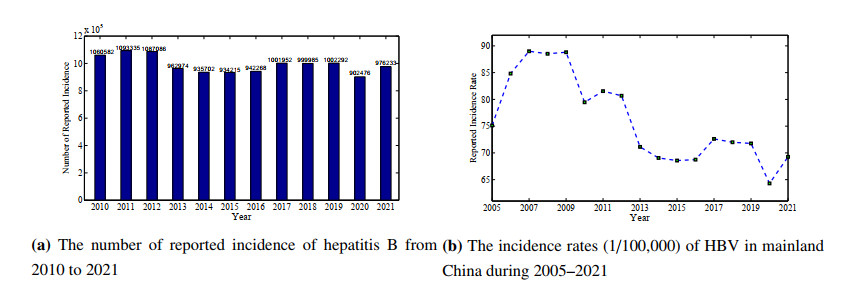
Hepatitis B virus (HBV) infection is a global public health problem and there are 257 million people living with chronic HBV infection throughout the world. In this paper, we investigate the dynamics of a stochastic HBV transmission model with media coverage and saturated incidence rate. Firstly, we prove the existence and uniqueness of positive solution for the stochastic model. Then the condition on the extinction of HBV infection is obtained, which implies that media coverage helps to control the disease spread and the noise intensities on the acute and chronic HBV infection play a key role in disease eradication. Furthermore, we verify that the system has a unique stationary distribution under certain conditions, and the disease will prevail from the biological perspective. Numerical simulations are conducted to illustrate our theoretical results intuitively. As a case study, we fit our model to the available hepatitis B data of mainland China from 2005 to 2021.
Citation: Jiying Ma, Shasha Ma. Dynamics of a stochastic hepatitis B virus transmission model with media coverage and a case study of China[J]. Mathematical Biosciences and Engineering, 2023, 20(2): 3070-3098. doi: 10.3934/mbe.2023145
[1] | Kangbo Bao, Qimin Zhang, Xining Li . A model of HBV infection with intervention strategies: dynamics analysis and numerical simulations. Mathematical Biosciences and Engineering, 2019, 16(4): 2562-2586. doi: 10.3934/mbe.2019129 |
[2] | Tingting Xue, Long Zhang, Xiaolin Fan . Dynamic modeling and analysis of Hepatitis B epidemic with general incidence. Mathematical Biosciences and Engineering, 2023, 20(6): 10883-10908. doi: 10.3934/mbe.2023483 |
[3] | Tailei Zhang, Hui Li, Na Xie, Wenhui Fu, Kai Wang, Xiongjie Ding . Mathematical analysis and simulation of a Hepatitis B model with time delay: A case study for Xinjiang, China. Mathematical Biosciences and Engineering, 2020, 17(2): 1757-1775. doi: 10.3934/mbe.2020092 |
[4] | Maysaa Al Qurashi, Saima Rashid, Fahd Jarad . A computational study of a stochastic fractal-fractional hepatitis B virus infection incorporating delayed immune reactions via the exponential decay. Mathematical Biosciences and Engineering, 2022, 19(12): 12950-12980. doi: 10.3934/mbe.2022605 |
[5] | Fathalla A. Rihan, Hebatallah J. Alsakaji . Analysis of a stochastic HBV infection model with delayed immune response. Mathematical Biosciences and Engineering, 2021, 18(5): 5194-5220. doi: 10.3934/mbe.2021264 |
[6] | Dong-Me Li, Bing Chai, Qi Wang . A model of hepatitis B virus with random interference infection rate. Mathematical Biosciences and Engineering, 2021, 18(6): 8257-8297. doi: 10.3934/mbe.2021410 |
[7] | Dwi Lestari, Noorma Yulia Megawati, Nanang Susyanto, Fajar Adi-Kusumo . Qualitative behaviour of a stochastic hepatitis C epidemic model in cellular level. Mathematical Biosciences and Engineering, 2022, 19(2): 1515-1535. doi: 10.3934/mbe.2022070 |
[8] | Suxia Zhang, Hongbin Guo, Robert Smith? . Dynamical analysis for a hepatitis B transmission model with immigration and infection age. Mathematical Biosciences and Engineering, 2018, 15(6): 1291-1313. doi: 10.3934/mbe.2018060 |
[9] | Sarah Kadelka, Stanca M Ciupe . Mathematical investigation of HBeAg seroclearance. Mathematical Biosciences and Engineering, 2019, 16(6): 7616-7658. doi: 10.3934/mbe.2019382 |
[10] | Xiaojing Wang, Yu Liang, Jiahui Li, Maoxing Liu . Modeling COVID-19 transmission dynamics incorporating media coverage and vaccination. Mathematical Biosciences and Engineering, 2023, 20(6): 10392-10403. doi: 10.3934/mbe.2023456 |
Hepatitis B virus (HBV) infection is a global public health problem and there are 257 million people living with chronic HBV infection throughout the world. In this paper, we investigate the dynamics of a stochastic HBV transmission model with media coverage and saturated incidence rate. Firstly, we prove the existence and uniqueness of positive solution for the stochastic model. Then the condition on the extinction of HBV infection is obtained, which implies that media coverage helps to control the disease spread and the noise intensities on the acute and chronic HBV infection play a key role in disease eradication. Furthermore, we verify that the system has a unique stationary distribution under certain conditions, and the disease will prevail from the biological perspective. Numerical simulations are conducted to illustrate our theoretical results intuitively. As a case study, we fit our model to the available hepatitis B data of mainland China from 2005 to 2021.
Hepatitis B, a viral liver infection caused by the hepatitis B virus (HBV), is a major threat to public health and security around the whole world. HBV can cause both acute and chronic hepatitis. Acute hepatitis B refers to the virus infection less than half a year and part of the patients can recover and get lifetime immunity. The disease course of chronic hepatitis B can be quite long, and it can lead to severe liver disease such as cirrhosis and liver cancer [1]. Globally 257 million people were living with chronic hepatitis B infection, which resulted in estimated 887,000 deaths in 2015. According to the latest fact sheets released by the World Health Organization (WHO), 296 million people were living with chronic hepatitis B infection in 2019, and there are 1.5 million new infections each year [2]. Worldwide, hepatitis B resulted in an estimated 820,000 deaths in 2019, mostly from cirrhosis and hepatocellular carcinoma (primary liver cancer) [2]. Hence, hepatitis B epidemic is still a major global health problem.
In China, hepatitis B is one of the top three infectious diseases reported by the Chinese Center for Disease Control and Prevention (CDC) [3]. Based on the sero-epidemiological investigation of hepatitis B in 1992, about 9.75% of the general population in China was chronic HBV carriers. That is, around 130 million people of mainland China were carriers of HBV in the 1990s [1]. Then an effective and national vaccination program has been conducted by the government, especially the hepatitis B planned immunization for newborn babies and children. The sero-epidemiological survey in 2006 showed about 7.18% of the population was hepatitis B carriers, and the number of hepatitis B carriers was reduced by 30 million [3]. Lately in 2014, an epidemiology survey of people younger than 29 reported that the HBV infection rates were 0.32% among the group of children aged one to four, 0.94% in the group aged 5 to 14, and 4.18% in the group aged 15 to 29, respectively. The government of mainland China has achieved remarkable results in the prevention and treatment of hepatitis B. Nevertheless, the epidemic situation remains to be severe and complicated. At present there are 80 million HBV carriers in mainland China, among whom 28 million carriers need immediate medical treatment. During the past decade, the number of reported incidence of hepatitis B is around one million each year (Figure 1(a)). The incidence rates of hepatitis B in mainland China from 2005 to 2021 are presented in Figure 1(b), and the incidence rates varied from 89.00 per 100,000 in 2007 (the highest during 2005–2021) to 64.29 per 100,000 in 2020 (the lowest during 2005–2021).
The incidence of hepatitis B is different among regions, and it could be influenced by various factors such as vaccination and economy. Figure 2 displays the hepatitis B incidence rates in 31 provinces and municipalities of mainland China, and the data in 2007 and 2020 are chosen and displayed. The figure shows that the incidence decreased for most provinces (22 of 31), especially for Ningxia, Gansu, Henan, Xinjiang and Qinghai. This strongly reveals that the immunization program with hepatitis B vaccine is successful in most provinces, and the achievement is remarkable for western areas in China. However, it should be pointed out, in 2020, the incidence varied from 6.15 per 100,000 in Beijing to 150.99 per 100,000 in Qinghai. There is still a gap between the impoverished west and the prosperous east.
In the last three decades, the research on hepatitis B has attracted more and more attention from the perspective of epidemiology and biomathematics. For instance, in 1991, Anderson and May used a simple mathematical model to study the impacts of carriers on the transmission of HBV [4]. Zhao et al. [5] studied the dynamics of HBV transmission and proposed vaccination strategy to prevent the prevalence in the population. In 2010, Zou et al. [1] formulated a high dimensional deterministic model to analyze the transmission dynamics of HBV infection in China. Then in 2015 Zou et al. [6] investigated the sexual transmission dynamics of HBV in China. Zhang et al. [7] proposed a four dimensional HBV epidemic model involving an exponential birth rate and vertical transmission, and fitted the parameters to the public data in Xinjiang, China. Recently Din and Li [8] analyzed a stochastic hepatitis B epidemic model with vaccination effect and showed a case study for Pakistan. For more mathematical epidemic models on HBV infection, one can refer to [9,10,11,12] and the references therein.
Besides the classical compartmental models for hepatitis B epidemic, a vibrant field of research on the virus transmission dynamics has emerged and developed rapidly [12]. The modeling approaches usually incorporate the interaction among intracellular viral dynamics, multicellular infection process, and immune responses [13,14,15,16]. For instance, Wang et al. [13] formulated a multi-scale computational model of SARS-CoV-2 infection using a combination of differential equations and stochastic modeling, and revealed heterogeneity among COVID-19 patients. Rihan and Alsakaji [14] analyzed a stochastic delay HBV infection model with cell-to-cell transmission and cytotoxic T lymphocytes (CTLs) immune response. Recently Mohajerani et al. [17] proposed a multiscale modeling of HBV capsid assembly pathways, by constructing Markov state models and employing transition path theory.
