳ) it outperforms some other competing models in real-world applications to medical data and the percentage of household spending on education out of total household expenditure from the Household Income, Expenditure, and Consumption Survey data for North Sinai Governorate.
In light of these merits, the LBTLo distribution is a great choice to use in various fields. As a consequence, we present a novel generated family that is based on the LBTLo distribution. In order to define the LBTLo-G family, let G(x;ζ) and g(x;ζ) be the baseline cdf and pdf, respectively, of a continuous distribution, and ζ is a vector of parameters. The generalized B-G generator specified in (1.1) and the LBTLo distribution (2.2) are combined to generate the cdf of the LBTLo-G family:
F(x;α,ζ)=α(1−α)Λ(α)∫G(x;ζ)0t(1+t)−α−1dt=Λ(α)[(1+G(x;ζ))−α(1+αG(x;ζ))−1],x∈R,α>0, | (2.3) |
where α is a shape parameter. Therefore, the pdf of the LBTLo-G family is given by
f(x;α,ζ)=α(1−α)Λ(α)g(x;ζ)G(x;ζ)(1+G(x;ζ))−α−1,x∈R,α>0. | (2.4) |
A random variable X with the pdf (2.4) is designated as X∼ LBTLo-G from here on out. The complementary cdf (ccdf), and hrf, are, provided by
S(x;α,ζ)=1−Λ(α)[(1+G(x;ζ))−α(1+αG(x;ζ))−1], |
h(x;α,ζ)=α(1−α)Λ(α)g(x)G(x)(1+G(x))−α−11−Λ(α)[(1+G(x;ζ))−α(1+αG(x;ζ))−1]. |
We create four new LBTLo-G family sub-distributions in the subsections that follow: LBTLo-inverse exponential, LBTLo-uniform, LBTLo-Weibull, and LBTLo-Kumaraswamy distributions.
The cdf and pdf of the LBTLo-inverse exponential (LBTLoIE) distribution are obtained from (2.3) and (2.4) for G(x;β)=e−(β/x),β,x>0, as follows:
F(x;α,β)=Λ(α)[(1+e−(β/x))−α(1+αe−(β/x))−1],x>0,α,β>0, |
f(x;α,β)=α(1−α)Λ(α)βx−2e−2(β/x)(1+e−(β/x))−α−1. |
Further, the hrf is as follows:
h(x;α,β)=α(1−α)Λ(α)βx−2e−2(β/x)(1+e−(β/x))−α−11−Λ(α)[(1+e−(β/x))−α(1+αe−(β/x))−1]. |
The cdf and pdf of the LBTLo-uniform (LBTLoU) distribution are derived from (2.3) and (2.4) by taking G(x;β)=β−1x,0<x<β, as follows:
F(x;α,β)=[(1+αβ−1x)(1+β−1x)−α−1]Λ(α),0<x<β,α,β>0, |
f(x;α,β)=αβ−2x(1+β−1x)−α−1(1−α)Λ(α). |
Further, the hrf is as follows:
h(x;α,β)=αβ−2x(1+β−1x)−α−1(1−α)Λ(α)1−Λ(α)[(1+αβ−1x)(1+β−1x)−α−1]. |
The cdf and pdf of the LBTLoW distribution are derived from (2.3) and (2.4) taking G(x;β,γ)=1−e−βxγ,x,β,γ>0, as follows:
F(x;α,β,γ)=[(2−e−βxγ)−α(1+α−αe−βxγ)−1]Λ(α),x>0,α,β,γ>0, | (2.5) |
f(x;α,β,γ)=αβγ(1−α)xγ−1e−βxγ(1−e−βxγ)(2−e−βxγ)−α−1Λ(α). | (2.6) |
Further, the hrf is:
h(x;α,β,γ)=αβγ(1−α)xγ−1e−βxγ(1−e−βxγ)(2−e−βxγ)−α−1Λ(α)1−Λ(α)[(2−e−βxγ)−α(1+α−αe−βxγ)−1]. |
The cdf and pdf of the LBTLo- Kumaraswamy (LBTLoKw) distribution are obtained from (2.3) and (2.4) by taking G(x;μ,b)=1−(1−xμ)b,0<x<1,b,μ>0, as follows:
F(x;α,μ,b)=[(2−(1−xμ)b)−α(1+α−α(1−xμ)b)−1]Λ(α),0<x<1,α,μ,b>0, |
f(x;α,μ,b)=αμb(1−α)xμ−1(1−xμ)b−1(1−(1−xμ)b)×(2−(1−xμ)b)−α−1Λ(α). |
Further, the hrf is as follows:
h(x;α,μ,b)=αμb(1−α)xμ−1(1−xμ)b−1(1−(1−xμ)b)Λ(α)(2−(1−xμ)b)−α−11−Λ(α)[(2−(1−xμ)b)−α(1+α−α(1−xμ)b)−1]. |
The plots of pdf and hrf for the LBTLoIE, LBTLoU, LBTLoW and LBTLoKw distributions are given in Figures 1 and 2, respectively.
The pdfs of the investigated distributions can have a variety of forms, including right- and left-skewed, bathtub, uni-modal, declining, and symmetric shapes, as shown in Figure 1. The corresponding hrf can take any form, including U, J, reverse J, growing, or decreasing, as seen in Figure 1.
In this part, we give some statistical properties of the LBTLo-G family.
The LBTLo-G family representations in pdf and cdf format are displayed here. The generalized binomial theorem says that
(1+z)−β=∞∑i=0(−1)i(β+i−1i)zi, | (3.1) |
for |z|<1. Hence, by using (3.1) in (2.4), the pdf of the LBTLo-G family can be written as follows:
f(x;α,ζ)=∞∑i=0ϑig(x;ζ)G(x;ζ)i+1,x∈R, | (3.2) |
where ϑi=(−1)iα(1−α)Λ(α)(α+ii). For example, the expansion of pdf of the LBTLoW distribution is derived from (3.2) as follows:
f(x;α,β,γ)=βγ∞∑i=0ϑixγ−1e−βxγ(1−e−βxγ)i+1,x>0,α,β,γ>0. | (3.3) |
But, in the special case where b is a positive integer, the standard generalized binomial theorem says that
(1−z)b=b∑ν=0(−1)ν(bν)zν. | (3.4) |
Then using the binomial expansion (3.4) in (3.3), we get
f(x;α,β,γ)=∞∑i=0i+1∑ν=0ϑi,νxγ−1e−β(ν+1)xγ, | (3.5) |
where ϑi,ν=βγϑi(−1)ν(i+1ν). In what follows, an expansion for F(x;α,ζ)his derived, for h is an integer, again, the exponential and the binomial expansions are worked out:
F(x;α,ζ)h=Λ(α)h[(1+G(x;ζ))−α(1+αG(x;ζ))−1]h. | (3.6) |
Using the binomial expansion (3.4) in (3.6), we get
F(x;α,ζ)h=Λ(α)hh∑j=0(−1)h−j(hj)(1+G(x;ζ))−αj(1+αG(x;ζ))j. | (3.7) |
Using the binomial expansion (3.1), we obtain
F(x;α,ζ)h=Λ(α)h∞∑d=0h∑j=0(−1)d+h−j(hj)(αj+d−1d)×G(x;ζ)d(1+αG(x;ζ))j. | (3.8) |
By using (3.4) in (3.8), we obtain
F(x;α,ζ)h=∞∑d=0ϖd,j,mG(x;ζ)d+m, | (3.9) |
where ϖd,j,m=∑hj=0∑jm=0(−1)d+m+h−jαm(hj)(jm)(αj+d−1d)Λ(α)h.
For example, the expansion of the cdf of the LBTLoW distribution is derived from (3.9), where G(x,ζ)=1−e−βxγ, as follows:
F(x;α,β,γ)h=∞∑d=0ϖd,j,m(1−e−βxγ)d+m. |
By using the binomial expansion (3.4) in the last term of the previous equation, we get
F(x;α,β,γ)h=∞∑d=0d+m∑l=0(−1)l(d+ml)ϖd,j,me−βlxγ. | (3.10) |
The above representations are of interest to express various important moment measures as series. By truncating the index of summation, we can have a precise approximation with a reasonable computation cost.
As a special class of moments, the probability weighted moments (PWMs) have been proposed in [29]. This class is used to derive estimates of the parameters and quantiles of distributions expressible in inverse form. Let X be a random variable with pdf and cdf f(x) and F(x), respectively, and r and q be non-negative integers. Then, the (r,q)th PWM of X, denoted by πr,q, can be calculated through the following relation:
πr,q=E[XrF(X)q]=∫∞−∞xrf(x)F(x)qdx. | (3.11) |
On this basis, the (r,q)th PMW of X with pdf and cdf of the LBTLo-G family is obtained by substituting (3.2) and (3.9) into (3.11), as follows:
πr,q=E[XrF(X;α,ζ)q]=∫∞−∞∞∑i,d=0ϑiϖd,j,mxrg(x;ζ)[G(x;ζ)]i+d+m+1dx. |
Then, provided that the interchange of the integral and sum is valid, depending on the definitions of g(x;ζ) and G(x;ζ), we have
πr,q=∞∑i,d=0ϑiϖd,j,mρr,i+d+m+1, |
where
ρr,i+d+m+1=∫∞−∞xrg(x;ζ)[G(x;ζ)]i+d+m+1dx. |
For example, the (r,q)th of a random variable X that follows the LBTLoW distribution can be obtained by substituting (3.5) and (3.10) into (3.11), and replacing h with q. We thus obtain
πr,q=∞∑i,d=0i+1∑v=0d+m∑l=0(−1)lϑi,vϖd,j,m(β(ν+l+1))rγ+1(d+ml)Γ(rγ+1), |
where Γ(.) stands for gamma function.
In this part, for any non-negative integer r, the rth moment associated with the LBTLo-G family is derived.
Let X be a random variable having the pdf of the LBTLo-G family. Then, the rth moment of X is obtained as follows:
μ′r=E(Xr)=∫∞−∞∞∑i=0ϑixrg(x;ζ)[G(x;ζ)]i+1dx=∞∑i=0ϑiυr,i+1, |
where υr,i+1 is the (r,i+1)th PWM of the baseline distribution. For example, after some developments, the rth moment associated with LBTLoW distribution is given by
μ′r=∞∑i=0i+1∑ν=0ϑi,ν(β(ν+1))rγ+1Γ(rγ+1). |
Tables 1–3 show the numerical values of the first four moments μ′1, μ′2, μ′3, μ′4, also the numerical values of variance (σ2), coefficient of skewness (CS), coefficient of kurtosis (CK) and coefficient of variation (CV) associated with the LBTLoW and LBTLoIE distribution.
γ | α | μ′1 | μ′2 | μ′3 | μ′4 | σ2 | CS | CK | CV |
0.4 | 0.2 | 2.629 | 8.323 | 30.531 | 126.387 | 1.413 | 0.729 | 3.596 | 0.452 |
0.6 | 0.5 | 1.558 | 2.937 | 6.430 | 15.922 | 0.508 | 0.746 | 3.627 | 0.457 |
0.8 | 0.8 | 1.184 | 1.700 | 2.847 | 5.403 | 0.300 | 0.765 | 3.660 | 0.463 |
1.1 | 1.2 | 0.925 | 1.044 | 1.380 | 2.074 | 0.189 | 0.793 | 3.717 | 0.470 |
1.3 | 1.5 | 0.805 | 0.794 | 0.920 | 1.216 | 0.146 | 0.814 | 3.758 | 0.475 |
1.7 | 1.8 | 0.705 | 0.615 | 0.634 | 0.748 | 0.117 | 0.856 | 3.853 | 0.486 |
1.9 | 2.0 | 0.655 | 0.532 | 0.513 | 0.568 | 0.103 | 0.878 | 3.906 | 0.491 |
2.4 | 2.3 | 0.582 | 0.425 | 0.371 | 0.374 | 0.086 | 0.937 | 4.058 | 0.503 |
2.7 | 2.6 | 0.530 | 0.355 | 0.285 | 0.266 | 0.073 | 0.974 | 4.162 | 0.511 |
3.2 | 3.0 | 0.469 | 0.280 | 0.203 | 0.172 | 0.060 | 1.038 | 4.359 | 0.522 |
γ | α | μ′1 | μ′2 | μ′3 | μ′4 | σ2 | CS | CK | CV |
0.4 | 0.2 | 1.964 | 4.277 | 10.154 | 25.945 | 0.420 | 0.380 | 3.017 | 0.330 |
0.6 | 0.5 | 1.347 | 2.017 | 3.298 | 5.813 | 0.202 | 0.395 | 3.026 | 0.334 |
0.8 | 0.8 | 1.105 | 1.360 | 1.829 | 2.658 | 0.139 | 0.411 | 3.037 | 0.337 |
1.1 | 1.2 | 0.924 | 0.954 | 1.081 | 1.325 | 0.100 | 0.435 | 3.058 | 0.343 |
1.3 | 1.5 | 0.836 | 0.783 | 0.805 | 0.897 | 0.084 | 0.453 | 3.075 | 0.346 |
1.7 | 1.8 | 0.760 | 0.649 | 0.612 | 0.627 | 0.072 | 0.489 | 3.116 | 0.353 |
1.9 | 2.0 | 0.720 | 0.584 | 0.524 | 0.511 | 0.066 | 0.508 | 3.140 | 0.356 |
2.4 | 2.3 | 0.661 | 0.494 | 0.411 | 0.374 | 0.058 | 0.557 | 3.213 | 0.365 |
2.7 | 2.6 | 0.617 | 0.433 | 0.339 | 0.290 | 0.052 | 0.588 | 3.265 | 0.369 |
3.2 | 3.0 | 0.565 | 0.364 | 0.263 | 0.209 | 0.045 | 0.641 | 3.366 | 0.377 |
β | α | μ′1 | μ′2 | μ′3 | μ′4 | σ2 | CS | CK | CV |
1.5 | 0.2 | 0.029 | 0.026 | 0.023 | 0.021 | 0.025 | 5.273 | 29.151 | 5.386 |
0.5 | 0.032 | 0.028 | 0.025 | 0.023 | 0.027 | 5.014 | 26.463 | 5.138 | |
0.8 | 0.035 | 0.031 | 0.028 | 0.025 | 0.030 | 4.772 | 24.069 | 4.906 | |
1.2 | 0.039 | 0.035 | 0.031 | 0.028 | 0.033 | 4.472 | 21.275 | 4.622 | |
1.5 | 0.043 | 0.038 | 0.033 | 0.03 | 0.036 | 4.264 | 19.441 | 4.424 | |
1.8 | 0.046 | 0.041 | 0.036 | 0.033 | 0.038 | 4.069 | 17.801 | 4.241 | |
2 | 0.049 | 0.043 | 0.038 | 0.034 | 0.040 | 3.946 | 16.806 | 4.125 | |
2.3 | 0.053 | 0.046 | 0.041 | 0.037 | 0.043 | 3.771 | 15.445 | 3.961 | |
2.6 | 0.056 | 0.049 | 0.044 | 0.039 | 0.046 | 3.607 | 14.225 | 3.809 | |
3 | 0.062 | 0.054 | 0.048 | 0.043 | 0.050 | 3.405 | 12.794 | 3.621 | |
2.5 | 0.2 | 0.006 | 0.005 | 0.005 | 0.004 | 0.005 | 12.420 | 156.328 | 12.403 |
0.5 | 0.007 | 0.006 | 0.006 | 0.005 | 0.006 | 11.672 | 138.195 | 11.665 | |
0.8 | 0.007 | 0.007 | 0.006 | 0.006 | 0.007 | 10.981 | 122.434 | 10.984 | |
1.2 | 0.009 | 0.008 | 0.007 | 0.007 | 0.008 | 10.140 | 104.548 | 10.156 | |
1.5 | 0.01 | 0.009 | 0.008 | 0.007 | 0.009 | 9.563 | 93.121 | 9.589 | |
1.8 | 0.011 | 0.010 | 0.009 | 0.008 | 0.010 | 9.030 | 83.140 | 9.066 | |
2 | 0.012 | 0.011 | 0.010 | 0.009 | 0.010 | 8.697 | 77.190 | 8.739 | |
2.3 | 0.013 | 0.012 | 0.011 | 0.010 | 0.012 | 8.227 | 69.194 | 8.279 | |
2.6 | 0.014 | 0.013 | 0.012 | 0.011 | 0.013 | 7.792 | 62.179 | 7.853 | |
3 | 0.017 | 0.015 | 0.014 | 0.013 | 0.015 | 7.261 | 54.128 | 7.334 |
It can be seen from Tables 1–3 that, when the value of α,γ increases for a fixed value of β, the first four moments and σ2 decrease, while the CS, CK, and CV measures increase. When the value of β increases for a fixed value of α and γ, we observe that the first four moments and σ decrease and then increase, while the CS, CK, and CV measures increase. The LBTLoW distribution is skewed to the right by leptokurtic curves.
Furthermore, if X is a random variable having the pdf of the LBTLo-G family, then the rth incomplete moment of X is obtained as follows:
φr(t)=E(XrI{X≤t})=∫t−∞xrf(x;α,ζ)dx=∫t−∞∞∑i=0ϑixrg(x;ζ)G(x;ζ)i+1dx. |
For example, after some developments, the rth incomplete moment associated with the LBTLoW distribution is given by
φr(t)=∞∑i=0i+1∑ν=0ϑi,ν[β(ν+1)]rγ+1Γ(rγ+1,β(ν+1)tγ), |
where Γ(.,x) is the lower incomplete gamma function.
Here, some uncertainty measures of the LBTLo-G family are derived. Then, these measures are specialized to the LBTLoW distribution. To begin, the Rényi entropy (RE), presented in [30], associated with a distribution with pdf f(x), is defined by
IR(ε)=11−εlog[∫∞−∞f(x)εdx],ε≠1,ε>0. |
A numerical study with integral calculus is possible; here, we focus on a series expansion. In what follows, an expansion for f(x;α,ζ)ε is derived, for ε is a non-integer (again, the generalized binomial expansion is worked out):
f(x;α,ζ)ε=∞∑i=0Δig(x;ζ)εG(x;ζ)i+ε, |
where
Δi=(−1)i[α(1−α)Λ(α)]ε(ε(α+1)+i−1i). |
Then, the RE associated with the LBTLo-G family is given by
IR(ε)=(1−ε)−1log{∫∞−∞∞∑i=0Δig(x;ζ)εG(x;ζ)i+εdx}. |
For example, the RE associated with the LBTLoW distribution can be obtained as follows:
IR(ε)=(1−ε)−1log{∞∑i,j=0Δi,jγ[β(ε+j)]−ε+(ε/γ)−(1/γ)Γ(ε−εγ+1γ)}. |
The Havrda and Charvát entropy (HaCE) (see [31]) associated with a distribution with pdf f(x) is defined by
HCR(ε)=121−ε−1({∫∞−∞f(x)εdx}1/ε−1),ε≠1,ε>0. |
Hence, the HaCE of the LBTLo-G family is given by
HCR(ε)=121−ε−1({∫∞−∞∞∑i=0Δig(x;ζ)εG(x;ζ)i+εdx}1/ε−1). |
For example, the HaCE of the LBTLoW distribution can be obtained as follows:
HCR(ε)=121−ε−1({∞∑i,j=0Δi,jγ[β(ε+j)]−ε+(ε/γ)−(1/γ)Γ(ε−εγ+1γ)}1/ε−1). |
The Arimoto entropy (ArE) (see [32]) associated with a distribution with pdf f(x) is defined by
AR(ε)=ε1−ε({∫∞−∞f(x)εdx}1/ε−1),ε≠1,ε>0. |
Hence, the ArE of the LBTLo-G family is given by
AR(ε)=ε1−ε({∫∞−∞∞∑i=0Δig(x;ζ)εG(x;ζ)i+εdx}1/ε−1). |
For example, the ArE of the LBTLoW distribution can be obtained as follows:
AR(ε)=ε1−ε({∞∑i,j=0Δi,jγ[β(ε+j)]−ε+(ε/γ)−(1/γ)Γ(ε−εγ+1γ)}1/ε−1). |
The Tsallis entropy (TsE) (see [33]) associated with a distribution with pdf f(x), is defined by
TR(ε)=1ε−1{1−∫∞−∞f(x)εdx},ε≠1,ε>0. |
Hence, the TsE of the LBTLo-G family is obtained as follows:
TR(ε)=1ε−1{1−∫∞−∞∞∑i=0Δig(x;ζ)εG(x;ζ)i+εdx}. |
For example, the TsE of the LBTLoW distribution can be obtained as follows:
TR(ε)=1ε−1{1−∞∑i,j=0Δi,jγ[β(ε+j)]−ε+(ε/γ)−(1/γ)Γ(ε−εγ+1γ)}. |
Some numerical values for the proposed entropy measures are obtained for the LBTLoW and LBTLoIE distribution in Tables 4 and 5.
