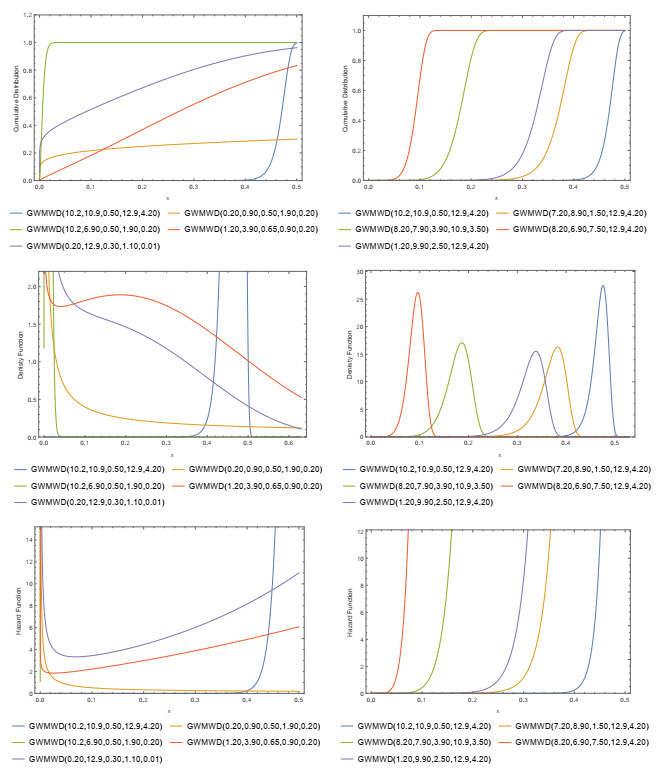
The combined-unified hybrid sampling approach was introduced as a general model that combines the unified hybrid censoring sampling approach and the combined hybrid censoring approach into a unified approach. In this paper, we apply this censoring sampling approach to improve the estimation of the parameter via a novel five-parameter expansion distribution, which we call the generalized Weibull-modified Weibull model. The new distribution contains five parameters and is therefore very flexible in terms of accommodating different types of data. The new distribution provides graphs of the probability density function, e.g., symmetric or right skewed. The graph of the risk function can have a shape similar to a monomer of the increasing or decreasing model. Using the Monte Carlo method, the maximum likelihood approach is used in the estimation procedure. The Copula model was used to discuss the two marginal univariate distributions. The asymptotic confidence intervals of the parameters were developed. We present some simulation results to validate the theoretical results. Finally, a data set with failure times for 50 electronic components was analyzed to illustrate the applicability and potential of the proposed model.
Citation: Walid Emam, Ghadah Alomani. Predictive modeling of reliability engineering data using a new version of the flexible Weibull model[J]. Mathematical Biosciences and Engineering, 2023, 20(6): 9948-9964. doi: 10.3934/mbe.2023436
[1] | Rashad A. R. Bantan, Zubair Ahmad, Faridoon Khan, Mohammed Elgarhy, Zahra Almaspoor, G. G. Hamedani, Mahmoud El-Morshedy, Ahmed M. Gemeay . Predictive modeling of the COVID-19 data using a new version of the flexible Weibull model and machine learning techniques. Mathematical Biosciences and Engineering, 2023, 20(2): 2847-2873. doi: 10.3934/mbe.2023134 |
[2] | Mahmoud El-Morshedy, Zubair Ahmad, Elsayed tag-Eldin, Zahra Almaspoor, Mohamed S. Eliwa, Zahoor Iqbal . A new statistical approach for modeling the bladder cancer and leukemia patients data sets: Case studies in the medical sector. Mathematical Biosciences and Engineering, 2022, 19(10): 10474-10492. doi: 10.3934/mbe.2022490 |
[3] | M. G. M. Ghazal, H. M. M. Radwan . A reduced distribution of the modified Weibull distribution and its applications to medical and engineering data. Mathematical Biosciences and Engineering, 2022, 19(12): 13193-13213. doi: 10.3934/mbe.2022617 |
[4] | Yinghui Zhou, Zubair Ahmad, Zahra Almaspoor, Faridoon Khan, Elsayed tag-Eldin, Zahoor Iqbal, Mahmoud El-Morshedy . On the implementation of a new version of the Weibull distribution and machine learning approach to model the COVID-19 data. Mathematical Biosciences and Engineering, 2023, 20(1): 337-364. doi: 10.3934/mbe.2023016 |
[5] | M. Nagy, Adel Fahad Alrasheedi . The lifetime analysis of the Weibull model based on Generalized Type-I progressive hybrid censoring schemes. Mathematical Biosciences and Engineering, 2022, 19(3): 2330-2354. doi: 10.3934/mbe.2022108 |
[6] | M. E. Bakr, Abdulhakim A. Al-Babtain, Zafar Mahmood, R. A. Aldallal, Saima Khan Khosa, M. M. Abd El-Raouf, Eslam Hussam, Ahmed M. Gemeay . Statistical modelling for a new family of generalized distributions with real data applications. Mathematical Biosciences and Engineering, 2022, 19(9): 8705-8740. doi: 10.3934/mbe.2022404 |
[7] | Amal S. Hassan, Najwan Alsadat, Christophe Chesneau, Ahmed W. Shawki . A novel weighted family of probability distributions with applications to world natural gas, oil, and gold reserves. Mathematical Biosciences and Engineering, 2023, 20(11): 19871-19911. doi: 10.3934/mbe.2023880 |
[8] | Said G. Nassr, Amal S. Hassan, Rehab Alsultan, Ahmed R. El-Saeed . Acceptance sampling plans for the three-parameter inverted Topp–Leone model. Mathematical Biosciences and Engineering, 2022, 19(12): 13628-13659. doi: 10.3934/mbe.2022636 |
[9] | Saralees Nadarajah . Remark on the Paper by Rao And Kakehashi (2005). Mathematical Biosciences and Engineering, 2006, 3(2): 385-387. doi: 10.3934/mbe.2006.3.385 |
[10] | Arni S.R. Srinivasa Rao, Masayuki Kakehashi . Incubation-time distribution in back-calculation applied to HIV/AIDS data in India. Mathematical Biosciences and Engineering, 2005, 2(2): 263-277. doi: 10.3934/mbe.2005.2.263 |
The combined-unified hybrid sampling approach was introduced as a general model that combines the unified hybrid censoring sampling approach and the combined hybrid censoring approach into a unified approach. In this paper, we apply this censoring sampling approach to improve the estimation of the parameter via a novel five-parameter expansion distribution, which we call the generalized Weibull-modified Weibull model. The new distribution contains five parameters and is therefore very flexible in terms of accommodating different types of data. The new distribution provides graphs of the probability density function, e.g., symmetric or right skewed. The graph of the risk function can have a shape similar to a monomer of the increasing or decreasing model. Using the Monte Carlo method, the maximum likelihood approach is used in the estimation procedure. The Copula model was used to discuss the two marginal univariate distributions. The asymptotic confidence intervals of the parameters were developed. We present some simulation results to validate the theoretical results. Finally, a data set with failure times for 50 electronic components was analyzed to illustrate the applicability and potential of the proposed model.
There are many phenomena in this world that need statistical description to be more understandable to the reader, but there is no specific statistical distribution that describes all of them. Therefore, many researchers have recently tried to develop new families by adding one, two or three parameters, e.g., [1,2,3,4,5]. By adding two additional parameters β and γ, Cordeiro et al. [6] introduced the generalized Weibull distribution family. We continue this line of research by proposing a novel family, namely, a generalized Weibull-modified Weibull model. A model was developed that can fit real data because the model has great flexibility in representing nonlinear dynamics. The model is useful for studying data science through statistical modeling and it has been applied to engineering data. Its application in engineering shows that the developed model is adaptable and flexible in terms of ability to represent complex data. For any cumulative function (CDF) W(x) and probability density function (PDF) w(x), the CDF and the PDF of the proposed family are respectively given by
ϝ(x;β,γ)=1−exp[−β(−log[1−W(x)])γ],x∈R;β,γ>0, | (1.1) |
and
f(x;β,γ)=βγw(x)1−W(x)(−log[1−W(x)])γ−1exp(−β(−log(1−W(x)))γ),x∈R;β,γ>0. | (1.2) |
Type-Ⅰ and Type-Ⅱ-censorship schemes are the two most common and popular censorship schemes. Type-Ⅰ and type-Ⅱ censorship schemes were merged by Epstein [7] in the hybrid censorship scheme. For a more brief review of censoring schemes, we refer the reader to [8–14]. Balakrishnan et al. [15] have proposed a unified hybrid censoring method. Huang and Yang [16] have considered a combined hybrid censoring sample. Emam and Sultan [17] combined the unified hybrid censoring sampling method and the combined hybrid censoring sampling method into a unified approach known as C-UHCS(m,r;T1,T2), which refers to the combined-unified hybrid censoring method. The likelihood function of C-UHCS(k,r;T1,T2) is
L(Ω|xk)=n!(n−k)![1−F(T)]n−kk∏i=1f(xi), | (1.3) |
where k and T can be chosen as:
L(C)(Ω|x) | L(U)(Ω|x) | |||
Cases | k | T | k | T |
1 : 0<T1<Xk:n<T2<Xr:n | m | Xm:n | D2 | T2 |
2 : 0<T1<Xk:n<Xr:n<T2 | m | Xm:n | r | Xr:n |
3 : 0<T1<T2<Xk:n<Xr:n | D2 | T2 | m | Xm:n |
4 : 0<Xk:n<Xr:n<T1<T2 | r | Xr:n | D1 | T1 |
5 : 0<Xk:n<T1<Xr:n<T2 | D1 | T1 | r | Xr:n |
6 : 0<Xk:n<T1<T2<Xr:n | D1 | T1 | D2 | T2 |
Then, for a parameter space Ω, the likelihood function of C-UHCS(m,r;T1,T2), which represents all possible likelihood functions under different values of k, T, and xk=(x1,x2,...,xk), can be written as
L(Ω|xk)=n!(n−k)!(k∏i=1f(xi))(1−F(T))n−k. | (1.4) |
The authors believe that this problem deserves investigation. The main motivations for using the GMW-X family in practice are as follows: 1) It is an excellent way to enter additional parameters to create an extended version of the basic model. 2) It can improve the properties of the traditional distributions. 3) It can create symmetric, right-skewed and left-skewed distributions. 4) It can provide a consistently better fit than other models. This was a good incentive to study the problem, and this was supported by the numerical results, which confirmed the superiority of the new model over many of the basic and competing models.
