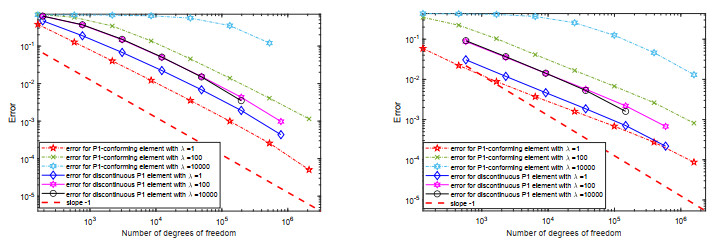
In order to improve the availability of fault data, the fault data of heat meters had been classified, and balances all kinds of fault data according to interpolation algorithms to meet the needs of fault diagnosis algorithms. Based on the voting mechanism, an integrated model of multi classifier fusion is established, and the weight of each classifier is optimally configured through pigeon swarm algorithm. In the experiment, three kinds of integration models are obtained according to the voting mechanism and pigeon swarm algorithm. The three integrated models are used to diagnose the fault of the heat meter, and the three indicators of precision, recall and F1 Core have achieved satisfactory results.
Citation: Shuchun Yu, Jinjian Tao, Jun Liu, Yanshu Miao. Research on fault diagnosis technology of heat meter based on multi classifier fusion of pigeon swarm algorithm[J]. Mathematical Biosciences and Engineering, 2023, 20(4): 6312-6326. doi: 10.3934/mbe.2023272
[1] | Justin W. L. Wan . Multigrid method for pricing European options under the CGMY process. AIMS Mathematics, 2019, 4(6): 1745-1767. doi: 10.3934/math.2019.6.1745 |
[2] | Shixian Ren, Yu Zhang, Ziqiang Wang . An efficient spectral-Galerkin method for a new Steklov eigenvalue problem in inverse scattering. AIMS Mathematics, 2022, 7(5): 7528-7551. doi: 10.3934/math.2022423 |
[3] | Song Lunji . A High-Order Symmetric Interior Penalty Discontinuous Galerkin Scheme to Simulate Vortex Dominated Incompressible Fluid Flow. AIMS Mathematics, 2016, 1(1): 43-63. doi: 10.3934/Math.2016.1.43 |
[4] | Yones Esmaeelzade Aghdam, Hamid Mesgarani, Zeinab Asadi, Van Thinh Nguyen . Investigation and analysis of the numerical approach to solve the multi-term time-fractional advection-diffusion model. AIMS Mathematics, 2023, 8(12): 29474-29489. doi: 10.3934/math.20231509 |
[5] | Shuangbing Guo, Xiliang Lu, Zhiyue Zhang . Finite element method for an eigenvalue optimization problem of the Schrödinger operator. AIMS Mathematics, 2022, 7(4): 5049-5071. doi: 10.3934/math.2022281 |
[6] | Lingling Sun, Hai Bi, Yidu Yang . A posteriori error estimates of mixed discontinuous Galerkin method for a class of Stokes eigenvalue problems. AIMS Mathematics, 2023, 8(9): 21270-21297. doi: 10.3934/math.20231084 |
[7] | Cagnur Corekli . The SIPG method of Dirichlet boundary optimal control problems with weakly imposed boundary conditions. AIMS Mathematics, 2022, 7(4): 6711-6742. doi: 10.3934/math.2022375 |
[8] | Yanhua Gu . High-order numerical method for the fractional Korteweg-de Vries equation using the discontinuous Galerkin method. AIMS Mathematics, 2025, 10(1): 1367-1383. doi: 10.3934/math.2025063 |
[9] | Zuliang Lu, Xiankui Wu, Fei Huang, Fei Cai, Chunjuan Hou, Yin Yang . Convergence and quasi-optimality based on an adaptive finite element method for the bilinear optimal control problem. AIMS Mathematics, 2021, 6(9): 9510-9535. doi: 10.3934/math.2021553 |
[10] | Lanyin Sun, Kunkun Pang . Numerical solution of unsteady elastic equations with C-Bézier basis functions. AIMS Mathematics, 2024, 9(1): 702-722. doi: 10.3934/math.2024036 |
In order to improve the availability of fault data, the fault data of heat meters had been classified, and balances all kinds of fault data according to interpolation algorithms to meet the needs of fault diagnosis algorithms. Based on the voting mechanism, an integrated model of multi classifier fusion is established, and the weight of each classifier is optimally configured through pigeon swarm algorithm. In the experiment, three kinds of integration models are obtained according to the voting mechanism and pigeon swarm algorithm. The three integrated models are used to diagnose the fault of the heat meter, and the three indicators of precision, recall and F1 Core have achieved satisfactory results.
The Steklov spectrum coincides with that of the Dirichlet-to-Neumann map for the Laplacian (see, e.g., [1]), and the Steklov eigenvalue problem for the Laplace operator has been well-studied in the mathematical community. In linear elasticity, the study of the Dirichlet-to-Neumann map is important in elastostatic problems, and has attracted the attention of scholars (see, e.g., [2,3,4]). In 2021, Domˊinguez [5] first introduced the Steklov-Lamé eigenvalue problem in which the spectral parameter appears on a Robin boundary condition. [5] investigated the existence of the countable spectrum of this problem and studied the conforming finite element methods for the Steklov Lamé problem. Later, Li and Bi [6] proposed a discontinuous finite element method for this problem and gave the a priori error estimates.
As we know, for numerical solutions of the problems in linear planar elasticity, standard conforming finite elements may suffer a deterioration in performance as the Lamˊe constant λ→∞, that is locking phenomenon (see [7,8]). To overcome the locking phenomenon, several numerical approaches have been developed including the p-version method [9], the PEERS method [10], the mixed method [11], the Galerkin least squares method [12], the nonconforming triangular elements [13,14] and the discontinuous finite element method [15,16,17], and so on.
On the other hand, based on standard finite element methods, people design many efficient discretization schemes/algorithms to get approximations with high accuracy or to reduce the computation costs. The finite element multigrid discretizations is one of such design approaches. This method benefits from the two-grid discretization scheme which was first proposed by Xu and Zhou [18,19]. The basic idea of the two-grid discretizations is to transform solving an eigenvalue problem on a fine grid into solving the eigenvalue problem on a coarse grid and solving a series of algebraic equations on the fine grid. This kind of method can save calculation time while keeping the accuracy of approximations, or improving the accuracy under the same degrees of freedom. So far, two-grid and multigrid finite element discretization schemes have been successfully applied to solving eigenvalue problems, such as elliptic eigenvalue problem [20], Steklov eigenvalue problem [21,22,23,24], biharmonic eigenvalue problem [25], semilinear elliptic eigenvalue problem [26], quantum eigenvalue problem [27], Stokes eigenvalue problem [28,29], Maxwell eigenvalue problem [30], 2m-order elliptic eigenvalue problem [31], etc.
At present, there is not much numerical research report on the Steklov-Lamé eigenproblem. In view of the characteristics of discontinuous finite element method (DGFEM) and multigrid discretizations and based on the work in [6,32], for the Steklov-Lamé eigenvalue problem we will design and analyze a multigrid discretization scheme of DGFEM based on the shifted-inverse iteration. The rest of this paper is organized as follows. In Section 2, the discontinuous finite element approximation of the Steklov-Lamé eigenvalue problem and its a prior error estimates are given. In Section 3, a multigrid discretization scheme of DGFEM based on the shifted-inverse iteration is established, and the error estimates of the proposed scheme is presented. Finally, in Section 4, an adaptive multigrid algorithm is provided coupled with some numerical experiment results. The numerical results show that our method is efficient and locking-free.
Before the discussion, let us specify some notations. Scalars are denoted by general letters, vectors are denoted by bold letters and tensors in bold Greek letters. For tensors σ,τ∈Rn×n, the double dot product notation σ:τ=tr(τTσ) where tr(⋅) denotes the trace of a tensor (sum of the main diagonal). This inner product induces the Frobenius norm for tensors which is denoted as ‖⋅‖. Let Hs(Ω) and Hs(∂Ω) be the usual Sobolev space with order s of scalar fields on Ω and ∂Ω, respectively, whereas for tensor fields we use the symbols Hs(Ω) and Hs(∂Ω) and each element in Hs belongs to Hs. The norm in Hs(Ω) and Hs(∂Ω) are denoted by ‖⋅‖s and ‖⋅‖s,∂Ω, respectively, and the same symbols are also used for the norms in Hs(Ω) and Hs(∂Ω) when there is no ambiguity. H0(∂Ω)=L2(∂Ω). Throughout this paper, we use the letter C, with or without subscript, to denote a generic positive constant independent of the mesh size h and the Lamé parameters, which may not be the same at each occurrence. We use the symbol a≲b to mean that a≤Cb.
Suppose that an isotropic and linearly elastic material occupies the region Ω in Rn (n=2or3) where Ω is a bounded convex polygonal with Lipschitz continuous boundary ∂Ω. Consider the following Steklov-Lamé eigenvalue problem: Find non-zero displacement vector u and the frequencies ω∈R satisfying
{−divσ(u)=0in Ω,σ(u)n=ωpuon ∂Ω, | (2.1) |
where n is the unit outward normal to ∂Ω, σ(u) is the Cauchy stress tensor defined as
σ(u)=2μϵ(u)+λtr(ϵ(u))I, |
where I∈Rn×n is the identity matrix, ϵ(u) is the strain tensor given by
ϵ(u)=12(∇u+(∇u)T), |
∇u is the displacement gradient tensor, and λ∈R and μ>0 are the Lamé parameters satisfying 0<μ1<μ<μ2 and 0<λ<∞.
Suppose that the density of material p∈L∞(∂Ω) has positive lower bound on ∂Ω.
Denote
RM(Ω):={v∈H1(Ω)|v(x)=a+Bx,a∈Rn,B∈Rn×n,BT=−B,x∈Ω}. |
It is obvious that 0 is an eigenvalue of (2.1) with the associated eigenfunction u∈RM(Ω) (see [5]). To find non-zero eigenvalues of (2.1), we adopt the following weak formulation: Seek (κ,u)∈R×H1(Ω) such that
a(u,v)=κb(u,v), ∀v∈H1(Ω), | (2.2) |
where κ=ω+1,
a(u,v):=∫Ωσ(u):ϵ(v)dx+∫∂Ωpu⋅vds=2μ∫Ωϵ(u):ϵ(v)dx+λ∫Ω(divu)(divv)dx+∫∂Ωpu⋅vds, ∀u,v∈H1(Ω),b(u,v):=∫∂Ωpu⋅vds, ∀u,v∈H1(Ω). |
Reference[5] proved that a(⋅,⋅) is a continuous and H1-coercive bilinear form in H1(Ω), b(⋅,⋅) is bounded.
Without losing generality, we assume that p≡1 in the rest of this paper. Denote ‖v‖b=b(v,v)12, then it is clear that ‖⋅‖b=‖⋅‖0,∂Ω.