When an infectious disease breaks out in one area, the center for disease control and prevention will release authoritative information through the mass media at the first moment, including the potential risk of the disease and prevention measures [18]. Media coverage helps to raise public health awareness, increase vaccine rates and reduce the spread of infections. Recently, a number of epidemic models have taken into account the influence of media coverage on the spread of infectious disease [19,20,21,22,23]. Their study suggests that media coverage is critical in disease eradication [19,20]. There are different forms of function to represent the effect of media coverage in epidemic models. Let S and I denote the number of the population that are susceptible and infectious respectively, and let β be the transmission rate. Cui et al. [19] used the exponential function βexp(−mI)SI to denote the incidence rate with media coverage, where m>0. In another work [20], the incidence rate incorporating media coverage takes the form: (β−β2f(I))SI, where β>β2 and the function f(I) satisfies f(0)=0, f′(I)≥0, limI→+∞f(I)=1. In the present paper, similar to the approach in the previous literatures [21,24,25], we adopt the most common form f(I)=Ib+I, where b is a half saturation constant.
Furthermore, the transmission of HBV is disturbed by various random factors in the environment, such as population mobility and unpredictable exposure to infections. An increasing number of researchers have formulated stochastic hepatitis B epidemic models considering environmental noise [8,10,11]. In fact, environment disturbances have an important effect on the evolution of infectious diseases [26,27,28,29,30], and Gaussian white noise is usually selected as an appropriate representation of environmental fluctuations [31]. For instance, Wang et al. [28] and Meng et al. [29] proved that a large disturbance of white noise can lead infectious diseases to extinction. A large number of works also indicate that stochastic disturbance can suppress disease outbreak [18,26].
In the present paper, motivated by the above discussion, we formulate and investigate a stochastic hepatitis B model with media coverage and saturated incidence rate. We obtain a sufficient condition for the extinction of the disease, and prove that the stochastic system has a unique stationary distribution under certain conditions. Moreover, as a case study, we utilize our model for fitting the available data of mainland China from 2005 to 2021. Based on our simulation result, the incidence rate of hepatitis B in China will remain around 50–60 per 100,000 in the long term.
The paper is organized as follows. In Section 2, we present the model formulation. In Section 3, the existence and uniqueness of positive solution is proved for the stochastic model. In Section 4, we obtain a sufficient condition for the extinction of the disease. In Section 5, the sufficient condition on the existence of stationary distribution is obtained, which indicates that all the compartments will be persistent. Moreover, we provide an estimation of lower bound of the expectation for the number of infected cases. In Section 6, numerical simulations are conducted to illustrate our theoretical results. As a case study, we fit our model to the available HBV data of mainland China from 2005 to 2021.
Recently, Khan et al. [10] proposed a stochastic model for the transmission of HBV. In their work, the population is divided into four compartments: susceptible humans S; acute HBV infections I1; chronical HBV infections I2; and recovered population R. They further assumed: (i) the contact of susceptible individuals with acutely and chronically infected hepatitis B individuals primarily causes acutely infected species; (ii) the transmission coefficient β is subject to random fluctuation, that is, βi→βi+ηi˙Bi(t) for i=1,2, where Bi(t) is standard Brownian motion with Bi(0)=0 and with the noise intensity η2i>0. Then the stochastic hepatitis B epidemic model in [10] was presented as follows
{dS(t)=[Λ−2∑i=1βiS(t)Ii(t)−(μ0+υ)S(t)]dt−2∑i=1ηiS(t)Ii(t)dBi(t),dI1(t)=[2∑i=1βiS(t)Ii(t)−(μ0+γ+γ1)I1(t)]dt+2∑i=1ηiS(t)Ii(t)dBi(t),dI2(t)=[γI1(t)−(μ0+μ1+γ2)I2(t)]dt,dR(t)=[γ1I1(t)+γ2I2(t)+υS(t)−μ0R(t)]dt, | (2.1) |
where Λ is the recruitment rate of the population, μ0 is the natural mortality rate, μ1 is the disease mortality rate, and υ represents the vaccination rate of hepatitis B. Moreover, βi (i=1,2) represent the transmission rate of hepatitis B, γ is the moving rate of acutely infected humans to chronic stage, and γi (i=1,2) denote the recovery rates of acutely and chronically infected hepatitis B individuals, respectively.
In the above model, the authors chose bilinear incidence rate, that is, βSI. As a matter of fact, the transmission of infectious diseases is complicated, and nonlinear incidence rates have been wildly utilized in epidemic model [26,29]. In 1978, to describe the phenomenon that incidence is increasing and the population is saturated with the infective, Capasso and Serio [32] proposed a saturated incidence rate βSI1+αI. Then such saturated incidence has been extensively used in epidemic models. For instance, Khan and Zaman [33] and Liu et al. [11] have formulated hepatitis B epidemic models with saturated incidence rate. In addition, as discussed in the introduction part, we also incorporate the impact of media coverage by using the function f(I)=Ib+I. Consequently, based on the deterministic part of model (2.1), we obtain the hepatitis B model with media coverage and saturated incidence rate as follows
{dS(t)dt=Λ−(β11−β12I1(t)b1+I1(t))S(t)I1(t)1+α1I1(t)−(β21−β22I2(t)b2+I2(t))S(t)I2(t)1+α2I2(t)−(μ0+υ)S(t),dI1(t)dt=(β11−β12I1(t)b1+I1(t))S(t)I1(t)1+α1I1(t)+(β21−β22I2(t)b2+I2(t))S(t)I2(t)1+α2I2(t)−(μ0+γ+γ1)I1(t),dI2(t)dt=γI1(t)−(μ0+μ1+γ2)I2(t),dR(t)dt=γ1I1(t)+γ2I2(t)+υS(t)−μ0R(t), | (2.2) |
where the forms SIi1+αiIi (i=1,2) represent the saturated incidence rates of acute and chronical infections, and the forms Iibi+Ii (i=1,2) denote the media coverage functions. Moreover, βi1 (i=1,2) represent the transmission rates for acute and chronical infections, and βi2 (i=1,2) denote the maximal reduced contact rates by mass media alert for acute and chronical infections, respectively. Recall that βi1>βi2 (i=1,2).
Furthermore, the disease transmission and biological populations are inevitably affected by environment noises. There are different approaches to add random perturbations to biological systems. The transmission rates βi (i=1,2) are assumed to be disturbed by Gaussian white noise in model (2.1). In this article, following the approach in [8,11,34,35], we assume that the environmental white noise is proportional to each state variable S(t),I1(t),I2(t) and R(t). Finally we extend model (2.2) to the following stochastic model
{dS(t)=[Λ−(β11−β12I1b1+I1)SI11+α1I1−(β21−β22I2b2+I2)SI21+α2I2−(μ0+υ)S]dt+σ1SdB1(t),dI1(t)=[(β11−β12I1b1+I1)SI11+α1I1+(β21−β22I2b2+I2)SI21+α2I2−(μ0+γ+γ1)I1]dt+σ2I1dB2(t),dI2(t)=[γI1−(μ0+μ1+γ2)I2]dt+σ3I2dB3(t),dR(t)=[γ1I1+γ2I2+υS−μ0R]dt+σ4RdB4(t), | (2.3) |
where Bi(t) (i=1,2,3,4) are independent standard Brownian motions with Bi(0)=0, and σ2i>0 (i=1,2,3,4) denote the intensities of white noise.
Throughout the paper, let (Ω,F,{Ft}t≥0,P) be a complete probability space with a filtration {Ft}t≥0 satisfying the usual conditions (i.e., it is increasing and right continuous while F0 contains all P-null sets), then Bi(t) (i=1,2,3,4) are defined on this complete probability space. We also introduce the following notations: Rd+={(x1,x2,...,xd)∈Rd:xi>0,i=1,2,...,d}, a∧b=min{a,b}, a∨b=max{a,b}, ⟨f⟩=1t∫t0f(r)dr.
Since S,I1,I2 and R in system (2.3) denote the number of individuals, they should be nonnegative from the viewpoint of biology. We first introduce some basic definitions that will be used in the reminder of the article [36]. In general, let X(t) be a homogeneous Markov process in the d-dimension Euclidean space Rd described by the stochastic differential equation
dX(t)=f(X)dt+k∑r=1σr(X)dBr(t), | (3.1) |
then the diffusion matrix is defined as
A(x)=(aij(x)),aij(x)=k∑r=1σir(x)σjr(x). |
Furthermore, the differential operator L is defined by
LV(x)=d∑i=1fi(x)∂V(x)∂xi+12d∑i,j=1aij(x)∂2V(x)∂xi∂xj, |
where V(x) is an arbitrary twice continuously differential real-value function.
In this section, we obtain the following theorem which guarantees the existence and uniqueness of positive solution for system (2.3).
Theorem 3.1. For any initial value (S(0),I1(0),I2(0),R(0))∈R4+, there is a unique positive solution (S(t),I1(t),I2(t),R(t)) for system (2.3) on t≥0 and the solution will remain in R4+ with probability one, namely, (S(t),I1(t),I2(t),R(t))∈R4+ for all t≥0 almost surely.
