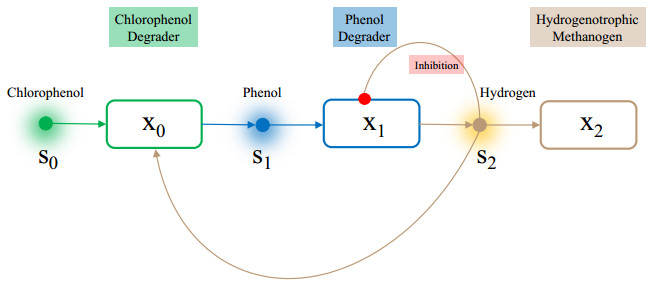
According to the mechanism of drug inhibition of hepatitis B virus and the analysis of clinical data, it is found that random factors in long-term treatment produced uncertainty and resistance to hepatitis B virus infection rate, a model of hepatitis B virus with random interference infection rate is established. By constructing Lyapunov function and using Ito's formula, it is proved that the stochastic hepatitis B model has a unique global positive solution. The sufficient conditions for the asymptotic behavior of solution are given. The relationship between noise intensity and oscillation amplitude is obtained. The effects of noise intensity on the asymptotic behavior of the model and antiviral therapy are simulated, and the conclusion of the theorem is verified. An interesting phenomenon is also found that with the increase of noise intensity, the number of drug-resistant viruses will decrease, which will affect the accuracy of a single test of HBV DNA. Therefore, it is suggested to increase the frequency and interval of tests.
Citation: Dong-Me Li, Bing Chai, Qi Wang. A model of hepatitis B virus with random interference infection rate[J]. Mathematical Biosciences and Engineering, 2021, 18(6): 8257-8297. doi: 10.3934/mbe.2021410
[1] | Jinhu Xu, Yicang Zhou . Bifurcation analysis of HIV-1 infection model with cell-to-cell transmission and immune response delay. Mathematical Biosciences and Engineering, 2016, 13(2): 343-367. doi: 10.3934/mbe.2015006 |
[2] | Amer Hassan Albargi, Miled El Hajji . Mathematical analysis of a two-tiered microbial food-web model for the anaerobic digestion process. Mathematical Biosciences and Engineering, 2023, 20(4): 6591-6611. doi: 10.3934/mbe.2023283 |
[3] | Boumediene Benyahia, Tewfik Sari . Effect of a new variable integration on steady states of a two-step Anaerobic Digestion Model. Mathematical Biosciences and Engineering, 2020, 17(5): 5504-5533. doi: 10.3934/mbe.2020296 |
[4] | Xiaomeng Ma, Zhanbing Bai, Sujing Sun . Stability and bifurcation control for a fractional-order chemostat model with time delays and incommensurate orders. Mathematical Biosciences and Engineering, 2023, 20(1): 437-455. doi: 10.3934/mbe.2023020 |
[5] | Ranjit Kumar Upadhyay, Swati Mishra, Yueping Dong, Yasuhiro Takeuchi . Exploring the dynamics of a tritrophic food chain model with multiple gestation periods. Mathematical Biosciences and Engineering, 2019, 16(5): 4660-4691. doi: 10.3934/mbe.2019234 |
[6] | Zhenliang Zhu, Yuming Chen, Zhong Li, Fengde Chen . Dynamic behaviors of a Leslie-Gower model with strong Allee effect and fear effect in prey. Mathematical Biosciences and Engineering, 2023, 20(6): 10977-10999. doi: 10.3934/mbe.2023486 |
[7] | Christian Cortés García, Jasmidt Vera Cuenca . Impact of alternative food on predator diet in a Leslie-Gower model with prey refuge and Holling Ⅱ functional response. Mathematical Biosciences and Engineering, 2023, 20(8): 13681-13703. doi: 10.3934/mbe.2023610 |
[8] | A. Aldurayhim, A. Elsonbaty, A. A. Elsadany . Dynamics of diffusive modified Previte-Hoffman food web model. Mathematical Biosciences and Engineering, 2020, 17(4): 4225-4256. doi: 10.3934/mbe.2020234 |
[9] | Mengyun Xing, Mengxin He, Zhong Li . Dynamics of a modified Leslie-Gower predator-prey model with double Allee effects. Mathematical Biosciences and Engineering, 2024, 21(1): 792-831. doi: 10.3934/mbe.2024034 |
[10] | Gunog Seo, Mark Kot . The dynamics of a simple Laissez-Faire model with two predators. Mathematical Biosciences and Engineering, 2009, 6(1): 145-172. doi: 10.3934/mbe.2009.6.145 |
According to the mechanism of drug inhibition of hepatitis B virus and the analysis of clinical data, it is found that random factors in long-term treatment produced uncertainty and resistance to hepatitis B virus infection rate, a model of hepatitis B virus with random interference infection rate is established. By constructing Lyapunov function and using Ito's formula, it is proved that the stochastic hepatitis B model has a unique global positive solution. The sufficient conditions for the asymptotic behavior of solution are given. The relationship between noise intensity and oscillation amplitude is obtained. The effects of noise intensity on the asymptotic behavior of the model and antiviral therapy are simulated, and the conclusion of the theorem is verified. An interesting phenomenon is also found that with the increase of noise intensity, the number of drug-resistant viruses will decrease, which will affect the accuracy of a single test of HBV DNA. Therefore, it is suggested to increase the frequency and interval of tests.
