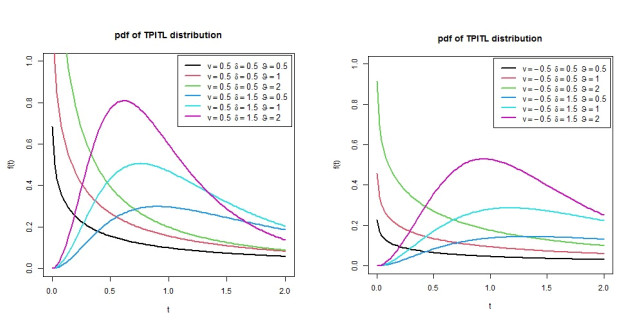
The quadratic rank transmutation map is used in this article to suggest a novel extension of the power inverted Topp–Leone distribution. The newly generated distribution is known as the transmuted power inverted Topp–Leone (TPITL) distribution. The power inverted Topp–Leone and the inverted Topp–Leone are included in the recommended distribution as specific models. Aspects of the offered model, including the quantile function, moments and incomplete moments, stochastic ordering, and various uncertainty measures, are all discussed. Plans for acceptance sampling are created for the TPITL model with the assumption that the life test will end at a specific time. The median lifetime of the TPITL distribution with the chosen variables is the truncation time. The smallest sample size is required to obtain the stated life test under a certain consumer's risk. Five conventional estimation techniques, including maximum likelihood, least squares, weighted least squares, maximum product of spacing, and Cramer-von Mises, are used to assess the characteristics of TPITL distribution. A rigorous Monte Carlo simulation study is used to evaluate the effectiveness of these estimators. To determine how well the most recent model handled data modeling, we tested it on a range of datasets. The simulation results demonstrated that, in most cases, the maximum likelihood estimates had the smallest mean squared errors among all other estimates. In some cases, the Cramer-von Mises estimates performed better than others. Finally, we observed that precision measures decrease for all estimation techniques when the sample size increases, indicating that all estimation approaches are consistent. Through two real data analyses, the suggested model's validity and adaptability are contrasted with those of other models, including the power inverted Topp–Leone, log-normal, Weibull, generalized exponential, generalized inverse exponential, inverse Weibull, inverse gamma, and extended inverse exponential distributions.
Citation: Said G. Nassr, Amal S. Hassan, Rehab Alsultan, Ahmed R. El-Saeed. Acceptance sampling plans for the three-parameter inverted Topp–Leone model[J]. Mathematical Biosciences and Engineering, 2022, 19(12): 13628-13659. doi: 10.3934/mbe.2022636
[1] | Ibrahim Alkhairy . Classical and Bayesian inference for the discrete Poisson Ramos-Louzada distribution with application to COVID-19 data. Mathematical Biosciences and Engineering, 2023, 20(8): 14061-14080. doi: 10.3934/mbe.2023628 |
[2] | Sue Wang, Chaohong Zhou, Saleem Riaz, Xuanchen Guo, Haider Zaman, Alsharef Mohammad, Ahmad Aziz Al-Ahmadi, Yasser Mohammed Alharbi, NasimUllah . Adaptive fuzzy-based stability control and series impedance correction for the grid-tied inverter. Mathematical Biosciences and Engineering, 2023, 20(2): 1599-1616. doi: 10.3934/mbe.2023073 |
[3] | Ghadah Alomani, Amer I. Al-Omari . Single acceptance sampling plans based on truncated lifetime tests for two-parameter Xgamma distribution with real data application. Mathematical Biosciences and Engineering, 2022, 19(12): 13321-13336. doi: 10.3934/mbe.2022624 |
[4] | Manal M. Yousef, Rehab Alsultan, Said G. Nassr . Parametric inference on partially accelerated life testing for the inverted Kumaraswamy distribution based on Type-II progressive censoring data. Mathematical Biosciences and Engineering, 2023, 20(2): 1674-1694. doi: 10.3934/mbe.2023076 |
[5] | Erin N. Bodine, Connor Cook, Mikayla Shorten . The potential impact of a prophylactic vaccine for Ebola in Sierra Leone. Mathematical Biosciences and Engineering, 2018, 15(2): 337-359. doi: 10.3934/mbe.2018015 |
[6] | Kaiwei Jia, Lujun Li . The moderate level of digital transformation: from the perspective of green total factor productivity. Mathematical Biosciences and Engineering, 2024, 21(2): 2254-2281. doi: 10.3934/mbe.2024099 |
[7] | Amal S. Hassan, Najwan Alsadat, Christophe Chesneau, Ahmed W. Shawki . A novel weighted family of probability distributions with applications to world natural gas, oil, and gold reserves. Mathematical Biosciences and Engineering, 2023, 20(11): 19871-19911. doi: 10.3934/mbe.2023880 |
[8] | Katarzyna Pichór, Ryszard Rudnicki . Stochastic models of population growth. Mathematical Biosciences and Engineering, 2025, 22(1): 1-22. doi: 10.3934/mbe.2025001 |
[9] | Mondal Hasan Zahid, Christopher M. Kribs . Ebola: Impact of hospital's admission policy in an overwhelmed scenario. Mathematical Biosciences and Engineering, 2018, 15(6): 1387-1399. doi: 10.3934/mbe.2018063 |
[10] | An Ma, Shuting Lyu, Qimin Zhang . Stationary distribution and optimal control of a stochastic population model in a polluted environment. Mathematical Biosciences and Engineering, 2022, 19(11): 11260-11280. doi: 10.3934/mbe.2022525 |
The quadratic rank transmutation map is used in this article to suggest a novel extension of the power inverted Topp–Leone distribution. The newly generated distribution is known as the transmuted power inverted Topp–Leone (TPITL) distribution. The power inverted Topp–Leone and the inverted Topp–Leone are included in the recommended distribution as specific models. Aspects of the offered model, including the quantile function, moments and incomplete moments, stochastic ordering, and various uncertainty measures, are all discussed. Plans for acceptance sampling are created for the TPITL model with the assumption that the life test will end at a specific time. The median lifetime of the TPITL distribution with the chosen variables is the truncation time. The smallest sample size is required to obtain the stated life test under a certain consumer's risk. Five conventional estimation techniques, including maximum likelihood, least squares, weighted least squares, maximum product of spacing, and Cramer-von Mises, are used to assess the characteristics of TPITL distribution. A rigorous Monte Carlo simulation study is used to evaluate the effectiveness of these estimators. To determine how well the most recent model handled data modeling, we tested it on a range of datasets. The simulation results demonstrated that, in most cases, the maximum likelihood estimates had the smallest mean squared errors among all other estimates. In some cases, the Cramer-von Mises estimates performed better than others. Finally, we observed that precision measures decrease for all estimation techniques when the sample size increases, indicating that all estimation approaches are consistent. Through two real data analyses, the suggested model's validity and adaptability are contrasted with those of other models, including the power inverted Topp–Leone, log-normal, Weibull, generalized exponential, generalized inverse exponential, inverse Weibull, inverse gamma, and extended inverse exponential distributions.
Symbols: h(t;Θ) : PDF; H(t;Θ) : CDF; S(t;Θ) : SF; λ(t;Θ) : HRF; ψ(τ) : AE; γ(τ) : H-CE; M(τ) : RE; ρ(τ) : Ts; L(p) : AP; Q(u) : QF; GM(Θ) : GM; −2logL : –2log likelihood ;p∗ : Consumer's risk
Abbreviations: AIC: Akaike information criterion; ASP: Acceptance sampling plan; AE: Arimoto entropy; AP: Acceptance probability of the lot as function of the failure probability; BIC: Bayesian information criterion; EIE: Extended inverse exponential; Ge-Ex: Generalized exponential; GIE: Generalized inverse exponential; in-Ga: inverse gamma; in-We: Inverse Weibull; K–S: Kolmogorov–Smirnov; CAIC: Corrected AIC; CvMEs: Cramer-von Mises estimators; CDF: Cumulative distribution function; HRF: Hazard rate function; H-CE: Havrda and Charvat entropy; ITL: Inverted Topp–Leone; Ku: Kurtosis; ; LSEs: Least squares estimators; MPSEs: Maximum product spacing estimators; PITL: Power ITL; PDF: Probability density function; PP: Probability-probability; QF: Quantile function; QRTM: Quadratic rank transmutation map; RE: Rényi entropy; Sw: Skewness; SE: Standard error; MSE: Mean squared error; med: Median lifetime; SO: Stochastic ordering
The validity of statistical investigations of real-world occurrences is determined by the appropriateness of the distributions used as models. Although well-known distribution families are frequently employed to simulate many events, their modeling capabilities may not always meet expectations. To address this issue, several scholars have presented new distribution families for ideally representing real-world events by employing generalizations of existing families or novel distribution generating procedures in the last two decades. In addition, several strategies for creating a new distribution have been described in the literature. For example, reference [1] devised a mechanism called the quadratic rank transmutation map (QRTM). This technique generates a transmuted distribution, as the name implies. In addition to having the same characteristics as baseline distributions, transmuted distributions are always more flexible.
Reference [1] defined the transmuted class with the following cumulative distribution function (CDF) and probability density function (PDF):
FTC(t)=G(t)[1+υ−υG(t)],|υ|≤1,t∈R, | (1) |
fTC(t)=g(t)[1+υ−2υG(t)], | (2) |
where υ is a transmuted parameter known as the transmutation parameter. The transmuted density (2) is a mixture of the baseline density and the exponentiated-G density with power parameter equal two for υ=0, the transmuted CDF FTC(t) and the baseline CDF, G(t) are identical. For more information about the generalization of the probability distributions via the QRTM method, the reader can refer to references [2,3,4,5,6,7,8]. Other generalization methods can be found in [9,10,11,12,13,14,15].
Reference [16] recently presented the inverted Topp–Leone (ITL) distribution as a probability distribution with only one shape parameter. The PDF and CDF of the ITL distribution are defined as follows:
G(t)=1−(1+2t)ϑ(1+t)−2ϑ;t,ϑ>0, |
g(t)=2ϑt(1+t)−2ϑ−1(1+2t)ϑ−1;t,ϑ>0. |
Many researchers have investigated the ITL distribution extensions and generalizations to increase flexibility in modeling a wide range of data. Reference [17] presented an "alpha power ITL distribution, " a new form of ITL model with an extra parameter based on the alpha power-G family. Reference [18] created a three-parameter ITL distribution for a particular case from the Kumaraswamy-G family. Reference [19] presented the two-parameter half-logistic ITL distribution, and parameter estimators were investigated under ranked samples. Reference [20] investigated parameter estimators using various estimating methods for the modified Kies ITL distribution. Reference [21] proposed a new two-parameter ITL model via the odd Weibull–G family. Our focus here is on a power ITL (PITL) distribution prepared in [22] with an extra shape parameter. The PDF and CDF of the PITL distribution are defined as follows:
G1(t)=1−(1+2tδ)ϑ(1+tδ)−2ϑ;t,ϑ,δ>0, | (3) |
g1(t)=2ϑδt2δ−1(1+tδ)−2ϑ−1(1+2tδ)ϑ−1;t,ϑ,δ>0. |
Acceptance sampling is used by industries worldwide to ensure the quality of incoming and outgoing goods, using statistical principles to create a plan for accepting or rejecting these goods. This process is called an acceptance sampling plan (ASP). It is one of the oldest quality assurance procedures, and it involves inspecting and deciding on quantities of goods to be accepted. An example of ASP in action is shown below:
● Required: A corporation gets a product shipment from a vendor. This product is frequently a component or raw material utilized during manufacturing.
● Sampling: A sample of the lot is obtained, and the relevant quality characteristics of the units in the sample are examined.
● Decision: Based on the information provided by the presented sample, a decision is made about whether to accept or reject the lot.
1) For accepted samples: Accepted lots are placed into production.
2) For rejected samples: Rejected lots may be transferred from the seller or subjected to further lot disposition actions.
Parameter estimation is essential to the study of any probability distribution. Maximum likelihood (ML) estimation is frequently used to estimate any model's parameters due to its desirable qualities. ML estimators are asymptotically consistent, unbiased, and impartial [23]. Over time, other methods for estimating distributions have emerged, including least squares (LS) and weighted LS (WLS) [24], maximum product of spacing (MPS) [25], and Cramer-von Mises (CvM) [26].
In this study, we established a new generalization method of PITL distribution to enhance the overall flexibility of the PITL model by employing QRTM. The newly created distribution is termed "transmuted PITL (TPITL) distribution". We are motivated to introduce the TPITL distribution due to the following reasons:
(i) This modification significantly impacts important distributional features, such as skewness, kurtosis, mean, and variance.
(ii) The related density and hazard rate functions have a large panel of monotonic and nonmonotonic forms, making them appropriate for data fitting and other applications.
(iii) It includes the PITL and ITL distributions as sub-models.
(iv) Important aspects of the presented model are explored, including quantile function, moments and incomplete moments, stochastic ordering, and some uncertainty measures.
(v) We created a sampling strategy, extracted its operational characteristic function, and gave it a decision rule.
(vi) The TPITL distribution parameters are evaluated using five traditional estimation methodologies.
(vii) An inclusive simulation study was conducted to determine if estimators based on accuracy criteria were successful.
(viii) The proposed model's validity and flexibility are compared with those of existing models, including the PITL, log-normal, Weibull, generalized inverse exponential, inverse Weibull, inverse gamma, generalized exponential, and extended inverse exponential distributions via two real data analyses.
The remaining paper is laid out as follows: Section 2 describes the CDF, survival function, and hazard rate function (HRF) of the TPITL distribution. In Section 3, the statistical features of the TPITL distribution are examined, and various helpful representations, measurements, and functions are derived. Section 4 discusses the suggested ASP's design under a truncated life test. In Section 5, the parametric estimate of the TPITL distribution is examined using some classical techniques. A simulation analysis ensuring its numerical performance is presented in Section 6. Section 7 discusses two real-world datasets to demonstrate how beneficial the TPITL model may be. Lastly, Section 8 contains a few findings.
We constructed the TPITL distribution using the CDF (3) of the PITL model as a baseline distribution in (1). The CDF of the TPITL distribution is given by:
H(t;Θ)=[1−{(1+2tδ)ϑ(1+tδ)2ϑ}][1+υ{(1+2tδ)ϑ(1+tδ)2ϑ}],|υ|≤1,t>0, | (4) |
where ϑ>0 and δ>0 are two shape parameters, υ is the transmuted parameter, and Θ≡(υ,ϑ,δ) is the set of parameters. A random variable with CDF (4) will be denoted by T TPITL(Θ). The PDF associated with (4) is given as follows:
h(t;Θ)=2ϑδt2δ−1(1+tδ)−2ϑ−1(1+2tδ)ϑ−1[1−υ+2υ{(1+2tδ)ϑ(1+tδ)2ϑ}],t>0. | (5) |
For υ=0, the PDF (5) reduces to PITL distribution (Abushal et al. [22]).
For υ=0, ϑ=1, the PDF (5) gives the ITL distribution (Hassen et al. [16]).
The form of the TPITL distribution is controlled by two shape parameters υ, and ϑ, similar to the PITL distribution. The parameter υ adds to the distribution's flexibility in addition to its role in influencing the behavior of the distribution. The survival function and HRF of the TPITL distribution are derived, respectively, as follows:
S(t;Θ)=1−{1−K(δ,ϑ)}[1+υ{K(δ,ϑ)}], |
λ(t;Θ)=2ϑδt2δ−1(1+tδ)−2ϑ−1(1+2tδ)ϑ−1[1−υ+2υ{K(δ,ϑ)}]1−{1−K(δ,ϑ)}[1+υ{K(δ,ϑ)}], |
where K(δ,ϑ)=(1+2tδ)ϑ(1+tδ)−2ϑ. For the given parameter values, the PDF and HRF plots are illustrated in Figures 1 and 2, respectively.
Reversed J-shaped, unimodal, nearly symmetrical, and positively skewed are all possible shapes for the PDF of the TPITL model. Additionally, the HRF of the TPITL model has a growing, decreasing, inverted J-shaped, upside-down form. These figures indicate the flexibility of the TPITL distribution to model right-skewed data as well as data with decreasing and upside-down bathtub shapes.
Furthermore, for estimation and simulation, quantiles are necessary. Inverting Equation (4) yields the quantile function, say, Q(u)=H−1(u), where u∈(0,1), as follows:
Q(u)={−1+(1−A)−1/ϑ+√(1−(1−A)−1/ϑ)2−1+(1−A)−1/ϑ}1/δ,A=((1+υ)−√(1+υ)2−4uυ2υ). | (6) |
Setting u=0.5 in (6), we obtain the median.
Herein, we present ordinary and incomplete moments, stochastic ordering (SO), and some uncertainty measures of the TPITL distribution.
Here, we obtain the mth moment, incomplete moments of the TPITL distribution. The mth moment for the TPITL model is derived as follows:
´μm=∫∞02ϑδtm+2δ−1(1+tδ)−2ϑ−1(1+2tδ)ϑ−1[1−υ+2υ{(1+2tδ)ϑ(1+tδ)2ϑ}]dt=(1−υ)I1+υI2, |
where
I1=∫∞02ϑδtm+2δ−1(1+tδ)−2ϑ−1(1+2tδ)ϑ−1dt, |
I2=∫∞04ϑδtm+2δ−1(1+tδ)−4ϑ−1(1+2tδ)2ϑ−1dt. |
Using the following generalized binomial expansion, for ϑ>0 is a real non-integer and |z|<1,
(1+z)ϑ−1=∑∞j=0(ϑ−1j)zj, |
in I1 and I2, then, we have
I1=∑∞j=02ϑ(ϑ−1j)B(mδ+j+2,ϑ−mδ),I2=∑∞i=04ϑ(2ϑ−1i)B(mδ+i+2,2ϑ−mδ). |
where B(.,.) is the beta function. Hence, the mth moment of the TPITL distribution can be written as follows:
´μm=(1−υ)∑∞j=02ϑ(ϑ−1j)B(mδ+j+2,ϑ−mδ)+υ∑∞i=04ϑ(2ϑ−1i)B(mδ+i+2,2ϑ−mδ). | (7) |
The first four moments about zero are obtained after putting m=1,2,3, and 4 in (7). The mth central moment (μm) of the TPITL distribution is given by:
μm=E(T−´μ1)m=∑mu=0(−1)u(mu)(´μ1)u´μm−u. |
Numerical values of the first four moments, variance (σ2), skewness (Sw), and kurtosis (Ku) of the TPITL distribution for ϑ=0.5, and at some choice values of parameters are displayed in Table 1.
´μm | δ=7 υ=−0.3 |
δ=7 υ=0.3 |
δ=9 υ=0.5 |
δ=9 υ=−0.5 |
δ=7 υ=−0.5 |
δ=7 υ=0.5 |
´μ1 | 1.567 | 1.389 | 1.236 | 1.439 | 1.626 | 1.33 |
´μ2 | 3.056 | 2.327 | 1.657 | 2.318 | 3.299 | 2.084 |
´μ3 | 11.183 | 6.932 | 2.603 | 4.584 | 12.6 | 5.515 |
´μ4 | 392.029 | 212.752 | 6.807 | 16.324 | 451.896 | 152.346 |
σ2 | 0.601 | 0.397 | 0.131 | 0.247 | 0.655 | 0.315 |
Sw | 9.669 | 10.388 | 4.904 | 4.377 | 9.618 | 10.8 |
Ku | 964.521 | 1206 | 124.816 | 22.875 | 934.164 | 1371 |
According to Table 1, all moments' values, variance, and skewness measures of the TPITL model are less for negative values of transmuted parameters than for positive ones. While the TPITL's kurtosis values was the highest for positive values of υ. The TPITL distribution is right-skewed according to the values of the skewness measure (Figure 1). The TPITL distribution is leptokurtic according to the values of the kurtosis measure (Figure 2).
Moreover, the mth incomplete moment, say ζm(z) of the TPITL distribution, is obtained as follows:
ζm(z)=2ϑδ(1−υ)z∫0tm+2δ−1(1+tδ)−2ϑ−1(1+2tδ)ϑ−1dt+4υϑδz∫0tm+2δ−1(1+tδ)−4ϑ−1(1+2tδ)2ϑ−1dt. |
After simplification, the mth incomplete moment of the TPITL distribution is obtained as:
ζm(z)=2ϑ(1−υ)∑∞j=0(ϑ−1j)B(mδ+j+2,ϑ−mδ,zδ1+zδ)+4ϑυ∑∞i=0(2ϑ−1i)B(mδ+i+2,2ϑ−mδ,zδ1+zδ), |
where B(.,.,x) is the incomplete beta function. Inequality measures, including the Bonferroni and Lorenz curves, are commonly utilized in various fields. These are the first incomplete moments' principal applications. The Lorenz and Bonferroni curves are formed, respectively, as follows:
Q=(1−υ)∑∞j=0(ϑ−1j)B(1δ+j+2,ϑ−1δ)+2υ∑∞i=0(2ϑ−1i)B(1δ+i+2,2ϑ−1δ)(1−υ)∑∞j=0(ϑ−1j)B(1δ+j+2,ϑ−1δ,zδ1+zδ)+2υ∑∞i=0(2ϑ−1i)B(1δ+i+2,2ϑ−1δ,zδ1+zδ), |
C=Q{[1−((1+2zδ)ϑ(1+zδ)2ϑ)][1+υ((1+2zδ)ϑ(1+zδ)2ϑ)]}−1. |
In reliability theory and other fields, SO is a well-studied notion in probability distributions, and it is used to assess the performance of random variables. Let Ti has the TPITL distribution with parameters Θi=(ϑi,δi,υi)i=1,2. Let Hi(t;Θi) indicates Ti 's CDF and hi(t;Θi) represents Ti 's PDF.
If h1(t;Θ1)/h2(t;Θ2), is a decreasing function ∀ t, then T1 is said to be stochastically less than T2 (represented by T1≤srT2) in terms of likelihood ratio order.
Let T1 TPITL(ϑ1,δ1,υ1) and T2 ITL(ϑ2,δ2,υ2) then the likelihood ratio ordering is
h1(t;Θ1)h2(t;Θ2)=ϑ1δ1t2δ1−1(1+tδ1)−2ϑ1−1(1+2tδ1)ϑ1−1[1−υ1+2υ1K(δ1,ϑ1)]ϑ2δ2t2δ2−1(1+tδ2)−2ϑ2−1(1+2tδ2)ϑ2−1[1−υ2+2υ2K(δ2,ϑ2)], |
ddtlogh1(t;Θ1)h2(t;Θ2)=2δ1−2δ2t−(2ϑ1+1)δ1tδ1−11+tδ1+(2ϑ2+1)δ2tδ2−11+tδ2+2(ϑ1−1)δ1tδ1−11+2tδ1 |
−2(ϑ2−1)δ2tδ2−11+2tδ2+2υ1[dK(δ1,ϑ1)/dt][1−υ1+2υ1K(δ1,ϑ1)]−2υ2[dK(δ2,ϑ2)/dt][1−υ2+2υ2K(δ2,ϑ2)], |
where, K(δi,ϑi)=(1+2tδi)ϑi(1+tδi)2ϑi, i=1,2 dK(δi,ϑi)dt=−2δiϑit2δi−1(1+2tδi)ϑi−1(1+tδi)2ϑi+1, i=1,2
At ϑ1<ϑ2, δ1<δ2, υ1<υ2, we get d/dt[logh1(t;Θ1)−logh2(t;Θ2)]<0, for all t≥0, hence, h1(t;Θ1)h2(t;Θ2) is decreasing in t, and hence, T1≤lrT2. Moreover, T1 is said to be smaller than T2 in other different orderings, such as SO (denoted by T1≤stT2), hazard rate order (denoted by T1≤hrT2), and reversed hazard rate order (denoted by T1≤rhrT2).