Proof. Since the coefficients of system (2.3) are locally Lipschitz continuous in R4+, then for any initial value (S(0),I1(0),I2(0),R(0))∈R4+, there exists a unique positive solution (S(t),I1(t),I2(t),R(t)) on t∈[0,τe), where τe is the explosion time [37]. Thus, it suffices to verify S(t), I1(t), I2(t) and R(t) do not explode to infinity in a finite time, that is, τe=∞ a.s. Let k0>0 be sufficiently large such that S(0), I1(0), I2(0) and R(0) all lie within the interval [1k0,k0]. For each integer k≥k0, define the stopping time
τk=inf{t∈[0,τe):min{S(t),I1(t),I2(t),R(t)}≤1kormax{S(t),I1(t),I2(t),R(t)}≥k}, |
and throughout this paper we set inf∅=∞ (as usual ∅ is the empty set). Clearly, τk is increasing as k→∞. Let τ∞=limk→∞τk, then τ∞≤τe a.s. Thus, τ∞=∞ a.s. implies τe=∞ a.s. Now we state that τ∞=∞. If this assertion is false, then there is a pair of constants T>0 and ϵ∈(0,1) such that
P{τ∞≤T}>ϵ. |
Thus there exists an integer k1≥k0 such that
P{τk≤T}≥ϵfor allk≥k1. | (3.2) |
Define a C2-function V:R4+→R+ by
V(S,I1,I2,R)=(S−a−alnSa)+(I1−b−blnI1b)+(I2−1−lnI2)+(R−1−lnR), |
where a and b are positive constants to be determined later. The nonnegativity of this function can be obtained from
v−1−lnv≥0for anyv>0. |
Apply Itô's formula [37] to V, and we obtain
dV(S,I1,I2,R)=LV(S,I1,I2,R)dt+σ1(S−a)dB1(t)+σ2(I1−b)dB2(t)+σ3(I2−1)dB3(t)+σ4(R−1)dB4(t), | (3.3) |
where
LV=(1−aS)[Λ−(β11−β12I1b1+I1)SI11+α1I1−(β21−β22I2b2+I2)SI21+α2I2−(μ0+υ)S]+aσ212+(1−bI1)[(β11−β12I1b1+I1)SI11+α1I1+(β21−β22I2b2+I2)SI21+α2I2−(μ0+γ+γ1)I1]+bσ222+(1−1I2)[γI1−(μ0+μ1+γ2)I2]+σ232+(1−1R)[γ1I1+γ2I2+υS−μ0R]+σ242=Λ−μ0S−aΛS+a(β11−β12I1b1+I1)I11+α1I1+a(β21−β22I2b2+I2)I21+α2I2+a(μ0+υ+σ212)−μ0I1−b(β11−β12I1b1+I1)S1+α1I1−b(β21−β22I2b2+I2)SI2I1(1+α2I2)+b(μ0+γ+γ1+σ222)−(μ0+μ1)I2−γI1I2+μ0+μ1+γ2+σ232−μ0R−γ1I1R−γ2I2R−υSR+μ0+σ242≤Λ−μ0S−μ0I1−(μ0+μ1)I2+aβ11I11+α1I1+aβ21I21+α2I2+a(μ0+υ+σ212)+bβ12I1S(b1+I1)(1+α1I1)+b(μ0+γ+γ1+σ222)+2μ0+μ1+γ2+σ232+σ242≤Λ−μ0S−μ0I1−(μ0+μ1)I2+aβ11I1+aβ21I2+a(μ0+υ+σ212)+bβ12Sb1α1+b(μ0+γ+γ1+σ222)+2μ0+μ1+γ2+σ232+σ242=Λ+(bβ12b1α1−μ0)S+(aβ11−μ0)I1+(aβ21−μ0−μ1)I2+a(μ0+υ+σ212)+b(μ0+γ+γ1+σ222)+2μ0+μ1+γ2+σ232+σ242. |
Choose a=min{μ0β11,μ0+μ1β21} and b=μ0b1α1β12, then
aβ11−μ0≤0,aβ21−μ0−μ1≤0andbβ12b1α1−μ0=0, |
and
LV(S,I1,I2,R)≤Λ+a(μ0+υ+σ212)+b(μ0+γ+γ1+σ222)+2μ0+μ1+γ2+σ232+σ242:=K, |
where K is a positive number. Thus, according to Eq (3.3), one can get
dV(S,I1,I2,R)≤Kdt+σ1(S−a)dB1(t)+σ2(I1−b)dB2(t)+σ3(I2−1)dB3(t)+σ4(R−1)dB4(t). |
Let k≥k1 and integrate the above inequality from 0 to τk∧T, then we have
∫τk∧T0dV(S,I1,I2,R)≤∫τk∧T0Kdt+∫τk∧T0σ1(S−a)dB1(t)+∫τk∧T0σ2(I1−b)dB2(t)+∫τk∧T0σ3(I2−1)dB3(t)+∫τk∧T0σ4(R−1)dB4(t). | (3.4) |
Taking the expectation of both sides of Eq (3.4) yields
E(V(S(τk∧T),I1(τk∧T),I2(τk∧T),R(τk∧T)))≤V(S(0),I1(0),I2(0),R(0))+KT. | (3.5) |
Set Ωk={ω∈Ω:τk=τk(ω)≤T} for k≥k1, then we have P(Ωk)≥ϵ by (3.2). Note that for every ω∈Ωk, at least one of S(τk,ω),I1(τk,ω),I2(τk,ω) and R(τk,ω) equals to either k or 1k, So V(S(τk,ω),I1(τk,ω),I2(τk,ω),R(τk,ω)) is no less than either
k−1−lnkor1k−1−ln1korn−a−alnkaor1k−a+aln(ka)ork−b−blnkbor1k−b+bln(kb). |
Thus, one can obtain
V(S(τk),I1(τk),I2(τk),R(τk))≥(k−1−lnk)∧(1k−1−ln1k)∧(k−a−alnka)∧(1k−a+aln(ka))∧(k−b−blnkb)∧(1k−b+bln(kb)). |
It then follows from Eq (3.5) that
∞>V(S(0),I1(0),I2(0),R(0))+KT≥E(IΩn(ω)V(S(τk),I1(τk),I2(τk),R(τk))=P(Ωk)V(S(τk),I1(τk),I2(τk),R(τk))≥ϵV(S(τk),I1(τk),I2(τk),R(τk))≥ϵ[(k−1−lnk)∧(1k−1−ln1k)∧(k−a−alnka)∧(1k−a+aln(ka))∧(k−b−blnkb)∧(1k−b+bln(kb))], |
where IΩk(ω) is the indicator function of Ωk. Taking k→∞ will induce ∞>V(S(0),I1(0),I2(0),R(0))+KT≥+∞, and it is a contradiction. Hence, we must have τ∞=∞ a.s.
The conclusion is confirmed.
In this section, we mainly discuss the extinction of hepatitis B under some conditions. To begin with, we present the following theorem.
Theorem 4.1. If min{μ0,μ1}>(σ21∨σ22∨σ23∨σ24)2, then the solution (S(t),I2(t),Sh(t),Ih(t)) of system (2.3) has the following properties
limt→∞S(t)t=0,limt→∞I1(t)t=0,limt→∞I2(t)t=0,limt→∞R(t)t=0a.s. |
and
limt→∞∫t0S(s)dB1(s)t=0,limt→∞∫t0I1(s)dB2(s)t=0,limt→∞∫t0I2(s)dB3(s)t=0,limt→∞∫t0R(s)dB4(s)t=0a.s. |
The proof is similar to those in [38] and hence is omitted here.
It is easy to compute that the deterministic system (2.2) admits a disease-free equilibrium point E0(Λμ0+υ,0,0,Λυμ0(μ0+υ)). For the stochastic system (2.3), we obtain a sufficient condition on the extinction of disease in the following theorem.
Theorem 4.2. Let (S(t),I1(t),I2(t),R(t)) be a solution of system (2.3) with initial value (S(0), I1(0), I2(0), R(0))∈R4+. If Rs0:=4Λ(2β11−β12+2β21−β22)μ0(σ22∧σ23)<1 and min{μ0,μ1}>(σ21∨σ22∨σ23∨σ24)2 hold, then the disease will tend to extinction with probability one, and the solution of system (2.3) satisfies
limt→∞I1(t)=0,limt→∞I2(t)=0,lim supt→∞⟨S(t)+R(t)⟩=Λμ0. |
Proof. Denote
Q(t)=I1(t)+I2(t), |
and applying Itô's formula to lnQ yields
dlnQ={1I1+I2[(β11−β12I1b1+I1)SI11+α1I1+(β21−β22I2b2+I2)SI21+α2I2−(μ0+γ1)I1−(μ0+μ1+γ2)I2]−12(I1+I2)2(σ22I21+σ23I22)}dt+σ2I1I1+I2dB2(t)+σ3I2I1+I2dB3(t)≤{(2β11−β12)S+(2β21−β22)S−σ22∧σ234(I21+I22)(I21+I22)}dt+σ2I1I1+I2dB2(t)+σ3I2I1+I2dB3(t)={(2β11−β12)S+(2β21−β22)S−σ22∧σ234}dt+σ2I1I1+I2dB2(t)+σ3I2I1+I2dB3(t). | (4.1) |
Integrate inequality (4.1) from 0 to t and divide by t on both sides, then we get
lnQ(t)−lnQ(0)t≤(2β11−β12+2β21−β22)⟨S⟩−σ22∧σ234+σ2t∫t0I1I1+I2dB2(s)+σ3t∫t0I2I1+I2dB3(s). | (4.2) |
According to system (2.3), we have
d(S+I1+I2+R)=[Λ−μ0(S+I1+I2+R)−μ1I2]dt+σ1SdB1(t)+σ2I1dB2(t)+σ3I2dB3(t)+σ4RdB4(t)≤[Λ−μ0(S+I1+I2+R)]dt+σ1SdB1(t)+σ2I1dB2(t)+σ3I2dB3(t)+σ4RdB4(t). | (4.3) |
Integrate the above inequality form 0 to t and divide by t on both sides, then
S(t)+I1(t)+I2(t)+R(t)t−S(0)+I1(0)+I2(0)+R(0)t≤Λ−μ0t∫t0(S+I1+I2+R)ds+σ1∫t0S(s)dB1(s)t+σ2∫t0I1(s)dB2(s)t+σ3∫t0I2(s)dB3(s)t+σ4∫t0R(s)dB4(s)t. | (4.4) |
Furthermore, it follows from Theorem 4.1 that
lim supt→∞⟨S+I1+I2+R⟩≤Λμ0a.s. | (4.5) |
Therefore,
lim supt→∞⟨S⟩≤Λμ0a.s. | (4.6) |
Take the superior limit on both sides of inequality (4.2), then we have
lim supt→∞lnQ(t)t≤(2β11−β12+2β21−β22)Λμ0−σ22∧σ234=σ22∧σ234(Rs0−1)<0a.s. |
Consequently,
limt→∞Q(t)=0a.s., |
which implies that
limt→∞I1(t)=0,limt→∞I2(t)=0a.s. | (4.7) |
On the other hand, according to Eq (4.3), we have
S(t)+I1(t)+I2(t)+R(t)t−S(0)+I1(0)+I2(0)+R(0)t=Λ−μ0t∫t0(S+I1+I2+R)ds−μ1t∫t0I2ds+σ1∫t0S(s)dB1(s)t+σ2∫t0I1(s)dB2(s)t+σ3∫t0I2(s)dB3(s)t+σ4∫t0R(s)dB4(s)t. | (4.8) |
According to Theorem 4.1 and Eq (4.7), we obtain
lim supt→∞⟨S(t)+R(t)⟩=Λμ0. |
The conclusion is confirmed.