A mathematical model of the complete anaerobic mineralisation of a generic monochlorophenol isomer (C6H4ClOH) by a canonical food-web has recently been described [1]. The system comprises three microbial species (chlorophenol degrader, phenol degrader, hydrogenotrophic methanogen). Their interactions are summarised as follows:
● Reductive dehalogenation of chlorophenol in the presence of hydrogen by the chlorophenol degrader producing phenol [2];
● Phenol is mineralised by a phenol degrader to acetate and hydrogen via the benzoyl-CoA pathway or a caproate intermediary [3];
● Anaerobic acetogenic (acetate producing) processes are known to be endergonic (the reaction results in a net loss of energy to the system). The production of hydrogen can lead to thermodynamic constraints, or inhibition, if its partial pressure is high enough. In other words, the reaction becomes decreasingly exergonic as more hydrogen is produced until it ceases to be thermodynamically favourable [4,5]. Hydrogen scavengers such as the hydrogenotrophic methanogen form a syntrophic partnership with the phenol degrader by maintaining the hydrogen partial pressure at concentrations low enough for the mineralisation reaction to proceed;
● Given that the chlorophenol degrader may also act as a syntrophic partner with the phenol degrader, a competitive interaction between the two hydrogen utilisers occurs. These positive and negative feedback loops reframe the ecological network from a simple food-chain to a more complex food-web that allows for the possibility of periodicity. This additionally allows for the case where the system reduces to a self-sustaining two-species network in which the chlorophenol degrader acts as the syntrophic partner to the phenol degrader.
A diagrammatic representation of the food-web is presented in Figure 1. The model is a simplified representation of the system at the population level, ignoring metabolic intermediates and 'dead-end' products such as methane, which do not contribute to the process dynamics. Acetoclastic methanogenesis, the conversion of acetate to methane, is also omitted from the model.
Hydrogen, however, has been shown to play an important role in stability of anaerobic microbial communities through the effects of inhibition and competition [6,7,8]. Given that external hydrogen addition will maintain the methanogen population (no washout when the methanogen growth rate is greater than the combined dilution and decay/maintenance rates) under a wider operating parameter regime (chlorophenol inflow and dilution rate) [1], a global analysis of the model can provide deeper insights into the ecological role of hydrogen through its association with community stability and criticality of the Hopf bifurcation.
Here, we focus on the mathematical analysis of the model, extending the work reported in the literature. For example, an analytical approach was taken to characterise the existence and stability of the system equilibria with and without inclusion of a microbial decay constant using a general representation of the species growth functions [9]. With no decay and without considering production of hydrogen by the phenol degrader, (an unusual constraint that appears to make the system entirely theoretical with extraneous addition of hydrogen necessary for complete chlorophenol mineralisation) local stability and the conditions giving rise to asymptotic coexistence of all three species have been shown analytically, with the possibility of periodic orbits not excluded [10]. Numerical analysis has suggested the presence of a Hopf bifurcation of the positive steady-state, with the concentration of influent chlorophenol as the bifurcating parameter [9].