ε | β | α | γ | RE | HaCE | ArE | TsE |
1.5 | 0.25 | 0.2 | 0.4 | 3.331 | 3.340 | 2.767 | 1.957 |
0.5 | 0.6 | 3.252 | 3.333 | 2.753 | 1.953 | ||
0.8 | 0.8 | 3.099 | 3.318 | 2.722 | 1.944 | ||
1.2 | 1.1 | 2.875 | 3.290 | 2.670 | 1.927 | ||
1.5 | 1.3 | 2.716 | 3.264 | 2.627 | 1.912 | ||
1.8 | 1.7 | 2.524 | 3.227 | 2.568 | 1.891 | ||
2.0 | 1.9 | 2.415 | 3.203 | 2.53 | 1.876 | ||
2.3 | 2.4 | 2.229 | 3.152 | 2.458 | 1.846 | ||
2.6 | 2.7 | 2.084 | 3.104 | 2.394 | 1.818 | ||
3.0 | 3.2 | 1.889 | 3.026 | 2.296 | 1.773 | ||
0.5 | 0.2 | 0.4 | 1.930 | 3.044 | 2.318 | 1.783 | |
0.5 | 0.6 | 1.924 | 3.042 | 2.315 | 1.782 | ||
0.8 | 0.8 | 1.824 | 2.996 | 2.260 | 1.755 | ||
1.2 | 1.1 | 1.660 | 2.909 | 2.161 | 1.704 | ||
1.5 | 1.3 | 1.541 | 2.835 | 2.081 | 1.661 | ||
1.8 | 1.7 | 1.392 | 2.726 | 1.969 | 1.597 | ||
2.0 | 1.9 | 1.308 | 2.657 | 1.901 | 1.556 | ||
2.3 | 2.4 | 1.161 | 2.517 | 1.770 | 1.475 | ||
2.6 | 2.7 | 1.051 | 2.397 | 1.661 | 1.404 | ||
3.0 | 3.2 | 0.903 | 2.207 | 1.500 | 1.293 | ||
2.0 | 0.25 | 0.2 | 0.4 | 2.180 | 1.987 | 1.837 | 0.993 |
0.5 | 0.6 | 2.167 | 1.986 | 1.835 | 0.993 | ||
0.8 | 0.8 | 2.053 | 1.982 | 1.812 | 0.991 | ||
1.2 | 1.1 | 1.876 | 1.973 | 1.769 | 0.987 | ||
1.5 | 1.3 | 1.753 | 1.965 | 1.734 | 0.982 | ||
1.8 | 1.7 | 1.595 | 1.949 | 1.681 | 0.975 | ||
2.0 | 1.9 | 1.510 | 1.938 | 1.648 | 0.969 | ||
2.3 | 2.4 | 1.358 | 1.912 | 1.581 | 0.956 | ||
2.6 | 2.7 | 1.247 | 1.887 | 1.524 | 0.943 | ||
3.0 | 3.2 | 1.098 | 1.840 | 1.435 | 0.920 | ||
0.5 | 0.2 | 0.4 | 1.210 | 1.877 | 1.503 | 0.938 | |
0.5 | 0.6 | 1.279 | 1.895 | 1.541 | 0.947 | ||
0.8 | 0.8 | 1.210 | 1.877 | 1.503 | 0.938 | ||
1.2 | 1.1 | 1.080 | 1.834 | 1.423 | 0.917 | ||
1.5 | 1.3 | 0.987 | 1.794 | 1.358 | 0.897 | ||
1.8 | 1.7 | 0.861 | 1.725 | 1.258 | 0.862 | ||
2.0 | 1.9 | 0.795 | 1.679 | 1.199 | 0.840 | ||
2.3 | 2.4 | 0.672 | 1.574 | 1.077 | 0.787 | ||
2.6 | 2.7 | 0.587 | 1.482 | 0.982 | 0.741 | ||
3.0 | 3.2 | 0.470 | 1.323 | 0.836 | 0.661 |
ε | β | α | RE | HaCE | ArE | TsE |
1.5 | 0.25 | 0.2 | 7.001 | 3.311 | 2.709 | 1.94 |
0.5 | 7.075 | 3.315 | 2.716 | 1.942 | ||
0.8 | 7.156 | 3.319 | 2.724 | 1.944 | ||
1.2 | 7.274 | 3.324 | 2.735 | 1.947 | ||
1.5 | 7.372 | 3.329 | 2.743 | 1.95 | ||
1.8 | 7.476 | 3.333 | 2.752 | 1.952 | ||
2 | 7.55 | 3.336 | 2.758 | 1.954 | ||
2.3 | 7.667 | 3.34 | 2.767 | 1.957 | ||
2.6 | 7.792 | 3.345 | 2.777 | 1.959 | ||
3 | 7.971 | 3.351 | 2.79 | 1.963 | ||
0.4 | 0.2 | 6.441 | 3.278 | 2.65 | 1.92 | |
0.5 | 6.452 | 3.279 | 2.651 | 1.921 | ||
0.8 | 6.469 | 3.28 | 2.653 | 1.921 | ||
1.2 | 6.503 | 3.282 | 2.657 | 1.923 | ||
1.5 | 6.536 | 3.284 | 2.66 | 1.924 | ||
1.8 | 6.577 | 3.287 | 2.665 | 1.925 | ||
2 | 6.608 | 3.289 | 2.668 | 1.927 | ||
2.3 | 6.661 | 3.292 | 2.674 | 1.928 | ||
2.6 | 6.721 | 3.296 | 2.681 | 1.931 | ||
3 | 6.813 | 3.301 | 2.69 | 1.934 | ||
2.0 | 0.25 | 0.2 | 4.376 | 1.975 | 1.776 | 0.987 |
0.5 | 4.429 | 1.976 | 1.782 | 0.988 | ||
0.8 | 4.487 | 1.977 | 1.788 | 0.989 | ||
1.2 | 4.57 | 1.979 | 1.796 | 0.99 | ||
1.5 | 4.639 | 1.981 | 1.803 | 0.99 | ||
1.8 | 4.713 | 1.982 | 1.81 | 0.991 | ||
2 | 4.765 | 1.983 | 1.815 | 0.991 | ||
2.3 | 4.847 | 1.984 | 1.823 | 0.992 | ||
2.6 | 4.934 | 1.986 | 1.83 | 0.993 | ||
3 | 5.058 | 1.987 | 1.841 | 0.994 | ||
0.4 | 0.2 | 3.975 | 1.962 | 1.726 | 0.981 | |
0.5 | 3.987 | 1.963 | 1.728 | 0.981 | ||
0.8 | 4.003 | 1.963 | 1.73 | 0.982 | ||
1.2 | 4.031 | 1.965 | 1.734 | 0.982 | ||
1.5 | 4.058 | 1.965 | 1.737 | 0.983 | ||
1.8 | 4.09 | 1.967 | 1.741 | 0.983 | ||
2 | 4.114 | 1.967 | 1.744 | 0.984 | ||
2.3 | 4.154 | 1.969 | 1.749 | 0.984 | ||
2.6 | 4.199 | 1.97 | 1.755 | 0.985 | ||
3 | 4.267 | 1.972 | 1.763 | 0.986 |
We can see from these tables that, as the value of ε rises, all entropy values decrease, providing more information. For a fixed value of β, as the values of α and γ rise, we infer that all entropy metrics decrease, indicating that there is less fluctuation. Additionally, we deduce that all entropies have less variability as the values of α, γ and β increase. When compared to other measures, the TsE measure values typically have the smallest values.
Let x(1)≤x(2)≤…≤x(n) be a T2C of size r resulting from a life test on n items whose lifetimes are described by the LBTLo-G family with a given set of parameters α and ζ, see [34,35,36,37]. The log-likelihood function of r failures and (n−r) censored values, is given by
logL(α,ζ)=rlogα+rlog(1−α)+rlogΛ(α)+r∑i=1logg(xi;ζ)+r∑i=1logG(xi;ζ)−(α+1)r∑i=1log(1+G(xi;ζ))+(n−r)log[Ar(α,ζ)], |
where Ar(α,ζ)=1−Λ(α)[(1+G(xr;ζ))−α(1+αG(xr;ζ))−1], and we write x(i)=xi for simplified form.
By maximizing the previous likelihood function, the MLEs of unknown parameters are determined. To achieve this, we can first compute the first derivative of the score function (Uα,Uζk), given as follows:
Uα=rα−r1−α+rΛ(α)(∂∂αΛ(α))−r∑i=1log(1+G(xi;ζ))+(n−r)Ar(α,ζ)(∂∂αAr(α,ζ)), |
Uζk=−(α+1)r∑i=111+G(xi;ζ)∂∂ζk(G(xi;ζ))+(n−r)Ar(α,ζ)∂∂ζkAr(α,ζ), |
where
∂∂αΛ(α)=[Λ(α)]22−α[(1+α)log2−1], |
∂∂αAr(α,ζ)=−∂∂αΛ(α)[(1+G(xr;ζ))−α(1+αG(xr;ζ))−1]+Λ(α)[(1+G(xr;ζ))−α{(1+αG(xr;ζ))log(1+G(xr;ζ))−G(xr;ζ)}], |
and
∂∂ζk(Ar(α,ζ))=α(α−1)Λ(α)G(xr;ζ)(1+G(xr;ζ))−α−1∂∂ζkG(xr;ζ). |
By putting Uα and Uζk equal to zero and solving these equations simultaneously, the MLEs of the LBTLo-G family are found. These equations are not amenable to analytical solution, however they are amenable to numerical solution by iterative techniques utilizing statistical software.
The confidence interval (CI) of the vector of the unknown parameters ξ=(α,ζ) could be obtained from the asymptotic distribution of the MLEs of the parameters as (ˆξMLE−ξ)→N2(0,I−1(ˆξMLE)), where I(ξ) is the Fisher information matrix. Under particular regularity conditions, the two-sided 100(1−v), asymptotic CI for the vector of unknown parameters ξ can be acquired in the following ways: ˆξMLE±zv/2√var(ˆξ), where var(ˆξ) is the element of the main diagonal of the asymptotic variance-covariance matrix I−1(ˆξMLE) and zv/2 is the upper vth/2 percentile of the standard normal distribution.
This section includes a simulation study to evaluate the performance of the MLEs for the LBTLoW model (α,β,γ), for complete and T2C. The Mathematica 9 package is used to get the mean squared error (MSE), lower bound (LB) of CI, upper bound (UB) of CI, average length (AL) of 95%, and coverage probability (CP) of 95% of the estimated values of α, β and γ. The algorithm is developed in the way described below:
1) From the LBTLoW distribution, 5000 random samples of sizes n = 50,100,150, and 200 are created.
2) Values of the unknown parameters (α,β,γ) are selected as Set 1 =(α=0.5,β=0.5,γ=0.5), Set 2 =(α=0.7,β=0.5,γ=0.25), Set 3 =(α=0.7,β=0.7,γ=0.5), and Set 4 =(α=0.6,β=0.3,γ=0.5).
3) Three levels of censorship are chosen: r = 70%, 80% (T2C), and 100% (complete sample).
4) The MLEs, Biases, and MSEs for all sample sizes and for all selected sets of parameters are computed. Furthermore, the LB, UB, AL, and CP with a confidence level of 0.95 for all sample sizes and for all selected sets of parameters are calculated.
5) Numerical outcomes are reported in Table 6. Based on complete and T2C samples, we can detect the following about the performance of the estimated parameters.
n | r | Set1 (α = 0.5, β = 0.5, γ = 0.5) | |||||||
MLE | Bias | MSE | LB | UB | AL | CP | |||
50 | 70% | α | 0.4204 | 0.0796 | 0.0064 | 0.0019 | 0.839 | 0.8370 | 97.4% |
β | 0.7041 | 0.2041 | 0.0471 | 0.5036 | 0.9046 | 0.4010 | 96.9% | ||
γ | 0.4201 | 0.0799 | 0.0069 | 0.3023 | 0.5379 | 0.2356 | 96.0% | ||
80% | α | 0.4218 | 0.0782 | 0.0061 | 0.0191 | 0.8245 | 0.8053 | 94.8% | |
β | 0.6382 | 0.1382 | 0.0242 | 0.4508 | 0.8256 | 0.3748 | 95.8% | ||
γ | 0.4386 | 0.0614 | 0.0053 | 0.3282 | 0.5490 | 0.2208 | 97.1% | ||
100% | α | 0.4234 | 0.0766 | 0.0059 | 0.0357 | 0.8111 | 0.7754 | 95.4% | |
β | 0.5177 | 0.0177 | 0.0056 | 0.3661 | 0.6694 | 0.3033 | 95.5% | ||
γ | 0.5316 | 0.0316 | 0.0027 | 0.4303 | 0.6328 | 0.2025 | 96.0% | ||
100 | 70% | α | 0.4213 | 0.0787 | 0.0062 | 0.0844 | 0.7583 | 0.6740 | 96.2% |
β | 0.6750 | 0.1750 | 0.0312 | 0.5375 | 0.8125 | 0.2750 | 95.9% | ||
γ | 0.4237 | 0.0763 | 0.0065 | 0.3389 | 0.5084 | 0.1694 | 96.0% | ||
80% | α | 0.4230 | 0.0770 | 0.0061 | 0.2099 | 0.6360 | 0.4262 | 96.2% | |
β | 0.6099 | 0.1099 | 0.0127 | 0.4819 | 0.7379 | 0.2560 | 96.1% | ||
γ | 0.4487 | 0.0513 | 0.0033 | 0.3652 | 0.5321 | 0.1669 | 97.3% | ||
100% | α | 0.4238 | 0.0762 | 0.0058 | 0.2501 | 0.5975 | 0.3473 | 95.6% | |
β | 0.4683 | 0.0317 | 0.0027 | 0.3558 | 0.5807 | 0.2249 | 95.8% | ||
γ | 0.4967 | 0.0033 | 0.0025 | 0.4199 | 0.5734 | 0.1535 | 96.0% | ||
150 | 70% | α | 0.4217 | 0.0783 | 0.0061 | 0.2710 | 0.5725 | 0.3015 | 95.2% |
β | 0.6626 | 0.1626 | 0.0281 | 0.5577 | 0.7675 | 0.2097 | 95.6% | ||
γ | 0.4277 | 0.0723 | 0.0058 | 0.3571 | 0.4983 | 0.1412 | 97.3% | ||
80% | α | 0.4236 | 0.0764 | 0.0059 | 0.3005 | 0.5466 | 0.2461 | 95.7% | |
β | 0.5977 | 0.0977 | 0.0113 | 0.4957 | 0.6997 | 0.2040 | 96.2% | ||
γ | 0.4649 | 0.0351 | 0.0022 | 0.3972 | 0.5325 | 0.1353 | 97.0% | ||
100% | α | 0.4238 | 0.0762 | 0.0058 | 0.3010 | 0.5467 | 0.2457 | 95.6% | |
β | 0.4766 | 0.0234 | 0.0023 | 0.3784 | 0.5749 | 0.1965 | 96.4% | ||
γ | 0.5277 | 0.0277 | 0.0015 | 0.4659 | 0.5894 | 0.1236 | 96.9% | ||
200 | 70% | α | 0.4219 | 0.0781 | 0.0061 | 0.3154 | 0.5285 | 0.2132 | 96.1% |
β | 0.6592 | 0.1592 | 0.0268 | 0.5675 | 0.7510 | 0.1835 | 96.3% | ||
γ | 0.4375 | 0.0625 | 0.0046 | 0.3789 | 0.4962 | 0.1173 | 96.7% | ||
80% | α | 0.4239 | 0.0761 | 0.0058 | 0.3236 | 0.5242 | 0.2006 | 96.3% | |
β | 0.5912 | 0.0912 | 0.0099 | 0.5074 | 0.6750 | 0.1676 | 97.0% | ||
γ | 0.4667 | 0.0333 | 0.0020 | 0.4101 | 0.5233 | 0.1132 | 97.5% | ||
100% | α | 0.4240 | 0.0760 | 0.0058 | 0.3372 | 0.5109 | 0.1737 | 96.5% | |
β | 0.4905 | 0.0095 | 0.0009 | 0.4167 | 0.5642 | 0.1475 | 96.7% | ||
γ | 0.5035 | 0.0035 | 0.0006 | 0.4524 | 0.5546 | 0.1022 | 97.1% | ||
n | r | Set2 (α = 0.7, β = 0.5, γ = 0.25) | |||||||
MLE | Bias | MSE | LB | UB | AL | CP | |||
50 | 70% | α | 0.4206 | 0.2794 | 0.0782 | 0.0055 | 0.8358 | 0.8304 | 97.7% |
β | 0.7016 | 0.2016 | 0.0462 | 0.5015 | 0.9016 | 0.4001 | 96.5% | ||
γ | 0.2172 | 0.0328 | 0.0017 | 0.1568 | 0.2776 | 0.1208 | 100% | ||
80% | α | 0.4214 | 0.2786 | 0.0776 | 0.2084 | 0.6345 | 0.4261 | 97.9% | |
β | 0.6361 | 0.1361 | 0.0243 | 0.4490 | 0.8231 | 0.3741 | 98.5% | ||
γ | 0.2297 | 0.0203 | 0.0010 | 0.1719 | 0.2874 | 0.1155 | 100% | ||
100% | α | 0.4234 | 0.2766 | 0.0765 | 0.2497 | 0.5970 | 0.3473 | 98.3% | |
β | 0.5161 | 0.0161 | 0.0062 | 0.3649 | 0.6673 | 0.3025 | 97.6% | ||
γ | 0.2565 | 0.0065 | 0.0007 | 0.2044 | 0.3086 | 0.1043 | 100% | ||
100 | 70% | α | 0.4210 | 0.2790 | 0.0779 | 0.0208 | 0.8212 | 0.8004 | 96.4% |
β | 0.7006 | 0.2006 | 0.0431 | 0.5593 | 0.8419 | 0.2826 | 98.0% | ||
γ | 0.2141 | 0.0359 | 0.0016 | 0.1720 | 0.2562 | 0.0842 | 100% | ||
80% | α | 0.4215 | 0.2785 | 0.0776 | 0.2708 | 0.5721 | 0.3013 | 97.2% | |
β | 0.6357 | 0.1357 | 0.0214 | 0.5035 | 0.7680 | 0.2645 | 97.7% | ||
γ | 0.2270 | 0.0230 | 0.0008 | 0.1866 | 0.2673 | 0.0807 | 100% | ||
100% | α | 0.4234 | 0.2766 | 0.0765 | 0.3006 | 0.5462 | 0.2456 | 97.3% | |
β | 0.5158 | 0.0158 | 0.0033 | 0.4088 | 0.6227 | 0.2140 | 98.2% | ||
γ | 0.2540 | 0.0040 | 0.0003 | 0.2176 | 0.2905 | 0.0729 | 100% | ||
150 | 70% | α | 0.4212 | 0.2788 | 0.0778 | 0.0330 | 0.8093 | 0.7763 | 97.7% |
β | 0.7000 | 0.2000 | 0.0419 | 0.5847 | 0.8153 | 0.2306 | 97.7% | ||
γ | 0.2122 | 0.0378 | 0.0016 | 0.1781 | 0.2463 | 0.0682 | 100% | ||
80% | α | 0.4215 | 0.2785 | 0.0776 | 0.2985 | 0.5445 | 0.2460 | 98.8% | |
β | 0.6350 | 0.1350 | 0.0203 | 0.5271 | 0.7430 | 0.2159 | 98.1% | ||
γ | 0.2259 | 0.0241 | 0.0008 | 0.1931 | 0.2587 | 0.0656 | 96.0% | ||
100% | α | 0.4234 | 0.2766 | 0.0765 | 0.3232 | 0.5237 | 0.2005 | 97.2% | |
β | 0.5151 | 0.0151 | 0.0023 | 0.4278 | 0.6024 | 0.1746 | 97.0% | ||
γ | 0.2529 | 0.0029 | 0.0002 | 0.2232 | 0.2825 | 0.0593 | 95.4% | ||
200 | 70% | α | 0.4209 | 0.2791 | 0.0779 | 0.0849 | 0.7569 | 0.6720 | 100% |
β | 0.6981 | 0.1981 | 0.0405 | 0.5984 | 0.7978 | 0.1994 | 97.2% | ||
γ | 0.2118 | 0.0382 | 0.0016 | 0.1823 | 0.2412 | 0.0589 | 97.3% | ||
80% | α | 0.4215 | 0.2785 | 0.0776 | 0.3150 | 0.5280 | 0.2131 | 100% | |
β | 0.6331 | 0.1331 | 0.0191 | 0.5398 | 0.7265 | 0.1867 | 98.2% | ||
γ | 0.2256 | 0.0244 | 0.0007 | 0.1973 | 0.2540 | 0.0567 | 98.0% | ||
100% | α | 0.4234 | 0.2766 | 0.0765 | 0.3366 | 0.5103 | 0.1737 | 100% | |
β | 0.5136 | 0.0136 | 0.0016 | 0.4381 | 0.5891 | 0.1510 | 98.8% | ||
γ | 0.2523 | 0.0023 | 0.0002 | 0.2267 | 0.2779 | 0.0512 | 100% | ||
n | r | Set3 (α = 0.7, β = 0.7, γ = 0.5) | |||||||
MLE | Bias | MSE | LB | UB | AL | CP | |||
50 | 70% | α | 0.4178 | 0.2822 | 0.0797 | 0.1937 | 0.6419 | 0.4482 | 96.2% |
β | 0.8994 | 0.1994 | 0.0477 | 0.6064 | 1.1923 | 0.5859 | 95.9% | ||
γ | 0.6151 | 0.1151 | 0.0239 | 0.3763 | 0.8540 | 0.4776 | 95.0% | ||
80% | α | 0.4193 | 0.2807 | 0.0788 | 0.2255 | 0.6131 | 0.3875 | 95.9% | |
β | 0.8238 | 0.1238 | 0.0218 | 0.5471 | 1.1006 | 0.5535 | 95.9% | ||
γ | 0.5695 | 0.0695 | 0.0182 | 0.3447 | 0.7943 | 0.4496 | 96.7% | ||
100% | α | 0.4211 | 0.2789 | 0.0778 | 0.2480 | 0.5942 | 0.3461 | 96.8% | |
β | 0.7612 | 0.0612 | 0.0104 | 0.5012 | 1.0213 | 0.5201 | 97.0% | ||
γ | 0.5425 | 0.0425 | 0.0163 | 0.3395 | 0.7456 | 0.4061 | 95.0% | ||
100 | 70% | α | 0.4174 | 0.2826 | 0.0798 | 0.2439 | 0.5910 | 0.3470 | 95.0% |
β | 0.8787 | 0.1787 | 0.0353 | 0.6426 | 1.1148 | 0.4722 | 96.3% | ||
γ | 0.5696 | 0.0696 | 0.0201 | 0.3697 | 0.7696 | 0.3998 | 96.0% | ||
80% | α | 0.4191 | 0.2809 | 0.0789 | 0.2690 | 0.5691 | 0.3001 | 95.5% | |
β | 0.7802 | 0.0802 | 0.0134 | 0.5600 | 1.0004 | 0.4404 | 95.7% | ||
γ | 0.5479 | 0.0479 | 0.0091 | 0.3814 | 0.7144 | 0.3330 | 96.0% | ||
100% | α | 0.4206 | 0.2794 | 0.0781 | 0.2866 | 0.5546 | 0.2680 | 95.6% | |
β | 0.7146 | 0.0146 | 0.0068 | 0.5069 | 0.9223 | 0.4154 | 95.7% | ||
γ | 0.5597 | 0.0597 | 0.0074 | 0.4063 | 0.7131 | 0.3067 | 96.0% | ||
150 | 70% | α | 0.4176 | 0.2824 | 0.0798 | 0.2949 | 0.5403 | 0.2454 | 95.8% |
β | 0.8697 | 0.1697 | 0.0305 | 0.7056 | 1.0338 | 0.3282 | 96.2% | ||
γ | 0.5924 | 0.0924 | 0.0174 | 0.4449 | 0.7399 | 0.2950 | 97.1% | ||
80% | α | 0.4193 | 0.2807 | 0.0788 | 0.3131 | 0.5254 | 0.2122 | 96.2% | |
β | 0.8023 | 0.1023 | 0.0124 | 0.6492 | 0.9555 | 0.3063 | 96.1% | ||
γ | 0.5524 | 0.0524 | 0.0058 | 0.4332 | 0.6716 | 0.2384 | 97.0% | ||
100% | α | 0.4209 | 0.2791 | 0.0779 | 0.3261 | 0.5156 | 0.1895 | 95.8% | |
β | 0.7374 | 0.0374 | 0.0032 | 0.5932 | 0.8817 | 0.2885 | 96.3% | ||
γ | 0.5507 | 0.0507 | 0.0041 | 0.4447 | 0.6568 | 0.2121 | 96.9% | ||
200 | 70% | α | 0.4175 | 0.2825 | 0.0798 | 0.3173 | 0.5177 | 0.2004 | 96.1% |
β | 0.8406 | 0.1406 | 0.0265 | 0.7075 | 0.9736 | 0.2661 | 97.2% | ||
γ | 0.5683 | 0.0683 | 0.0069 | 0.4569 | 0.6798 | 0.2230 | 96.9% | ||
80% | α | 0.4193 | 0.2807 | 0.0788 | 0.3327 | 0.5059 | 0.1733 | 96.3% | |
β | 0.8043 | 0.1043 | 0.0123 | 0.6790 | 0.9295 | 0.2505 | 96.6% | ||
γ | 0.5502 | 0.0502 | 0.0041 | 0.4533 | 0.6471 | 0.1938 | 97.0% | ||
100% | α | 0.4209 | 0.2791 | 0.0779 | 0.3435 | 0.4983 | 0.1548 | 96.1% | |
β | 0.7411 | 0.0411 | 0.0030 | 0.6229 | 0.8592 | 0.2362 | 97.0% | ||
γ | 0.5463 | 0.0463 | 0.0041 | 0.4589 | 0.6338 | 0.1749 | 96.2% | ||
n | r | Set4 (α = 0.6, β = 0.3, γ = 0.5) | |||||||
MLE | Bias | MSE | LB | UB | AL | CP | |||
50 | 70% | α | 0.4197 | 0.1803 | 0.0325 | 0.2456 | 0.5939 | 0.3482 | 98.1% |
β | 0.5719 | 0.2719 | 0.0744 | 0.3742 | 0.7696 | 0.3954 | 97.0% | ||
γ | 0.2990 | 0.2010 | 0.0406 | 0.1769 | 0.4211 | 0.2442 | 98.0% | ||
80% | α | 0.4221 | 0.1779 | 0.0317 | 0.2716 | 0.5725 | 0.3009 | 98.4% | |
β | 0.4934 | 0.1934 | 0.0384 | 0.3126 | 0.6742 | 0.3616 | 97.4% | ||
γ | 0.3593 | 0.1407 | 0.0200 | 0.2383 | 0.4803 | 0.2421 | 98.2% | ||
100% | α | 0.4246 | 0.1754 | 0.0308 | 0.2903 | 0.5589 | 0.2686 | 97.9% | |
β | 0.4198 | 0.1198 | 0.0150 | 0.2576 | 0.5820 | 0.3244 | 97.4% | ||
γ | 0.4172 | 0.0828 | 0.0078 | 0.2968 | 0.5376 | 0.2408 | 98.4% | ||
100 | 70% | α | 0.4198 | 0.1802 | 0.0325 | 0.2966 | 0.5429 | 0.2463 | 97.2% |
β | 0.5674 | 0.2674 | 0.0717 | 0.4278 | 0.7069 | 0.2791 | 97.7% | ||
γ | 0.3137 | 0.1863 | 0.0351 | 0.2278 | 0.3995 | 0.1717 | 98.0% | ||
80% | α | 0.4222 | 0.1778 | 0.0316 | 0.3157 | 0.5286 | 0.2128 | 97.9% | |
β | 0.4857 | 0.1857 | 0.0350 | 0.3584 | 0.6130 | 0.2546 | 97.9% | ||
γ | 0.3669 | 0.1331 | 0.0179 | 0.2818 | 0.4519 | 0.1701 | 98.7% | ||
100% | α | 0.4248 | 0.1752 | 0.0307 | 0.3242 | 0.5253 | 0.2011 | 98.1% | |
β | 0.4082 | 0.1082 | 0.0125 | 0.2944 | 0.5221 | 0.2277 | 97.8% | ||
γ | 0.4171 | 0.0829 | 0.0074 | 0.3349 | 0.4993 | 0.1644 | 98.3% | ||
150 | 70% | α | 0.4198 | 0.1802 | 0.0325 | 0.3248 | 0.5148 | 0.1899 | 97.2% |
β | 0.5653 | 0.2653 | 0.0706 | 0.4518 | 0.6788 | 0.2270 | 98.3% | ||
γ | 0.3140 | 0.1860 | 0.0350 | 0.2421 | 0.3860 | 0.1439 | 99.3% | ||
80% | α | 0.4222 | 0.1778 | 0.0316 | 0.3353 | 0.5091 | 0.1738 | 97.7% | |
β | 0.4832 | 0.1832 | 0.0338 | 0.3797 | 0.5866 | 0.2069 | 98.2% | ||
γ | 0.3677 | 0.1323 | 0.0178 | 0.2996 | 0.4358 | 0.1363 | 99.0% | ||
100% | α | 0.4248 | 0.1752 | 0.0307 | 0.3377 | 0.5119 | 0.1742 | 97.3% | |
β | 0.4018 | 0.1018 | 0.0107 | 0.3032 | 0.5004 | 0.1972 | 97.9% | ||
γ | 0.4248 | 0.0752 | 0.0061 | 0.3608 | 0.4888 | 0.1280 | 99.7% | ||
200 | 70% | α | 0.4198 | 0.1802 | 0.0325 | 0.3423 | 0.4973 | 0.1551 | 99.0% |
β | 0.5650 | 0.2650 | 0.0705 | 0.4738 | 0.6561 | 0.1823 | 99.1% | ||
γ | 0.3256 | 0.1744 | 0.0309 | 0.2637 | 0.3875 | 0.1239 | 98.7% | ||
80% | α | 0.4222 | 0.1778 | 0.0316 | 0.3470 | 0.4975 | 0.1505 | 99.6% | |
β | 0.4781 | 0.1781 | 0.0321 | 0.3882 | 0.5679 | 0.1798 | 99.3% | ||
γ | 0.3733 | 0.1267 | 0.0173 | 0.3129 | 0.4336 | 0.1206 | 99.5% | ||
100% | α | 0.4250 | 0.1750 | 0.0306 | 0.3578 | 0.4921 | 0.1343 | 98.7% | |
β | 0.3904 | 0.0904 | 0.0088 | 0.3106 | 0.4703 | 0.1596 | 99.6% | ||
γ | 0.4262 | 0.0738 | 0.0060 | 0.3681 | 0.4843 | 0.1162 | 100% |
A. For almost all the true values, the MSE of all the estimates decreases as the sample sizes and the censoring level r increase, demonstrating that the various estimates are consistent (see Table 6 and Figure 3).