Remark 1. According to the expression of Rs0, it is clear that the value of Rs0 decreases with the increase of σ2,3, β12 and β22. Since βi2 (i=1,2) represent the maximal reduced contact rates by mass media, the media coverage plays an important role in disease eradication.
In this section, we focus on the existence of stationary distribution for system (2.3). From the biological point of view, stationary distribution can be interpreted as a weak stability of the system, and the disease will be persistent in the time mean sense. We first present a fundamental lemma.
Lemma 5.1. ([39]) The Markov process X(t), the solution of system (3.1), has a unique ergodic stationary distribution π(⋅), if there exists a bounded domain D⊂Rd with regular boundary Γ and
(C.1) there is a positive number M such that ∑di,j=1aij(x)ξiξj≥M|ξ|2, x∈D, ξ∈Rd,
(C.2) there exists a nonnegative C2-function V such that LV is negative for any x∈Rd∖D. Then
Px{limT→∞1T∫T0f(X(t))dt=∫Rdf(x)π(dx)}=1, |
for all x∈Rd, where f(⋅) is a function integrable with respect to the measure π.
Define
ˆRs0=Λ(μ0+γ+γ1+σ222)(2β11−β12α1+2β21−β22α2+μ0+υ+σ212)[β11−β121+α1Λμ0+γ+γ1+γ(β21−β22)μ0+μ1+γ2+σ232]. |
Theorem 5.2. If ˆRs0>1, then there exists a unique stationary distribution for system (2.3) and it has the ergodic property.
Proof. We will prove the theorem by verifying the conditions in Lemma 5.1. The diffusion matrix of model (2.3) is given by
A=[σ21S20000σ22I210000σ23I220000σ24R2]. |
Apparently, the matrix A is positive definite for any compact subset of R4+, so the condition (C.1) in Lemma 5.1 holds.
Define V1=−lnS, then
LV1=−ΛS+(β11−β12I1b1+I1)I11+α1I1+(β21−β22I2b2+I2)I21+α2I2+μ0+υ+σ212. |
Define
V2=−lnI1+a1V1+a2α1μ0+γ+γ1I1+c1V1−c2lnI2+c3α2μ0+μ1+γ2I2+a2α1μ0+γ+γ1(S+R), |
where a1,a2,c1,c2 and c3 are positive constants to be chosen later. By the use of Itô's formula, we obtain
LV2=−(β11−β12I1b1+I1)S1+α1I1−(β21−β22I2b2+I2)SI2(1+α2I2)I1+(μ0+γ+γ1+σ222)−a1ΛS+a1(β11−β12I1b1+I1)I11+α1I1+a1(β21−β22I2b2+I2)I21+α2I2+a1(μ0+υ+σ212)+a2−a2(α1I1+1)−c1ΛS+c1(β11−β12I1b1+I1)I11+α1I1+c1(β21−β22I2b2+I2)I21+α2I2+c1(μ0+υ+σ212)−c2γI1I2+c2(μ0+μ1+γ2+σ232)+c3α2γμ0+μ1+γ2I1−c3(α2I2+1)+c3+a2α1Λμ1+γ+γ1−μ0a2α1μ1+γ+γ1S+a2α1γ1μ0+γ+γ1I1+a2α1γ2μ0+γ+γ1I2−a2α1μ0μ0+γ+γ1R≤−(β11−β12)S1+α1I1−(β21−β22)SI2(1+α2I2)I1+(μ0+γ+γ1+σ222)−a1ΛS+a1(μ0+υ+σ212)+a1(2β11−β12)α1+a1(2β21−β22)α2−a2(α1I1+1)+a2−c1ΛS+c1(2β11−β12)α1+c1(2β21−β22)α2+c1(μ0+υ+σ212)−c2γI1I2+c2(μ0+μ1+γ2+σ232)+c3α2γμ0+μ1+γ2I1−c3(α2I2+1)+c3+a2α1Λμ1+γ+γ1+a2α1γ1μ0+γ+γ1I1+a2α1γ2μ0+γ+γ1I2≤−33√(β11−β12)a1a2Λ+a1(2β11−β12α1+2β21−β22α2+μ0+υ+σ212)+a2(1+α1Λμ1+γ+γ1)−44√(β21−β22)c1c2c3γΛ+c1(2β11−β12α1+2β21−β22α2+μ0+υ+σ212)+c2(μ0+μ1+γ2+σ232)+c3+(c3α2γμ0+μ1+γ2+a2α1γ1μ0+γ+γ1)I1+a2α1γ2μ0+γ+γ1I2+μ0+γ+γ1+σ222. | (5.1) |
Choose
a1=Λ(β11−β12)(2β11−β12α1+2β21−β22α2+μ0+υ+σ212)2(1+α1Λμ0+γ+γ1), |
a2=Λ(β11−β12)(2β11−β12α1+2β21−β22α2+μ0+υ+σ212)(1+α1Λμ0+γ+γ1)2, |
c1=Λγ(β21−β22)(2β11−β12α1+2β21−β22α2+μ0+υ+σ212)2(μ0+μ1+γ2+σ232), |
c2=Λγ(β21−β22)(2β11−β12α1+2β21−β22α2+μ0+υ+σ212)(μ0+μ1+γ2+σ232)2, |
and
c3=Λγ(β21−β22)(2β11−β12α1+2β21−β22α2+μ0+υ+σ212)(μ0+μ1+γ2+σ232). |
It follows from inequality (5.1), that
LV2≤−Λ(β11−β12)(2β11−β12α1+2β21−β22α2+μ0+υ+σ212)(1+α1Λμ1+γ+γ1)−Λγ(β21−β22)(2β11−β12α1+2β21−β22α2+μ0+υ+σ212)(μ0+μ1+γ2+σ232)+μ0+γ+γ1+σ222+(c3α1γμ0+μ1+γ2+a2α1γ1μ0+γ+γ1)I1+a2α1γ2μ0+γ+γ1I2=−(μ0+γ+γ1+σ222)(ˆRs0−1)+(c3α2γμ0+μ1+γ2+a2α1γ1μ0+γ+γ1)I1+a2α1γ2μ0+γ+γ1I2. | (5.2) |
Define
V3=V2+a2α1γ2(μ0+γ+γ1)(μ0+μ1+γ2)I2, |
then by inequality (5.2) one can derive
LV3≤−(μ0+γ+γ1+σ222)(ˆRs0−1)+(a2α1γ1μ0+γ+γ1+a2α1γ2γ(μ0+γ+γ1)(μ0+μ1+γ2)+c3α2γμ0+μ1+γ2)I1≤−λ+λ1I1, | (5.3) |
where
λ=(μ0+γ+γ1+σ222)(ˆRs0−1)>0, |
and
λ1=c3α2γμ0+μ1+γ2+a2α1γ1μ0+γ+γ1+a2α1γ2γ(μ0+γ+γ1)(μ0+μ1+γ2). |
Define
V4=−lnS,V5=−lnI2,V6=−lnR,V7=1θ+1(S+I1+I2+R)θ+1, |
where θ is a positive constant that is less than one. Thus, we obtain
LV4=−ΛS+(β11−β12I1b1+I1)I11+α1I1+(β21−β22I2b2+I2)I21+α2I2+(μ0+υ+σ212)≤−ΛS+(2β11−β12)I1+(2β21−β22)I2+(μ0+υ+σ212), | (5.4) |
LV5=−1I2[γI1−(μ0+μ1+γ2)I2]+σ232=−γI1I2+μ0+μ1+γ2+σ232, | (5.5) |
LV6=−1R[γ1I1+γ2I2+υS−μ0R]+σ242=−γ1I1R−γ2I2R−υSR+μ0+σ242, | (5.6) |
and
LV7=(S+I1+I2+R)θ[Λ−μ0(S+I1+I2+R)−μ1I2]+θ2(S+I1+I2+R)θ−1(σ21S2+σ22I21+σ23I22+σ24R2)≤(S+I1+I2+R)θ[Λ−μ0(S+I1+I2+R)]+θ2(S+I1+I2+R)θ+1(σ21∨σ22∨σ23∨σ24)=Λ(S+I1+I2+R)θ−μ0(S+I1+I2+R)θ+1+θ2(S+I1+I2+R)θ+1(σ21∨σ22∨σ23∨σ24)=Λ(S+I1+I2+R)θ−[μ0−θ2(σ21∨σ22∨σ23∨σ24)](S+I1+I2+R)θ+1≤A−12[μ0−θ2(σ21∨σ22∨σ23∨σ24)](S+I1+I2+R)θ+1≤A−12[μ0−θ2(σ21∨σ22∨σ23∨σ24)](Sθ+1+Iθ+11+Iθ+12+Rθ+1), | (5.7) |
where
A=sup(S,I1,I2,R)∈R4+{Λ(S+I1+I2+R)θ−12[μ0−θ2(σ21∨σ22∨σ23∨σ24)](Sθ+1+Iθ+11+Iθ+12+Rθ+1)}<∞. |
Define a C2-function ˜V:R4+→R by
˜V=MV3+V4+V5+V6+V7. |
Choose a positive constant M such that
−Mλ+E≤−2, | (5.8) |
where