The rest of this work is presented here as follows. The generalized Weibull-modified Weibull distribution (GWMWD) is presented in Section 2. The bivariate extension of the generalized Weibull-modified Weibull model is discussed in Section 3. Based on C-UHCS(m,r;T1,T2), Section 4 is devoted to applying the maximum likelihood approach to the GWMWD. Section 5 presents the Monte Carlo procedure. Section 6 applies the GWMWD to a data set of 50 electronic component failures. Section 7 shows some conclusions.
Let X be a random variable (R.V.) with the modified Weibull distribution (ω,θ,ν) distribution suggested by Sarhan and Zaindin [18]; then, its CDF is
W(x;ω,δ,ν)=1−exp(−ωx−δxν),x>0, | (2.1) |
and its PDF is given by
w(x;ω,δ,ν)=(ω+δνxν−1)exp(−ωx−δxν),x>0, | (2.2) |
where ω≥0 is a scale parameter, while δ≥0 and ν>0 are shape parameters such that ν+δ>0.
The generalized Weibull distribution family generalizes the generalized Weibull normal distribution when β=γ=1, the generalized Weibull Gumbel distribution when the generalized Weibull family βγ=1, and the generalized Weibull logistic distribution. The GWMWD is defined from (1.1) by replacing W(x) and w(x) with W(x;ω,δ,ν) and w(x;ω,δ,ν), respectively. The CDF and PDF of GWMWD, respectively, are
ϝ(x;β,γ,ω,δ,ν)=1−e−β(xω+xνδ)γ,x>0;β,γ,ω,δ,ν>0, | (2.3) |
and
f(x;β,γ,ω,δ,ν)=e−β(xω+xνδ)γβγ(ω+xν−1δν)(xω+xνδ)γ−1,x>0;β,γ,ω,δ,ν>0. | (2.4) |
The survival function (SF) and hazard rate function (HRF) via the GWMWD of time t, respectively, are
S(t;β,γ,ω,δ,ν)=e−β(tω+tνδ)γ, | (2.5) |
and
H(t;β,γ,ω,δ,ν)=βγ(tω+tνδ)γ−1(ω+tν−1δν). | (2.6) |
In particular, the GWMWD generalizes the generalized Weibull-Weibull distribution (when ω=0), the generalized Weibull-Rayleigh distribution (when ω=0 and ν=2), the generalized linear Weibull exponential distribution (when ν=2 and δ=ω/2, ω>0), and the generalized Weibull exponential distribution (for ν=0). In what follows, an R.V. X with the GWMWD PDF (2.3) is written as X∼GWMWD(β,γ,ω,δ,ν).
Some possible behaviors of the CDF, PDF, and HRF for GWMWD (β,γ,ω,δ,ν) are shown in Figure 1. The left panel shows GWMWD (10.2, 10.9, 0.50, 12.9, 4.20), GWMWD(0.20, 0.90, 0.50, 1.90, 0.20), GWMWD (10.20, 6.90, 0.50, 1.90, 0.20), GWMWD (1.20, 3.90, 0.65, 0.90, 0.20), and GWMWD (0.20, 12.9, 0.30, 1.10, 0.01), while the right panel shows GWMWD (10.2, 10.9, 0.50, 12.9, 4.20), GWMWD (7.20, 8.90, 1.50, 12.9, 4.20), GWMWD (8.20, 7.90, 3.90, 10.9, 3.50), GWMWD (8.20, 6.90, 7.50, 12.9, 4.20), and GWMWD (1.20, 9.90, 2.50, 12.9, 4.20). From Figure 1, it can be seen that the CDF increases faster with increasing x for the parameters β and δ>1; then, it is constant and the graph grows exponentially, the PDF increases faster with increasing x for the parameters β and δ>1, and the proposed GWMWD has strong spurs; the HRF is constant and then increases faster with increasing x when the parameters β,γ,δ and α>1. The PDF shape is at times very flexible. It appears to approximate a bell curve with some twist. At other times it appears to have strong tails. Because of the divergent behavior of the proposed model, it could be a good candidate for modeling semi-normal and strong-tailed data in various industrial, financial, and medical applications.
The copula model was introduced by Morgenstern [19] to represent the joint CDF of the two marginal univariate distributions. Let ϝ(xj) be the CDF of Xj,j=1,2. Conway [20] introduced the joint CDF and PDF of the copula model, respectively, as
ϝ(x1,x2)=ϝ(x1)ϝ(x2)[1+ρ(1−ϝ(x1))(1−ϝ(x2))],−1<ρ<1, | (3.1) |
and
f(x1,x2)=f(x1)f(x2)[1+ρ(1−2ϝ(x1))(1−2ϝ(x2))], | (3.2) |
where ρ is the dependence measure between X1 and X2. Let the R.V.s X1∼ GWMWD (β1,γ1,ω1,δ1,ν1) and X2∼ GWMWD (β2,γ2,ω2,δ2,ν2); then, the corresponding joint CDF and PDF are, respectively, given by
ϝ(x1,x2)=(1−e−β1(x1ω1+xν11δ1)γ1)(1−e−β2(x2ω2+xν22δ2)γ2)×(1+ρe−β1(x1ω1+xν11δ1)γ1−β2(x2ω2+xν22δ2)γ2), | (3.3) |
and
f(x1,x2)=β1β2γ1γ2e−β1(x1ω1+xν11δ1)γ1−β2(x2ω2+xν22δ2)γ2(x1ω1+xν11δ1)γ1−1×(x2ω2+xν22δ2)ω1−1(ω1+xν−111δ1ν1)(ω2+xν−122δ2ν2)×(1+ρ(1−2e−β1(x1ω1+xν11δ1)γ1β1γ1(x1ω1+xν11δ1)γ−12(ω1+xν−111δ1ν1))×(1−2e−β2(x2ω2+xν22δ2)γ2β2γ2(x2ω2+xν22δ2)γ−12(ω2+xν−122δ2ν2))). | (3.4) |
Figure 2 presents the CDF for the bivariate GWMWD (0.1, 0.8, 4.4, 6.1, 3.20) and GWMWD (0.1, 0.8, 4.4, 6.1, 0.20) when the parameter ν increases, and for ρ=0.2.
The copula function is a way to construct bivariate distributions. Other methods can be reviewed and may be helpful to introduce some new bivariate distributions (see, Xu et al. [21] and Luo et al.[22].)