The entropy of a random variable with PDF (5) is a measure of the uncertainty's fluctuation. A high entropy number indicates that the data is more unpredictable. The Rényi entropy (RE) [27] is defined by:
M(τ)=(1−τ)−1log(∫∞−∞(h(t))τdt),τ≠1,τ>0. | (8) |
To obtain M(τ) of the TPITL model, we must obtain (h(t;Θ))τ, as follows:
(h(t;Θ))τ=Ξ1Ξ2, |
where, Ξ1=(1−υ)τ(2ϑδ)τtτ(2δ−1)(1+tδ)−τ(2ϑ+1)(1+2tδ)τ(ϑ−1) and Ξ2=[1+2υ1−υK(δ,ϑ)]τ.
Using the binomial expansion, then Ξ1 and Ξ2 noticing that [2υ1−υK(δ,ϑ)]<1, we have
Ξ1=∑∞j=0(1−υ)τ(τ(ϑ−1)j)(2ϑδ)τtτ(2δ−1)+δj(1+tδ)−τϑ−2τ−j, |
Ξ2=∑∞i=0(τi)(2υ1−υ)i(1+tδ)−ϑi(1+tδ1+tδ)ϑi=∑∞i,k=0(τi)(ϑik)(2υ1−υ)itδk(1+tδ)−ϑi−k. |
Hence, (h(t;Θ))τ, is formed as
(h(t;Θ))τ=[Υjξi,ktτ(2δ−1)+δj+δk(1+tδ)−τϑ−2τ−j−ϑi−k], | (9) |
Υj=∑∞j=0(1−υ)τ(τ(ϑ−1)j)(2ϑδ)τ,ξi,k=∑∞i,k=0(τi)(ϑik)(2υ1−υ)i. |
Insert (9) in (8), then, we get the RE of the TPITL distribution as follows:
M(τ)=(1−τ)−1log{[ξi,kΥjδB(2τ−τδ+j+k+1δ,τϑ+ϑi+τδ−1δ)]}. |
The Tsallis entropy (TE) measure [28] is defined by:
ρ(τ)=1τ−1[1−∫∞−∞(h(t))τdt],τ≠1,τ>0. |
The TE of the TPITL distribution is obtained as follows:
ρ(τ)=1τ−1[1−{[ξi,kΥjδB(2τ−τδ+j+k+1δ,τϑ+ϑi+τδ−1δ)]}]. |
The Arimoto entropy (AE) measure ([29]) is given by:
ψ(τ)=τ1−τ[(∫∞−∞(h(t))τdt)1τ−1],τ≠1,τ>0. |
Hence, the AE of the TPITL distribution is given by:
ψ(τ)=τ1−τ{[ξi,kΥjδB(2τ−τδ+j+k+1δ,τϑ+ϑi+τδ−1δ)]1τ−1}. |
The Havrda and Charvat entropy (H–CE) measure ([30]) is defined by:
γ(τ)=121−τ−1[(∫∞−∞hτ(t)dt)1τ−1],τ≠1,τ>0. |
Hence, the H–CE of the TPITL distribution is given by:
γ(τ)=121−τ−1{[ξi,kΥjδB(2τ−τδ+j+k+1δ,τϑ+ϑi+τδ−1δ)]1τ−1}. |
Table 2 shows the numerical entropy values for some of the elected parameter values.
τ | (ϑ,δ,υ) | RE | TE | AE | H-CE |
0.5 | (0.5, 3, 0.3) | 3.28 | 8.308 | 25.563 | 61.716 |
(1, 3, 0.3) | 1.535 | 2.308 | 3.64 | 8.789 | |
(1.5, 3, 0.3) | 1.028 | 1.345 | 1.797 | 4.337 | |
(2, 3, 0.3) | 0.76 | 0.924 | 1.138 | 2.746 | |
(0.5, 5, –0.3) | 1.944 | 3.286 | 5.985 | 14.448 | |
(1, 5, –0.3) | 0.876 | 1.1 | 1.402 | 3.384 | |
(1.5, 5, –0.3) | 0.483 | 0.546 | 0.62 | 1.497 | |
(2, 5, –0.3) | 0.263 | 0.281 | 0.301 | 0.726 | |
2 | (0.5, 3, 0.3) | 1.163 | 0.687 | 0.882 | 0.882 |
(1, 3, 0.3) | 0.615 | 0.46 | 0.53 | 0.53 | |
(1.5, 3, 0.3) | 0.353 | 0.297 | 0.323 | 0.323 | |
(2, 3, 0.3) | 0.188 | 0.172 | 0.18 | 0.18 | |
(0.5, 5, –0.3) | 1.504 | 0.778 | 1.057 | 1.057 | |
(1, 5, –0.3) | 0.834 | 0.566 | 0.682 | 0.682 | |
(1.5, 5, –0.3) | 0.517 | 0.404 | 0.456 | 0.456 | |
(2, 5, –0.3) | 0.32 | 0.274 | 0.296 | 0.296 |
From Table 2, we observe the following:
● For a small value of τ, the H–CE measure takes the largest values for all values of (ϑ,δ,υ), compared to other entropy measures, which leads to less information.
● The RE measure provides more information due to its small values compared with other measures at τ=0.5 for all values of (ϑ,δ,υ).
● As the value of τ increases, the values of all entropy measures decrease.
● The TS entropy gets the smallest values for all values of (ϑ,δ,υ), at a large value of τ, which leads to less variability.
We assume that the lifetime of a product follows the TPITL distribution with parameters (Θ) defined by (4) and that the specified median lifetime (med) of the units claimed by a producer is med0. Our interest is to make an inference about the acceptance or rejection of the proposed lot based on the criterion that the actual med of the units is larger than the prescribed lifetime med0. A common practice in life testing is to terminate the life test at a predetermined time t0 and note the number of failures. Now to observe med, the experiment is run for a t0=a∗ med0 units of time, multiple of claimed med with any positive constant a. The idea of accepting the proposed lot based on the evidence that med ≥ med0, given probability of at least p∗ (consumer's risk) using a single ASP is as follows [31]: Draw a random sample of n number of units from the proposed lot and conduct an experiment for t0 units of time. If during the experiment, c or less number of units (acceptance number) fail, then accept the whole lot; otherwise, reject the lot. Observe the probability of accepting a lot, and consider sufficiently large-sized lots so that the binomial distribution can be applied under the proposed sampling plan given by:
L(p)=∑ci=0(ni)pi(1−p)n−i,i=1,…,n, |
where p=H(t0;ϑ), defined by (4). The function L(p) is the operating characteristic function of the sampling plan, i.e., the acceptance probability of the lot as a function of the failure probability. Further, using t0=a∗m0, p0 can be written as follows:
p0=[1−{(1+2(ma0)δ)ϑ(1+(ma0)δ)2ϑ}][1+υ{(1+2(ma0)δ)ϑ(1+(ma0)δ)2ϑ}]. | (10) |
Now, the problem is to determine for the given values of p∗(0<p∗<1), t0, and c, the smallest positive integer n such that
L(p0)=∑ci=0(ni)pi0(1−p0)n−i≤1−p∗, | (11) |
where p0 is given by (10). The minimum values of n satisfying the inequality (11) and its corresponding operating characteristic probability are obtained and displayed in Tables 3–8 for the assumed parameters as follows: p∗= 0.2, 0.4, 0.6, 0.99, c= 0.2 (0.2) 1 when a=1, thus, t0= med0 = 0.5 for all parameter values. The assumed parameter values of TPITL distribution are:
p∗ | c | a=0.2 | a=0.4 | a=0.6 | a=0.8 | a=1 | |||||
n | L(p0) | n | L(p0) | n | L(p0) | n | L(p0) | n | L(p0) | ||
0.2 | 0 | 1 | 1.0000 | 1 | 1.0000 | 1 | 1.0000 | 1 | 1.0000 | 1 | 1.0000 |
2 | 6 | 0.8346 | 5 | 0.8354 | 4 | 0.9162 | 4 | 0.8941 | 4 | 0.8750 | |
4 | 11 | 0.8468 | 9 | 0.8457 | 8 | 0.8627 | 8 | 0.8152 | 7 | 0.8906 | |
10 | 29 | 0.8030 | 23 | 0.8085 | 20 | 0.8438 | 19 | 0.8256 | 18 | 0.8338 | |
0.4 | 0 | 2 | 0.6983 | 2 | 0.6130 | 1 | 1.0000 | 1 | 1.0000 | 1 | 1.0000 |
2 | 8 | 0.6432 | 6 | 0.7047 | 6 | 0.6158 | 5 | 0.7268 | 5 | 0.6875 | |
4 | 14 | 0.6491 | 11 | 0.6652 | 10 | 0.6504 | 9 | 0.6939 | 9 | 0.6367 | |
10 | 33 | 0.6359 | 26 | 0.6373 | 23 | 0.6486 | 21 | 0.6797 | 20 | 0.6762 | |
0.6 | 0 | 3 | 0.4876 | 2 | 0.6130 | 2 | 0.5624 | 2 | 0.5270 | 2 | 0.5000 |
2 | 10 | 0.4582 | 8 | 0.4484 | 7 | 0.4667 | 6 | 0.5504 | 6 | 0.5000 | |
4 | 17 | 0.4439 | 13 | 0.4753 | 12 | 0.4300 | 11 | 0.4448 | 10 | 0.5000 | |
10 | 38 | 0.4151 | 29 | 0.4539 | 26 | 0.4330 | 24 | 0.4387 | 23 | 0.4159 | |
0.8 | 0 | 5 | 0.2377 | 4 | 0.2303 | 3 | 0.3163 | 3 | 0.2777 | 3 | 0.2500 |
2 | 13 | 0.2487 | 10 | 0.2570 | 9 | 0.2419 | 8 | 0.2731 | 8 | 0.2266 | |
4 | 21 | 0.2324 | 16 | 0.2487 | 14 | 0.2562 | 13 | 0.2501 | 12 | 0.2744 | |
10 | 44 | 0.2077 | 34 | 0.2099 | 30 | 0.2071 | 27 | 0.2408 | 26 | 0.2122 | |
0.99 | 0 | 13 | 0.0134 | 10 | 0.0122 | 9 | 0.0100 | 8 | 0.0113 | 7 | 0.0156 |
2 | 25 | 0.0114 | 19 | 0.0109 | 16 | 0.0136 | 15 | 0.0111 | 14 | 0.0112 | |
4 | 35 | 0.0110 | 26 | 0.0132 | 23 | 0.0113 | 21 | 0.0113 | 19 | 0.0154 | |
10 | 62 | 0.0107 | 47 | 0.0114 | 41 | 0.0113 | 37 | 0.0135 | 35 | 0.0122 |
p∗ | c | a=0.2 | a=0.4 | a=0.6 | a=0.8 | a=1 | |||||
n | L(p0) | n | L(p0) | n | L(p0) | n | L(p0) | n | L(p0) | ||
0.2 | 0 | 1 | 1.0000 | 1 | 1.0000 | 1 | 1.0000 | 1 | 1.0000 | 1 | 1.0000 |
2 | 8 | 0.8058 | 5 | 0.8890 | 5 | 0.8148 | 4 | 0.9037 | 4 | 0.8750 | |
4 | 15 | 0.8099 | 10 | 0.8553 | 9 | 0.8183 | 8 | 0.8360 | 7 | 0.8906 | |
10 | 38 | 0.8055 | 26 | 0.8223 | 22 | 0.8127 | 19 | 0.8563 | 18 | 0.8338 | |
0.4 | 0 | 3 | 0.6001 | 2 | 0.6668 | 1 | 1.0000 | 1 | 1.0000 | 1 | 1.0000 |
2 | 11 | 0.6005 | 7 | 0.6806 | 6 | 0.6737 | 5 | 0.7473 | 5 | 0.6875 | |
4 | 19 | 0.6174 | 13 | 0.6318 | 11 | 0.6202 | 10 | 0.6016 | 9 | 0.6367 | |
10 | 44 | 0.6278 | 30 | 0.6362 | 25 | 0.6308 | 22 | 0.6507 | 20 | 0.6762 | |
0.6 | 0 | 4 | 0.4649 | 3 | 0.4446 | 2 | 0.5949 | 2 | 0.5417 | 2 | 0.5000 |
2 | 14 | 0.4118 | 9 | 0.4685 | 8 | 0.4088 | 7 | 0.4248 | 6 | 0.5000 | |
4 | 23 | 0.4261 | 16 | 0.4044 | 13 | 0.4237 | 11 | 0.4828 | 10 | 0.5000 | |
10 | 51 | 0.4092 | 34 | 0.4354 | 28 | 0.4368 | 25 | 0.4214 | 23 | 0.4159 | |
0.8 | 0 | 7 | 0.2161 | 4 | 0.2964 | 4 | 0.2105 | 3 | 0.2934 | 3 | 0.2500 |
2 | 18 | 0.2274 | 12 | 0.2343 | 10 | 0.2223 | 9 | 0.2062 | 8 | 0.2266 | |
4 | 29 | 0.2108 | 19 | 0.2314 | 16 | 0.2056 | 14 | 0.2100 | 12 | 0.2744 | |
10 | 59 | 0.2126 | 39 | 0.2313 | 32 | 0.2275 | 28 | 0.2357 | 26 | 0.2122 | |
0.99 | 0 | 19 | 0.0101 | 12 | 0.0116 | 9 | 0.0157 | 8 | 0.0137 | 7 | 0.0156 |
2 | 34 | 0.0121 | 22 | 0.0129 | 18 | 0.0111 | 15 | 0.0146 | 14 | 0.0112 | |
4 | 48 | 0.0109 | 31 | 0.0123 | 25 | 0.0118 | 22 | 0.0104 | 19 | 0.0154 | |
10 | 85 | 0.0101 | 55 | 0.0124 | 45 | 0.0104 | 39 | 0.0109 | 35 | 0.0122 |
p* | c | a=0.2 | a=0.4 | a=0.6 | a=0.8 | a=1 | |||||
n | L(p0) | n | L(p0) | n | L(p0) | n | L(p0) | n | L(p0) | ||
0.2 | 0 | 5 | 0.8277 | 2 | 0.8220 | 1 | 1.0000 | 1 | 1.0000 | 1 | 1.0000 |
2 | 34 | 0.8059 | 9 | 0.8432 | 6 | 0.8221 | 4 | 0.9270 | 4 | 0.8750 | |
4 | 68 | 0.8032 | 18 | 0.8285 | 11 | 0.8303 | 8 | 0.8854 | 7 | 0.8906 | |
10 | 178 | 0.8031 | 47 | 0.8163 | 28 | 0.8108 | 21 | 0.8344 | 18 | 0.8339 | |
0.4 | 0 | 11 | 0.6234 | 3 | 0.6757 | 2 | 0.6890 | 1 | 1.0000 | 1 | 1.0000 |
2 | 50 | 0.6045 | 13 | 0.6370 | 8 | 0.6221 | 6 | 0.6512 | 5 | 0.6875 | |
4 | 90 | 0.6072 | 24 | 0.6093 | 14 | 0.6210 | 10 | 0.6950 | 9 | 0.6367 | |
10 | 214 | 0.6045 | 56 | 0.6126 | 32 | 0.6386 | 24 | 0.6498 | 20 | 0.6762 | |
0.6 | 0 | 20 | 0.4074 | 5 | 0.4566 | 3 | 0.4748 | 2 | 0.5821 | 2 | 0.5000 |
2 | 67 | 0.4073 | 17 | 0.4386 | 10 | 0.4339 | 7 | 0.5073 | 6 | 0.5000 | |
4 | 113 | 0.4064 | 29 | 0.4263 | 17 | 0.4121 | 12 | 0.4836 | 10 | 0.5000 | |
10 | 249 | 0.4037 | 64 | 0.4207 | 37 | 0.4098 | 27 | 0.4468 | 23 | 0.4159 | |
0.8 | 0 | 35 | 0.2005 | 9 | 0.2085 | 5 | 0.2254 | 3 | 0.3389 | 3 | 0.2500 |
2 | 92 | 0.2033 | 23 | 0.2225 | 13 | 0.2274 | 9 | 0.2792 | 8 | 0.2266 | |
4 | 145 | 0.2011 | 37 | 0.2072 | 21 | 0.2062 | 15 | 0.2352 | 12 | 0.2744 | |
10 | 294 | 0.2030 | 75 | 0.2118 | 42 | 0.2269 | 31 | 0.2271 | 26 | 0.2122 | |
0.99 | 0 | 98 | 0.0102 | 24 | 0.0110 | 13 | 0.0115 | 9 | 0.0132 | 7 | 0.0156 |
2 | 179 | 0.0103 | 44 | 0.0115 | 24 | 0.0120 | 17 | 0.0129 | 14 | 0.0112 | |
4 | 248 | 0.0102 | 62 | 0.0104 | 34 | 0.0107 | 24 | 0.0124 | 19 | 0.0154 | |
10 | 432 | 0.0101 | 108 | 0.0110 | 60 | 0.0107 | 43 | 0.0119 | 35 | 0.0122 |
p∗ | c | a=0.2 | a=0.4 | a=0.6 | a=0.8 | a=1 | |||||
n | L(p0) | n | L(p0) | n | L(p0) | n | n | L(p0) | n | ||
0.2 | 0 | 1 | 1.0000 | 1 | 1.0000 | 1 | 1.0000 | 1 | 1.0000 | 1 | 1.0000 |
2 | 7 | 0.8405 | 5 | 0.8776 | 5 | 0.8063 | 4 | 0.9018 | 4 | 0.8750 | |
4 | 14 | 0.8097 | 10 | 0.8354 | 9 | 0.8067 | 8 | 0.8317 | 7 | 0.8906 | |
10 | 35 | 0.8142 | 25 | 0.8284 | 21 | 0.8469 | 19 | 0.8502 | 18 | 0.8338 | |
0.4 | 0 | 2 | 0.7564 | 2 | 0.6543 | 1 | 1.0000 | 1 | 1.0000 | 1 | 1.0000 |
2 | 10 | 0.6185 | 7 | 0.6557 | 6 | 0.6610 | 5 | 0.7430 | 5 | 0.6875 | |
4 | 17 | 0.6530 | 12 | 0.6795 | 11 | 0.6019 | 9 | 0.7174 | 9 | 0.6367 | |
10 | 41 | 0.6205 | 29 | 0.6344 | 25 | 0.6030 | 22 | 0.6399 | 20 | 0.6762 | |
0.6 | 0 | 4 | 0.4327 | 3 | 0.4281 | 2 | 0.5877 | 2 | 0.5386 | 2 | 0.5000 |
2 | 13 | 0.4107 | 9 | 0.4381 | 7 | 0.5188 | 7 | 0.4186 | 6 | 0.5000 | |
4 | 21 | 0.4409 | 15 | 0.4361 | 13 | 0.4036 | 11 | 0.4747 | 10 | 0.5000 | |
10 | 47 | 0.4152 | 33 | 0.4246 | 28 | 0.4068 | 25 | 0.4093 | 23 | 0.4159 | |
0.8 | 0 | 6 | 0.2476 | 4 | 0.2801 | 4 | 0.2030 | 3 | 0.2901 | 3 | 0.2500 |
2 | 17 | 0.2135 | 12 | 0.2085 | 10 | 0.2093 | 9 | 0.2011 | 8 | 0.2266 | |
4 | 27 | 0.2046 | 18 | 0.2463 | 15 | 0.2484 | 14 | 0.2035 | 12 | 0.2744 | |
10 | 55 | 0.2022 | 38 | 0.2163 | 32 | 0.2037 | 28 | 0.2258 | 26 | 0.2122 | |
0.99 | 0 | 17 | 0.0115 | 11 | 0.0144 | 9 | 0.0142 | 8 | 0.0131 | 7 | 0.0156 |
2 | 32 | 0.0103 | 21 | 0.0134 | 17 | 0.0144 | 15 | 0.0138 | 14 | 0.0112 | |
4 | 44 | 0.0113 | 30 | 0.0114 | 24 | 0.0142 | 21 | 0.0147 | 19 | 0.0154 | |
10 | 78 | 0.0105 | 53 | 0.0119 | 44 | 0.0107 | 38 | 0.0138 | 35 | 0.0122 |
p∗ | c | a=0.2 | a=0.4 | a=0.6 | a=0.8 | a=1 | |||||
n | L(p0) | n | L(p0) | n | L(p0) | n | L(p0) | n | L(p0) | ||
0.2 | 0 | 2 | 0.8116 | 1 | 1.0000 | 1 | 1.0000 | 1 | 1.0000 | 1 | 1.0000 |
2 | 9 | 0.8220 | 6 | 0.8359 | 5 | 0.8398 | 4 | 0.9104 | 4 | 0.8750 | |
4 | 17 | 0.8319 | 11 | 0.8484 | 9 | 0.8515 | 8 | 0.8504 | 7 | 0.8906 | |
10 | 45 | 0.8064 | 29 | 0.8062 | 23 | 0.8192 | 20 | 0.8219 | 18 | 0.8338 | |
0.4 | 0 | 3 | 0.6588 | 2 | 0.6992 | 2 | 0.6170 | 1 | 1.0000 | 1 | 1.0000 |
2 | 12 | 0.6560 | 8 | 0.6453 | 6 | 0.7115 | 5 | 0.7619 | 5 | 0.6875 | |
4 | 22 | 0.6392 | 14 | 0.6520 | 11 | 0.6751 | 10 | 0.6275 | 9 | 0.6367 | |
10 | 53 | 0.6120 | 33 | 0.6404 | 26 | 0.6530 | 23 | 0.6131 | 20 | 0.6762 | |
0.6 | 0 | 5 | 0.4340 | 3 | 0.4889 | 2 | 0.6170 | 2 | 0.5526 | 2 | 0.5000 |
2 | 16 | 0.4429 | 10 | 0.4608 | 8 | 0.4574 | 7 | 0.4467 | 6 | 0.5000 | |
4 | 28 | 0.4058 | 17 | 0.4472 | 14 | 0.4014 | 12 | 0.4039 | 10 | 0.5000 | |
10 | 61 | 0.4084 | 38 | 0.4200 | 30 | 0.4142 | 25 | 0.4640 | 23 | 0.4159 | |
0.8 | 0 | 8 | 0.2320 | 5 | 0.2390 | 4 | 0.2349 | 3 | 0.3053 | 3 | 0.2500 |
2 | 22 | 0.2147 | 14 | 0.2008 | 10 | 0.2651 | 9 | 0.2245 | 8 | 0.2266 | |
4 | 35 | 0.2059 | 21 | 0.2352 | 17 | 0.2035 | 14 | 0.2337 | 12 | 0.2744 | |
10 | 71 | 0.2089 | 44 | 0.2116 | 34 | 0.2239 | 29 | 0.2215 | 26 | 0.2122 | |
0.99 | 0 | 23 | 0.0101 | 13 | 0.0137 | 10 | 0.0130 | 8 | 0.0157 | 7 | 0.0156 |
2 | 42 | 0.0105 | 25 | 0.0116 | 19 | 0.0119 | 16 | 0.0112 | 14 | 0.0112 | |
4 | 58 | 0.0110 | 35 | 0.0114 | 27 | 0.0105 | 22 | 0.0134 | 19 | 0.0154 | |
10 | 102 | 0.0108 | 62 | 0.0111 | 48 | 0.0101 | 40 | 0.0112 | 35 | 0.0122 |
p∗ | c | a=0.2 | a=0.4 | a=0.6 | a=0.8 | a=1 | |||||
n | L(p0) | n | L(p0) | n | L(p0) | n | L(p0) | n | L(p0) | ||
0.2 | 0 | 19 | 0.8043 | 3 | 0.8513 | 2 | 0.8010 | 1 | 1.0000 | 1 | 1.0000 |
2 | 128 | 0.8027 | 21 | 0.8019 | 8 | 0.8536 | 5 | 0.8740 | 4 | 0.8750 | |
4 | 258 | 0.8008 | 41 | 0.8058 | 17 | 0.8012 | 10 | 0.8291 | 7 | 0.8906 | |
10 | 680 | 0.8003 | 107 | 0.8034 | 42 | 0.8220 | 25 | 0.8180 | 18 | 0.8338 | |
0.4 | 0 | 43 | 0.6016 | 7 | 0.6169 | 3 | 0.6416 | 2 | 0.6505 | 1 | 1.0000 |
2 | 190 | 0.6028 | 30 | 0.6086 | 12 | 0.6206 | 7 | 0.6481 | 5 | 0.6875 | |
4 | 345 | 0.6020 | 54 | 0.6087 | 21 | 0.6339 | 12 | 0.6696 | 9 | 0.6367 | |
10 | 821 | 0.6006 | 128 | 0.6061 | 50 | 0.6182 | 29 | 0.6182 | 20 | 0.6762 | |
0.6 | 0 | 76 | 0.4036 | 12 | 0.4125 | 5 | 0.4116 | 3 | 0.4231 | 2 | 0.5000 |
2 | 258 | 0.4018 | 40 | 0.4103 | 16 | 0.4017 | 9 | 0.4290 | 6 | 0.5000 | |
4 | 435 | 0.4017 | 68 | 0.4010 | 26 | 0.4255 | 15 | 0.4243 | 10 | 0.5000 | |
10 | 957 | 0.4012 | 149 | 0.4010 | 58 | 0.4019 | 33 | 0.4070 | 23 | 0.4159 | |
0.8 | 0 | 134 | 0.2001 | 20 | 0.2166 | 8 | 0.2115 | 4 | 0.2752 | 3 | 0.2500 |
2 | 355 | 0.2011 | 55 | 0.2017 | 21 | 0.2091 | 12 | 0.2011 | 8 | 0.2266 | |
4 | 558 | 0.2007 | 86 | 0.2043 | 33 | 0.2082 | 18 | 0.2361 | 12 | 0.2744 | |
10 | 1134 | 0.2003 | 175 | 0.2037 | 67 | 0.2113 | 38 | 0.2025 | 26 | 0.2122 | |
0.99 | 0 | 381 | 0.0101 | 58 | 0.0102 | 21 | 0.0118 | 11 | 0.0136 | 7 | 0.0156 |
2 | 696 | 0.0101 | 106 | 0.0103 | 39 | 0.0117 | 21 | 0.0123 | 14 | 0.0112 | |
4 | 962 | 0.0100 | 147 | 0.0101 | 55 | 0.0105 | 30 | 0.0103 | 19 | 0.0154 | |
10 | 1671 | 0.0100 | 256 | 0.0101 | 96 | 0.0112 | 53 | 0.0103 | 35 | 0.0122 |
(i) (υ,δ,ϑ)=(0.5,0.5,0.5), (ii) (υ,δ,ϑ)=(0.5,0.5,1.5), (iii) (υ,δ,ϑ)=(0.5,1.5,0.5), (iv) (υ,δ,ϑ)=(1.5,0.5,0.5), (v) (υ,δ,ϑ)=(1.5,0.5,1.5), and (vi) (υ,δ,ϑ)=(1.5,1.5,1.5). We provide the R codes of numerical value in Appendix A. From the results obtained in Tables 3–8, one can notice that:
● Regarding the ASP parameters, when p∗ and c are increasing, the required sample sizes n and L(p0) are also increasing.