In this work, we extend the analysis of the model to provide a proof of the existence and uniqueness of system equilibria and their stability for the most general case where up to three external inputs (chlorophenol, phenol, hydrogen) may be included. The procedure we use allows us to identify sufficient conditions for the emergence of a Hopf bifurcation as the inflow concentration parameters vary. We are also able to prove that there is a range of parameters for which the model is uniformly persistent, i.e., all populations avoid extinction, a new result for the system. Two-parameter bifurcation diagrams reveal rich dynamics that include Bogdanov-Takens and Bautin bifurcations and, hence, homoclinic and saddle-node of limit cycle bifurcations.
We first present concisely the original given by [1] using the identical scaling:
{x′0=−αx0+μ0(s0,s2)x0−kAx0,x′1=−αx1+μ1(s1,s2)x1−kBx1,x′2=−αx2+μ2(s2)x2−kCx2,s′0=α(uf−s0)−μ0(s0,s2)x0,s′1=α(ug−s1)+ω0μ0(s0,s2)x0−μ1(s1,s2)x1,s′2=α(uh−s2)−ω2μ0(s0,s2)x0+ω1μ1(s1,s2)x1−μ2(s2)x2, | (2.1) |
with
α=Dkm,chYch,uf=Sch,inKS,ch,ug=Sph,inKS,ph,uh=SH2,inKS,H2,ω0=KS,chKS,ph224208(1−Ych),ω1=KS,phKS,H232224(1−Yph),ω2=16208KS,chKS,H2,ϕ1=km,phYphkm,chYch,ϕ2=km,H2YH2km,chYch,KP=KS,H2,cKS,H2,KI=KS,H2KI,H2,kA=kdec,chkm,chYch,kB=kdec,phkm,chYch,kC=kdec,H2km,chYch. |
μ0(s0,s2)=s01+s0s2KP+s2,μ1(s1,s2)=ϕ1s11+s111+KIs2,μ2(s2)=ϕ2s21+s2, | (2.2) |
where α is the dilution rate, uf,ug,uh are the chlorophenol, phenol, and hydrogen inflow concentrations, respectively, and kA,kB,kC are the decay (or maintenance) terms. These are scaled to be dimensionless, as are the other parameters using the scaling provided by [1]. Briefly, km,⋅ are the specific growth rates, KS,⋅ are the half-saturation coefficients, Y⋅ are the substrate yield coefficients, KI,H2 is the kinetic inhibition constant of hydrogen on the phenol degrader, and kdec,⋅ are the unscaled decay terms. The numeric values indicate the stoichiometric coefficients given in terms of units of Chemical Oxygen Demand rather than molarity, as is common for environmental engineering models. The μn(⋅),n=0,1,2 are the species growth functions described by double Monod, Monod with product inhibition, and Monod kinetics, respectively. Subscripts ⋅ch, ⋅ph, ⋅H2 relate to the chlorophenol degrader, phenol degrader, and methanogen, respectively.
For the numerical bifurcation analysis given in Section 4.4, we consider the same parameter values as provided in the original work in [1], as shown in Table 1.