Let Th={K} be a shape-regular partition of Ω, and h=max{hK:K∈Th} is the diameter of Th where hK is the diameter of element K. When n=2, K is a triangle and a tetrahedron when n=3. Let e∈∂K be an edge/face of element K with diameter he, and let Γh=Γih∪Γbh where Γih denotes the interior edges/faces set and Γbh denotes the set of edges/faces lying on the boundary ∂Ω. In the following, when there is no confusion we always use n to represent the unit outward normal on the boundary of Ω or element K.
Define the broken Sobolev space:
Hs(Th)={v∈[L2(Ω)]n:v∣K∈[Hs(K)]n,∀K∈Th}. |
For any v∈Hs(Th), define the jump [[v]] and the average {v} on e as follows:
[[v]]={v+−v−,e∈Γih,v+ ,e∈Γbh,{v}={v++v−2,e∈Γih,v+ , e∈Γbh, |
where v+=v|K+,v−=v|K−, e∈∂K+∩∂K−.
Define the DGFEM space:
Sh={v∈[L2(Ω)]n:v∣K∈[Pk(K)]n,∀K∈Th}, |
where Pk(K) is the space of polynomials defined on K with degree less than or equal to k≥1.
The DGFEM discretization for the problem (2.2) is to find (κh,uh)∈R×Sh,uh≠0,κh=ωh+1, such that
ah(uh,vh)=κhbh(uh,vh), ∀vh∈Sh, | (2.3) |
where
ah(uh,vh)=2μ(∑K∈Th∫Kϵ(uh):ϵ(vh)dx−∑e∈Γih∫e{ϵ(uh)n}⋅[[vh]]ds−∑e∈Γih∫e{ϵ(vh)n}⋅[[uh]]ds+∑e∈Γihγμhe∫e[[uh]]⋅[[vh]]ds)+λ(∑K∈Th∫K(divuh)(divvh)dx−∑e∈Γih∫e{divuh}[[vh⋅n]]ds−∑e∈Γih∫e{divvh}[[uh⋅n]]ds+∑e∈Γihγλhe∫e[[uh⋅n]][[vh⋅n]]ds)+∑e∈Γbh∫euh⋅vhds,bh(uh,vh)=∑e∈Γbh∫euh⋅vhds, |
and the penalty constants γμ,γλ are independent of the shape of K and h. The determination of γμ and γλ is to ensure that (2.4) is valid. It is easy to see that the discretization (2.3) is symmetric which is called symmetric internal penalty method (SIPG) in DGFEM.
Define the DG norm:
‖uh‖2G=2μ∑K∈Th‖ϵ(uh)‖20,K+2μ∑e∈Γihγμh−1e‖[[uh]]‖20,e+λ∑K∈Th‖divuh‖20,K+λ∑e∈Γihγλh−1e‖[[uh⋅n]]‖20,e+∑e∈Γbh‖uh‖20,e, |
and the energy-like norm:
‖uh‖2h=‖uh‖2G+2μ∑e∈Γihhe‖{ϵ(uh)n}‖20,e+λ∑e∈Γihhe‖{divuh}‖20,e. |
From Lemma 4 in [33] we know that there exist constants Cμ and Cλ, independent of h,he,μ and λ, such that
‖h1/2eϵ(v)n‖20,e≤Cμ‖ϵ(v)‖20,K,‖h1/2edivv‖20,e≤Cλ‖divv‖20,K. |
Then, for 0<β<1, when γμ≥Cμ/(1−β)2, γλ≥Cλ/(1−β)2, the bilinear form ah(⋅,⋅) is coercive on Sh (see Lemma 2.2 in [6]):
β‖vh‖2G≤ah(vh,vh),∀vh∈Sh. | (2.4) |
Using Cauchy-Schwartz inequality, it is easy to prove that the bilinear form ah(⋅,⋅) is continuous:
|ah(u,v)|≤M‖u‖h‖v‖h,∀u,v∈H1+s(Th),s>12. |
In order to derive the convergence and the error estimates of DG approximations by using Babu˘ska-Osborn spectral approximation theory, we consider the following source problem associated with the eigenvalue problem (2.2): find w∈H1(Ω) such that
a(w,v)=b(f,v), ∀v∈H1(Ω). | (2.5) |
The DG approximation of (2.5) is to find wh∈Sh such that
ah(wh,vh)=bh(f,vh), ∀vh∈Sh. | (2.6) |
Since a(⋅,⋅) and ah(⋅,⋅) are continuous and coercive on H1(Ω) and Sh, respectively, b(⋅,⋅) and bh(⋅,⋅) are bounded, from Lax-Milgram Theorem we know that (2.5) and (2.6) admit the unique solution w and wh, respectively.
The following regularity estimates of the solution of (2.5) has been discussed in Lemma 3.1 of [6].
(1) Let w be the solution of (2.5). If f∈Hr−12(∂Ω), then w∈Hr+1(Ω) and
‖w‖r+1+λ‖divw‖r≤CR‖f‖r−12,∂Ω, |
where r=1 when Ω is a convex polygonal, and r can be large enough when ∂Ω is sufficiently smooth;
(2) If f∈H−12(∂Ω), then w∈H1(Ω) and
‖w‖1+λ‖divw‖0≤CR‖f‖−12,∂Ω; |
(3) If f∈L2(∂Ω), then w∈H1+12(Ω) and
‖w‖1+12+λ‖divw‖12≤CR‖f‖0,∂Ω, | (2.7) |
where the constant CR is independent of μ and λ.
For any given f∈L2(∂Ω), from (2.7) we have w∈H1+r(Ω),r<12 and r can be arbitrarily close to 12, and
‖w‖1+r+λ‖divw‖r≤CR‖f‖0,∂Ω. | (2.8) |
Let w and wh be the solution of (2.5) and (2.6), respectively, then the SIPG approximation (2.6) of (2.5) is consistent (see Lemma 3.3 in [6]):
ah(w−wh,vh)=0, ∀vh∈Sh. | (2.9) |
For the source problem (2.5), let f∈L2(∂Ω), define the solution operator A: L2(∂Ω)→H1(Ω) by
a(Af,v)=b(f,v), ∀v∈H1(Ω), |
and define the operator T: L2(∂Ω)→L2(∂Ω):
Tf=(Af)′, |
where ′ denotes the restriction on ∂Ω. Then, (2.2) has the following equivalent operator form:
Au=1κu. |
Similarly, from (2.6) we can define the discrete solution operator Ah: L2(∂Ω)→Sh by
ah(Ahf,v)=bh(f,v), ∀v∈Sh, |
and the operator Th:L2(∂Ω)→δSh⊂L2(∂Ω) satisfying
Thf=(Ahf)′, |
where δSh is the restriction of Sh on ∂Ω. Then (2.3) has the following equivalent operator form:
Ahuh=1κhuh. |
Denote ρ=1κ,ρh=1κh. In this paper, κ, κh and ρ, ρh are all called eigenvalues.
From the definition of Ah and (2.4), noticing that ‖⋅‖h and ‖⋅‖G are equivalent on Sh, we can deduce that
‖Ahf‖2h≲ah(Ahf,Ahf)=bh(f,Ahf)≲‖f‖0,∂Ω‖Ahf‖0,∂Ω≲‖f‖0,∂Ω‖Ahf‖h, |
thus,
‖Ahf‖h⩽C‖f‖0,∂Ω≤C‖f‖h. | (2.10) |
Reference [6] gave the a priori error estimates of DG approximation of (2.5).
Theorem 2.1. For any given f∈L2(∂Ω), let w∈H1+r(Ω)(0<r<12) be the solution of (2.5), and let wh be the solution of (2.6). Assume that the regularity estimate (2.8) is valid, then there hold
‖w−wh‖G≲hr‖f‖0,∂Ω,‖w−wh‖0,∂Ω≲h2r‖f‖0,∂Ω; |
Further, when w∈H1+s(Ω)(12<s≤k), there hold
‖w−wh‖h≲hr(√2μ‖w‖1+r+√λ‖divw‖r),‖w−wh‖0,∂Ω≲hr+s(√2μ‖w‖1+s+√λ‖divw‖s). |
Proof. See Theorems 3.6–3.8 in [6].
Suppose that κ is the jth eigenvalue of (2.2) with algebraic multiplicity q, i.e., κ=κj=κj+1=⋅⋅⋅=κj+q−1. [5] proved that ‖T−Th‖0,∂Ω→0 when h→0, therefore, q eigenvalues κj,h,κj+1,h,⋯,κj+q−1,h of (2.3) will converge to κ. Let M(κ) be the space of eigenfunctions of (2.2) associated with eigenvalue κ, and Mh(κ) be the direct sum of the generalized eigenspace of (2.3) associated with κh that converge to κ, M(ρ)=M(κ) and Mh(ρ)=Mh(κ). From [34] we have the following error estimates.
Theorem 2.2. Assume that the regularity estimate (2.8) is valid, and let M(κ)⊂H1+s(Ω)(12<s),t=min{k,s}, then there holds
|κ−κh|≲h2t; | (2.11) |
Let uh∈Mh(κ) be an eigenfunction of (2.3), then there exists u∈M(κ) such that
‖u−uh‖0,∂Ω≲hr+t, | (2.12) |
‖u−uh‖h≲ht; | (2.13) |
Let u∈M(κ) be an eigenfunction of (2.2), then there exists uh∈Mh(κ) such that
‖u−uh‖h≲ht. | (2.14) |
Proof. See Theorem 3.10 in [6] for the proofs of (2.11)–(2.13). By similar arguments we can get (2.14).
Let {Thi}l0 be a family of regular meshes of Ω, hi−1≫hi, and let Shi be the DG space defined on Thi. Denote TH=Th0,SH=Sh0. Now, for the eigenvalue problem (2.3) we give the following multigrid discretization scheme of DGFEM based on the shifted inverse iteration.
Scheme 3.1. Given the iteration times l .
Step 1: Solve (2.3) on SH: Find (κH,uH)∈R×SH, such that ‖uH‖0,∂Ω=1 and
aH(uH,v)=κHbH(uH,v), ∀v∈SH. |
Step 2: uh0⇐uH,κh0⇐κH, i⇐1.
Step 3: Solve a linear system on Shi: Find u′∈Shi such that
ahi(u′,v)−κhi−1bhi(u′,v)=bhi(uhi−1,v), ∀v∈Shi. |
Set uhi=u′‖u′‖0,∂Ω.
Step 4: Compute the Rayleigh quotient
κhi=ahi(uhi,uhi)bhi(uhi,uhi). |
Step 5: If i=l, then output (κhl,uhl), stop; else, i⇐i+1 and return to Step 3.
Next we will conduct the error analysis on Scheme 3.1.
From (2.9) we define the projection operator Ph:H1(Ω)+Sh→Sh⊂L2(∂Ω) satifying
ah(u−Phu,vh)=0,∀vh∈Sh. | (3.1) |
Then, from (2.9) and (3.1) together with Af=w,Ahf=wh, we can prove easily that Ah=PhA.
We first give the following lemmas to prepare for the error analysis.