E=sup(S,I1I2,R)∈R4+{−12[μ0−θ2(σ21∨σ22∨σ23∨σ24)](Sθ+1+Iθ+11+Iθ+12+Rθ+1)+(2β21−β22)I2+A+3μ0+υ+μ1+γ2+σ212+σ232+σ242}. |
It is easy to check that
lim infn→∞(S,I1,I2,R)∈R4+∖Dn˜V(S,I1,I2,R)=∞, |
here, Dn=(1n,n)×(1n,n)×(1n,n)×(1n,n). Thus one can see that there exists at least one minimum point (S∗,I∗1,I∗2,R∗) for the function ˜V(S,I1,I2,R). Define a nonnegative C2-function V:R4+→R+ by
V(S(t),I1(t),I2(t),R(t))=˜V(S(t),I1(t),I2(t),R(t))−˜V(S∗,I∗1,I∗2,R∗). |
According to inequalities (5.3)–(5.7), we obtain
LV≤−Mλ+Mλ1I1−ΛS+(2β11−β12)I1+(2β21−β22)I2−γI1I2−γ1I1R−γ2I2R−υSR+A+3μ0−12[μ0−θ2(σ21∨σ22∨σ23∨σ24)](Sθ+1+Iθ+11+Iθ+12+Rθ+1)+υ+μ1+γ2+σ212+σ232+σ242. |
Now we denote a bounded closed set as follows
Dϵ={(S,I1,I2,R)∈R4+:ϵ<S<1ϵ,ϵ<I1<1ϵ,ϵ<I2<1ϵ,ϵ<R<1ϵ}, |
where ϵ>0, and we choose ϵ sufficiently small such that the following conditions hold in the set R4+∖Dϵ
−min{Λ,υ,γ,γ1,γ2}ϵ+D≤−1, | (5.9) |
−Mλ+Mλ1ϵ+(2β11−β12)ϵ+E≤−1, | (5.10) |
−14[μ0−θ2(σ21∨σ22∨σ23∨σ24)]1ϵθ+1+F≤−1, | (5.11) |
−14[μ0−θ2(σ21∨σ22∨σ23∨σ24)]1ϵθ+1+G≤−1, | (5.12) |
−14[μ0−θ2(σ21∨σ22∨σ23∨σ24)]1ϵθ+1+H≤−1, | (5.13) |
−14[μ0−θ2(σ21∨σ22∨σ23∨σ24)]1ϵθ+1+J≤−1, | (5.14) |
where D,F,G,H,J are all positive constants which will be determined later. Hence, R4+∖Dϵ can be divided into the following ten domains,
D1ϵ={(S,I1,I2,R)∈R4+:0≤S≤ϵ},D2ϵ={(S,I1,I2,R)∈R4+:0≤R≤ϵ,S>ϵ},D3ϵ={(S,I1,I2,R)∈R4+:0≤I1≤ϵ},D4ϵ={(S,I1,I2,R)∈R4+:0≤R≤ϵ,I1>ϵ},D5ϵ={(S,I1,I2,R)∈R4+:0≤I2≤ϵ,I1>ϵ},D6ϵ={(S,I1,I2,R)∈R4+:0≤R≤ϵ,I2>ϵ},D7ϵ={(S,I1,I2,R)∈R4+:S≥1ϵ},D8ϵ={(S,I1,I2,R)∈R4+:I1≥1ϵ},D9ϵ={(S,I1,I2,R)∈R4+:I2≥1ϵ},D10ϵ={(S,I1,I2,R)∈R4+:R≥1ϵ}. |
Obviously, R4+∖Dϵ=D1ϵ⋃D2ϵ⋃D3ϵ⋃D4ϵ⋃D5ϵ⋃D6ϵ⋃D7ϵ⋃D8ϵ⋃D9ϵ⋃D10ϵ. Our next task is to verify LV≤−1 on R4+∖Dϵ.
Case 1. If (S,I1,I2,R)∈D1ϵ, then
LV≤−ΛS+Mλ1I1+(2β11−β12)I1+(2β21−β22)I2+3μ0+μ1+γ2+υ+A+σ212+σ232+σ242−12[μ0−θ2(σ21∨σ22∨σ23∨σ24)](Sθ+1+Iθ+11+Iθ+12+Rθ+1)≤−ΛS+D≤−Λϵ+D, | (5.15) |
where
D=sup(S,I1,I2,R)∈R4+{−12[μ0−θ2(σ21∨σ22∨σ23∨σ24)](Sθ+1+Iθ+11+Iθ+12+Rθ+1)+Mλ1I1+(2β11−β12)I1+(2β21−β22)I2+3μ0+μ1+γ2+υ+A+σ212+σ232+σ242}. |
According to inequality (5.9), we obtain that LV≤−1 for all (S,I1,I2,R)∈D1ϵ.
Case 2. If (S,I1,I2,R)∈D2ϵ, then
LV≤−υSR+Mλ1I1+(2β11−β12)I1+(β21−β22)I2+3μ0+μ1+γ2+υ+A+σ212+σ232+σ242−12[μ0−θ2(σ21∨σ22∨σ23∨σ24)](Sθ+1+Iθ+11+Iθ+12+Rθ+1)≤−υSR+D≤−1ϵ+D, | (5.16) |
It then follows from inequality (5.9) that LV≤−1 for all (S,I1,I2,R)∈D2ϵ.
Case 3. If (S,I1,I2,R)∈D3ϵ, then
LV≤−Mλ+Mλ1I1+(2β11−β12)I1+(β21−β22)I2+3μ0+μ1+γ2+υ+A+σ212+σ232+σ242−12[μ0−θ2(σ21∨σ22∨σ23∨σ24)](Sθ+1+Iθ+11+Iθ+12+Rθ+1)≤−Mλ+Mλ1I1+(2β11−β12)I1+E≤−Mλ+Mλ1ε+(2β11−β12)ϵ+E. | (5.17) |
Combining inequalities (5.8) and (5.10) we derive that LV≤−1 for all (S,I1,I2,R)∈D3ϵ.
Case 4. If (S,I1,I2,R)∈D4ϵ, then
LV≤−γ1I1R+D≤−γ1ϵ+D. | (5.18) |
By inequality (5.9), one can derive that LV≤−1 for all (S,I1,I2,R)∈D4ϵ.
Case 5. If (S,I1,I2,R)∈D5ϵ, then
LV≤−γI1I2+D≤−γϵ+D. | (5.19) |
Combining with inequality (5.9), we obtain that LV≤−1 for all (S,I1,I2,R)∈D5ϵ.
Case 6. If (S,I1,I2,R)∈D6ϵ, we have
LV≤−γ2I2R+D≤−γ2ϵ+D. | (5.20) |
According to inequality (5.9), we can deduce that LV≤−1 for all (S,I1,I2,R)∈D6ϵ.
Case 7. If (S,I1,I2,R)∈D7ϵ, we have
LV≤−14[μ0−θ2(σ21∨σ22∨σ23∨σ24)]Sθ+1−14[μ0−θ2(σ21∨σ22∨σ23∨σ24)]Sθ+1−12[μ0−θ2(σ21∨σ22∨σ23∨σ24)](Iθ+11+Iθ+12+Rθ+1)+Mλ1I1+(2β11−β12)I1+(2β21−β22)I2+3μ0+μ1+γ2+υ+A+σ212+σ232+σ242≤−14[μ0−θ2(σ21∨σ22∨σ23∨σ24)]Sθ+1+F≤−14[μ0−θ2(σ21∨σ21∨σ23∨σ24)]1ϵθ+1+F, | (5.21) |
where
F=sup(S,I1,I2,R)∈R4+{−14[μ0−θ2(σ21∨σ22∨σ23∨σ24)]Sθ+1+Mλ1I1+(2β21−β22)I2+(2β21−β22)I2−12[μ0−θ2(σ21∨σ22∨σ23∨σ24)](Iθ+11+Iθ+12+Rθ+1)+3μ0+μ1+γ2+υ+A+σ212+σ232+σ242}. |
Combining with inequality (5.11), we can derive that LV≤−1 for all (S,I1,I2,R)∈D7ϵ.
Case 8. If (S,I1,I2,R)∈D8ϵ, then
LV≤−14[μ0−θ2(σ21∨σ22∨σ23∨σ24)]Iθ+11−14[μ0−θ2(σ21∨σ22∨σ23∨σ24)]Iθ+11−12[μ0−θ2(σ21∨σ22∨σ23∨σ24)](Sθ+1+Iθ+12+Rθ+1)+Mλ1I1+(2β11−β12)I1+(2β21−β22)I2+3μ0+μ1+γ2+υ+A+σ212+σ232+σ242≤−14[μ0−θ2(σ21∨σ22∨σ23∨σ24)]Iθ+11+G≤−14[μ0−θ2(σ21∨σ22∨σ23∨σ24)]1ϵθ+1+G, | (5.22) |
where
G=sup(S,I1,I2,R)∈R4+{−14[μ0−θ2(σ21∨σ22∨σ23∨σ24)]Iθ+11+Mλ1I1+(2β11−β12)I1+(2β21−β22)I2−12[μ0−θ2(σ21∨σ22∨σ23∨σ24)](Sθ+1+Iθ+12+Rθ+1)+3μ0+μ1+γ2+υ+A+σ212+σ232+σ242}. |
By virtue of inequality (5.12), we obtain that LV≤−1 for all (S,I1,I2,R)∈D8ϵ.
Case 9. If (S,I1,I2,R)∈D9ϵ, then
LV≤−14[μ0−θ2(σ21∨σ22∨σ23∨σ24)]Iθ+12−14[μ0−θ2(σ21∨σ22∨σ23∨σ24)]Iθ+12−12[μ0−θ2(σ21∨σ22∨σ23∨σ24)](Sθ+1+Iθ+11+Rθ+1)+Mλ1I1+(2β11−β12)I1+(2β21−β22)I2+3μ0+μ1+γ2+υ+A+σ232+σ232+σ242≤−14[μ0−θ2(σ21∨σ22∨σ23∨σ24)]Iθ+12+H≤−14[μ0−θ2(σ21∨σ22∨σ23∨σ24)]1ϵθ+1+H, | (5.23) |
where
H=sup(S,I1,I2,R)∈R4+{−14[μ0−θ2(σ21∨σ22∨σ23∨σ24)]Iθ+12+Mλ1I1+(2β11−β12)I1+(2β21−β22)I2−12[μ0−θ2(σ21∨σ22∨σ23∨σ24)](Sθ+1+Iθ+11+Rθ+1)+3μ0+μ1+γ2+υ+A+σ212+σ232+σ242}. |
By virtue of inequality (5.13), we can conclude that LV≤−1 for all (S,I1,I2,R)∈D9ϵ.