B. For all true parameter values, the ALs of all the estimates decrease as the sample sizes and the censoring level r increase (see Table 6 and Figure 4).
C. For all true parameter values, the CP of all the estimates increases as the sample sizes and the censoring level r increase (see Table 6).
D. The MSE of the estimate of α at the true value of Set1 yields the lowest values in comparison to the other actual parameter values for all sample sizes (see Table 6 and Figure 5).
E. At all actual values, the MSE of the estimate of β produces the largest results for all sample sizes (see Table 6 and Figure 6). Also, it is evident that except for n=50 and 200, the MSE of β estimates obtains the smallest values for the actual value of Set1 compared to the other actual sets at the censoring level 70%. At the censoring level 80%, the MSE of β estimates gets the smallest values at all sets of parameters except at n=50.
F. The MSE of the estimate of γ at the true value of Set2 gets the smallest values in comparison to the other actual parameter values for all sample sizes (see Table 6 and Figure 7).
G. The MSEs, biases, and ALs of γ are smaller than the other estimates of α and β in almost all of the cases.
H. As n rises, the CI's lengths get shorter.
I. As n increases, parameter estimates grow increasingly accurate, suggesting that they are asymptotically unbiased.
J. For the parameter values examined, the CI's overall performance is fairly strong.
Here, we provide applications to four real data sets to illustrate the importance and potentiality of the LBTLoW distribution. The goodness-of-fit statistics for these distributions and other competitive distributions are compared, and the MLEs of their parameters are provided.
The first real data set [38] on the relief times of twenty patients receiving an analgesic is 1.1, 1.4, 3, 1.7, 2.3, 1.4, 1.3, 1.7, 2.2, 1.7, 2.7, 4.1, 1.8, 1.5, 1.9, 1.8, 1.6, 1.2, 1.6, 2.
The second dataset illustrates the proportion of global reserves of natural gas in various countries as of the year 2020. In contrast to other nations, Russia possesses the largest natural gas reserves globally and maintains its position as the leading exporter of natural gas. Iran, on the other hand, ranks second in terms of natural gas reserves worldwide. Qatar, although holding slightly over 13% of the total global natural gas reserves, also plays a significant role in the natural gas market. Lastly, Saudi Arabia possesses the fifth-largest natural gas reserves globally. The electronic address from which it was taken is as follows: https://worldpopulationreview.com/. The data set is reported in Table 7.
Rank | Country | % Global Reserves | Rank | Country | % Global Reserves |
1 | Russia | 19.9 | 23 | Ukraine | 0.6 |
2 | Iran | 17.1 | 24 | Malaysia | 0.5 |
3 | Qatar | 13.1 | 25 | Uzbekistan | 0.4 |
4 | Turkmenistan | 7.2 | 26 | Oman | 0.4 |
5 | United States | 6.7 | 27 | Vietnam | 0.3 |
6 | China | 4.5 | 28 | Israel | 0.3 |
7 | Venezuela | 3.3 | 29 | Argentina | 0.2 |
8 | Saudi Arabia | 3.2 | 30 | Pakistan | 0.2 |
9 | United Arab Emirates | 3.2 | 31 | Trinidad | 0.2 |
10 | Nigeria | 2.9 | 32 | Brazil | 0.2 |
11 | Iraq | 1.9 | 33 | Myanmar | 0.2 |
12 | Canada | 1.3 | 34 | United Kingdom | 0.1 |
13 | Australia | 1.3 | 35 | Thailand | 0.1 |
14 | Azerbaijan | 1.3 | 36 | Mexico | 0.1 |
15 | Algeria | 1.2 | 37 | Bangladesh | 0.1 |
16 | Kazakhstan | 1.2 | 38 | Netherlands | 0.1 |
17 | Egypt | 1.1 | 39 | Bolivia | 0.1 |
18 | Kuwait | 0.9 | 40 | Brunei | 0.1 |
19 | Norway | 0.8 | 41 | Peru | 0.1 |
20 | Libya | 0.8 | 42 | Syria | 0.1 |
21 | Indonesia | 0.7 | 43 | Yemen | 0.1 |
22 | India | 0.7 | 44 | Papua New Guinea | 0.1 |
The third dataset pertains to the Top 20 Countries with the Largest Oil Reserves, measured in thousand million barrels. Crude oil serves as the predominant fuel source globally and is the primary source of energy on a wide scale. In the year 2020, global oil consumption reached around 88.6 million barrels per day, or 30.1% of the overall primary energy consumption. Venezuela possesses the largest oil reserves globally, over 300 billion barrels in total. Saudi Arabia holds the world's second-largest oil reserves, with 297.5 billion barrels. The United States is the world's leading producer of oil as well as the world's greatest user of oil, necessitating additional imports from dozens of other oil-producing countries. Despite having the world's highest oil production, the United States is only 9th in the world in terms of available oil reserves. It was obtained from the following electronic address: https://worldpopulationreview.com/. The data set is reported in Table 8.
Rank | Country | reserves2020 | Rank | Country | reserves2020 |
1 | Venezuela | 303.8 | 11 | Nigeria | 36.9 |
2 | Saudi Arabia | 297.5 | 12 | Kazakhstan | 30 |
3 | Canada | 168.1 | 13 | China | 26 |
4 | Iran | 157.8 | 14 | Qatar | 25.2 |
5 | Iraq | 145 | 15 | Algeria | 12.2 |
6 | Russia | 107.8 | 16 | Brazil | 11.9 |
7 | Kuwait | 101.5 | 17 | Norway | 7.9 |
8 | United Arab Emirates | 97.8 | 18 | Angola | 7.8 |
9 | United States | 68.8 | 19 | Azerbaijan | 7 |
10 | Libya | 48.4 | 20 | Mexico | 6.1 |
The fourth data set represents the Top 100 central banks that owned the largest gold Reserves (in thousand tons). Because of its safety, liquidity, and return qualities-the three major investment objectives for central banks-gold is an essential component of central bank reserves. As such, they are significant gold holders, accounting for around one-fifth of all gold extracted throughout history. They present gold reserve data derived using IMF IFS figures to help comprehend this sector of the gold market, which records central banks' (and other official institutions, when appropriate) reported purchases and sales of gold as a percentage of their international reserves. It was obtained from the following electronic address: https://www.gold.org/. The data set is reported in Table 9.
Rank | Country | Reserves of Gold | Rank | Country | Reserves of Gold | Rank | Country | Reserves of Gold |
1 | USA | 8.1335 | 35 | LBY | 0.1166 | 68 | CYP | 0.0139 |
2 | DEU | 3.3585 | 36 | GRC | 0.1141 | 69 | CUW | 0.0131 |
3 | IMF | 2.814 | 37 | ROK | 0.1045 | 70 | MUS | 0.0124 |
4 | ITA | 2.4518 | 38 | ROU | 0.1036 | 71 | IRL | 0.012 |
5 | FRA | 2.4365 | 39 | BIS | 0.102 | 72 | CZE | 0.0109 |
6 | RUS | 2.2985 | 40 | IRQ | 0.0964 | 73 | KGZ | 0.0102 |
7 | CHN | 1.9483 | 41 | HUN | 0.0945 | 74 | GHA | 0.0087 |
8 | CHE | 1.04 | 42 | AUS | 0.0798 | 75 | PRY | 0.0082 |
9 | JPN | 0.846 | 43 | KWT | 0.079 | 76 | NPL | 0.008 |
10 | IND | 0.7604 | 44 | IDN | 0.0786 | 77 | MNG | 0.0076 |
11 | NLD | 0.6125 | 45 | DNK | 0.0666 | 78 | MMR | 0.0073 |
12 | ECB | 0.5048 | 46 | PAK | 0.0647 | 79 | GTM | 0.0069 |
13 | TUR | 0.4311 | 47 | ARG | 0.0617 | 80 | MKD | 0.0069 |
14 | TAI | 0.4236 | 48 | ARE | 0.0553 | 81 | TUN | 0.0068 |
15 | PRT | 0.3826 | 49 | BLR | 0.0535 | 82 | LVA | 0.0067 |
16 | KAZ | 0.3681 | 50 | QAT | 0.0513 | 83 | LTU | 0.0058 |
17 | UZB | 0.3375 | 51 | KHM | 0.0504 | 84 | COL | 0.0047 |
18 | SAU | 0.3231 | 52 | FIN | 0.049 | 85 | BHR | 0.0047 |
19 | GBR | 0.3103 | 53 | JOR | 0.0435 | 86 | BRN | 0.0046 |
20 | LBN | 0.2868 | 54 | BOL | 0.0425 | 87 | GIN | 0.0042 |
21 | ESP | 0.2816 | 55 | BGR | 0.0408 | 88 | MOZ | 0.0039 |
22 | AUT | 0.28 | 56 | MYS | 0.0389 | 89 | SVN | 0.0032 |
23 | THA | 0.2442 | 57 | SRB | 0.0378 | 90 | ABW | 0.0031 |
24 | POL | 0.2287 | 58 | WAEMU | 0.0365 | 91 | BIH | 0.003 |
25 | BEL | 0.2274 | 59 | PER | 0.0347 | 92 | ALB | 0.0028 |
26 | DZA | 0.1736 | 60 | SVK | 0.0317 | 93 | LUX | 0.0022 |
27 | VEN | 0.1612 | 61 | UKR | 0.0271 | 94 | HKG | 0.0021 |
28 | PHL | 0.1563 | 62 | SYR | 0.0258 | 95 | ISL | 0.002 |
29 | SGP | 0.1537 | 63 | MAR | 0.0221 | 96 | TTO | 0.0019 |
30 | BRA | 0.1297 | 64 | ECU | 0.0219 | 97 | HTI | 0.0018 |
31 | SWE | 0.1257 | 65 | AFG | 0.0219 | 98 | YEM | 0.0016 |
32 | ZAF | 0.1254 | 66 | NGA | 0.0215 | 99 | SUR | 0.0015 |
33 | EGY | 0.125 | 67 | BGD | 0.014 | 100 | SLV | 0.0014 |
34 | MEX | 0.1199 |
The descriptive analysis of all the data sets is reported in Table 10.
n | Mean | Median | Skewness | Kurtosis | Range | Min | Max | Sum | |
Data1 | 20 | 1.900 | 1.700 | 1.860 | 4.185 | 3.000 | 1.100 | 4.100 | 38.000 |
Data2 | 44 | 2.248 | 0.650 | 2.990 | 8.864 | 19.800 | 0.100 | 19.900 | 98.900 |
Data3 | 20 | 83.375 | 42.650 | 1.430 | 1.420 | 297.700 | 6.100 | 303.800 | 1667.500 |
Data4 | 100 | 0.347 | 0.050 | 5.590 | 38.257 | 8.130 | 0.001 | 8.133 | 34.676 |
These real data sets are utilized to assess the goodness of fit of the LBTLoW distribution. The suggested model is compared with exponentiated transmuted generalized Rayleigh (ETGR) [39], beta Weibull (BW) [40], transmuted Lindley (T-Li) [41], McDonald log-logistic (McLL) [42], new modified Weibull (NMW) [43], weighted exponentiated inverted Weibull (WEIW) [44], transmuted complementary Weibull geometric (TCWG) [45], transmuted modified Weibull (TMW) [46], exponentiated Kumaraswamy Weibull (EKW) [47] and Weibull (W) models.
The maximum likelihood estimators (MLEs) and standard errors (SEs) of the model parameters are computed. In order to assess the distribution models, various criteria are taken into account, including the Akaike information criterion (AIC), correct AIC (CAIC), Bayesian IC (BIC), Hannan-Quinn IC (HQIC), Kolmogorov-Smirnov (KS) test, and p-value (PV) test. In contrast, the broader dissemination is associated with reduced values of AIC, CAIC, BIC, HQIC, KS, and the highest magnitude of PV. The maximum likelihood estimators (MLEs) of the competitive models, along with their standard errors (SEs) and values of AIC, CAIC, BIC, HQIC, PV, and KS for the suggested data sets, are displayed in Tables 11-18. It has been observed that the LBTLoW distribution, characterized by three parameters, exhibits superior goodness of fit compared to alternative models. This distribution exhibits the lowest values of AIC, CAIC, BIC, HQIC, and KS, and the highest value of PV among the distributions under consideration in this analysis. Furthermore, Figures 8-15 exhibit the graphical representations of the estimated pdf, cdf, ccdf, and probability-probability (PP) plots for the competitive model applied to the given data sets.
Distributions | MLE and SE | ||||
α | β | γ | λ | θ | |
LBTLoW | 8.648 | 3.074 | 0.042 | ||
(3.545) | (0.474) | (0.025) | |||
ETGR | 0.103 | 0.692 | 23.539 | -0.342 | |
(0.436) | (0.086) | (105.137) | (1.971) | ||
BW | 0.831 | 0.613 | 29.947 | 11.632 | |
(0.954) | (0.340) | (40.414) | (21.900) | ||
T-Li | 0.665 | 0.359 | |||
(0.332) | (0.048) | ||||
McLL | 0.881 | 2.070 | 1.926 | 19.225 | 32.033 |
(0.109) | (3.693) | (5.165) | (22.341) | (43.081) | |
NMW | 0.121 | 2.784 | 2.787 | 0.003 | 0.008 |
(0.056) | (20.370) | (0.428) | (0.025) | (0.002) | |
W | 0.122 | 2.787 | |||
(0.056) | (0.427) |
Distributions | AIC | CAIC | BIC | HQIC | KS | PV |
LBTLoW | 40.140 | 41.640 | 38.040 | 40.720 | 0.146 | 0.790 |
ETGR | 44.860 | 47.520 | 42.060 | 45.630 | 0.190 | 0.465 |
BW | 42.400 | 45.060 | 39.600 | 43.170 | 0.160 | 0.683 |
T-Li | 65.730 | 66.440 | 64.330 | 66.120 | 0.380 | 0.006 |
McLL | 43.850 | 48.140 | 40.360 | 44.830 | 0.147 | 0.734 |
NMW | 51.170 | 55.460 | 47.680 | 52.150 | 0.190 | 0.501 |
W | 45.170 | 45.880 | 43.780 | 45.560 | 0.180 | 0.509 |
Distributions | MLE and SE | ||||
α | β | γ | λ | θ | |
LBTLoW | 6.268 | 0.623 | 0.484 | ||
(2.631) | (0.066) | (0.210) | |||
ETGR | 0.055 | 0.071 | 8.773 | 0.947 | |
(0.027) | (0.029) | (7.043) | (0.081) | ||
TCWG | 34.076 | 0.802 | 0.005 | 1.12 | |
(81.023) | (0.021) | (0.013) | (0.285) | ||
EKW | 0.221 | 400.298 | 5.215 | 1 | 3.823 |
(0.038) | (718.99) | (0.649) | (0.004) | (3.036) | |
TMW | 0.851 | 1.159 | -0.554 | 0.519 | |
(0.163) | (1.026) | (0.985) | (0.379) | ||
BW | 2.861 | 0.075 | 78.550 | 42.576 | |
(69.095) | (0.090) | (167.320) | (187.300) | ||
T-Li | 0.604 | 0.671 | |||
(0.155) | (0.074) | ||||
McLL | 0.181 | 1.565 | 1.286 | 21.234 | 28.124 |
(0.193) | (9.254) | (5.432) | (34.701) | (45.757) | |
NMW | 6.8 x 10−8 | 0.680 | 0.223 | 0.015 | 0.806 |
(0.623) | (0.110) | (617.48) | (0.015) | (0.418) | |
W | 0.799 | 0.621 | |||
(0.136) | (0.068) |
Distributions | AIC | CAIC | BIC | HQIC | KS | PV |
LBTLoW | 132.210 | 132.810 | 131.140 | 134.200 | 0.130 | 0.425 |
ETGR | 143.470 | 144.490 | 142.040 | 146.110 | 0.180 | 0.118 |
TCWG | 137.690 | 138.710 | 136.260 | 140.330 | 0.150 | 0.251 |
EKW | 133.890 | 135.470 | 132.110 | 140.330 | 0.140 | 0.355 |
TMW | 140.900 | 142.480 | 139.120 | 144.210 | 0.150 | 0.276 |
BW | 133.180 | 134.200 | 131.750 | 135.820 | 0.130 | 0.408 |
T-Li | 174.360 | 174.660 | 173.650 | 175.690 | 0.200 | 0.057 |
McLL | 134.830 | 136.410 | 133.040 | 138.130 | 0.130 | 0.419 |
NMW | 143.780 | 145.360 | 142.000 | 147.090 | 0.160 | 0.243 |
W | 138.650 | 138.940 | 137.940 | 139.970 | 0.170 | 0.139 |
Distributions | MLE and SE | ||||
α | β | γ | λ | θ | |
LBTLoW | 2.515 | 0.756 | 0.040 | ||
(3.877) | (0.166) | (0.048) | |||
WEIW | 0.909 | 0.871 | 7.225 | ||
(106700) | (0.152) | (384700) | |||
TMW | 0.998 | 0.459 | -0.443 | 0.202 | |
(0.081) | (18.537) | (18.537) | (0.769) | ||
T-Li | 0.021 | 0.384 | |||
(0.345) | (0.004) | ||||
McLL | 0.208 | 93.978 | 1.279 | 24.759 | 32.815 |
(0.499) | (1721) | (19.272) | (142.806) | (161.611) | |
NMW | 10.7 x 10−8 | 0.930 | 0.859 | 7.46 x 10−8 | 0.017 |
(0.001) | (0.250) | (1.216) | (0.002) | (0.017) | |
EKW | 0.167 | 261.64 | 45.725 | 1.201 | 2.138 |
(0.079) | (1709) | (219.725) | (0.741) | (7.209) |
Distributions | AIC | CAIC | BIC | HQIC | KS | PV |
LBTLoW | 221.690 | 223.190 | 219.600 | 222.280 | 0.135 | 0.857 |
WEIW | 223.400 | 224.900 | 221.300 | 223.980 | 0.157 | 0.708 |
TMW | 226.410 | 230.690 | 222.910 | 227.380 | 0.153 | 0.734 |
T-Li | 230.480 | 231.180 | 229.080 | 230.870 | 0.265 | 0.120 |
McLL | 225.990 | 230.280 | 222.500 | 222.500 | 0.146 | 0.789 |
NMW | 226.570 | 230.860 | 223.080 | 227.540 | 0.140 | 0.826 |
EKW | 226.290 | 230.570 | 222.790 | 229.300 | 0.148 | 0.776 |
Distributions | MLE and SE | ||||
α | β | γ | λ | θ | |
LBTLoW | 6.498 | 0.482 | 1.490 | ||
(2.301) | (0.034) | (0.573) | |||
EKW | 0.221 | 1096 | 4.424 | 1 | 1.717 |
(0.030) | (1376) | (1.817) | (0.001) | (0.901) | |
TMW | 0.596 | 2.612 | 0.588 | -0.523 | |
(0.057) | (0.689) | (0.256) | (0.346) | ||
BW | 134.832 | 0.073 | 49.149 | 22.930 | |
(956.622) | (0.060) | (74.497) | (46.500) | ||
WEIW | 27.512 | 0.549 | 0.094 | ||
(3272000) | (0.042) | (856.967) | |||
W | 2.648 | 0.489 | |||
(0.281) | (0.035) |
Distributions | AIC | CAIC | BIC | HQIC | KS | PV |
LBTLoW | –170.510 | –170.260 | –170.510 | –167.350 | 0.070 | 0.704 |
ETGR | –167.710 | –167.070 | –167.710 | –157.710 | 0.078 | 0.584 |
BW | –158.180 | –157.540 | –158.180 | –152.910 | 0.077 | 0.593 |
T-Li | –170.220 | –169.800 | –170.220 | –166.010 | 0.071 | 0.703 |
McLL | –170.200 | –169.950 | –170.200 | –167.040 | 0.091 | 0.383 |
W | –157.390 | –157.260 | –157.390 | –155.280 | 0.100 | 0.269 |
From the previous figures, we conclude that the LBTLoW model clearly gives the best overall fit and so may be picked as the most appropriate model for explaining data.