● As the value of a increases, the required sample sizes n and L(p0) decrease.
● Regarding the TPITL distribution parameters, the desired sample size n increases while L(p0) decreases with an increased value of ϑ for fixed υ and δ
● The required sample size n increases while L(p0) decreases as the value of δ increases for fixed values of υ and ϑ.
● The required sample size n increases while L(p0) decreases with increasing values of δ and fixed values of υ and ϑ.
● As the value of υ increases for fixed values of ϑ, and δ, the required sample size n increases, while L(p0) decreases.
● For all results, we have obtained and verified that L(p0)≤1−p∗. Also, when a=1, we have p0=0.5 as t0=m0; hence, all results (n,L(p0)) for any vector of parameters (υ,δ,ϑ) are similar.
In this section, the parameter estimation of the TPITL distribution is discussed using classical methods, including ML, MPS, LS, WLS, and CvM.
Let T1,T2,…Tn be the TPITL distribution's observed random sample. Then, the TPITL distribution's log-likelihood function, denoted by ln l, for parameters, based on a complete sample, is given by
ln l=n ln (2ϑδ)+(2δ−1)∑ni=1 ln ti−(2ϑ+1)∑ni=1 ln Wi+(ϑ−1)∑ni=1 ln Si+∑ni=1 ln [1−υ+2υ(SϑiW−2ϑi)]. |
where, Wi=Wi(δ)=(1+tδi) and Si=Si(δ)=(1+2tδi). The partial derivatives of ln l with respect to υ,δ and ϑ are given by:
∂ ln l∂υ=∑ni=1[2(1−SϑiW−2ϑi)−1][1−υ+2υ(SϑiW−2ϑi)]−1, |
∂ ln l∂δ=nδ+2n∑i=1 ln ti−(2ϑ+1)n∑i=1tδi ln tiW−1i+2(ϑ−1)n∑i=1tδi ln tiS−1i |
+4ϑυ∑ni=1{tδi ln ti[W−2ϑiSϑ−1i−SϑiW−2ϑ−1i]}[1−υ+2υ(SϑiW−2ϑi)]−1, |
∂ ln l∂ϑ=nϑ−2∑ni=1 ln Wi+∑ni=1 ln Si+2υ∑ni=1SϑiW−2ϑi( ln Si−2 ln Wi)[1−υ+2υ(SϑiW−2ϑi)]−1. |
To obtain the ML estimators of υ,δ and ϑ, say ˆυ,ˆδ and ˆϑ we use the nonlinear equations, ∂ ln l/∂υ=0, ∂ ln l/∂δ=0 and ∂ ln l/∂ϑ=0 to solve them using an iterative approach.
Reference [25] proposed the MPS estimation of population parameters. The notion of discrepancies between the CDF values at consecutive data points may be used to create this approach. Assume that T(1), T(2), …, T(n), are the ordered observations of TPITL distribution. Then, the uniform spacings may be determined based on a random sample of size n from the TPITL distribution, as follows:
Di(Θ)=H(t(i)|Θ)−H(t(i−1)|Θ),i=1,2,…,n+1,H(t(0)|Θ)=0,H(t(n+1)|Θ)=1,∑n+1i=1Di(Θ)=1. |
For simplicity of notation, we write ti=t(i), hence, the MPS estimates obtained by ˆυMPS,ˆδMPS and ˆϑMPS can be obtained by maximizing the geometric mean of the spacings
GM(Θ)=1n+1∑n+1i=1log{[(1−SϑiW−2ϑi)(1+υ−υ(1−SϑiW−2ϑi))]−[(1−Sϑi−1W−2ϑi−1)(1+υ−υ(1−Sϑi−1W−2ϑi−1))]}, |
with respect to υ,δ and ϑ, or by solving the following equations:
∂GM(Θ)∂υ=1n+1∑n+1i=1ω1(ti|Θ)−ω1(ti−1|Θ)Di(Θ),∂GM(Θ)∂ϑ=1n+1∑n+1i=1ω2(ti|Θ)−ω2(ti−1|Θ)Di(Θ), |
∂GM(Θ)∂δ=1n+1∑n+1i=1ω3(ti|Θ)−ω3(ti−1|Θ)Di(Θ). |
ω1(ti|Θ)=(1−SϑiW−2ϑi)(SϑiW−2ϑi), | (12) |
ω2(ti|Θ)=SϑiW−2ϑi{(1−SϑiW−2ϑi)υ[ ln Si−2 ln Wi]+(1+υSϑiW−2ϑi)[2 ln Wi− ln Si]}, | (13) |
ω3(ti|Θ)=2ϑtδi ln tiSϑiW−2ϑi[W−1i−S−1i][υ(S−1i−W−1i)]. | (14) |
Let T(1),T(2),….T(n) be the observed ordered sample and T1,T2,….Tn a TPITL-generated random sample of size n. Then, the LS estimates (LSEs) and WLS estimates (WLSEs) of υ,δ and ϑ can be obtained by minimizing the following function with respect to υ,δ and ϑ :
Λ(Θ)=∑ni=1ui{[(1−SϑiW−2ϑi)(1+υSϑiW−2ϑi)]−in+1}2. |
where ti=t(i), we can get the LSEs designated by ˆυLS,ˆδLS and ˆϑLS by setting ui=1, whereas we can get the WLSEs denoted by ˆυWLS,ˆδWLS and ˆϑWLS by setting ui=(n+1)2(n+2)i(n−i+1). These estimates can also be obtained by solving the equations stated below:
∂Λ(Θ)∂υ=∑ni=12ui{[(1−SϑiW−2ϑi)(1+υSϑiW−2ϑi)]−in+1}ω1(ti|Θ), |
∂Λ(Θ)∂ϑ=∑ni=12ui{[(1−SϑiW−2ϑi)(1+υSϑiW−2ϑi)]−in+1}ω2(ti|Θ), |
and
∂Λ(Θ)∂δ=∑ni=12ui{[(1−SϑiW−2ϑi)(1+υSϑiW−2ϑi)]−in+1}ω3(ti|Θ). |
ω1(ti|Θ), ω2(ti|Θ) and ω3(ti|Θ) are defined in (12)–(14).
The CvM estimates (CvMEs) denoted by ˆυCME,ˆδCME and ˆϑCME of υ,δ and ϑ can be obtained by minimizing the following function with respect to υ,δ and ϑ :
CvM(Θ)=112n+n∑i=1{[(1−SϑiW−2ϑi)(1+υSϑiW−2ϑi)]−2i−12n}2, |
where ti=t(i). These estimates can also be obtained by solving the following equations:
∂CvM(Θ)∂υ=∑ni=12{[(1−SϑiW−2ϑi)(1+υSϑiW−2ϑi)]−2i−12n}ω1(ti|Θ), |
∂CvM(Θ)∂ϑ=∑ni=12{[(1−SϑiW−2ϑi)(1+υSϑiW−2ϑi)]−2i−12n}ω2(ti|Θ), |
and
∂CvM(Θ)∂δ=∑ni=12{[(1−SϑiW−2ϑi)(1+υSϑiW−2ϑi)]−2i−12n}ω3(ti|Θ). |
where ω1(ti|Θ), ω2(ti|Θ) and ω3(ti|Θ) are defined in (12)–(14).
As mentioned in the previous section, expressions for the derived estimators are hard to obtain. Therefore, we designed a simulation study to clarify the theoretical results. The behavior of estimates was examined in terms of their mean squared error (MSE) and standard error (SE). We performed the following steps:
Step 1: Random samples (10,000) of sizes 20, 30, 50, 75 and 150 were generated from the TPITL distribution. The chosen parameter values are as follows:
(δ=0.75,ϑ=0.25,υ=−0.25), (δ=1.5,ϑ=0.5,υ=−0.25), (δ=0.75,ϑ=0.25,υ=−0.75), and (δ=1.5,ϑ=0.5,υ=−0.75).
Step 2: ML estimate (MLE), MPSE, LSE, WLSE, and CvMEs of the parameters were obtained.
Step 3: We computed MSEs and SEs of all estimates, and the results are listed in Tables 9–12. We noticed the following about the performance of estimates:
n | Parameters | MLE | LSE | WLSE | MPSE | CvME | |||||
MSE | SE | MSE | SE | MSE | SE | MSE | SE | MSE | SE | ||
20 | δ=0.75 | 0.0634 | 0.0512 | 0.0462 | 0.0471 | 0.0321 | 0.0392 | 0.0318 | 0.0385 | 0.0457 | 0.0476 |
ϑ=0.25 | 4.2070* | 0.0104 | 5.9938* | 0.0168 | 3.7685* | 0.0135 | 1.8682* | 9.6702* | 5.7922* | 0.0166 | |
υ=−0.25 | 0.0563 | 3.5521* | 0.1807 | 0.0249 | 0.1933 | 0.0222 | 0.2111 | 0.0204 | 0.2275 | 0.0281 | |
30 | δ=0.75 | 0.0316 | 0.0295 | 0.0327 | 0.0309 | 0.0242 | 0.0270 | 0.0193 | 0.0248 | 0.0328 | 0.0321 |
ϑ=0.25 | 3.5439* | 7.0577* | 3.0803* | 9.8808* | 2.7990* | 9.6063* | 1.0694* | 5.5847* | 4.3042* | 0.0118 | |
υ=−0.25 | 0.0558 | 2.0435* | 0.1807 | 0.0176 | 0.1915 | 0.0147 | 0.1973 | 0.0116 | 0.2110 | 0.0199 | |
50 | δ=0.75 | 0.0317 | 0.0213 | 0.0162 | 0.0174 | 0.0101 | 0.0140 | 0.0157 | 0.0165 | 0.0162 | 0.0178 |
ϑ=0.25 | 3.8108* | 4.7838* | 1.1283* | 4.7452* | 0.5372* | 3.0750* | 1.1926* | 3.7336* | 1.1844* | 4.8565* | |
υ=−0.25 | 0.0561 | 1.3275* | 0.1649 | 8.5725* | 0.1800 | 5.9981* | 0.1874 | 5.1355* | 0.1824 | 8.9142* | |
75 | \delta =0.75 | 0.0400 | 0.0202 | 0.0101 | 0.0104 | 6.1135* | 8.6267* | 0.0106 | 0.0112 | 8.3405* | 9.6595* |
\vartheta =0.25 | 3.6780* | 3.5785* | 0.4110* | 2.3153* | 0.3427* | 1.8551* | 1.0702* | 2.6413* | 0.3617* | 2.1469* | |
\upsilon =-0.25 | 0.0555 | 1.4264* | 0.1659 | 5.9550* | 0.1788 | 3.7775* | 0.1838 | 3.2474* | 0.1763 | 5.9837* | |
100 | \delta =0.75 | 0.0194 | 0.0113 | 7.9590* | 7.7147* | 5.3663* | 6.9967* | 8.3169* | 8.5896* | 7.3736* | 7.4625* |
\vartheta =0.25 | 3.1715* | 2.4667* | 0.4031* | 1.9978* | 0.3735* | 1.7288* | 0.9853* | 2.0216* | 0.3708* | 1.9209* | |
\upsilon =-0.25 | 0.0558 | 0.7247* | 0.1641 | 4.0990* | 0.1780 | 2.5736* | 0.1811 | 2.2216* | 0.1713 | 4.2937* | |
150 | \delta =0.75 | 0.0174 | 8.6607* | 4.7108* | 4.4809* | 3.5370* | 4.4433* | 4.2869* | 5.3664* | 4.3355* | 4.4987* |
\vartheta =0.25 | 3.0945* | 1.8702* | 0.2082* | 1.1216* | 0.2407* | 0.8882* | 0.6736* | 1.4636* | 0.2130* | 1.1105* | |
\upsilon =-0.25 | 0.0557 | 0.4741* | 0.1543 | 3.4320* | 0.1758 | 1.6379* | 0.1533 | 1.7328* | 0.1703 | 3.1378* |
n | Parameters | MLE | LSE | WLSE | MPSE | CvME | |||||
MSE | SE | MSE | SE | MSE | SE | MSE | SE | MSE | SE | ||
20 | \delta =1.5 | 0.5272 | 0.1158 | 0.2419 | 0.0810 | 0.2248 | 0.0707 | 0.4151 | 0.1004 | 0.3123 | 0.0825 |
\vartheta =0.5 | 0.0346 | 0.0142 | 0.0184 | 6.5972* | 0.0190 | 5.5531* | 0.0256 | 0.0107 | 0.0205 | 6.0177* | |
\upsilon =-0.25 | 0.0375 | 9.2001* | 0.4592 | 0.0446 | 0.4543 | 0.0345 | 0.3840 | 0.0235 | 0.5443 | 0.0447 | |
30 | \delta =1.5 | 0.4394 | 0.0833 | 0.1267 | 0.0477 | 0.1531 | 0.0502 | 0.2508 | 0.0594 | 0.1548 | 0.0482 |
\vartheta =0.5 | 0.0334 | 0.0100 | 0.0138 | 6.2299* | 0.0146 | 5.9041* | 0.0205 | 8.3477* | 0.0144 | 5.2513* | |
\upsilon =-0.25 | 0.0336 | 0.0101 | 0.4306 | 0.0254 | 0.4516 | 0.0219 | 0.4126 | 0.0164 | 0.4962 | 0.0278 | |
50 | \delta =1.5 | 0.4512 | 0.0672 | 0.1199 | 0.0348 | 0.1158 | 0.0321 | 0.1586 | 0.0376 | 0.1261 | 0.0324 |
\vartheta =0.5 | 0.0356 | 8.5213* | 0.0144 | 4.0590* | 0.0142 | 3.7567* | 0.0190 | 4.9860* | 0.0146 | 3.3391* | |
\upsilon =-0.25 | 0.0353 | 6.0854* | 0.4172 | 0.0154 | 0.4337 | 0.0122 | 0.3878 | 9.5065* | 0.4603 | 0.0164 | |
75 | \delta =1.5 | 0.4150 | 0.0512 | 0.1116 | 0.0226 | 0.1003 | 0.0193 | 0.1555 | 0.0300 | 0.1234 | 0.0216 |
\vartheta =0.5 | 0.0351 | 6.0012* | 0.0140 | 2.7917* | 0.0135 | 2.6442* | 0.0201 | 4.4084* | 0.0146 | 2.4824* | |
\upsilon =-0.25 | 0.0358 | 4.3681* | 0.4250 | 0.0103 | 0.4419 | 8.0752* | 0.3809 | 6.4523* | 0.4510 | 0.0106 | |
100 | \delta =1.5 | 0.4328 | 0.0426 | 0.1056 | 0.0171 | 0.0920 | 0.0172 | 0.1647 | 0.0282 | 0.1011 | 0.0153 |
\vartheta =0.5 | 0.0363 | 5.5815* | 0.0136 | 2.3123* | 0.0121 | 2.7129* | 0.0189 | 4.3207* | 0.0131 | 1.8163* | |
\upsilon =-0.25 | 0.0339 | 3.6981* | 0.4570 | 7.3339* | 0.4564 | 5.6197* | 0.3973 | 5.2128* | 0.4761 | 7.1705* | |
150 | \delta =1.5 | 0.3750 | 0.0254 | 0.0874 | 6.4564* | 0.0663 | 6.6803* | 0.1223 | 0.0146 | 0.0980 | 7.1989* |
\vartheta =0.5 | 0.0333 | 3.0071* | 0.0134 | 1.1206* | 0.0121 | 1.2057* | 0.0189 | 3.0867* | 0.0135 | 1.1276* | |
\upsilon =-0.25 | 0.0336 | 1.9440* | 0.4335 | 2.6577* | 0.4322 | 1.7794* | 0.3768 | 2.8150* | 0.4433 | 2.4982* |
n | Parameters | MLE | LSE | WLSE | MPSE | CvME | |||||
MSE | SE | MSE | SE | MSE | SE | MSE | SE | MSE | SE | ||
20 | \delta =0.75 | 0.0276 | 0.0320 | 0.0650 | 0.0400 | 0.0521 | 0.0350 | 0.0312 | 0.0370 | 0.0656 | 0.0420 |
\vartheta =0.25 | 2.9819* | 9.6410* | 8.3113* | 0.0200 | 6.9943* | 0.0180 | 2.1731* | 0.0100 | 0.0109 | 0.0220 | |
\upsilon =-0.75 | 0.5469 | 1.3110* | 0.9473 | 0.0340 | 0.9252 | 0.0260 | 0.8917 | 0.0210 | 1.0580 | 0.0370 | |
30 | \delta =0.75 | 0.0240 | 0.0250 | 0.0523 | 0.0240 | 0.0435 | 0.0250 | 0.0217 | 0.0250 | 0.0539 | 0.0260 |
\vartheta =0.25 | 3.4648* | 7.9550* | 5.8641* | 0.0140 | 5.8410* | 0.0140 | 2.2441* | 6.4740* | 6.4372* | 0.0140 | |
\upsilon =-0.75 | 0.5458 | 1.4640* | 0.9186 | 0.0210 | 0.9199 | 0.0190 | 0.8715 | 0.0150 | 0.9848 | 0.0230 | |
50 | \delta =0.75 | 0.0173 | 0.0170 | 0.0441 | 0.0170 | 0.0377 | 0.0170 | 0.0129 | 0.0140 | 0.0452 | 0.0180 |
\vartheta =0.25 | 3.8093* | 5.0600* | 3.6101* | 8.5390* | 4.1827* | 9.1340* | 2.3664* | 3.4760* | 3.8059* | 8.7550* | |
\upsilon =-0.75 | 0.5438 | 0.9780* | 0.8972 | 0.0110 | 0.8821 | 8.3640* | 0.8377 | 6.2060* | 0.9392 | 0.0110 | |
75 | \delta =0.75 | 0.0238 | 0.0157 | 0.0410 | 0.0140 | 0.0307 | 0.0130 | 9.5963* | 9.3015* | 0.0413 | 0.0148 |
\vartheta =0.25 | 2.9710* | 4.3074* | 3.6810* | 7.0170* | 3.2999* | 6.5592* | 1.9662* | 2.2798* | 4.7127* | 7.9185* | |
\upsilon =-0.75 | 0.5459 | 0.6425* | 0.9595 | 8.5172* | 0.8979 | 5.5828* | 0.8356 | 3.6097* | 0.9863 | 8.7994* | |
100 | \delta =0.75 | 0.0227 | 0.0134 | 0.0298 | 0.0100 | 0.0137 | 7.9828* | 4.3208* | 5.2667* | 0.0297 | 9.1020* |
\vartheta =0.25 | 2.3568* | 4.0206* | 2.8294* | 5.5969* | 1.5028* | 2.2740* | 1.5569* | 1.4654* | 2.5345* | 5.3057* | |
\upsilon =-0.75 | 0.5416 | 0.6058* | 0.9242 | 5.0194* | 0.8670 | 2.3480* | 0.8194 | 3.1607* | 0.9360 | 5.1240* | |
150 | \delta =0.75 | 9.1118* | 2.7224* | 0.0249 | 5.3565* | 8.5464* | 7.9564* | 4.1972* | 4.5636* | 0.0241 | 5.8262* |
\vartheta =0.25 | 1.7642* | 0.9286* | 1.5173* | 1.8264* | 0.8638* | 0.4991* | 1.0472* | 0.9626* | 1.0430* | 2.3932* | |
\upsilon =-0.75 | 0.5329 | 1.0329* | 0.9163 | 5.0756* | 0.8485 | 1.7051* | 0.7717 | 1.7450* | 0.9272 | 4.3014* |
n | Parameters | MLE | LSE | WLSE | MPSE | CvME | |||||
MSE | SE | MSE | SE | MSE | SE | MSE | SE | MSE | SE | ||
20 | \delta =1.5 | 0.3166 | 0.1074 | 0.0752 | 0.0611 | 0.0688 | 0.0584 | 0.1069 | 0.0688 | 0.0855 | 0.0628 |
\vartheta =0.5 | 0.0371 | 0.0136 | 0.0161 | 7.9040* | 0.0156 | 8.2771* | 0.0170 | 0.0104 | 0.0172 | 7.9114* | |
\upsilon =-0.75 | 0.4460 | 0.0347 | 1.4360 | 0.0421 | 1.4374 | 0.0389 | 1.3799 | 0.0355 | 1.6197 | 0.0471 | |
30 | \delta =1.5 | 0.2908 | 0.0852 | 0.0450 | 0.0385 | 0.0401 | 0.0359 | 0.0525 | 0.0419 | 0.0446 | 0.0385 |
\vartheta =0.5 | 0.0361 | 0.0105 | 0.0136 | 5.8095* | 0.0121 | 5.5192* | 0.0125 | 7.1990* | 0.0141 | 5.2089* | |
\upsilon =-0.75 | 0.4180 | 0.0143 | 1.4336 | 0.0260 | 1.4175 | 0.0237 | 1.3529 | 0.0213 | 1.5567 | 0.0286 | |
50 | \delta =1.5 | 0.2901 | 0.0662 | 0.0281 | 0.0235 | 0.0269 | 0.0219 | 0.0337 | 0.0256 | 0.0273 | 0.0234 |
\vartheta =0.5 | 0.0352 | 7.9137* | 0.0134 | 3.6008* | 0.0111 | 3.5586* | 0.0121 | 5.2926* | 0.0138 | 3.4227* | |
\upsilon =-0.75 | 0.4176 | 0.0131 | 1.4264 | 0.0148 | 1.3670 | 0.0112 | 1.2780 | 0.0106 | 1.4927 | 0.0155 | |
75 | \delta =1.5 | 0.1622 | 0.0418 | 0.0195 | 0.0158 | 0.0229 | 0.0153 | 0.0315 | 0.0192 | 0.0185 | 0.0157 |
\vartheta =0.5 | 0.0301 | 5.3795* | 0.0131 | 2.5415* | 0.0103 | 2.9067* | 0.0112 | 3.9476* | 0.0134 | 2.4295* | |
\upsilon =-0.75 | 0.4141 | 9.7076* | 1.4082 | 0.0101 | 1.3617 | 6.9812* | 1.2608 | 6.7749* | 1.4829 | 0.0101 | |
100 | \delta =1.5 | 0.2589 | 0.0436 | 0.0151 | 0.0122 | 0.0181 | 0.0107 | 0.0204 | 0.0132 | 0.0159 | 0.0126 |
\vartheta =0.5 | 0.0357 | 5.4308* | 0.0129 | 2.0247* | 9.3446* | 2.0512* | 0.0106 | 3.7361* | 0.0132 | 2.0816* | |
\upsilon =-0.75 | 0.4123 | 6.1602* | 1.3819 | 7.3598* | 1.3518 | 5.2508* | 1.2430 | 5.0394* | 1.4739 | 7.9705* | |
150 | \delta =1.5 | 0.1303 | 0.0258 | 0.0113 | 8.2565* | 0.0176 | 0.0105 | 0.0189 | 7.0110* | 0.0134 | 9.3264* |
\vartheta =0.5 | 0.0312 | 4.0948* | 0.0124 | 1.4042* | 7.8324* | 2.2745* | 8.8525* | 2.0483* | 0.0128 | 1.6189* | |
\upsilon =-0.75 | 0.4096 | 4.3745* | 1.3245 | 5.5974* | 1.3308 | 3.0557* | 1.2360 | 2.7320* | 1.4591 | 5.2175* | |
*Note: * Indicate that the value multiply {10}^{-3} in Tables 9–12. |
■ For all estimating methods, it was evident that MSEs and SEs reduced with n .