Case 10. If (S,I1,I2,R)∈D10ϵ, then
LV≤−14[μ0−θ2(σ21∨σ22∨σ23∨σ24)]Rθ+1−14[μ0−θ2(σ21∨σ22∨σ23∨σ24)]Rθ+1−12[μ0−θ2(σ21∨σ22∨σ23∨σ24)](Sθ+1+Iθ+11+Iθ+12)+Mλ1I1+(2β11−β12)I1+(2β21−β22)I2+3μ0+μ1+γ2+υ+A+σ212+σ232+σ242≤−14[μ0−θ2(σ21∨σ22∨σ23∨σ24)]Rθ+1+J≤−14[μ0−θ2(σ21∨σ22∨σ23∨σ24)]1ϵθ+1+J, | (5.24) |
where
J=sup(S,I1,I2,R)∈R4+{−14[μ0−θ2(σ21∨σ22∨σ23∨σ24)]Rθ+1+Mλ1I1+(2β11−β12)I1+(2β21−β22)I2−12[μ0−θ2(σ21∨σ22∨σ23∨σ24)](Sθ+1+Iθ+11+Iθ+12)+3μ0+μ1+γ2+υ+A+σ212+σ232+σ242}. |
Together with inequality (5.14), one can obtain that LV≤−1 for all (S,I1,I2,R)∈D10ϵ.
Combining inequalities (5.15)–(5.24), we finally get a sufficiently small ϵ such that LV≤−1 for all (S,I1,I2,R)∈R4+∖Dϵ. Therefore the condition (C.2) of Lemma 5.1 holds. According to Lemma 5.1, system (2.3) has a unique stationary distribution and it has the ergodic property.
The conclusion is confirmed.
Consequently, we provide an estimation of lower bound for the expectation of infective population in the following theorem.
Theorem 5.3. Let (S(t),I1(t),I2(t),R(t)) be a solution of system (2.3) for any initial value (S(0), I1(0), I2(0), R(0))∈R4+. If ˆRs0>1, then
lim inft→∞⟨I1+I2⟩≥(μ0+γ+γ1+σ222)(ˆRs0−1)λ2a.s., | (5.25) |
where λ2=max{c3α2γμ0+μ1+γ2+a2α1γμ0+γ+γ1,a2α1γ2μ0+γ+γ1}.
Proof. Recall the function V2 in the proof of Theorem 5.2
V2=−lnI1+a1V1+a2α1μ0+γ+γ1I1+c1V1−c2lnI2+c3α2μ0+μ1+γ2I2+a2α1μ0+γ+γ1(S+R). |
Apply Itô's formula to V2, then by inequality (5.2) we have
dV2=LV2dt−(a1+c1)σ1dB1(t)+a2α1σ1μ0+γ+γ1SdB1(t)−σ2dB2(t)+a2α1σ2μ0+γ+γ1I1dB2(t)−c2σ3dB3(t)+c3α2σ3μ0+μ1+γ2I2dB3(t)+a2α1σ4μ0+γ+γ1RdB4(t)≤[−(μ0+γ+γ1+σ222)(ˆRs0−1)+(c3α2γμ0+μ1+γ2+a2α1γ1μ0+γ+γ1)I1+a2α1γ2I2μ0+γ+γ1]dt−(a1+c1)σ1dB1(t)+a2α1σ1Sμ0+γ+γ1dB1(t)−σ2dB2(t)+a2α1σ2μ0+γ+γ1I1dB2(t)−c2σ3dB3(t)+c3α2σ3μ0+μ1+γ2I2dB3(t)+a2α1σ4μ0+γ+γ1RdB4(t). | (5.26) |
Integrate the inequality (5.26) from 0 to t and divide by t on both sides, then we obtain
V2(S(t),I1(t),I2(t),R(t))−V2(S(0),I1(0),I2(0),R(0))t≤−(μ0+γ+γ1+σ222)(ˆRs0−1)+(c3α2γμ0+μ1+γ2+a2α1γ1μ0+γ+γ1)⟨I1⟩+a2α1γ2μ0+γ+γ1⟨I2⟩−ψ(t)t, | (5.27) |
where
ψ(t)=∫t0(a1+c1)σ1dB1(s)−∫t0a2α1σ1μ0+γ+γ1SdB1(s)+∫t0σ2dB2(s)−∫t0a2α1σ2μ0+γ+γ1I1dB2(s)+∫t0c2σ3dB3(s)−∫t0c3α2σ3μ0+μ1+γ2I2dB3(s)−∫t0a2α1σ4μ0+γ+γ1RdB4(s). |
According to the strong law of large numbers [37] and Theorem 4.1, it then follows that
limt→∞ψ(t)t=0a.s. |
Therefore,
lim inft→∞λ2⟨I1+I2⟩≥(μ0+γ+γ1+σ222)(ˆRs0−1)+limt→∞ψ(t)t+lim inft→∞V2(S(t),I1(t),I2(t),R(t))−V2(S(0),I1(0),I2(0),R(0))t=(μ0+γ+γ1+σ222)(ˆRs0−1)>0a.s., |
namely, lim inft→∞⟨I1+I2⟩≥(μ0+γ+γ1+σ222)(ˆRs0−1)λ2a.s.
Remark 2. From the viewpoint of biology, the existence of stationary distribution indicates that all the compartments will be persistent in the time mean sense. As is discussed in [40], the lower bound for the expectation of infective populations in Theorem 5.3 clearly shows that the disease will prevail if ˆRs0>1.
In this section, we provide some numerical simulations for system (2.3) to illustrate the feasibility of our theoretical results. Applying Milstein's higher order method [41] to system (2.3), we obtain the corresponding discretization equation as follows
{Sk+1=Sk+[Λ−(β11−β12I1,kb1+I1,k)SkI1,k1+α1I1,k−(β21−β22I2,kb2+I2,k)SkI2,k1+α2I2,k−(μ0+υ)Sk]Δt+σ1Sk√Δtξk+σ212Sk(ξ2k−1)Δt,I1,k+1=I1,k+[(β11−β12I1,kb1+I1,k)SkI1,k1+α1I1,k+(β21−β22I2,kb2+I2,k)SkI2,k1+α2I2,k−(μ0+γ+γ1)I1,k]Δt+σ2I1,k√Δtηk+σ222I1,k(η2k−1)Δt,I2,k+1=I2,k+[γI1,k−(μ0+μ1+γ2)I2,k]Δt+σ3I2,k√Δtζk+σ232I2,k(ζ2k−1)Δt,Rk+1=Rk+[γ1I1,k+γ2I2,k+υSk−μ0Rk]Δt+σ4Rk√Δtςk+σ242Rk(ς2k−1)Δt, | (6.1) |
where Δt>0, and ξk,ηk,ζk,ςk (k=1,2,...n) are independent Gaussian random variables N(0,1), and σ2i>0 (i=1,2,3,4) are the intensities of white noise.
First of all, take the data of hepatitis B of mainland China as a case study. We have provided the reported incidence rates (1/100, 000) of HBV in mainland China during 2005–2021 in Figure 1(b). Besides, the incidence rates of hepatitis B in 31 provinces are displayed in Figure 2. By comparing Figure 1(c) in [1] and Figure 2 (the data of 2007), one can see that the reported incidence rates of HBV were taken as acute incidence rates therein. It is reasonable because the clinical difference between acute and chronic HBV infections depends on the length of infection, and acute hepatitis B usually refers to the virus infection less than six months. Therefore, inspired by the method in [1], we simulate the reported incidence rates in Figure 1(b) by computing the percentage of acute infection. In the process of model fitting, we first fix the initial values such that the percentage of acute infection is close to the incidence rate 75/100, 000. Then similar to the approaches in Table 3 of [8], we fix a relatively large value of the parameter Λ. The rest of the parameters are estimated and selected partially according to the values in Table 1 of [1]. More specifically, we choose the initial value (S(0),I1(0),I2(0),R(0)) as (5000,0.4,20,10), and the parameters in model (2.3) are taken as
Λ=250,β11=0.0168,β12=0.009,b1=0.5,α1=10,β21=0.0042,β22=0.002,b2=0.02,α2=10,μ0=0.6,υ=0.4,γ=0.2,γ1=0.3,γ2=0.2,μ1=0.65,σ1=0.08,σ2=0.05,σ3=0.05,σ4=0.02. |
Then we compute the percentage of acute HBV infection by Eq (6.1), and compare it with the incidence rates of HBV in mainland China during 2005–2021. The simulation is displayed in Figure 3(a), which shows that stochastic model fits the data well by selecting appropriate parameter values. The long-term solution is given in Figure 3(b), and one can see that the incidence rate of HBV in China will remain around 50–60 per 100,000 in the long term.
More simulations are conducted to illustrate our theoretical results. Firstly, let the initial value (S(0),I1(0),I2(0),R(0))=(0.9,0.4,0.2,0.1), and we choose the parameter values in the stochastic model (2.3) as follows
Λ=0.1,β11=0.25,β12=0.1,b1=0.5,α1=5,β21=0.2,β22=0.1,b2=0.02,α2=5,μ0=0.5,υ=0.4,γ=0.1,γ1=0.4,γ2=0.3,μ1=0.45,σ1=0.2,σ2=0.8,σ3=0.9,σ4=0.1. |
It is easy to compute that
Rs0=0.875<1,min{μ0,μ1}>(σ21∨σ22∨σ23∨σ24)2. |
Thus, the condition of Theorem 4.2 is satisfied and the disease will tend to extinction. The numerical simulation is depicted in Figure 4.