Parameters | Value |
ω0 | 0.1854 |
ω1 | 1656.69 |
ω2 | 163.08 |
ϕ1 | 1.8875 |
ϕ2 | 3.8113 |
KP | 0.04 |
KI | 7.1429 |
We are able to obtain many theoretical results assuming general forms of the growth functions provided we assume the death rates of the microbial populations are insignificant compared to the dilution rate. We thus consider the following system that is identical to system (2.1), except that we assume ki=0,
i∈{A,B,C}:
{x′0=−αx0+μ0(s0,s2)x0,x′1=−αx1+μ1(s1,s2)x1,x′2=−αx2+μ2(s2)x2,s′0=α(uf−s0)−μ0(s0,s2)x0,s′1=α(ug−s1)+ω0μ0(s0,s2)x0−μ1(s1,s2)x1,s′2=α(uh−s2)−ω2μ0(s0,s2)x0+ω1μ1(s1,s2)x1−μ2(s2)x2, | (3.1) |
xi(0)≥0,si(0)≥0,i∈{0,1,2}. |
We assume that μ0(s0,s2), μ1(s1,s2), μ2(s2) are C1 functions that satisfy the following general conditions:
(H1) For all s0≥0 and s2≥0, μ0(0,s2)=0, μ0(s0,0)=0. As a consequence, ∂s0μ0(s0,0)=0, ∂s2μ0(0,s2)=0. Thus we assume that the chlorophenol degrader cannot grow in the absence of either chlorophenol or hydrogen;
(H2) For all s0>0 and s2>0, ∂s0μ0(s0,s2)>0, ∂s2μ0(s0,s2)>0. Thus we assume that the chlorophenol degrader grows on both chlorophenol and hydrogen;
(H3) For all s2≥0, μ1(0,s2)=0. As a consequence ∂s2μ1(0,s2)=0. Thus we assume that the phenol degrader cannot grow in the absence of phenol;
(H4) For all s1>0 and s2>0, ∂s1μ1(s1,s2)>0, ∂s2μ1(s1,s2)<0. Thus we assume that the supply of phenol results in growth of the phenol degrader, and that hydrogen inhibits its growth;
(H5) μ2(0)=0 and μ′2(s2)>0 for all s2>0. Thus we assume that the methanogen cannot grow without the presence of hydrogen, and that increasing the supply of hydrogen results in faster growth of the methanogen.
We use the prototypes μ0, μ1, and μ2 defined in Eq (2.2), which satisfy these conditions, when we are unable to prove results in general, and when providing numerical simulations or bifurcation diagrams.
The proof of the following lemma, needed for well-posedness of system (3.7), is standard, and hence omitted.
Lemma 3.1. All solutions of system (3.1) with positive initial conditions remain positive and bounded for all positive times and if xi(0)=0, i∈{0,1,2}, then xi(t)=0 for all t≥0.
We can further simplify analysis of system (3.1) using the following lemma.
Lemma 3.2. The omega limit set of any solution of system (3.1) is contained in a positively invariant attracting set Ω⊂R6 defined as
Ω={(x0,x1,x2,s0,s1,s2)∈R6:xi,si≥0,i=0,1,2;x0+s0=uf,x1+ω0s0+s1=ω0uf+ug,ω2x0+x2+ω0ω1s0+ω1s1+s2=ω0ω1uf+ω1ug+uh}. | (3.2) |
Proof. By adding the first and the fourth equations of system (3.1), we obtain
x′0+s′0=−α(x0+s0−uf), |
hence
(x0+s0−uf)′=−α(x0+s0−uf), |
which implies that
x0(t)+s0(t)=uf+(x0(0)+s0(0)−uf)e−αt. | (3.3) |
Similarly, we obtain
x1(t)+ω0s0(t)+s1(t)=ω0uf+ug+(x1(0)+ω0s0(0)+s1(0)−ω0uf−ug)e−αt, | (3.4) |
and
ω2x0(t)+x2(t)+ω0ω1s0(t)+ω1s1(t)+s2(t)=ω0ω1uf+ω1ug+uh+(ω0x0(0)+x2(0)+ω0ω1s0(0)+ω1s1(0)+s2(0)−ω0ω1uf−ω1ug−uh)e−αt. | (3.5) |
By taking the limit as t→∞ in Eqs (3.3)–(3.5) we obtain
limt→∞(x0(t)+s0(t))=uf,limt→∞(x1(t)+ω0s0(t)+s1(t))=ω0uf+ug,limt→∞(ω2x0(t)+x2(t)+ω0ω1s0(t)+ω1s1(t)+s2(t))=ω0ω1uf+ω1ug+uh, |
which yields the desired result.