■ The MSE and SE for \widehat{\upsilon } performed better than the other estimates as the sample size increased.
■ The LSE, WLSE, WLSE, MPSE, and CvMEs for \upsilon and \vartheta had analogous results, while MLE had a slightly different result in terms of MSE and SE (Figure 3).
■ As shown in the left panel of Figures 4–5, the LSE, WLSE, MPSE, and CvME outperformed MLE in terms of MSE, especially for \vartheta and \delta .
■ Generally, under all approaches, we noticed that MSE of \widehat{\upsilon } had the least value, while MSE of {\widehat{\vartheta }}_{WLS} took the smallest value.
■ For a fixed value of the parameters \left(\delta , \vartheta \right) and as \upsilon increased, the MSEs and SEs of \upsilon estimate increased while the MSEs and SEs of \left(\delta , \vartheta \right) estimate decreased for different methods.
■ The general conclusion from the aforementioned figures is that as the sample size increases, all MSE and SE graphs for all parameters will reach zero. This confirms the accuracy of these estimation methods.
■ It should be emphasized that, in most situations, the ML technique outperformed other methods in terms of MSE.
■ In some situations, MPSE comes in as the second-best performing estimator, followed by LSE.
This section discussed two applications for the TPITL model using two different real datasets. The first dataset represents 30 observations of the March precipitation (in inches) in Minneapolis/St Paul. The proposed dataset was first recorded in [32] and recently used to fit the PITL distribution in [22].
We checked whether the TPITL distribution is suitable for analyzing this dataset. We reported the MLEs of the parameters and the values of the negative –2 log-likelihood \left(-2\mathrm{log}L\right) , Akaike's information criterion (AIC), Bayesian IC (BIC), corrected AIC (CAIC), and the Kolmogorov–Smirnov (K–S) test statistic, as well as associated P-value to judge the goodness of fit with comparison to PITL, generalized inverse exponential (GIE), inverse Weibull (in-We), inverse gamma (in-Ga) and Burr XII distributions. The lower the values of these criteria, the better the fit. Table 13 shows the parameter estimators, SE, and some goodness of fit statistics. Plots of estimated PDF and CDF are provided in Figure 6. The probability–probability (PP) plots of estimated densities are given in Figure 7.
Model | MLE | SE | -2LogL | AIC | BIC | CAIC | K-S | P-value |
TPITL | \widehat{\upsilon }=-0.373 | 0.610 | 77.89 | 82.66 | 85.09 | 83.10 | 0.1069 | 0.8831 |
\widehat{\delta }=1.723 | 0.587 | |||||||
\widehat{\vartheta }=1.758 | 1.003 | |||||||
PITL | \widehat{\alpha }=1.313 | 0.322 | 78.66 | 83.32 | 85.46 | 83.77 | 0.1182 | 0.7961 |
\widehat{\vartheta }=1.974 | 0.365 | |||||||
GIE | \widehat{\alpha }=3.300 | 1.065 | 79.32 | 83.89 | 86.13 | 84.81 | 0.1266 | 0.7224 |
\widehat{\lambda }=2.199 | 0.433 | |||||||
Burr XII | \widehat{\vartheta }=3.216 | 0.645 | 80.55 | 84.55 | 87.36 | 85 | 0.1287 | 0.7033 |
\widehat{\beta }=0.563 | 0.137 | |||||||
in-Ga | \widehat{\lambda }=2.596 | 0.631 | 80.61 | 85.61 | 87.42 | 85.06 | 0.1375 | 0.6218 |
\widehat{\beta }=2.966 | 0.795 | |||||||
in-We | \widehat{\alpha }=1.550 | 0.198 | 83.83 | 87.83 | 90.64 | 88.28 | 0.1523 | 0.4896 |
\widehat{\lambda }=1.025 | 0.203 |
The second dataset represents the survival times (in days) of 72 guinea pigs infected with virulent tubercle bacilli. The above dataset was first recorded in [33] and was recently used to fit the inverse Weibull distribution by reference [34] under Type-II right censoring. Reference [35] also used it to fit extended inverse power Lomax under Type-I right censoring.
We checked whether the TPITL distribution is suitable for analyzing this dataset. We reported the MLEs of the parameters and the values of the –2log L, AIC, BIC, and the K–S test statistics to judge the goodness of fit with comparison to PITL, log-normal (log-N), Weibull (We), generalized exponential (Ge-Ex), and extended inverse exponential (EIE) distributions. The lower the values of these criteria, the better the fit. In Table 14, MLEs, SE, and some measures are obtained. Plots of estimated PDF and CDF are provided in Figure 8. The PP plots of estimated densities are given in Figure 9. It can be seen that the TPITL provides the overall best fit based on Tables 13 and 14. The R codes of data analysis are provided in Appendix B.
Model | MLE | SE | -2LogL | AIC | BIC | CAIC | K-S | P-value |
TPITL | \hat{v}=-0.794 | 0.193 | 194.002 | 198.002 | 202.610 | 198.171 | 0.089 | 0.601 |
\hat{\delta}=1.640 | 0.270 | |||||||
\hat{\vartheta}=2.010 | 0.407 | |||||||
Ge-Ex | \hat{\alpha}=3.565 | 0.696 | 195.184 | 200.889 | 205.498 | 201.026 | 0.090 | 0.588 |
\hat{\lambda}=0.891 | 0.103 | |||||||
We | \hat{\eta}=1.820 | 0.156 | 196.889 | 201.184 | 208.096 | 201.558 | 0.100 | 0.448 |
\hat{\beta}=1.989 | 0.134 | |||||||
log-N | \hat{\mu}=0.393 | 0.073 | 200.565 | 204.565 | 209.174 | 204.734 | 0.101 | 0.437 |
\hat{\sigma}=0.632 | 0.052 | |||||||
PITL | \hat{\alpha}=1.142 | 0.189 | 200.604 | 204.604 | 209.213 | 204.774 | 0.118 | 0.254 |
\hat{\vartheta}=2.154 | 0.274 | |||||||
EIE | \hat{\alpha}=2.858 | 0.586 | 221.525 | 225.525 | 230.133 | 225.694 | 0.145 | 0.072 |
\hat{\lambda}=2.082 | 0.271 |
Figures 6 and 7 demonstrate that, among the two datasets, the TPITL model provided an excellent fit to the observed distribution (i.e., to the histogram). Also, we conclude that from these figures, the TPITL model offered the best agreement to the empirical CDF for the two datasets. Figures 8 and 9 also show that the TPITL model matches the two real-world datasets more closely than the other competing models. Consequently, the TPITL distribution can be chosen as a suitable model when compared with other distributions for explaining the studied data.
The QRTM is used to suggest a novel extension of the power inverted Topp–Leone distribution in this article. The developed distribution is known as the TPITL distribution. The PITL and ITL are included in the proposed distribution as specific models. The significant characteristics of the provided model, including quantile function, moments and incomplete moments, SO, and some uncertainty measures, are also discussed. The ASPs are created for the TPITL model, assuming the life test is terminated at a specific period. The median lifetime of the TPITL distribution with pre-specified variables is used to calculate the truncation time. The smallest sample size is necessary to get the claimed life test at a given consumer risk. Different estimating approaches are used to analyze the TPITL distribution characteristics. To assess the efficacy of estimations based on precision criteria, a comprehensive simulation research is conducted.
Regarding the simulation outcomes, it should be noted that the ML estimation approach often outperforms other methods. In some cases, the next best performing estimator is MPSE, followed by LSE. Finally, we observed that MSE decreases across all estimation techniques as the sample size grows, indicating that all estimation approaches are consistent. Two real data analyses are used to compare the proposed model's validity and adaptability to the alternative models, such as the PITL, log-normal, Weibull, GIE, inverse Weibull, inverse gamma, Ge-Ex, and EIE models. In the future, we will examine the statistical inference of this suggested model using Bayesian estimates under various censored schemes. Other researchers can employ this model in the future to investigate its statistical inference using Bayesian and E-Bayesian estimations under various complete and censored schemes.
The authors would like to thank the Deanship of Scientific Research at Umm Al-Qura University for supporting this work by Grant Code: (22UQU4340290DSR02). Additionally, the authors thank the editor and the reviewers for their careful reading of the research article and for their constructive comments that greatly improved this paper. The authors also thank all those who help in creating or revising this work. Thanks, is also extended to Enago® Office for proofreading and editing the paper. The authors extend their appreciation to the Deanship of Scientific Research at Umm Al-Qura University for supporting and funding this work this work by Grant Code: (22UQU4340290DSR02).
All authors declare no conflicts of interest in this paper.
######## Compute sampling plan and probability of acceptance
accept < - function(pstar, v, delta, vartheta, a, c)
{
######## Compute median life time from quantile function (t_0)
m0 < - t_0(0.5, v, delta, vartheta)
######## Compute truncation time at a
t0 < - a*m0
######## Compute probability (p) from cdf
p0 < - Fx(t0, v, delta, vartheta)
######## Determine optimal sample size (n)
for(n0 in 1:10^9)
{
s = 0
l_p = 0
for(i in 0:c)
{
s = s+choose(n0, i)*p0^i*(1-p0)^(n0-i)
l_p = l_p+choose((n0-1), i)*p0^i*(1-p0)^((n0-1)-i)
}
######## print minimum sample size
if (s < = 1-pstar)
{
print(c(c = c, n = n0, OC = l_p))
return(c(c, n0, l_p))
break()
}
}
}
######## Define pdf and cdf of PITL distribution
Pdf_PITL < - function(parm, x){
alpha < - parm[1]
theta < - parm[2]
a < - 2*alpha*theta*x^(2*theta-1)
b < - (1+x^theta)^(-2*alpha-1)
c < - (1+2*x^theta)^(alpha-1)
Pdf_PITL < - a*b*c
}
Cdf_PITL < - function(parm, x){
alpha < - parm[1]
theta < - parm[2]
a < - (1+x^theta)^(2*alpha)
b < - (1+2*x^theta)^alpha
Cdf_PITL < - 1-(b/a)
}
######## define pdf and cdf of inv. Weibull (invWe) Distribution
Pdf_inWE < - function(parm, x){
lambda < - parm[1]
beta < - parm[2]
Pdf_inWE < - lambda*beta*x^(-beta-1)*exp(-lambda/x^beta)
}
Cdf_inWE < - function(parm, x){
lambda < - parm[1]
beta < - parm[2]
Cdf_inWE < - exp(-lambda/x^beta)
}
######## define pdf and cdf for inverse gamma (invGa) Distribution
Pdf_inGa < - function(parm, x){
alpha < - parm[1]
lambda < - parm[2]
Pdf_inGa < - dinvgamma(x, alpha, rate = lambda)
}
Cdf_inGa < - function(parm, x){
alpha < - parm[1]
lambda < - parm[2]
Cdf_inGa < - pinvgamma(x, alpha, rate = lambda)
}
######## define pdf and cdf for Weibull Distribution
Pdf_We < - function(parm, x){
alpha < - parm[1]
lambda < - parm[2]
Pdf_We < - (alpha*x^(alpha-1)*exp(-(x/lambda)^alpha))/lambda^alpha
}
Cdf_We < - function(parm, x){
alpha < - parm[1]
lambda < - parm[2]
Cdf_We < - 1-exp(-(x/lambda)^alpha)
}
######## define pdf and cdf for log-Normal Distribution
Pdf_log < - function(parm, x){
alpha < - parm[1]
lambda < - parm[2]
Pdf_log < - dlnorm(x, alpha, lambda)
}
Cdf_log < - function(parm, x){
alpha < - parm[1]
lambda < - parm[2]
Cdf_log < - plnorm(x, alpha, lambda)
}
######## define pdf and cdf for Gamma Distribution
Pdf_Ga < - function(parm, x){
alpha < - parm[1]
lambda < - parm[2]
Pdf_Ga < - dgamma(x, shape = alpha, scale = lambda)
}
Cdf_Ga < - function(parm, x){
alpha < - parm[2]
lambda < - parm[1]
Cdf_Ga < - pgamma(x, shape = alpha, scale = lambda)
}
######## define pdf and cdf for Generalized Exponential Distribution
Pdf_Gex < - function(parm, x){
alpha < - parm[1]
lambda < - parm[2]
Pdf_Gex < - (alpha/lambda)*exp(-x/lambda)*(1-exp(-x/lambda))^(alpha-1)
}
Cdf_Gex < - function(parm, x){
alpha < - parm[1]
lambda < - parm[2]
Cdf_Gex < - (1-exp(-x/lambda))^alpha
}
######## define pdf and cdf for Generalized inverted Exponential (GIE) Distribution
Pdf_GIE < - function(parm, x){
alpha < - parm[1]
lambda < - parm[2]
Pdf_GIE < - (alpha*lambda/x^2)*exp(-lambda/x)*(1-exp(-lambda/x))^(alpha-1)
}
Cdf_GIE < - function(parm, x){
alpha < - parm[1]
lambda < - parm[2]
Cdf_GIE < - 1-(1-exp(-lambda/x))^alpha
}
######## define pdf and cdf for Extendend inverse exponential (EIE) Distribution
Pdf_EIE < - function(parm, x){
alpha < - parm[1]
theta < - parm[2]
a < - exp(-theta/x)
Pdf_EIE < - ((alpha*theta)/x^2)*a*(1/(1-a)^2)*exp(-alpha*(a/(1-a)))
}
Cdf_EIE < - function(parm, x){
alpha < - parm[1]
theta < - parm[2]
a < - exp(-theta/x)
Cdf_EIE < - 1-exp(-alpha*(a/(1-a)))
}
######## Define TPITL distribution
Pdf_TPITL < - function(parm, x){
v < - parm[1]
delta < - parm[2]
vartheta < - parm[3]
a = (1+2*x^delta)^vartheta
b = (1+x^delta)^(2*vartheta)
d = (1-(a/b))
aa = 2*vartheta*delta*x^(2*delta-1)*(1+x^delta)^(-2*vartheta-1)*(1+2*x^delta)^(vartheta-1)
Pdf_TPITL < - aa*(1+v-2*v*d)
}
Cdf_TPITL < - function(parm, x){
v < - parm[1]
delta < - parm[2]
vartheta < - parm[3]
a = (1+2*x^delta)^vartheta
b = (1+x^delta)^(2*vartheta)
d = (1-(a/b))
Cdf_TPITL < - d*(1+v-v*(d))
}
######## define pdf and cdf of Burr XII dist.
Pdf_Burr < - function(parm, x){
theta < - parm[1]
beta < - parm[2]
Pdf_Burr < - theta*beta*x^(theta-1)*(1+x^theta)^(-(beta+1))
}
Cdf_Burr < - function(parm, x){
theta < - parm[1]
beta < - parm[2]
Cdf_Burr < - 1-(1+x^theta)^(-beta)
}
######## log-likhood
LL_PITL < - function(alpha, theta){-sum(log(Pdf_PITL(c(alpha, theta), x)))}
LL_inWE < - function(lambda, beta){-sum(log(Pdf_inWE(c(lambda, beta), x)))}
LL_inGa < - function(alpha, lambda){-sum(log(Pdf_inGa(c(alpha, lambda), x)))}
LL_GIE < - function(alpha, lambda){-sum(log(Pdf_GIE(c(alpha, lambda), x)))}
LL_EIE < - function(alpha, theta){-sum(log(Pdf_EIE(c(alpha, theta), x)))}
LL_TPITL < - function(v, delta, vartheta){-sum(log(Pdf_TPITL(c(v, delta, vartheta), x)))}
LL_Burr < - function(theta, beta){-sum(log(Pdf_Burr(c(theta, beta), x)))}
LL_We < - function(alpha, lambda){-sum(log(Pdf_We(c(alpha, lambda), x)))}
LL_Ga < - function(alpha, lambda){-sum(log(Pdf_Ga(c(alpha, lambda), x)))}
LL_Gex < - function(alpha, lambda){-sum(log(Pdf_Gex(c(alpha, lambda), x)))}
LL_log < - function(alpha, lambda){-sum(log(Pdf_log(c(alpha, lambda), x)))}
########################################################################
######## Real data set I: -------------------------------------------------------------
# The data contain 30 observations of the March precipitation (in inches) in Minneapolis/St Paul
######## D. Hinkley, "On quick choice of power transformation, " Applied Statistics, vol. 26, no. 1,
X1 = c(0.77, 1.74, 0.81, 1.20, 1.95, 1.2, 0.47, 1.43, 3.37, 2.2, 3, 3.09, 1.51, 2.1, 0.52, 1.62, 1.31, 0.32, 0.59, 0.81, 2.81, 1.87, 1.18, 1.35, 4.75, 2.48, 0.96, 1.89, 0.9, 2.05)
######## Real data set II: -------------------------------------------------------------
######## The survival times (in days) of 72 guinea pigs infected with virulent tubercle bacilli,