Then we fix the same initial value (S(0),I1(0),I2(0),R(0))=(0.9,0.4,0.2,0.1), and the parameter values in the stochastic model (2.3) are taken as
Λ=9,β11=0.8,β12=0.01,b1=0.5,α1=10,β21=0.8,β22=0.02,b2=0.02,α2=10,μ0=0.6,υ=0.4,γ=0.4,γ1=0.3,γ2=0.2,μ1=0.65,σ1=0.3,σ2=0.4,σ3=0.3,σ4=0.5. |
One can compute that
ˆRs0=1.3353>1. |
Therefore, the condition of Theorem 5.2 is satisfied, and system (2.3) has a stationary distribution. The numerical simulation is shown in Figures 5 and 6, which indicates that the system will be persistent in mean and the disease will prevail.
Since most realistic systems are disturbed by various stochastic factors, in this paper, we have investigated a stochastic HBV transmission model with media coverage and saturated incidence rate. To begin with, we prove the existence and uniqueness of global positive solution of system (2.3). Then we prove that hepatitis B will tend to extinction if Rs0<1 and min{μ0,μ1}>(σ21∨σ22∨σ23∨σ24)2, while system (2.3) has a unique ergodic stationary distribution if ˆRs0>1. According to the expression of Rs0 and ˆRs0, the noise intensities and mass media alert are crucial factors in the disease control. We also obtain an estimation of lower bound of the expectation for the number of infectious cases. Moreover, as a case study, we fit our stochastic model to the data of reported incidence rates of HBV in mainland China, and it is anticipated that the incidence rate of HBV in China will remain around 50–60 per 100,000 for a long time to come.
It should be mentioned that the present HBV transmission model is formulated from a standard SIR epidemic model. This modeling method often divides the total population into several compartments under the assumption that individuals are homogeneous [42]. In other words, our modeling approach is conducted on the single spatial scale, without the consideration of human behavior, contact heterogeneity, and population spatial or social structure [12]. In recent years, multiscale models have been introduced to improve the modeling of disease transmission [43]. Guo et al. [42] developed a heterogeneous graph modeling approach to describe the dynamic process of influenza virus transmission. Since the outbreak of coronavirus disease 2019 (COVID-19) throughout the world, the modeling of SARS-CoV-2 dynamics has further motivated the trends on multiscale modeling [44,45], from the small scale of the virus itself and cells to the large scale of individuals and further up to the collective behavior of populations [46]. For instance, Hayden et al. [47] extended a classical SIR model to a SIRC model by considering the coronavirus concentration in the air (denoted by C). The researchers proposed multi-scale epidemic models by linking the disease transmission to information dissemination dynamics [48] and to the behavior change dynamics [49]. Such multiscale modeling approaches provide important insights into HBV transmission dynamics.
Some interesting research topics deserve further consideration. In stochastic epidemic modeling, Gaussian white noise has been usually adopted to represent environment disturbances and to reflect the fluctuations of disease transmission. Meanwhile different types of noise has also been investigated in the literatures [50,51,52,53,54]. For instance, Lévy noise is introduced to represent some abrupt environmental shocks and disasters [53], and telephone noise (also known as telegraph noise or burst noise) can be regarded as instantaneous transitions between different regimes [54]. We hope to formulate more realistic models considering different types of noise in future research.
The authors are grateful to the anonymous referees for their valuable comments and suggestions which led to the improvement of this paper.
The authors declare that there is no conflict of interest regarding the publication of this manuscript.
[1] |
L. Zou, W. Zhang, S. Ruan, Modeling the transmission dynamics and control of hepatitis B virus in China, J. Theor. Biol., 262 (2010), 330–338. https://doi.org/10.1016/j.jtbi.2009.09.035 doi: 10.1016/j.jtbi.2009.09.035
![]() |
[2] | World health organization, Hepatitis B, Key facts, 2022. Available from: https://www.who.int/news-room/fact-sheets/detail/hepatitis-b |
[3] | Chinese Center for Disease Control and Prevention, Chinese Center for Disease Control and Prevention, 2022. Available from: https://www.chinacdc.cn |
[4] | R. M. Anderson, R. M. May, Infectious Disease of Humans: Dynamics and Control, Oxford University Press, Oxford, 1991. |
[5] |
S. Zhao, Z. Xu, Y. Lu, A mathematical model of hepatitis B virus transmission and its application for vaccination strategy in China, Int. J. Epidemiol., 29 (2000), 744–752. https://doi.org/10.1093/ije/29.4.744 doi: 10.1093/ije/29.4.744
![]() |
[6] |
L. Zou, S. Ruan, W. Zhang, On the sexual transmission dynamics of hepatitis B virus in China, J. Theor. Biol., 369 (2015), 1–12. https://doi.org/10.1016/j.jtbi.2015.01.005 doi: 10.1016/j.jtbi.2015.01.005
![]() |
[7] |
T. Zhang, K. Wang, X. Zhang, Modeling and analyzing the transmission dynamics of HBV epidemic in Xinjiang, China, PLoS ONE, 10 (2015), e0138765. https://doi.org/10.1371/journal.pone.0138765 doi: 10.1371/journal.pone.0138765
![]() |
[8] |
A. Din, Y. Li, Mathematical analysis of a new nonlinear stochastic hepatitis B epidemic model with vaccination effect and a case study, Eur. Phys. J. Plus, 137 (2022), 558. https://doi.org/10.1140/epjp/s13360-022-02748-x doi: 10.1140/epjp/s13360-022-02748-x
![]() |
[9] |
T. Khan, A. Khan, G. Zaman, The extinction and persistence of the stochastic hepatitis B epidemic model, Chaos Soliton Fract., 108 (2018), 123–128. https://doi.org/10.1016/j.chaos.2018.01.036 doi: 10.1016/j.chaos.2018.01.036
![]() |
[10] |
T. Khan, I. H. Jung, G. Zaman, A stochastic model for the transmission dynamics of hepatitis B virus, J. Biol. Dyn., 13 (2019), 328–344. https://doi.org/10.1080/17513758.2019.1600750 doi: 10.1080/17513758.2019.1600750
![]() |
[11] |
L. Liu, D. Jiang, T. Hayat, B. Ahmad, Dynamics of a hepatitis B model with saturated incidence, Acta Math. Sci., 38 (2018), 1731–1750. https://doi.org/10.1016/S0252-9602(18)30842-7 doi: 10.1016/S0252-9602(18)30842-7
![]() |
[12] |
Z. Wang, C. T. Bauch, S. Bhattacharyya, A. d'Onofrio, P. Manfredi, M. Perc, et al., Statistical physics of vaccination, Phys. Rep., 664 (2016), 1–113. https://doi.org/10.1016/j.physrep.2016.10.006 doi: 10.1016/j.physrep.2016.10.006
![]() |
[13] |
S. Wang, M. Hao, Z. Pan, J. Lei, X. Zou, Data-driven multi-scale mathematical modeling of SARS-CoV-2 infection reveals heterogeneity among COVID-19 patients, PLoS Comput. Biol., 17 (2021), e1009587. https://doi.org/10.1371/journal.pcbi.1009587 doi: 10.1371/journal.pcbi.1009587
![]() |
[14] |
F. A. Rihan, H. J. Alsakaji, Analysis of a stochastic HBV infection model with delayed immune response, Math. Biosci. Eng., 18 (2021), 5194–5220. https://doi.org/10.3934/mbe.2021264 doi: 10.3934/mbe.2021264
![]() |
[15] |
T. Luzyanina, G. Bocharov, Stochastic modeling of the impact of random forcing on persistent hepatitis B virus infection, Math. Comput. Simul., 96 (2014), 54–65. https://doi.org/10.1016/j.matcom.2011.10.002 doi: 10.1016/j.matcom.2011.10.002
![]() |
[16] |
X. Wang, Y. Tan, Y. Cai, K. Wang, W. Wang, Dynamics of a stochastic HBV infection model with cell-to-cell transmission and immune response, Math. Biosci. Eng., 18 (2021), 616–642. https://doi.org/10.3934/mbe.2021034 doi: 10.3934/mbe.2021034
![]() |
[17] |
F. Mohajerani, B. Tyukodi, C. J. Schlicksup, J. A. Hadden-Perilla, A. Zlotnick, M. F. Hagan, Multiscale modeling of hepatitis B virus capsid assembly and its dimorphism, ACS Nano, 16 (2022), 13845–13859. https://doi.org/10.1021/acsnano.2c02119 doi: 10.1021/acsnano.2c02119
![]() |
[18] |
Y. Cai, Y. Kang, M. Banerjee, W. Wang, A stochastic SIRS epidemic model with infectious force under intervention strategies, J. Differ. Equations, 259 (2015), 7463–7502. https://doi.org/10.1016/j.jde.2015.08.024 doi: 10.1016/j.jde.2015.08.024
![]() |
[19] |
J. Cui, Y. Sun, H. Zhu, The impact of media on the control of infectious diseases, J. Dyn. Differ. Equations, 20 (2008), 31–53. https://doi.org/10.1007/s10884-007-9075-0 doi: 10.1007/s10884-007-9075-0
![]() |
[20] |
J. Cui, X. Tao, H. Zhu, An SIS infection model incorporating media coverage, Rocky Mountain J. Math., 38 (2008), 1323–1334. https://doi.org/10.1216/RMJ-2008-38-5-1323 doi: 10.1216/RMJ-2008-38-5-1323
![]() |
[21] |