The LBTLo-G family of distributions is explored in this article. The LBTLo-G family of probability distributions has a number of desirable characteristics, including being very flexible and simple, containing a number of new distributions, the ability for the generated distributions' pdfs to be unimodal, decreasing, bathtub-shaped, right-skewed, and symmetric, and the ability for their hrf shapes to be increasing, decreasing, U-shaped, upside-down-shaped, or J-shaped. These include discussion of the characteristics of the LBTLo-G family, including expansion for the density function, moments, incomplete moments, and certain entropy metrics. Estimating the model parameters is done using the ML technique. A simulation study demonstrated that the estimates of the model parameters are not far from their true values. Also, the biases and mean squared errors of estimates based on censored samples are larger than those based on complete samples. As the censoring levels and sample sizes increase, the coverage probability of estimates increases in approximately most cases.
As one distribution of the LBTLo-G family, the real datasets for global reserves of oil, gold, and natural gas were chosen to fit the LBTLoW distribution. The first data set proposed was the lifetime data relating to relief times (in minutes) of patients receiving an analgesic. The second data set provides the percent of global reserves of natural gas for 44 countries. We have considered the third real data analysis of the countries with the largest oil reserves in 20 countries. We consider another real-data analysis of the central bank owning the largest gold reserves in 100 countries. This gold reserve data, compiled using international monetary funds and international financial statistics, tracks central banks' reported purchases and sales of gold as a percentage of their international reserves. The LBTLoW model typically provides superior fits in comparison to certain other alternative models, as shown by real-world data applications.
The authors declare that they have not used artificial intelligence tools in the creation of this article.
Researchers Supporting Project number (RSPD2023R548), King Saud University, Riyadh, Saudi Arabia.
The authors declare that there are no conflicts of interest.
[1] | C. R. Rao, On Discrete Distributions Arising out of Methods of Ascertainment, in Classical and Contagious Discrete Distribution, (Eds. G. P. Patil), Pergamon Press, Calcutta, (1965), 320–332. |
[2] |
R. A. Fisher, The effects of methods of ascertainment upon the estimation of frequencies, Ann. Eugen., 6 (1934), 13–25. https://doi.org/10.1111/j.1469-1809.1934.tb02105.x doi: 10.1111/j.1469-1809.1934.tb02105.x
![]() |
[3] |
G. P. Patil, C. R. Rao, Weighted distributions and size-biased sampling with applications to wildlife populations and human families, Biometrics, 34 (1978), 179–189. https://doi.org/10.2307/2530008 doi: 10.2307/2530008
![]() |
[4] | R. C. Gupta, J. P. Keating, Relations for reliability measures under length biased sampling, Scand. J. Stat., (1986), 49–56. |
[5] |
A. K. Nanda, K. Jain, Some weighted distribution results on univariate and bivariate cases, J. Stat. Plan. Inference, 77 (1999), 169–180. https://doi.org/10.1016/S0378-3758(98)00190-6 doi: 10.1016/S0378-3758(98)00190-6
![]() |
[6] | S. Sen, N. Chandra, S. S. Maiti, The weighted X-Gamma distribution: Properties and application, J. Reliab. Stat. Stud., 10 (2017), 43–58. |
[7] |
S. Abbas, G. Ozal, S. H. Shahbaz, M. Q. Shahbaz, A new generalized weighted weibull distribution, Pakistan J. Stat. Oper. Res., 15 (2019), 161–178. https://doi.org/10.18187/pjsor.v15i1.2782 doi: 10.18187/pjsor.v15i1.2782
![]() |
[8] |
A. M. T. A. El-Bar, I. E. Ragab, On weighted exponential-gompertz distribution: Properties and application, J. Taibah Univ. Sci., 13 (2019), 616–627. https://doi.org/10.1080/16583655.2019.1600277 doi: 10.1080/16583655.2019.1600277
![]() |
[9] |
D. Aydin, The new weighted inverse rayleigh distribution and its application, Math. Inf., 34 (2019), 511–523. https://doi.org/10.22190/FUMI1903511A doi: 10.22190/FUMI1903511A
![]() |
[10] |
S. Mudiasir, S. P. Ahmad, Weighted version of generalized inverse weibull distribution, J. Mod. Appl. Stat. Methods, 17 (2019), 18. https://doi.org/10.22237/jmasm/1555506264 doi: 10.22237/jmasm/1555506264
![]() |
[11] |
A. Mallick, I. Ghosh, S. Dey, D. Kumar, Bounded weighted exponential distribution with applications, Am. J. Math. Manage. Sci., 40 (2020), 68–87. https://doi.org/10.1080/01966324.2020.1834893 doi: 10.1080/01966324.2020.1834893
![]() |
[12] |
H. S. Bakouch, C. Chesneau, M. G. Enany, A new weighted exponential distribution as an alternative to the weibull distribution and its fit to reliability data, Int. J. Data Sci., 6 (2021), 223–240. https://doi.org/10.1504/IJDS.2021.121096 doi: 10.1504/IJDS.2021.121096
![]() |
[13] | A. S. Hassan, E. M. Almetwally, M. A. Khaleel, H. F. Nagy, Weightedpower lomax distribution and its length biased version: Properties and estimation based on censored samples, Pak. J. Stat. Oper. Res., 17 (2021), 343–356. |
[14] | S. Abbas, S. Zaniab, O. Mehmood, G. Ozal, M. Q. Shahbaz, A new generalized weighted exponential distribution: Properties and applications, Thailand Stat., 20 (2022), 271–283. |
[15] |
A. A. Alahmadi, M. Alqawba, W. Almutiry, A. W. Shawki, S. Alrajhi, S. Al-Marzouki, et al., A new version of weighted Weibull distribution: Modelling to COVID-19 data, Discrete Dyn. Nat. Soc., 2022 (2022), 1–12. https://doi.org/10.1155/2022/3994361 doi: 10.1155/2022/3994361
![]() |
[16] |
C. Chesneau, V. Kumar, M. Khetan, M. Arshad, On amodified weighted exponential distribution with applications, Math. Comput. Appl., 27 (2022), 17. https://doi.org/10.3390/mca27010017 doi: 10.3390/mca27010017
![]() |
[17] | M. Mohiuddin, S. A. Dar, A. A. Khan, M. Ahajeeth, H. A. Bayatti, On weighted Nwikpe distribution: Properties and applications, Inf. Sci. Lett., 11 (2022), 85–96. |
[18] |
A. A. H. Ahmadini, M. Elgarhy, A. W. Shawki, H. Baaqeel, O. Bazighifan, Statistical analysis of the people fully vaccinated against COVID-19 in two different regions, Appl. Bionics Biomech., 2022 (2022). https://doi.org/10.1155/2022/7104960 doi: 10.1155/2022/7104960
![]() |
[19] |
R. Bantan, A. S. Hassan, E. Almetwally, M. Elgarhy, F. Jamal, C. Chesneau, et al., Bayesian analysis in partially accelerated life tests for weighted Lomax distribution, Comput. Mater. Continua, 68 (2021), 2859–2875. https://doi.org/10.32604/cmc.2021.015422 doi: 10.32604/cmc.2021.015422
![]() |
[20] |
A.S. Hassan, M. Elgarhy, Z. Ahmad, Type Ⅱ generalized topp leone family of distributions: Properties and applications, J. Data Sci., 17 (2019), 638–659. https://doi.org/10.6339/JDS.201910_17(4).0001 doi: 10.6339/JDS.201910_17(4).0001
![]() |
[21] |
A. Algarni, A. M. Almarashi, I. Elbatal, A. S. Hassan, E. M. Almetwally, A. M. Daghistani, et al., Type Ⅰ half logistic Burr X-G family: Properties, Bayesian, and non-Bayesian estimation under censored samples and applications to COVID-19 data, Math. Prob. Eng., 2021 (2021), 1–21. https://doi.org/10.1155/2021/5461130 doi: 10.1155/2021/5461130
![]() |
[22] |
A. S. Hassan, A. I. AlOmari, R. R. Hassan, G. Alomani, The odd inverted Topp Leone-H family of distributions: Estimation and applications, J. Radiat. Res. Appl. Sci., 15 (2022), 365–379. https://doi.org/10.1016/j.jrras.2022.08.006 doi: 10.1016/j.jrras.2022.08.006
![]() |
[23] |
N. C. Eugene, C. Lee, F. Famoye, Beta-normal distribution and its applications, Commun. Stat. Theory Methods, 31 (2002), 497–512. https://doi.org/10.1081/STA-120003130 doi: 10.1081/STA-120003130
![]() |
[24] | N. H. Al-Noor, L. K. Hussein, Weighted exponential-G Family of probability distributions, Saudi J. Eng. Technol., 3 (2018), 51–59. |
[25] | Z. Ahmad, G. Hamedani, M. Elgarhy, The weighted exponentiated family of distributions: Properties, applications and characterizations, J. Iran. Stat. Soc., 19 (2020), 209–228. |
[26] |
H. Bakouch, C. Chesneau, M. Enany, A weighted general family of distributions: Theory and practice, Comput. Math. Methods, 3 (2020). https://doi.org/10.1002/cmm4.1135 doi: 10.1002/cmm4.1135
![]() |
[27] | M. Hashempour, Weighted topp-leone g family of distributions: Properties, applications for modelling reliability data and different method of estimation, Hacettepe J. Math. Stat., 51 (2022), 1420–1441. |
[28] | A. S. Hassan, A. W. Shawki, H. Z. Muhammed, Analysis of HIECS research data for north Sinai governorate in Egypt using length biased truncated Lomax distribution, Stat. Optim. Inf. Comput., 11 (2023). |
[29] |
J. A. Greenwood, J. M. Landwehr, N. C. Matalas, J. R. Wallis, Probability-weighted moments: Definition andrelation to parameters of several distributions expressible in inverse form, Water Resour. Res., 15 (1979), 1049–1054. https://doi.org/10.1029/WR015i005p01049 doi: 10.1029/WR015i005p01049
![]() |
[30] | A. Re′nyi, On measures of entropy and information, in Proceedings of the 4th Fourth Berkeley Symposium on Mathematical Statistics and Probability, (1961), 547–561. |
[31] | J. Havrda, F. Charvat, Quantification method of classification processes. concept of structural-entropy, Kybernetika, 3 (1967), 30–35. |
[32] |
S. Arimoto, Information-theoretical considerations on estimation problems, Inf. Control, 19 (1971), 181–194. https://doi.org/10.1016/S0019-9958(71)90065-9 doi: 10.1016/S0019-9958(71)90065-9
![]() |
[33] |
C. Tsallis, The role of constraints within generalized nonextensive statistics, Physica, 261 (1998), 547–561. https://doi.org/10.1016/S0378-4371(98)00437-3 doi: 10.1016/S0378-4371(98)00437-3
![]() |
[34] |
S. Zhou, A. Xu, Y. Tang, Fast Bayesian inference of reparameterized Gamma process with random effects, IEEE Trans. Reliabil., (2023). https://doi.org/10.1109/TR.2023.3263940 doi: 10.1109/TR.2023.3263940
![]() |
[35] | K. A. Tasias, Integrated quality, maintenance and production model for multivariate processes: A Bayesian approach, J. Manuf. Syst., 63 (2022), 35–51. |
[36] |
L. Zhuang, A. Xu, X. L. Wang, A prognostic driven predictive maintenance framework based on Bayesian deep learning, Reliab. Eng. Syst. Safety, 234 (2023), 109–181. https://doi.org/10.1016/j.ress.2023.109181 doi: 10.1016/j.ress.2023.109181
![]() |
[37] |
C. Luo, L. Shen, A. Xu, Modelling and estimation of system reliability under dynamic operating environments and lifetime ordering constraints, Reliab. Eng. Syst. Safety, 218 (2022), 108–136. https://doi.org/10.1016/j.ress.2021.108136 doi: 10.1016/j.ress.2021.108136
![]() |
[38] | A. J. Gross, V. A. Clark, Survival Distributions: Reliability Applications in the Biomedical Sciences, John Wiley, New York, 1975. |
[39] |
A. Z. Afify, G. M. Cordeiro, H. M. Yousof, A. Alzaatreh, Z. M. Nofal, The Kumaraswamy transmuted-G family of distributions: Properties and applications, J. Data Sci., 14 (2016), 245–270. https://doi.org/10.6339/JDS.201604_14(2).0004 doi: 10.6339/JDS.201604_14(2).0004
![]() |
[40] |
C. Lee, F. Famoye, O. Olumolade, Beta-Weibull distribution: Some properties and applications to censored data, J. Modern Appl. Stat. Methods, 6 (2007), 173–186. https://doi.org/10.22237/jmasm/1177992960 doi: 10.22237/jmasm/1177992960
![]() |
[41] |
F. Merovci, Transmuted lindley distribution, Int. J. Open Prob. Comput. Sci. Math., 6 (2013), 63–72. https://doi.org/10.12816/0006170 doi: 10.12816/0006170
![]() |
[42] |
M. H. Tahir, M. Mansoor, M. Zubair, G. Hamedani, McDonald log-logistic distribution with an application to breast cancer data, J. Stat. Theory Appl., 13 (2014), 65–82. https://doi.org/10.2991/jsta.2014.13.1.6 doi: 10.2991/jsta.2014.13.1.6
![]() |
[43] |
S. J. Almalki, J. Yuan, A new modified Weibull distribution, Reliab. Eng. Syst. Safety, 111 (2013), 164–170. https://doi.org/10.1016/j.ress.2012.10.018 doi: 10.1016/j.ress.2012.10.018
![]() |
[44] | A. Saghir, S. Tazeema, I. Ahmad, The weighted exponentiated inverted Weibull distribution: Properties and application, J. Inf. Math. Sci., 9 (2017), 137–151. |
[45] | A. Z. Afify, Z. M. Nofal, N. S. Butt, Transmuted complementary Weibull geometric distribution, Pak. J. Stat. Oper. Res., 10 (2014), 435–454. |
[46] |
M. S. Khan, R. King, I. L. Hudson, Transmuted modified Weibull distribution: Properties and application, Eur. J. Pure Appl. Math., 11 (2018), 362–374. https://doi.org/10.29020/nybg.ejpam.v11i2.3208 doi: 10.29020/nybg.ejpam.v11i2.3208
![]() |
[47] |
F. H. Eissa, The exponentiated Kumaraswamy–Weibull distribution with application to real data, Int. J. Stat. Probab., 6 (2017), 167–182. https://doi.org/10.5539/ijsp.v6n6p167 doi: 10.5539/ijsp.v6n6p167
![]() |
1. | Najwan Alsadat, Amal S Hassan, Mohammed Elgarhy, Vasili B V Nagarjuna, Sid Ahmed Benchiha, Ahmed M Gemeay, A novel asymmetric extension of power XLindley distribution: properties, inference and applications to engineering data, 2024, 99, 0031-8949, 105262, 10.1088/1402-4896/ad77fa | |
2. | Mohammed Elgarhy, Arne Johannssen, Mohamed Kayid, An extended Rayleigh Weibull model with actuarial measures and applications, 2024, 10, 24058440, e32143, 10.1016/j.heliyon.2024.e32143 | |
3. | Naif Alotaibi, A.S. Al-Moisheer, Amal S. Hassan, Ibrahim Elbatal, Salem A. Alyami, Ehab M. Almetwally, Epidemiological modeling of COVID-19 data with Advanced statistical inference based on Type-II progressive censoring, 2024, 10, 24058440, e36774, 10.1016/j.heliyon.2024.e36774 | |