# observed and reported by Bjerkedal (1960).
X2 = c(0.1, 0.33, 0.44, 0.56, 0.59, 0.59, 0.72, 0.74, 0.92, 0.93, 0.96, 1, 1, 1.02, 1.05, 1.07, 1.07, 1.08, 1.08, 1.08, 1.09, 1.12, 1.13, 1.15, 1.16, 1.2, 1.21, 1.22, 1.22, 1.24, 1.3, 1.34, 1.36, 1.39, 1.44, 1.46, 1.53, 1.59, 1.6, 1.63, 1.63, 1.68, 1.71, 1.72, 1.76, 1.83, 1.95, 1.96, 1.97, 2.02, 2.13, 2.15, 2.16, 2.22, 2.3, 2.31, 2.4, 2.45, 2.51, 2.53, 2.54, 2.54, 2.78, 2.93, 3.27, 3.42, 3.47, 3.61, 4.02, 4.32, 4.58, 5.55, 2.54, 0.77)
######## Use one data set:
x = X1
######## obtain MLE
fit_TPITL < - mle2(minuslog = LL_TPITL, start = list(v = 1, delta = 0.6, vartheta = 0.05),
data = list(x), method = "Nelder-Mead")
fit_invWe < - mle2(minuslog = LL_inWE, start = list(lambda = 1, beta = 1),
data = list(x), method = "Nelder-Mead")
fit_invGa < - mle2(minuslog = LL_inGa, start = list(alpha = 1, lambda = 3),
data = list(x), method = "Nelder-Mead")
fit_GIE < - mle2(minuslog = LL_GIE, start = list(alpha = 1, lambda = 1),
data = list(x), method = "Nelder-Mead")
fit_EIE < - mle2(minuslog = LL_EIE, start = list(alpha = 1, theta = 1),
data = list(x), method = "Nelder-Mead")
fit_Burr < - mle2(minuslog = LL_Burr, start = list(theta = 1, beta = 1),
data = list(x), method = "Nelder-Mead")
fit_We < - mle2(minuslog = LL_We, start = list(alpha = 1, lambda = 1),
data = list(x), method = "Nelder-Mead")
fit_Ga < - mle2(minuslog = LL_Ga, start = list(alpha = 1, lambda = 1),
data = list(x), method = "Nelder-Mead")
fit_Gex < - mle2(minuslog = LL_Gex, start = list(alpha = 1, lambda = 1),
data = list(x), method = "Nelder-Mead")
fit_log < - mle2(minuslog = LL_log, start = list(alpha = 1, lambda = 1),
data = list(x), method = "Nelder-Mead")
################################################################
######## goodness of fit
gof.test_TPITL < - goodness.fit(Pdf_TPITL, Cdf_TPITL, starts = c(coef(fit_TPITL)), data = x,
method = "N", domain = c(0, 1000))
gof.test_invWE < - goodness.fit(Pdf_inWE, Cdf_inWE, starts = c(coef(fit_invWe)), data = x,
method = "N", domain = c(0, 10000))
gof.test_invGa < - goodness.fit(Pdf_inGa, Cdf_inGa, starts = c(coef(fit_invGa)), data = x,
lim_inf = c(0.001, 0.001), lim_sup = c(10, 10))
gof.test_GIE < - goodness.fit(Pdf_GIE, Cdf_GIE, starts = c(coef(fit_GIE)), data = x,
lim_inf = c(0.001, 0.001), lim_sup = c(10, 20))
gof.test_EIE < - goodness.fit(Pdf_EIE, Cdf_EIE, starts = c(coef(fit_EIE)), data = x,
lim_inf = c(0.00001, 0.00001), lim_sup = c(10, 10))
gof.test_Burr < - goodness.fit(Pdf_Burr, Cdf_Burr, starts = c(coef(fit_Burr)), data = x,
lim_inf = c(0.001, 0.001), lim_sup = c(10, 10))
gof.test_We < - goodness.fit(Pdf_We, Cdf_We, starts = c(coef(fit_We)), data = x,
lim_inf = c(0.001, 0.001), lim_sup = c(10, 10))
gof.test_Ga < - goodness.fit(Pdf_Ga, Cdf_Ga, starts = c(coef(fit_Ga)), data = x,
lim_inf = c(0.001, 0.001), lim_sup = c(10, 10))
gof.test_Gex < - goodness.fit(Pdf_Gex, Cdf_Gex, starts = c(coef(fit_Gex)), data = x,
lim_inf = c(0.001, 0.001), lim_sup = c(10, 10))
gof.test_log < - goodness.fit(Pdf_log, Cdf_log, starts = c(coef(fit_log)), data = x,
lim_inf = c(0.001, 0.001), lim_sup = c(10, 10))
[1] | W. T. Shaw, I. R. C. Buckley, The alchemy of probability distributions: Beyond Gram–Charlier expansions, and a skew-kurtotic normal distribution from a rank transmutation map, preprint, arXiv: 0901.0434. |
[2] |
M. S. Khan, R. King, I. L. Hudson, Transmuted Weibull distribution: properties and estimation, Commun. Stat. Theory Methods, 46 (2017), 5394–5418. https://doi.org/10.1080/03610926.2015.1100744 doi: 10.1080/03610926.2015.1100744
![]() |
[3] |
Z. M. Nofal, A. Z. Afify, H. M. Yousof, G. M. Cordeiro, The generalized transmuted-G family of distributions, Commun. Stat. Theory Methods, 46 (2017), 4119–4136. https://doi.org/10.1080/03610926.2015.1078478 doi: 10.1080/03610926.2015.1078478
![]() |
[4] |
M. Elgarhy, I. Elbatal, A. S. Hassan, Transmuted Kumaraswamy quasi-Lindley distribution with applications, Ann. Data Sci., 5 (2018), 565–581. https://doi.org/10.1007/s40745-018-0153-4 doi: 10.1007/s40745-018-0153-4
![]() |
[5] |
H. D. Biçer, Properties and inference for a new class of XGamma distributions with an application, Math. Sci., 13 (2019), 335–346. https://doi.org/10.1007/s40096-019-00303-x doi: 10.1007/s40096-019-00303-x
![]() |
[6] | A. S. Hassan, S. M. Assar, A. M. Abd Elghaffar, Bayesian estimation of power transmuted inverse Rayleigh distributions, Thailand Stat., 19 (2021), 393–410. |
[7] |
A. S. Hassan, M. A. Khaleel, S. G. Nassr, Transmuted Topp–Leone power function distribution: Theory and application, J. Stat. Appl. Probab., 10 (2021), 215–227. https://doi.org/10.18576/jsap/100120 doi: 10.18576/jsap/100120
![]() |
[8] | A. Shafiq, S. A. Lone, T. N. Sindhu, Y. E. Khatib, Q. M. Al-Mdallal, T. Muhammad, A new modified Kies Fréchet distribution: Applications of mortality rate of COVID-19, Results Phys., 28 (2021), 104638, 1–17. https://doi.org/10.1016/j.rinp.2021.104638 |
[9] |
A. W. Marshall, I. Olkin, A new method for adding a parameter to a family of distributions with applications to the exponential and Weibull families, Biometrika, 84 (1997), 641–652. https://doi.org/10.1093/biomet/84.3.641 doi: 10.1093/biomet/84.3.641
![]() |
[10] | O. Nave, V. Gol'dshtein, A combination of two semi-analytical method called "singular perturbed homotopy analysis method, (SPHAM)" applied to combustion of spray fuel droplets, Cogent Math., 3 (2016), 1256467. https://doi.org/10.1080/23311835.2016.1256467 |
[11] | A. Algarni, A. M. Almarashi, I. Elbatal, A. S. Hassan, E. M. Almetwally, A. M. Daghistani, et al., Type I half lLogistic Burr X-G family: Properties, Bayesian, and non-Bayesian estimation under censored samples and applications to COVID-19 data, Math. Probl. Eng., 2021 (2021), 1–21. https://doi.org/10.1155/2021/5461130 |
[12] |
A. S. Hassan, M. A. H. Sabry, A. M. Elsehetry, A new probability distribution family arising from truncated power Lomax distribution with application to Weibull model, Pak. J. Stat. Oper. Res., 16 (2020), 661–674. https://doi.org/10.18187/pjsor.v16i4.3442 doi: 10.18187/pjsor.v16i4.3442
![]() |
[13] | A. S. Hassan, A. W. Shawkia, H. Z. Muhammeda, Weighted Weibull-G family of distributions: Theory and application in the analysis of renewable energy sources, J. Posit. Sch. Psychol., 6 (2022), 9201–9216. |
[14] | A. S. Hassan, A. Al-Omari, R. R. Hassan, G. A. Alomani, The odd inverted Topp Leone–H family of distributions: Estimation and applications, J. Radiat. Res. Appl. Sci., 2022. https://doi.org/10.1016/j.jrras.2022.08.006 |
[15] |
A. Shafiq, T. N. Sindhu, N. Alotaibi, A novel extended model with versatile shaped failure rate: Statistical inference with Covid-19 applications, Results Phys., 3 (2022), 105398. https://doi.org/10.1016/j.rinp.2022.105398 doi: 10.1016/j.rinp.2022.105398
![]() |
[16] |
A. S. Hassan, M. Elgarhy, R. Ragab, Statistical properties and estimation of inverted Topp–Leone distribution, J. Stat. Appl. Probab., 9 (2020), 319–331. https://doi.org/10.18576/jsap/090212 doi: 10.18576/jsap/090212
![]() |
[17] |
G. M. Ibrahim, A. S. Hassan, E. M. Almetwally, H. M. Almongy, Parameter estimation of alpha power inverted Topp–Leone distribution with applications, Intell. Autom. Soft Comput., 29 (2021), 353–371. https://doi.org/10.32604/iasc.2021.017586 doi: 10.32604/iasc.2021.017586
![]() |
[18] |
A. S. Hassan, E. M. Almetwally, G. M. Ibrahim, Kumaraswamy inverted Topp–Leone distribution with applications to COVID-19 data, Comput. Mater. Contin., 68 (2021), 337–358. https://doi.org/10.32604/cmc.2021.013971 doi: 10.32604/cmc.2021.013971
![]() |
[19] |
R. Bantan, M. Elsehetry, A. S. Hassan, M. Elgarhy, D. Sharma, C. Chesneau, et al., A two-parameter model: Properties and estimation under ranked sampling, MDPI Math., 9 (2021), 1214. https://doi.org/10.3390/math9111214 doi: 10.3390/math9111214
![]() |
[20] |
E. M. Almetwally, R. Alharbi, D. Alnagar, E. H. Hafez, A new inverted Topp-Leone distribution: Applications to the COVID-19 mortality rate in two different countries, Axioms, 10 (2021), 25. https://doi.org/10.3390/axioms10010025 doi: 10.3390/axioms10010025
![]() |
[21] |
E. M. Almetwally, The odd Weibull inverse Topp–Leone distribution with applications to COVID-19 data, Ann. Data Sci., 9 (2022), 121–140. https://doi.org/10.1007/s40745-021-00329-w doi: 10.1007/s40745-021-00329-w
![]() |
[22] |
T. A. Abushal, A. S. Hassan, A. R. El-Saeed, S. G. Nassr, Power inverted Topp–Leone distribution in acceptance sampling plans, Comput. Mater. Contin., 67 (2021), 991–1011. https://doi.org/10.32604/cmc.2021.014620 doi: 10.32604/cmc.2021.014620
![]() |
[23] | E. Lehmann, Elements of Large-sample Theory, Springer-Verlag, New York, 1999. https://doi.org/10.1007/b98855 |
[24] |
J. J. Swain, S. Venkatraman, J. R. Wilson, Least squares estimation of distribution function in Johnson's system, J. Stat. Comput. Simul., 29 (1988), 271–297. https://doi.org/10.1080/00949658808811068 doi: 10.1080/00949658808811068
![]() |
[25] |
R. C. H. Cheng, N. A. K. Amin, Estimating parameters in continuous univariate distributions with a shifted origin, J. R. Stat. Soc., 45 (1983), 394–403. https://doi.org/10.1111/j.2517-6161.1983.tb01268.x doi: 10.1111/j.2517-6161.1983.tb01268.x
![]() |
[26] |
P. D. M. MacDonald, Comment on "An estimation procedure for mixtures of distributions" by Choi and Bulgren, J. R. Stat. Soc. Series B (Methodological), 33 (1971), 326–329. https://doi.org/10.1111/j.2517-6161.1971.tb00884.x doi: 10.1111/j.2517-6161.1971.tb00884.x
![]() |
[27] | A. Rényi, On measures of entropy and information, in Proceedings of the 4th Berkeley Symposium on Mathematical Statistics and Probability, 1 (1960), 547–561. |
[28] |
C. Tsallis, Possible generalization of Boltzmann–Gibbs statistics, J. Stat. Phys., 52 (1988), 479–487. https://doi.org/10.1007/BF01016429 doi: 10.1007/BF01016429
![]() |
[29] |
S. Arimoto, Information-theoretical considerations on estimation problems, Inf. Control, 19 (1971), 181–194. https://doi.org/10.1016/S0019-9958(71)90065-9 doi: 10.1016/S0019-9958(71)90065-9
![]() |
[30] | J. Havrda, F. Charvat, Quantification method of classification processes, concept of structural a-entropy, Kybernetika, 3 (1967), 30–35 |
[31] |
S. Singh, Y. M. Tripathi, Acceptance sampling plans for inverse Weibull distribution based on truncated life test, Life Cycle Reliab. Saf. Eng., 6 (2017), 169–178. https://doi.org/10.1007/s41872-017-0022-8 doi: 10.1007/s41872-017-0022-8
![]() |
[32] |
D. Hinkley, On quick choice of power transformation, Appl. Stat., 26 (1977), 67–69. https://doi.org/10.2307/2346869 doi: 10.2307/2346869
![]() |
[33] |
T. Bjerkedal, Acquisition of resistance in guinea pigs infected with different doses of virulent tubercle bacilli, Am. J. Hyg., 72 (1960), 130–148. https://doi.org/10.1093/oxfordjournals.aje.a120129 doi: 10.1093/oxfordjournals.aje.a120129
![]() |
[34] |
D. Kundu, H. Howlader, Bayesian inference and prediction of the inverse Weibull distribution for Type-II censored data, Comput. Stat. Data Anal., 54 (2010), 1547–1558. https://doi.org/10.1016/j.csda.2010.01.003 doi: 10.1016/j.csda.2010.01.003
![]() |
[35] |
A. S. Hassan, S. G. Nassr, Parameter estimation of an extended inverse power Lomax distribution with Type I right censored data, Commun. Stat. Appl. Methods, 28 (2021), 99–118. https://doi.org/10.29220/CSAM.2021.28.2.099 doi: 10.29220/CSAM.2021.28.2.099
![]() |
1. | Osama H. Mahmoud Hassan, Ibrahim Elbatal, Abdullah H. Al-Nefaie, Ahmed R. El-Saeed, Statistical Inference of the Beta Binomial Exponential 2 Distribution with Application to Environmental Data, 2022, 11, 2075-1680, 740, 10.3390/axioms11120740 | |
2. | Ali Hussein AL-Marshadi, Muhammad Aslam, Abdullah Alharbey, Design of double acceptance sampling plan for Weibull distribution under indeterminacy, 2023, 8, 2473-6988, 13294, 10.3934/math.2023672 | |
3. | Najwan Alsadat, Amal S. Hassan, Mohammed Elgarhy, Christophe Chesneau, Ahmed R. El-Saeed, Sampling Plan for the Kavya–Manoharan Generalized Inverted Kumaraswamy Distribution with Statistical Inference and Applications, 2023, 12, 2075-1680, 739, 10.3390/axioms12080739 | |
4. | Ahmed R. El-Saeed, Amal S. Hassan, Neema M. Elharoun, Aned Al Mutairi, Rana H. Khashab, Said G. Nassr, A class of power inverted Topp-Leone distribution: Properties, different estimation methods & applications, 2023, 16, 16878507, 100643, 10.1016/j.jrras.2023.100643 | |
5. | Said G. Nassr, Amal S. Hassan, Ehab M. Almetwally, Aned Al Mutairi, Rana H. Khashab, Neema M. ElHaroun, Statistical inference of the inverted exponentiated Lomax distribution using generalized order statistics with application to COVID-19, 2023, 13, 2158-3226, 10.1063/5.0174540 | |
6. | Fatehi Yahya Eissa, Chhaya Dhanraj Sonar, Osama Abdulaziz Alamri, Ahlam H. Tolba, Statistical Inferences about Parameters of the Pseudo Lindley Distribution with Acceptance Sampling Plans, 2024, 13, 2075-1680, 443, 10.3390/axioms13070443 | |
7. | Huda M. Alshanbari, Gadde Srinivasa Rao, Jin-Taek Seong, Sultan Salem, Saima K. Khosa, A New Cosine-Originated Probability Distribution with Symmetrical and Asymmetrical Behaviors: Repetitive Acceptance Sampling with Reliability Application, 2023, 15, 2073-8994, 2187, 10.3390/sym15122187 | |
8. | Amani S. Alghamdi, Lulah Alnaji, Hilary Izuchukwu Okagbue, A new extended Chen distribution for modelling COVID-19 data, 2025, 20, 1932-6203, e0316235, 10.1371/journal.pone.0316235 |
\stackrel{´}{{\mu }_{m}} | \delta =7 \upsilon =-0.3 |
\delta =7 \upsilon =0.3 |
\delta =9 \upsilon =0.5 |
\delta =9 \upsilon =-0.5 |
\delta =7 \upsilon =-0.5 |
\delta =7 \upsilon =0.5 |
\stackrel{´}{{\mu }_{1}} | 1.567 | 1.389 | 1.236 | 1.439 | 1.626 | 1.33 |
\stackrel{´}{{\mu }_{2}} | 3.056 | 2.327 | 1.657 | 2.318 | 3.299 | 2.084 |
\stackrel{´}{{\mu }_{3}} | 11.183 | 6.932 | 2.603 | 4.584 | 12.6 | 5.515 |
\stackrel{´}{{\mu }_{4}} | 392.029 | 212.752 | 6.807 | 16.324 | 451.896 | 152.346 |
{\sigma }^{2} | 0.601 | 0.397 | 0.131 | 0.247 | 0.655 | 0.315 |
Sw | 9.669 | 10.388 | 4.904 | 4.377 | 9.618 | 10.8 |
Ku | 964.521 | 1206 | 124.816 | 22.875 | 934.164 | 1371 |
\tau | \left(\vartheta , \delta , \upsilon \right) | RE | TE | AE | H-CE |
0.5 | (0.5, 3, 0.3) | 3.28 | 8.308 | 25.563 | 61.716 |
(1, 3, 0.3) | 1.535 | 2.308 | 3.64 | 8.789 | |
(1.5, 3, 0.3) | 1.028 | 1.345 | 1.797 | 4.337 | |
(2, 3, 0.3) | 0.76 | 0.924 | 1.138 | 2.746 | |
(0.5, 5, –0.3) | 1.944 | 3.286 | 5.985 | 14.448 | |
(1, 5, –0.3) | 0.876 | 1.1 | 1.402 | 3.384 | |
