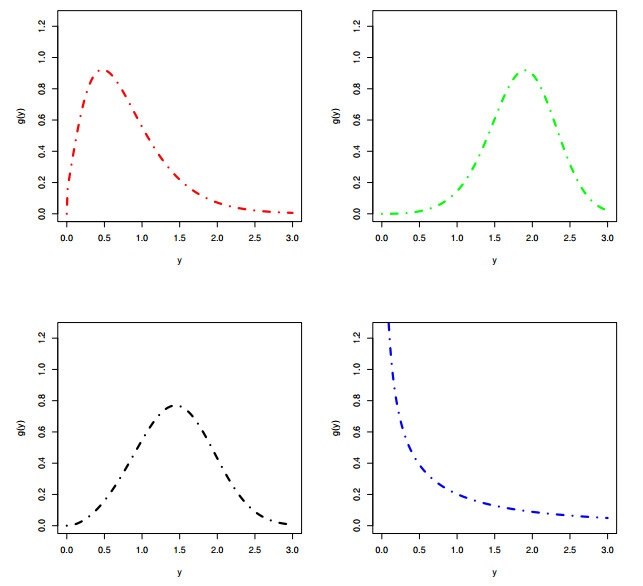
Statistical methods are frequently used in numerous healthcare and other related sectors. One of the possible applications of the statistical methods is to provide the best description of the data sets in the healthcare sector. Keeping in view the applicability of statistical methods in the medical sector, numerous models have been introduced. In this paper, we also introduce a novel statistical method called, a new modified-G family of distributions. Several mathematical properties of the new modified-G family are derived. Based on the new modified-G method, a new updated version of the Weibull model called, a new modified-Weibull distribution is introduced. Furthermore, the estimators of the parameters of the new modified-G distributions are also obtained. Finally, the applicability of the new modified-Weibull distribution is illustrated by analyzing two medical sets. Using certain analytical tools, it is observed that the new modified-Weibull distribution is the best choice to deal with the medical data sets.
Citation: Mahmoud El-Morshedy, Zubair Ahmad, Elsayed tag-Eldin, Zahra Almaspoor, Mohamed S. Eliwa, Zahoor Iqbal. A new statistical approach for modeling the bladder cancer and leukemia patients data sets: Case studies in the medical sector[J]. Mathematical Biosciences and Engineering, 2022, 19(10): 10474-10492. doi: 10.3934/mbe.2022490
[1] | Yinghui Zhou, Zubair Ahmad, Zahra Almaspoor, Faridoon Khan, Elsayed tag-Eldin, Zahoor Iqbal, Mahmoud El-Morshedy . On the implementation of a new version of the Weibull distribution and machine learning approach to model the COVID-19 data. Mathematical Biosciences and Engineering, 2023, 20(1): 337-364. doi: 10.3934/mbe.2023016 |
[2] | Rashad A. R. Bantan, Zubair Ahmad, Faridoon Khan, Mohammed Elgarhy, Zahra Almaspoor, G. G. Hamedani, Mahmoud El-Morshedy, Ahmed M. Gemeay . Predictive modeling of the COVID-19 data using a new version of the flexible Weibull model and machine learning techniques. Mathematical Biosciences and Engineering, 2023, 20(2): 2847-2873. doi: 10.3934/mbe.2023134 |
[3] | Eugene Kashdan, Svetlana Bunimovich-Mendrazitsky . Hybrid discrete-continuous model of invasive bladder cancer. Mathematical Biosciences and Engineering, 2013, 10(3): 729-742. doi: 10.3934/mbe.2013.10.729 |
[4] | K. E. Starkov, Svetlana Bunimovich-Mendrazitsky . Dynamical properties and tumor clearance conditions for a nine-dimensional model of bladder cancer immunotherapy. Mathematical Biosciences and Engineering, 2016, 13(5): 1059-1075. doi: 10.3934/mbe.2016030 |
[5] | Guobing Lin, Cunming Zhang, Xuanyu Chen, Junwei Wang, Song Chen, Siyuan Tang, Tianqiang Yu . Identification of circulating miRNAs as novel prognostic biomarkers for bladder cancer. Mathematical Biosciences and Engineering, 2020, 17(1): 834-844. doi: 10.3934/mbe.2020044 |
[6] | M. E. Bakr, Abdulhakim A. Al-Babtain, Zafar Mahmood, R. A. Aldallal, Saima Khan Khosa, M. M. Abd El-Raouf, Eslam Hussam, Ahmed M. Gemeay . Statistical modelling for a new family of generalized distributions with real data applications. Mathematical Biosciences and Engineering, 2022, 19(9): 8705-8740. doi: 10.3934/mbe.2022404 |
[7] | Svetlana Bunimovich-Mendrazitsky, Yakov Goltser . Use of quasi-normal form to examine stability of tumor-free equilibrium in a mathematical model of bcg treatment of bladder cancer. Mathematical Biosciences and Engineering, 2011, 8(2): 529-547. doi: 10.3934/mbe.2011.8.529 |
[8] | Amal S. Hassan, Najwan Alsadat, Christophe Chesneau, Ahmed W. Shawki . A novel weighted family of probability distributions with applications to world natural gas, oil, and gold reserves. Mathematical Biosciences and Engineering, 2023, 20(11): 19871-19911. doi: 10.3934/mbe.2023880 |
[9] | Liang-Sian Lin, Susan C Hu, Yao-San Lin, Der-Chiang Li, Liang-Ren Siao . A new approach to generating virtual samples to enhance classification accuracy with small data—a case of bladder cancer. Mathematical Biosciences and Engineering, 2022, 19(6): 6204-6233. doi: 10.3934/mbe.2022290 |
[10] | Zekun Xin, Yang Li, Lingyin Meng, Lijun Dong, Jing Ren, Jianlong Men . Elevated expression of the MYB proto-oncogene like 2 (MYBL2)-encoding gene as a prognostic and predictive biomarker in human cancers. Mathematical Biosciences and Engineering, 2022, 19(2): 1825-1842. doi: 10.3934/mbe.2022085 |
Statistical methods are frequently used in numerous healthcare and other related sectors. One of the possible applications of the statistical methods is to provide the best description of the data sets in the healthcare sector. Keeping in view the applicability of statistical methods in the medical sector, numerous models have been introduced. In this paper, we also introduce a novel statistical method called, a new modified-G family of distributions. Several mathematical properties of the new modified-G family are derived. Based on the new modified-G method, a new updated version of the Weibull model called, a new modified-Weibull distribution is introduced. Furthermore, the estimators of the parameters of the new modified-G distributions are also obtained. Finally, the applicability of the new modified-Weibull distribution is illustrated by analyzing two medical sets. Using certain analytical tools, it is observed that the new modified-Weibull distribution is the best choice to deal with the medical data sets.
It is a well-recognized fact that statistical methodologies are effectively used in the analysis of healthcare data sets. For example, (i) the number of admitted patients to the hospital during a week, month, or year, (ii) the survival times of the patients, (iii) the death rate of the patients, (iv) the survival rate of the patients, (v) the recovered rate of the patients, (iv) the number of discharged patients during a week, month, or year. In the healthcare sector, several studies about the implementation of statistical methods have appeared. For detailed information, please see El-Morshedy et al. [1], de Villiers et al. [2], Eliwa et al. [3], Sivaparthipan et al. [4], Urlacher [5], Sandberg et al. [6], Altun et al. [7], Altaf-Ul-Amin et al. [8], Eliwa et al. [9], Ratnovsky et al. [10], Onchonga et al. [11], El-Morshedy et al. [12], El-Morshedy et al. [13], and Illescas et al. [14].