4. | Sara Almakhareez, Loai Alzoubi, Extension to Benrabia distribution with applications and parameter estimation, 2024, 2, 2768-4520, 10.1080/27684520.2024.2393597 | |
5. | Abdullah M. Alomair, Ayesha Babar, Muhammad Ahsan-ul-Haq, Saadia Tariq, An improved extension of Xgamma distribution: Its properties, estimation and application on failure time data, 2025, 24058440, e41976, 10.1016/j.heliyon.2025.e41976 | |
6. | Ahmed W. Shawki, Mohamed Kayid, Oluwafemi Samson Balogun, Tamer S. Helal, Modeling to radiotherapy, environmental and engineering data: Using a new approach to generating family of distributions, 2025, 18, 16878507, 101317, 10.1016/j.jrras.2025.101317 | |
7. | Mohammed Elgarhy, Amal S. Hassan, Najwan Alsadat, Oluwafemi Samson Balogun, Ahmed W. Shawki, Ibrahim E. Ragab, A Heavy Tailed Model Based on Power XLindley Distribution with Actuarial Data Applications, 2025, 142, 1526-1506, 2547, 10.32604/cmes.2025.058362 |
γ | α | μ′1 | μ′2 | μ′3 | μ′4 | σ2 | CS | CK | CV |
0.4 | 0.2 | 2.629 | 8.323 | 30.531 | 126.387 | 1.413 | 0.729 | 3.596 | 0.452 |
0.6 | 0.5 | 1.558 | 2.937 | 6.430 | 15.922 | 0.508 | 0.746 | 3.627 | 0.457 |
0.8 | 0.8 | 1.184 | 1.700 | 2.847 | 5.403 | 0.300 | 0.765 | 3.660 | 0.463 |
1.1 | 1.2 | 0.925 | 1.044 | 1.380 | 2.074 | 0.189 | 0.793 | 3.717 | 0.470 |
1.3 | 1.5 | 0.805 | 0.794 | 0.920 | 1.216 | 0.146 | 0.814 | 3.758 | 0.475 |
1.7 | 1.8 | 0.705 | 0.615 | 0.634 | 0.748 | 0.117 | 0.856 | 3.853 | 0.486 |
1.9 | 2.0 | 0.655 | 0.532 | 0.513 | 0.568 | 0.103 | 0.878 | 3.906 | 0.491 |
2.4 | 2.3 | 0.582 | 0.425 | 0.371 | 0.374 | 0.086 | 0.937 | 4.058 | 0.503 |
2.7 | 2.6 | 0.530 | 0.355 | 0.285 | 0.266 | 0.073 | 0.974 | 4.162 | 0.511 |
3.2 | 3.0 | 0.469 | 0.280 | 0.203 | 0.172 | 0.060 | 1.038 | 4.359 | 0.522 |
γ | α | μ′1 | μ′2 | μ′3 | μ′4 | σ2 | CS | CK | CV |
0.4 | 0.2 | 1.964 | 4.277 | 10.154 | 25.945 | 0.420 | 0.380 | 3.017 | 0.330 |
0.6 | 0.5 | 1.347 | 2.017 | 3.298 | 5.813 | 0.202 | 0.395 | 3.026 | 0.334 |
0.8 | 0.8 | 1.105 | 1.360 | 1.829 | 2.658 | 0.139 | 0.411 | 3.037 | 0.337 |
1.1 | 1.2 | 0.924 | 0.954 | 1.081 | 1.325 | 0.100 | 0.435 | 3.058 | 0.343 |
1.3 | 1.5 | 0.836 | 0.783 | 0.805 | 0.897 | 0.084 | 0.453 | 3.075 | 0.346 |
1.7 | 1.8 | 0.760 | 0.649 | 0.612 | 0.627 | 0.072 | 0.489 | 3.116 | 0.353 |
1.9 | 2.0 | 0.720 | 0.584 | 0.524 | 0.511 | 0.066 | 0.508 | 3.140 | 0.356 |
2.4 | 2.3 | 0.661 | 0.494 | 0.411 | 0.374 | 0.058 | 0.557 | 3.213 | 0.365 |
2.7 | 2.6 | 0.617 | 0.433 | 0.339 | 0.290 | 0.052 | 0.588 | 3.265 | 0.369 |
3.2 | 3.0 | 0.565 | 0.364 | 0.263 | 0.209 | 0.045 | 0.641 | 3.366 | 0.377 |
β | α | μ′1 | μ′2 | μ′3 | μ′4 | σ2 | CS | CK | CV |
1.5 | 0.2 | 0.029 | 0.026 | 0.023 | 0.021 | 0.025 | 5.273 | 29.151 | 5.386 |
0.5 | 0.032 | 0.028 | 0.025 | 0.023 | 0.027 | 5.014 | 26.463 | 5.138 | |
0.8 | 0.035 | 0.031 | 0.028 | 0.025 | 0.030 | 4.772 | 24.069 | 4.906 | |
1.2 | 0.039 | 0.035 | 0.031 | 0.028 | 0.033 | 4.472 | 21.275 | 4.622 | |
1.5 | 0.043 | 0.038 | 0.033 | 0.03 | 0.036 | 4.264 | 19.441 | 4.424 | |
1.8 | 0.046 | 0.041 | 0.036 | 0.033 | 0.038 | 4.069 | 17.801 | 4.241 | |
2 | 0.049 | 0.043 | 0.038 | 0.034 | 0.040 | 3.946 | 16.806 | 4.125 | |
2.3 | 0.053 | 0.046 | 0.041 | 0.037 | 0.043 | 3.771 | 15.445 | 3.961 | |
2.6 | 0.056 | 0.049 | 0.044 | 0.039 | 0.046 | 3.607 | 14.225 | 3.809 | |
3 | 0.062 | 0.054 | 0.048 | 0.043 | 0.050 | 3.405 | 12.794 | 3.621 | |
2.5 | 0.2 | 0.006 | 0.005 | 0.005 | 0.004 | 0.005 | 12.420 | 156.328 | 12.403 |
0.5 | 0.007 | 0.006 | 0.006 | 0.005 | 0.006 | 11.672 | 138.195 | 11.665 | |
0.8 | 0.007 | 0.007 | 0.006 | 0.006 | 0.007 | 10.981 | 122.434 | 10.984 | |
1.2 | 0.009 | 0.008 | 0.007 | 0.007 | 0.008 | 10.140 | 104.548 | 10.156 | |
1.5 | 0.01 | 0.009 | 0.008 | 0.007 | 0.009 | 9.563 | 93.121 | 9.589 | |
1.8 | 0.011 | 0.010 | 0.009 | 0.008 | 0.010 | 9.030 | 83.140 | 9.066 | |
2 | 0.012 | 0.011 | 0.010 | 0.009 | 0.010 | 8.697 | 77.190 | 8.739 | |
2.3 | 0.013 | 0.012 | 0.011 | 0.010 | 0.012 | 8.227 | 69.194 | 8.279 | |
2.6 | 0.014 | 0.013 | 0.012 | 0.011 | 0.013 | 7.792 | 62.179 | 7.853 | |
3 | 0.017 | 0.015 | 0.014 | 0.013 | 0.015 | 7.261 | 54.128 | 7.334 |
ε | β | α | γ | RE | HaCE | ArE | TsE |
1.5 | 0.25 | 0.2 | 0.4 | 3.331 | 3.340 | 2.767 | 1.957 |
0.5 | 0.6 | 3.252 | 3.333 | 2.753 | 1.953 | ||
0.8 | 0.8 | 3.099 | 3.318 | 2.722 | 1.944 | ||
1.2 | 1.1 | 2.875 | 3.290 | 2.670 | 1.927 | ||
1.5 | 1.3 | 2.716 | 3.264 | 2.627 | 1.912 | ||
1.8 | 1.7 | 2.524 | 3.227 | 2.568 | 1.891 | ||
2.0 | 1.9 | 2.415 | 3.203 | 2.53 | 1.876 | ||
2.3 | 2.4 | 2.229 | 3.152 | 2.458 | 1.846 | ||
2.6 | 2.7 | 2.084 | 3.104 | 2.394 | 1.818 | ||
3.0 | 3.2 | 1.889 | 3.026 | 2.296 | 1.773 | ||
0.5 | 0.2 | 0.4 | 1.930 | 3.044 | 2.318 | 1.783 | |
0.5 | 0.6 | 1.924 | 3.042 | 2.315 | 1.782 | ||
0.8 | 0.8 | 1.824 | 2.996 | 2.260 | 1.755 | ||
1.2 | 1.1 | 1.660 | 2.909 | 2.161 | 1.704 | ||
1.5 | 1.3 | 1.541 | 2.835 | 2.081 | 1.661 | ||
1.8 | 1.7 | 1.392 | 2.726 | 1.969 | 1.597 | ||
2.0 | 1.9 | 1.308 | 2.657 | 1.901 | 1.556 | ||
2.3 | 2.4 | 1.161 | 2.517 | 1.770 | 1.475 | ||
2.6 | 2.7 | 1.051 | 2.397 | 1.661 | 1.404 | ||
3.0 | 3.2 | 0.903 | 2.207 | 1.500 | 1.293 | ||
2.0 | 0.25 | 0.2 | 0.4 | 2.180 | 1.987 | 1.837 | 0.993 |
0.5 | 0.6 | 2.167 | 1.986 | 1.835 | 0.993 | ||
0.8 | 0.8 | 2.053 | 1.982 | 1.812 | 0.991 | ||
1.2 | 1.1 | 1.876 | 1.973 | 1.769 | 0.987 | ||
1.5 | 1.3 | 1.753 | 1.965 | 1.734 | 0.982 | ||
1.8 | 1.7 | 1.595 | 1.949 | 1.681 | 0.975 | ||
2.0 | 1.9 | 1.510 | 1.938 | 1.648 | 0.969 | ||
2.3 | 2.4 | 1.358 | 1.912 | 1.581 | 0.956 | ||
2.6 | 2.7 | 1.247 | 1.887 | 1.524 | 0.943 | ||
3.0 | 3.2 | 1.098 | 1.840 | 1.435 | 0.920 | ||
0.5 | 0.2 | 0.4 | 1.210 | 1.877 | 1.503 | 0.938 | |
0.5 | 0.6 | 1.279 | 1.895 | 1.541 | 0.947 | ||
0.8 | 0.8 | 1.210 | 1.877 | 1.503 | 0.938 | ||
1.2 | 1.1 | 1.080 | 1.834 | 1.423 | 0.917 | ||
1.5 | 1.3 | 0.987 | 1.794 | 1.358 | 0.897 | ||
1.8 | 1.7 | 0.861 | 1.725 | 1.258 | 0.862 | ||
2.0 | 1.9 | 0.795 | 1.679 | 1.199 | 0.840 | ||
2.3 | 2.4 | 0.672 | 1.574 | 1.077 | 0.787 | ||
2.6 | 2.7 | 0.587 | 1.482 | 0.982 | 0.741 | ||
3.0 | 3.2 | 0.470 | 1.323 | 0.836 | 0.661 |
ε | β | α | RE | HaCE | ArE | TsE |
1.5 | 0.25 | 0.2 | 7.001 | 3.311 | 2.709 | 1.94 |
0.5 | 7.075 | 3.315 | 2.716 | 1.942 | ||
0.8 | 7.156 | 3.319 | 2.724 | 1.944 | ||
1.2 | 7.274 | 3.324 | 2.735 | 1.947 | ||
1.5 | 7.372 | 3.329 | 2.743 | 1.95 | ||
1.8 | 7.476 | 3.333 | 2.752 | 1.952 | ||
2 | 7.55 | 3.336 | 2.758 | 1.954 | ||
2.3 | 7.667 | 3.34 | 2.767 | 1.957 | ||
2.6 | 7.792 | 3.345 | 2.777 | 1.959 | ||
3 | 7.971 | 3.351 | 2.79 | 1.963 | ||
0.4 | 0.2 | 6.441 | 3.278 | 2.65 | 1.92 | |
0.5 | 6.452 | 3.279 | 2.651 | 1.921 | ||
0.8 | 6.469 | 3.28 | 2.653 | 1.921 | ||
1.2 | 6.503 | 3.282 | 2.657 | 1.923 | ||
1.5 | 6.536 | 3.284 | 2.66 | 1.924 | ||
1.8 | 6.577 | 3.287 | 2.665 | 1.925 | ||
2 | 6.608 | 3.289 | 2.668 | 1.927 | ||
2.3 | 6.661 | 3.292 | 2.674 | 1.928 | ||
2.6 | 6.721 | 3.296 | 2.681 | 1.931 | ||
3 | 6.813 | 3.301 | 2.69 | 1.934 | ||
2.0 | 0.25 | 0.2 | 4.376 | 1.975 | 1.776 | 0.987 |
0.5 | 4.429 | 1.976 | 1.782 | 0.988 | ||
0.8 | 4.487 | 1.977 | 1.788 | 0.989 | ||
1.2 | 4.57 | 1.979 | 1.796 | 0.99 | ||
1.5 | 4.639 | 1.981 | 1.803 | 0.99 | ||
1.8 | 4.713 | 1.982 | 1.81 | 0.991 | ||
2 | 4.765 | 1.983 | 1.815 | 0.991 | ||
2.3 | 4.847 | 1.984 | 1.823 | 0.992 | ||
2.6 | 4.934 | 1.986 | 1.83 | 0.993 | ||
3 | 5.058 | 1.987 | 1.841 | 0.994 | ||
0.4 | 0.2 | 3.975 | 1.962 | 1.726 | 0.981 | |
0.5 | 3.987 | 1.963 | 1.728 | 0.981 | ||
0.8 | 4.003 | 1.963 | 1.73 | 0.982 | ||
1.2 | 4.031 | 1.965 | 1.734 | 0.982 | ||
1.5 | 4.058 | 1.965 | 1.737 | 0.983 | ||
1.8 | 4.09 | 1.967 | 1.741 | 0.983 | ||
2 | 4.114 | 1.967 | 1.744 | 0.984 | ||
2.3 | 4.154 | 1.969 | 1.749 | 0.984 | ||
2.6 | 4.199 | 1.97 | 1.755 | 0.985 | ||
3 | 4.267 | 1.972 | 1.763 | 0.986 |
n | r | Set1 (α = 0.5, β = 0.5, γ = 0.5) | |||||||
MLE | Bias | MSE | LB | UB | AL | CP | |||
50 | 70% | α | 0.4204 | 0.0796 | 0.0064 | 0.0019 | 0.839 | 0.8370 | 97.4% |
β | 0.7041 | 0.2041 | 0.0471 | 0.5036 | 0.9046 | 0.4010 | 96.9% | ||
γ | 0.4201 | 0.0799 | 0.0069 | 0.3023 | 0.5379 | 0.2356 | 96.0% | ||
80% | α | 0.4218 | 0.0782 | 0.0061 | 0.0191 | 0.8245 | 0.8053 | 94.8% | |
β | 0.6382 | 0.1382 | 0.0242 | 0.4508 | 0.8256 | 0.3748 | 95.8% | ||
γ | 0.4386 | 0.0614 | 0.0053 | 0.3282 | 0.5490 | 0.2208 | 97.1% | ||
100% | α | 0.4234 | 0.0766 | 0.0059 | 0.0357 | 0.8111 | 0.7754 | 95.4% | |
β | 0.5177 | 0.0177 | 0.0056 | 0.3661 | 0.6694 | 0.3033 | 95.5% | ||
γ | 0.5316 | 0.0316 | 0.0027 | 0.4303 | 0.6328 | 0.2025 | 96.0% | ||
100 | 70% | α | 0.4213 | 0.0787 | 0.0062 | 0.0844 | 0.7583 | 0.6740 | 96.2% |
β | 0.6750 | 0.1750 | 0.0312 | 0.5375 | 0.8125 | 0.2750 | 95.9% | ||
γ | 0.4237 | 0.0763 | 0.0065 | 0.3389 | 0.5084 | 0.1694 | 96.0% | ||
80% | α | 0.4230 | 0.0770 | 0.0061 | 0.2099 | 0.6360 | 0.4262 | 96.2% | |
β | 0.6099 | 0.1099 | 0.0127 | 0.4819 | 0.7379 | 0.2560 | 96.1% | ||
γ | 0.4487 | 0.0513 | 0.0033 | 0.3652 | 0.5321 | 0.1669 | 97.3% | ||
100% | α | 0.4238 | 0.0762 | 0.0058 | 0.2501 | 0.5975 | 0.3473 | 95.6% | |
β | 0.4683 | 0.0317 | 0.0027 | 0.3558 | 0.5807 | 0.2249 | 95.8% | ||
γ | 0.4967 | 0.0033 | 0.0025 | 0.4199 | 0.5734 | 0.1535 | 96.0% | ||
150 | 70% | α | 0.4217 | 0.0783 | 0.0061 | 0.2710 | 0.5725 | 0.3015 | 95.2% |
β | 0.6626 | 0.1626 | 0.0281 | 0.5577 | 0.7675 | 0.2097 | 95.6% | ||
γ | 0.4277 | 0.0723 | 0.0058 | 0.3571 | 0.4983 | 0.1412 | 97.3% | ||
80% | α | 0.4236 | 0.0764 | 0.0059 | 0.3005 | 0.5466 | 0.2461 | 95.7% | |
β | 0.5977 | 0.0977 | 0.0113 | 0.4957 | 0.6997 | 0.2040 | 96.2% | ||
γ | 0.4649 | 0.0351 | 0.0022 | 0.3972 | 0.5325 | 0.1353 | 97.0% | ||
100% | α | 0.4238 | 0.0762 | 0.0058 | 0.3010 | 0.5467 | 0.2457 | 95.6% | |
β | 0.4766 | 0.0234 | 0.0023 | 0.3784 | 0.5749 | 0.1965 | 96.4% | ||
γ | 0.5277 | 0.0277 | 0.0015 | 0.4659 | 0.5894 | 0.1236 | 96.9% | ||
200 | 70% | α | 0.4219 | 0.0781 | 0.0061 | 0.3154 | 0.5285 | 0.2132 | 96.1% |
β | 0.6592 | 0.1592 | 0.0268 | 0.5675 | 0.7510 | 0.1835 | 96.3% | ||
γ | 0.4375 | 0.0625 | 0.0046 | 0.3789 | 0.4962 | 0.1173 | 96.7% | ||
80% | α | 0.4239 | 0.0761 | 0.0058 | 0.3236 | 0.5242 | 0.2006 | 96.3% | |
β | 0.5912 | 0.0912 | 0.0099 | 0.5074 | 0.6750 | 0.1676 | 97.0% | ||
γ | 0.4667 | 0.0333 | 0.0020 | 0.4101 | 0.5233 | 0.1132 | 97.5% | ||
100% | α | 0.4240 | 0.0760 | 0.0058 | 0.3372 | 0.5109 | 0.1737 | 96.5% | |
β | 0.4905 | 0.0095 | 0.0009 | 0.4167 | 0.5642 | 0.1475 | 96.7% | ||
γ | 0.5035 | 0.0035 | 0.0006 | 0.4524 | 0.5546 | 0.1022 | 97.1% | ||
n | r | Set2 (α = 0.7, β = 0.5, γ = 0.25) | |||||||
MLE | Bias | MSE | LB | UB | AL | CP | |||
50 | 70% | α | 0.4206 | 0.2794 | 0.0782 | 0.0055 | 0.8358 | 0.8304 | 97.7% |
β | 0.7016 | 0.2016 | 0.0462 | 0.5015 | 0.9016 | 0.4001 | 96.5% | ||
γ | 0.2172 | 0.0328 | 0.0017 | 0.1568 | 0.2776 | 0.1208 | 100% | ||
80% | α | 0.4214 | 0.2786 | 0.0776 | 0.2084 | 0.6345 | 0.4261 | 97.9% | |
β | 0.6361 | 0.1361 | 0.0243 | 0.4490 | 0.8231 | 0.3741 | 98.5% | ||
γ | 0.2297 | 0.0203 | 0.0010 | 0.1719 | 0.2874 | 0.1155 | 100% | ||
100% | α | 0.4234 | 0.2766 | 0.0765 | 0.2497 | 0.5970 | 0.3473 | 98.3% | |
β | 0.5161 | 0.0161 | 0.0062 | 0.3649 | 0.6673 | 0.3025 | 97.6% | ||
γ | 0.2565 | 0.0065 | 0.0007 | 0.2044 | 0.3086 | 0.1043 | 100% | ||
100 | 70% | α | 0.4210 | 0.2790 | 0.0779 | 0.0208 | 0.8212 | 0.8004 | 96.4% |
β | 0.7006 | 0.2006 | 0.0431 | 0.5593 | 0.8419 | 0.2826 | 98.0% | ||
γ | 0.2141 | 0.0359 | 0.0016 | 0.1720 | 0.2562 | 0.0842 | 100% | ||
80% | α | 0.4215 | 0.2785 | 0.0776 | 0.2708 | 0.5721 | 0.3013 | 97.2% | |
β | 0.6357 | 0.1357 | 0.0214 | 0.5035 | 0.7680 | 0.2645 | 97.7% | ||
γ | 0.2270 | 0.0230 | 0.0008 | 0.1866 | 0.2673 | 0.0807 | 100% | ||
100% | α | 0.4234 | 0.2766 | 0.0765 | 0.3006 | 0.5462 | 0.2456 | 97.3% | |
β | 0.5158 | 0.0158 | 0.0033 | 0.4088 | 0.6227 | 0.2140 | 98.2% | ||
γ | 0.2540 | 0.0040 | 0.0003 | 0.2176 | 0.2905 | 0.0729 | 100% | ||
150 | 70% | α | 0.4212 | 0.2788 | 0.0778 | 0.0330 | 0.8093 | 0.7763 | 97.7% |
β | 0.7000 | 0.2000 | 0.0419 | 0.5847 | 0.8153 | 0.2306 | 97.7% | ||
γ | 0.2122 | 0.0378 | 0.0016 | 0.1781 | 0.2463 | 0.0682 | 100% | ||
80% | α | 0.4215 | 0.2785 | 0.0776 | 0.2985 | 0.5445 | 0.2460 | 98.8% | |
β | 0.6350 | 0.1350 | 0.0203 | 0.5271 | 0.7430 | 0.2159 | 98.1% | ||
γ | 0.2259 | 0.0241 | 0.0008 | 0.1931 | 0.2587 | 0.0656 | 96.0% | ||
100% | α | 0.4234 | 0.2766 | 0.0765 | 0.3232 | 0.5237 | 0.2005 | 97.2% | |
β | 0.5151 | 0.0151 | 0.0023 | 0.4278 | 0.6024 | 0.1746 | 97.0% | ||
γ | 0.2529 | 0.0029 | 0.0002 | 0.2232 | 0.2825 | 0.0593 | 95.4% | ||
200 | 70% | α | 0.4209 | 0.2791 | 0.0779 | 0.0849 | 0.7569 | 0.6720 | 100% |
β | 0.6981 | 0.1981 | 0.0405 | 0.5984 | 0.7978 | 0.1994 | 97.2% | ||
γ | 0.2118 | 0.0382 | 0.0016 | 0.1823 | 0.2412 | 0.0589 | 97.3% | ||
80% | α | 0.4215 | 0.2785 | 0.0776 | 0.3150 | 0.5280 | 0.2131 | 100% | |
β | 0.6331 | 0.1331 | 0.0191 | 0.5398 | 0.7265 | 0.1867 | 98.2% | ||
γ | 0.2256 | 0.0244 | 0.0007 | 0.1973 | 0.2540 | 0.0567 | 98.0% | ||
100% | α | 0.4234 | 0.2766 | 0.0765 | 0.3366 | 0.5103 | 0.1737 | 100% | |
β | 0.5136 | 0.0136 | 0.0016 | 0.4381 | 0.5891 | 0.1510 | 98.8% | ||
γ | 0.2523 | 0.0023 | 0.0002 | 0.2267 | 0.2779 | 0.0512 | 100% | ||
n | r | Set3 (α = 0.7, β = 0.7, γ = 0.5) | |||||||
MLE | Bias | MSE | LB | UB | AL | CP | |||
50 | 70% | α | 0.4178 | 0.2822 | 0.0797 | 0.1937 | 0.6419 | 0.4482 | 96.2% |