Lemma 3.1. Let (κ,u) be an eigenpair of (2.2), then for any v∈Sh and ‖v‖b≠0, the Rayleigh quotient R(v)=ah(v,v)‖v‖2b satisfies
R(v)−κ=ah(v−u,v−u)‖v‖2b−κ‖v−u‖2b‖v‖2b. | (3.2) |
Proof. From (2.9) we have
ah(u,v)=b(κu,v)=bh(κu,v),∀v∈Sh, |
thus,
ah(v−u,v−u)−κb(v−u,v−u)=ah(v,v)−2ah(u,v)+ah(u,u)−κb(v,v)+2κb(u,v)−κb(u,u)=ah(v,v)−2b(κu,v)+a(u,u)−κb(v,v)+2κb(u,v)−κb(u,u)=ah(v,v)−κb(v,v), |
dividing both sides by ‖v‖2b we obtain (3.2).
Lemma 3.2. For any non-zero elements u,v in any normed linear space (V,‖⋅‖), it is valid that
‖u‖u‖−v‖v‖‖≤2‖u−v‖‖u‖ , ‖u‖u‖−v‖v‖‖≤2‖u−v‖‖v‖ . |
Proof. See Lemma 3.1 in [20].
Denote d=dimSh, dist(u,Sh)=infv∈Sh‖u−v‖h. Referring to Lemma 4.1 in [20] we prove the following result which plays an important role in our analysis.
Lemma 3.3. Let (ρ0,w0) be an approximation of the jth eigenpair (ρ,u) of (2.2) where ρ0 is not an eigenvalue of Ah, w0∈Sh,‖w0‖0,∂Ω=1. And let u0=Ahw0‖Ahw0‖0,∂Ω. Suppose that
(C1)infv∈Mh(ρ)‖w0−v‖0,∂Ω≤12;
(C2)|ρ0−ρ|≤ϑ4,|ρm,h−ρm|≤ϑ4,m=j−1,j,j+q(m≠0), where ϑ=minm≠j|ρm−ρ| is the separate constant of the eigenvalue ρ;
(C3)u′∈Sh and uh∈Sh satisfy
(ρ0−Ah)u′=u0,uh=u′‖u′‖0,∂Ω. | (3.3) |
Then
dist(uh,Mh(ρ))≤Cϑmaxj≤m≤j+q−1|ρ0−ρm,h|dist(w0,Mh(ρ)). |
Proof. Let {um,h}dm=1 be eigenfunctions of Ah satisfying b(um,h,ui,h)=δm,i. Then
u0=d∑m=1b(u0,um,h)um,h. |
Since ρ0 is not an eigenvalue of Ah, from (3.3) we can get
(ρ0−ρj,h)u′=(ρ0−ρj,h)(ρ0−Ah)−1u0=d∑m=1ρ0−ρj,hρ0−ρm,hb(u0,um,h)um,h. | (3.4) |
Using triangle inequality and the condition (C2) we derive
|ρ0−ρj,h|≤|ρ0−ρ|+|ρ−ρj,h|≤ϑ4+ϑ4=ϑ2,|ρ0−ρm,h|≥|ρ−ρm|−|ρ0−ρ|−|ρm−ρm,h|≥ϑ−ϑ4−ϑ4=ϑ2, |
where m=j−1,j+q(m≠0). Hence, we have
|ρ0−ρm,h|≥ϑ2m≠j,j+1,⋯,j+q−1. | (3.5) |
Because the operator Th is selfadjoint with respect to b(⋅,⋅), in fact, for ∀f∈L2(∂Ω), from the symmetry of ah(⋅,⋅) and b(⋅,⋅) and b(⋅,⋅)=bh(⋅,⋅) we have b(Thf,vh)=b(vh,Thf)=bh(vh,Thf)=ah(Thvh,Thf)= ah(Thf,Thvh)=bh(f,Thvh)=b(f,Thvh) and Ahuh=ρhuh, then, for m=1,2,⋯,d, there holds
b(Thw0,um,h)um,h=b(w0,Thum,h)um,h=b(w0,ρm,hum,h)um,h=b(w0,um,h)ρm,hum,h=b(w0,um,h)Ahum,h. | (3.6) |
Noticing that {um,h}j+q−1m=j is a standard orthogonal basis of Mh(ρ) with respect to the L2(∂Ω) inner product b(⋅,⋅), from u0=Ahw0‖Ahw0‖0,∂Ω, (3.4), (3.6), (2.10) and (3.5) we deduce
‖(ρ0−ρj,h)u′−j+q−1∑m=jρ0−ρj,hρ0−ρm,hb(u0,um,h)um,h‖h=‖∑m≠j,j+1,⋯,j+q−1ρ0−ρj,hρ0−ρm,hb(u0,um,h)um,h‖h=1‖Ahw0‖0,∂Ω‖∑m≠j,j+1,⋯,j+q−1ρ0−ρj,hρ0−ρm,hb(Ahw0,um,h)um,h‖h=1‖Ahw0‖0,∂Ω‖∑m≠j,j+1,⋯,j+q−1ρ0−ρj,hρ0−ρm,hb(Thw0,um,h)um,h‖h=1‖Ahw0‖0,∂Ω‖∑m≠j,j+1,⋯,j+q−1ρ0−ρj,hρ0−ρm,hb(w0,um,h)Ahum,h‖h=1‖Ahw0‖0,∂Ω‖Ah(∑m≠j,j+1,⋯,j+q−1ρ0−ρj,hρ0−ρm,hb(w0,um,h)um,h)‖h≤C‖Ahw0‖0,∂Ω‖∑m≠j,j+1,⋯,j+q−1ρ0−ρj,hρ0−ρm,hb(w0,um,h)um,h‖0,∂Ω≤2Cϑ‖Ahw0‖0,∂Ω|ρ0−ρj,h|(∑m≠j,j+1,⋯,j+q−1b2(w0,um,h))12≤2Cϑ‖Ahw0‖0,∂Ω|ρ0−ρj,h|‖w0−j+q−1∑m=jb(w0,um,h)um,h‖0,∂Ω=2Cϑ‖Ahw0‖0,∂Ω|ρ0−ρj,h|infv∈Mh(ρ)‖w0−v‖0,∂Ω≤2Cϑ‖Ahw0‖0,∂Ω|ρ0−ρj,h|dist(w0,Mh(ρ)). | (3.7) |
Taking the norm on both sides of (3.4), and noting that u0=Ahw0‖Ahw0‖0,∂Ω, the condition (C1) and (3.6), we get
‖(ρ0−ρj,h)u′‖0,∂Ω=1‖Ahw0‖0,∂Ω‖d∑m=1ρ0−ρj,hρ0−ρm,hb(Ahw0,um,h)um,h‖0,∂Ω=1‖Ahw0‖0,∂Ω‖d∑m=1ρ0−ρj,hρ0−ρm,hb(Thw0,um,h)um,h‖0,∂Ω=1‖Ahw0‖0,∂Ω(d∑m=1(ρ0−ρj,hρ0−ρm,hb2(w0,ρm,hum,h)))12≥C‖Ahw0‖0,∂Ωminj≤m≤j+q−1|ρ0−ρj,hρ0−ρm,h|(j+q−1∑m=jb2(w0,um,h))12=C‖Ahw0‖0,∂Ωminj≤m≤j+q−1|ρ0−ρj,hρ0−ρm,h|‖w0−(w0−j+q−1∑m=jb(w0,um,h)um,h)‖0,∂Ω≥C2‖Ahw0‖0,∂Ωminj≤m≤j+q−1|ρ0−ρj,hρ0−ρm,h|. | (3.8) |
From (3.7) and (3.8) we derive
dist(uh,Mh(ρ))=dist(sign(ρ0−ρj,h)uh,Mh(ρ))≤‖sign(ρ0−ρj,h)uh−1‖(ρ0−ρj,h)u′‖0,∂Ωj+q−1∑m=jρ0−ρj,hρ0−ρm,hb(u0,um,h)um,h‖h=‖(ρ0−ρj,h)u′‖(ρ0−ρj,h)u′‖0,∂Ω−1‖(ρ0−ρj,h)u′‖0,∂Ωj+q−1∑m=jρ0−ρj,hρ0−ρm,hb(u0,um,h)um,h‖h≤2C‖Ahw0‖0,∂Ωmaxj≤m≤j+q−1|ρ0−ρm,hρ0−ρj,h|‖(ρ0−ρj,h)u′−j+q−1∑m=jρ0−ρj,hρ0−ρm,hb(u0,um,h)um,h‖h≤Cϑmaxj≤m≤j+q−1|ρ0−ρm,h|dist(w0,Mh(ρ)). |
The proof is completed.
Now we can analyze the error of multigrid discretization scheme 3.1 by using Theorem 2.2 and Lemma 3.3. We first consider the case of l=1. Denote H=h0,h=h1.
Theorem 3.1. Suppose that M(κj)⊂H1+s(Ω)(s≥r), and t=min{k,s}. Let (κhj,uhj) be an approximate eigenpair obtained by Scheme 3.1 (l=1) and H is sufficiently small, then there exists uj∈M(κj) such that
‖uhj−uj‖h≤C(H3t+ht), | (3.9) |
‖uhj−uj‖0,∂Ω≤C(H3t+hr+t), | (3.10) |
|κhj−κj|≤C(H3t+ht)2. | (3.11) |
Proof. We will use Lemma 3.3 to complete the proof. Take ρ0=1κH,w0=uH and u0=AhuH‖AhuH‖0,∂Ω. From (2.13) we know that there exists ˉu∈M(κj) such that
‖uH−ˉu‖h≤CHt. |
From the triangle inequality and (2.14) we have
dist(uH,Mh(κj))≤‖uH−ˉu‖H+dist(ˉu,Mh(κj))≤C(Ht+ht)≤CHt, | (3.12) |
thus,
infv∈Mh(κj)‖uH−v‖0,∂Ω≤CHt, |
when H is small enough, the condition (C1) in Lemma 3.3 is valid.
From (2.11) we get
|ρ0−ρj|=|κH−κj||κHκj|≤CH2t≤ϑ4;|ρm−ρm,h|=|κm,h−κm||κm,hκm|≤Ch2t≤ϑ4,m=j−1,j,…,j+q,m≠0, |
i.e., the condition (C2) in Lemma 3.3 holds.
From the definition of Ah we know that Step 3 in Scheme 3.1 is equivalent to the following:
ah(u′,v)−κHah(Ahu′,v)=ah(AhuH,v)∀v∈Sh, |
and uhj=u′‖u′‖0,∂Ω, i.e.,
(κ−1H−Ah)u′=κ−1HAhuH,uhj=u′‖u′‖0,∂Ω. |
Note that κ−1HAhuH and u0 differ by only one constant, thus, Step 3 in Scheme 3.1 is equivalent to
(κ−1H−Ah)u′=u0,uhj=u′‖u′‖0,∂Ω. |
So far, all conditions of Lemma 3.3 are valid.