Due to the recognized importance of statistical methodologies and models in the healthcare sector, numerous statistical models have been introduced and implemented. For example, (i) Jones et al. [15] used a bivariate version of the power generalized Weibull (PG-Wei) distribution for survival analysis, (ii) Looha et al. [16] introduced a mixture of the Weibull model for analyzing the survival times of male and female patients, (iii) Kumar et al. [17] used a generalized version of the Log-Weibull (L-Wei) distribution for modeling the medical data sets. (iv) Liu et al. [18] proposed a new statistical distribution for analyzing the survival times of the COVID-19 infected patients. (v) Omer et al. [19] implemented a mixture of the generalized modified Weibull (GM-Wei) distribution for analyzing a medical data set related to leukemia patients. (vi) Mohammed et al. [20] proposed a new logarithmic version of the Weibull distribution for analyzing the bladder cancer data, and (vii) Klakattawi [21] implemented a new extended Weibull (NEx-Wei) distribution for conducting the survival analysis of the cancer patient's data set.
To further improve the fitting power of the statistical distributions, numerous approaches have appeared in the literature. For example, (i) a new family of heavy-tailed (NFHT) models introduced by Arif et al. [22]. (ii) a new generalized-X family studied by Wang et al. [23], (iii) a new extended alpha power transformed (NEAPTrans) family introduced by Ahmad et al. [24], (iv) a class of claim distributions of Ahmad et al. [25], and (v) a new flexible exponentiated-X (NFEx-X) family of Arif et al. [26]. For the latest review about the recent development of statistical methods; see Ahmad et al. [27]
In this paper, we also introduce a new approach for data modeling in the medical sector. The new approach is called, a new modified-G (for 'NModi-G') family of distributions.
Definition: A random variable Y has the NModi-G family, if its DF G(y;σ,ϕ) is given by
G(y;σ,ϕ)=F(y;ϕ)σ[σ−1+F(y;ϕ)], | (1.1) |
where σ≥1,σ≤−1,y∈R, and F(y;ϕ) is a baseline DF. The proofs in Propositions 1 and 2, we show that G(y;σ,ϕ) is a compact DF.
Proposition 1. Using the DF G(y;σ,ϕ) given in Eq (1.1), we have to prove that limy→−∞G(y;σ,ϕ)=0 and limy→∞G(y;σ,ϕ)=1.
Proof.
limy→−∞G(y;σ,ϕ)=limy→−∞{F(y;ϕ)σ[σ−1+F(y;ϕ)]}, |
limy→−∞G(y;σ,ϕ)=F(−∞;ϕ)σ[σ−1+F(−∞;ϕ)], |
limy→−∞G(y;σ,ϕ)=0. |
Also, we have
limy→∞G(y;σ,ϕ)=limy→∞{F(y;ϕ)σ[σ−1+F(y;ϕ)]}, |
limy→∞G(y;σ,ϕ)=F(∞;ϕ)σ[σ−1+F(∞;ϕ)], |
limy→−∞G(y;σ,ϕ)=1. |
Proposition 2. The DF G(y;σ,ϕ) in Eq (1.1), is differentiable and right continuous.
Proof.
ddyG(y;σ,ϕ)=g(y;σ,ϕ). |
From the mathematical results proved in Propositions 1 and 2, we can see that G(y;σ,ϕ) is a valid DF.
For y∈R, corresponding to G(y;σ,ϕ), the PDF (probability density function) g(y;σ,ϕ), is given by
g(y;σ,ϕ)=f(y;ϕ)σ[σ−1+2F(y;ϕ)], | (1.2) |
where ddyF(y;ϕ)=f(y;ϕ).
In link to G(y;σ,ϕ) and g(y;σ,ϕ), the SF (survival function) S(y;σ,ϕ)=1−G(y;σ,ϕ), HF (hazard function) h(y;σ,ϕ)=g(y;σ,ϕ)1−G(y;σ,ϕ), and cumulative HF (CHF) H(y;σ,ϕ)=−log[1−G(y;σ,ϕ)], are given by
S(y;σ,ϕ)=1−F(y;ϕ)σ[σ−1+F(y;ϕ)], |
h(y;σ,ϕ)=f(y;ϕ)[σ−1+2F(y;ϕ)]σ−F(y;ϕ)[σ−1+F(y;ϕ)], |
and
H(y;σ,ϕ)=−log(1−F(y;ϕ)σ[σ−1+F(y;ϕ)]), |
respectively.
By implementing the DF G(y;σ,ϕ) in Eq (1.1), we introduce an updated version of the Weibull distribution, called a NModi-Weibull distribution. The NModi-Weibull distribution can be considered a special member of the NModi-G family. In Section 2, the expressions for the DF, PDF, SF, HF, and CHF of the NModi-Weibull distribution are obtained.
Consider the DF F(y;ϕ) of the Weibull distribution is given by
F(y;ϕ)=1−e−δyθ,y≥0,θ>0,δ>0, | (2.1) |
with PDF f(y;ϕ) given by
f(y;ϕ)=θδyθ−1e−δyθ,y>0,θ>0,δ>0, | (2.2) |
where ϕ=(θ,δ).
Using Eq (2.1) in Eq (1.1), we get the DF of the NModi-Weibull distribution. Let Y have the NModi-Weibull distribution with parameters δ>0,θ>0,σ≥1, and σ≤−1, then, its DF G(y;σ,ϕ) is given by
G(y;σ,ϕ)=(1−e−δyθ)σ[σ−e−δyθ],y≥0. | (2.3) |
For y>0, the PDF of the NModi-Weibull distribution is given by
g(y;σ,ϕ)=θδyθ−1e−δyθσ[σ+1−2e−δyθ]. | (2.4) |
Different plots of g(y;σ,ϕ) of the NModi-Weibull distribution are provided in Figure 1. The plots of g(y;σ,ϕ) are obtained for (i) θ=1.2,δ=1.8,σ=1.2 (red curve), (ii) θ=3.8,δ=0.1,σ=1.4 (green curve), (iii) θ=2.9,δ=0.3,σ=3.2 (black curve), and (iv) θ=0.5,δ=1.3,σ=3.4 (blue curve).
From the plots of g(y;σ,ϕ) in Figure 1, we can see that the shape of g(y;σ,ϕ) of the NModi-Weibull can be (i) positively skewed (red curve), (ii) left skewed (green curve), (iii) symmetrical (black curve), or (iv) decreasing (blue curve).
Furthermore, the SF, HF, and CHF of the NModi-Weibull distribution are given by
S(y;σ,ϕ)=1−(1−e−δyθ)σ[σ−e−δyθ], |
h(y;σ,ϕ)=θδyθ−1e−δyθ[σ+1−2e−δyθ]σ−(1−e−δyθ)[σ−e−δyθ], |
and
H(y;σ,ϕ)=−log(1−(1−e−δyθ)σ[σ−e−δyθ]), |
respectively.
Different behaviors of h(y;σ,ϕ) of the NModi-Weibull distribution are shown in Figure 2. The visual behaviors of h(y;σ,ϕ) are obtained for (i) θ=1.2,δ=1.8,σ=1.2 (blue curve), (ii) θ=0.5,δ=1.3,σ=3.2 (red curve), and (iii) θ=0.9,δ=0.6,σ=1.7 (green curve).
From the visual behaviors of h(y;σ,ϕ), it can be observed that the shape of the HF of the NModi-Weibull distribution can be (i) decreasing (blue curve), (ii) increasing (red curve), or (iii) bathtub shaped (green curve).
Here, we derive some mathematical properties of the NModi-G distributions. These properties include (i) the quantile function (QF), (ii) the identifiability property (IP), (iii) the rth moment, and moment generating function (MGF).