Suppose that {x1,x2,...,xk} is an observed sample from X∼GWMWD(β,γ,ω,δ,ν). The likelihood function of β,γ,ω,δ, and ν becomes
l=n!(n−k)!e−β(n−k)(Tω+Tνδ)γ(βγ)ke−β∑ki=1(xiω+xνiδ)γk∏i=1(ω+xν−1iδν)(xiω+xνiδ)γ−1, | (4.1) |
and the log-likelihood function (L) is
L=log[n!(n−k)!]−β(n−k)(Tω+Tνδ)γ+klog[βγ]−βk∑i=1(xiω+xνiδ)γ+k∑i=1log[ω+xν−1iδν]+(γ−1)k∑i=1log[xiω+xνiδ]. | (4.2) |
Let Q(x)=ωx+δxν. The first partial derivatives of (4.2) with respect to β,γ,ω,δ and ν are given by
∂L∂β=kβ−(n−k)Q(T)γ−k∑i=1Q(xi)γ, | (4.3) |
∂L∂γ=kγ−(n−k)βQ(T)γlogQ(T)+k∑i=1logQ(xi)−βk∑i=1Q(xi)γlogQ(xi), | (4.4) |
∂L∂ω=−(n−k)TβγQ(T)γ−1+k∑i=11ω+δνxν−1i+(γ−1)k∑i=1xiQ(xi)−βγk∑i=1xiQ(xi)γ−1, | (4.5) |
∂L∂δ=−(n−k)TνβγQ(T)γ−1+k∑i=1νxν−1iω+δνxν−1i+(γ−1)k∑i=1xνiQ(xi)−βγk∑i=1xνiQ(xi)γ−1, | (4.6) |
∂L∂ν=−(n−k)TνβγδQ(T)γ−1log[T]+δk∑i=1xν−1i+νlog[xi]xν−1iω+δνxν−1i+(γ−1)δk∑i=1log[xi]xνiQ(xi)−βγδk∑i=1log[xi]xνiQ(xi)γ−1. | (4.7) |
The maximum likelihood estimators ˆβML,ˆγML,ˆωML,ˆδML, and ˆνML of the GWMWD (β,γ,ω,δ,ν) parameters are the solutions of (4.3)–(4.7). The asymptotic confidence intervals of the parameters β,γ,ω,δ and ν can be calculated. ˆV=V(ˆβML,ˆγML,ˆωML,ˆδML,ˆνML) is the observed variance covariance matrix, such that
V(β,γ,ω,δ,ν)=−[∂2L∂β2∂2L∂β∂γ∂2L∂β∂ω∂2L∂β∂δ∂2L∂β∂ν∂2L∂γ∂β∂2L∂γ2∂2L∂γ∂ω∂2L∂γ∂δ∂2L∂γ∂ν∂2L∂ω∂β∂2L∂ω∂γ∂2L∂ω2∂2L∂ω∂δ∂2L∂ω∂ν∂2L∂δ∂β∂2L∂δ∂γ∂2L∂δ∂ω∂2L∂δ2∂2L∂δ∂ν∂2L∂ν∂β∂2L∂ν∂γ∂2L∂ν∂ω∂2L∂ν∂δ∂2L∂ν2]−1, | (4.8) |
where
∂2L∂β2=−kβ2, | (4.9) |
∂2L∂β∂γ=(k−n)Q(T)γlogδ2−k∑i=1Q(xi)γlogQ(xi), | (4.10) |
∂2L∂β∂ω=(k−n)TγQ(T)γ−1−γk∑i=1xiQ(xi)γ−1, | (4.11) |
∂2L∂β∂δ=(k−n)TνγQ(T)γ−1−γk∑i=1xνiQ(xi)γ−1, | (4.12) |
∂2L∂β∂ν=(k−n)TνγδQ(T)γ−1log[T]−γδk∑i=1xνiQ(xi)γ−1log[xi], | (4.13) |
∂2L∂γ2=−kγ2(k−n)βQ(T)γlogQ(T)2−βk∑i=1Q(xi)γlog[Q(xi)]2, | (4.14) |
∂2L∂γ∂ω=(k−n)2T2β2γQ(T)2γ−2logQ(T)+k∑i=1xiQ(xi)−βk∑i=1xiQ(xi)γ−1(1+γlogQ(xi)), | (4.15) |
∂2L∂γ∂δ=(k−n)2T2νβ2γQ(T)2γ−2logQ(T)+k∑i=1xνiQ(xi)−βk∑i=1xνiQ(xi)γ−1(1+γlogQ(xi)), | (4.16) |
∂2L∂γ∂ν=(k−n)TνβδQ(T)γ−1log[T](1+γlogQ(T))+k∑i=1δlog[xi]xνiQ(xi)−βδk∑i=1xνiQ(xi)γ−1log[xi](1+γlogQ(xi)), | (4.17) |
∂2L∂ω2=(k−n)T2β(γ−1)γQ(T)γ−2+k∑i=1−1Q(xi)2−(γ−1)k∑i=1x2iQ(xi)2+βγ(1−γ)k∑i=1xi2Q(xi)γ−2, | (4.18) |
∂2L∂ω∂δ=(k−n)T1+νβ(γ−1)γQ(T)γ−2+k∑i=1−νxν−1i(ω+δνxν−1i)2−(γ−1)k∑i=1x1+νiQ(xi)2+βγk∑i=1xiν+1Q(xi)γ−2(1−γ), | (4.19) |
∂2L∂ω∂ν=(k−n)T1+νβ(γ−1)γδQ(T)γ−2log[T]−(γ−1)k∑i=1δlog[xi]x1+νiQ(xi)2−δk∑i=1(xν−1i(ω+δνxν−1i)2+νlog[xi]xν−1i(ω+δνxν−1i)2)+βγδk∑i=1xiν+1Q(xi)γ−2log[xi](1−γ), | (4.20) |
∂2L∂δ2=(k−n)T2νβ(γ−1)γQ(T)γ−2−k∑i=1ν2x−2+2νi(ω+δνxν−1i)2−k∑i=1(γ−1)x2νiQ(xi)2+βγk∑i=1x2νiQ(xi)γ−2(1+γ), | (4.21) |
∂2L∂δ∂ν=(k−n)TνβγQ(T)γ−1log[T](Tν(γ−1)δ(ωT+δTν)−1−1)+(γ−1)k∑i=1log[xi]xνiQ(xi)(1−δ xνiQ(xi))+k∑i=1xν−1iω+δνxν−1i(1+νlog[xi]−νxν−1i(δ(1−νlog[xi])ω+δνxν−1i))−βγk∑i=1xνilog[xi](Q(xi)γ−1+(γ−1)δQ(x−i)γ−2), | (4.22) |
∂2L∂ν2=(k−n)2T3νβ2(γ−1)γ2δ3Q(T)2γ−3(log[T]2)2+(γ−1)k∑i=1(−δ2log[xi]2x2νiQ(xi)2+δlog[xi]2xνiQ(xi))+2δlog[xi]xν−1i+δνlog[xi]2xν−1iω+δνxν−1i−δ2k∑i=1x2ν−2i(1+νlog[xi])(1(ω+νxν−1i)2+νlog[xi](ω+δνxν−1i)2)−βγδk∑i=1xνiQ(xi)γ−1log[xi](log[xi]+(xνi)−1(γ−1)). | (4.23) |
An approximate 100(1−ϵ)% two-sided C.Is for the parameters β,γ,ω,δ and ν are
ˆβ±zϵ/2√V(ˆβ), | (4.24) |
ˆγ±zϵ/2√V(ˆγ), | (4.25) |
ˆω±zϵ/2√V(ˆω), | (4.26) |
ˆδ±zϵ/2√V(ˆδ), | (4.27) |
and
ˆν±zϵ/2√V(ˆν), | (4.28) |
respectively, where the diagonal elements of ˆV V(ˆβ), V(ˆγ), V(ˆω), V(ˆδ), and V(ˆν) are the estimated variances of ˆβML,ˆγML,ˆωML,ˆδML, and ˆνML, and zϵ/2 is the upper (ϵ2) percentile of the normal(0, 1) distribution.