The three equalities in the definition of Ω given in system (3.2) are called "conservation principles". Using them, we can compute s0, s1, and s2 as functions of x0, x1, x2:
s0=−x0+uf,s1=ω0x0−x1+ug,s2=−ω2x0+ω1x1−x2+uh. | (3.6) |
Now, we can reduce the analysis of the original system (3.1) to the analysis of the following three-dimensional limiting system on the invariant set Ω:
{x′0=−αx0+μ0(−x0+uf,−ω2x0+ω1x1−x2+uh)x0,x′1=−αx1+μ1(ω0x0−x1+ug,−ω2x0+ω1x1−x2+uh)x1,x′2=−αx2+μ2(−ω2x0+ω1x1−x2+uh)x2. | (3.7) |
From now on, we will study the reduced system (3.7). We begin by analysing all the possible equilibria.
The equilibria are found by setting the right hand sides of Eq (3.7) equal to zero. Below, we list all the possibilities obtained this way. Since Eq (3.6) give a one-to-one correspondence of the equilibria of system (3.7) with the equilibria of system (3.1), we also list the corresponding steady states (x0,x1,x2,s0,s1,s2) of the six-dimensional system in each case. Each equilibrium is preceded by a subscript, indicating which population of microorganisms survives. That is, subscript (100) implies that x0>0, x1=x2=0, (110) that x0, x1>0, x2=0, and so on.
Types of equilibria of system (3.7):
● Zero equilibrium (000)E=(0,0,0). The corresponding equilibrium (000)E in the six-dimensional system:
(000)E=(0,0,0,uf,ug,uh). |
In this case, all the populations die out, hence the only source for the substrates comes from the inflows uf, ug and uh.
● Boundary equilibria:
- (100)E=((100)x0,0,0), where x0=(100)x0>0 is a solution (if it exists) of
μ0(−x0+uf,−ω2x0+uh)=α. |
The corresponding equilibrium (100)E in the six-dimensional system:
(100)E=((100)x0,0,0,−(100)x0+uf,ω0(100)x0+ug,−ω2(100)x0+uh). |
In this case, the only microorganism surviving is the chlorophenol degrader. It consumes the chlorophenol, hence the value of s0 is given as the balance between this consumption, and the supply inflow uf. Since x0 produces phenol, this value is added to ug to obtain the total phenol amount s1. Since x0 consumes hydrogen as well, the value ω2(100)x0 is subtracted from s2. This steady state is not desirable, because of the phenol build-up in the system.
- (010)E=(0,(010)x1,0), where x1=(010)x1>0 is a solution (if it exists) of
μ1(−x1+ug,ω1x1+uh)=α. |
The corresponding equilibrium (010)E in the six-dimensional system:
(010)E=(0,(010)x1,0,uf,−(010)x1+ug,ω1(010)x1+uh). |
In this case, only the phenol degrader survives, and hence the value of s1 at the equilibrium is equal to the balance between its consumption and inflow ug. Chlorophenol is not being consumed, hence its total amount equals the inflow concentration uf. Hydrogen is being produced by the phenol degrader, and also its value is increased by the inflow uh.
- (001)E=(0,0,(001)x2), where x2=(001)x2>0 is a solution (if it exists) of
μ2(−x2+uh)=α. |
The corresponding equilibrium (001)E in the six-dimensional system:
(001)E=(0,0,(001)x2,uf,ug,−(001)x2+uh). |
Here, only the methanogen is present, hence the values of chlorophenol and phenol are equal to the the inflow concentrations uf and ug, respectively.
- (101)E=((101)x0,0,−ω2(101)x0+uh−μ−12(α)),
where x0=(101)x0>0 is a solution (if it exists) of
μ0(−x0+uf,μ−12(α))=α. |
The corresponding equilibrium (101)E in the six-dimensional system:
(101)E=((101)x0,0,−ω2(101)x0+uh−μ−12(α),−(101)x0+uf,ω0(101)x0+ug,μ(−1)2(α)). |
In this case, both the chlorophenol degrader and the methanogen are present. The lack of the phenol degrader results in phenol build-up. We can also observe competition for hydrogen between the chlorophenol degrader and the methanogen.
- (011)E=(0,(011)x1,ω1(011)x1+uh−μ−12(α)),
where x1=(011)x1>0 is a solution (if it exists) of
μ1(−x1+ug,μ−12(α))=α. |
The corresponding equilibrium (011)E in the six-dimensional system:
(011)E=(0,(011)x1,ω1(011)x1+uh−μ−12(α),uf,−(011)x1+ug,μ−12(α)). |
This steady state represents a two-tiered food chain, with the phenol degrader and methanogen present. Hydrogen has an inhibiting effect on the phenol degrader.