The QF of the NModi-G distributions can be derived as
y=Q(u)=G−1(u)=F−1(k), |
where k is the solution of (σ−1)k+k2−σu.
This subsection offers the derivation of the identifiability property of the NModi-G distributions using the additional parameter σ. Suppose that the parameter σ1 has the DF G(y;σ1,ϕ) and the parameter σ2 has the DF G(y;σ2,ϕ). The parameter σ is said to be identifiable, if σ1=σ2. Consider
G(y;σ1,ϕ)=G(y;σ2,ϕ). | (3.1) |
Using Eq (1.1) in Eq (3.1), we have
F(y;ϕ)σ1[σ1−1+F(y;ϕ)]=F(y;ϕ)σ2[σ2−1+F(y;ϕ)], |
σ2F(y;ϕ)[σ1−1+F(y;ϕ)]=σ1F(y;ϕ)[σ2−1+F(y;ϕ)], |
σ1σ2F(y;ϕ)−σ2F(y;ϕ)+σ2[F(y;ϕ)]2=σ1σ2F(y;ϕ)−σ1F(y;ϕ)+σ1[F(y;ϕ)]2, |
σ1F(y;ϕ)−σ2F(y;ϕ)+σ2[F(y;ϕ)]2−σ1[F(y;ϕ)]2=0, |
F(y;ϕ)(σ1−σ2)−[F(y;ϕ)]2(σ1−σ2)=0, |
(σ1−σ2)(F(y;ϕ)−[F(y;ϕ)]2)=0, |
(σ1−σ2)=0, |
σ1=σ2. |
This subsection considers the derivation of the rth moment of the NModi-G distributions. Suppose Y has the NModi-G distributions, then the rth moment of Y, say μ′r, is derived as
μ′r=∫Ωyrg(y;σ,ϕ)dy. | (3.2) |
Using Eq (1.2) in Eq (3.2), we get
μ′r=∫Ωyrf(y;ϕ)σ[σ−1+2F(y;ϕ)]dy, |
μ′r=(σ−1σ)∫Ωyrf(y;ϕ)dy+2σ∫Ωyrf(y;ϕ)F(y;ϕ)dy, |
μ′r=(σ−1σ)Δ1+2σΔ2, |
where
Δ1=∫Ωyrf(y;ϕ)dy, |
and
Δ2=∫Ωyrf(y;ϕ)F(y;ϕ)dy. |
The MGF Mt(y) of the NModi-G distributions is given by
μ′r=∞∑r=1trr!(σ−1σ)Δ1+∞∑r=1trr!2σΔ2. |
In this section, we discuss and implement the method of ML (maximum likelihood) to obtain the ML estimators (MLEs) (ˆσMLE,ˆϕMLE) of the parameters (σ,ϕ) of the NModi-G distributions. Furthermore, the performances of ˆσMLE and ˆϕMLE are evaluated using a simulation study.
The method of ML estimation is one of the prominent methods to obtain the MLEs. The estimators obtained via implementing the ML approach enjoy numerous properties such as (i) efficiency, (ii) consistency, and (iii) asymptotic normality. Therefore, we use the ML approach to obtain the estimators of the NModi-G distributions with PDF g(y;σ,ϕ).
Let Y1,Y2,...,Ys be a collection of random samples, say s, taken from the NModi-G distributions. Corresponding to g(y;σ,ϕ), the LikF (likelihood function), say λ(σ,ϕ|y1,y2,...,ys), is given by
λ(σ,ϕ|y1,y2,...,ys)=s∏a=1g(yi;σ,ϕ). | (4.1) |
Using Eq (1.2) in Eq (4.1), we get
λ(σ,ϕ|y1,y2,...,ys)=s∏a=1f(yi;ϕ)σ[σ−1+2F(yi;ϕ)]. | (4.2) |
Corresponding to λ(σ,ϕ|y1,y2,...,ys) presented in Eq (4.2), the log LikF, say λ(y1,y2,...,ys|σ,ϕ), is given by
λ(σ,ϕ|y1,y2,...,ys)=−slogσ+s∑a=1logf(yi;ϕ)+s∑a=1log[σ−1+2F(yi;ϕ)]. |
In link to λ(σ,ϕ|y1,y2,...,ys), the partial derivates are given by
∂∂σλ(σ,ϕ|y1,y2,...,ys)=−sσ+s∑a=11[σ−1+2F(yi;ϕ)], |
and
∂∂ϕλ(σ,ϕ|y1,y2,...,ys)=s∑a=1∂∂ϕf(yi;ϕ)f(yi;ϕ)+2s∑a=1∂∂ϕF(yi;ϕ)[σ−1+2F(yi;ϕ)]. |
By solving ∂∂σλ(σ,ϕ|y1,y2,...,ys)=0 and ∂∂ϕλ(σ,ϕ|y1,y2,...,ys)=0, we obtain the MLEs (ˆσMLE,ˆϕMLE) of the parameters (σ,ϕ).
A Monte Carlo simulation study (MCSS) is implemented to review/evaluate the performance of the estimation method. Here, we provide MCSS to evaluate the MLEs ˆσMLE and ˆϕMLE. To carry out the MCSS, random samples (RSs) from the PDF g(yi;σ,ϕ) are generated using the formula
y=Q(u)=G−1(u)=F−1(k), | (4.3) |
where k is the solution of (σ−1)k+k2−σu.
The MCSS is conducted for (a) θ=1.6,δ=0.5,σ=1.3, and (b) θ=1.5,δ=0.8,σ=1.2. Using the function in Eq (4.3) and the above specific values of θ,δ, and σ, RSs of sizes s=25,50,75,...,500 are generated for the MCSS.
After obtaining the RSs, two statistical measures, such as (a) MSE (mean square error) and (b) Bias are selected to evaluate ˆσMLE and ˆϕMLE. These two quantities are, respectively, computed as
MSE(ˆΞ)=1500500∑i=1(^Ξi−Ξ)2, |
and
Bias(ˆΞ)=1500500∑i=1(^Ξi−Ξ), |
where Ξ=(σ,ϕ).
Corresponding to (a) θ=1.6,δ=0.5,σ=1.3, and (b) θ=1.5,δ=0.8,σ=1.2, the MCSS results for the NModo-Weibull model are presented in Tables 1 and 2, respectively. Furthermore, corresponding to Tables 1 and 2, visual illustrations of the MCSS results are presented in Figures 3 and 4, respectively.