Let U have a uniform (0, 1) distribution. The GWMWD can be simulated by using the solution of the nonlinear equation
0=1−u−exp−β(xω+xνδ)γ. | (5.1) |
We simulate the GWMWD for two sets of the parameters: Set 1: β=1.4,γ=3.0,ω=0.7,δ=1.3,ν=0.4, and Set 2: β=0.4,γ=1.0,ω=1.7,δ=1.5,ν=1.8. The empirical results of the Monte Carlo simulation study are given in Table 1 for Set 1. The empirical results of the Monte Carlo simulation study are given in Table 2 for Set 2. Suppose that the data were observed for the GWMWD under the censoring scheme C-UHCS(m,r;T1,T2) and set the arbitrary values for termination as T=Xk and k=45n. The simulation study is carried out as follows
Par. | n | Bias | MSEs | 90%L | 90%U | 90%W | 95%L | 95%U | 95%W |
ˆβ | 25 | 1.284 | 1.955 | 0.001 | 5.89 | 5.89 | 0.001 | 6.516 | 6.516 |
50 | 1.163 | 1.479 | 0.137 | 4.989 | 4.852 | 0.001 | 5.462 | 5.462 | |
100 | 1.124 | 1.325 | 0.35 | 4.698 | 4.347 | 0.001 | 5.122 | 5.122 | |
200 | 1.099 | 1.241 | 0.464 | 4.533 | 4.069 | 0.067 | 4.93 | 4.863 | |
400 | 1.08 | 1.18 | 0.545 | 4.415 | 3.87 | 0.167 | 4.793 | 4.625 | |
600 | 1.076 | 1.169 | 0.559 | 4.394 | 3.835 | 0.185 | 4.768 | 4.583 | |
800 | 1.079 | 1.171 | 0.559 | 4.399 | 3.841 | 0.184 | 4.774 | 4.59 | |
1000 | 1.078 | 1.169 | 0.561 | 4.395 | 3.834 | 0.187 | 4.77 | 4.582 | |
ˆγ | 25 | -0.307 | 0.403 | 2.032 | 3.354 | 1.322 | 1.903 | 3.483 | 1.58 |
50 | -0.428 | 0.311 | 2.061 | 3.082 | 1.021 | 1.962 | 3.182 | 1.22 | |
100 | -0.475 | 0.288 | 2.053 | 2.997 | 0.944 | 1.961 | 3.089 | 1.129 | |
200 | -0.502 | 0.281 | 2.038 | 2.959 | 0.921 | 1.948 | 3.049 | 1.101 | |
400 | -0.51 | 0.276 | 2.038 | 2.941 | 0.904 | 1.949 | 3.03 | 1.08 | |
600 | -0.52 | 0.28 | 2.02 | 2.939 | 0.919 | 1.931 | 3.029 | 1.099 | |
800 | -0.521 | 0.279 | 2.021 | 2.937 | 0.915 | 1.932 | 3.026 | 1.094 | |
1000 | -0.524 | 0.28 | 2.018 | 2.935 | 0.918 | 1.928 | 3.025 | 1.097 | |
ˆω | 25 | 0.99 | 1.265 | 0.616 | 4.764 | 4.148 | 0.212 | 5.169 | 4.957 |
50 | 0.886 | 0.921 | 1.076 | 4.096 | 3.02 | 0.781 | 4.39 | 3.609 | |
100 | 0.827 | 0.743 | 1.308 | 3.746 | 2.438 | 1.07 | 3.984 | 2.914 | |
200 | 0.798 | 0.668 | 1.403 | 3.593 | 2.19 | 1.189 | 3.807 | 2.617 | |
400 | 0.783 | 0.629 | 1.452 | 3.515 | 2.062 | 1.251 | 3.716 | 2.465 | |
600 | 0.78 | 0.619 | 1.466 | 3.495 | 2.029 | 1.268 | 3.693 | 2.425 | |
800 | 0.777 | 0.61 | 1.476 | 3.477 | 2.002 | 1.281 | 3.673 | 2.392 | |
1000 | 0.772 | 0.601 | 1.486 | 3.457 | 1.971 | 1.294 | 3.649 | 2.355 | |
ˆδ | 25 | 1.102 | 1.355 | 0.379 | 4.824 | 4.445 | -0.054 | 5.258 | 5.312 |
50 | 1.057 | 1.212 | 0.57 | 4.544 | 3.974 | 0.182 | 4.932 | 4.75 | |
100 | 1.017 | 1.086 | 0.735 | 4.298 | 3.563 | 0.387 | 4.646 | 4.258 | |
200 | 1.011 | 1.052 | 0.786 | 4.236 | 3.449 | 0.45 | 4.572 | 4.123 | |
400 | 0.986 | 0.987 | 0.867 | 4.105 | 3.238 | 0.551 | 4.421 | 3.869 | |
600 | 0.978 | 0.966 | 0.893 | 4.063 | 3.17 | 0.584 | 4.373 | 3.788 | |
800 | 0.978 | 0.965 | 0.896 | 4.06 | 3.164 | 0.588 | 4.369 | 3.781 | |
1000 | 0.977 | 0.961 | 0.902 | 4.052 | 3.151 | 0.594 | 4.36 | 3.765 | |
ˆν | 25 | 0.4 | 0.16 | 0.938 | 1.462 | 0.525 | 0.886 | 1.514 | 0.627 |
50 | 0.4 | 0.16 | 0.938 | 1.462 | 0.525 | 0.886 | 1.514 | 0.627 | |
100 | 0.4 | 0.16 | 0.938 | 1.462 | 0.525 | 0.886 | 1.514 | 0.627 | |
200 | 0.4 | 0.16 | 0.938 | 1.462 | 0.525 | 0.886 | 1.514 | 0.627 | |
400 | 0.4 | 0.16 | 0.938 | 1.462 | 0.525 | 0.886 | 1.514 | 0.627 | |
600 | 0.4 | 0.16 | 0.938 | 1.462 | 0.525 | 0.886 | 1.514 | 0.627 | |
800 | 0.4 | 0.16 | 0.938 | 1.462 | 0.525 | 0.886 | 1.514 | 0.627 | |
1000 | 0.4 | 0.16 | 0.938 | 1.462 | 0.525 | 0.886 | 1.514 | 0.627 |
Par. | n | Bias | MSEs | 90%L | 90%U | 90%W | 95%L | 95%U | 95%W |
ˆβ | 25 | 0.498 | 0.303 | 0.401 | 1.394 | 0.994 | 0.304 | 1.491 | 1.187 |
ˆβ | 25 | 0.498 | 0.303 | 0.401 | 1.394 | 0.994 | 0.304 | 1.491 | 1.187 |
50 | 0.458 | 0.226 | 0.487 | 1.229 | 0.742 | 0.415 | 1.302 | 0.887 | |
100 | 0.437 | 0.197 | 0.514 | 1.161 | 0.647 | 0.451 | 1.224 | 0.774 | |
200 | 0.426 | 0.187 | 0.519 | 1.133 | 0.613 | 0.459 | 1.193 | 0.733 | |
400 | 0.41 | 0.177 | 0.519 | 1.101 | 0.582 | 0.462 | 1.158 | 0.695 | |
600 | 0.404 | 0.175 | 0.517 | 1.091 | 0.574 | 0.461 | 1.147 | 0.686 | |
800 | 0.396 | 0.171 | 0.516 | 1.077 | 0.561 | 0.461 | 1.131 | 0.67 | |
1000 | 0.385 | 0.165 | 0.514 | 1.055 | 0.541 | 0.461 | 1.108 | 0.647 | |
ˆγ | 25 | -0.098 | 0.073 | 0.783 | 1.021 | 0.238 | 0.76 | 1.044 | 0.284 |
50 | -0.147 | 0.034 | 0.797 | 0.908 | 0.111 | 0.787 | 0.919 | 0.132 | |
100 | -0.159 | 0.03 | 0.792 | 0.89 | 0.098 | 0.782 | 0.9 | 0.117 | |
200 | -0.164 | 0.03 | 0.787 | 0.884 | 0.097 | 0.778 | 0.894 | 0.116 | |
400 | -0.16 | 0.028 | 0.794 | 0.885 | 0.091 | 0.785 | 0.894 | 0.109 | |
600 | -0.158 | 0.027 | 0.798 | 0.887 | 0.089 | 0.789 | 0.896 | 0.107 | |
800 | -0.157 | 0.027 | 0.799 | 0.888 | 0.089 | 0.79 | 0.896 | 0.106 | |
1000 | -0.154 | 0.027 | 0.802 | 0.89 | 0.088 | 0.794 | 0.898 | 0.105 | |
ˆω | 25 | -0.796 | 0.691 | 0.001 | 2.037 | 2.037 | 0.001 | 2.258 | 2.258 |
50 | -0.836 | 0.716 | 0.001 | 2.038 | 2.038 | 0.001 | 2.267 | 2.267 | |
100 | -0.855 | 0.741 | 0.001 | 2.06 | 2.06 | 0.001 | 2.297 | 2.297 | |
200 | -0.849 | 0.737 | 0.001 | 2.059 | 2.059 | 0.001 | 2.295 | 2.295 | |
400 | -0.83 | 0.723 | 0.001 | 2.057 | 2.057 | 0.001 | 2.288 | 2.288 | |
600 | -0.815 | 0.709 | 0.001 | 2.049 | 2.049 | 0.001 | 2.276 | 2.276 | |
800 | -0.802 | 0.699 | 0.001 | 2.044 | 2.044 | 0.001 | 2.268 | 2.268 | |
1000 | -0.783 | 0.683 | 0.001 | 2.037 | 2.037 | 0.001 | 2.255 | 2.255 | |
ˆδ | 25 | -0.597 | 0.415 | 0.222 | 1.584 | 1.362 | 0.089 | 1.717 | 1.628 |
50 | -0.637 | 0.422 | 0.17 | 1.556 | 1.386 | 0.035 | 1.691 | 1.656 | |
100 | -0.654 | 0.436 | 0.131 | 1.561 | 1.43 | 0.001 | 1.701 | 1.709 | |
200 | -0.652 | 0.436 | 0.134 | 1.563 | 1.429 | 0.001 | 1.702 | 1.707 | |
400 | -0.657 | 0.441 | 0.12 | 1.565 | 1.445 | 0.001 | 1.706 | 1.706 | |
600 | -0.651 | 0.435 | 0.136 | 1.563 | 1.427 | 0.001 | 1.702 | 1.702 | |
800 | -0.638 | 0.428 | 0.161 | 1.564 | 1.403 | 0.024 | 1.701 | 1.677 | |
1000 | -0.627 | 0.421 | 0.183 | 1.563 | 1.38 | 0.048 | 1.698 | 1.65 | |
ˆν | 25 | -0.899 | 0.863 | 0.001 | 2.316 | 2.316 | 0.001 | 2.592 | 2.592 |
50 | -0.941 | 0.904 | 0.001 | 2.341 | 2.341 | 0.001 | 2.63 | 2.63 | |
100 | -0.953 | 0.921 | 0.001 | 2.356 | 2.356 | 0.001 | 2.651 | 2.651 | |
200 | -0.948 | 0.918 | 0.001 | 2.358 | 2.358 | 0.001 | 2.652 | 2.652 | |
400 | -0.924 | 0.897 | 0.001 | 2.347 | 2.347 | 0.001 | 2.635 | 2.635 | |
600 | -0.905 | 0.876 | 0.001 | 2.332 | 2.332 | 0.001 | 2.612 | 2.612 | |
800 | -0.894 | 2.33 | 0.001 | 2.848 | 2.848 | 0.001 | 2.6005 | 2.601 | |
1000 | -0.874 | 2.319 | 0.001 | 2.785 | 2.785 | 0.001 | 2.59 | 2.59 |
1) Random samples of size n=25,50,...,1000 were simulated from the GWMWD.
2) The model parameters were estimated via the maximum likelihood method.