β | 0.8994 | 0.1994 | 0.0477 | 0.6064 | 1.1923 | 0.5859 | 95.9% | ||
γ | 0.6151 | 0.1151 | 0.0239 | 0.3763 | 0.8540 | 0.4776 | 95.0% | ||
80% | α | 0.4193 | 0.2807 | 0.0788 | 0.2255 | 0.6131 | 0.3875 | 95.9% | |
β | 0.8238 | 0.1238 | 0.0218 | 0.5471 | 1.1006 | 0.5535 | 95.9% | ||
γ | 0.5695 | 0.0695 | 0.0182 | 0.3447 | 0.7943 | 0.4496 | 96.7% | ||
100% | α | 0.4211 | 0.2789 | 0.0778 | 0.2480 | 0.5942 | 0.3461 | 96.8% | |
β | 0.7612 | 0.0612 | 0.0104 | 0.5012 | 1.0213 | 0.5201 | 97.0% | ||
γ | 0.5425 | 0.0425 | 0.0163 | 0.3395 | 0.7456 | 0.4061 | 95.0% | ||
100 | 70% | α | 0.4174 | 0.2826 | 0.0798 | 0.2439 | 0.5910 | 0.3470 | 95.0% |
β | 0.8787 | 0.1787 | 0.0353 | 0.6426 | 1.1148 | 0.4722 | 96.3% | ||
γ | 0.5696 | 0.0696 | 0.0201 | 0.3697 | 0.7696 | 0.3998 | 96.0% | ||
80% | α | 0.4191 | 0.2809 | 0.0789 | 0.2690 | 0.5691 | 0.3001 | 95.5% | |
β | 0.7802 | 0.0802 | 0.0134 | 0.5600 | 1.0004 | 0.4404 | 95.7% | ||
γ | 0.5479 | 0.0479 | 0.0091 | 0.3814 | 0.7144 | 0.3330 | 96.0% | ||
100% | α | 0.4206 | 0.2794 | 0.0781 | 0.2866 | 0.5546 | 0.2680 | 95.6% | |
β | 0.7146 | 0.0146 | 0.0068 | 0.5069 | 0.9223 | 0.4154 | 95.7% | ||
γ | 0.5597 | 0.0597 | 0.0074 | 0.4063 | 0.7131 | 0.3067 | 96.0% | ||
150 | 70% | α | 0.4176 | 0.2824 | 0.0798 | 0.2949 | 0.5403 | 0.2454 | 95.8% |
β | 0.8697 | 0.1697 | 0.0305 | 0.7056 | 1.0338 | 0.3282 | 96.2% | ||
γ | 0.5924 | 0.0924 | 0.0174 | 0.4449 | 0.7399 | 0.2950 | 97.1% | ||
80% | α | 0.4193 | 0.2807 | 0.0788 | 0.3131 | 0.5254 | 0.2122 | 96.2% | |
β | 0.8023 | 0.1023 | 0.0124 | 0.6492 | 0.9555 | 0.3063 | 96.1% | ||
γ | 0.5524 | 0.0524 | 0.0058 | 0.4332 | 0.6716 | 0.2384 | 97.0% | ||
100% | α | 0.4209 | 0.2791 | 0.0779 | 0.3261 | 0.5156 | 0.1895 | 95.8% | |
β | 0.7374 | 0.0374 | 0.0032 | 0.5932 | 0.8817 | 0.2885 | 96.3% | ||
γ | 0.5507 | 0.0507 | 0.0041 | 0.4447 | 0.6568 | 0.2121 | 96.9% | ||
200 | 70% | α | 0.4175 | 0.2825 | 0.0798 | 0.3173 | 0.5177 | 0.2004 | 96.1% |
β | 0.8406 | 0.1406 | 0.0265 | 0.7075 | 0.9736 | 0.2661 | 97.2% | ||
γ | 0.5683 | 0.0683 | 0.0069 | 0.4569 | 0.6798 | 0.2230 | 96.9% | ||
80% | α | 0.4193 | 0.2807 | 0.0788 | 0.3327 | 0.5059 | 0.1733 | 96.3% | |
β | 0.8043 | 0.1043 | 0.0123 | 0.6790 | 0.9295 | 0.2505 | 96.6% | ||
γ | 0.5502 | 0.0502 | 0.0041 | 0.4533 | 0.6471 | 0.1938 | 97.0% | ||
100% | α | 0.4209 | 0.2791 | 0.0779 | 0.3435 | 0.4983 | 0.1548 | 96.1% | |
β | 0.7411 | 0.0411 | 0.0030 | 0.6229 | 0.8592 | 0.2362 | 97.0% | ||
γ | 0.5463 | 0.0463 | 0.0041 | 0.4589 | 0.6338 | 0.1749 | 96.2% | ||
n | r | Set4 (α = 0.6, β = 0.3, γ = 0.5) | |||||||
MLE | Bias | MSE | LB | UB | AL | CP | |||
50 | 70% | α | 0.4197 | 0.1803 | 0.0325 | 0.2456 | 0.5939 | 0.3482 | 98.1% |
β | 0.5719 | 0.2719 | 0.0744 | 0.3742 | 0.7696 | 0.3954 | 97.0% | ||
γ | 0.2990 | 0.2010 | 0.0406 | 0.1769 | 0.4211 | 0.2442 | 98.0% | ||
80% | α | 0.4221 | 0.1779 | 0.0317 | 0.2716 | 0.5725 | 0.3009 | 98.4% | |
β | 0.4934 | 0.1934 | 0.0384 | 0.3126 | 0.6742 | 0.3616 | 97.4% | ||
γ | 0.3593 | 0.1407 | 0.0200 | 0.2383 | 0.4803 | 0.2421 | 98.2% | ||
100% | α | 0.4246 | 0.1754 | 0.0308 | 0.2903 | 0.5589 | 0.2686 | 97.9% | |
β | 0.4198 | 0.1198 | 0.0150 | 0.2576 | 0.5820 | 0.3244 | 97.4% | ||
γ | 0.4172 | 0.0828 | 0.0078 | 0.2968 | 0.5376 | 0.2408 | 98.4% | ||
100 | 70% | α | 0.4198 | 0.1802 | 0.0325 | 0.2966 | 0.5429 | 0.2463 | 97.2% |
β | 0.5674 | 0.2674 | 0.0717 | 0.4278 | 0.7069 | 0.2791 | 97.7% | ||
γ | 0.3137 | 0.1863 | 0.0351 | 0.2278 | 0.3995 | 0.1717 | 98.0% | ||
80% | α | 0.4222 | 0.1778 | 0.0316 | 0.3157 | 0.5286 | 0.2128 | 97.9% | |
β | 0.4857 | 0.1857 | 0.0350 | 0.3584 | 0.6130 | 0.2546 | 97.9% | ||
γ | 0.3669 | 0.1331 | 0.0179 | 0.2818 | 0.4519 | 0.1701 | 98.7% | ||
100% | α | 0.4248 | 0.1752 | 0.0307 | 0.3242 | 0.5253 | 0.2011 | 98.1% | |
β | 0.4082 | 0.1082 | 0.0125 | 0.2944 | 0.5221 | 0.2277 | 97.8% | ||
γ | 0.4171 | 0.0829 | 0.0074 | 0.3349 | 0.4993 | 0.1644 | 98.3% | ||
150 | 70% | α | 0.4198 | 0.1802 | 0.0325 | 0.3248 | 0.5148 | 0.1899 | 97.2% |
β | 0.5653 | 0.2653 | 0.0706 | 0.4518 | 0.6788 | 0.2270 | 98.3% | ||
γ | 0.3140 | 0.1860 | 0.0350 | 0.2421 | 0.3860 | 0.1439 | 99.3% | ||
80% | α | 0.4222 | 0.1778 | 0.0316 | 0.3353 | 0.5091 | 0.1738 | 97.7% | |
β | 0.4832 | 0.1832 | 0.0338 | 0.3797 | 0.5866 | 0.2069 | 98.2% | ||
γ | 0.3677 | 0.1323 | 0.0178 | 0.2996 | 0.4358 | 0.1363 | 99.0% | ||
100% | α | 0.4248 | 0.1752 | 0.0307 | 0.3377 | 0.5119 | 0.1742 | 97.3% | |
β | 0.4018 | 0.1018 | 0.0107 | 0.3032 | 0.5004 | 0.1972 | 97.9% | ||
γ | 0.4248 | 0.0752 | 0.0061 | 0.3608 | 0.4888 | 0.1280 | 99.7% | ||
200 | 70% | α | 0.4198 | 0.1802 | 0.0325 | 0.3423 | 0.4973 | 0.1551 | 99.0% |
β | 0.5650 | 0.2650 | 0.0705 | 0.4738 | 0.6561 | 0.1823 | 99.1% | ||
γ | 0.3256 | 0.1744 | 0.0309 | 0.2637 | 0.3875 | 0.1239 | 98.7% | ||
80% | α | 0.4222 | 0.1778 | 0.0316 | 0.3470 | 0.4975 | 0.1505 | 99.6% | |
β | 0.4781 | 0.1781 | 0.0321 | 0.3882 | 0.5679 | 0.1798 | 99.3% | ||
γ | 0.3733 | 0.1267 | 0.0173 | 0.3129 | 0.4336 | 0.1206 | 99.5% | ||
100% | α | 0.4250 | 0.1750 | 0.0306 | 0.3578 | 0.4921 | 0.1343 | 98.7% | |
β | 0.3904 | 0.0904 | 0.0088 | 0.3106 | 0.4703 | 0.1596 | 99.6% | ||
γ | 0.4262 | 0.0738 | 0.0060 | 0.3681 | 0.4843 | 0.1162 | 100% |
Rank | Country | % Global Reserves | Rank | Country | % Global Reserves |
1 | Russia | 19.9 | 23 | Ukraine | 0.6 |
2 | Iran | 17.1 | 24 | Malaysia | 0.5 |
3 | Qatar | 13.1 | 25 | Uzbekistan | 0.4 |
4 | Turkmenistan | 7.2 | 26 | Oman | 0.4 |
5 | United States | 6.7 | 27 | Vietnam | 0.3 |
6 | China | 4.5 | 28 | Israel | 0.3 |
7 | Venezuela | 3.3 | 29 | Argentina | 0.2 |
8 | Saudi Arabia | 3.2 | 30 | Pakistan | 0.2 |
9 | United Arab Emirates | 3.2 | 31 | Trinidad | 0.2 |
10 | Nigeria | 2.9 | 32 | Brazil | 0.2 |
11 | Iraq | 1.9 | 33 | Myanmar | 0.2 |
12 | Canada | 1.3 | 34 | United Kingdom | 0.1 |
13 | Australia | 1.3 | 35 | Thailand | 0.1 |
14 | Azerbaijan | 1.3 | 36 | Mexico | 0.1 |
15 | Algeria | 1.2 | 37 | Bangladesh | 0.1 |
16 | Kazakhstan | 1.2 | 38 | Netherlands | 0.1 |
17 | Egypt | 1.1 | 39 | Bolivia | 0.1 |
18 | Kuwait | 0.9 | 40 | Brunei | 0.1 |
19 | Norway | 0.8 | 41 | Peru | 0.1 |
20 | Libya | 0.8 | 42 | Syria | 0.1 |
21 | Indonesia | 0.7 | 43 | Yemen | 0.1 |
22 | India | 0.7 | 44 | Papua New Guinea | 0.1 |
Rank | Country | reserves2020 | Rank | Country | reserves2020 |
1 | Venezuela | 303.8 | 11 | Nigeria | 36.9 |
2 | Saudi Arabia | 297.5 | 12 | Kazakhstan | 30 |
3 | Canada | 168.1 | 13 | China | 26 |
4 | Iran | 157.8 | 14 | Qatar | 25.2 |
5 | Iraq | 145 | 15 | Algeria | 12.2 |
6 | Russia | 107.8 | 16 | Brazil | 11.9 |
7 | Kuwait | 101.5 | 17 | Norway | 7.9 |
8 | United Arab Emirates | 97.8 | 18 | Angola | 7.8 |
9 | United States | 68.8 | 19 | Azerbaijan | 7 |
10 | Libya | 48.4 | 20 | Mexico | 6.1 |
Rank | Country | Reserves of Gold | Rank | Country | Reserves of Gold | Rank | Country | Reserves of Gold |
1 | USA | 8.1335 | 35 | LBY | 0.1166 | 68 | CYP | 0.0139 |
2 | DEU | 3.3585 | 36 | GRC | 0.1141 | 69 | CUW | 0.0131 |
3 | IMF | 2.814 | 37 | ROK | 0.1045 | 70 | MUS | 0.0124 |
4 | ITA | 2.4518 | 38 | ROU | 0.1036 | 71 | IRL | 0.012 |
5 | FRA | 2.4365 | 39 | BIS | 0.102 | 72 | CZE | 0.0109 |
6 | RUS | 2.2985 | 40 | IRQ | 0.0964 | 73 | KGZ | 0.0102 |
7 | CHN | 1.9483 | 41 | HUN | 0.0945 | 74 | GHA | 0.0087 |
8 | CHE | 1.04 | 42 | AUS | 0.0798 | 75 | PRY | 0.0082 |
9 | JPN | 0.846 | 43 | KWT | 0.079 | 76 | NPL | 0.008 |
10 | IND | 0.7604 | 44 | IDN | 0.0786 | 77 | MNG | 0.0076 |
11 | NLD | 0.6125 | 45 | DNK | 0.0666 | 78 | MMR | 0.0073 |
12 | ECB | 0.5048 | 46 | PAK | 0.0647 | 79 | GTM | 0.0069 |
13 | TUR | 0.4311 | 47 | ARG | 0.0617 | 80 | MKD | 0.0069 |
14 | TAI | 0.4236 | 48 | ARE | 0.0553 | 81 | TUN | 0.0068 |
15 | PRT | 0.3826 | 49 | BLR | 0.0535 | 82 | LVA | 0.0067 |
16 | KAZ | 0.3681 | 50 | QAT | 0.0513 | 83 | LTU | 0.0058 |
17 | UZB | 0.3375 | 51 | KHM | 0.0504 | 84 | COL | 0.0047 |
18 | SAU | 0.3231 | 52 | FIN | 0.049 | 85 | BHR | 0.0047 |
19 | GBR | 0.3103 | 53 | JOR | 0.0435 | 86 | BRN | 0.0046 |
20 | LBN | 0.2868 | 54 | BOL | 0.0425 | 87 | GIN | 0.0042 |
21 | ESP | 0.2816 | 55 | BGR | 0.0408 | 88 | MOZ | 0.0039 |
22 | AUT | 0.28 | 56 | MYS | 0.0389 | 89 | SVN | 0.0032 |
23 | THA | 0.2442 | 57 | SRB | 0.0378 | 90 | ABW | 0.0031 |
24 | POL | 0.2287 | 58 | WAEMU | 0.0365 | 91 | BIH | 0.003 |
25 | BEL | 0.2274 | 59 | PER | 0.0347 | 92 | ALB | 0.0028 |
26 | DZA | 0.1736 | 60 | SVK | 0.0317 | 93 | LUX | 0.0022 |
27 | VEN | 0.1612 | 61 | UKR | 0.0271 | 94 | HKG | 0.0021 |
28 | PHL | 0.1563 | 62 | SYR | 0.0258 | 95 | ISL | 0.002 |
29 | SGP | 0.1537 | 63 | MAR | 0.0221 | 96 | TTO | 0.0019 |
30 | BRA | 0.1297 | 64 | ECU | 0.0219 | 97 | HTI | 0.0018 |
31 | SWE | 0.1257 | 65 | AFG | 0.0219 | 98 | YEM | 0.0016 |
32 | ZAF | 0.1254 | 66 | NGA | 0.0215 | 99 | SUR | 0.0015 |
33 | EGY | 0.125 | 67 | BGD | 0.014 | 100 | SLV | 0.0014 |
34 | MEX | 0.1199 |
n | Mean | Median | Skewness | Kurtosis | Range | Min | Max | Sum | |
Data1 | 20 | 1.900 | 1.700 | 1.860 | 4.185 | 3.000 | 1.100 | 4.100 | 38.000 |
Data2 | 44 | 2.248 | 0.650 | 2.990 | 8.864 | 19.800 | 0.100 | 19.900 | 98.900 |
Data3 | 20 | 83.375 | 42.650 | 1.430 | 1.420 | 297.700 | 6.100 | 303.800 | 1667.500 |
Data4 | 100 | 0.347 | 0.050 | 5.590 | 38.257 | 8.130 | 0.001 | 8.133 | 34.676 |
Distributions | MLE and SE | ||||
α | β | γ | λ | θ | |
LBTLoW | 8.648 | 3.074 | 0.042 | ||
(3.545) | (0.474) | (0.025) | |||
ETGR | 0.103 | 0.692 | 23.539 | -0.342 | |
(0.436) | (0.086) | (105.137) | (1.971) | ||
BW | 0.831 | 0.613 | 29.947 | 11.632 | |
(0.954) | (0.340) | (40.414) | (21.900) | ||
T-Li | 0.665 | 0.359 | |||
(0.332) | (0.048) | ||||
McLL | 0.881 | 2.070 | 1.926 | 19.225 | 32.033 |
(0.109) | (3.693) | (5.165) | (22.341) | (43.081) | |
NMW | 0.121 | 2.784 | 2.787 | 0.003 | 0.008 |
(0.056) | (20.370) | (0.428) | (0.025) | (0.002) | |
W | 0.122 | 2.787 | |||
(0.056) | (0.427) |
Distributions | AIC | CAIC | BIC | HQIC | KS | PV |
LBTLoW | 40.140 | 41.640 | 38.040 | 40.720 | 0.146 | 0.790 |
ETGR | 44.860 | 47.520 | 42.060 | 45.630 | 0.190 | 0.465 |
BW | 42.400 | 45.060 | 39.600 | 43.170 | 0.160 | 0.683 |
T-Li | 65.730 | 66.440 | 64.330 | 66.120 | 0.380 | 0.006 |
McLL | 43.850 | 48.140 | 40.360 | 44.830 | 0.147 | 0.734 |
NMW | 51.170 | 55.460 | 47.680 | 52.150 | 0.190 | 0.501 |
W | 45.170 | 45.880 | 43.780 | 45.560 | 0.180 | 0.509 |
Distributions | MLE and SE | ||||
α | β | γ | λ | θ | |
LBTLoW | 6.268 | 0.623 | 0.484 | ||
(2.631) | (0.066) | (0.210) | |||
ETGR | 0.055 | 0.071 | 8.773 | 0.947 | |
(0.027) | (0.029) | (7.043) | (0.081) | ||
TCWG | 34.076 | 0.802 | 0.005 | 1.12 | |
(81.023) | (0.021) | (0.013) | (0.285) | ||
EKW | 0.221 | 400.298 | 5.215 | 1 | 3.823 |
(0.038) | (718.99) | (0.649) | (0.004) | (3.036) | |
TMW | 0.851 | 1.159 | -0.554 | 0.519 | |
(0.163) | (1.026) | (0.985) | (0.379) | ||
BW | 2.861 | 0.075 | 78.550 | 42.576 | |
(69.095) | (0.090) | (167.320) | (187.300) | ||
T-Li | 0.604 | 0.671 | |||
(0.155) | (0.074) | ||||
McLL | 0.181 | 1.565 | 1.286 | 21.234 | 28.124 |
(0.193) | (9.254) | (5.432) | (34.701) | (45.757) | |
NMW | 6.8 x 10−8 | 0.680 | 0.223 | 0.015 | 0.806 |
(0.623) | (0.110) | (617.48) | (0.015) | (0.418) | |
W | 0.799 | 0.621 | |||
(0.136) | (0.068) |
Distributions | AIC | CAIC | BIC | HQIC | KS | PV |
LBTLoW | 132.210 | 132.810 | 131.140 | 134.200 | 0.130 | 0.425 |
ETGR | 143.470 | 144.490 | 142.040 | 146.110 | 0.180 | 0.118 |
TCWG | 137.690 | 138.710 | 136.260 | 140.330 | 0.150 | 0.251 |
EKW | 133.890 | 135.470 | 132.110 | 140.330 | 0.140 | 0.355 |
TMW | 140.900 | 142.480 | 139.120 | 144.210 | 0.150 | 0.276 |
BW | 133.180 | 134.200 | 131.750 | 135.820 | 0.130 | 0.408 |
T-Li | 174.360 | 174.660 | 173.650 | 175.690 | 0.200 | 0.057 |
McLL | 134.830 | 136.410 | 133.040 | 138.130 | 0.130 | 0.419 |
NMW | 143.780 | 145.360 | 142.000 | 147.090 | 0.160 | 0.243 |
W | 138.650 | 138.940 | 137.940 | 139.970 | 0.170 | 0.139 |
Distributions | MLE and SE | ||||
α | β | γ | λ | θ | |
LBTLoW | 2.515 | 0.756 | 0.040 | ||
(3.877) | (0.166) | (0.048) | |||
WEIW | 0.909 | 0.871 | 7.225 | ||
(106700) | (0.152) | (384700) | |||
TMW | 0.998 | 0.459 | -0.443 | 0.202 | |
(0.081) | (18.537) | (18.537) | (0.769) | ||
T-Li | 0.021 | 0.384 | |||
(0.345) | (0.004) | ||||
McLL | 0.208 | 93.978 | 1.279 | 24.759 | 32.815 |
(0.499) | (1721) | (19.272) | (142.806) | (161.611) | |
NMW | 10.7 x 10−8 | 0.930 | 0.859 | 7.46 x 10−8 | 0.017 |
(0.001) | (0.250) | (1.216) | (0.002) | (0.017) | |
EKW | 0.167 | 261.64 | 45.725 | 1.201 | 2.138 |
(0.079) | (1709) | (219.725) | (0.741) | (7.209) |
Distributions | AIC | CAIC | BIC | HQIC | KS | PV |
LBTLoW | 221.690 | 223.190 | 219.600 | 222.280 | 0.135 | 0.857 |
WEIW | 223.400 | 224.900 | 221.300 | 223.980 | 0.157 | 0.708 |
TMW | 226.410 | 230.690 | 222.910 | 227.380 | 0.153 | 0.734 |
T-Li | 230.480 | 231.180 | 229.080 | 230.870 | 0.265 | 0.120 |
McLL | 225.990 | 230.280 | 222.500 | 222.500 | 0.146 | 0.789 |
NMW | 226.570 | 230.860 | 223.080 | 227.540 | 0.140 | 0.826 |
EKW | 226.290 | 230.570 | 222.790 | 229.300 | 0.148 | 0.776 |
Distributions | MLE and SE | ||||
α | β | γ | λ | θ | |
LBTLoW | 6.498 | 0.482 | 1.490 | ||
(2.301) | (0.034) | (0.573) | |||
EKW | 0.221 | 1096 | 4.424 | 1 | 1.717 |
(0.030) | (1376) | (1.817) | (0.001) | (0.901) | |
TMW | 0.596 | 2.612 | 0.588 | -0.523 | |
(0.057) | (0.689) | (0.256) | (0.346) | ||
BW | 134.832 | 0.073 | 49.149 | 22.930 | |
(956.622) | (0.060) | (74.497) | (46.500) | ||
WEIW | 27.512 | 0.549 | 0.094 | ||