(1.5, 5, –0.3) | 0.483 | 0.546 | 0.62 | 1.497 | |
(2, 5, –0.3) | 0.263 | 0.281 | 0.301 | 0.726 | |
2 | (0.5, 3, 0.3) | 1.163 | 0.687 | 0.882 | 0.882 |
(1, 3, 0.3) | 0.615 | 0.46 | 0.53 | 0.53 | |
(1.5, 3, 0.3) | 0.353 | 0.297 | 0.323 | 0.323 | |
(2, 3, 0.3) | 0.188 | 0.172 | 0.18 | 0.18 | |
(0.5, 5, –0.3) | 1.504 | 0.778 | 1.057 | 1.057 | |
(1, 5, –0.3) | 0.834 | 0.566 | 0.682 | 0.682 | |
(1.5, 5, –0.3) | 0.517 | 0.404 | 0.456 | 0.456 | |
(2, 5, –0.3) | 0.32 | 0.274 | 0.296 | 0.296 |
{p}^{*} | c | a=0.2 | a=0.4 | a=0.6 | a=0.8 | a=1 | |||||
n | L\left({p}_{0}\right) | n | L\left({p}_{0}\right) | n | L\left({p}_{0}\right) | n | L\left({p}_{0}\right) | n | L\left({p}_{0}\right) | ||
0.2 | 0 | 1 | 1.0000 | 1 | 1.0000 | 1 | 1.0000 | 1 | 1.0000 | 1 | 1.0000 |
2 | 6 | 0.8346 | 5 | 0.8354 | 4 | 0.9162 | 4 | 0.8941 | 4 | 0.8750 | |
4 | 11 | 0.8468 | 9 | 0.8457 | 8 | 0.8627 | 8 | 0.8152 | 7 | 0.8906 | |
10 | 29 | 0.8030 | 23 | 0.8085 | 20 | 0.8438 | 19 | 0.8256 | 18 | 0.8338 | |
0.4 | 0 | 2 | 0.6983 | 2 | 0.6130 | 1 | 1.0000 | 1 | 1.0000 | 1 | 1.0000 |
2 | 8 | 0.6432 | 6 | 0.7047 | 6 | 0.6158 | 5 | 0.7268 | 5 | 0.6875 | |
4 | 14 | 0.6491 | 11 | 0.6652 | 10 | 0.6504 | 9 | 0.6939 | 9 | 0.6367 | |
10 | 33 | 0.6359 | 26 | 0.6373 | 23 | 0.6486 | 21 | 0.6797 | 20 | 0.6762 | |
0.6 | 0 | 3 | 0.4876 | 2 | 0.6130 | 2 | 0.5624 | 2 | 0.5270 | 2 | 0.5000 |
2 | 10 | 0.4582 | 8 | 0.4484 | 7 | 0.4667 | 6 | 0.5504 | 6 | 0.5000 | |
4 | 17 | 0.4439 | 13 | 0.4753 | 12 | 0.4300 | 11 | 0.4448 | 10 | 0.5000 | |
10 | 38 | 0.4151 | 29 | 0.4539 | 26 | 0.4330 | 24 | 0.4387 | 23 | 0.4159 | |
0.8 | 0 | 5 | 0.2377 | 4 | 0.2303 | 3 | 0.3163 | 3 | 0.2777 | 3 | 0.2500 |
2 | 13 | 0.2487 | 10 | 0.2570 | 9 | 0.2419 | 8 | 0.2731 | 8 | 0.2266 | |
4 | 21 | 0.2324 | 16 | 0.2487 | 14 | 0.2562 | 13 | 0.2501 | 12 | 0.2744 | |
10 | 44 | 0.2077 | 34 | 0.2099 | 30 | 0.2071 | 27 | 0.2408 | 26 | 0.2122 | |
0.99 | 0 | 13 | 0.0134 | 10 | 0.0122 | 9 | 0.0100 | 8 | 0.0113 | 7 | 0.0156 |
2 | 25 | 0.0114 | 19 | 0.0109 | 16 | 0.0136 | 15 | 0.0111 | 14 | 0.0112 | |
4 | 35 | 0.0110 | 26 | 0.0132 | 23 | 0.0113 | 21 | 0.0113 | 19 | 0.0154 | |
10 | 62 | 0.0107 | 47 | 0.0114 | 41 | 0.0113 | 37 | 0.0135 | 35 | 0.0122 |
{p}^{*} | c | a=0.2 | a=0.4 | a=0.6 | a=0.8 | a=1 | |||||
n | L\left({p}_{0}\right) | n | L\left({p}_{0}\right) | n | L\left({p}_{0}\right) | n | L\left({p}_{0}\right) | n | L\left({p}_{0}\right) | ||
0.2 | 0 | 1 | 1.0000 | 1 | 1.0000 | 1 | 1.0000 | 1 | 1.0000 | 1 | 1.0000 |
2 | 8 | 0.8058 | 5 | 0.8890 | 5 | 0.8148 | 4 | 0.9037 | 4 | 0.8750 | |
4 | 15 | 0.8099 | 10 | 0.8553 | 9 | 0.8183 | 8 | 0.8360 | 7 | 0.8906 | |
10 | 38 | 0.8055 | 26 | 0.8223 | 22 | 0.8127 | 19 | 0.8563 | 18 | 0.8338 | |
0.4 | 0 | 3 | 0.6001 | 2 | 0.6668 | 1 | 1.0000 | 1 | 1.0000 | 1 | 1.0000 |
2 | 11 | 0.6005 | 7 | 0.6806 | 6 | 0.6737 | 5 | 0.7473 | 5 | 0.6875 | |
4 | 19 | 0.6174 | 13 | 0.6318 | 11 | 0.6202 | 10 | 0.6016 | 9 | 0.6367 | |
10 | 44 | 0.6278 | 30 | 0.6362 | 25 | 0.6308 | 22 | 0.6507 | 20 | 0.6762 | |
0.6 | 0 | 4 | 0.4649 | 3 | 0.4446 | 2 | 0.5949 | 2 | 0.5417 | 2 | 0.5000 |
2 | 14 | 0.4118 | 9 | 0.4685 | 8 | 0.4088 | 7 | 0.4248 | 6 | 0.5000 | |
4 | 23 | 0.4261 | 16 | 0.4044 | 13 | 0.4237 | 11 | 0.4828 | 10 | 0.5000 | |
10 | 51 | 0.4092 | 34 | 0.4354 | 28 | 0.4368 | 25 | 0.4214 | 23 | 0.4159 | |
0.8 | 0 | 7 | 0.2161 | 4 | 0.2964 | 4 | 0.2105 | 3 | 0.2934 | 3 | 0.2500 |
2 | 18 | 0.2274 | 12 | 0.2343 | 10 | 0.2223 | 9 | 0.2062 | 8 | 0.2266 | |
4 | 29 | 0.2108 | 19 | 0.2314 | 16 | 0.2056 | 14 | 0.2100 | 12 | 0.2744 | |
10 | 59 | 0.2126 | 39 | 0.2313 | 32 | 0.2275 | 28 | 0.2357 | 26 | 0.2122 | |
0.99 | 0 | 19 | 0.0101 | 12 | 0.0116 | 9 | 0.0157 | 8 | 0.0137 | 7 | 0.0156 |
2 | 34 | 0.0121 | 22 | 0.0129 | 18 | 0.0111 | 15 | 0.0146 | 14 | 0.0112 | |
4 | 48 | 0.0109 | 31 | 0.0123 | 25 | 0.0118 | 22 | 0.0104 | 19 | 0.0154 | |
10 | 85 | 0.0101 | 55 | 0.0124 | 45 | 0.0104 | 39 | 0.0109 | 35 | 0.0122 |
p* | c | a=0.2 | a=0.4 | a=0.6 | a=0.8 | a=1 | |||||
n | L(p0) | n | L(p0) | n | L(p0) | n | L(p0) | n | L(p0) | ||
0.2 | 0 | 5 | 0.8277 | 2 | 0.8220 | 1 | 1.0000 | 1 | 1.0000 | 1 | 1.0000 |
2 | 34 | 0.8059 | 9 | 0.8432 | 6 | 0.8221 | 4 | 0.9270 | 4 | 0.8750 | |
4 | 68 | 0.8032 | 18 | 0.8285 | 11 | 0.8303 | 8 | 0.8854 | 7 | 0.8906 | |
10 | 178 | 0.8031 | 47 | 0.8163 | 28 | 0.8108 | 21 | 0.8344 | 18 | 0.8339 | |
0.4 | 0 | 11 | 0.6234 | 3 | 0.6757 | 2 | 0.6890 | 1 | 1.0000 | 1 | 1.0000 |
2 | 50 | 0.6045 | 13 | 0.6370 | 8 | 0.6221 | 6 | 0.6512 | 5 | 0.6875 | |
4 | 90 | 0.6072 | 24 | 0.6093 | 14 | 0.6210 | 10 | 0.6950 | 9 | 0.6367 | |
10 | 214 | 0.6045 | 56 | 0.6126 | 32 | 0.6386 | 24 | 0.6498 | 20 | 0.6762 | |
0.6 | 0 | 20 | 0.4074 | 5 | 0.4566 | 3 | 0.4748 | 2 | 0.5821 | 2 | 0.5000 |
2 | 67 | 0.4073 | 17 | 0.4386 | 10 | 0.4339 | 7 | 0.5073 | 6 | 0.5000 | |
4 | 113 | 0.4064 | 29 | 0.4263 | 17 | 0.4121 | 12 | 0.4836 | 10 | 0.5000 | |
10 | 249 | 0.4037 | 64 | 0.4207 | 37 | 0.4098 | 27 | 0.4468 | 23 | 0.4159 | |
0.8 | 0 | 35 | 0.2005 | 9 | 0.2085 | 5 | 0.2254 | 3 | 0.3389 | 3 | 0.2500 |
2 | 92 | 0.2033 | 23 | 0.2225 | 13 | 0.2274 | 9 | 0.2792 | 8 | 0.2266 | |
4 | 145 | 0.2011 | 37 | 0.2072 | 21 | 0.2062 | 15 | 0.2352 | 12 | 0.2744 | |
10 | 294 | 0.2030 | 75 | 0.2118 | 42 | 0.2269 | 31 | 0.2271 | 26 | 0.2122 | |
0.99 | 0 | 98 | 0.0102 | 24 | 0.0110 | 13 | 0.0115 | 9 | 0.0132 | 7 | 0.0156 |
2 | 179 | 0.0103 | 44 | 0.0115 | 24 | 0.0120 | 17 | 0.0129 | 14 | 0.0112 | |
4 | 248 | 0.0102 | 62 | 0.0104 | 34 | 0.0107 | 24 | 0.0124 | 19 | 0.0154 | |
10 | 432 | 0.0101 | 108 | 0.0110 | 60 | 0.0107 | 43 | 0.0119 | 35 | 0.0122 |
{p}^{*} | c | a=0.2 | a=0.4 | a=0.6 | a=0.8 | a=1 | |||||
n | L\left({p}_{0}\right) | n | L\left({p}_{0}\right) | n | L\left({p}_{0}\right) | n | n | L\left({p}_{0}\right) | n | ||
0.2 | 0 | 1 | 1.0000 | 1 | 1.0000 | 1 | 1.0000 | 1 | 1.0000 | 1 | 1.0000 |
2 | 7 | 0.8405 | 5 | 0.8776 | 5 | 0.8063 | 4 | 0.9018 | 4 | 0.8750 | |
4 | 14 | 0.8097 | 10 | 0.8354 | 9 | 0.8067 | 8 | 0.8317 | 7 | 0.8906 | |
10 | 35 | 0.8142 | 25 | 0.8284 | 21 | 0.8469 | 19 | 0.8502 | 18 | 0.8338 | |
0.4 | 0 | 2 | 0.7564 | 2 | 0.6543 | 1 | 1.0000 | 1 | 1.0000 | 1 | 1.0000 |
2 | 10 | 0.6185 | 7 | 0.6557 | 6 | 0.6610 | 5 | 0.7430 | 5 | 0.6875 | |
4 | 17 | 0.6530 | 12 | 0.6795 | 11 | 0.6019 | 9 | 0.7174 | 9 | 0.6367 | |
10 | 41 | 0.6205 | 29 | 0.6344 | 25 | 0.6030 | 22 | 0.6399 | 20 | 0.6762 | |
0.6 | 0 | 4 | 0.4327 | 3 | 0.4281 | 2 | 0.5877 | 2 | 0.5386 | 2 | 0.5000 |
2 | 13 | 0.4107 | 9 | 0.4381 | 7 | 0.5188 | 7 | 0.4186 | 6 | 0.5000 | |
4 | 21 | 0.4409 | 15 | 0.4361 | 13 | 0.4036 | 11 | 0.4747 | 10 | 0.5000 | |
10 | 47 | 0.4152 | 33 | 0.4246 | 28 | 0.4068 | 25 | 0.4093 | 23 | 0.4159 | |
0.8 | 0 | 6 | 0.2476 | 4 | 0.2801 | 4 | 0.2030 | 3 | 0.2901 | 3 | 0.2500 |
2 | 17 | 0.2135 | 12 | 0.2085 | 10 | 0.2093 | 9 | 0.2011 | 8 | 0.2266 | |
4 | 27 | 0.2046 | 18 | 0.2463 | 15 | 0.2484 | 14 | 0.2035 | 12 | 0.2744 | |
10 | 55 | 0.2022 | 38 | 0.2163 | 32 | 0.2037 | 28 | 0.2258 | 26 | 0.2122 | |
0.99 | 0 | 17 | 0.0115 | 11 | 0.0144 | 9 | 0.0142 | 8 | 0.0131 | 7 | 0.0156 |
2 | 32 | 0.0103 | 21 | 0.0134 | 17 | 0.0144 | 15 | 0.0138 | 14 | 0.0112 | |
4 | 44 | 0.0113 | 30 | 0.0114 | 24 | 0.0142 | 21 | 0.0147 | 19 | 0.0154 | |
10 | 78 | 0.0105 | 53 | 0.0119 | 44 | 0.0107 | 38 | 0.0138 | 35 | 0.0122 |
{p}^{*} | c | a=0.2 | a=0.4 | a=0.6 | a=0.8 | a=1 | |||||
n | L\left({p}_{0}\right) | n | L\left({p}_{0}\right) | n | L\left({p}_{0}\right) | n | L\left({p}_{0}\right) | n | L\left({p}_{0}\right) | ||
0.2 | 0 | 2 | 0.8116 | 1 | 1.0000 | 1 | 1.0000 | 1 | 1.0000 | 1 | 1.0000 |
2 | 9 | 0.8220 | 6 | 0.8359 | 5 | 0.8398 | 4 | 0.9104 | 4 | 0.8750 | |
4 | 17 | 0.8319 | 11 | 0.8484 | 9 | 0.8515 | 8 | 0.8504 | 7 | 0.8906 | |
10 | 45 | 0.8064 | 29 | 0.8062 | 23 | 0.8192 | 20 | 0.8219 | 18 | 0.8338 | |
0.4 | 0 | 3 | 0.6588 | 2 | 0.6992 | 2 | 0.6170 | 1 | 1.0000 | 1 | 1.0000 |
2 | 12 | 0.6560 | 8 | 0.6453 | 6 | 0.7115 | 5 | 0.7619 | 5 | 0.6875 | |
4 | 22 | 0.6392 | 14 | 0.6520 | 11 | 0.6751 | 10 | 0.6275 | 9 | 0.6367 | |
10 | 53 | 0.6120 | 33 | 0.6404 | 26 | 0.6530 | 23 | 0.6131 | 20 | 0.6762 | |
0.6 | 0 | 5 | 0.4340 | 3 | 0.4889 | 2 | 0.6170 | 2 | 0.5526 | 2 | 0.5000 |
2 | 16 | 0.4429 | 10 | 0.4608 | 8 | 0.4574 | 7 | 0.4467 | 6 | 0.5000 | |
4 | 28 | 0.4058 | 17 | 0.4472 | 14 | 0.4014 | 12 | 0.4039 | 10 | 0.5000 | |
10 | 61 | 0.4084 | 38 | 0.4200 | 30 | 0.4142 | 25 | 0.4640 | 23 | 0.4159 | |
0.8 | 0 | 8 | 0.2320 | 5 | 0.2390 | 4 | 0.2349 | 3 | 0.3053 | 3 | 0.2500 |
2 | 22 | 0.2147 | 14 | 0.2008 | 10 | 0.2651 | 9 | 0.2245 | 8 | 0.2266 | |
4 | 35 | 0.2059 | 21 | 0.2352 | 17 | 0.2035 | 14 | 0.2337 | 12 | 0.2744 | |
10 | 71 | 0.2089 | 44 | 0.2116 | 34 | 0.2239 | 29 | 0.2215 | 26 | 0.2122 | |
0.99 | 0 | 23 | 0.0101 | 13 | 0.0137 | 10 | 0.0130 | 8 | 0.0157 | 7 | 0.0156 |
2 | 42 | 0.0105 | 25 | 0.0116 | 19 | 0.0119 | 16 | 0.0112 | 14 | 0.0112 | |
4 | 58 | 0.0110 | 35 | 0.0114 | 27 | 0.0105 | 22 | 0.0134 | 19 | 0.0154 | |
10 | 102 | 0.0108 | 62 | 0.0111 | 48 | 0.0101 | 40 | 0.0112 | 35 | 0.0122 |
{p}^{*} | c | a=0.2 | a=0.4 | a=0.6 | a=0.8 | a=1 | |||||
n | L\left({p}_{0}\right) | n | L\left({p}_{0}\right) | n | L\left({p}_{0}\right) | n | L\left({p}_{0}\right) | n | L\left({p}_{0}\right) | ||
0.2 | 0 | 19 | 0.8043 | 3 | 0.8513 | 2 | 0.8010 | 1 | 1.0000 | 1 | 1.0000 |
2 | 128 | 0.8027 | 21 | 0.8019 | 8 | 0.8536 | 5 | 0.8740 | 4 | 0.8750 | |
4 | 258 | 0.8008 | 41 | 0.8058 | 17 | 0.8012 | 10 | 0.8291 | 7 | 0.8906 | |
10 | 680 | 0.8003 | 107 | 0.8034 | 42 | 0.8220 | 25 | 0.8180 | 18 | 0.8338 | |
0.4 | 0 | 43 | 0.6016 | 7 | 0.6169 | 3 | 0.6416 | 2 | 0.6505 | 1 | 1.0000 |
2 | 190 | 0.6028 | 30 | 0.6086 | 12 | 0.6206 | 7 | 0.6481 | 5 | 0.6875 | |
4 | 345 | 0.6020 | 54 | 0.6087 | 21 | 0.6339 | 12 | 0.6696 | 9 | 0.6367 | |
10 | 821 | 0.6006 | 128 | 0.6061 | 50 | 0.6182 | 29 | 0.6182 | 20 | 0.6762 | |
0.6 | 0 | 76 | 0.4036 | 12 | 0.4125 | 5 | 0.4116 | 3 | 0.4231 | 2 | 0.5000 |
2 | 258 | 0.4018 | 40 | 0.4103 | 16 | 0.4017 | 9 | 0.4290 | 6 | 0.5000 | |
4 | 435 | 0.4017 | 68 | 0.4010 | 26 | 0.4255 | 15 | 0.4243 | 10 | 0.5000 | |
10 | 957 | 0.4012 | 149 | 0.4010 | 58 | 0.4019 | 33 | 0.4070 | 23 | 0.4159 | |
0.8 | 0 | 134 | 0.2001 | 20 | 0.2166 | 8 | 0.2115 | 4 | 0.2752 | 3 | 0.2500 |
2 | 355 | 0.2011 | 55 | 0.2017 | 21 | 0.2091 | 12 | 0.2011 | 8 | 0.2266 | |
4 | 558 | 0.2007 | 86 | 0.2043 | 33 | 0.2082 | 18 | 0.2361 | 12 | 0.2744 | |
10 | 1134 | 0.2003 | 175 | 0.2037 | 67 | 0.2113 | 38 | 0.2025 | 26 | 0.2122 | |
0.99 | 0 | 381 | 0.0101 | 58 | 0.0102 | 21 | 0.0118 | 11 | 0.0136 | 7 | 0.0156 |
2 | 696 | 0.0101 | 106 | 0.0103 | 39 | 0.0117 | 21 | 0.0123 | 14 | 0.0112 | |
4 | 962 | 0.0100 | 147 | 0.0101 | 55 | 0.0105 | 30 | 0.0103 | 19 | 0.0154 | |
10 | 1671 | 0.0100 | 256 | 0.0101 | 96 | 0.0112 | 53 | 0.0103 | 35 | 0.0122 |
n | Parameters | MLE | LSE | WLSE | MPSE | CvME | |||||
MSE | SE | MSE | SE | MSE | SE | MSE | SE | MSE | SE | ||
20 | \delta =0.75 | 0.0634 | 0.0512 | 0.0462 | 0.0471 | 0.0321 | 0.0392 | 0.0318 | 0.0385 | 0.0457 | 0.0476 |
\vartheta =0.25 | 4.2070* | 0.0104 | 5.9938* | 0.0168 | 3.7685* | 0.0135 | 1.8682* | 9.6702* | 5.7922* | 0.0166 | |
\upsilon =-0.25 | 0.0563 | 3.5521* | 0.1807 | 0.0249 | 0.1933 | 0.0222 | 0.2111 | 0.0204 | 0.2275 | 0.0281 | |
30 | \delta =0.75 | 0.0316 | 0.0295 | 0.0327 | 0.0309 | 0.0242 | 0.0270 | 0.0193 | 0.0248 | 0.0328 | 0.0321 |
\vartheta =0.25 | 3.5439* | 7.0577* | 3.0803* | 9.8808* | 2.7990* | 9.6063* | 1.0694* | 5.5847* | 4.3042* | 0.0118 | |
\upsilon =-0.25 | 0.0558 | 2.0435* | 0.1807 | 0.0176 | 0.1915 | 0.0147 | 0.1973 | 0.0116 | 0.2110 | 0.0199 | |
50 | \delta =0.75 | 0.0317 | 0.0213 | 0.0162 | 0.0174 | 0.0101 | 0.0140 | 0.0157 | 0.0165 | 0.0162 | 0.0178 |
\vartheta =0.25 | 3.8108* | 4.7838* | 1.1283* | 4.7452* | 0.5372* | 3.0750* | 1.1926* | 3.7336* | 1.1844* | 4.8565* | |
\upsilon =-0.25 | 0.0561 | 1.3275* | 0.1649 | 8.5725* | 0.1800 | 5.9981* | 0.1874 | 5.1355* | 0.1824 | 8.9142* | |
75 | \delta =0.75 | 0.0400 | 0.0202 | 0.0101 | 0.0104 | 6.1135* | 8.6267* | 0.0106 | 0.0112 | 8.3405* | 9.6595* |
\vartheta =0.25 | 3.6780* | 3.5785* | 0.4110* | 2.3153* | 0.3427* | 1.8551* | 1.0702* | 2.6413* | 0.3617* | 2.1469* | |
\upsilon =-0.25 | 0.0555 | 1.4264* | 0.1659 | 5.9550* | 0.1788 | 3.7775* | 0.1838 | 3.2474* | 0.1763 | 5.9837* | |
100 | \delta =0.75 | 0.0194 | 0.0113 | 7.9590* | 7.7147* | 5.3663* | 6.9967* | 8.3169* | 8.5896* | 7.3736* | 7.4625* |
\vartheta =0.25 | 3.1715* | 2.4667* | 0.4031* | 1.9978* | 0.3735* | 1.7288* | 0.9853* | 2.0216* | 0.3708* | 1.9209* | |
\upsilon =-0.25 | 0.0558 | 0.7247* | 0.1641 | 4.0990* | 0.1780 | 2.5736* | 0.1811 | 2.2216* | 0.1713 | 4.2937* | |
150 | \delta =0.75 | 0.0174 | 8.6607* | 4.7108* | 4.4809* | 3.5370* | 4.4433* | 4.2869* | 5.3664* | 4.3355* | 4.4987* |
\vartheta =0.25 | 3.0945* | 1.8702* | 0.2082* | 1.1216* | 0.2407* | 0.8882* | 0.6736* | 1.4636* | 0.2130* | 1.1105* | |
\upsilon =-0.25 | 0.0557 | 0.4741* | 0.1543 | 3.4320* | 0.1758 | 1.6379* | 0.1533 | 1.7328* | 0.1703 | 3.1378* |
n | Parameters | MLE | LSE | WLSE | MPSE | CvME | |||||
MSE | SE | MSE | SE | MSE | SE | MSE | SE | MSE | SE | ||
20 | \delta =1.5 | 0.5272 | 0.1158 | 0.2419 | 0.0810 | 0.2248 | 0.0707 | 0.4151 | 0.1004 | 0.3123 | 0.0825 |
\vartheta =0.5 | 0.0346 | 0.0142 | 0.0184 | 6.5972* | 0.0190 | 5.5531* | 0.0256 | 0.0107 | 0.0205 | 6.0177* | |
\upsilon =-0.25 | 0.0375 | 9.2001* | 0.4592 | 0.0446 | 0.4543 | 0.0345 | 0.3840 | 0.0235 | 0.5443 | 0.0447 | |
30 | \delta =1.5 | 0.4394 | 0.0833 | 0.1267 | 0.0477 | 0.1531 | 0.0502 | 0.2508 | 0.0594 | 0.1548 | 0.0482 |
\vartheta =0.5 | 0.0334 | 0.0100 | 0.0138 | 6.2299* | 0.0146 | 5.9041* | 0.0205 | 8.3477* | 0.0144 | 5.2513* | |
\upsilon =-0.25 | 0.0336 | 0.0101 | 0.4306 | 0.0254 | 0.4516 | 0.0219 | 0.4126 | 0.0164 | 0.4962 | 0.0278 | |
50 | \delta =1.5 | 0.4512 | 0.0672 | 0.1199 | 0.0348 | 0.1158 | 0.0321 | 0.1586 | 0.0376 | 0.1261 | 0.0324 |
\vartheta =0.5 | 0.0356 | 8.5213* | 0.0144 | 4.0590* | 0.0142 | 3.7567* | 0.0190 | 4.9860* | 0.0146 | 3.3391* | |
\upsilon =-0.25 | 0.0353 | 6.0854* | 0.4172 | 0.0154 | 0.4337 | 0.0122 | 0.3878 | 9.5065* | 0.4603 | 0.0164 | |
75 | \delta =1.5 | 0.4150 | 0.0512 | 0.1116 | 0.0226 | 0.1003 | 0.0193 | 0.1555 | 0.0300 | 0.1234 | 0.0216 |
\vartheta =0.5 | 0.0351 | 6.0012* | 0.0140 | 2.7917* | 0.0135 | 2.6442* | 0.0201 | 4.4084* | 0.0146 | 2.4824* | |
\upsilon =-0.25 | 0.0358 | 4.3681* | 0.4250 | 0.0103 | 0.4419 | 8.0752* | 0.3809 | 6.4523* | 0.4510 | 0.0106 | |
100 | \delta =1.5 | 0.4328 | 0.0426 | 0.1056 | 0.0171 | 0.0920 | 0.0172 | 0.1647 | 0.0282 | 0.1011 | 0.0153 |