3) 1000 iterations were made to obtain the MLEs, biases and MSEs of these estimators.
4) Let ˆϑ be the MLE of ϑ=(β,γ,ω,δ,ν). The MLEs, biases and MSEs are given, respectively, by
ˆϑ=11000M∑i=1ˆϑi, | (5.2) |
Bias(ˆϑ)=11000M∑i=1(ˆϑi−ϑ), | (5.3) |
and
MSE(ˆϑ)=110001000∑i=1(ˆϑi−ϑ)2, | (5.4) |
5) The 90% and 95% approximate confidence intervals with their width were calculated.
This section is devoted for illustrating the GWMWD through the analysis of a reliability engineering application. The data set represents the failure times of 50 electronic components (per 1000 h); see Aryal and Elbatal [23]. Suppose that the data was observed from GWMWD under the censoring scheme C-UHCS(m,r;T1,T2), and set the arbitrary values for termination k=45 and T=10.943. Table 3 shows a summary of the reliability engineering data. The boxplot and Q-Q plot for the reliability engineering data are shown in Figure 4. The estimated parameters are ˆβ=0.058,ˆγ=9.986,ˆω=0.005,ˆδ=1.239, and ˆν=0.056. Plots of the fitted density and distribution functions of the GWMWD model are shown in Figure 5. The likelihood probability (PP) and Kaplan-Meier survival curve are shown in Figure 6.
Min. | 1st Qu. | Median | Mean | 3rd Qu. | Max. |
0.058 | 0.254 | 1.600 | 3.410 | 4.534 | 15.080 |
Table 4 compares the GWMWD based on some detection criteria, such as the Akaike information criterion (AIC), Bayesian information criterion (BIC), Hannan-Quinn information criterion (HQIC) and consistent Akaike information criterion (CAIC). The goodness-of-fit results of the GWMWD model are compared with some other models, including the generalized Weibull distribution (GWWD), the generalized Weibull-Rayleigh distribution (GWRD), the generalized linear Weibull exponential distribution (GLWEXPD) and the generalized Weibull exponential distribution (GWEXPD). Table 5 compares the GWMWD with the Kolmogorov-Smirnov test for one sample. The results in Tables 4 and 5 suggest that the GWMWD provides a better fit than other competing models and could be chosen as a suitable model for analyzing heavy-tailed electronic data.
Model | AIC | CAIC | BIC | HQIC |
GWMWD(0.058, 9.986, 0.005, 1.239, 0.056) | 212.9574 | 214.3211 | 222.5175 | 216.5980 |
GWWD(1.916, 0.972, 0.326, 0.635) | 213.6829 | 214.5718 | 221.3310 | 216.5953 |
GWRD(0.360, 0.246, 3.774) | 221.4256 | 221.9473 | 227.1617 | 223.609 |
GLWEXPD(0.466, 0.455, 0.715) | 213.2008 | 213.7226 | 218.9369 | 215.3852 |
GWEXPD(0.465, 0.640, 0.902, 0.007) | 216.9577 | 217.8465 | 224.6058 | 219.8701 |
Model | KS | p-value |
GWMWD(0.058, 9.986, 0.005, 1.239, 0.056) | 0.13263 | 0.3144 |
GWWD(1.916, 0.972, 0.326, 0.635) | 0.16936 | 0.2247 |
GWRD(0.360, 0.246, 3.774) | 0.14916 | 0.1952 |
GLWEXPD(0.466, 0.455, 0.715) | 0.14875 | 0.1977 |
GWEXPD(0.465, 0.640, 0.902, 0.007) | 0.17732 | 0.07589 |
A new extension of the Weibull distribution i.e., the generalized modified Weibull distribution with five parameters, is presented. The model has a high degree of flexibility to fit the data appropriately. The provided model exhibits strong tail-heavy behavior and has unimodal increasing failure rate functions. Based on a combined-unified hybrid sample, the maximum likelihood estimators of the intended model parameters and a Monte Carlo simulation study were obtained. To illustrate the applicability and potential of the intended distribution, a dataset of failure times of 50 electronic components was analyzed. The mean square errors and biases decrease with increasing sample size. It is clear that the proposed model agrees well with the estimated PDF and CDF plots. The boxplot shows that the electronic downtime data set has a highly right skewed tail. The new generalized modified Weibull distribution based on the one-sample Kolmogorov-Smirnov test provides a better fit than other competing models. The proposed model fits the Kaplan-Meier survival plot very well. The results indicate that the generalized Weibull distribution (modified Weibull distribution) is considered ideal for modeling the intended engineering data. For future studies, we hope to discuss the accelerated life testing based on the new distribution by using stress-strength models (Zhang et al. [24,25]).
The study was funded by Princess Nourah bint Abdulrahman University Researchers Supporting Project number (PNURSP2023R226), Princess Nourah bint Abdulrahman University, Riyadh, Saudi Arabia.
The authors declare that they have no conflicts of interest.
[1] |
W. Emam, Y. Tashkandy, The Weibull claim model: Bivariate extension, Bayesian, and maximum likelihood estimations, Math. Probl. Eng., 2022 (2022), 1–10. https://doi.org/10.1155/2022/8729529 doi: 10.1155/2022/8729529
![]() |
[2] |
W. Emam, Y. Tashkandy, A new generalized modified Weibull model: Simulating and modeling the dynamics of the COVID-19 pandemic in Saudi Arabia and Egypt, Math. Probl. Eng., 2022 (2022), 1–9. https://doi.org/10.1155/2022/1947098 doi: 10.1155/2022/1947098
![]() |
[3] |
X. Liu, Z. Ahmad, S. K. Khosa, M. Yusuf, O. Alamri, W. Emam, A new flexible statistical model: Simulating and modeling the survival times of COVID-19 patients in China, Complexity, 2021 (2021), 1–16. https://doi.org/10.1155/2021/6915742 doi: 10.1155/2021/6915742
![]() |
[4] |
W. Emam, Y. Tashkandy, The arcsine Kumaraswamy-generalized family: Bayesian and classical estimates and application, Symmetry, 14 (2022), 2311. https://doi.org/10.3390/sym14112311 doi: 10.3390/sym14112311
![]() |
[5] |
W. Emam, Y. Tashkandy, Khalil new generalized Weibull distribution based on ranked samples: Estimation, mathematical properties, and application to COVID-19 data, Symmetry, 14 (2022), 853. https://doi.org/10.3390/sym14050853 doi: 10.3390/sym14050853
![]() |
[6] |
G. M. Cordeiro, E. M. M. Ortega, T. G. Ramires, A new generalized Weibull family of distributions: Mathematical properties and applications, J. Stat. Distrib. Appl., 2 (2015), 1–25. https://doi.org/10.1186/s40488-015-0036-6 doi: 10.1186/s40488-015-0036-6