(3272000) | (0.042) | (856.967) | |||
W | 2.648 | 0.489 | |||
(0.281) | (0.035) |
Distributions | AIC | CAIC | BIC | HQIC | KS | PV |
LBTLoW | –170.510 | –170.260 | –170.510 | –167.350 | 0.070 | 0.704 |
ETGR | –167.710 | –167.070 | –167.710 | –157.710 | 0.078 | 0.584 |
BW | –158.180 | –157.540 | –158.180 | –152.910 | 0.077 | 0.593 |
T-Li | –170.220 | –169.800 | –170.220 | –166.010 | 0.071 | 0.703 |
McLL | –170.200 | –169.950 | –170.200 | –167.040 | 0.091 | 0.383 |
W | –157.390 | –157.260 | –157.390 | –155.280 | 0.100 | 0.269 |
γ | α | μ′1 | μ′2 | μ′3 | μ′4 | σ2 | CS | CK | CV |
0.4 | 0.2 | 2.629 | 8.323 | 30.531 | 126.387 | 1.413 | 0.729 | 3.596 | 0.452 |
0.6 | 0.5 | 1.558 | 2.937 | 6.430 | 15.922 | 0.508 | 0.746 | 3.627 | 0.457 |
0.8 | 0.8 | 1.184 | 1.700 | 2.847 | 5.403 | 0.300 | 0.765 | 3.660 | 0.463 |
1.1 | 1.2 | 0.925 | 1.044 | 1.380 | 2.074 | 0.189 | 0.793 | 3.717 | 0.470 |
1.3 | 1.5 | 0.805 | 0.794 | 0.920 | 1.216 | 0.146 | 0.814 | 3.758 | 0.475 |
1.7 | 1.8 | 0.705 | 0.615 | 0.634 | 0.748 | 0.117 | 0.856 | 3.853 | 0.486 |
1.9 | 2.0 | 0.655 | 0.532 | 0.513 | 0.568 | 0.103 | 0.878 | 3.906 | 0.491 |
2.4 | 2.3 | 0.582 | 0.425 | 0.371 | 0.374 | 0.086 | 0.937 | 4.058 | 0.503 |
2.7 | 2.6 | 0.530 | 0.355 | 0.285 | 0.266 | 0.073 | 0.974 | 4.162 | 0.511 |
3.2 | 3.0 | 0.469 | 0.280 | 0.203 | 0.172 | 0.060 | 1.038 | 4.359 | 0.522 |
γ | α | μ′1 | μ′2 | μ′3 | μ′4 | σ2 | CS | CK | CV |
0.4 | 0.2 | 1.964 | 4.277 | 10.154 | 25.945 | 0.420 | 0.380 | 3.017 | 0.330 |
0.6 | 0.5 | 1.347 | 2.017 | 3.298 | 5.813 | 0.202 | 0.395 | 3.026 | 0.334 |
0.8 | 0.8 | 1.105 | 1.360 | 1.829 | 2.658 | 0.139 | 0.411 | 3.037 | 0.337 |
1.1 | 1.2 | 0.924 | 0.954 | 1.081 | 1.325 | 0.100 | 0.435 | 3.058 | 0.343 |
1.3 | 1.5 | 0.836 | 0.783 | 0.805 | 0.897 | 0.084 | 0.453 | 3.075 | 0.346 |
1.7 | 1.8 | 0.760 | 0.649 | 0.612 | 0.627 | 0.072 | 0.489 | 3.116 | 0.353 |
1.9 | 2.0 | 0.720 | 0.584 | 0.524 | 0.511 | 0.066 | 0.508 | 3.140 | 0.356 |
2.4 | 2.3 | 0.661 | 0.494 | 0.411 | 0.374 | 0.058 | 0.557 | 3.213 | 0.365 |
2.7 | 2.6 | 0.617 | 0.433 | 0.339 | 0.290 | 0.052 | 0.588 | 3.265 | 0.369 |
3.2 | 3.0 | 0.565 | 0.364 | 0.263 | 0.209 | 0.045 | 0.641 | 3.366 | 0.377 |
β | α | μ′1 | μ′2 | μ′3 | μ′4 | σ2 | CS | CK | CV |
1.5 | 0.2 | 0.029 | 0.026 | 0.023 | 0.021 | 0.025 | 5.273 | 29.151 | 5.386 |
0.5 | 0.032 | 0.028 | 0.025 | 0.023 | 0.027 | 5.014 | 26.463 | 5.138 | |
0.8 | 0.035 | 0.031 | 0.028 | 0.025 | 0.030 | 4.772 | 24.069 | 4.906 | |
1.2 | 0.039 | 0.035 | 0.031 | 0.028 | 0.033 | 4.472 | 21.275 | 4.622 | |
1.5 | 0.043 | 0.038 | 0.033 | 0.03 | 0.036 | 4.264 | 19.441 | 4.424 | |
1.8 | 0.046 | 0.041 | 0.036 | 0.033 | 0.038 | 4.069 | 17.801 | 4.241 | |
2 | 0.049 | 0.043 | 0.038 | 0.034 | 0.040 | 3.946 | 16.806 | 4.125 | |
2.3 | 0.053 | 0.046 | 0.041 | 0.037 | 0.043 | 3.771 | 15.445 | 3.961 | |
2.6 | 0.056 | 0.049 | 0.044 | 0.039 | 0.046 | 3.607 | 14.225 | 3.809 | |
3 | 0.062 | 0.054 | 0.048 | 0.043 | 0.050 | 3.405 | 12.794 | 3.621 | |
2.5 | 0.2 | 0.006 | 0.005 | 0.005 | 0.004 | 0.005 | 12.420 | 156.328 | 12.403 |
0.5 | 0.007 | 0.006 | 0.006 | 0.005 | 0.006 | 11.672 | 138.195 | 11.665 | |
0.8 | 0.007 | 0.007 | 0.006 | 0.006 | 0.007 | 10.981 | 122.434 | 10.984 | |
1.2 | 0.009 | 0.008 | 0.007 | 0.007 | 0.008 | 10.140 | 104.548 | 10.156 | |
1.5 | 0.01 | 0.009 | 0.008 | 0.007 | 0.009 | 9.563 | 93.121 | 9.589 | |
1.8 | 0.011 | 0.010 | 0.009 | 0.008 | 0.010 | 9.030 | 83.140 | 9.066 | |
2 | 0.012 | 0.011 | 0.010 | 0.009 | 0.010 | 8.697 | 77.190 | 8.739 | |
2.3 | 0.013 | 0.012 | 0.011 | 0.010 | 0.012 | 8.227 | 69.194 | 8.279 | |
2.6 | 0.014 | 0.013 | 0.012 | 0.011 | 0.013 | 7.792 | 62.179 | 7.853 | |
3 | 0.017 | 0.015 | 0.014 | 0.013 | 0.015 | 7.261 | 54.128 | 7.334 |
ε | β | α | γ | RE | HaCE | ArE | TsE |
1.5 | 0.25 | 0.2 | 0.4 | 3.331 | 3.340 | 2.767 | 1.957 |
0.5 | 0.6 | 3.252 | 3.333 | 2.753 | 1.953 | ||
0.8 | 0.8 | 3.099 | 3.318 | 2.722 | 1.944 | ||
1.2 | 1.1 | 2.875 | 3.290 | 2.670 | 1.927 | ||
1.5 | 1.3 | 2.716 | 3.264 | 2.627 | 1.912 | ||
1.8 | 1.7 | 2.524 | 3.227 | 2.568 | 1.891 | ||
2.0 | 1.9 | 2.415 | 3.203 | 2.53 | 1.876 | ||
2.3 | 2.4 | 2.229 | 3.152 | 2.458 | 1.846 | ||
2.6 | 2.7 | 2.084 | 3.104 | 2.394 | 1.818 | ||
3.0 | 3.2 | 1.889 | 3.026 | 2.296 | 1.773 | ||
0.5 | 0.2 | 0.4 | 1.930 | 3.044 | 2.318 | 1.783 | |
0.5 | 0.6 | 1.924 | 3.042 | 2.315 | 1.782 | ||
0.8 | 0.8 | 1.824 | 2.996 | 2.260 | 1.755 | ||
1.2 | 1.1 | 1.660 | 2.909 | 2.161 | 1.704 | ||
1.5 | 1.3 | 1.541 | 2.835 | 2.081 | 1.661 | ||
1.8 | 1.7 | 1.392 | 2.726 | 1.969 | 1.597 | ||
2.0 | 1.9 | 1.308 | 2.657 | 1.901 | 1.556 | ||
2.3 | 2.4 | 1.161 | 2.517 | 1.770 | 1.475 | ||
2.6 | 2.7 | 1.051 | 2.397 | 1.661 | 1.404 | ||
3.0 | 3.2 | 0.903 | 2.207 | 1.500 | 1.293 | ||
2.0 | 0.25 | 0.2 | 0.4 | 2.180 | 1.987 | 1.837 | 0.993 |
0.5 | 0.6 | 2.167 | 1.986 | 1.835 | 0.993 | ||
0.8 | 0.8 | 2.053 | 1.982 | 1.812 | 0.991 | ||
1.2 | 1.1 | 1.876 | 1.973 | 1.769 | 0.987 | ||
1.5 | 1.3 | 1.753 | 1.965 | 1.734 | 0.982 | ||
1.8 | 1.7 | 1.595 | 1.949 | 1.681 | 0.975 | ||
2.0 | 1.9 | 1.510 | 1.938 | 1.648 | 0.969 | ||
2.3 | 2.4 | 1.358 | 1.912 | 1.581 | 0.956 | ||
2.6 | 2.7 | 1.247 | 1.887 | 1.524 | 0.943 | ||
3.0 | 3.2 | 1.098 | 1.840 | 1.435 | 0.920 | ||
0.5 | 0.2 | 0.4 | 1.210 | 1.877 | 1.503 | 0.938 | |
0.5 | 0.6 | 1.279 | 1.895 | 1.541 | 0.947 | ||
0.8 | 0.8 | 1.210 | 1.877 | 1.503 | 0.938 | ||
1.2 | 1.1 | 1.080 | 1.834 | 1.423 | 0.917 | ||
1.5 | 1.3 | 0.987 | 1.794 | 1.358 | 0.897 | ||
1.8 | 1.7 | 0.861 | 1.725 | 1.258 | 0.862 | ||
2.0 | 1.9 | 0.795 | 1.679 | 1.199 | 0.840 | ||
2.3 | 2.4 | 0.672 | 1.574 | 1.077 | 0.787 | ||
2.6 | 2.7 | 0.587 | 1.482 | 0.982 | 0.741 | ||
3.0 | 3.2 | 0.470 | 1.323 | 0.836 | 0.661 |
ε | β | α | RE | HaCE | ArE | TsE |
1.5 | 0.25 | 0.2 | 7.001 | 3.311 | 2.709 | 1.94 |
0.5 | 7.075 | 3.315 | 2.716 | 1.942 | ||
0.8 | 7.156 | 3.319 | 2.724 | 1.944 | ||
1.2 | 7.274 | 3.324 | 2.735 | 1.947 | ||
1.5 | 7.372 | 3.329 | 2.743 | 1.95 | ||
1.8 | 7.476 | 3.333 | 2.752 | 1.952 | ||
2 | 7.55 | 3.336 | 2.758 | 1.954 | ||
2.3 | 7.667 | 3.34 | 2.767 | 1.957 | ||
2.6 | 7.792 | 3.345 | 2.777 | 1.959 | ||
3 | 7.971 | 3.351 | 2.79 | 1.963 | ||
0.4 | 0.2 | 6.441 | 3.278 | 2.65 | 1.92 | |
0.5 | 6.452 | 3.279 | 2.651 | 1.921 | ||
0.8 | 6.469 | 3.28 | 2.653 | 1.921 | ||
1.2 | 6.503 | 3.282 | 2.657 | 1.923 | ||
1.5 | 6.536 | 3.284 | 2.66 | 1.924 | ||
1.8 | 6.577 | 3.287 | 2.665 | 1.925 | ||
2 | 6.608 | 3.289 | 2.668 | 1.927 | ||
2.3 | 6.661 | 3.292 | 2.674 | 1.928 | ||
2.6 | 6.721 | 3.296 | 2.681 | 1.931 | ||
3 | 6.813 | 3.301 | 2.69 | 1.934 | ||
2.0 | 0.25 | 0.2 | 4.376 | 1.975 | 1.776 | 0.987 |
0.5 | 4.429 | 1.976 | 1.782 | 0.988 | ||
0.8 | 4.487 | 1.977 | 1.788 | 0.989 | ||
1.2 | 4.57 | 1.979 | 1.796 | 0.99 | ||
1.5 | 4.639 | 1.981 | 1.803 | 0.99 | ||
1.8 | 4.713 | 1.982 | 1.81 | 0.991 | ||
2 | 4.765 | 1.983 | 1.815 | 0.991 | ||
2.3 | 4.847 | 1.984 | 1.823 | 0.992 | ||
2.6 | 4.934 | 1.986 | 1.83 | 0.993 | ||
3 | 5.058 | 1.987 | 1.841 | 0.994 | ||
0.4 | 0.2 | 3.975 | 1.962 | 1.726 | 0.981 | |
0.5 | 3.987 | 1.963 | 1.728 | 0.981 | ||
0.8 | 4.003 | 1.963 | 1.73 | 0.982 | ||
1.2 | 4.031 | 1.965 | 1.734 | 0.982 | ||
1.5 | 4.058 | 1.965 | 1.737 | 0.983 | ||
1.8 | 4.09 | 1.967 | 1.741 | 0.983 | ||
2 | 4.114 | 1.967 | 1.744 | 0.984 | ||
2.3 | 4.154 | 1.969 | 1.749 | 0.984 | ||
2.6 | 4.199 | 1.97 | 1.755 | 0.985 | ||
3 | 4.267 | 1.972 | 1.763 | 0.986 |
n | r | Set1 (α = 0.5, β = 0.5, γ = 0.5) | |||||||
MLE | Bias | MSE | LB | UB | AL | CP | |||
50 | 70% | α | 0.4204 | 0.0796 | 0.0064 | 0.0019 | 0.839 | 0.8370 | 97.4% |
β | 0.7041 | 0.2041 | 0.0471 | 0.5036 | 0.9046 | 0.4010 | 96.9% | ||
γ | 0.4201 | 0.0799 | 0.0069 | 0.3023 | 0.5379 | 0.2356 | 96.0% | ||
80% | α | 0.4218 | 0.0782 | 0.0061 | 0.0191 | 0.8245 | 0.8053 | 94.8% | |
β | 0.6382 | 0.1382 | 0.0242 | 0.4508 | 0.8256 | 0.3748 | 95.8% | ||
γ | 0.4386 | 0.0614 | 0.0053 | 0.3282 | 0.5490 | 0.2208 | 97.1% | ||
100% | α | 0.4234 | 0.0766 | 0.0059 | 0.0357 | 0.8111 | 0.7754 | 95.4% | |
β | 0.5177 | 0.0177 | 0.0056 | 0.3661 | 0.6694 | 0.3033 | 95.5% | ||
γ | 0.5316 | 0.0316 | 0.0027 | 0.4303 | 0.6328 | 0.2025 | 96.0% | ||
100 | 70% | α | 0.4213 | 0.0787 | 0.0062 | 0.0844 | 0.7583 | 0.6740 | 96.2% |
β | 0.6750 | 0.1750 | 0.0312 | 0.5375 | 0.8125 | 0.2750 | 95.9% | ||
γ | 0.4237 | 0.0763 | 0.0065 | 0.3389 | 0.5084 | 0.1694 | 96.0% | ||
80% | α | 0.4230 | 0.0770 | 0.0061 | 0.2099 | 0.6360 | 0.4262 | 96.2% | |
β | 0.6099 | 0.1099 | 0.0127 | 0.4819 | 0.7379 | 0.2560 | 96.1% | ||
γ | 0.4487 | 0.0513 | 0.0033 | 0.3652 | 0.5321 | 0.1669 | 97.3% | ||
100% | α | 0.4238 | 0.0762 | 0.0058 | 0.2501 | 0.5975 | 0.3473 | 95.6% | |
β | 0.4683 | 0.0317 | 0.0027 | 0.3558 | 0.5807 | 0.2249 | 95.8% | ||
γ | 0.4967 | 0.0033 | 0.0025 | 0.4199 | 0.5734 | 0.1535 | 96.0% | ||
150 | 70% | α | 0.4217 | 0.0783 | 0.0061 | 0.2710 | 0.5725 | 0.3015 | 95.2% |
β | 0.6626 | 0.1626 | 0.0281 | 0.5577 | 0.7675 | 0.2097 | 95.6% | ||
γ | 0.4277 | 0.0723 | 0.0058 | 0.3571 | 0.4983 | 0.1412 | 97.3% | ||
80% | α | 0.4236 | 0.0764 | 0.0059 | 0.3005 | 0.5466 | 0.2461 | 95.7% | |
β | 0.5977 | 0.0977 | 0.0113 | 0.4957 | 0.6997 | 0.2040 | 96.2% | ||
γ | 0.4649 | 0.0351 | 0.0022 | 0.3972 | 0.5325 | 0.1353 | 97.0% | ||
100% | α | 0.4238 | 0.0762 | 0.0058 | 0.3010 | 0.5467 | 0.2457 | 95.6% | |
β | 0.4766 | 0.0234 | 0.0023 | 0.3784 | 0.5749 | 0.1965 | 96.4% | ||
γ | 0.5277 | 0.0277 | 0.0015 | 0.4659 | 0.5894 | 0.1236 | 96.9% | ||
200 | 70% | α | 0.4219 | 0.0781 | 0.0061 | 0.3154 | 0.5285 | 0.2132 | 96.1% |
β | 0.6592 | 0.1592 | 0.0268 | 0.5675 | 0.7510 | 0.1835 | 96.3% | ||
γ | 0.4375 | 0.0625 | 0.0046 | 0.3789 | 0.4962 | 0.1173 | 96.7% | ||
80% | α | 0.4239 | 0.0761 | 0.0058 | 0.3236 | 0.5242 | 0.2006 | 96.3% | |
β | 0.5912 | 0.0912 | 0.0099 | 0.5074 | 0.6750 | 0.1676 | 97.0% | ||
γ | 0.4667 | 0.0333 | 0.0020 | 0.4101 | 0.5233 | 0.1132 | 97.5% | ||
100% | α | 0.4240 | 0.0760 | 0.0058 | 0.3372 | 0.5109 | 0.1737 | 96.5% | |
β | 0.4905 | 0.0095 | 0.0009 | 0.4167 | 0.5642 | 0.1475 | 96.7% | ||
γ | 0.5035 | 0.0035 | 0.0006 | 0.4524 | 0.5546 | 0.1022 | 97.1% | ||
n | r | Set2 (α = 0.7, β = 0.5, γ = 0.25) | |||||||
MLE | Bias | MSE | LB | UB | AL | CP | |||
50 | 70% | α | 0.4206 | 0.2794 | 0.0782 | 0.0055 | 0.8358 | 0.8304 | 97.7% |
β | 0.7016 | 0.2016 | 0.0462 | 0.5015 | 0.9016 | 0.4001 | 96.5% | ||
γ | 0.2172 | 0.0328 | 0.0017 | 0.1568 | 0.2776 | 0.1208 | 100% | ||
80% | α | 0.4214 | 0.2786 | 0.0776 | 0.2084 | 0.6345 | 0.4261 | 97.9% | |
β | 0.6361 | 0.1361 | 0.0243 | 0.4490 | 0.8231 | 0.3741 | 98.5% | ||
γ | 0.2297 | 0.0203 | 0.0010 | 0.1719 | 0.2874 | 0.1155 | 100% | ||
100% | α | 0.4234 | 0.2766 | 0.0765 | 0.2497 | 0.5970 | 0.3473 | 98.3% | |
β | 0.5161 | 0.0161 | 0.0062 | 0.3649 | 0.6673 | 0.3025 | 97.6% | ||
γ | 0.2565 | 0.0065 | 0.0007 | 0.2044 | 0.3086 | 0.1043 | 100% | ||
100 | 70% | α | 0.4210 | 0.2790 | 0.0779 | 0.0208 | 0.8212 | 0.8004 | 96.4% |
β | 0.7006 | 0.2006 | 0.0431 | 0.5593 | 0.8419 | 0.2826 | 98.0% | ||
γ | 0.2141 | 0.0359 | 0.0016 | 0.1720 | 0.2562 | 0.0842 | 100% | ||
80% | α | 0.4215 | 0.2785 | 0.0776 | 0.2708 | 0.5721 | 0.3013 | 97.2% | |
β | 0.6357 | 0.1357 | 0.0214 | 0.5035 | 0.7680 | 0.2645 | 97.7% | ||
γ | 0.2270 | 0.0230 | 0.0008 | 0.1866 | 0.2673 | 0.0807 | 100% | ||
100% | α | 0.4234 | 0.2766 | 0.0765 | 0.3006 | 0.5462 | 0.2456 | 97.3% | |
β | 0.5158 | 0.0158 | 0.0033 | 0.4088 | 0.6227 | 0.2140 | 98.2% | ||
γ | 0.2540 | 0.0040 | 0.0003 | 0.2176 | 0.2905 | 0.0729 | 100% | ||
150 | 70% | α | 0.4212 | 0.2788 | 0.0778 | 0.0330 | 0.8093 | 0.7763 | 97.7% |
β | 0.7000 | 0.2000 | 0.0419 | 0.5847 | 0.8153 | 0.2306 | 97.7% | ||
γ | 0.2122 | 0.0378 | 0.0016 | 0.1781 | 0.2463 | 0.0682 | 100% | ||
80% | α | 0.4215 | 0.2785 | 0.0776 | 0.2985 | 0.5445 | 0.2460 | 98.8% | |
β | 0.6350 | 0.1350 | 0.0203 | 0.5271 | 0.7430 | 0.2159 | 98.1% | ||
γ | 0.2259 | 0.0241 | 0.0008 | 0.1931 | 0.2587 | 0.0656 | 96.0% | ||
100% | α | 0.4234 | 0.2766 | 0.0765 | 0.3232 | 0.5237 | 0.2005 | 97.2% | |
β | 0.5151 | 0.0151 | 0.0023 | 0.4278 | 0.6024 | 0.1746 | 97.0% | ||
γ | 0.2529 | 0.0029 | 0.0002 | 0.2232 | 0.2825 | 0.0593 | 95.4% | ||
200 | 70% | α | 0.4209 | 0.2791 | 0.0779 | 0.0849 | 0.7569 | 0.6720 | 100% |
β | 0.6981 | 0.1981 | 0.0405 | 0.5984 | 0.7978 | 0.1994 | 97.2% | ||
γ | 0.2118 | 0.0382 | 0.0016 | 0.1823 | 0.2412 | 0.0589 | 97.3% | ||
80% | α | 0.4215 | 0.2785 | 0.0776 | 0.3150 | 0.5280 | 0.2131 | 100% | |
β | 0.6331 | 0.1331 | 0.0191 | 0.5398 | 0.7265 | 0.1867 | 98.2% | ||
γ | 0.2256 | 0.0244 | 0.0007 | 0.1973 | 0.2540 | 0.0567 | 98.0% | ||
100% | α | 0.4234 | 0.2766 | 0.0765 | 0.3366 | 0.5103 | 0.1737 | 100% | |
β | 0.5136 | 0.0136 | 0.0016 | 0.4381 | 0.5891 | 0.1510 | 98.8% | ||
γ | 0.2523 | 0.0023 | 0.0002 | 0.2267 | 0.2779 | 0.0512 | 100% | ||
n | r | Set3 (α = 0.7, β = 0.7, γ = 0.5) | |||||||
MLE | Bias | MSE | LB | UB | AL | CP | |||
50 | 70% | α | 0.4178 | 0.2822 | 0.0797 | 0.1937 | 0.6419 | 0.4482 | 96.2% |
β | 0.8994 | 0.1994 | 0.0477 | 0.6064 | 1.1923 | 0.5859 | 95.9% | ||
γ | 0.6151 | 0.1151 | 0.0239 | 0.3763 | 0.8540 | 0.4776 | 95.0% | ||
80% | α | 0.4193 | 0.2807 | 0.0788 | 0.2255 | 0.6131 | 0.3875 | 95.9% | |
β | 0.8238 | 0.1238 | 0.0218 | 0.5471 | 1.1006 | 0.5535 | 95.9% | ||
γ | 0.5695 | 0.0695 | 0.0182 | 0.3447 | 0.7943 | 0.4496 | 96.7% | ||
100% | α | 0.4211 | 0.2789 | 0.0778 | 0.2480 | 0.5942 | 0.3461 | 96.8% | |
β | 0.7612 | 0.0612 | 0.0104 | 0.5012 | 1.0213 | 0.5201 | 97.0% | ||
γ | 0.5425 | 0.0425 | 0.0163 | 0.3395 | 0.7456 | 0.4061 | 95.0% | ||