Since Mh(κj) is a q-dimensional space, there must exist u∗∈Mh(κj) such that
‖uhj−u∗‖h=dist(uhj,Mh(κj)). |
For m=j,j+1,…,j+q−1, according to (2.11) we have
∣ρ0−ρm,h∣=|1κH−1κm,h|≤|κH−κm,h||κHκm,h|≤C(|κH−κj|+|κj−κm,h|)≤CH2t. | (3.13) |
Therefore, from Lemma 3.3, (3.12) and (3.13) we get
‖uhj−u∗‖h=dist(uhj,Mh(κj))≤Cϑmaxj≤m≤j+q−1|ρ0−ρm,h|dist(uH,Mh(κj))≤CH3t. | (3.14) |
From (2.13) we know that there exists uj∈M(κj), such that ‖u∗−uj‖h=dist(u∗,M(κj)), and
‖u∗−uj‖h≤Cht, |
then
‖uhj−uj‖h≤‖uhj−u∗‖h+‖u∗−uj‖h≤C(H3t+ht), |
that is (3.9).
Next, we will prove (3.10). From (2.12) we have
‖u∗−uj‖0,∂Ω≤Chr+t, |
which together with (3.14) yields
‖uhj−uj‖0,∂Ω≤‖uhj−u∗‖0,∂Ω+‖u∗−uj‖0,∂Ω≤C(H3t+hr+t). |
Finally, we use Lemma 3.1 to derive (3.11). From Step 4 of Scheme 3.1, Lemma 3.1, (3.9) and (3.10) we deduce that
|κhj−κj|=|ah(uhj−uj,uhj−uj)‖uhj‖2b−κjb(uhj−uj,uhj−uj)‖uhj‖2b|≤C(‖uhj−uj‖2h+|κj|‖uhj−uj‖20,∂Ω)≤C(H3t+ht)2. |
The proof is completed.
Remark 3.1. Using Theorem 3.1 and referring to Theorem 4.2 in [32], we can give the error estimates of Scheme 3.1. To ensure that the error is independent of the number of iterations in the multigrid refinement, we also need the following conditions.
Condition 3.1. For any given ε∈(0,1), there exists ti∈(1,2−ε](i=1,2,⋯), such that hi=O(htii−1), and hi→0(i→∞).
Condition 3.1 is easy to be satisfied. For instance, for smooth eigenfunctions, using uniform meshes and linear elements and taking ε=0.1,h0=√28,h1=√232,h2=√2128,⋯, then ti=log(hi)log(hi−1)=log(hi−1−log(4)log(hi−1), thus, t1≈1.80,t2≈1.44,t3≈1.31,⋯, and ti↘1 when i→∞.
Theorem 3.2. Suppose that Condition 3.1 holds and M(κj)⊂H1+s(Ω)(s≥r), and t=min{k,s}. Let (κhlj,uhlj) be an approximate eigenpair obtained by Scheme 3.1, then, when h0=H is small enough, there exists uj∈M(κj) such that
‖uhlj−uj‖h≤Chtl, | (3.15) |
‖uhlj−uj‖0,∂Ω≤Chr+tl, | (3.16) |
|κhlj−κj|≤Ch2tl,l≥1. | (3.17) |
In this section, we will report some numerical experiments to show the efficiency of the DG-multigrid method (Scheme 3.1) for solving the Steklov-Lamé eigenproblem. We conduct the numerical experiments on the MATLAB 2022a on a ThinkBook 14p Gen 2 PC with 16G memory, and our program makes use of the package of iFEM [35]. The test domains are set to be the unit square ΩS=(0,1)2 and the L-shaped domain ΩL=(−1,1)2∖[0,1)2.
Example 4.1. We use Scheme 3.1 to compute the approximation for the 1st eigenvalue κ1 of the problem (2.2). We adopt piecewise polynomial of degree 1 (P1 element) to compute on uniform isosceles right triangulations. We produce the initial coarse grid TH=Th0 and refine the coarse grid in a uniform way (each triangle is divided into four congruent triangles) repeatedly to obtain fine grids Thi,i=1,2,...,l. By using the basis functions of SH, the eigenvalue problem on the initial coarse grid in Step 1 of Scheme 3.1 can be rewritten as a generalized matrix eigenvalue problem
KHˉu1,H=κ1,HMHˉu1,H, | (4.1) |
where the elements of array ˉu1,H are the coordinates of u1,H under the basis functions in SH. Similarly, by using the basis functions of Shi, the algebraic system in Step 3 of Scheme 3.1 can be rewritten as
(Khi−κhi−11Mhi)ˆu=Mhiˆuhi−11 | (4.2) |
and ˉuhi1=ˆu√ˆuTMhiˆu where ˆuhi−11 is actually the projection of the solution ˉuhi−11 obtained on the previous grid Thi−1 in Thi. For example, if Th0 contains NT=2 elements with the associated solution ˉuh01=ˉu1,H, denote ˉuh01=[uh01,uh02]T with uh01=[a11,a21,a12,a22,a13,a23], uh02=[b11,b21,b12,b22,b13,b23] and aιj,bιj(j=1,2,3) being the coordinates of the basis function {1,x,y} on the element ι in Th0, and encrypt Th0 once (each triangle is divided into four congruent triangles) to get Th1 which contains 4NT=8 elements, then the projection of ˉuh01 in Th1 is as follows:
ˆuh01=Q1ˉuh01, |
where Q1 is the projection (restriction) operator:
Q1=[Q1100Q12],Q11=Q12=[I4NT000I4NT000I4NT],I4NT=[INTINTINTINT],INT=I2=[1001]. |
If bisecting encryption is used, that is, each triangle is divided into two triangles, then just replace I4NT with I2NT=[INTINT]. We use the command "eigs" of MATLAB to solve the discrete algebraic eigenvalue problem (4.1), and use the command "∖" in MATLAB to solve the linear system (4.2). Further, there has no difficulty with solving the system (4.2) (see Lecture 27.4 in [36]).
For comparison, we also use the multigrid method of conforming finite elements by adopting P1 element to compute. The error curves are depicted in Figure 1 where the reference value are taken as the most accurate approximations that we can compute. From Figure 1, we can see that as the Lamé parameter λ increases, the DG-multigrid method is robust compared with the multigrid method of conforming finite elements, which is a major advantage of using DG method to solve elastic problems.
Example 4.2. Adaptive computation.
Adaptive algorithm based on the a posterior error estimation is an efficient and important numerical approach for solving partial differential equations. Referring to [37], we combine the multigrid scheme 3.1 and the a posteriori error indicator to establish the adaptive multigrid algorithm. Referring to the a posterior error indicator (25) for the linear elastic source problem in [37], we formally give the following local error indicator for the underlying eigenvalue problem:
ζ2K(κh,uh)=h2K‖divσ(uh)‖2L2(K)+hK(‖Σn(uh)−n⋅σ(uh)‖2L2(∂K∖∂Ω)+‖Σn(uh)−n⋅σ(uh)‖2L2(∂K∩∂Ω))+h−1K‖[[uh]]‖2L2(∂K∖∂Ω), |
where
Σn(uh):={n⋅{σ(uh)}−γμh−1e[[uh]]−h−1eγλn(n⋅[[uh]]),on∂K∖∂Ω,(κh−1)uh,on∂K∩∂Ω. |
Define the global error indicator:
ζΩ=(∑K∈Thζ2K(κh,uh))12. |
Based on the above error indicators and Scheme 3.1, we design the following adaptive multigrid algorithm bases on the shifted inverse iteration.
Algorithm 4.1. Choose parameter 0<α<1.
Step 1: Pick any initial mesh Th0.
Step 2: Solve (2.3) on Th0 for discrete solution (κj,h0,uj,h0).
Step 3: Let l=1. uh1j⇐uj,h0,κh1j⇐κj,h0.
Step 4: Compute the local indicator ζK(κhlj,uhlj).
Step 5: Construct ˆThl⊂ Thl by Mark Strategy and parameter α.
Step 6: Refine Thl to get a new mesh Thl+1 by procedure REFINE.
Step 7: Find ˜u∈Shl+1 such that
ahl+1(˜u,ψ)−κhljbhl+1(˜u,ψ)=bhl+1(uhlj,ψ), ∀ψ∈Shl+1. |
Denote uhl+1j=˜u‖˜u‖0,∂Ω and compute the Rayleigh quotient
κhl+1j=ahl+1(uhl+1j,uhl+1j)bhl+1(uhl+1j,uhl+1j). |
Step 8: Let l=l+1 and go to Step 4.
Mark Strategy
Given parameter 0<α<1.
Step 1: Construct a minimal subset ˆThl of Thl by selecting some elements in Thl such that
∑K∈ˆThlζ2K(κhlj,uhlj)≥α∑K∈Thlζ2K(κhlj,uhlj). |
Step 2: Mark all elements in ˆThl.
Mark Strategy was first proposed in [38], and the procedure REFINE is some iterative or recursive bisection (see, e.g., [39,40]) of elements with the minimal refinement condition that marked elements are bisected at least once.
In addition, to investigate the efficiency of Algorithm 4.1, referring to the standard popular adaptive algorithm [41] we give the following Algorithm 4.2 for comparison.
Algorithm 4.2. Choose parameter 0<α<1.
Step 1: Pick any initial mesh Th0.
Step 2: Solve (2.3) on Th0 for discrete solution (κj,h1,uj,h1).
Step 3: Let l=1.
Step 4: Compute the local indicators ζK(κj,hl,uj,hl).
Step 5: Construct ˆThl⊂ Thl by Mark Strategy and parameter α.
Step 6: Refine Thl to get a new mesh Thl+1 by procedure REFINE.
Step 7: Solve (2.3) on Thl+1 for discrete solution (κj,hl+1,uj,hl+1).
Step 8: Let l=l+1 and go to Step 4.
We use the adaptive DG-multigrid method (Algorithm 4.1) with polynomials of degree 1 (P1 element) and degree 2 (P2 element) to compute, and take α=0.5. For convenience of reading, we specify the following notations in our tables and figures.
− Nj,l: the degrees of freedom at the lth iteration;
− κhlj: the jth eigenvalue obtained by Algorithm 4.1 at the l th iteration;
− κj,hl: the jth eigenvalue obtained by Algorithm 4.2 at the l th iteration;
− CPUj,l(s): the CPU time(s) from the first iteration beginning to the calculate results of the lth iteration appearing by using Algorithm 4.1/4.2;
− ej: the error of the jth approximate eigenvalue by Algorithm 4.1;
− ζj: the error indicator of the jth approximate eigenvalue by Algorithm 4.1.
We first give a numerical experiment comparison between using DGFEM to solve directly on fine meshes and using the adaptive DG-multigrid method (Algorithm 4.1) for the 1st nonzero eigenvalue of (2.3). The error curves are shown in Figures 2 and 3. An observation of the left and right subgraphs in Figures 2 and 3 tells us that the regularity of the eigenfunction in ΩL is lower than that in ΩS, which is consistent with the general conclusion of the regularity of solutions to PDEs. From Figure 2 we can see that the error curves of adaptive DG-multigrid method are all parallel to the line with slope −1 but the error curves of directly computing by DGFEM do not parallel, which indicates that the approximate eigenvalues obtained by the adaptive DG-multigrid method achieve the optimal convergence order. The same conclusion can be seen from Figure 3.