\vartheta =0.5 | 0.0363 | 5.5815* | 0.0136 | 2.3123* | 0.0121 | 2.7129* | 0.0189 | 4.3207* | 0.0131 | 1.8163* | |
\upsilon =-0.25 | 0.0339 | 3.6981* | 0.4570 | 7.3339* | 0.4564 | 5.6197* | 0.3973 | 5.2128* | 0.4761 | 7.1705* | |
150 | \delta =1.5 | 0.3750 | 0.0254 | 0.0874 | 6.4564* | 0.0663 | 6.6803* | 0.1223 | 0.0146 | 0.0980 | 7.1989* |
\vartheta =0.5 | 0.0333 | 3.0071* | 0.0134 | 1.1206* | 0.0121 | 1.2057* | 0.0189 | 3.0867* | 0.0135 | 1.1276* | |
\upsilon =-0.25 | 0.0336 | 1.9440* | 0.4335 | 2.6577* | 0.4322 | 1.7794* | 0.3768 | 2.8150* | 0.4433 | 2.4982* |
n | Parameters | MLE | LSE | WLSE | MPSE | CvME | |||||
MSE | SE | MSE | SE | MSE | SE | MSE | SE | MSE | SE | ||
20 | \delta =0.75 | 0.0276 | 0.0320 | 0.0650 | 0.0400 | 0.0521 | 0.0350 | 0.0312 | 0.0370 | 0.0656 | 0.0420 |
\vartheta =0.25 | 2.9819* | 9.6410* | 8.3113* | 0.0200 | 6.9943* | 0.0180 | 2.1731* | 0.0100 | 0.0109 | 0.0220 | |
\upsilon =-0.75 | 0.5469 | 1.3110* | 0.9473 | 0.0340 | 0.9252 | 0.0260 | 0.8917 | 0.0210 | 1.0580 | 0.0370 | |
30 | \delta =0.75 | 0.0240 | 0.0250 | 0.0523 | 0.0240 | 0.0435 | 0.0250 | 0.0217 | 0.0250 | 0.0539 | 0.0260 |
\vartheta =0.25 | 3.4648* | 7.9550* | 5.8641* | 0.0140 | 5.8410* | 0.0140 | 2.2441* | 6.4740* | 6.4372* | 0.0140 | |
\upsilon =-0.75 | 0.5458 | 1.4640* | 0.9186 | 0.0210 | 0.9199 | 0.0190 | 0.8715 | 0.0150 | 0.9848 | 0.0230 | |
50 | \delta =0.75 | 0.0173 | 0.0170 | 0.0441 | 0.0170 | 0.0377 | 0.0170 | 0.0129 | 0.0140 | 0.0452 | 0.0180 |
\vartheta =0.25 | 3.8093* | 5.0600* | 3.6101* | 8.5390* | 4.1827* | 9.1340* | 2.3664* | 3.4760* | 3.8059* | 8.7550* | |
\upsilon =-0.75 | 0.5438 | 0.9780* | 0.8972 | 0.0110 | 0.8821 | 8.3640* | 0.8377 | 6.2060* | 0.9392 | 0.0110 | |
75 | \delta =0.75 | 0.0238 | 0.0157 | 0.0410 | 0.0140 | 0.0307 | 0.0130 | 9.5963* | 9.3015* | 0.0413 | 0.0148 |
\vartheta =0.25 | 2.9710* | 4.3074* | 3.6810* | 7.0170* | 3.2999* | 6.5592* | 1.9662* | 2.2798* | 4.7127* | 7.9185* | |
\upsilon =-0.75 | 0.5459 | 0.6425* | 0.9595 | 8.5172* | 0.8979 | 5.5828* | 0.8356 | 3.6097* | 0.9863 | 8.7994* | |
100 | \delta =0.75 | 0.0227 | 0.0134 | 0.0298 | 0.0100 | 0.0137 | 7.9828* | 4.3208* | 5.2667* | 0.0297 | 9.1020* |
\vartheta =0.25 | 2.3568* | 4.0206* | 2.8294* | 5.5969* | 1.5028* | 2.2740* | 1.5569* | 1.4654* | 2.5345* | 5.3057* | |
\upsilon =-0.75 | 0.5416 | 0.6058* | 0.9242 | 5.0194* | 0.8670 | 2.3480* | 0.8194 | 3.1607* | 0.9360 | 5.1240* | |
150 | \delta =0.75 | 9.1118* | 2.7224* | 0.0249 | 5.3565* | 8.5464* | 7.9564* | 4.1972* | 4.5636* | 0.0241 | 5.8262* |
\vartheta =0.25 | 1.7642* | 0.9286* | 1.5173* | 1.8264* | 0.8638* | 0.4991* | 1.0472* | 0.9626* | 1.0430* | 2.3932* | |
\upsilon =-0.75 | 0.5329 | 1.0329* | 0.9163 | 5.0756* | 0.8485 | 1.7051* | 0.7717 | 1.7450* | 0.9272 | 4.3014* |
n | Parameters | MLE | LSE | WLSE | MPSE | CvME | |||||
MSE | SE | MSE | SE | MSE | SE | MSE | SE | MSE | SE | ||
20 | \delta =1.5 | 0.3166 | 0.1074 | 0.0752 | 0.0611 | 0.0688 | 0.0584 | 0.1069 | 0.0688 | 0.0855 | 0.0628 |
\vartheta =0.5 | 0.0371 | 0.0136 | 0.0161 | 7.9040* | 0.0156 | 8.2771* | 0.0170 | 0.0104 | 0.0172 | 7.9114* | |
\upsilon =-0.75 | 0.4460 | 0.0347 | 1.4360 | 0.0421 | 1.4374 | 0.0389 | 1.3799 | 0.0355 | 1.6197 | 0.0471 | |
30 | \delta =1.5 | 0.2908 | 0.0852 | 0.0450 | 0.0385 | 0.0401 | 0.0359 | 0.0525 | 0.0419 | 0.0446 | 0.0385 |
\vartheta =0.5 | 0.0361 | 0.0105 | 0.0136 | 5.8095* | 0.0121 | 5.5192* | 0.0125 | 7.1990* | 0.0141 | 5.2089* | |
\upsilon =-0.75 | 0.4180 | 0.0143 | 1.4336 | 0.0260 | 1.4175 | 0.0237 | 1.3529 | 0.0213 | 1.5567 | 0.0286 | |
50 | \delta =1.5 | 0.2901 | 0.0662 | 0.0281 | 0.0235 | 0.0269 | 0.0219 | 0.0337 | 0.0256 | 0.0273 | 0.0234 |
\vartheta =0.5 | 0.0352 | 7.9137* | 0.0134 | 3.6008* | 0.0111 | 3.5586* | 0.0121 | 5.2926* | 0.0138 | 3.4227* | |
\upsilon =-0.75 | 0.4176 | 0.0131 | 1.4264 | 0.0148 | 1.3670 | 0.0112 | 1.2780 | 0.0106 | 1.4927 | 0.0155 | |
75 | \delta =1.5 | 0.1622 | 0.0418 | 0.0195 | 0.0158 | 0.0229 | 0.0153 | 0.0315 | 0.0192 | 0.0185 | 0.0157 |
\vartheta =0.5 | 0.0301 | 5.3795* | 0.0131 | 2.5415* | 0.0103 | 2.9067* | 0.0112 | 3.9476* | 0.0134 | 2.4295* | |
\upsilon =-0.75 | 0.4141 | 9.7076* | 1.4082 | 0.0101 | 1.3617 | 6.9812* | 1.2608 | 6.7749* | 1.4829 | 0.0101 | |
100 | \delta =1.5 | 0.2589 | 0.0436 | 0.0151 | 0.0122 | 0.0181 | 0.0107 | 0.0204 | 0.0132 | 0.0159 | 0.0126 |
\vartheta =0.5 | 0.0357 | 5.4308* | 0.0129 | 2.0247* | 9.3446* | 2.0512* | 0.0106 | 3.7361* | 0.0132 | 2.0816* | |
\upsilon =-0.75 | 0.4123 | 6.1602* | 1.3819 | 7.3598* | 1.3518 | 5.2508* | 1.2430 | 5.0394* | 1.4739 | 7.9705* | |
150 | \delta =1.5 | 0.1303 | 0.0258 | 0.0113 | 8.2565* | 0.0176 | 0.0105 | 0.0189 | 7.0110* | 0.0134 | 9.3264* |
\vartheta =0.5 | 0.0312 | 4.0948* | 0.0124 | 1.4042* | 7.8324* | 2.2745* | 8.8525* | 2.0483* | 0.0128 | 1.6189* | |
\upsilon =-0.75 | 0.4096 | 4.3745* | 1.3245 | 5.5974* | 1.3308 | 3.0557* | 1.2360 | 2.7320* | 1.4591 | 5.2175* | |
*Note: * Indicate that the value multiply {10}^{-3} in Tables 9–12. |
Model | MLE | SE | -2LogL | AIC | BIC | CAIC | K-S | P-value |
TPITL | \widehat{\upsilon }=-0.373 | 0.610 | 77.89 | 82.66 | 85.09 | 83.10 | 0.1069 | 0.8831 |
\widehat{\delta }=1.723 | 0.587 | |||||||
\widehat{\vartheta }=1.758 | 1.003 | |||||||
PITL | \widehat{\alpha }=1.313 | 0.322 | 78.66 | 83.32 | 85.46 | 83.77 | 0.1182 | 0.7961 |
\widehat{\vartheta }=1.974 | 0.365 | |||||||
GIE | \widehat{\alpha }=3.300 | 1.065 | 79.32 | 83.89 | 86.13 | 84.81 | 0.1266 | 0.7224 |
\widehat{\lambda }=2.199 | 0.433 | |||||||
Burr XII | \widehat{\vartheta }=3.216 | 0.645 | 80.55 | 84.55 | 87.36 | 85 | 0.1287 | 0.7033 |
\widehat{\beta }=0.563 | 0.137 | |||||||
in-Ga | \widehat{\lambda }=2.596 | 0.631 | 80.61 | 85.61 | 87.42 | 85.06 | 0.1375 | 0.6218 |
\widehat{\beta }=2.966 | 0.795 | |||||||
in-We | \widehat{\alpha }=1.550 | 0.198 | 83.83 | 87.83 | 90.64 | 88.28 | 0.1523 | 0.4896 |
\widehat{\lambda }=1.025 | 0.203 |
Model | MLE | SE | -2LogL | AIC | BIC | CAIC | K-S | P-value |
TPITL | \hat{v}=-0.794 | 0.193 | 194.002 | 198.002 | 202.610 | 198.171 | 0.089 | 0.601 |
\hat{\delta}=1.640 | 0.270 | |||||||
\hat{\vartheta}=2.010 | 0.407 | |||||||
Ge-Ex | \hat{\alpha}=3.565 | 0.696 | 195.184 | 200.889 | 205.498 | 201.026 | 0.090 | 0.588 |
\hat{\lambda}=0.891 | 0.103 | |||||||
We | \hat{\eta}=1.820 | 0.156 | 196.889 | 201.184 | 208.096 | 201.558 | 0.100 | 0.448 |
\hat{\beta}=1.989 | 0.134 | |||||||
log-N | \hat{\mu}=0.393 | 0.073 | 200.565 | 204.565 | 209.174 | 204.734 | 0.101 | 0.437 |
\hat{\sigma}=0.632 | 0.052 | |||||||
PITL | \hat{\alpha}=1.142 | 0.189 | 200.604 | 204.604 | 209.213 | 204.774 | 0.118 | 0.254 |
\hat{\vartheta}=2.154 | 0.274 | |||||||
EIE | \hat{\alpha}=2.858 | 0.586 | 221.525 | 225.525 | 230.133 | 225.694 | 0.145 | 0.072 |
\hat{\lambda}=2.082 | 0.271 |
\stackrel{´}{{\mu }_{m}} | \delta =7 \upsilon =-0.3 |
\delta =7 \upsilon =0.3 |
\delta =9 \upsilon =0.5 |
\delta =9 \upsilon =-0.5 |
\delta =7 \upsilon =-0.5 |
\delta =7 \upsilon =0.5 |
\stackrel{´}{{\mu }_{1}} | 1.567 | 1.389 | 1.236 | 1.439 | 1.626 | 1.33 |
\stackrel{´}{{\mu }_{2}} | 3.056 | 2.327 | 1.657 | 2.318 | 3.299 | 2.084 |
\stackrel{´}{{\mu }_{3}} | 11.183 | 6.932 | 2.603 | 4.584 | 12.6 | 5.515 |
\stackrel{´}{{\mu }_{4}} | 392.029 | 212.752 | 6.807 | 16.324 | 451.896 | 152.346 |
{\sigma }^{2} | 0.601 | 0.397 | 0.131 | 0.247 | 0.655 | 0.315 |
Sw | 9.669 | 10.388 | 4.904 | 4.377 | 9.618 | 10.8 |
Ku | 964.521 | 1206 | 124.816 | 22.875 | 934.164 | 1371 |
\tau | \left(\vartheta , \delta , \upsilon \right) | RE | TE | AE | H-CE |
0.5 | (0.5, 3, 0.3) | 3.28 | 8.308 | 25.563 | 61.716 |
(1, 3, 0.3) | 1.535 | 2.308 | 3.64 | 8.789 | |
(1.5, 3, 0.3) | 1.028 | 1.345 | 1.797 | 4.337 | |
(2, 3, 0.3) | 0.76 | 0.924 | 1.138 | 2.746 | |
(0.5, 5, –0.3) | 1.944 | 3.286 | 5.985 | 14.448 | |
(1, 5, –0.3) | 0.876 | 1.1 | 1.402 | 3.384 | |
(1.5, 5, –0.3) | 0.483 | 0.546 | 0.62 | 1.497 | |
(2, 5, –0.3) | 0.263 | 0.281 | 0.301 | 0.726 | |
2 | (0.5, 3, 0.3) | 1.163 | 0.687 | 0.882 | 0.882 |
(1, 3, 0.3) | 0.615 | 0.46 | 0.53 | 0.53 | |
(1.5, 3, 0.3) | 0.353 | 0.297 | 0.323 | 0.323 | |
(2, 3, 0.3) | 0.188 | 0.172 | 0.18 | 0.18 | |
(0.5, 5, –0.3) | 1.504 | 0.778 | 1.057 | 1.057 | |
(1, 5, –0.3) | 0.834 | 0.566 | 0.682 | 0.682 | |
(1.5, 5, –0.3) | 0.517 | 0.404 | 0.456 | 0.456 | |
(2, 5, –0.3) | 0.32 | 0.274 | 0.296 | 0.296 |
{p}^{*} | c | a=0.2 | a=0.4 | a=0.6 | a=0.8 | a=1 | |||||
n | L\left({p}_{0}\right) | n | L\left({p}_{0}\right) | n | L\left({p}_{0}\right) | n | L\left({p}_{0}\right) | n | L\left({p}_{0}\right) | ||
0.2 | 0 | 1 | 1.0000 | 1 | 1.0000 | 1 | 1.0000 | 1 | 1.0000 | 1 | 1.0000 |
2 | 6 | 0.8346 | 5 | 0.8354 | 4 | 0.9162 | 4 | 0.8941 | 4 | 0.8750 | |
4 | 11 | 0.8468 | 9 | 0.8457 | 8 | 0.8627 | 8 | 0.8152 | 7 | 0.8906 | |
10 | 29 | 0.8030 | 23 | 0.8085 | 20 | 0.8438 | 19 | 0.8256 | 18 | 0.8338 | |
0.4 | 0 | 2 | 0.6983 | 2 | 0.6130 | 1 | 1.0000 | 1 | 1.0000 | 1 | 1.0000 |
2 | 8 | 0.6432 | 6 | 0.7047 | 6 | 0.6158 | 5 | 0.7268 | 5 | 0.6875 | |
4 | 14 | 0.6491 | 11 | 0.6652 | 10 | 0.6504 | 9 | 0.6939 | 9 | 0.6367 | |
10 | 33 | 0.6359 | 26 | 0.6373 | 23 | 0.6486 | 21 | 0.6797 | 20 | 0.6762 | |
0.6 | 0 | 3 | 0.4876 | 2 | 0.6130 | 2 | 0.5624 | 2 | 0.5270 | 2 | 0.5000 |
2 | 10 | 0.4582 | 8 | 0.4484 | 7 | 0.4667 | 6 | 0.5504 | 6 | 0.5000 | |
4 | 17 | 0.4439 | 13 | 0.4753 | 12 | 0.4300 | 11 | 0.4448 | 10 | 0.5000 | |
10 | 38 | 0.4151 | 29 | 0.4539 | 26 | 0.4330 | 24 | 0.4387 | 23 | 0.4159 | |
0.8 | 0 | 5 | 0.2377 | 4 | 0.2303 | 3 | 0.3163 | 3 | 0.2777 | 3 | 0.2500 |
2 | 13 | 0.2487 | 10 | 0.2570 | 9 | 0.2419 | 8 | 0.2731 | 8 | 0.2266 | |
4 | 21 | 0.2324 | 16 | 0.2487 | 14 | 0.2562 | 13 | 0.2501 | 12 | 0.2744 | |
10 | 44 | 0.2077 | 34 | 0.2099 | 30 | 0.2071 | 27 | 0.2408 | 26 | 0.2122 | |
0.99 | 0 | 13 | 0.0134 | 10 | 0.0122 | 9 | 0.0100 | 8 | 0.0113 | 7 | 0.0156 |
2 | 25 | 0.0114 | 19 | 0.0109 | 16 | 0.0136 | 15 | 0.0111 | 14 | 0.0112 | |
4 | 35 | 0.0110 | 26 | 0.0132 | 23 | 0.0113 | 21 | 0.0113 | 19 | 0.0154 | |
10 | 62 | 0.0107 | 47 | 0.0114 | 41 | 0.0113 | 37 | 0.0135 | 35 | 0.0122 |
{p}^{*} | c | a=0.2 | a=0.4 | a=0.6 | a=0.8 | a=1 | |||||
n | L\left({p}_{0}\right) | n | L\left({p}_{0}\right) | n | L\left({p}_{0}\right) | n | L\left({p}_{0}\right) | n | L\left({p}_{0}\right) | ||
0.2 | 0 | 1 | 1.0000 | 1 | 1.0000 | 1 | 1.0000 | 1 | 1.0000 | 1 | 1.0000 |
2 | 8 | 0.8058 | 5 | 0.8890 | 5 | 0.8148 | 4 | 0.9037 | 4 | 0.8750 | |
4 | 15 | 0.8099 | 10 | 0.8553 | 9 | 0.8183 | 8 | 0.8360 | 7 | 0.8906 | |
10 | 38 | 0.8055 | 26 | 0.8223 | 22 | 0.8127 | 19 | 0.8563 | 18 | 0.8338 | |
0.4 | 0 | 3 | 0.6001 | 2 | 0.6668 | 1 | 1.0000 | 1 | 1.0000 | 1 | 1.0000 |
2 | 11 | 0.6005 | 7 | 0.6806 | 6 | 0.6737 | 5 | 0.7473 | 5 | 0.6875 | |
4 | 19 | 0.6174 | 13 | 0.6318 | 11 | 0.6202 | 10 | 0.6016 | 9 | 0.6367 | |
10 | 44 | 0.6278 | 30 | 0.6362 | 25 | 0.6308 | 22 | 0.6507 | 20 | 0.6762 | |
0.6 | 0 | 4 | 0.4649 | 3 | 0.4446 | 2 | 0.5949 | 2 | 0.5417 | 2 | 0.5000 |
2 | 14 | 0.4118 | 9 | 0.4685 | 8 | 0.4088 | 7 | 0.4248 | 6 | 0.5000 | |
4 | 23 | 0.4261 | 16 | 0.4044 | 13 | 0.4237 | 11 | 0.4828 | 10 | 0.5000 | |
10 | 51 | 0.4092 | 34 | 0.4354 | 28 | 0.4368 | 25 | 0.4214 | 23 | 0.4159 | |
0.8 | 0 | 7 | 0.2161 | 4 | 0.2964 | 4 | 0.2105 | 3 | 0.2934 | 3 | 0.2500 |
2 | 18 | 0.2274 | 12 | 0.2343 | 10 | 0.2223 | 9 | 0.2062 | 8 | 0.2266 | |
4 | 29 | 0.2108 | 19 | 0.2314 | 16 | 0.2056 | 14 | 0.2100 | 12 | 0.2744 | |
10 | 59 | 0.2126 | 39 | 0.2313 | 32 | 0.2275 | 28 | 0.2357 | 26 | 0.2122 | |
0.99 | 0 | 19 | 0.0101 | 12 | 0.0116 | 9 | 0.0157 | 8 | 0.0137 | 7 | 0.0156 |
2 | 34 | 0.0121 | 22 | 0.0129 | 18 | 0.0111 | 15 | 0.0146 | 14 | 0.0112 | |
4 | 48 | 0.0109 | 31 | 0.0123 | 25 | 0.0118 | 22 | 0.0104 | 19 | 0.0154 | |
10 | 85 | 0.0101 | 55 | 0.0124 | 45 | 0.0104 | 39 | 0.0109 | 35 | 0.0122 |
p* | c | a=0.2 | a=0.4 | a=0.6 | a=0.8 | a=1 | |||||
n | L(p0) | n | L(p0) | n | L(p0) | n | L(p0) | n | L(p0) | ||
0.2 | 0 | 5 | 0.8277 | 2 | 0.8220 | 1 | 1.0000 | 1 | 1.0000 | 1 | 1.0000 |
2 | 34 | 0.8059 | 9 | 0.8432 | 6 | 0.8221 | 4 | 0.9270 | 4 | 0.8750 | |
4 | 68 | 0.8032 | 18 | 0.8285 | 11 | 0.8303 | 8 | 0.8854 | 7 | 0.8906 | |
10 | 178 | 0.8031 | 47 | 0.8163 | 28 | 0.8108 | 21 | 0.8344 | 18 | 0.8339 | |
0.4 | 0 | 11 | 0.6234 | 3 | 0.6757 | 2 | 0.6890 | 1 | 1.0000 | 1 | 1.0000 |
2 | 50 | 0.6045 | 13 | 0.6370 | 8 | 0.6221 | 6 | 0.6512 | 5 | 0.6875 | |
4 | 90 | 0.6072 | 24 | 0.6093 | 14 | 0.6210 | 10 | 0.6950 | 9 | 0.6367 | |
10 | 214 | 0.6045 | 56 | 0.6126 | 32 | 0.6386 | 24 | 0.6498 | 20 | 0.6762 | |
0.6 | 0 | 20 | 0.4074 | 5 | 0.4566 | 3 | 0.4748 | 2 | 0.5821 | 2 | 0.5000 |
2 | 67 | 0.4073 | 17 | 0.4386 | 10 | 0.4339 | 7 | 0.5073 | 6 | 0.5000 | |
4 | 113 | 0.4064 | 29 | 0.4263 | 17 | 0.4121 | 12 | 0.4836 | 10 | 0.5000 | |
10 | 249 | 0.4037 | 64 | 0.4207 | 37 | 0.4098 | 27 | 0.4468 | 23 | 0.4159 | |
0.8 | 0 | 35 | 0.2005 | 9 | 0.2085 | 5 | 0.2254 | 3 | 0.3389 | 3 | 0.2500 |
2 | 92 | 0.2033 | 23 | 0.2225 | 13 | 0.2274 | 9 | 0.2792 | 8 | 0.2266 | |
4 | 145 | 0.2011 | 37 | 0.2072 | 21 | 0.2062 | 15 | 0.2352 | 12 | 0.2744 | |
10 | 294 | 0.2030 | 75 | 0.2118 | 42 | 0.2269 | 31 | 0.2271 | 26 | 0.2122 | |
0.99 | 0 | 98 | 0.0102 | 24 | 0.0110 | 13 | 0.0115 | 9 | 0.0132 | 7 | 0.0156 |
2 | 179 | 0.0103 | 44 | 0.0115 | 24 | 0.0120 | 17 | 0.0129 | 14 | 0.0112 | |
4 | 248 | 0.0102 | 62 | 0.0104 | 34 | 0.0107 | 24 | 0.0124 | 19 | 0.0154 | |
10 | 432 | 0.0101 | 108 | 0.0110 | 60 | 0.0107 | 43 | 0.0119 | 35 | 0.0122 |
{p}^{*} | c | a=0.2 | a=0.4 | a=0.6 | a=0.8 | a=1 | |||||
n | L\left({p}_{0}\right) | n | L\left({p}_{0}\right) | n | L\left({p}_{0}\right) | n | n | L\left({p}_{0}\right) | n | ||
0.2 | 0 | 1 | 1.0000 | 1 | 1.0000 | 1 | 1.0000 | 1 | 1.0000 | 1 | 1.0000 |
2 | 7 | 0.8405 | 5 | 0.8776 | 5 | 0.8063 | 4 | 0.9018 | 4 | 0.8750 | |
4 | 14 | 0.8097 | 10 | 0.8354 | 9 | 0.8067 | 8 | 0.8317 | 7 | 0.8906 | |
10 | 35 | 0.8142 | 25 | 0.8284 | 21 | 0.8469 | 19 | 0.8502 | 18 | 0.8338 | |
0.4 | 0 | 2 | 0.7564 | 2 | 0.6543 | 1 | 1.0000 | 1 | 1.0000 | 1 | 1.0000 |
2 | 10 | 0.6185 | 7 | 0.6557 | 6 | 0.6610 | 5 | 0.7430 | 5 | 0.6875 | |
4 | 17 | 0.6530 | 12 | 0.6795 | 11 | 0.6019 | 9 | 0.7174 | 9 | 0.6367 | |
10 | 41 | 0.6205 | 29 | 0.6344 | 25 | 0.6030 | 22 | 0.6399 | 20 | 0.6762 | |
0.6 | 0 | 4 | 0.4327 | 3 | 0.4281 | 2 | 0.5877 | 2 | 0.5386 | 2 | 0.5000 |
2 | 13 | 0.4107 | 9 | 0.4381 | 7 | 0.5188 | 7 | 0.4186 | 6 | 0.5000 | |
4 | 21 | 0.4409 | 15 | 0.4361 | 13 | 0.4036 | 11 | 0.4747 | 10 | 0.5000 | |
10 | 47 | 0.4152 | 33 | 0.4246 | 28 | 0.4068 | 25 | 0.4093 | 23 | 0.4159 | |
0.8 | 0 | 6 | 0.2476 | 4 | 0.2801 | 4 | 0.2030 | 3 | 0.2901 | 3 | 0.2500 |