Set 1: θ=1.6,δ=0.5,σ=1.3. | ||||
n | parameters | MLEs | MSEs | Biass |
θ | 2.0264250 | 0.34424293 | 0.42642528 | |
25 | δ | 0.3308959 | 0.04919103 | -0.16910411 |
σ | 3.0607790 | 6.24781140 | 1.96077890 | |
θ | 1.9068750 | 0.18418145 | 0.30687538 | |
50 | δ | 0.3664297 | 0.03407172 | -0.13357025 |
σ | 2.5546780 | 4.57668210 | 1.45467770 | |
θ | 1.8398460 | 0.12796033 | 0.23984625 | |
75 | δ | 0.3861830 | 0.02637042 | -0.11381702 |
σ | 2.2494480 | 3.56365220 | 1.14944810 | |
θ | 1.8022450 | 0.09987249 | 0.20224504 | |
100 | δ | 0.4007103 | 0.02282122 | -0.09928972 |
σ | 2.0751470 | 2.99863540 | 0.97514660 | |
θ | 1.7642540 | 0.07597643 | 0.16425421 | |
150 | δ | 0.4199996 | 0.01762115 | -0.08000036 |
σ | 1.8684900 | 2.37178320 | 0.76848950 | |
θ | 1.7198750 | 0.05149042 | 0.11987515 | |
200 | δ | 0.4394083 | 0.01318933 | -0.06059166 |
σ | 1.6652230 | 1.68975300 | 0.56522340 | |
θ | 1.7109000 | 0.04665635 | 0.11089982 | |
250 | δ | 0.4460993 | 0.01147129 | -0.05390074 |
σ | 1.6108190 | 1.55819453 | 0.51081890 | |
θ | 1.6837020 | 0.03669438 | 0.08370244 | |
300 | δ | 0.4577645 | 0.00964167 | -0.04223553 |
σ | 1.5188100 | 1.34898910 | 0.41881030 | |
θ | 1.7011510 | 0.04038710 | 0.10115076 | |
350 | δ | 0.4484196 | 0.01051719 | -0.05158038 |
σ | 1.5852900 | 1.53231090 | 0.48529030 | |
θ | 1.6654520 | 0.02587484 | 0.06545179 | |
400 | δ | 0.4674469 | 0.00704898 | -0.03255313 |
σ | 1.3942840 | 0.88039980 | 0.29428350 | |
θ | 1.6390040 | 0.02636183 | 0.06900377 | |
450 | δ | 0.4840139 | 0.00717385 | -0.03598612 |
σ | 1.3522010 | 0.88759460 | 0.30220140 | |
θ | 1.6136070 | 0.01555444 | 0.04360732 | |
500 | δ | 0.4975956 | 0.00451764 | -0.02240439 |
σ | 1.2923990 | 0.45577130 | 0.17239860 |
Set 2: θ=1.5,δ=0.8,σ=1.2. | ||||
n | parameters | MLEs | MSEs | Biass |
θ | 2.0216330 | 0.42346514 | 0.52163299 | |
25 | δ | 0.5268598 | 0.10444894 | -0.27314024 |
σ | 3.0711290 | 6.50104860 | 2.05112910 | |
θ | 1.8599560 | 0.22470984 | 0.35995562 | |
50 | δ | 0.5787362 | 0.07833671 | -0.22126382 |
σ | 2.6975860 | 5.59054410 | 1.67758650 | |
θ | 1.7832010 | 0.15911705 | 0.28320139 | |
75 | δ | 0.6200479 | 0.06027562 | -0.17995208 |
σ | 2.3359260 | 4.28183600 | 1.31592640 | |
θ | 1.7617930 | 0.13213407 | 0.26179258 | |
100 | δ | 0.6318675 | 0.05207615 | -0.16813250 |
σ | 2.1996040 | 3.84146220 | 1.17960390 | |
θ | 1.7056680 | 0.08848033 | 0.20566761 | |
150 | δ | 0.6625591 | 0.03944994 | -0.13744086 |
σ | 1.9168200 | 2.92731720 | 0.89682010 | |
θ | 1.6492930 | 0.06256641 | 0.14929256 | |
200 | δ | 0.6994846 | 0.02682993 | -0.10051539 |
σ | 1.6056450 | 1.77894570 | 0.58564520 | |
θ | 1.6322050 | 0.05351188 | 0.13220512 | |
250 | δ | 0.7095510 | 0.02370518 | -0.09044900 |
σ | 1.5534750 | 1.63002940 | 0.53347460 | |
θ | 1.6085810 | 0.03601538 | 0.10858060 | |
300 | δ | 0.7255111 | 0.01709929 | -0.07448886 |
σ | 1.4167820 | 1.16321090 | 0.39678200 | |
θ | 1.5798770 | 0.02485567 | 0.07987703 | |
350 | δ | 0.7456060 | 0.01108549 | -0.05439403 |
σ | 1.2851130 | 0.72728290 | 0.26511340 | |
θ | 1.5515160 | 0.02252164 | 0.08151622 | |
400 | δ | 0.7679470 | 0.01058925 | -0.05720534 |
σ | 1.2542670 | 0.59473740 | 0.23426730 | |
θ | 1.5345300 | 0.01671347 | 0.06453007 | |
450 | δ | 0.7857109 | 0.00806090 | -0.04428908 |
σ | 1.1957780 | 0.41036160 | 0.17877810 | |
θ | 1.5112700 | 0.01539761 | 0.06127049 | |
500 | δ | 0.7976776 | 0.00717589 | -0.04232238 |
σ | 1.1987100 | 0.36360180 | 0.15671040 |
This section illustrates the applicability of the NModi-Weibull distribution in the medical sector. We apply the NModi-Weibull to two medical data sets. The very first data set refers to the bladder cancer patients' data; see Lee et al. [28]. While the second data set refers to the lifetimes of leukemia patients; see El-Gohary et al. [29]. The key measures of the first data set are given by minimum = 0.080, Q1 = 3.348, Q2 = 6.395, Q3 = 11.838, mean/average = 9.366, maximum = 79.050, variance = 110.425, skewness = 3.286569, kurtosis = 18.48308, and range = 8.97. For the second data set, the key measures are given by Minimum = 1.150, Q1 = 8.025, Q2 = 12.220, Q3 = 15.562, mean/average = 11.370, maximum = 18.520, variance = 23.19414, skewness = -0.4879275, kurtosis = 2.271123, and range = 17.37. It is important to note that Q1 refers to the 1st quartile, Q2 refers to the 2nd quartile or median, and Q3 refers to the 3rd quartile.
Using the bladder cancer and leukemia patients' data sets, the comparison of the NModi-Weibull distribution is made with the (i) Weibull distribution, (ii) novel exponent power-Weibull (NEPow-Weibull) distribution, and (iii) an exponential T-X exponentiated exponential (ET-XEE) distribution. The DFs of these models are given by
F(y;ϕ)=1−e−δyθ,y≥0,θ>0,δ>0, |
F(y;β,ϕ)=1−(1−1−e−δyθee−δyθ)β,y≥0,β>0,θ>0,δ>0, |
and
F(y;θ,δ,α)=1−α[1−(1−e−δy)θ]α−(1−e−δy)θ,y≥0,θ>0,δ>0,α>1, |
respectively.
The next step is to choose certain statistical measures to show the best model for the bladder cancer and leukemia patients' data sets. To figure out the best model for medical data sets, three statistical measures (i) Cramer-von Mises (CM), (ii) Anderson-Darling (AD), and (iii) Kolmogorov–Smirnov (SK) test with the p-value are considered.
After carrying out the numerical analysis using the bladder cancer and leukemia patients' data sets, the results of the competing models are presented in Tables 3–6. Tables 3 and 4 offer the MLEs and statistical measures of the fitted models using the bladder cancer data, respectively. Whereas, Tables 5 and 6 offer the MLEs and statistical measures of the fitted models using the leukemia data, respectively. From the numerical evaluations presented in Tables 4 and 6, we can see the NModi-Weibull distribution is the best choice to apply for modeling the medical data sets.