Now we use Algorithms 4.1 and 4.2 with P1 and P2 elements to compute the first 7 non-zero eigenvalues of (2.3) in \Omega_S and \Omega_L , respectively. When using P1 element, the parameters \mu = 1, \lambda = 1, \gamma_\mu = \gamma_\lambda = 10 and the diameter of initial mesh is taken as \frac{\sqrt{2}}{16} . Limited to space, we list the 1st, the 3rd, the 4th and the 6th approximate eigenvalue in Tables 1 and 2. We also depict the error curves of approximate eigenvalues by Algorithm 4.1 and the curve of error indicators in Figure 4, where the reference values are taken as the most accurate approximations that we can compute. In addition, for the 1st non-zero eigenvalue of (2.3), we investigate the influence of Lamé parameter by taking \lambda = 1, 10,100, 1000, 10000 , and the corresponding error curves are shown in Figure 5. When using P2 element, the parameters \mu = \lambda = 1, \gamma_\mu = \gamma_\lambda = 40 and the diameter of initial mesh is taken as \frac{\sqrt{2}}{8} . In Tables 3 and 4 we list the 1st, the 3rd, the 4th and the 6th approximate eigenvalue. We also plot the error curves of approximate eigenvalues by Algorithm 4.1 and the curve of error indicators in Figure 6. For the 1st non-zero eigenvalue of (2.3), we investigate the influence of Lamé parameter by taking \lambda = 1, 10,100, 1000, 10000 , and the corresponding error curves are shown in Figure 7.
j | l | N_{j, l} | \kappa_{j}^{h_l} | CPU_{j, l}(s) | l | N_{j, l} | \kappa_{j, h_l} | CPU_{j, l}(s) |
1 | 1 | 3600 | 2.5834151705 | 0.05433 | 1 | 3600 | 2.5834151539 | 0.06679 |
1 | 8 | 26328 | 2.5365029347 | 1.62136 | 8 | 26328 | 2.5365029348 | 2.52953 |
1 | 21 | 1208232 | 2.5310939833 | 249.69061 | 21 | 1208232 | 2.5310939827 | 391.04103 |
1 | 22 | 1570164 | 2.5310583228 | 485.28739 | 22 | 1570164 | 2.5310583228 | 651.02095 |
1 | 23 | 2050932 | 2.5310346038 | 817.8231911 | 23 | 2050932 | 2.531034604 | 1167.502765 |
3 | 1 | 3324 | 2.7404121518 | 0.02745 | 1 | 3324 | 2.7414582353 | 0.07244 |
3 | 11 | 30156 | 2.6778807957 | 1.75582 | 16 | 43908 | 2.6778901580 | 7.73674 |
3 | 20 | 428676 | 2.6740736381 | 57.92155 | 31 | 605400 | 2.6740696701 | 244.04938 |
3 | 23 | 1026540 | 2.6739097335 | 212.85588 | 34 | 1025280 | 2.6739660124 | 488.60793 |
3 | 24 | 1349388 | 2.6738786576 | 395.09095 | 35 | 1219860 | 2.6739372698 | 624.67912 |
4 | 1 | 3888 | 3.7164345114 | 0.05795 | 1 | 3888 | 3.7164345114 | 0.10192 |
4 | 9 | 44460 | 3.7115741607 | 3.84681 | 9 | 44460 | 3.7115741607 | 5.61523 |
4 | 22 | 1762944 | 3.7111432638 | 194.69801 | 22 | 1762944 | 3.7111432638 | 341.67732 |
4 | 23 | 2344044 | 3.7111398968 | 269.71127 | 23 | 2344044 | 3.7111398969 | 470.55583 |
4 | 24 | 3028620 | 3.7111378848 | 385.01813 | 24 | 3028620 | 3.7111378848 | 654.91996 |
6 | 1 | 3792 | 5.2873876626 | 0.06042 | 1 | 3792 | 5.2873876273 | 0.09334 |
6 | 5 | 11784 | 5.2632333578 | 0.70311 | 5 | 11784 | 5.2632333578 | 1.11777 |
6 | 20 | 942528 | 5.2537917257 | 198.46971 | 20 | 942528 | 5.2537917253 | 306.96848 |
6 | 21 | 1259328 | 5.2537597122 | 334.17056 | 21 | 1259328 | 5.2537597120 | 452.24058 |
6 | 22 | 1636512 | 5.2537348070 | 605.55218 | 22 | 1636512 | 5.2537348072 | 796.76924 |
j | l | N_{j, l} | \kappa_{j}^{h_l} | CPU_{j, l}(s) | l | N_{j, l} | \kappa_{j, h_l} | CPU_{j, l}(s) |
1 | 1 | 9252 | 1.1578406889 | 0.08684 | 1 | 9252 | 1.1578406889 | 0.21686 |
1 | 4 | 9960 | 1.1568717901 | 0.51428 | 4 | 9960 | 1.1568717901 | 1.05144 |
1 | 22 | 854196 | 1.1551704849 | 83.61915 | 22 | 854520 | 1.1551704801 | 143.97265 |
1 | 23 | 1125306 | 1.1551665423 | 116.92568 | 23 | 1125690 | 1.1551665389 | 193.87293 |
1 | 24 | 1469730 | 1.1551634294 | 330.33730 | 24 | 1470228 | 1.1551634258 | 431.50241 |
3 | 1 | 9852 | 2.0253499592 | 0.10770 | 1 | 9852 | 2.0253499590 | 0.24006 |
3 | 3 | 13236 | 2.0200276681 | 0.62807 | 3 | 13236 | 2.0200276680 | 1.21075 |
3 | 18 | 1072320 | 2.0137654513 | 221.81309 | 18 | 1072320 | 2.0137654513 | 344.53795 |
3 | 19 | 1431672 | 2.0137487824 | 351.63554 | 19 | 1431672 | 2.0137487822 | 590.05031 |
3 | 20 | 1924536 | 2.0137351315 | 711.94221 | 20 | 1924536 | 2.0137351315 | 1029.63061 |
4 | 1 | 9540 | 2.1396949065 | 0.10559 | 1 | 9540 | 2.1396949061 | 0.23500 |
4 | 4 | 13446 | 2.1322409848 | 0.70466 | 4 | 13446 | 2.1322409847 | 1.33492 |
4 | 19 | 823536 | 2.1258417612 | 147.60184 | 19 | 823536 | 2.1258417612 | 260.40436 |
4 | 20 | 1085094 | 2.1258166076 | 225.83332 | 20 | 1085094 | 2.1258166075 | 386.16427 |
4 | 21 | 1437324 | 2.1257991175 | 324.24898 | 21 | 1437324 | 2.1257991172 | 566.48046 |
6 | 1 | 9828 | 2.7939491213 | 0.15553 | 1 | 9828 | 2.7939490858 | 0.31958 |
6 | 3 | 12894 | 2.7668920157 | 0.78224 | 3 | 12894 | 2.7668920151 | 1.00058 |
6 | 18 | 965010 | 2.7367899041 | 163.71460 | 18 | 965010 | 2.7367899035 | 335.07813 |
6 | 19 | 1292184 | 2.7367099740 | 271.08934 | 19 | 1292184 | 2.7367099727 | 503.74878 |
6 | 20 | 1729368 | 2.7366439994 | 494.77192 | 20 | 1729368 | 2.7366439984 | 797.84910 |
j | l | N_{j, l} | \kappa_{j}^{h_l} | CPU_{j, l}(s) | l | N_{j, l} | \kappa_{j, h_l} | CPU_{j, l}(s) |
1 | 1 | 1632 | 2.5399573563 | 0.20754 | 1 | 1632 | 2.5399573531 | 0.33385 |
1 | 5 | 3600 | 2.5321126514 | 0.80285 | 5 | 3600 | 2.5321126514 | 1.16189 |
1 | 24 | 760152 | 2.5309641786 | 211.46084 | 24 | 760152 | 2.5309641786 | 239.26807 |
1 | 25 | 1003368 | 2.5309641677 | 278.22507 | 25 | 1003368 | 2.5309641677 | 320.01464 |
1 | 26 | 1339404 | 2.5309641607 | 378.58015 | 26 | 1339404 | 2.5309641607 | 439.05795 |
3 | 1 | 1584 | 2.6862908794 | 0.12511 | 1 | 1584 | 2.6862921826 | 0.40313 |
3 | 18 | 43920 | 2.6737990072 | 8.60574 | 24 | 46896 | 2.6737995054 | 17.94984 |
3 | 30 | 813360 | 2.6737894142 | 210.84097 | 42 | 823224 | 2.6737894180 | 409.26749 |
3 | 31 | 1021272 | 2.6737894039 | 275.45868 | 44 | 1129272 | 2.6737894027 | 587.31383 |
3 | 32 | 1276776 | 2.6737893962 | 363.84821 | 45 | 1328904 | 2.6737893981 | 708.17810 |
4 | 1 | 1824 | 3.7114311740 | 0.13369 | 1 | 1824 | 3.7114311740 | 0.21961 |
4 | 5 | 6360 | 3.7111583273 | 0.89324 | 5 | 6360 | 3.7111583273 | 1.89695 |
4 | 22 | 754512 | 3.7111313470 | 178.28979 | 22 | 754512 | 3.7111313471 | 250.31766 |
4 | 23 | 939408 | 3.7111313465 | 244.08245 | 23 | 939408 | 3.7111313466 | 341.16368 |
4 | 24 | 1218768 | 3.7111313461 | 339.61412 | 24 | 1218768 | 3.7111313462 | 460.76539 |
6 | 2 | 1920 | 5.2557235447 | 0.49529 | 2 | 1920 | 5.2557235444 | 0.59788 |
6 | 8 | 8904 | 5.2537750836 | 1.87161 | 8 | 8904 | 5.2537750836 | 2.28091 |
6 | 24 | 838920 | 5.2536682063 | 197.38184 | 24 | 838920 | 5.2536682064 | 267.40500 |
6 | 25 | 1119096 | 5.2536682008 | 270.08041 | 25 | 1119096 | 5.2536682009 | 376.06184 |
6 | 26 | 1456968 | 5.2536681969 | 380.87500 | 26 | 1456968 | 5.2536681971 | 520.85769 |
j | l | N_{j, l} | \kappa_{j}^{h_l} | CPU_{j, l}(s) | l | N_{j, l} | \kappa_{j, h_l} | CPU_{j, l}(s) |
1 | 1 | 4656 | 1.1562458918 | 0.38986 | 1 | 4656 | 1.1562458918 | 0.55317 |
1 | 25 | 66996 | 1.1551541513 | 18.31403 | 25 | 66996 | 1.1551541513 | 22.07079 |
1 | 38 | 774480 | 1.1551532609 | 260.06329 | 38 | 774480 | 1.1551532609 | 325.52552 |
1 | 39 | 923052 | 1.1551532590 | 320.61556 | 39 | 923052 | 1.1551532591 | 400.40419 |
1 | 40 | 1099044 | 1.1551532576 | 398.76151 | 40 | 1099044 | 1.1551532576 | 496.73607 |
3 | 1 | 4680 | 2.0159225406 | 0.46936 | 1 | 4680 | 2.0159225405 | 0.57221 |