- (110)E=((110)x0,(110)x1,0), where x0=(110)x0>0 and x1=(110)x1>0 are solutions of
μ0(−x0+uf,−ω2x0+ω1x1+uh)=α,μ1(ω0x0−x1+ug,−ω2x0+ω1x1+uh)=α. |
The corresponding equilibrium (110)E in the six-dimensional system:
(110)E=((110)x0,(110)x1,0,−(110)x0+uf,ω0(110)x0−(110)x1+ug,−ω2(110)x0+ω1(110)x1+uh). |
In this case, both the chlorophenol and phenol degraders are present, however the methanogen is washed out. Thus, full mineralisation to methane is not possible. Hence, the hydrogen accumulates to some theoretical maximum, balanced such that the the inhibitory effect on the phenol degrader does not induce washout, whilst providing enough hydrogen for the chlorophenol degrader activity.
● Positive (interior) equilibrium (111)E=(∗x0,∗x1,∗x2), where x0=∗x0>0, x1=∗x1>0, and x2=∗x2>0 are solutions of
μ0(−x0+uf,−ω2x0+ω1x1−x2+uh)=α,μ1(ω0x0−x1+ug,−ω2x0+ω1x1−x2+uh)=α,μ2(−ω2x0+ω1x1−x2+uh)=α. |
The corresponding equilibrium (111)E in the six-dimensional system:
(111)E=(∗x0,∗x1,∗x2,−∗x0+uf,ω0∗x0−∗x1+ug,μ(−1)2(α)). |
Here, all species are present, and thus we observe full chlorophenol mineralisation. For this reason, asymptotic stability of this equilibrium is the desired operational state.
The issues regarding the conditions on existence, uniqueness, and local stability of equilibria of the system have been fully answered in two recent preprints [11,12]. The system considered in these works is more general, as it allows non-zero death rates. It is related to our system (3.1) by a linear change of variables, that is, by replacing x0 by ω0ω1x0, s0 by ω0ω1s0, x1 by ω1s1, s1 by ω1s1, and replacing ω0, ω1, and ω2 by a single parameter ω=ω2ω0ω1. It follows that all results described in [11,12] apply to system (3.1) under this rescaling. Specifically, it has been shown that all equilibria, except (110)E, are unique, when they exist. Also, system (3.1) can have at most two equilibria of the form (110)E. Note that, following [1], different steady-state notation is adopted in [11,12], i.e., (000)E=SS1, (001)E=SS2, (100)E=SS3, (110)E=SS4, (101)E=SS5, (111)E=SS6, (010)E=SS7, (011)E=SS8. For more details, we refer readers to the aforementioned preprints, as this paper is focused more on possible bifurcations and persistence analysis of system (3.7).
We begin by ruling out the possibility of having a periodic orbit in one of the invariant faces of Ω. This can be done by using general forms of prototypes μ0, μ1 and μ2.
Lemma 4.1. There are no periodic orbits on the invariant faces of the set Ω of system (3.7).
Proof. First, consider system (3.7) on the part of Ω with x0=0, i.e..
{x′1=(−α+μ1(−x1+ug,ω1x1−x2+uh))x1,x′2=(−α+μ2(ω1x1−x2+uh))x2. | (4.1) |
The domain for system (4.1) is given by the following set Ω12:
Ω12={(x1,x2)∈R2:0≤x1≤ug,0≤x2≤uh+ω1x1}. |
Notice that no periodic orbit can intersect the axes x1=0 or x2=0 since they are invariant. Now, let us define an auxiliary function
φ0(x1,x2)=1x1x2,(x1,x2)∈Ω12∖({x1=0}∪{x2=0}). |
Then
∇⋅(φ0(−α+μ1)x1,φ0(−α+μ2)x2)=−∂s1μ1+ω1∂s2μ1x2−μ′2x1<0 |
for all (x1,x2) in the domain of φ0, by Conditions (H4) and (H5). Thus, by the Dulac's Criterion [13], there are no periodic orbits in the x1x2 face.