![]() |
[7] | B. Epstein, Truncated life tests in the exponential case, Ann. Math. Stat., 25 (1954), 555–564. |
[8] |
H. S. Jeong, J. I. Park, B. J. Yum, Development of (r,T) hybrid sampling plans for exponential lifetime distributions, J. Appl. Stat., 23 (1996), 601–607. https://doi.org/10.1080/02664769623964 doi: 10.1080/02664769623964
![]() |
[9] |
A. Childs, B. Chandrasekhar, N. Balakrishnan, D. Kundu, Exact likelihood inference based on type-I and type-Ⅱ hybrid censored samples from the exponential distribution, Ann. Inst. Stat. Math., 55 (2003), 319–330. https://doi.org/10.1007/BF02530502 doi: 10.1007/BF02530502
![]() |
[10] |
X. P. Xiao, H. Guo, S. H. Mao, The modeling mechanism, extension and optimization of grey GM (1, 1) model, Appl. Math. Modell., 38 (2014), 1896–1910. https://doi.org/10.1016/j.apm.2013.10.004 doi: 10.1016/j.apm.2013.10.004
![]() |
[11] |
N. Balakrishnan, D. Kundu, Hybrid censoring: Models, inferential results and applications, Comput. Stat. Data Anal., 57 (2013), 166–209. https://doi.org/10.1016/j.csda.2012.03.025 doi: 10.1016/j.csda.2012.03.025
![]() |
[12] |
S. Chen, G. K. Bhattacharya, Exact confidence bounds for an exponential parameter under hybrid censoring, Commun. Stat. Theory Methods, 16 (1987), 2429–2442. https://doi.org/10.1080/03610928708829516 doi: 10.1080/03610928708829516
![]() |
[13] |
N. Draper, I. Guttman, Bayesian analysis of hybrid life tests with exponential failure times, Ann. Inst. Stat. Math., 39 (1987), 219–225. https://doi.org/10.1007/BF02491461 doi: 10.1007/BF02491461
![]() |
[14] | K. Fairbanks, R. Madson, R. Dykstra, A confidence interval for an exponential parameter from a hybrid life test, J. Am. Stat. Assoc., 77 (1982), 137–140. |
[15] |
N. Balakrishnan, A. Rasouli, N. S. Farsipour, Exact likelihood inference based on an unified hybrid censored sample from the exponential distribution. J. Stat. Comput. Simul., 78 (2008), 475–488. https://doi.org/10.1080/00949650601158336 doi: 10.1080/00949650601158336
![]() |
[16] | W. T. Huang, K. C. Yang, A new hybrid censoring scheme and some of its properties, Tamsui Oxford J. Math. Sci., 26 (2010), 355–367. |
[17] |
W. Emam, K. S. Sultan, Bayesian and maximum likelihood estimations of the Dagum parameters under combined-unified hybrid censoring, Math. Biosci. Eng., 18 (2021), 2930–2951. http://dx.doi.org/10.3934/mbe.2021148 doi: 10.3934/mbe.2021148
![]() |
[18] | A. M. Sarhan, M. Zaindin, Modified Weibull distribution, APPS Appl. Sci., 11 (2009), 123–136. |
[19] | D. Morgenstern, Einfache beispiele zweidimensionaler verteilungen, Mitt. Math. Statist., 8 (1956), 234–235. |
[20] | D. A. Conway, Farlie-Gumbel-Morgenstern distributions, in Encyclopedia of Statistical Sciences, (eds. Kotz and N. L. Johnson), Wiley, (1983), 28–31. |
[21] |
A. Xu, S. Zhou, Y. Tang, A unified model for system reliability evaluation under dynamic operating conditions, IEEE Trans. Reliab., 70 (2019), 65–72. https://doi.org/10.1109/TR.2019.2948173 doi: 10.1109/TR.2019.2948173
![]() |
[22] |
C. Luo, L. Shen, A. Xu, Modelling and estimation of system reliability under dynamic operating environments and lifetime ordering constraints, Reliab. Eng. Syst. Saf., 218 (2022), 108136. https://doi.org/10.1016/j.ress.2021.108136 doi: 10.1016/j.ress.2021.108136
![]() |
[23] |
G. Aryal, I. Elbatal, On the exponentiated generalized modified Weibull distribution, Commun. Stat. Appl. Methods, 22 (2015), 333–348. http://dx.doi.org/10.5351/CSAM.2015.22.4.333 doi: 10.5351/CSAM.2015.22.4.333
![]() |
[24] |
L. Zhang, A. Xu, L. An, M. Li, Bayesian inference of system reliability for multicomponent stress-strength model under Marshall-Olkin Weibull distribution, Systems, 10 (2022), 196. https://doi.org/10.3390/systems10060196 doi: 10.3390/systems10060196
![]() |
[25] |
L. Zhuang, A. Xu, B. Wang, Y. Xue, S. Zhang, Data analysis of progressive-stress accelerated life tests with group effects, Qual. Technol. Quant. Manage., 2022 (2022), 1–21. https://doi.org/10.1080/16843703.2022.2147690 doi: 10.1080/16843703.2022.2147690
![]() |
1. | Patchanok Srisuradetchai, Jatuporn Somsamai, Wikanda Phaphan, Modified likelihood approach for Wald-typed interval of the shape parameter in Weibull distribution, 2025, 10, 2473-6988, 1, 10.3934/math.2025001 |
Par. | n | Bias | MSEs | 90%L | 90%U | 90%W | 95%L | 95%U | 95%W |
ˆβ | 25 | 1.284 | 1.955 | 0.001 | 5.89 | 5.89 | 0.001 | 6.516 | 6.516 |
50 | 1.163 | 1.479 | 0.137 | 4.989 | 4.852 | 0.001 | 5.462 | 5.462 | |
100 | 1.124 | 1.325 | 0.35 | 4.698 | 4.347 | 0.001 | 5.122 | 5.122 | |
200 | 1.099 | 1.241 | 0.464 | 4.533 | 4.069 | 0.067 | 4.93 | 4.863 | |
400 | 1.08 | 1.18 | 0.545 | 4.415 | 3.87 | 0.167 | 4.793 | 4.625 | |
600 | 1.076 | 1.169 | 0.559 | 4.394 | 3.835 | 0.185 | 4.768 | 4.583 | |
800 | 1.079 | 1.171 | 0.559 | 4.399 | 3.841 | 0.184 | 4.774 | 4.59 | |
1000 | 1.078 | 1.169 | 0.561 | 4.395 | 3.834 | 0.187 | 4.77 | 4.582 | |
ˆγ | 25 | -0.307 | 0.403 | 2.032 | 3.354 | 1.322 | 1.903 | 3.483 | 1.58 |
50 | -0.428 | 0.311 | 2.061 | 3.082 | 1.021 | 1.962 | 3.182 | 1.22 | |
100 | -0.475 | 0.288 | 2.053 | 2.997 | 0.944 | 1.961 | 3.089 | 1.129 | |
200 | -0.502 | 0.281 | 2.038 | 2.959 | 0.921 | 1.948 | 3.049 | 1.101 | |
400 | -0.51 | 0.276 | 2.038 | 2.941 | 0.904 | 1.949 | 3.03 | 1.08 | |
600 | -0.52 | 0.28 | 2.02 | 2.939 | 0.919 | 1.931 | 3.029 | 1.099 | |
800 | -0.521 | 0.279 | 2.021 | 2.937 | 0.915 | 1.932 | 3.026 | 1.094 | |
1000 | -0.524 | 0.28 | 2.018 | 2.935 | 0.918 | 1.928 | 3.025 | 1.097 | |
ˆω | 25 | 0.99 | 1.265 | 0.616 | 4.764 | 4.148 | 0.212 | 5.169 | 4.957 |
50 | 0.886 | 0.921 | 1.076 | 4.096 | 3.02 | 0.781 | 4.39 | 3.609 | |
100 | 0.827 | 0.743 | 1.308 | 3.746 | 2.438 | 1.07 | 3.984 | 2.914 | |
200 | 0.798 | 0.668 | 1.403 | 3.593 | 2.19 | 1.189 | 3.807 | 2.617 | |
400 | 0.783 | 0.629 | 1.452 | 3.515 | 2.062 | 1.251 | 3.716 | 2.465 | |
600 | 0.78 | 0.619 | 1.466 | 3.495 | 2.029 | 1.268 | 3.693 | 2.425 | |