3 | 9 | 19224 | 2.0137381688 | 4.00481 | 9 | 19224 | 2.0137381688 | 5.20982 |
3 | 23 | 976428 | 2.0136927626 | 233.96942 | 23 | 976428 | 2.0136927626 | 313.17266 |
3 | 24 | 1280052 | 2.0136927550 | 324.92148 | 24 | 1280052 | 2.0136927550 | 421.48383 |
3 | 25 | 1679592 | 2.0136927505 | 458.31975 | 25 | 1679592 | 2.0136927505 | 578.37647 |
4 | 10 | 11316 | 2.1259084959 | 3.98294 | 10 | 11316 | 2.1259084959 | 4.01302 |
4 | 21 | 105648 | 2.1257416544 | 27.89323 | 21 | 105648 | 2.1257416544 | 37.43586 |
4 | 32 | 822120 | 2.1257391878 | 297.07841 | 32 | 822120 | 2.1257391878 | 368.95873 |
4 | 33 | 980688 | 2.1257391755 | 362.49125 | 33 | 980688 | 2.1257391755 | 456.94719 |
4 | 34 | 1181328 | 2.1257391658 | 446.73985 | 34 | 1181328 | 2.1257391658 | 572.04947 |
6 | 1 | 4680 | 2.7468959498 | 0.40303 | 1 | 4680 | 2.7468959503 | 0.61874 |
6 | 3 | 5232 | 2.7405213916 | 0.92105 | 3 | 5232 | 2.7405213907 | 1.21365 |
6 | 23 | 1000704 | 2.7364519542 | 234.72412 | 23 | 1000704 | 2.7364519542 | 334.60146 |
6 | 24 | 1312716 | 2.7364519227 | 327.39089 | 24 | 1312716 | 2.7364519227 | 448.35877 |
6 | 25 | 1726080 | 2.7364519037 | 468.01995 | 25 | 1726080 | 2.7364519038 | 608.05296 |
It can be seen from Tables 1–4 that to get the same accurate approximate eigenvalues, Algorithm 4.1 uses less time or less degrees of freedom than Algorithm 4.2. In Figure 4, the error curves e_1, e_3, e_4 and e_6 are all parallel to the line with slope -1 , and in Figure 6 the error curves e_1, e_3, e_4 and e_6 are parallel to the line with slope -2 , which indicate that the approximate eigenvalues obtained by Algorithm 4.1 achieve the optimal convergence order. Meanwhile, in Figure 5, the error curves e_1, e_3, e_4 and e_6 are almost parallel to the curve of \zeta_1, \zeta_3, \zeta_4 and \zeta_6 respectively, and in Figure 7, the curves of e_1, e_3, e_4 and e_6 are parallel to \zeta_1, \zeta_3, \zeta_4 and \zeta_6 , which indicate that the error indicators are reliable and efficient. Figures 5 and 7 then show that Algorithm 4.1 is robust in both \Omega_S and \Omega_L .
In this paper, we discussed a multigrid discretization scheme of DGFEM based on the shifted-inverse iteration. Theoretical analysis and numerical results all showed that this method can efficiently solve the Steklov-Lamé eigenproblem as we expected. Generally, the time of solving a linear algebraic system is much less than that of solving an eigenvalue problem. Further, we observe from Tables 1–4 that although the CPU time of the adaptive DG-multigrid method is less than that of the standard adaptive DGFEM, the advantage is not obvious. We think that this may be because we use " \setminus " to solve linear algebraic systems. We notice that in recent research, the multigrid method has been combined with other methods to form many efficient algorithms and applied to many problems, as combined with the DG method in this paper. For example, the multigrid-homotopy method to diffusion equation [42], the multigrid method for the semilinear interface problem based on the modified two-grid method [43], the multigrid method for nonlinear eigenvalue problems based on Newton iteration [44], etc. It is of interest for us to explore more applications of multigrid methods and more efficient solvers for solving linear algebraic equations in multigrid methods.
This work was supported by the National Natural Science Foundation of China (No. 12261024) and Science and Technology Planning Project of Guizhou Province (Guizhou Kehe fundamental research-ZK[2022] No.324).
This work does not have any conflicts of interest.
[1] |
M. H. Abokersh, M Vallès, L. F. Cabeza, D. Boer, A framework for the optimal integration of solar assisted district heating in different urban sized communities: A robust machine learning approach incorporating global sensitivity analysis, Appl. Energy, 267 (2020), 114903. https://doi.org/10.1016/j.apenergy.2020.114903 doi: 10.1016/j.apenergy.2020.114903
![]() |
[2] |
I. Mitiche, A. Nesbitt, S. Conner, P. Boreham, G. Morison, 1D-CNN based real-time fault detection system for power asset diagnostics, IET Gener. Transm. Distrib. 14 (2020), 5766–5773. https://doi.org/10.1049/iet-gtd.2020.0773 doi: 10.1049/iet-gtd.2020.0773
![]() |
[3] |
M. Biswal, S. Ghore, O. P. Malik, R. C. Bansal, Development of time-frequency based approach to detect high impedance fault in an inverter interfaced distribution system, IEEE Trans. Power Delivery, 36 (2021), 3825–3833. https://doi.org/10.1109/TPWRD.2021.3049572 doi: 10.1109/TPWRD.2021.3049572
![]() |
[4] |
K. Koziy, B. Gou, J. Aslakson, A low-cost power-quality meter with series arc-fault detection capability for smart grid, IEEE Trans. Power Delivery, 28 (2013), 1584–1591. https://doi.org/10.1109/TPWRD.2013.2251753 doi: 10.1109/TPWRD.2013.2251753
![]() |
[5] |
O. Namaki-Shoushtari, B. Huang, Bayesian control loop diagnosis by combining historical data and process knowledge of fault signatures, IEEE Trans. Ind. Electron., 62 (2015), 3696–3704. https://doi.org/10.1109/TIE.2014.2375253 doi: 10.1109/TIE.2014.2375253
![]() |
[6] |
O. Lyashevska, F. Malone, E. Maccarthy, J. Fiehler, J. Buhk, L. Morris, Class imbalance in gradient boosting classification algorithms: Application to experimental stroke data, Stat. Methods Med. Res., 30 (2021), 916–925. https://doi.org/10.1177/0962280220980484 doi: 10.1177/0962280220980484
![]() |
[7] |
A. Yagi, L. Nguyen, T. V. Ta, A sustainability condition for stochastic forest model, Commun. Pure Appl. Anal., 16 (2017), 699–718. https://doi.org/10.3934/cpaa.2017034 doi: 10.3934/cpaa.2017034
![]() |
[8] |
B. de Silva, J. Callaham, J. Jonker, N. Goebel, J. Klemisch, D. McDonald, et al., Hybrid learning approach to sensor fault detection with flight test data, AIAA J., 59 (2021), 3490–3503. https://doi.org/10.2514/1.J059943 doi: 10.2514/1.J059943
![]() |
[9] |
J. Hage, P. Xu, P. Bonnifait, J. Ibanez-Guzman, Localization integrity for intelligent vehicles through fault detection and position error characterization, IEEE Trans. Intell. Transp. Syst., 23 (2020), 2978–2990. https://doi.org/10.1109/TITS.2020.3027433 doi: 10.1109/TITS.2020.3027433
![]() |
[10] |
M. Ma, P. He, Y. Li, H. Li, M. Jiang, Y. Wu, Fault diagnosis method based on multi-source information fusion for weak interturn short circuit in synchronous condensers, IET Electr. Power Appl., 15 (2021), 1245–1260. https://doi.org/10.1049/elp2.12094 doi: 10.1049/elp2.12094
![]() |
[11] |
J. Sonne, C. Seavey, J. Groshong, Rapid immunohistological measurement of tyrosine hydroxylase in rat midbrain by near-infrared instrument-based detection, arXiv preprint, (2021). https://doi.org/10.1016/j.jchemneu.2021.101992 doi: 10.1016/j.jchemneu.2021.101992
![]() |
[12] |
Y. Yamazaki, S. Kanaji, T. Matsuda, T. Oshikiri, T. Nakamuraet, S. Suzuki, et al., Automated surgical instrument detection from laparoscopic gastrectomy video images using an open source convolutional neural network platform, J. Am. Coll. Surg., 230 (2020), 725–732. https://doi.org/10.1016/j.jamcollsurg.2020.01.037 doi: 10.1016/j.jamcollsurg.2020.01.037
![]() |
[13] |
T. Zhou, T. Han, E. Droguett, Towards trustworthy machine fault diagnosis: A probabilistic Bayesian deep learning framework, Reliab. Eng. Syst. Saf., 224 (2022), 1065–1077. https://doi.org/10.1016/j.ress.2022.108525 doi: 10.1016/j.ress.2022.108525