Now, consider system (3.7) on the part of Ω with x1=0, i.e..
{x′0=(−α+μ0(−x0+uf,−ω2x0−x2+uh))x0,x′2=(−α+μ2(−ω2x0−x2+uh))x2, |
defined on Ω02 given by
Ω02={(x0,x2)∈R2:0≤x0≤min{uf,uh−x2ω2},0≤x2≤−ω2x0+uh}. |
For auxiliary function
φ1(x0,x2)=1x0x2,(x0,x2)∈Ω02∖({x0=0}∪{x2=0}) |
we have, using Conditions (H2) and (H5),
∇⋅(φ1(−α+μ1)x0,φ1(−α+μ2)x2)=−∂s0μ0−ω2∂s2μ0x2−μ′2x0<0. |
This, together with the fact that the axes x0=0, x2=0 are invariant, shows that there are no periodic orbits in the x0x2 face.
Finally, consider system (3.7) on the part of Ω with x2=0, i.e..
{x′0=(−α+μ0(−x0+uf,−ω2x0+ω1x1+uh))x0,x′1=(−α+μ1(ω0x0−x1+ug,−ω2x0+ω1x1+uh))x1. |
defined on Ω01 given by
Ω01={(x0,x1)∈R2:0≤x0≤uf,max{0,ω2x0−uhω1}≤x1≤ω0x0+ug}. |
Analogously to the previous cases, we define
φ2(x0,x1)=1x0x1,(x0,x1)∈Ω01∖({x0=0}∪{x1=0}) |
and note that by Conditions (H2) and (H4)
∇⋅(φ(−α+μ0)x0,φ(−α+μ1)x1)=−∂s0μ0−ω2∂s2μ0x1+−∂s1μ1+ω1∂s2μ1x0<0. |
Since the axes x0=0, x1=0 are invariant, by Dulac's Criterion, there are no periodic orbits in the x0x1 face.
In this work we are especially interested in developing a more systematic approach to studying the Hopf bifurcation of the interior equilibrium. That Hopf bifurcation that occurs in this model was previously observed numerically [9]. The occurrence of a stable periodic orbit in system (3.7) represents a situation in which the concentrations of all three populations of microorganisms oscillate indefinitely, and as a consequence, the substrate concentrations fluctuate.
The characteristic polynomial of the Jacobian corresponding to the interior equilibrium is given by
λ3+a2λ2+a1λ+a0=0, |
where
a2=−∗x0(−∂μ0∂s0−ω2∂μ0∂s2)−∗x1(−∂μ1∂s1+ω1∂μ1∂s2)+∗x2μ′2, | (4.2) |
a1=∗x1∂μ1∂s1(∗x0∂μ0∂s0−(ω0ω1−ω2)∗x0∂μ0∂s2+∗x2μ′2)+∗x0∂μ0∂s0(−ω1∗x1∂μ1∂s2+∗x2μ′2), | (4.3) |
a0=∗x0∗x1∗x2∂μ0∂s0∂μ1∂s1μ′2, | (4.4) |
and the coefficients a2, a1, and a0 depend on the parameters uf, ug, uh, and α. The coefficients a2 and a0 are sign-definite (they are both positive by Conditions (H2), (H4) and (H5)), but a1 might possibly change sign. Notice first that since the polynomial is of order three, a real eigenvalue always exists. By the Routh-Hurwitz criterion, the above polynomial has a pair of purely imaginary eigenvalues if and only if
a2a1=a0(which implies a1>0). |
In that case, we also have
λ3+a2λ2+a1λ+a0=λ3+a2λ2+a1λ+a2a1=(λ+a2)(λ2+a1). |
Hence, the eigenvalues are λ1=−a2 and λ2,3=±√a1i. In the following theorem, we prove that under certain nondegeneracy conditions, system (3.7) exhibits a Hopf bifurcation when the eigenvalues λ1,2 cross the imaginary axis. We explore the possibility of a Hopf bifurcation in either of the three parameters uf, ug, or uh. Additionally, the effect of α on possible bifurcations in the system is explored numerically, by constructing bifurcation diagrams in Subsection 4.4. To derive our results, we use specific forms of the growth functions (2.2) and the values of the parameters given in Table 1.