800 | 0.777 | 0.61 | 1.476 | 3.477 | 2.002 | 1.281 | 3.673 | 2.392 | |
1000 | 0.772 | 0.601 | 1.486 | 3.457 | 1.971 | 1.294 | 3.649 | 2.355 | |
ˆδ | 25 | 1.102 | 1.355 | 0.379 | 4.824 | 4.445 | -0.054 | 5.258 | 5.312 |
50 | 1.057 | 1.212 | 0.57 | 4.544 | 3.974 | 0.182 | 4.932 | 4.75 | |
100 | 1.017 | 1.086 | 0.735 | 4.298 | 3.563 | 0.387 | 4.646 | 4.258 | |
200 | 1.011 | 1.052 | 0.786 | 4.236 | 3.449 | 0.45 | 4.572 | 4.123 | |
400 | 0.986 | 0.987 | 0.867 | 4.105 | 3.238 | 0.551 | 4.421 | 3.869 | |
600 | 0.978 | 0.966 | 0.893 | 4.063 | 3.17 | 0.584 | 4.373 | 3.788 | |
800 | 0.978 | 0.965 | 0.896 | 4.06 | 3.164 | 0.588 | 4.369 | 3.781 | |
1000 | 0.977 | 0.961 | 0.902 | 4.052 | 3.151 | 0.594 | 4.36 | 3.765 | |
ˆν | 25 | 0.4 | 0.16 | 0.938 | 1.462 | 0.525 | 0.886 | 1.514 | 0.627 |
50 | 0.4 | 0.16 | 0.938 | 1.462 | 0.525 | 0.886 | 1.514 | 0.627 | |
100 | 0.4 | 0.16 | 0.938 | 1.462 | 0.525 | 0.886 | 1.514 | 0.627 | |
200 | 0.4 | 0.16 | 0.938 | 1.462 | 0.525 | 0.886 | 1.514 | 0.627 | |
400 | 0.4 | 0.16 | 0.938 | 1.462 | 0.525 | 0.886 | 1.514 | 0.627 | |
600 | 0.4 | 0.16 | 0.938 | 1.462 | 0.525 | 0.886 | 1.514 | 0.627 | |
800 | 0.4 | 0.16 | 0.938 | 1.462 | 0.525 | 0.886 | 1.514 | 0.627 | |
1000 | 0.4 | 0.16 | 0.938 | 1.462 | 0.525 | 0.886 | 1.514 | 0.627 |
Par. | n | Bias | MSEs | 90%L | 90%U | 90%W | 95%L | 95%U | 95%W |
ˆβ | 25 | 0.498 | 0.303 | 0.401 | 1.394 | 0.994 | 0.304 | 1.491 | 1.187 |
ˆβ | 25 | 0.498 | 0.303 | 0.401 | 1.394 | 0.994 | 0.304 | 1.491 | 1.187 |
50 | 0.458 | 0.226 | 0.487 | 1.229 | 0.742 | 0.415 | 1.302 | 0.887 | |
100 | 0.437 | 0.197 | 0.514 | 1.161 | 0.647 | 0.451 | 1.224 | 0.774 | |
200 | 0.426 | 0.187 | 0.519 | 1.133 | 0.613 | 0.459 | 1.193 | 0.733 | |
400 | 0.41 | 0.177 | 0.519 | 1.101 | 0.582 | 0.462 | 1.158 | 0.695 | |
600 | 0.404 | 0.175 | 0.517 | 1.091 | 0.574 | 0.461 | 1.147 | 0.686 | |
800 | 0.396 | 0.171 | 0.516 | 1.077 | 0.561 | 0.461 | 1.131 | 0.67 | |
1000 | 0.385 | 0.165 | 0.514 | 1.055 | 0.541 | 0.461 | 1.108 | 0.647 | |
ˆγ | 25 | -0.098 | 0.073 | 0.783 | 1.021 | 0.238 | 0.76 | 1.044 | 0.284 |
50 | -0.147 | 0.034 | 0.797 | 0.908 | 0.111 | 0.787 | 0.919 | 0.132 | |
100 | -0.159 | 0.03 | 0.792 | 0.89 | 0.098 | 0.782 | 0.9 | 0.117 | |
200 | -0.164 | 0.03 | 0.787 | 0.884 | 0.097 | 0.778 | 0.894 | 0.116 | |
400 | -0.16 | 0.028 | 0.794 | 0.885 | 0.091 | 0.785 | 0.894 | 0.109 | |
600 | -0.158 | 0.027 | 0.798 | 0.887 | 0.089 | 0.789 | 0.896 | 0.107 | |
800 | -0.157 | 0.027 | 0.799 | 0.888 | 0.089 | 0.79 | 0.896 | 0.106 | |
1000 | -0.154 | 0.027 | 0.802 | 0.89 | 0.088 | 0.794 | 0.898 | 0.105 | |
ˆω | 25 | -0.796 | 0.691 | 0.001 | 2.037 | 2.037 | 0.001 | 2.258 | 2.258 |
50 | -0.836 | 0.716 | 0.001 | 2.038 | 2.038 | 0.001 | 2.267 | 2.267 | |
100 | -0.855 | 0.741 | 0.001 | 2.06 | 2.06 | 0.001 | 2.297 | 2.297 | |
200 | -0.849 | 0.737 | 0.001 | 2.059 | 2.059 | 0.001 | 2.295 | 2.295 | |
400 | -0.83 | 0.723 | 0.001 | 2.057 | 2.057 | 0.001 | 2.288 | 2.288 | |
600 | -0.815 | 0.709 | 0.001 | 2.049 | 2.049 | 0.001 | 2.276 | 2.276 | |
800 | -0.802 | 0.699 | 0.001 | 2.044 | 2.044 | 0.001 | 2.268 | 2.268 | |
1000 | -0.783 | 0.683 | 0.001 | 2.037 | 2.037 | 0.001 | 2.255 | 2.255 | |
ˆδ | 25 | -0.597 | 0.415 | 0.222 | 1.584 | 1.362 | 0.089 | 1.717 | 1.628 |
50 | -0.637 | 0.422 | 0.17 | 1.556 | 1.386 | 0.035 | 1.691 | 1.656 | |
100 | -0.654 | 0.436 | 0.131 | 1.561 | 1.43 | 0.001 | 1.701 | 1.709 | |
200 | -0.652 | 0.436 | 0.134 | 1.563 | 1.429 | 0.001 | 1.702 | 1.707 | |
400 | -0.657 | 0.441 | 0.12 | 1.565 | 1.445 | 0.001 | 1.706 | 1.706 | |
600 | -0.651 | 0.435 | 0.136 | 1.563 | 1.427 | 0.001 | 1.702 | 1.702 | |
800 | -0.638 | 0.428 | 0.161 | 1.564 | 1.403 | 0.024 | 1.701 | 1.677 | |
1000 | -0.627 | 0.421 | 0.183 | 1.563 | 1.38 | 0.048 | 1.698 | 1.65 | |
ˆν | 25 | -0.899 | 0.863 | 0.001 | 2.316 | 2.316 | 0.001 | 2.592 | 2.592 |
50 | -0.941 | 0.904 | 0.001 | 2.341 | 2.341 | 0.001 | 2.63 | 2.63 | |
100 | -0.953 | 0.921 | 0.001 | 2.356 | 2.356 | 0.001 | 2.651 | 2.651 | |
200 | -0.948 | 0.918 | 0.001 | 2.358 | 2.358 | 0.001 | 2.652 | 2.652 | |
400 | -0.924 | 0.897 | 0.001 | 2.347 | 2.347 | 0.001 | 2.635 | 2.635 | |
600 | -0.905 | 0.876 | 0.001 | 2.332 | 2.332 | 0.001 | 2.612 | 2.612 | |
800 | -0.894 | 2.33 | 0.001 | 2.848 | 2.848 | 0.001 | 2.6005 | 2.601 | |
1000 | -0.874 | 2.319 | 0.001 | 2.785 | 2.785 | 0.001 | 2.59 | 2.59 |
Min. | 1st Qu. | Median | Mean | 3rd Qu. | Max. |
0.058 | 0.254 | 1.600 | 3.410 | 4.534 | 15.080 |
Model | AIC | CAIC | BIC | HQIC |
GWMWD(0.058, 9.986, 0.005, 1.239, 0.056) | 212.9574 | 214.3211 | 222.5175 | 216.5980 |
GWWD(1.916, 0.972, 0.326, 0.635) | 213.6829 | 214.5718 | 221.3310 | 216.5953 |
GWRD(0.360, 0.246, 3.774) | 221.4256 | 221.9473 | 227.1617 | 223.609 |
GLWEXPD(0.466, 0.455, 0.715) | 213.2008 | 213.7226 | 218.9369 | 215.3852 |
GWEXPD(0.465, 0.640, 0.902, 0.007) | 216.9577 | 217.8465 | 224.6058 | 219.8701 |
Model | KS | p-value |
GWMWD(0.058, 9.986, 0.005, 1.239, 0.056) | 0.13263 | 0.3144 |
GWWD(1.916, 0.972, 0.326, 0.635) | 0.16936 | 0.2247 |
GWRD(0.360, 0.246, 3.774) | 0.14916 | 0.1952 |
GLWEXPD(0.466, 0.455, 0.715) | 0.14875 | 0.1977 |
GWEXPD(0.465, 0.640, 0.902, 0.007) | 0.17732 | 0.07589 |
Par. | n | Bias | MSEs | 90%L | 90%U | 90%W | 95%L | 95%U | 95%W |
ˆβ | 25 | 1.284 | 1.955 | 0.001 | 5.89 | 5.89 | 0.001 | 6.516 | 6.516 |
50 | 1.163 | 1.479 | 0.137 | 4.989 | 4.852 | 0.001 | 5.462 | 5.462 | |
100 | 1.124 | 1.325 | 0.35 | 4.698 | 4.347 | 0.001 | 5.122 | 5.122 | |
200 | 1.099 | 1.241 | 0.464 | 4.533 | 4.069 | 0.067 | 4.93 | 4.863 | |
400 | 1.08 | 1.18 | 0.545 | 4.415 | 3.87 | 0.167 | 4.793 | 4.625 | |
600 | 1.076 | 1.169 | 0.559 | 4.394 | 3.835 | 0.185 | 4.768 | 4.583 | |
800 | 1.079 | 1.171 | 0.559 | 4.399 | 3.841 | 0.184 | 4.774 | 4.59 | |
1000 | 1.078 | 1.169 | 0.561 | 4.395 | 3.834 | 0.187 | 4.77 | 4.582 | |
ˆγ | 25 | -0.307 | 0.403 | 2.032 | 3.354 | 1.322 | 1.903 | 3.483 | 1.58 |
50 | -0.428 | 0.311 | 2.061 | 3.082 | 1.021 | 1.962 | 3.182 | 1.22 | |
100 | -0.475 | 0.288 | 2.053 | 2.997 | 0.944 | 1.961 | 3.089 | 1.129 | |