Models | θ | δ | σ | β | α |
NModi-Weibull | 0.75418 | 0.30260 | 1.98970 | - | |
Weibull | 1.05357 | 0.09165 | - | - | |
NEPow-Weibull | 0.91046 | 0.68725 | - | 0.22336 | |
ET-XEE | 1.147472 | 0.12483 | - | - | 6.91687 |
Models | CM | AD | KS | P-value |
NModi-Weibull | 0.05557 | 0.35149 | 0.04976 | 0.90920 |
Weibull | 0.13241 | 0.79257 | 0.07429 | 0.47980 |
NEPow-Weibull | 0.06679 | 0.41012 | 0.05615 | 0.81440 |
ET-XEE | 0.13209 | 0.78746 | 0.07982 | 0.38850 |
Models | θ | δ | σ | β | α |
NModi-Weibull | 2.44343 | 0.00225 | 4.42298 | - | |
Weibull | 2.42921 | 0.00230 | - | - | |
NEPow-Weibull | 1.92969 | 0.12835 | - | 0.06098 | |
ET-XEE | 1.39012 | 0.32552 | - | - | 1.02808 |
Models | CM | AD | KS | P-value |
NModi-Weibull | 0.12637 | 0.82294 | 0.11752 | 0.63850 |
Weibull | 0.14137 | 0.91039 | 0.16746 | 0.21190 |
NEPow-Weibull | 0.21028 | 1.30936 | 0.14832 | 0.34230 |
ET-XEE | 0.10453 | 0.85098 | 0.12098 | 0.58690 |
In addition to the numerical evaluation presented in Tables 4 and 6, a visual evaluation of the NModi-Weibull distribution is also presented; see Figures 5 and 6. From the plots in Figures 5 and 6, we can see that the NModi-Weibull distribution closely follows the fitted PDF, DF, PP (probability-probability), SF, and QQ (quantile-quantile) functions.
When a dataset is impure with a single or few outliers, it causes a serious problem in estimating the parameters. To overcome this problem, we use the robust estimation (RoE) method. The RoE is an important technique for analyzing the data sets that contain outliers.
The robust estimators (RoEs) have a large family such as the least absolute deviation (LAD), the least quantile of squares, the least median of squares, and M-estimation methods. For a brief discussion about the RoEs, we refer to Fang et al. [30], Kantar et al. [31], and Almetwally et al. [32].
In this section, we implement the LAD method to obtain the RoEs of the proposed model. Consider a sample of size s from the NModi-Weibull distribution, then, the RoEs of the NModi-Weibull distribution can be derived as follows
yi=Qi(θ,δ,σ)+ξi,i=1,2,...,n, | (6.1) |
where Qi(θ,δ,σ) is the quantile function of the NModi-Weibull distribution and ξi is the error term with mean equals zero and known variance.
For the RoE method, it is necessary to scale the invariant error (ςi) as follows ςi=ξiΛ, where ξi denotes the ith residual, and Λ=Median{|ξi−Median(ξi)|}0.6745. The constant 0.6745 is chosen so that the RoEs become asymptotically unbiased for a normal error case. The LAD method is obtained by minimizing ςi, where LAD(θ,δ,σ)=min|ςi|.
After differentiating Eq (6.1) with respect to the parameters θ,δ and σ, we get three nonlinear equations that cannot be solved analytically. Hence, iterative procedures like the Newton-Raphson algorithms can be utilized to solve for the solution of the ˆθLAD,ˆδLAD, and ˆσLAD numerically.
As we discussed earlier that the RoE methods can be implemented when there are outliers in the data. In this paper, we have analyzed two data sets; see Section A. The first data set (i.e., the bladder cancer patients' data) has some outliers. Whereas, the second data set (i.e., the lifetimes of leukemia patients) has no outliers. Therefore, we provide the RoE of the NModi-Weibull distribution using the bladder cancer patients data set, only.
The initial density shape is listed using the non-parametric kernel density estimation approach in Figure 7. From Figure 7, we can see that the shape of the density is asymmetric. The normality condition is checked via the QQ plot; see Figure 7. The outliers can also be spotted using the box plot; see Figure 7. Henceforth, we can say that there are outliers in the data.
Based on the LAD approach, the RoEs of the NModi-Weibull parameters are ˆθ=2.3178,ˆδ=0.00127, and ˆσ=4.50187 with P-value =0.71279. It is noted that the LAD approach is the best as compared to the MLE technique due to its high p-value.
Statistical distributions have proven great applicability in healthcare-related sectors. For modeling and describing the medical data sets, numerous statistical distributions have been introduced and implemented. Keeping in view the recognized importance of the statistical methods in the healthcare sector, a new modified-G family was introduced in this paper. Certain mathematical properties of the NM-G distributions were derived. A special sub-case of the NM-G distributions by taking the Weibull distribution as a baseline model was discussed. The special model of the NM-G distributions was named a NModi-Weibull distribution. A simulation study based on the NModi-Weibull distribution has also been carried out. At last, the importance of the NModi-Weibull distribution was illustrated by modeling two data sets (the bladder cancer patient's data and the lifetimes of leukemia patient's data) taken from the healthcare sector. Using these data sets, it is observed that the NModi-Weibull distribution was the best competitor for dealing with the medical data sets.
The authors declare there is no conflict of interest.
[1] |
M. El-Morshedy, M. S. Eliwa, The odd flexible Weibull-H family of distributions: Properties and estimation with applications to complete and upper record data, Filomat, 33 (2019), 2635–2652. https://doi.org/10.2298/FIL1909635E doi: 10.2298/FIL1909635E
![]() |
[2] |
C. B. de Villiers, M. Kroese, S. Moorthie, Understanding polygenic models, their development and the potential application of polygenic scores in healthcare, J. Med. Genet., 57 (2020), 725–732. https://doi.org/10.1136/jmedgenet-2019-106763 doi: 10.1136/jmedgenet-2019-106763
![]() |
[3] |
M. S. Eliwa, Z. A. Alhussain, M. El-Morshedy, Discrete Gompertz-G family of distributions for over-and under-dispersed data with properties, estimation, and applications, Mathematics, 8 (2020), 358. https://doi.org/10.3390/math8030358 doi: 10.3390/math8030358
![]() |