2 | 17 | 0.2135 | 12 | 0.2085 | 10 | 0.2093 | 9 | 0.2011 | 8 | 0.2266 | |
4 | 27 | 0.2046 | 18 | 0.2463 | 15 | 0.2484 | 14 | 0.2035 | 12 | 0.2744 | |
10 | 55 | 0.2022 | 38 | 0.2163 | 32 | 0.2037 | 28 | 0.2258 | 26 | 0.2122 | |
0.99 | 0 | 17 | 0.0115 | 11 | 0.0144 | 9 | 0.0142 | 8 | 0.0131 | 7 | 0.0156 |
2 | 32 | 0.0103 | 21 | 0.0134 | 17 | 0.0144 | 15 | 0.0138 | 14 | 0.0112 | |
4 | 44 | 0.0113 | 30 | 0.0114 | 24 | 0.0142 | 21 | 0.0147 | 19 | 0.0154 | |
10 | 78 | 0.0105 | 53 | 0.0119 | 44 | 0.0107 | 38 | 0.0138 | 35 | 0.0122 |
{p}^{*} | c | a=0.2 | a=0.4 | a=0.6 | a=0.8 | a=1 | |||||
n | L\left({p}_{0}\right) | n | L\left({p}_{0}\right) | n | L\left({p}_{0}\right) | n | L\left({p}_{0}\right) | n | L\left({p}_{0}\right) | ||
0.2 | 0 | 2 | 0.8116 | 1 | 1.0000 | 1 | 1.0000 | 1 | 1.0000 | 1 | 1.0000 |
2 | 9 | 0.8220 | 6 | 0.8359 | 5 | 0.8398 | 4 | 0.9104 | 4 | 0.8750 | |
4 | 17 | 0.8319 | 11 | 0.8484 | 9 | 0.8515 | 8 | 0.8504 | 7 | 0.8906 | |
10 | 45 | 0.8064 | 29 | 0.8062 | 23 | 0.8192 | 20 | 0.8219 | 18 | 0.8338 | |
0.4 | 0 | 3 | 0.6588 | 2 | 0.6992 | 2 | 0.6170 | 1 | 1.0000 | 1 | 1.0000 |
2 | 12 | 0.6560 | 8 | 0.6453 | 6 | 0.7115 | 5 | 0.7619 | 5 | 0.6875 | |
4 | 22 | 0.6392 | 14 | 0.6520 | 11 | 0.6751 | 10 | 0.6275 | 9 | 0.6367 | |
10 | 53 | 0.6120 | 33 | 0.6404 | 26 | 0.6530 | 23 | 0.6131 | 20 | 0.6762 | |
0.6 | 0 | 5 | 0.4340 | 3 | 0.4889 | 2 | 0.6170 | 2 | 0.5526 | 2 | 0.5000 |
2 | 16 | 0.4429 | 10 | 0.4608 | 8 | 0.4574 | 7 | 0.4467 | 6 | 0.5000 | |
4 | 28 | 0.4058 | 17 | 0.4472 | 14 | 0.4014 | 12 | 0.4039 | 10 | 0.5000 | |
10 | 61 | 0.4084 | 38 | 0.4200 | 30 | 0.4142 | 25 | 0.4640 | 23 | 0.4159 | |
0.8 | 0 | 8 | 0.2320 | 5 | 0.2390 | 4 | 0.2349 | 3 | 0.3053 | 3 | 0.2500 |
2 | 22 | 0.2147 | 14 | 0.2008 | 10 | 0.2651 | 9 | 0.2245 | 8 | 0.2266 | |
4 | 35 | 0.2059 | 21 | 0.2352 | 17 | 0.2035 | 14 | 0.2337 | 12 | 0.2744 | |
10 | 71 | 0.2089 | 44 | 0.2116 | 34 | 0.2239 | 29 | 0.2215 | 26 | 0.2122 | |
0.99 | 0 | 23 | 0.0101 | 13 | 0.0137 | 10 | 0.0130 | 8 | 0.0157 | 7 | 0.0156 |
2 | 42 | 0.0105 | 25 | 0.0116 | 19 | 0.0119 | 16 | 0.0112 | 14 | 0.0112 | |
4 | 58 | 0.0110 | 35 | 0.0114 | 27 | 0.0105 | 22 | 0.0134 | 19 | 0.0154 | |
10 | 102 | 0.0108 | 62 | 0.0111 | 48 | 0.0101 | 40 | 0.0112 | 35 | 0.0122 |
{p}^{*} | c | a=0.2 | a=0.4 | a=0.6 | a=0.8 | a=1 | |||||
n | L\left({p}_{0}\right) | n | L\left({p}_{0}\right) | n | L\left({p}_{0}\right) | n | L\left({p}_{0}\right) | n | L\left({p}_{0}\right) | ||
0.2 | 0 | 19 | 0.8043 | 3 | 0.8513 | 2 | 0.8010 | 1 | 1.0000 | 1 | 1.0000 |
2 | 128 | 0.8027 | 21 | 0.8019 | 8 | 0.8536 | 5 | 0.8740 | 4 | 0.8750 | |
4 | 258 | 0.8008 | 41 | 0.8058 | 17 | 0.8012 | 10 | 0.8291 | 7 | 0.8906 | |
10 | 680 | 0.8003 | 107 | 0.8034 | 42 | 0.8220 | 25 | 0.8180 | 18 | 0.8338 | |
0.4 | 0 | 43 | 0.6016 | 7 | 0.6169 | 3 | 0.6416 | 2 | 0.6505 | 1 | 1.0000 |
2 | 190 | 0.6028 | 30 | 0.6086 | 12 | 0.6206 | 7 | 0.6481 | 5 | 0.6875 | |
4 | 345 | 0.6020 | 54 | 0.6087 | 21 | 0.6339 | 12 | 0.6696 | 9 | 0.6367 | |
10 | 821 | 0.6006 | 128 | 0.6061 | 50 | 0.6182 | 29 | 0.6182 | 20 | 0.6762 | |
0.6 | 0 | 76 | 0.4036 | 12 | 0.4125 | 5 | 0.4116 | 3 | 0.4231 | 2 | 0.5000 |
2 | 258 | 0.4018 | 40 | 0.4103 | 16 | 0.4017 | 9 | 0.4290 | 6 | 0.5000 | |
4 | 435 | 0.4017 | 68 | 0.4010 | 26 | 0.4255 | 15 | 0.4243 | 10 | 0.5000 | |
10 | 957 | 0.4012 | 149 | 0.4010 | 58 | 0.4019 | 33 | 0.4070 | 23 | 0.4159 | |
0.8 | 0 | 134 | 0.2001 | 20 | 0.2166 | 8 | 0.2115 | 4 | 0.2752 | 3 | 0.2500 |
2 | 355 | 0.2011 | 55 | 0.2017 | 21 | 0.2091 | 12 | 0.2011 | 8 | 0.2266 | |
4 | 558 | 0.2007 | 86 | 0.2043 | 33 | 0.2082 | 18 | 0.2361 | 12 | 0.2744 | |
10 | 1134 | 0.2003 | 175 | 0.2037 | 67 | 0.2113 | 38 | 0.2025 | 26 | 0.2122 | |
0.99 | 0 | 381 | 0.0101 | 58 | 0.0102 | 21 | 0.0118 | 11 | 0.0136 | 7 | 0.0156 |
2 | 696 | 0.0101 | 106 | 0.0103 | 39 | 0.0117 | 21 | 0.0123 | 14 | 0.0112 | |
4 | 962 | 0.0100 | 147 | 0.0101 | 55 | 0.0105 | 30 | 0.0103 | 19 | 0.0154 | |
10 | 1671 | 0.0100 | 256 | 0.0101 | 96 | 0.0112 | 53 | 0.0103 | 35 | 0.0122 |
n | Parameters | MLE | LSE | WLSE | MPSE | CvME | |||||
MSE | SE | MSE | SE | MSE | SE | MSE | SE | MSE | SE | ||
20 | \delta =0.75 | 0.0634 | 0.0512 | 0.0462 | 0.0471 | 0.0321 | 0.0392 | 0.0318 | 0.0385 | 0.0457 | 0.0476 |
\vartheta =0.25 | 4.2070* | 0.0104 | 5.9938* | 0.0168 | 3.7685* | 0.0135 | 1.8682* | 9.6702* | 5.7922* | 0.0166 | |
\upsilon =-0.25 | 0.0563 | 3.5521* | 0.1807 | 0.0249 | 0.1933 | 0.0222 | 0.2111 | 0.0204 | 0.2275 | 0.0281 | |
30 | \delta =0.75 | 0.0316 | 0.0295 | 0.0327 | 0.0309 | 0.0242 | 0.0270 | 0.0193 | 0.0248 | 0.0328 | 0.0321 |
\vartheta =0.25 | 3.5439* | 7.0577* | 3.0803* | 9.8808* | 2.7990* | 9.6063* | 1.0694* | 5.5847* | 4.3042* | 0.0118 | |
\upsilon =-0.25 | 0.0558 | 2.0435* | 0.1807 | 0.0176 | 0.1915 | 0.0147 | 0.1973 | 0.0116 | 0.2110 | 0.0199 | |
50 | \delta =0.75 | 0.0317 | 0.0213 | 0.0162 | 0.0174 | 0.0101 | 0.0140 | 0.0157 | 0.0165 | 0.0162 | 0.0178 |
\vartheta =0.25 | 3.8108* | 4.7838* | 1.1283* | 4.7452* | 0.5372* | 3.0750* | 1.1926* | 3.7336* | 1.1844* | 4.8565* | |
\upsilon =-0.25 | 0.0561 | 1.3275* | 0.1649 | 8.5725* | 0.1800 | 5.9981* | 0.1874 | 5.1355* | 0.1824 | 8.9142* | |
75 | \delta =0.75 | 0.0400 | 0.0202 | 0.0101 | 0.0104 | 6.1135* | 8.6267* | 0.0106 | 0.0112 | 8.3405* | 9.6595* |
\vartheta =0.25 | 3.6780* | 3.5785* | 0.4110* | 2.3153* | 0.3427* | 1.8551* | 1.0702* | 2.6413* | 0.3617* | 2.1469* | |
\upsilon =-0.25 | 0.0555 | 1.4264* | 0.1659 | 5.9550* | 0.1788 | 3.7775* | 0.1838 | 3.2474* | 0.1763 | 5.9837* | |
100 | \delta =0.75 | 0.0194 | 0.0113 | 7.9590* | 7.7147* | 5.3663* | 6.9967* | 8.3169* | 8.5896* | 7.3736* | 7.4625* |
\vartheta =0.25 | 3.1715* | 2.4667* | 0.4031* | 1.9978* | 0.3735* | 1.7288* | 0.9853* | 2.0216* | 0.3708* | 1.9209* | |
\upsilon =-0.25 | 0.0558 | 0.7247* | 0.1641 | 4.0990* | 0.1780 | 2.5736* | 0.1811 | 2.2216* | 0.1713 | 4.2937* | |
150 | \delta =0.75 | 0.0174 | 8.6607* | 4.7108* | 4.4809* | 3.5370* | 4.4433* | 4.2869* | 5.3664* | 4.3355* | 4.4987* |
\vartheta =0.25 | 3.0945* | 1.8702* | 0.2082* | 1.1216* | 0.2407* | 0.8882* | 0.6736* | 1.4636* | 0.2130* | 1.1105* | |
\upsilon =-0.25 | 0.0557 | 0.4741* | 0.1543 | 3.4320* | 0.1758 | 1.6379* | 0.1533 | 1.7328* | 0.1703 | 3.1378* |
n | Parameters | MLE | LSE | WLSE | MPSE | CvME | |||||
MSE | SE | MSE | SE | MSE | SE | MSE | SE | MSE | SE | ||
20 | \delta =1.5 | 0.5272 | 0.1158 | 0.2419 | 0.0810 | 0.2248 | 0.0707 | 0.4151 | 0.1004 | 0.3123 | 0.0825 |
\vartheta =0.5 | 0.0346 | 0.0142 | 0.0184 | 6.5972* | 0.0190 | 5.5531* | 0.0256 | 0.0107 | 0.0205 | 6.0177* | |
\upsilon =-0.25 | 0.0375 | 9.2001* | 0.4592 | 0.0446 | 0.4543 | 0.0345 | 0.3840 | 0.0235 | 0.5443 | 0.0447 | |
30 | \delta =1.5 | 0.4394 | 0.0833 | 0.1267 | 0.0477 | 0.1531 | 0.0502 | 0.2508 | 0.0594 | 0.1548 | 0.0482 |
\vartheta =0.5 | 0.0334 | 0.0100 | 0.0138 | 6.2299* | 0.0146 | 5.9041* | 0.0205 | 8.3477* | 0.0144 | 5.2513* | |
\upsilon =-0.25 | 0.0336 | 0.0101 | 0.4306 | 0.0254 | 0.4516 | 0.0219 | 0.4126 | 0.0164 | 0.4962 | 0.0278 | |
50 | \delta =1.5 | 0.4512 | 0.0672 | 0.1199 | 0.0348 | 0.1158 | 0.0321 | 0.1586 | 0.0376 | 0.1261 | 0.0324 |
\vartheta =0.5 | 0.0356 | 8.5213* | 0.0144 | 4.0590* | 0.0142 | 3.7567* | 0.0190 | 4.9860* | 0.0146 | 3.3391* | |
\upsilon =-0.25 | 0.0353 | 6.0854* | 0.4172 | 0.0154 | 0.4337 | 0.0122 | 0.3878 | 9.5065* | 0.4603 | 0.0164 | |
75 | \delta =1.5 | 0.4150 | 0.0512 | 0.1116 | 0.0226 | 0.1003 | 0.0193 | 0.1555 | 0.0300 | 0.1234 | 0.0216 |
\vartheta =0.5 | 0.0351 | 6.0012* | 0.0140 | 2.7917* | 0.0135 | 2.6442* | 0.0201 | 4.4084* | 0.0146 | 2.4824* | |
\upsilon =-0.25 | 0.0358 | 4.3681* | 0.4250 | 0.0103 | 0.4419 | 8.0752* | 0.3809 | 6.4523* | 0.4510 | 0.0106 | |
100 | \delta =1.5 | 0.4328 | 0.0426 | 0.1056 | 0.0171 | 0.0920 | 0.0172 | 0.1647 | 0.0282 | 0.1011 | 0.0153 |
\vartheta =0.5 | 0.0363 | 5.5815* | 0.0136 | 2.3123* | 0.0121 | 2.7129* | 0.0189 | 4.3207* | 0.0131 | 1.8163* | |
\upsilon =-0.25 | 0.0339 | 3.6981* | 0.4570 | 7.3339* | 0.4564 | 5.6197* | 0.3973 | 5.2128* | 0.4761 | 7.1705* | |
150 | \delta =1.5 | 0.3750 | 0.0254 | 0.0874 | 6.4564* | 0.0663 | 6.6803* | 0.1223 | 0.0146 | 0.0980 | 7.1989* |
\vartheta =0.5 | 0.0333 | 3.0071* | 0.0134 | 1.1206* | 0.0121 | 1.2057* | 0.0189 | 3.0867* | 0.0135 | 1.1276* | |
\upsilon =-0.25 | 0.0336 | 1.9440* | 0.4335 | 2.6577* | 0.4322 | 1.7794* | 0.3768 | 2.8150* | 0.4433 | 2.4982* |
n | Parameters | MLE | LSE | WLSE | MPSE | CvME | |||||
MSE | SE | MSE | SE | MSE | SE | MSE | SE | MSE | SE | ||
20 | \delta =0.75 | 0.0276 | 0.0320 | 0.0650 | 0.0400 | 0.0521 | 0.0350 | 0.0312 | 0.0370 | 0.0656 | 0.0420 |
\vartheta =0.25 | 2.9819* | 9.6410* | 8.3113* | 0.0200 | 6.9943* | 0.0180 | 2.1731* | 0.0100 | 0.0109 | 0.0220 | |
\upsilon =-0.75 | 0.5469 | 1.3110* | 0.9473 | 0.0340 | 0.9252 | 0.0260 | 0.8917 | 0.0210 | 1.0580 | 0.0370 | |
30 | \delta =0.75 | 0.0240 | 0.0250 | 0.0523 | 0.0240 | 0.0435 | 0.0250 | 0.0217 | 0.0250 | 0.0539 | 0.0260 |
\vartheta =0.25 | 3.4648* | 7.9550* | 5.8641* | 0.0140 | 5.8410* | 0.0140 | 2.2441* | 6.4740* | 6.4372* | 0.0140 | |
\upsilon =-0.75 | 0.5458 | 1.4640* | 0.9186 | 0.0210 | 0.9199 | 0.0190 | 0.8715 | 0.0150 | 0.9848 | 0.0230 | |
50 | \delta =0.75 | 0.0173 | 0.0170 | 0.0441 | 0.0170 | 0.0377 | 0.0170 | 0.0129 | 0.0140 | 0.0452 | 0.0180 |
\vartheta =0.25 | 3.8093* | 5.0600* | 3.6101* | 8.5390* | 4.1827* | 9.1340* | 2.3664* | 3.4760* | 3.8059* | 8.7550* | |
\upsilon =-0.75 | 0.5438 | 0.9780* | 0.8972 | 0.0110 | 0.8821 | 8.3640* | 0.8377 | 6.2060* | 0.9392 | 0.0110 | |
75 | \delta =0.75 | 0.0238 | 0.0157 | 0.0410 | 0.0140 | 0.0307 | 0.0130 | 9.5963* | 9.3015* | 0.0413 | 0.0148 |
\vartheta =0.25 | 2.9710* | 4.3074* | 3.6810* | 7.0170* | 3.2999* | 6.5592* | 1.9662* | 2.2798* | 4.7127* | 7.9185* | |
\upsilon =-0.75 | 0.5459 | 0.6425* | 0.9595 | 8.5172* | 0.8979 | 5.5828* | 0.8356 | 3.6097* | 0.9863 | 8.7994* | |
100 | \delta =0.75 | 0.0227 | 0.0134 | 0.0298 | 0.0100 | 0.0137 | 7.9828* | 4.3208* | 5.2667* | 0.0297 | 9.1020* |
\vartheta =0.25 | 2.3568* | 4.0206* | 2.8294* | 5.5969* | 1.5028* | 2.2740* | 1.5569* | 1.4654* | 2.5345* | 5.3057* | |
\upsilon =-0.75 | 0.5416 | 0.6058* | 0.9242 | 5.0194* | 0.8670 | 2.3480* | 0.8194 | 3.1607* | 0.9360 | 5.1240* | |
150 | \delta =0.75 | 9.1118* | 2.7224* | 0.0249 | 5.3565* | 8.5464* | 7.9564* | 4.1972* | 4.5636* | 0.0241 | 5.8262* |
\vartheta =0.25 | 1.7642* | 0.9286* | 1.5173* | 1.8264* | 0.8638* | 0.4991* | 1.0472* | 0.9626* | 1.0430* | 2.3932* | |
\upsilon =-0.75 | 0.5329 | 1.0329* | 0.9163 | 5.0756* | 0.8485 | 1.7051* | 0.7717 | 1.7450* | 0.9272 | 4.3014* |
n | Parameters | MLE | LSE | WLSE | MPSE | CvME | |||||
MSE | SE | MSE | SE | MSE | SE | MSE | SE | MSE | SE | ||
20 | \delta =1.5 | 0.3166 | 0.1074 | 0.0752 | 0.0611 | 0.0688 | 0.0584 | 0.1069 | 0.0688 | 0.0855 | 0.0628 |
\vartheta =0.5 | 0.0371 | 0.0136 | 0.0161 | 7.9040* | 0.0156 | 8.2771* | 0.0170 | 0.0104 | 0.0172 | 7.9114* | |
\upsilon =-0.75 | 0.4460 | 0.0347 | 1.4360 | 0.0421 | 1.4374 | 0.0389 | 1.3799 | 0.0355 | 1.6197 | 0.0471 | |
30 | \delta =1.5 | 0.2908 | 0.0852 | 0.0450 | 0.0385 | 0.0401 | 0.0359 | 0.0525 | 0.0419 | 0.0446 | 0.0385 |
\vartheta =0.5 | 0.0361 | 0.0105 | 0.0136 | 5.8095* | 0.0121 | 5.5192* | 0.0125 | 7.1990* | 0.0141 | 5.2089* | |
\upsilon =-0.75 | 0.4180 | 0.0143 | 1.4336 | 0.0260 | 1.4175 | 0.0237 | 1.3529 | 0.0213 | 1.5567 | 0.0286 | |
50 | \delta =1.5 | 0.2901 | 0.0662 | 0.0281 | 0.0235 | 0.0269 | 0.0219 | 0.0337 | 0.0256 | 0.0273 | 0.0234 |
\vartheta =0.5 | 0.0352 | 7.9137* | 0.0134 | 3.6008* | 0.0111 | 3.5586* | 0.0121 | 5.2926* | 0.0138 | 3.4227* | |
\upsilon =-0.75 | 0.4176 | 0.0131 | 1.4264 | 0.0148 | 1.3670 | 0.0112 | 1.2780 | 0.0106 | 1.4927 | 0.0155 | |
75 | \delta =1.5 | 0.1622 | 0.0418 | 0.0195 | 0.0158 | 0.0229 | 0.0153 | 0.0315 | 0.0192 | 0.0185 | 0.0157 |
\vartheta =0.5 | 0.0301 | 5.3795* | 0.0131 | 2.5415* | 0.0103 | 2.9067* | 0.0112 | 3.9476* | 0.0134 | 2.4295* | |
\upsilon =-0.75 | 0.4141 | 9.7076* | 1.4082 | 0.0101 | 1.3617 | 6.9812* | 1.2608 | 6.7749* | 1.4829 | 0.0101 | |
100 | \delta =1.5 | 0.2589 | 0.0436 | 0.0151 | 0.0122 | 0.0181 | 0.0107 | 0.0204 | 0.0132 | 0.0159 | 0.0126 |
\vartheta =0.5 | 0.0357 | 5.4308* | 0.0129 | 2.0247* | 9.3446* | 2.0512* | 0.0106 | 3.7361* | 0.0132 | 2.0816* | |
\upsilon =-0.75 | 0.4123 | 6.1602* | 1.3819 | 7.3598* | 1.3518 | 5.2508* | 1.2430 | 5.0394* | 1.4739 | 7.9705* | |
150 | \delta =1.5 | 0.1303 | 0.0258 | 0.0113 | 8.2565* | 0.0176 | 0.0105 | 0.0189 | 7.0110* | 0.0134 | 9.3264* |
\vartheta =0.5 | 0.0312 | 4.0948* | 0.0124 | 1.4042* | 7.8324* | 2.2745* | 8.8525* | 2.0483* | 0.0128 | 1.6189* | |
\upsilon =-0.75 | 0.4096 | 4.3745* | 1.3245 | 5.5974* | 1.3308 | 3.0557* | 1.2360 | 2.7320* | 1.4591 | 5.2175* | |
*Note: * Indicate that the value multiply {10}^{-3} in Tables 9–12. |
Model | MLE | SE | -2LogL | AIC | BIC | CAIC | K-S | P-value |
TPITL | \widehat{\upsilon }=-0.373 | 0.610 | 77.89 | 82.66 | 85.09 | 83.10 | 0.1069 | 0.8831 |
\widehat{\delta }=1.723 | 0.587 | |||||||
\widehat{\vartheta }=1.758 | 1.003 | |||||||
PITL | \widehat{\alpha }=1.313 | 0.322 | 78.66 | 83.32 | 85.46 | 83.77 | 0.1182 | 0.7961 |
\widehat{\vartheta }=1.974 | 0.365 | |||||||
GIE | \widehat{\alpha }=3.300 | 1.065 | 79.32 | 83.89 | 86.13 | 84.81 | 0.1266 | 0.7224 |
\widehat{\lambda }=2.199 | 0.433 | |||||||
Burr XII | \widehat{\vartheta }=3.216 | 0.645 | 80.55 | 84.55 | 87.36 | 85 | 0.1287 | 0.7033 |
\widehat{\beta }=0.563 | 0.137 | |||||||
in-Ga | \widehat{\lambda }=2.596 | 0.631 | 80.61 | 85.61 | 87.42 | 85.06 | 0.1375 | 0.6218 |
\widehat{\beta }=2.966 | 0.795 | |||||||
in-We | \widehat{\alpha }=1.550 | 0.198 | 83.83 | 87.83 | 90.64 | 88.28 | 0.1523 | 0.4896 |
\widehat{\lambda }=1.025 | 0.203 |
Model | MLE | SE | -2LogL | AIC | BIC | CAIC | K-S | P-value |
TPITL | \hat{v}=-0.794 | 0.193 | 194.002 | 198.002 | 202.610 | 198.171 | 0.089 | 0.601 |
\hat{\delta}=1.640 | 0.270 | |||||||
\hat{\vartheta}=2.010 | 0.407 | |||||||
Ge-Ex | \hat{\alpha}=3.565 | 0.696 | 195.184 | 200.889 | 205.498 | 201.026 | 0.090 | 0.588 |
\hat{\lambda}=0.891 | 0.103 | |||||||
We | \hat{\eta}=1.820 | 0.156 | 196.889 | 201.184 | 208.096 | 201.558 | 0.100 | 0.448 |
\hat{\beta}=1.989 | 0.134 | |||||||
log-N | \hat{\mu}=0.393 | 0.073 | 200.565 | 204.565 | 209.174 | 204.734 | 0.101 | 0.437 |
\hat{\sigma}=0.632 | 0.052 | |||||||
PITL | \hat{\alpha}=1.142 | 0.189 | 200.604 | 204.604 | 209.213 | 204.774 | 0.118 | 0.254 |
\hat{\vartheta}=2.154 | 0.274 | |||||||
EIE | \hat{\alpha}=2.858 | 0.586 | 221.525 | 225.525 | 230.133 | 225.694 | 0.145 | 0.072 |
\hat{\lambda}=2.082 | 0.271 |