200 | -0.502 | 0.281 | 2.038 | 2.959 | 0.921 | 1.948 | 3.049 | 1.101 | |
400 | -0.51 | 0.276 | 2.038 | 2.941 | 0.904 | 1.949 | 3.03 | 1.08 | |
600 | -0.52 | 0.28 | 2.02 | 2.939 | 0.919 | 1.931 | 3.029 | 1.099 | |
800 | -0.521 | 0.279 | 2.021 | 2.937 | 0.915 | 1.932 | 3.026 | 1.094 | |
1000 | -0.524 | 0.28 | 2.018 | 2.935 | 0.918 | 1.928 | 3.025 | 1.097 | |
ˆω | 25 | 0.99 | 1.265 | 0.616 | 4.764 | 4.148 | 0.212 | 5.169 | 4.957 |
50 | 0.886 | 0.921 | 1.076 | 4.096 | 3.02 | 0.781 | 4.39 | 3.609 | |
100 | 0.827 | 0.743 | 1.308 | 3.746 | 2.438 | 1.07 | 3.984 | 2.914 | |
200 | 0.798 | 0.668 | 1.403 | 3.593 | 2.19 | 1.189 | 3.807 | 2.617 | |
400 | 0.783 | 0.629 | 1.452 | 3.515 | 2.062 | 1.251 | 3.716 | 2.465 | |
600 | 0.78 | 0.619 | 1.466 | 3.495 | 2.029 | 1.268 | 3.693 | 2.425 | |
800 | 0.777 | 0.61 | 1.476 | 3.477 | 2.002 | 1.281 | 3.673 | 2.392 | |
1000 | 0.772 | 0.601 | 1.486 | 3.457 | 1.971 | 1.294 | 3.649 | 2.355 | |
ˆδ | 25 | 1.102 | 1.355 | 0.379 | 4.824 | 4.445 | -0.054 | 5.258 | 5.312 |
50 | 1.057 | 1.212 | 0.57 | 4.544 | 3.974 | 0.182 | 4.932 | 4.75 | |
100 | 1.017 | 1.086 | 0.735 | 4.298 | 3.563 | 0.387 | 4.646 | 4.258 | |
200 | 1.011 | 1.052 | 0.786 | 4.236 | 3.449 | 0.45 | 4.572 | 4.123 | |
400 | 0.986 | 0.987 | 0.867 | 4.105 | 3.238 | 0.551 | 4.421 | 3.869 | |
600 | 0.978 | 0.966 | 0.893 | 4.063 | 3.17 | 0.584 | 4.373 | 3.788 | |
800 | 0.978 | 0.965 | 0.896 | 4.06 | 3.164 | 0.588 | 4.369 | 3.781 | |
1000 | 0.977 | 0.961 | 0.902 | 4.052 | 3.151 | 0.594 | 4.36 | 3.765 | |
ˆν | 25 | 0.4 | 0.16 | 0.938 | 1.462 | 0.525 | 0.886 | 1.514 | 0.627 |
50 | 0.4 | 0.16 | 0.938 | 1.462 | 0.525 | 0.886 | 1.514 | 0.627 | |
100 | 0.4 | 0.16 | 0.938 | 1.462 | 0.525 | 0.886 | 1.514 | 0.627 | |
200 | 0.4 | 0.16 | 0.938 | 1.462 | 0.525 | 0.886 | 1.514 | 0.627 | |
400 | 0.4 | 0.16 | 0.938 | 1.462 | 0.525 | 0.886 | 1.514 | 0.627 | |
600 | 0.4 | 0.16 | 0.938 | 1.462 | 0.525 | 0.886 | 1.514 | 0.627 | |
800 | 0.4 | 0.16 | 0.938 | 1.462 | 0.525 | 0.886 | 1.514 | 0.627 | |
1000 | 0.4 | 0.16 | 0.938 | 1.462 | 0.525 | 0.886 | 1.514 | 0.627 |
Par. | n | Bias | MSEs | 90%L | 90%U | 90%W | 95%L | 95%U | 95%W |
ˆβ | 25 | 0.498 | 0.303 | 0.401 | 1.394 | 0.994 | 0.304 | 1.491 | 1.187 |
ˆβ | 25 | 0.498 | 0.303 | 0.401 | 1.394 | 0.994 | 0.304 | 1.491 | 1.187 |
50 | 0.458 | 0.226 | 0.487 | 1.229 | 0.742 | 0.415 | 1.302 | 0.887 | |
100 | 0.437 | 0.197 | 0.514 | 1.161 | 0.647 | 0.451 | 1.224 | 0.774 | |
200 | 0.426 | 0.187 | 0.519 | 1.133 | 0.613 | 0.459 | 1.193 | 0.733 | |
400 | 0.41 | 0.177 | 0.519 | 1.101 | 0.582 | 0.462 | 1.158 | 0.695 | |
600 | 0.404 | 0.175 | 0.517 | 1.091 | 0.574 | 0.461 | 1.147 | 0.686 | |
800 | 0.396 | 0.171 | 0.516 | 1.077 | 0.561 | 0.461 | 1.131 | 0.67 | |
1000 | 0.385 | 0.165 | 0.514 | 1.055 | 0.541 | 0.461 | 1.108 | 0.647 | |
ˆγ | 25 | -0.098 | 0.073 | 0.783 | 1.021 | 0.238 | 0.76 | 1.044 | 0.284 |
50 | -0.147 | 0.034 | 0.797 | 0.908 | 0.111 | 0.787 | 0.919 | 0.132 | |
100 | -0.159 | 0.03 | 0.792 | 0.89 | 0.098 | 0.782 | 0.9 | 0.117 | |
200 | -0.164 | 0.03 | 0.787 | 0.884 | 0.097 | 0.778 | 0.894 | 0.116 | |
400 | -0.16 | 0.028 | 0.794 | 0.885 | 0.091 | 0.785 | 0.894 | 0.109 | |
600 | -0.158 | 0.027 | 0.798 | 0.887 | 0.089 | 0.789 | 0.896 | 0.107 | |
800 | -0.157 | 0.027 | 0.799 | 0.888 | 0.089 | 0.79 | 0.896 | 0.106 | |
1000 | -0.154 | 0.027 | 0.802 | 0.89 | 0.088 | 0.794 | 0.898 | 0.105 | |
ˆω | 25 | -0.796 | 0.691 | 0.001 | 2.037 | 2.037 | 0.001 | 2.258 | 2.258 |
50 | -0.836 | 0.716 | 0.001 | 2.038 | 2.038 | 0.001 | 2.267 | 2.267 | |
100 | -0.855 | 0.741 | 0.001 | 2.06 | 2.06 | 0.001 | 2.297 | 2.297 | |
200 | -0.849 | 0.737 | 0.001 | 2.059 | 2.059 | 0.001 | 2.295 | 2.295 | |
400 | -0.83 | 0.723 | 0.001 | 2.057 | 2.057 | 0.001 | 2.288 | 2.288 | |
600 | -0.815 | 0.709 | 0.001 | 2.049 | 2.049 | 0.001 | 2.276 | 2.276 | |
800 | -0.802 | 0.699 | 0.001 | 2.044 | 2.044 | 0.001 | 2.268 | 2.268 | |
1000 | -0.783 | 0.683 | 0.001 | 2.037 | 2.037 | 0.001 | 2.255 | 2.255 | |
ˆδ | 25 | -0.597 | 0.415 | 0.222 | 1.584 | 1.362 | 0.089 | 1.717 | 1.628 |
50 | -0.637 | 0.422 | 0.17 | 1.556 | 1.386 | 0.035 | 1.691 | 1.656 | |
100 | -0.654 | 0.436 | 0.131 | 1.561 | 1.43 | 0.001 | 1.701 | 1.709 | |
200 | -0.652 | 0.436 | 0.134 | 1.563 | 1.429 | 0.001 | 1.702 | 1.707 | |
400 | -0.657 | 0.441 | 0.12 | 1.565 | 1.445 | 0.001 | 1.706 | 1.706 | |
600 | -0.651 | 0.435 | 0.136 | 1.563 | 1.427 | 0.001 | 1.702 | 1.702 | |
800 | -0.638 | 0.428 | 0.161 | 1.564 | 1.403 | 0.024 | 1.701 | 1.677 | |
1000 | -0.627 | 0.421 | 0.183 | 1.563 | 1.38 | 0.048 | 1.698 | 1.65 | |
ˆν | 25 | -0.899 | 0.863 | 0.001 | 2.316 | 2.316 | 0.001 | 2.592 | 2.592 |
50 | -0.941 | 0.904 | 0.001 | 2.341 | 2.341 | 0.001 | 2.63 | 2.63 | |
100 | -0.953 | 0.921 | 0.001 | 2.356 | 2.356 | 0.001 | 2.651 | 2.651 | |
200 | -0.948 | 0.918 | 0.001 | 2.358 | 2.358 | 0.001 | 2.652 | 2.652 | |
400 | -0.924 | 0.897 | 0.001 | 2.347 | 2.347 | 0.001 | 2.635 | 2.635 | |
600 | -0.905 | 0.876 | 0.001 | 2.332 | 2.332 | 0.001 | 2.612 | 2.612 | |
800 | -0.894 | 2.33 | 0.001 | 2.848 | 2.848 | 0.001 | 2.6005 | 2.601 | |
1000 | -0.874 | 2.319 | 0.001 | 2.785 | 2.785 | 0.001 | 2.59 | 2.59 |
Min. | 1st Qu. | Median | Mean | 3rd Qu. | Max. |
0.058 | 0.254 | 1.600 | 3.410 | 4.534 | 15.080 |
Model | AIC | CAIC | BIC | HQIC |
GWMWD(0.058, 9.986, 0.005, 1.239, 0.056) | 212.9574 | 214.3211 | 222.5175 | 216.5980 |
GWWD(1.916, 0.972, 0.326, 0.635) | 213.6829 | 214.5718 | 221.3310 | 216.5953 |
GWRD(0.360, 0.246, 3.774) | 221.4256 | 221.9473 | 227.1617 | 223.609 |
GLWEXPD(0.466, 0.455, 0.715) | 213.2008 | 213.7226 | 218.9369 | 215.3852 |
GWEXPD(0.465, 0.640, 0.902, 0.007) | 216.9577 | 217.8465 | 224.6058 | 219.8701 |
Model | KS | p-value |
GWMWD(0.058, 9.986, 0.005, 1.239, 0.056) | 0.13263 | 0.3144 |
GWWD(1.916, 0.972, 0.326, 0.635) | 0.16936 | 0.2247 |
GWRD(0.360, 0.246, 3.774) | 0.14916 | 0.1952 |
GLWEXPD(0.466, 0.455, 0.715) | 0.14875 | 0.1977 |
GWEXPD(0.465, 0.640, 0.902, 0.007) | 0.17732 | 0.07589 |