100 | 70% | α | 0.4174 | 0.2826 | 0.0798 | 0.2439 | 0.5910 | 0.3470 | 95.0% |
β | 0.8787 | 0.1787 | 0.0353 | 0.6426 | 1.1148 | 0.4722 | 96.3% | ||
γ | 0.5696 | 0.0696 | 0.0201 | 0.3697 | 0.7696 | 0.3998 | 96.0% | ||
80% | α | 0.4191 | 0.2809 | 0.0789 | 0.2690 | 0.5691 | 0.3001 | 95.5% | |
β | 0.7802 | 0.0802 | 0.0134 | 0.5600 | 1.0004 | 0.4404 | 95.7% | ||
γ | 0.5479 | 0.0479 | 0.0091 | 0.3814 | 0.7144 | 0.3330 | 96.0% | ||
100% | α | 0.4206 | 0.2794 | 0.0781 | 0.2866 | 0.5546 | 0.2680 | 95.6% | |
β | 0.7146 | 0.0146 | 0.0068 | 0.5069 | 0.9223 | 0.4154 | 95.7% | ||
γ | 0.5597 | 0.0597 | 0.0074 | 0.4063 | 0.7131 | 0.3067 | 96.0% | ||
150 | 70% | α | 0.4176 | 0.2824 | 0.0798 | 0.2949 | 0.5403 | 0.2454 | 95.8% |
β | 0.8697 | 0.1697 | 0.0305 | 0.7056 | 1.0338 | 0.3282 | 96.2% | ||
γ | 0.5924 | 0.0924 | 0.0174 | 0.4449 | 0.7399 | 0.2950 | 97.1% | ||
80% | α | 0.4193 | 0.2807 | 0.0788 | 0.3131 | 0.5254 | 0.2122 | 96.2% | |
β | 0.8023 | 0.1023 | 0.0124 | 0.6492 | 0.9555 | 0.3063 | 96.1% | ||
γ | 0.5524 | 0.0524 | 0.0058 | 0.4332 | 0.6716 | 0.2384 | 97.0% | ||
100% | α | 0.4209 | 0.2791 | 0.0779 | 0.3261 | 0.5156 | 0.1895 | 95.8% | |
β | 0.7374 | 0.0374 | 0.0032 | 0.5932 | 0.8817 | 0.2885 | 96.3% | ||
γ | 0.5507 | 0.0507 | 0.0041 | 0.4447 | 0.6568 | 0.2121 | 96.9% | ||
200 | 70% | α | 0.4175 | 0.2825 | 0.0798 | 0.3173 | 0.5177 | 0.2004 | 96.1% |
β | 0.8406 | 0.1406 | 0.0265 | 0.7075 | 0.9736 | 0.2661 | 97.2% | ||
γ | 0.5683 | 0.0683 | 0.0069 | 0.4569 | 0.6798 | 0.2230 | 96.9% | ||
80% | α | 0.4193 | 0.2807 | 0.0788 | 0.3327 | 0.5059 | 0.1733 | 96.3% | |
β | 0.8043 | 0.1043 | 0.0123 | 0.6790 | 0.9295 | 0.2505 | 96.6% | ||
γ | 0.5502 | 0.0502 | 0.0041 | 0.4533 | 0.6471 | 0.1938 | 97.0% | ||
100% | α | 0.4209 | 0.2791 | 0.0779 | 0.3435 | 0.4983 | 0.1548 | 96.1% | |
β | 0.7411 | 0.0411 | 0.0030 | 0.6229 | 0.8592 | 0.2362 | 97.0% | ||
γ | 0.5463 | 0.0463 | 0.0041 | 0.4589 | 0.6338 | 0.1749 | 96.2% | ||
n | r | Set4 (α = 0.6, β = 0.3, γ = 0.5) | |||||||
MLE | Bias | MSE | LB | UB | AL | CP | |||
50 | 70% | α | 0.4197 | 0.1803 | 0.0325 | 0.2456 | 0.5939 | 0.3482 | 98.1% |
β | 0.5719 | 0.2719 | 0.0744 | 0.3742 | 0.7696 | 0.3954 | 97.0% | ||
γ | 0.2990 | 0.2010 | 0.0406 | 0.1769 | 0.4211 | 0.2442 | 98.0% | ||
80% | α | 0.4221 | 0.1779 | 0.0317 | 0.2716 | 0.5725 | 0.3009 | 98.4% | |
β | 0.4934 | 0.1934 | 0.0384 | 0.3126 | 0.6742 | 0.3616 | 97.4% | ||
γ | 0.3593 | 0.1407 | 0.0200 | 0.2383 | 0.4803 | 0.2421 | 98.2% | ||
100% | α | 0.4246 | 0.1754 | 0.0308 | 0.2903 | 0.5589 | 0.2686 | 97.9% | |
β | 0.4198 | 0.1198 | 0.0150 | 0.2576 | 0.5820 | 0.3244 | 97.4% | ||
γ | 0.4172 | 0.0828 | 0.0078 | 0.2968 | 0.5376 | 0.2408 | 98.4% | ||
100 | 70% | α | 0.4198 | 0.1802 | 0.0325 | 0.2966 | 0.5429 | 0.2463 | 97.2% |
β | 0.5674 | 0.2674 | 0.0717 | 0.4278 | 0.7069 | 0.2791 | 97.7% | ||
γ | 0.3137 | 0.1863 | 0.0351 | 0.2278 | 0.3995 | 0.1717 | 98.0% | ||
80% | α | 0.4222 | 0.1778 | 0.0316 | 0.3157 | 0.5286 | 0.2128 | 97.9% | |
β | 0.4857 | 0.1857 | 0.0350 | 0.3584 | 0.6130 | 0.2546 | 97.9% | ||
γ | 0.3669 | 0.1331 | 0.0179 | 0.2818 | 0.4519 | 0.1701 | 98.7% | ||
100% | α | 0.4248 | 0.1752 | 0.0307 | 0.3242 | 0.5253 | 0.2011 | 98.1% | |
β | 0.4082 | 0.1082 | 0.0125 | 0.2944 | 0.5221 | 0.2277 | 97.8% | ||
γ | 0.4171 | 0.0829 | 0.0074 | 0.3349 | 0.4993 | 0.1644 | 98.3% | ||
150 | 70% | α | 0.4198 | 0.1802 | 0.0325 | 0.3248 | 0.5148 | 0.1899 | 97.2% |
β | 0.5653 | 0.2653 | 0.0706 | 0.4518 | 0.6788 | 0.2270 | 98.3% | ||
γ | 0.3140 | 0.1860 | 0.0350 | 0.2421 | 0.3860 | 0.1439 | 99.3% | ||
80% | α | 0.4222 | 0.1778 | 0.0316 | 0.3353 | 0.5091 | 0.1738 | 97.7% | |
β | 0.4832 | 0.1832 | 0.0338 | 0.3797 | 0.5866 | 0.2069 | 98.2% | ||
γ | 0.3677 | 0.1323 | 0.0178 | 0.2996 | 0.4358 | 0.1363 | 99.0% | ||
100% | α | 0.4248 | 0.1752 | 0.0307 | 0.3377 | 0.5119 | 0.1742 | 97.3% | |
β | 0.4018 | 0.1018 | 0.0107 | 0.3032 | 0.5004 | 0.1972 | 97.9% | ||
γ | 0.4248 | 0.0752 | 0.0061 | 0.3608 | 0.4888 | 0.1280 | 99.7% | ||
200 | 70% | α | 0.4198 | 0.1802 | 0.0325 | 0.3423 | 0.4973 | 0.1551 | 99.0% |
β | 0.5650 | 0.2650 | 0.0705 | 0.4738 | 0.6561 | 0.1823 | 99.1% | ||
γ | 0.3256 | 0.1744 | 0.0309 | 0.2637 | 0.3875 | 0.1239 | 98.7% | ||
80% | α | 0.4222 | 0.1778 | 0.0316 | 0.3470 | 0.4975 | 0.1505 | 99.6% | |
β | 0.4781 | 0.1781 | 0.0321 | 0.3882 | 0.5679 | 0.1798 | 99.3% | ||
γ | 0.3733 | 0.1267 | 0.0173 | 0.3129 | 0.4336 | 0.1206 | 99.5% | ||
100% | α | 0.4250 | 0.1750 | 0.0306 | 0.3578 | 0.4921 | 0.1343 | 98.7% | |
β | 0.3904 | 0.0904 | 0.0088 | 0.3106 | 0.4703 | 0.1596 | 99.6% | ||
γ | 0.4262 | 0.0738 | 0.0060 | 0.3681 | 0.4843 | 0.1162 | 100% |
Rank | Country | % Global Reserves | Rank | Country | % Global Reserves |
1 | Russia | 19.9 | 23 | Ukraine | 0.6 |
2 | Iran | 17.1 | 24 | Malaysia | 0.5 |
3 | Qatar | 13.1 | 25 | Uzbekistan | 0.4 |
4 | Turkmenistan | 7.2 | 26 | Oman | 0.4 |
5 | United States | 6.7 | 27 | Vietnam | 0.3 |
6 | China | 4.5 | 28 | Israel | 0.3 |
7 | Venezuela | 3.3 | 29 | Argentina | 0.2 |
8 | Saudi Arabia | 3.2 | 30 | Pakistan | 0.2 |
9 | United Arab Emirates | 3.2 | 31 | Trinidad | 0.2 |
10 | Nigeria | 2.9 | 32 | Brazil | 0.2 |
11 | Iraq | 1.9 | 33 | Myanmar | 0.2 |
12 | Canada | 1.3 | 34 | United Kingdom | 0.1 |
13 | Australia | 1.3 | 35 | Thailand | 0.1 |
14 | Azerbaijan | 1.3 | 36 | Mexico | 0.1 |
15 | Algeria | 1.2 | 37 | Bangladesh | 0.1 |
16 | Kazakhstan | 1.2 | 38 | Netherlands | 0.1 |
17 | Egypt | 1.1 | 39 | Bolivia | 0.1 |
18 | Kuwait | 0.9 | 40 | Brunei | 0.1 |
19 | Norway | 0.8 | 41 | Peru | 0.1 |
20 | Libya | 0.8 | 42 | Syria | 0.1 |
21 | Indonesia | 0.7 | 43 | Yemen | 0.1 |
22 | India | 0.7 | 44 | Papua New Guinea | 0.1 |
Rank | Country | reserves2020 | Rank | Country | reserves2020 |
1 | Venezuela | 303.8 | 11 | Nigeria | 36.9 |
2 | Saudi Arabia | 297.5 | 12 | Kazakhstan | 30 |
3 | Canada | 168.1 | 13 | China | 26 |
4 | Iran | 157.8 | 14 | Qatar | 25.2 |
5 | Iraq | 145 | 15 | Algeria | 12.2 |
6 | Russia | 107.8 | 16 | Brazil | 11.9 |
7 | Kuwait | 101.5 | 17 | Norway | 7.9 |
8 | United Arab Emirates | 97.8 | 18 | Angola | 7.8 |
9 | United States | 68.8 | 19 | Azerbaijan | 7 |
10 | Libya | 48.4 | 20 | Mexico | 6.1 |
Rank | Country | Reserves of Gold | Rank | Country | Reserves of Gold | Rank | Country | Reserves of Gold |
1 | USA | 8.1335 | 35 | LBY | 0.1166 | 68 | CYP | 0.0139 |
2 | DEU | 3.3585 | 36 | GRC | 0.1141 | 69 | CUW | 0.0131 |
3 | IMF | 2.814 | 37 | ROK | 0.1045 | 70 | MUS | 0.0124 |
4 | ITA | 2.4518 | 38 | ROU | 0.1036 | 71 | IRL | 0.012 |
5 | FRA | 2.4365 | 39 | BIS | 0.102 | 72 | CZE | 0.0109 |
6 | RUS | 2.2985 | 40 | IRQ | 0.0964 | 73 | KGZ | 0.0102 |
7 | CHN | 1.9483 | 41 | HUN | 0.0945 | 74 | GHA | 0.0087 |
8 | CHE | 1.04 | 42 | AUS | 0.0798 | 75 | PRY | 0.0082 |
9 | JPN | 0.846 | 43 | KWT | 0.079 | 76 | NPL | 0.008 |
10 | IND | 0.7604 | 44 | IDN | 0.0786 | 77 | MNG | 0.0076 |
11 | NLD | 0.6125 | 45 | DNK | 0.0666 | 78 | MMR | 0.0073 |
12 | ECB | 0.5048 | 46 | PAK | 0.0647 | 79 | GTM | 0.0069 |
13 | TUR | 0.4311 | 47 | ARG | 0.0617 | 80 | MKD | 0.0069 |
14 | TAI | 0.4236 | 48 | ARE | 0.0553 | 81 | TUN | 0.0068 |
15 | PRT | 0.3826 | 49 | BLR | 0.0535 | 82 | LVA | 0.0067 |
16 | KAZ | 0.3681 | 50 | QAT | 0.0513 | 83 | LTU | 0.0058 |
17 | UZB | 0.3375 | 51 | KHM | 0.0504 | 84 | COL | 0.0047 |
18 | SAU | 0.3231 | 52 | FIN | 0.049 | 85 | BHR | 0.0047 |
19 | GBR | 0.3103 | 53 | JOR | 0.0435 | 86 | BRN | 0.0046 |
20 | LBN | 0.2868 | 54 | BOL | 0.0425 | 87 | GIN | 0.0042 |
21 | ESP | 0.2816 | 55 | BGR | 0.0408 | 88 | MOZ | 0.0039 |
22 | AUT | 0.28 | 56 | MYS | 0.0389 | 89 | SVN | 0.0032 |
23 | THA | 0.2442 | 57 | SRB | 0.0378 | 90 | ABW | 0.0031 |
24 | POL | 0.2287 | 58 | WAEMU | 0.0365 | 91 | BIH | 0.003 |
25 | BEL | 0.2274 | 59 | PER | 0.0347 | 92 | ALB | 0.0028 |
26 | DZA | 0.1736 | 60 | SVK | 0.0317 | 93 | LUX | 0.0022 |
27 | VEN | 0.1612 | 61 | UKR | 0.0271 | 94 | HKG | 0.0021 |
28 | PHL | 0.1563 | 62 | SYR | 0.0258 | 95 | ISL | 0.002 |
29 | SGP | 0.1537 | 63 | MAR | 0.0221 | 96 | TTO | 0.0019 |
30 | BRA | 0.1297 | 64 | ECU | 0.0219 | 97 | HTI | 0.0018 |
31 | SWE | 0.1257 | 65 | AFG | 0.0219 | 98 | YEM | 0.0016 |
32 | ZAF | 0.1254 | 66 | NGA | 0.0215 | 99 | SUR | 0.0015 |
33 | EGY | 0.125 | 67 | BGD | 0.014 | 100 | SLV | 0.0014 |
34 | MEX | 0.1199 |
n | Mean | Median | Skewness | Kurtosis | Range | Min | Max | Sum | |
Data1 | 20 | 1.900 | 1.700 | 1.860 | 4.185 | 3.000 | 1.100 | 4.100 | 38.000 |
Data2 | 44 | 2.248 | 0.650 | 2.990 | 8.864 | 19.800 | 0.100 | 19.900 | 98.900 |
Data3 | 20 | 83.375 | 42.650 | 1.430 | 1.420 | 297.700 | 6.100 | 303.800 | 1667.500 |
Data4 | 100 | 0.347 | 0.050 | 5.590 | 38.257 | 8.130 | 0.001 | 8.133 | 34.676 |
Distributions | MLE and SE | ||||
α | β | γ | λ | θ | |
LBTLoW | 8.648 | 3.074 | 0.042 | ||
(3.545) | (0.474) | (0.025) | |||
ETGR | 0.103 | 0.692 | 23.539 | -0.342 | |
(0.436) | (0.086) | (105.137) | (1.971) | ||
BW | 0.831 | 0.613 | 29.947 | 11.632 | |
(0.954) | (0.340) | (40.414) | (21.900) | ||
T-Li | 0.665 | 0.359 | |||
(0.332) | (0.048) | ||||
McLL | 0.881 | 2.070 | 1.926 | 19.225 | 32.033 |
(0.109) | (3.693) | (5.165) | (22.341) | (43.081) | |
NMW | 0.121 | 2.784 | 2.787 | 0.003 | 0.008 |
(0.056) | (20.370) | (0.428) | (0.025) | (0.002) | |
W | 0.122 | 2.787 | |||
(0.056) | (0.427) |
Distributions | AIC | CAIC | BIC | HQIC | KS | PV |
LBTLoW | 40.140 | 41.640 | 38.040 | 40.720 | 0.146 | 0.790 |
ETGR | 44.860 | 47.520 | 42.060 | 45.630 | 0.190 | 0.465 |
BW | 42.400 | 45.060 | 39.600 | 43.170 | 0.160 | 0.683 |
T-Li | 65.730 | 66.440 | 64.330 | 66.120 | 0.380 | 0.006 |
McLL | 43.850 | 48.140 | 40.360 | 44.830 | 0.147 | 0.734 |
NMW | 51.170 | 55.460 | 47.680 | 52.150 | 0.190 | 0.501 |
W | 45.170 | 45.880 | 43.780 | 45.560 | 0.180 | 0.509 |
Distributions | MLE and SE | ||||
α | β | γ | λ | θ | |
LBTLoW | 6.268 | 0.623 | 0.484 | ||
(2.631) | (0.066) | (0.210) | |||
ETGR | 0.055 | 0.071 | 8.773 | 0.947 | |
(0.027) | (0.029) | (7.043) | (0.081) | ||
TCWG | 34.076 | 0.802 | 0.005 | 1.12 | |
(81.023) | (0.021) | (0.013) | (0.285) | ||
EKW | 0.221 | 400.298 | 5.215 | 1 | 3.823 |
(0.038) | (718.99) | (0.649) | (0.004) | (3.036) | |
TMW | 0.851 | 1.159 | -0.554 | 0.519 | |
(0.163) | (1.026) | (0.985) | (0.379) | ||
BW | 2.861 | 0.075 | 78.550 | 42.576 | |
(69.095) | (0.090) | (167.320) | (187.300) | ||
T-Li | 0.604 | 0.671 | |||
(0.155) | (0.074) | ||||
McLL | 0.181 | 1.565 | 1.286 | 21.234 | 28.124 |
(0.193) | (9.254) | (5.432) | (34.701) | (45.757) | |
NMW | 6.8 x 10−8 | 0.680 | 0.223 | 0.015 | 0.806 |
(0.623) | (0.110) | (617.48) | (0.015) | (0.418) | |
W | 0.799 | 0.621 | |||
(0.136) | (0.068) |
Distributions | AIC | CAIC | BIC | HQIC | KS | PV |
LBTLoW | 132.210 | 132.810 | 131.140 | 134.200 | 0.130 | 0.425 |
ETGR | 143.470 | 144.490 | 142.040 | 146.110 | 0.180 | 0.118 |
TCWG | 137.690 | 138.710 | 136.260 | 140.330 | 0.150 | 0.251 |
EKW | 133.890 | 135.470 | 132.110 | 140.330 | 0.140 | 0.355 |
TMW | 140.900 | 142.480 | 139.120 | 144.210 | 0.150 | 0.276 |
BW | 133.180 | 134.200 | 131.750 | 135.820 | 0.130 | 0.408 |
T-Li | 174.360 | 174.660 | 173.650 | 175.690 | 0.200 | 0.057 |
McLL | 134.830 | 136.410 | 133.040 | 138.130 | 0.130 | 0.419 |
NMW | 143.780 | 145.360 | 142.000 | 147.090 | 0.160 | 0.243 |
W | 138.650 | 138.940 | 137.940 | 139.970 | 0.170 | 0.139 |
Distributions | MLE and SE | ||||
α | β | γ | λ | θ | |
LBTLoW | 2.515 | 0.756 | 0.040 | ||
(3.877) | (0.166) | (0.048) | |||
WEIW | 0.909 | 0.871 | 7.225 | ||
(106700) | (0.152) | (384700) | |||
TMW | 0.998 | 0.459 | -0.443 | 0.202 | |
(0.081) | (18.537) | (18.537) | (0.769) | ||
T-Li | 0.021 | 0.384 | |||
(0.345) | (0.004) | ||||
McLL | 0.208 | 93.978 | 1.279 | 24.759 | 32.815 |
(0.499) | (1721) | (19.272) | (142.806) | (161.611) | |
NMW | 10.7 x 10−8 | 0.930 | 0.859 | 7.46 x 10−8 | 0.017 |
(0.001) | (0.250) | (1.216) | (0.002) | (0.017) | |
EKW | 0.167 | 261.64 | 45.725 | 1.201 | 2.138 |
(0.079) | (1709) | (219.725) | (0.741) | (7.209) |
Distributions | AIC | CAIC | BIC | HQIC | KS | PV |
LBTLoW | 221.690 | 223.190 | 219.600 | 222.280 | 0.135 | 0.857 |
WEIW | 223.400 | 224.900 | 221.300 | 223.980 | 0.157 | 0.708 |
TMW | 226.410 | 230.690 | 222.910 | 227.380 | 0.153 | 0.734 |
T-Li | 230.480 | 231.180 | 229.080 | 230.870 | 0.265 | 0.120 |
McLL | 225.990 | 230.280 | 222.500 | 222.500 | 0.146 | 0.789 |
NMW | 226.570 | 230.860 | 223.080 | 227.540 | 0.140 | 0.826 |
EKW | 226.290 | 230.570 | 222.790 | 229.300 | 0.148 | 0.776 |
Distributions | MLE and SE | ||||
α | β | γ | λ | θ | |
LBTLoW | 6.498 | 0.482 | 1.490 | ||
(2.301) | (0.034) | (0.573) | |||
EKW | 0.221 | 1096 | 4.424 | 1 | 1.717 |
(0.030) | (1376) | (1.817) | (0.001) | (0.901) | |
TMW | 0.596 | 2.612 | 0.588 | -0.523 | |
(0.057) | (0.689) | (0.256) | (0.346) | ||
BW | 134.832 | 0.073 | 49.149 | 22.930 | |
(956.622) | (0.060) | (74.497) | (46.500) | ||
WEIW | 27.512 | 0.549 | 0.094 | ||
(3272000) | (0.042) | (856.967) | |||
W | 2.648 | 0.489 | |||
(0.281) | (0.035) |
Distributions | AIC | CAIC | BIC | HQIC | KS | PV |
LBTLoW | –170.510 | –170.260 | –170.510 | –167.350 | 0.070 | 0.704 |
ETGR | –167.710 | –167.070 | –167.710 | –157.710 | 0.078 | 0.584 |
BW | –158.180 | –157.540 | –158.180 | –152.910 | 0.077 | 0.593 |
T-Li | –170.220 | –169.800 | –170.220 | –166.010 | 0.071 | 0.703 |
McLL | –170.200 | –169.950 | –170.200 | –167.040 | 0.091 | 0.383 |
W | –157.390 | –157.260 | –157.390 | –155.280 | 0.100 | 0.269 |