[4] |
C. B. Sivaparthipan, N. Karthikeyan, S. Karthik, Designing statistical assessment healthcare information system for diabetics analysis using big data, Multimedia Tools Appl., 79 (2020), 8431–8444. https://doi.org/10.1007/s11042-018-6648-3 doi: 10.1007/s11042-018-6648-3
![]() |
[5] |
B. R. Urlacher, Complexity, causality, and control in statistical modeling, Am. Behav. Sci., 64 (2020), 55–73. https://doi.org/10.1177/0002764219859641 doi: 10.1177/0002764219859641
![]() |
[6] |
L. Sandberg, H. Taavola, Y. Aoki, R. Chandler, G. N. Norén, Risk factor considerations in statistical signal detection: using subgroup disproportionality to uncover risk groups for adverse drug reactions in VigiBase, Drug Safety, 43 (2020), 999–1009. https://doi.org/10.1007/s40264-020-00957-w doi: 10.1007/s40264-020-00957-w
![]() |
[7] |
E. Altun, M. Ç. Korkmaz, M. El-Morshedy, M. S. Eliwa, A new flexible family of continuous distributions: the additive odd-G family, Mathematics, 9 (2021), 1837. https://doi.org/10.3390/math9161837 doi: 10.3390/math9161837
![]() |
[8] |
M. Altaf-Ul-Amin, S. Kanaya, N. Ono, M. Huang, Recent trends in computational biomedical research, Life, 12 (2021), 27. https://doi.org/10.3390/life12010027 doi: 10.3390/life12010027
![]() |
[9] |
M. S. Eliwa, M. El-Morshedy, S. Ali, Exponentiated odd Chen-G family of distributions: statistical properties, Bayesian and non-Bayesian estimation with applications, J. Appl. Stat., 48 (2021), 1948–1974. https://doi.org/10.1080/02664763.2020.1783520 doi: 10.1080/02664763.2020.1783520
![]() |
[10] |
A. Ratnovsky, S. Rozenes, E. Bloch, P. Halpern, Statistical learning methodologies and admission prediction in an emergency department, Australas. Emergency Care, 24 (2021), 241–247. https://doi.org/10.1016/j.auec.2020.11.004 doi: 10.1016/j.auec.2020.11.004
![]() |
[11] |
D. Onchonga, E. Ngetich, W. Makunda, P. Wainaina, D. Wangeshi, Anxiety and depression due to 2019 SARS-CoV-2 among frontier healthcare workers in Kenya, Heliyon, 7 (2021), e06351. https://doi.org/10.1016/j.heliyon.2021.e06351 doi: 10.1016/j.heliyon.2021.e06351
![]() |
[12] | M. El-Morshedy, E. Altun, M. S. Eliwa, A new statistical approach to model the counts of novel coronavirus cases, Math. Sci., (2021), 1–14. https://doi.org/10.21203/rs.3.rs-31163/v1 |
[13] | M. El-Morshedy, M. S. Eliwa, A. Tyagi, A discrete analogue of odd Weibull-G family of distributions: properties, classical and Bayesian estimation with applications to count data, J. Appl. Stat., (2021), 1–25. https://doi.org/10.1080/02664763.2021.1928018 |
[14] |
A. Illescas, H. Zhong, C. Cozowicz, A. Gonzalez Della Valle, J. Liu, S. G. Memtsoudis, et al., Health services research in anesthesia: a brief overview of common methodologies, Anesth. Analg., 134 (2021), 540–547. https://doi.org/10.1213/ANE.0000000000005884 doi: 10.1213/ANE.0000000000005884
![]() |
[15] |
M. C. Jones, A. Noufaily, K. Burke, A bivariate power generalized Weibull distribution: a flexible parametric model for survival analysis, Stat. Methods Med. Res., 29 (2020), 2295–2306. https://doi.org/10.1177/0962280219890893 doi: 10.1177/0962280219890893
![]() |
[16] |
M. A. Looha, E. Zarean, F. Masaebi, M. A. Pourhoseingholi, M. R. Zali, Assessment of prognostic factors in long-term survival of male and female patients with colorectal cancer using non-mixture cure model based on the Weibull distribution, Surg. Oncol., 38 (2021), 101562. https://doi.org/10.1016/j.suronc.2021.101562 doi: 10.1016/j.suronc.2021.101562
![]() |
[17] |
C. S. Kumar, S. R. Nair, A generalized Log-Weibull distribution with bio-medical appligcations, Int. J. Stat. Med. Res., 10 (2021), 10–21. https://doi.org/10.6000/1929-6029.2021.10.02 doi: 10.6000/1929-6029.2021.10.02
![]() |
[18] |
X. Liu, Z. Ahmad, A. M. Gemeay, A. T. Abdulrahman, E. H. Hafez, N. Khalil, Modeling the survival times of the COVID-19 patients with a new statistical model: A case study from China, Plos One, 16 (2021), e0254999. https://doi.org/10.1371/journal.pone.0254999 doi: 10.1371/journal.pone.0254999
![]() |
[19] |
M. E. Omer, M. A. Bakar, M. Adam, M. Mustafa, Utilization of a mixture cure rate model based on the generalized modified Weibull distribution for the analysis of leukemia patients, Asian Pac. J. Cancer Prev., 22 (2021), 1045. https://doi.org/10.31557/APJCP.2021.22.4.1045 doi: 10.31557/APJCP.2021.22.4.1045
![]() |
[20] |
H. S. Mohammed, Z. Ahmad, A. T. Abdulrahman, S. K. Khosa, E. H. Hafez, M. M. Abd El-Raouf, et al., Statistical modelling for Bladder cancer disease using the NLT-W distribution, AIMS Math., 6 (2021), 9262–9276. https://doi.org/10.3934/math.2021538 doi: 10.3934/math.2021538
![]() |
[21] |
H. S. Klakattawi, Survival analysis of cancer patients using a new extended Weibull distribution, Plos One, 17 (2021), e0264229. https://doi.org/10.1371/journal.pone.0264229 doi: 10.1371/journal.pone.0264229
![]() |
[22] |
M. Arif, D. M. Khan, S. K. Khosa, M. Aamir, A. Aslam, Z. Ahmad, et al., Modelling insurance losses with a new family of heavy-tailed distributions, Comput. Mater. Continua, 66 (2021), 537–550. https://doi.org/10.32604/cmc.2020.012420 doi: 10.32604/cmc.2020.012420
![]() |
[23] |
W. Wang, Z. Ahmad, O. Kharazmi, C. B. Ampadu, E. H. Hafez, M. M. Mohie El-Din, New generalized-X family: modeling the reliability engineering applications, Plos One, 16 (2021), e0248312. https://doi.org/10.1371/journal.pone.0248312 doi: 10.1371/journal.pone.0248312
![]() |
[24] |
Z. Ahmad, E. Mahmoudi, G. G. Hamedani, A new extended alpha power transformed family of distributions: properties, characterizations and an application to a data set in the insurance sciences, Commun. Stat. Appl. Methods, 28 (2021), 1–19. https://doi.org/10.29220/CSAM.2021.28.1.001 doi: 10.29220/CSAM.2021.28.1.001
![]() |
[25] |