Y. Zhao, L. Zhang, S. Yuan, The effect of media coverage on threshold dynamics for a stochastic SIS epidemic model, Phys. A, 512 (2018), 248–260. https://doi.org/10.1016/j.physa.2018.08.113 doi: 10.1016/j.physa.2018.08.113
![]() |
[22] |
W. Guo, Y. Cai, Q. Zhang, W. Wang, Stochastic persistence and stationary distribution in an SIS epidemic model with media coverage, Phys. A, 492 (2018), 2220–2236. https://doi.org/10.1016/j.physa.2017.11.137 doi: 10.1016/j.physa.2017.11.137
![]() |
[23] |
Y. Zhang, K. Fan, S. Gao, Y. Liu, S. Chen, Ergodic stationary distribution of a stochastic SIRS epidemic model incorporating media coverage and saturated incidence rate, Phys. A, 514 (2019), 671–685. https://doi.org/10.1016/j.physa.2018.09.124 doi: 10.1016/j.physa.2018.09.124
![]() |
[24] |
W. Liu, Q. Zheng, A stochastic sis epidemic model incorporating media coverage in a two patch setting, Appl. Math. Comput., 262 (2015), 160–168. https://doi.org/10.1016/j.amc.2015.04.025 doi: 10.1016/j.amc.2015.04.025
![]() |
[25] |
M. A. Khan, S. Islam, G. Zaman, Media coverage campaign in Hepatitis B transmission model, Appl. Math. Comput., 331 (2018), 378–393. https://doi.org/10.1016/j.amc.2018.03.029 doi: 10.1016/j.amc.2018.03.029
![]() |
[26] |
D. Li, J. Cui, M. Liu, S. Liu, The evolutionary dynamics of stochastic epidemic model with nonlinear incidence rate, Bull. Math. Biol., 77 (2015), 1705–1743. https://doi.org/10.1007/s11538-015-0101-9 doi: 10.1007/s11538-015-0101-9
![]() |
[27] |
Q. Liu, D. Jiang, Stationary distribution and extinction of a stochastic SIR model with nonlinear perturbation, Appl. Math. Lett., 73 (2017), 8–15. https://doi.org/10.1016/j.aml.2017.04.021 doi: 10.1016/j.aml.2017.04.021
![]() |
[28] |
W. Wang, Y. Cai, J. Li, Z. Gui, Periodic behavior in a FIV model with seasonality as well as environment fluctuations, J. Franklin Inst., 354 (2017), 7410–7428. https://doi.org/10.1016/j.jfranklin.2017.08.034 doi: 10.1016/j.jfranklin.2017.08.034
![]() |
[29] |
X. Meng, S. Zhao, T. Feng, T. Zhang, Dynamic of a novel nonlinear stochastic SIS epidemic model with double epidemic hypothesis, J. Math. Anal. Appl., 433 (2016), 227–242. https://doi.org/10.1016/j.jmaa.2015.07.056 doi: 10.1016/j.jmaa.2015.07.056
![]() |
[30] |
Y. Cai, J. Jiao, Z. Gui, Y. Liu, W. Wang, Environmental variability in a stochastic epidemic model, Appl. Math. Comput., 329 (2018), 210–226. https://doi.org/10.1016/j.amc.2018.02.009 doi: 10.1016/j.amc.2018.02.009
![]() |
[31] |
F. A. Rihan, H. J. Alsakaji, Persistence and extinction for stochastic delay differential model of prey predator system with hunting cooperation in predators, Adv. Differ.Equations, 2020 (2020), 124. https://doi.org/10.1186/s13662-020-02579-z doi: 10.1186/s13662-020-02579-z
![]() |
[32] |
V. Capasso, G. Serio, A generalization of the Kermack-McKendrick deterministic epidemic model, Math. Biosci., 42 (1978), 43–61. https://doi.org/10.1016/0025-5564(78)90006-8 doi: 10.1016/0025-5564(78)90006-8
![]() |
[33] |
T. Khan, G. Zaman, Classification of different Hepatitis B infected individuals with saturated incidence rate, SpringerPlus, 5 (2016), 1082. https://doi.org/10.1186/s40064-016-2706-3 doi: 10.1186/s40064-016-2706-3
![]() |
[34] |
F. Zhang, X. Zhang, The threshold of a stochastic avian-human influenza epidemic model with psychological effect, Phys. A, 492 (2018), 485–495. https://doi.org/10.1016/j.physa.2017.10.043 doi: 10.1016/j.physa.2017.10.043
![]() |
[35] |
Z. Shi, X. Zhang, D. Jiang, Dynamics of an avian influenza model with half-saturated incidence, Appl. Math. Comput., 355 (2019), 399–416. https://doi.org/10.1016/j.amc.2019.02.070 doi: 10.1016/j.amc.2019.02.070
![]() |
[36] | X. Mao, C. Yuan, Stochastic Differential Equations with Markovian Switching, Imperial College Press, London, 2006. |
[37] | X. Mao, Stochastic Differential Equations and Applications, Woodhead Publishing, Cambridge, 1997. |
[38] |
Y. Zhao, D. Jiang, The threshold of a stochastic SIS epidemic model with vaccination, Appl. Math. Comput., 243 (2014), 718–727. https://doi.org/10.1016/j.amc.2014.05.124 doi: 10.1016/j.amc.2014.05.124
![]() |
[39] | R. Z. Has'minskii, Stochastic Stability of Differential Equatious, 2nd edition, Springer, Berlin, 2012. |
[40] |
X. Zhang, S. Chang, Q. Shi, H. Huo, Qualitative study of a stochastic SIS epidemic model with vertical transmission, Phys. A, 505 (2018), 805–817. https://doi.org/10.1016/j.physa.2018.04.022 doi: 10.1016/j.physa.2018.04.022
![]() |
[41] |
D. J. Higham, An algorithmic introduction to numerical simulation of stochastic differential equations, SIAM Rev., 43 (2001), 525–546. https://doi.org/10.1137/S0036144500378302 doi: 10.1137/S0036144500378302
![]() |
[42] |
D. Guo, K. C. Li, T. R. Peters, B. M. Snively, K. A. Poehling, X. Zhou, Multi-scale modeling for the transmission of influenza and the evaluation of interventions toward it, Sci. Rep., 5 (2015), 8980. https://doi.org/10.1038/srep08980 doi: 10.1038/srep08980
![]() |
[43] |
V. Reinharz, A. Churkin, S. Lewkiewicz, H. Dahari, D. Barash, A parameter estimation method for multiscale models of hepatitis C virus dynamics, Bull. Math. Biol., 81 (2019), 3675–3721. https://doi.org/10.1007/s11538-019-00644-7 doi: 10.1007/s11538-019-00644-7
![]() |
[44] |
N. Bellomo, D. Burini, N. Outada, Multiscale models of Covid-19 with mutations and variants, Netw. Heterog. Media, 17 (2022), 293–310. https://doi.org/10.3934/nhm.2022008 doi: 10.3934/nhm.2022008
![]() |
[45] |
A. Goyal, D. B. Reeves, J. T. Schiffer, Multi-scale modelling reveals that early super-spreader events are a likely contributor to novel variant predominance, J. R. Soc. Interface, 19 (2022), 20210811. https://doi.org/10.1098/rsif.2021.0811 doi: 10.1098/rsif.2021.0811
![]() |
[46] |
N. Bellomo, D. Burini, N. Outada, Pandemics of mutating virus and society: A multi-scale active particles approach, Phil. Trans. R. Soc. A., 380 (2022), 20210161. https://doi.org/10.1098/rsta.2021.0161 doi: 10.1098/rsta.2021.0161
![]() |
[47] |
M. Hayden, B. Morrow, W. Yang, J. Wang, Quantifying the role of airborne transmission in the spread of COVID-19, Math. Biosci. Eng., 20 (2023), 587–612. https://doi.org/10.3934/mbe.2023027 doi: 10.3934/mbe.2023027
![]() |
[48] |
T. Li, Y. Xiao, Linking the disease transmission to information dissemination dynamics: An insight from a multi-scale model study, J. Theor. Biol., 526 (2021), 110796. https://doi.org/10.1016/j.jtbi.2021.110796 doi: 10.1016/j.jtbi.2021.110796
![]() |
[49] |
B. Tang, W. Zhou, X. Wang, H. Wu, Y. Xiao, Controlling multiple COVID-19 epidemic waves: an insight from a multi-scale model linking the behaviour change dynamics to the disease transmission dynamics, Bull. Math. Biol., 84 (2022), 106. https://doi.org/10.1007/s11538-022-01061-z doi: 10.1007/s11538-022-01061-z
![]() |
[50] |
X. Cao, Z. Jin, N-intertwined SIS epidemic model with Markovian switching, Stoch. Dyn., 19 (2019), 1950031. https://doi.org/10.1142/S021949371950031X doi: 10.1142/S021949371950031X
![]() |
[51] |
Y. Lin, L. Wang, X. Dong, Long-time behavior of a regime-switching SIRS epidemic model with degenerate diffusion, Phys. A, 529 (2019), 121551. https://doi.org/10.1016/j.physa.2019.121551 doi: 10.1016/j.physa.2019.121551
![]() |
[52] |
X. Zhang, H. Peng, Stationary distribution of a stochastic cholera epidemic model with vaccination under regime switching, Appl. Math. Lett., 102 (2020), 106095. https://doi.org/10.1016/j.aml.2019.106095 doi: 10.1016/j.aml.2019.106095
![]() |
[53] |
B. Boukanjime, M. El Fatini, A stochastic Hepatitis B epidemic model driven by Lévy noise, Phys. A, 521 (2019), 796–806. https://doi.org/10.1016/j.physa.2019.01.097 doi: 10.1016/j.physa.2019.01.097
![]() |
[54] |
M. Liu, J. Yu, P. S. Mandal, Dynamics of a stochastic delay competitive model with harvesting and Markovian switching, Appl. Math. Comput., 337 (2018), 335–349. https://doi.org/10.1016/j.amc.2018.03.044 doi: 10.1016/j.amc.2018.03.044
![]() |
![]() |
![]() |
1. | Sayed Murad Ali Shah, Yufeng Nie, Anwarud Din, Abdulwasea Alkhazzan, Stochastic Optimal Control Analysis for HBV Epidemic Model with Vaccination, 2024, 16, 2073-8994, 1306, 10.3390/sym16101306 | |
2. | Liangwei Wang, Fengying Wei, Zhen Jin, Xuerong Mao, Shaojian Cai, Guangmin Chen, Kuicheng Zheng, Jianfeng Xie, HCV transmission model with protection awareness in an SEACTR community, 2025, 24680427, 10.1016/j.idm.2024.12.014 | |
3. | Nabeela Anwar, Iftikhar Ahmad, Hijab Javaid, Adiqa Kausar Kiani, Muhammad Shoaib, Muhammad Asif Zahoor Raja, Dynamical analysis of hepatitis B virus through the stochastic and the deterministic model, 2025, 1025-5842, 1, 10.1080/10255842.2025.2470798 |