![]() |
[14] |
J. Gompel, D. Spina, C. Develder, Satellite based fault diagnosis of photovoltaic systems using recurrent neural networks, Appl. Energy, 305 (2022), 117874. https://doi.org/10.1016/j.apenergy.2021.117874 doi: 10.1016/j.apenergy.2021.117874
![]() |
1. | Noura Alhouiti, Fatemah Mofarreh, Fatemah Abdullah Alghamdi, Akram Ali, Piscoran-Ioan Laurian, Geometric topology of CR-warped products in six-dimensional sphere, 2024, 9, 2473-6988, 25114, 10.3934/math.20241224 |
j | l | N_{j, l} | \kappa_{j}^{h_l} | CPU_{j, l}(s) | l | N_{j, l} | \kappa_{j, h_l} | CPU_{j, l}(s) |
1 | 1 | 3600 | 2.5834151705 | 0.05433 | 1 | 3600 | 2.5834151539 | 0.06679 |
1 | 8 | 26328 | 2.5365029347 | 1.62136 | 8 | 26328 | 2.5365029348 | 2.52953 |
1 | 21 | 1208232 | 2.5310939833 | 249.69061 | 21 | 1208232 | 2.5310939827 | 391.04103 |
1 | 22 | 1570164 | 2.5310583228 | 485.28739 | 22 | 1570164 | 2.5310583228 | 651.02095 |
1 | 23 | 2050932 | 2.5310346038 | 817.8231911 | 23 | 2050932 | 2.531034604 | 1167.502765 |
3 | 1 | 3324 | 2.7404121518 | 0.02745 | 1 | 3324 | 2.7414582353 | 0.07244 |
3 | 11 | 30156 | 2.6778807957 | 1.75582 | 16 | 43908 | 2.6778901580 | 7.73674 |
3 | 20 | 428676 | 2.6740736381 | 57.92155 | 31 | 605400 | 2.6740696701 | 244.04938 |
3 | 23 | 1026540 | 2.6739097335 | 212.85588 | 34 | 1025280 | 2.6739660124 | 488.60793 |
3 | 24 | 1349388 | 2.6738786576 | 395.09095 | 35 | 1219860 | 2.6739372698 | 624.67912 |
4 | 1 | 3888 | 3.7164345114 | 0.05795 | 1 | 3888 | 3.7164345114 | 0.10192 |
4 | 9 | 44460 | 3.7115741607 | 3.84681 | 9 | 44460 | 3.7115741607 | 5.61523 |
4 | 22 | 1762944 | 3.7111432638 | 194.69801 | 22 | 1762944 | 3.7111432638 | 341.67732 |
4 | 23 | 2344044 | 3.7111398968 | 269.71127 | 23 | 2344044 | 3.7111398969 | 470.55583 |
4 | 24 | 3028620 | 3.7111378848 | 385.01813 | 24 | 3028620 | 3.7111378848 | 654.91996 |
6 | 1 | 3792 | 5.2873876626 | 0.06042 | 1 | 3792 | 5.2873876273 | 0.09334 |
6 | 5 | 11784 | 5.2632333578 | 0.70311 | 5 | 11784 | 5.2632333578 | 1.11777 |
6 | 20 | 942528 | 5.2537917257 | 198.46971 | 20 | 942528 | 5.2537917253 | 306.96848 |
6 | 21 | 1259328 | 5.2537597122 | 334.17056 | 21 | 1259328 | 5.2537597120 | 452.24058 |
6 | 22 | 1636512 | 5.2537348070 | 605.55218 | 22 | 1636512 | 5.2537348072 | 796.76924 |
j | l | N_{j, l} | \kappa_{j}^{h_l} | CPU_{j, l}(s) | l | N_{j, l} | \kappa_{j, h_l} | CPU_{j, l}(s) |
1 | 1 | 9252 | 1.1578406889 | 0.08684 | 1 | 9252 | 1.1578406889 | 0.21686 |
1 | 4 | 9960 | 1.1568717901 | 0.51428 | 4 | 9960 | 1.1568717901 | 1.05144 |
1 | 22 | 854196 | 1.1551704849 | 83.61915 | 22 | 854520 | 1.1551704801 | 143.97265 |
1 | 23 | 1125306 | 1.1551665423 | 116.92568 | 23 | 1125690 | 1.1551665389 | 193.87293 |
1 | 24 | 1469730 | 1.1551634294 | 330.33730 | 24 | 1470228 | 1.1551634258 | 431.50241 |
3 | 1 | 9852 | 2.0253499592 | 0.10770 | 1 | 9852 | 2.0253499590 | 0.24006 |
3 | 3 | 13236 | 2.0200276681 | 0.62807 | 3 | 13236 | 2.0200276680 | 1.21075 |
3 | 18 | 1072320 | 2.0137654513 | 221.81309 | 18 | 1072320 | 2.0137654513 | 344.53795 |
3 | 19 | 1431672 | 2.0137487824 | 351.63554 | 19 | 1431672 | 2.0137487822 | 590.05031 |
3 | 20 | 1924536 | 2.0137351315 | 711.94221 | 20 | 1924536 | 2.0137351315 | 1029.63061 |
4 | 1 | 9540 | 2.1396949065 | 0.10559 | 1 | 9540 | 2.1396949061 | 0.23500 |
4 | 4 | 13446 | 2.1322409848 | 0.70466 | 4 | 13446 | 2.1322409847 | 1.33492 |
4 | 19 | 823536 | 2.1258417612 | 147.60184 | 19 | 823536 | 2.1258417612 | 260.40436 |
4 | 20 | 1085094 | 2.1258166076 | 225.83332 | 20 | 1085094 | 2.1258166075 | 386.16427 |
4 | 21 | 1437324 | 2.1257991175 | 324.24898 | 21 | 1437324 | 2.1257991172 | 566.48046 |
6 | 1 | 9828 | 2.7939491213 | 0.15553 | 1 | 9828 | 2.7939490858 | 0.31958 |
6 | 3 | 12894 | 2.7668920157 | 0.78224 | 3 | 12894 | 2.7668920151 | 1.00058 |
6 | 18 | 965010 | 2.7367899041 | 163.71460 | 18 | 965010 | 2.7367899035 | 335.07813 |
6 | 19 | 1292184 | 2.7367099740 | 271.08934 | 19 | 1292184 | 2.7367099727 | 503.74878 |
6 | 20 | 1729368 | 2.7366439994 | 494.77192 | 20 | 1729368 | 2.7366439984 | 797.84910 |
j | l | N_{j, l} | \kappa_{j}^{h_l} | CPU_{j, l}(s) | l | N_{j, l} | \kappa_{j, h_l} | CPU_{j, l}(s) |
1 | 1 | 1632 | 2.5399573563 | 0.20754 | 1 | 1632 | 2.5399573531 | 0.33385 |
1 | 5 | 3600 | 2.5321126514 | 0.80285 | 5 | 3600 | 2.5321126514 | 1.16189 |
1 | 24 | 760152 | 2.5309641786 | 211.46084 | 24 | 760152 | 2.5309641786 | 239.26807 |
1 | 25 | 1003368 | 2.5309641677 | 278.22507 | 25 | 1003368 | 2.5309641677 | 320.01464 |
1 | 26 | 1339404 | 2.5309641607 | 378.58015 | 26 | 1339404 | 2.5309641607 | 439.05795 |
3 | 1 | 1584 | 2.6862908794 | 0.12511 | 1 | 1584 | 2.6862921826 | 0.40313 |
3 | 18 | 43920 | 2.6737990072 | 8.60574 | 24 | 46896 | 2.6737995054 | 17.94984 |
3 | 30 | 813360 | 2.6737894142 | 210.84097 | 42 | 823224 | 2.6737894180 | 409.26749 |
3 | 31 | 1021272 | 2.6737894039 | 275.45868 | 44 | 1129272 | 2.6737894027 | 587.31383 |
3 | 32 | 1276776 | 2.6737893962 | 363.84821 | 45 | 1328904 | 2.6737893981 | 708.17810 |
4 | 1 | 1824 | 3.7114311740 | 0.13369 | 1 | 1824 | 3.7114311740 | 0.21961 |
4 | 5 | 6360 | 3.7111583273 | 0.89324 | 5 | 6360 | 3.7111583273 | 1.89695 |
4 | 22 | 754512 | 3.7111313470 | 178.28979 | 22 | 754512 | 3.7111313471 | 250.31766 |
4 | 23 | 939408 | 3.7111313465 | 244.08245 | 23 | 939408 | 3.7111313466 | 341.16368 |
4 | 24 | 1218768 | 3.7111313461 | 339.61412 | 24 | 1218768 | 3.7111313462 | 460.76539 |
6 | 2 | 1920 | 5.2557235447 | 0.49529 | 2 | 1920 | 5.2557235444 | 0.59788 |
6 | 8 | 8904 | 5.2537750836 | 1.87161 | 8 | 8904 | 5.2537750836 | 2.28091 |
6 | 24 | 838920 | 5.2536682063 | 197.38184 | 24 | 838920 | 5.2536682064 | 267.40500 |
6 | 25 | 1119096 | 5.2536682008 | 270.08041 | 25 | 1119096 | 5.2536682009 | 376.06184 |
6 | 26 | 1456968 | 5.2536681969 | 380.87500 | 26 | 1456968 | 5.2536681971 | 520.85769 |
j | l | N_{j, l} | \kappa_{j}^{h_l} | CPU_{j, l}(s) | l | N_{j, l} | \kappa_{j, h_l} | CPU_{j, l}(s) |
1 | 1 | 4656 | 1.1562458918 | 0.38986 | 1 | 4656 | 1.1562458918 | 0.55317 |
1 | 25 | 66996 | 1.1551541513 | 18.31403 | 25 | 66996 | 1.1551541513 | 22.07079 |
1 | 38 | 774480 | 1.1551532609 | 260.06329 | 38 | 774480 | 1.1551532609 | 325.52552 |
1 | 39 | 923052 | 1.1551532590 | 320.61556 | 39 | 923052 | 1.1551532591 | 400.40419 |
1 | 40 | 1099044 | 1.1551532576 | 398.76151 | 40 | 1099044 | 1.1551532576 | 496.73607 |
3 | 1 | 4680 | 2.0159225406 | 0.46936 | 1 | 4680 | 2.0159225405 | 0.57221 |
3 | 9 | 19224 | 2.0137381688 | 4.00481 | 9 | 19224 | 2.0137381688 | 5.20982 |
3 | 23 | 976428 | 2.0136927626 | 233.96942 | 23 | 976428 | 2.0136927626 | 313.17266 |
3 | 24 | 1280052 | 2.0136927550 | 324.92148 | 24 | 1280052 | 2.0136927550 | 421.48383 |
3 | 25 | 1679592 | 2.0136927505 | 458.31975 | 25 | 1679592 | 2.0136927505 | 578.37647 |
4 | 10 | 11316 | 2.1259084959 | 3.98294 | 10 | 11316 | 2.1259084959 | 4.01302 |
4 | 21 | 105648 | 2.1257416544 | 27.89323 | 21 | 105648 | 2.1257416544 | 37.43586 |
4 | 32 | 822120 | 2.1257391878 | 297.07841 | 32 | 822120 | 2.1257391878 | 368.95873 |
4 | 33 | 980688 | 2.1257391755 | 362.49125 | 33 | 980688 | 2.1257391755 | 456.94719 |
4 | 34 | 1181328 | 2.1257391658 | 446.73985 | 34 | 1181328 | 2.1257391658 | 572.04947 |
6 | 1 | 4680 | 2.7468959498 | 0.40303 | 1 | 4680 | 2.7468959503 | 0.61874 |
6 | 3 | 5232 | 2.7405213916 | 0.92105 | 3 | 5232 | 2.7405213907 | 1.21365 |