Z. Ahmad, E. Mahmoudi, G. Hamedani, A class of claim distributions: properties, characterizations and applications to insurance claim data, Commun. Stat. Theory Methods, 51 (2022), 2183–2208. https://doi.org/10.1080/03610926.2020.1772306 doi: 10.1080/03610926.2020.1772306
![]() |
[26] | M. Arif, D. M. Khan, M. Aamir, M. El-Morshedy, Z. Ahmad, Z. Khan, A new flexible exponentiated-X family of distributions: characterizations and applications to lifetime data, IETE J. Res., (2022), 1–13. https://doi.org/10.1080/03772063.2022.2034537 |
[27] |
Z. Ahmad, G. G. Hamedani, N. S. Butt, Recent developments in distribution theory: a brief survey and some new generalized classes of distributions, Pak. J. Stat. Oper. Res., 15 (2019), 87–110. https://doi.org/10.18187/pjsor.v15i1.2803 doi: 10.18187/pjsor.v15i1.2803
![]() |
[28] | E. T. Lee, J. Wang, Statistical Methods for Survival Data Analysis, John Wiley & Sons, 2003. https://doi.org/10.1002/0471458546 |
[29] |
A. El-Gohary, A. H. El-Bassiouny, M. El-Morshedy, Exponentiated flexible Weibull extension distribution, Int. J. Math. Appl., 3 (2015), 1–12. https://doi.org/10.18576/jsap/050106 doi: 10.18576/jsap/050106
![]() |
[30] |
Y. Fang, L. Zhao, Approximation to the distribution of LAD estimators for censored regression by random weighting method, J. Stat. Plann. Inference, 136 (2006), 1302–1316. https://doi.org/10.1016/j.jspi.2004.09.010 doi: 10.1016/j.jspi.2004.09.010
![]() |
[31] |
Y. M. Kantar, V. Yildirim, Robust estimation for parameters of the extended Burr Type III distribution, Commun. Stat. Simul. Comput., 44 (2015), 1901–1930. https://doi.org/10.1080/03610918.2013.839032 doi: 10.1080/03610918.2013.839032
![]() |
[32] | E. M. Almetwally, H. M. Almogy, Comparison between M-estimation, S-estimation, and MM estimation methods of robust estimation with application and simulation, Int. J. Math. Arch., 9 (2018), 55–63. |
1. | John Kwadey Okutu, Nana K. Frempong, Simon K. Appiah, Atinuke O. Adebanji, Generalization of Odd Ramos-Louzada generated family of distributions: Properties, characterizations, and applications to diabetes and cancer survival datasets, 2024, 10, 24058440, e30690, 10.1016/j.heliyon.2024.e30690 | |
2. | Huda M. Alshanbari, Gadde Srinivasa Rao, Jin-Taek Seong, Saima K. Khosa, A New Sine-Based Distributional Method with Symmetrical and Asymmetrical Natures: Control Chart with Industrial Implication, 2023, 15, 2073-8994, 1892, 10.3390/sym15101892 | |
3. | Huda M. Alshanbari, Omalsad Hamood Odhah, Zubair Ahmad, Faridoon Khan, Abd Al-Aziz Hosni El-Bagoury, A New Probability Distribution: Model, Theory and Analyzing the Recovery Time Data, 2023, 12, 2075-1680, 477, 10.3390/axioms12050477 | |
4. | Divine-Favour N. Ekemezie, Kizito E. Anyiam, Mohammed Kayid, Oluwafemi Samson Balogun, Okechukwu J. Obulezi, DUS Topp–Leone-G Family of Distributions: Baseline Extension, Properties, Estimation, Simulation and Useful Applications, 2024, 26, 1099-4300, 973, 10.3390/e26110973 | |
5. | Beatriz L. Simões e Silva, Cira E. G. Otiniano, Eduardo Y. Nakano, The return period of heterogeneous climate data with a new invertible distribution, 2024, 38, 1436-3240, 2283, 10.1007/s00477-024-02679-2 |
Set 1: θ=1.6,δ=0.5,σ=1.3. | ||||
n | parameters | MLEs | MSEs | Biass |
θ | 2.0264250 | 0.34424293 | 0.42642528 | |
25 | δ | 0.3308959 | 0.04919103 | -0.16910411 |
σ | 3.0607790 | 6.24781140 | 1.96077890 | |
θ | 1.9068750 | 0.18418145 | 0.30687538 | |
50 | δ | 0.3664297 | 0.03407172 | -0.13357025 |
σ | 2.5546780 | 4.57668210 | 1.45467770 | |
θ | 1.8398460 | 0.12796033 | 0.23984625 | |
75 | δ | 0.3861830 | 0.02637042 | -0.11381702 |
σ | 2.2494480 | 3.56365220 | 1.14944810 | |
θ | 1.8022450 | 0.09987249 | 0.20224504 | |
100 | δ | 0.4007103 | 0.02282122 | -0.09928972 |
σ | 2.0751470 | 2.99863540 | 0.97514660 | |
θ | 1.7642540 | 0.07597643 | 0.16425421 | |
150 | δ | 0.4199996 | 0.01762115 | -0.08000036 |
σ | 1.8684900 | 2.37178320 | 0.76848950 | |
θ | 1.7198750 | 0.05149042 | 0.11987515 | |
200 | δ | 0.4394083 | 0.01318933 | -0.06059166 |
σ | 1.6652230 | 1.68975300 | 0.56522340 | |
θ | 1.7109000 | 0.04665635 | 0.11089982 | |
250 | δ | 0.4460993 | 0.01147129 | -0.05390074 |
σ | 1.6108190 | 1.55819453 | 0.51081890 | |
θ | 1.6837020 | 0.03669438 | 0.08370244 | |
300 | δ | 0.4577645 | 0.00964167 | -0.04223553 |
σ | 1.5188100 | 1.34898910 | 0.41881030 | |
θ | 1.7011510 | 0.04038710 | 0.10115076 | |
350 | δ | 0.4484196 | 0.01051719 | -0.05158038 |
σ | 1.5852900 | 1.53231090 | 0.48529030 | |
θ | 1.6654520 | 0.02587484 | 0.06545179 | |
400 | δ | 0.4674469 | 0.00704898 | -0.03255313 |
σ | 1.3942840 | 0.88039980 | 0.29428350 | |
θ | 1.6390040 | 0.02636183 | 0.06900377 | |
450 | δ | 0.4840139 | 0.00717385 | -0.03598612 |
σ | 1.3522010 | 0.88759460 | 0.30220140 | |
θ | 1.6136070 | 0.01555444 | 0.04360732 | |
500 | δ | 0.4975956 | 0.00451764 | -0.02240439 |
σ | 1.2923990 | 0.45577130 | 0.17239860 |
Set 2: θ=1.5,δ=0.8,σ=1.2. | ||||
n | parameters | MLEs | MSEs | Biass |
θ | 2.0216330 | 0.42346514 | 0.52163299 | |
25 | δ | 0.5268598 | 0.10444894 | -0.27314024 |
σ | 3.0711290 | 6.50104860 | 2.05112910 | |
θ | 1.8599560 | 0.22470984 | 0.35995562 | |
50 | δ | 0.5787362 | 0.07833671 | -0.22126382 |
σ | 2.6975860 | 5.59054410 | 1.67758650 | |
θ | 1.7832010 | 0.15911705 | 0.28320139 | |
75 | δ | 0.6200479 | 0.06027562 | -0.17995208 |
σ | 2.3359260 | 4.28183600 | 1.31592640 | |
θ | 1.7617930 | 0.13213407 | 0.26179258 | |
100 | δ | 0.6318675 | 0.05207615 | -0.16813250 |
σ | 2.1996040 | 3.84146220 | 1.17960390 | |
θ | 1.7056680 | 0.08848033 | 0.20566761 | |
150 | δ | 0.6625591 | 0.03944994 | -0.13744086 |
σ | 1.9168200 | 2.92731720 | 0.89682010 | |
θ | 1.6492930 | 0.06256641 | 0.14929256 | |
200 | δ | 0.6994846 | 0.02682993 | -0.10051539 |
σ | 1.6056450 | 1.77894570 | 0.58564520 | |
θ | 1.6322050 | 0.05351188 | 0.13220512 | |
250 | δ | 0.7095510 | 0.02370518 | -0.09044900 |
σ | 1.5534750 | 1.63002940 | 0.53347460 | |
θ | 1.6085810 | 0.03601538 | 0.10858060 | |
300 | δ | 0.7255111 | 0.01709929 | -0.07448886 |