6 | 23 | 1000704 | 2.7364519542 | 234.72412 | 23 | 1000704 | 2.7364519542 | 334.60146 |
6 | 24 | 1312716 | 2.7364519227 | 327.39089 | 24 | 1312716 | 2.7364519227 | 448.35877 |
6 | 25 | 1726080 | 2.7364519037 | 468.01995 | 25 | 1726080 | 2.7364519038 | 608.05296 |
j | l | N_{j, l} | \kappa_{j}^{h_l} | CPU_{j, l}(s) | l | N_{j, l} | \kappa_{j, h_l} | CPU_{j, l}(s) |
1 | 1 | 3600 | 2.5834151705 | 0.05433 | 1 | 3600 | 2.5834151539 | 0.06679 |
1 | 8 | 26328 | 2.5365029347 | 1.62136 | 8 | 26328 | 2.5365029348 | 2.52953 |
1 | 21 | 1208232 | 2.5310939833 | 249.69061 | 21 | 1208232 | 2.5310939827 | 391.04103 |
1 | 22 | 1570164 | 2.5310583228 | 485.28739 | 22 | 1570164 | 2.5310583228 | 651.02095 |
1 | 23 | 2050932 | 2.5310346038 | 817.8231911 | 23 | 2050932 | 2.531034604 | 1167.502765 |
3 | 1 | 3324 | 2.7404121518 | 0.02745 | 1 | 3324 | 2.7414582353 | 0.07244 |
3 | 11 | 30156 | 2.6778807957 | 1.75582 | 16 | 43908 | 2.6778901580 | 7.73674 |
3 | 20 | 428676 | 2.6740736381 | 57.92155 | 31 | 605400 | 2.6740696701 | 244.04938 |
3 | 23 | 1026540 | 2.6739097335 | 212.85588 | 34 | 1025280 | 2.6739660124 | 488.60793 |
3 | 24 | 1349388 | 2.6738786576 | 395.09095 | 35 | 1219860 | 2.6739372698 | 624.67912 |
4 | 1 | 3888 | 3.7164345114 | 0.05795 | 1 | 3888 | 3.7164345114 | 0.10192 |
4 | 9 | 44460 | 3.7115741607 | 3.84681 | 9 | 44460 | 3.7115741607 | 5.61523 |
4 | 22 | 1762944 | 3.7111432638 | 194.69801 | 22 | 1762944 | 3.7111432638 | 341.67732 |
4 | 23 | 2344044 | 3.7111398968 | 269.71127 | 23 | 2344044 | 3.7111398969 | 470.55583 |
4 | 24 | 3028620 | 3.7111378848 | 385.01813 | 24 | 3028620 | 3.7111378848 | 654.91996 |
6 | 1 | 3792 | 5.2873876626 | 0.06042 | 1 | 3792 | 5.2873876273 | 0.09334 |
6 | 5 | 11784 | 5.2632333578 | 0.70311 | 5 | 11784 | 5.2632333578 | 1.11777 |
6 | 20 | 942528 | 5.2537917257 | 198.46971 | 20 | 942528 | 5.2537917253 | 306.96848 |
6 | 21 | 1259328 | 5.2537597122 | 334.17056 | 21 | 1259328 | 5.2537597120 | 452.24058 |
6 | 22 | 1636512 | 5.2537348070 | 605.55218 | 22 | 1636512 | 5.2537348072 | 796.76924 |
j | l | N_{j, l} | \kappa_{j}^{h_l} | CPU_{j, l}(s) | l | N_{j, l} | \kappa_{j, h_l} | CPU_{j, l}(s) |
1 | 1 | 9252 | 1.1578406889 | 0.08684 | 1 | 9252 | 1.1578406889 | 0.21686 |
1 | 4 | 9960 | 1.1568717901 | 0.51428 | 4 | 9960 | 1.1568717901 | 1.05144 |
1 | 22 | 854196 | 1.1551704849 | 83.61915 | 22 | 854520 | 1.1551704801 | 143.97265 |
1 | 23 | 1125306 | 1.1551665423 | 116.92568 | 23 | 1125690 | 1.1551665389 | 193.87293 |
1 | 24 | 1469730 | 1.1551634294 | 330.33730 | 24 | 1470228 | 1.1551634258 | 431.50241 |
3 | 1 | 9852 | 2.0253499592 | 0.10770 | 1 | 9852 | 2.0253499590 | 0.24006 |
3 | 3 | 13236 | 2.0200276681 | 0.62807 | 3 | 13236 | 2.0200276680 | 1.21075 |
3 | 18 | 1072320 | 2.0137654513 | 221.81309 | 18 | 1072320 | 2.0137654513 | 344.53795 |
3 | 19 | 1431672 | 2.0137487824 | 351.63554 | 19 | 1431672 | 2.0137487822 | 590.05031 |
3 | 20 | 1924536 | 2.0137351315 | 711.94221 | 20 | 1924536 | 2.0137351315 | 1029.63061 |
4 | 1 | 9540 | 2.1396949065 | 0.10559 | 1 | 9540 | 2.1396949061 | 0.23500 |
4 | 4 | 13446 | 2.1322409848 | 0.70466 | 4 | 13446 | 2.1322409847 | 1.33492 |
4 | 19 | 823536 | 2.1258417612 | 147.60184 | 19 | 823536 | 2.1258417612 | 260.40436 |
4 | 20 | 1085094 | 2.1258166076 | 225.83332 | 20 | 1085094 | 2.1258166075 | 386.16427 |
4 | 21 | 1437324 | 2.1257991175 | 324.24898 | 21 | 1437324 | 2.1257991172 | 566.48046 |
6 | 1 | 9828 | 2.7939491213 | 0.15553 | 1 | 9828 | 2.7939490858 | 0.31958 |
6 | 3 | 12894 | 2.7668920157 | 0.78224 | 3 | 12894 | 2.7668920151 | 1.00058 |
6 | 18 | 965010 | 2.7367899041 | 163.71460 | 18 | 965010 | 2.7367899035 | 335.07813 |
6 | 19 | 1292184 | 2.7367099740 | 271.08934 | 19 | 1292184 | 2.7367099727 | 503.74878 |
6 | 20 | 1729368 | 2.7366439994 | 494.77192 | 20 | 1729368 | 2.7366439984 | 797.84910 |
j | l | N_{j, l} | \kappa_{j}^{h_l} | CPU_{j, l}(s) | l | N_{j, l} | \kappa_{j, h_l} | CPU_{j, l}(s) |
1 | 1 | 1632 | 2.5399573563 | 0.20754 | 1 | 1632 | 2.5399573531 | 0.33385 |
1 | 5 | 3600 | 2.5321126514 | 0.80285 | 5 | 3600 | 2.5321126514 | 1.16189 |
1 | 24 | 760152 | 2.5309641786 | 211.46084 | 24 | 760152 | 2.5309641786 | 239.26807 |
1 | 25 | 1003368 | 2.5309641677 | 278.22507 | 25 | 1003368 | 2.5309641677 | 320.01464 |
1 | 26 | 1339404 | 2.5309641607 | 378.58015 | 26 | 1339404 | 2.5309641607 | 439.05795 |
3 | 1 | 1584 | 2.6862908794 | 0.12511 | 1 | 1584 | 2.6862921826 | 0.40313 |
3 | 18 | 43920 | 2.6737990072 | 8.60574 | 24 | 46896 | 2.6737995054 | 17.94984 |
3 | 30 | 813360 | 2.6737894142 | 210.84097 | 42 | 823224 | 2.6737894180 | 409.26749 |
3 | 31 | 1021272 | 2.6737894039 | 275.45868 | 44 | 1129272 | 2.6737894027 | 587.31383 |
3 | 32 | 1276776 | 2.6737893962 | 363.84821 | 45 | 1328904 | 2.6737893981 | 708.17810 |
4 | 1 | 1824 | 3.7114311740 | 0.13369 | 1 | 1824 | 3.7114311740 | 0.21961 |
4 | 5 | 6360 | 3.7111583273 | 0.89324 | 5 | 6360 | 3.7111583273 | 1.89695 |
4 | 22 | 754512 | 3.7111313470 | 178.28979 | 22 | 754512 | 3.7111313471 | 250.31766 |
4 | 23 | 939408 | 3.7111313465 | 244.08245 | 23 | 939408 | 3.7111313466 | 341.16368 |
4 | 24 | 1218768 | 3.7111313461 | 339.61412 | 24 | 1218768 | 3.7111313462 | 460.76539 |
6 | 2 | 1920 | 5.2557235447 | 0.49529 | 2 | 1920 | 5.2557235444 | 0.59788 |
6 | 8 | 8904 | 5.2537750836 | 1.87161 | 8 | 8904 | 5.2537750836 | 2.28091 |
6 | 24 | 838920 | 5.2536682063 | 197.38184 | 24 | 838920 | 5.2536682064 | 267.40500 |
6 | 25 | 1119096 | 5.2536682008 | 270.08041 | 25 | 1119096 | 5.2536682009 | 376.06184 |
6 | 26 | 1456968 | 5.2536681969 | 380.87500 | 26 | 1456968 | 5.2536681971 | 520.85769 |
j | l | N_{j, l} | \kappa_{j}^{h_l} | CPU_{j, l}(s) | l | N_{j, l} | \kappa_{j, h_l} | CPU_{j, l}(s) |
1 | 1 | 4656 | 1.1562458918 | 0.38986 | 1 | 4656 | 1.1562458918 | 0.55317 |
1 | 25 | 66996 | 1.1551541513 | 18.31403 | 25 | 66996 | 1.1551541513 | 22.07079 |
1 | 38 | 774480 | 1.1551532609 | 260.06329 | 38 | 774480 | 1.1551532609 | 325.52552 |
1 | 39 | 923052 | 1.1551532590 | 320.61556 | 39 | 923052 | 1.1551532591 | 400.40419 |
1 | 40 | 1099044 | 1.1551532576 | 398.76151 | 40 | 1099044 | 1.1551532576 | 496.73607 |
3 | 1 | 4680 | 2.0159225406 | 0.46936 | 1 | 4680 | 2.0159225405 | 0.57221 |
3 | 9 | 19224 | 2.0137381688 | 4.00481 | 9 | 19224 | 2.0137381688 | 5.20982 |
3 | 23 | 976428 | 2.0136927626 | 233.96942 | 23 | 976428 | 2.0136927626 | 313.17266 |
3 | 24 | 1280052 | 2.0136927550 | 324.92148 | 24 | 1280052 | 2.0136927550 | 421.48383 |
3 | 25 | 1679592 | 2.0136927505 | 458.31975 | 25 | 1679592 | 2.0136927505 | 578.37647 |
4 | 10 | 11316 | 2.1259084959 | 3.98294 | 10 | 11316 | 2.1259084959 | 4.01302 |
4 | 21 | 105648 | 2.1257416544 | 27.89323 | 21 | 105648 | 2.1257416544 | 37.43586 |
4 | 32 | 822120 | 2.1257391878 | 297.07841 | 32 | 822120 | 2.1257391878 | 368.95873 |
4 | 33 | 980688 | 2.1257391755 | 362.49125 | 33 | 980688 | 2.1257391755 | 456.94719 |
4 | 34 | 1181328 | 2.1257391658 | 446.73985 | 34 | 1181328 | 2.1257391658 | 572.04947 |
6 | 1 | 4680 | 2.7468959498 | 0.40303 | 1 | 4680 | 2.7468959503 | 0.61874 |
6 | 3 | 5232 | 2.7405213916 | 0.92105 | 3 | 5232 | 2.7405213907 | 1.21365 |
6 | 23 | 1000704 | 2.7364519542 | 234.72412 | 23 | 1000704 | 2.7364519542 | 334.60146 |
6 | 24 | 1312716 | 2.7364519227 | 327.39089 | 24 | 1312716 | 2.7364519227 | 448.35877 |
6 | 25 | 1726080 | 2.7364519037 | 468.01995 | 25 | 1726080 | 2.7364519038 | 608.05296 |