σ | 1.4167820 | 1.16321090 | 0.39678200 | |
θ | 1.5798770 | 0.02485567 | 0.07987703 | |
350 | δ | 0.7456060 | 0.01108549 | -0.05439403 |
σ | 1.2851130 | 0.72728290 | 0.26511340 | |
θ | 1.5515160 | 0.02252164 | 0.08151622 | |
400 | δ | 0.7679470 | 0.01058925 | -0.05720534 |
σ | 1.2542670 | 0.59473740 | 0.23426730 | |
θ | 1.5345300 | 0.01671347 | 0.06453007 | |
450 | δ | 0.7857109 | 0.00806090 | -0.04428908 |
σ | 1.1957780 | 0.41036160 | 0.17877810 | |
θ | 1.5112700 | 0.01539761 | 0.06127049 | |
500 | δ | 0.7976776 | 0.00717589 | -0.04232238 |
σ | 1.1987100 | 0.36360180 | 0.15671040 |
Models | θ | δ | σ | β | α |
NModi-Weibull | 0.75418 | 0.30260 | 1.98970 | - | |
Weibull | 1.05357 | 0.09165 | - | - | |
NEPow-Weibull | 0.91046 | 0.68725 | - | 0.22336 | |
ET-XEE | 1.147472 | 0.12483 | - | - | 6.91687 |
Models | CM | AD | KS | P-value |
NModi-Weibull | 0.05557 | 0.35149 | 0.04976 | 0.90920 |
Weibull | 0.13241 | 0.79257 | 0.07429 | 0.47980 |
NEPow-Weibull | 0.06679 | 0.41012 | 0.05615 | 0.81440 |
ET-XEE | 0.13209 | 0.78746 | 0.07982 | 0.38850 |
Models | θ | δ | σ | β | α |
NModi-Weibull | 2.44343 | 0.00225 | 4.42298 | - | |
Weibull | 2.42921 | 0.00230 | - | - | |
NEPow-Weibull | 1.92969 | 0.12835 | - | 0.06098 | |
ET-XEE | 1.39012 | 0.32552 | - | - | 1.02808 |
Models | CM | AD | KS | P-value |
NModi-Weibull | 0.12637 | 0.82294 | 0.11752 | 0.63850 |
Weibull | 0.14137 | 0.91039 | 0.16746 | 0.21190 |
NEPow-Weibull | 0.21028 | 1.30936 | 0.14832 | 0.34230 |
ET-XEE | 0.10453 | 0.85098 | 0.12098 | 0.58690 |
Set 1: θ=1.6,δ=0.5,σ=1.3. | ||||
n | parameters | MLEs | MSEs | Biass |
θ | 2.0264250 | 0.34424293 | 0.42642528 | |
25 | δ | 0.3308959 | 0.04919103 | -0.16910411 |
σ | 3.0607790 | 6.24781140 | 1.96077890 | |
θ | 1.9068750 | 0.18418145 | 0.30687538 | |
50 | δ | 0.3664297 | 0.03407172 | -0.13357025 |
σ | 2.5546780 | 4.57668210 | 1.45467770 | |
θ | 1.8398460 | 0.12796033 | 0.23984625 | |
75 | δ | 0.3861830 | 0.02637042 | -0.11381702 |
σ | 2.2494480 | 3.56365220 | 1.14944810 | |
θ | 1.8022450 | 0.09987249 | 0.20224504 | |
100 | δ | 0.4007103 | 0.02282122 | -0.09928972 |
σ | 2.0751470 | 2.99863540 | 0.97514660 | |
θ | 1.7642540 | 0.07597643 | 0.16425421 | |
150 | δ | 0.4199996 | 0.01762115 | -0.08000036 |
σ | 1.8684900 | 2.37178320 | 0.76848950 | |
θ | 1.7198750 | 0.05149042 | 0.11987515 | |
200 | δ | 0.4394083 | 0.01318933 | -0.06059166 |
σ | 1.6652230 | 1.68975300 | 0.56522340 | |
θ | 1.7109000 | 0.04665635 | 0.11089982 | |
250 | δ | 0.4460993 | 0.01147129 | -0.05390074 |
σ | 1.6108190 | 1.55819453 | 0.51081890 | |
θ | 1.6837020 | 0.03669438 | 0.08370244 | |
300 | δ | 0.4577645 | 0.00964167 | -0.04223553 |
σ | 1.5188100 | 1.34898910 | 0.41881030 | |
θ | 1.7011510 | 0.04038710 | 0.10115076 | |
350 | δ | 0.4484196 | 0.01051719 | -0.05158038 |
σ | 1.5852900 | 1.53231090 | 0.48529030 | |
θ | 1.6654520 | 0.02587484 | 0.06545179 | |
400 | δ | 0.4674469 | 0.00704898 | -0.03255313 |
σ | 1.3942840 | 0.88039980 | 0.29428350 | |
θ | 1.6390040 | 0.02636183 | 0.06900377 | |
450 | δ | 0.4840139 | 0.00717385 | -0.03598612 |
σ | 1.3522010 | 0.88759460 | 0.30220140 | |
θ | 1.6136070 | 0.01555444 | 0.04360732 | |
500 | δ | 0.4975956 | 0.00451764 | -0.02240439 |
σ | 1.2923990 | 0.45577130 | 0.17239860 |
Set 2: θ=1.5,δ=0.8,σ=1.2. | ||||
n | parameters | MLEs | MSEs | Biass |
θ | 2.0216330 | 0.42346514 | 0.52163299 | |
25 | δ | 0.5268598 | 0.10444894 | -0.27314024 |
σ | 3.0711290 | 6.50104860 | 2.05112910 | |
θ | 1.8599560 | 0.22470984 | 0.35995562 | |
50 | δ | 0.5787362 | 0.07833671 | -0.22126382 |
σ | 2.6975860 | 5.59054410 | 1.67758650 | |
θ | 1.7832010 | 0.15911705 | 0.28320139 | |
75 | δ | 0.6200479 | 0.06027562 | -0.17995208 |
σ | 2.3359260 | 4.28183600 | 1.31592640 | |
θ | 1.7617930 | 0.13213407 | 0.26179258 | |
100 | δ | 0.6318675 | 0.05207615 | -0.16813250 |
σ | 2.1996040 | 3.84146220 | 1.17960390 | |
θ | 1.7056680 | 0.08848033 | 0.20566761 | |
150 | δ | 0.6625591 | 0.03944994 | -0.13744086 |
σ | 1.9168200 | 2.92731720 | 0.89682010 | |
θ | 1.6492930 | 0.06256641 | 0.14929256 | |
200 | δ | 0.6994846 | 0.02682993 | -0.10051539 |
σ | 1.6056450 | 1.77894570 | 0.58564520 | |
θ | 1.6322050 | 0.05351188 | 0.13220512 | |
250 | δ | 0.7095510 | 0.02370518 | -0.09044900 |
σ | 1.5534750 | 1.63002940 | 0.53347460 | |
θ | 1.6085810 | 0.03601538 | 0.10858060 | |
300 | δ | 0.7255111 | 0.01709929 | -0.07448886 |
σ | 1.4167820 | 1.16321090 | 0.39678200 | |
θ | 1.5798770 | 0.02485567 | 0.07987703 | |
350 | δ | 0.7456060 | 0.01108549 | -0.05439403 |
σ | 1.2851130 | 0.72728290 | 0.26511340 | |
θ | 1.5515160 | 0.02252164 | 0.08151622 | |
400 | δ | 0.7679470 | 0.01058925 | -0.05720534 |
σ | 1.2542670 | 0.59473740 | 0.23426730 | |
θ | 1.5345300 | 0.01671347 | 0.06453007 | |
450 | δ | 0.7857109 | 0.00806090 | -0.04428908 |
σ | 1.1957780 | 0.41036160 | 0.17877810 | |
θ | 1.5112700 | 0.01539761 | 0.06127049 | |
500 | δ | 0.7976776 | 0.00717589 | -0.04232238 |
σ | 1.1987100 | 0.36360180 | 0.15671040 |
Models | θ | δ | σ | β | α |
NModi-Weibull | 0.75418 | 0.30260 | 1.98970 | - | |
Weibull | 1.05357 | 0.09165 | - | - | |
NEPow-Weibull | 0.91046 | 0.68725 | - | 0.22336 | |
ET-XEE | 1.147472 | 0.12483 | - | - | 6.91687 |
Models | CM | AD | KS | P-value |
NModi-Weibull | 0.05557 | 0.35149 | 0.04976 | 0.90920 |
Weibull | 0.13241 | 0.79257 | 0.07429 | 0.47980 |
NEPow-Weibull | 0.06679 | 0.41012 | 0.05615 | 0.81440 |
ET-XEE | 0.13209 | 0.78746 | 0.07982 | 0.38850 |
Models | θ | δ | σ | β | α |
NModi-Weibull | 2.44343 | 0.00225 | 4.42298 | - | |
Weibull | 2.42921 | 0.00230 | - | - | |
NEPow-Weibull | 1.92969 | 0.12835 | - | 0.06098 | |
ET-XEE | 1.39012 | 0.32552 | - | - | 1.02808 |
Models | CM | AD | KS | P-value |
NModi-Weibull | 0.12637 | 0.82294 | 0.11752 | 0.63850 |
Weibull | 0.14137 | 0.91039 | 0.16746 | 0.21190 |
NEPow-Weibull | 0.21028 | 1.30936 | 0.14832 | 0.34230 |
ET-XEE | 0.10453 | 0.85098 | 0.12098 | 0.58690 |