Theorem 4.1. Consider system (3.7) with the prototypes given by Eq (2.2) and with the values of parameters from Table 1 fixed. Define f:R4+→R as f(uf,ug,uh,α)=a2a1−a0 where a0, a1, a2 are given in Eqs (4.2)–(4.4). Furthermore, assume that there exists a point (uf,ug,uh,α)=(u∗f,u∗g,u∗h,α∗) such that f(u∗f,u∗g,u∗h,α∗)=0. For fixed ug=u∗g, uh=u∗h, and α=α∗, denote the resulting third-order polynomial f(uf,u∗g,u∗h,α∗) as
f(uf,u∗g,u∗h,α∗)=b3u3f+b2u2f+b1uf+b0, |
for fixed uf=u∗f, uh=u∗h, and α=α∗, denote the resulting third-order polynomial f(u∗f,ug,u∗h,α∗) as
f(u∗f,ug,u∗h,α∗)=c3u3g+c2u2g+c1ug+c0, |
and for fixed uf=u∗f, ug=u∗g, and α=α∗, denote the second-order polynomial f(u∗f,u∗g,uh,α∗) as
f(u∗f,u∗g,uh,α∗)=d2u2h+d1uh+d0. |
There exists a δ>0 such that, if ‖(uf,ug,uh,α)−(u∗f,u∗g,u∗h,α∗)‖<δ and in addition:
I.
u∗f≠−b2±√b22−3b1b33b3, |
then there is a Hopf bifurcation in uf when uf=u∗f, or
II.
u∗g≠−c2±√c22−3c1c33c3, |
then there is a Hopf bifurcation in ug when ug=u∗g, or
III.
d21−4d2d0≠0, |
then there is a Hopf bifurcation in uh when uh=u∗h.
Proof. Since eigenvalues are continuous functions of the parameters, we can see that if there is some (uf,ug,uh,α)=(u∗f,u∗g,u∗h,α∗) such that f(u∗f,u∗g,u∗h,α∗)=0 (i.e., a2a1=a0), then if we denote by λ1 the real eigenvalue (that always exists), there is some δ>0 such that for ‖(uf,ug,uh,α)−(u∗f,u∗g,u∗h,α∗)‖<δ, we always have λ1<0. By Lemma 5.1, Section 5.2 in [13], this implies the existence of a parameter-dependent, smooth, attracting, two dimensional, center manifold Wc(uf,ug,uh,α). In the following analysis, we consider only parameters that are in the δ-neighbourhood of (uf,ug,uh,α), in order to ensure that the real eigenvalue λ1 is negative.
By the Routh-Hurwitz criterion (since when (111)E exists, a0 and a2 are always positive), a Hopf bifurcation occurs when the function f changes sign as a parameter varies. This ensures that the real part of a pair of complex eigenvalues with nonzero imaginary part passes through 0 and changes sign. This is related to the transversality condition: the derivative of the real part of the eigenvalue with respect to the bifurcation parameter evaluated at the critical value when the real parts are zero is non-zero. We check this condition for specific forms of the functions μ0, μ1, and μ2, given in Eq (2.2). We begin our analysis by choosing uf as the free parameter. We have
f(uf,u∗g,u∗h,α∗)=b3u3f+b2u2f+b1uf+b0, |
for some constants bi, i∈{0,1,2,3}, and by the hypothesis, for uf=u∗f, we have f(u∗f,u∗g,u∗h,α∗)=0. We want to find conditions on the coefficients of f that guarantee that its derivative with respect to uf is not equal to zero when uf=u∗f. We have
∂uff(uf,u∗g,u∗h,α∗)=3b3u2f+2b2uf+b1. |
Thus ∂uff(uf,u∗g,u∗h,α∗)≠0 if and only if
u∗f≠−b2±√b22−3b1b33b3. |
We have thus obtained a sufficient condition for a Hopf bifurcation.
If we choose ug as the free parameter, we have
f(u∗f,ug,u∗h,α∗)=c3u3g+c2u2g+c1ug+c0, |
for some constants ci, i∈{0,1,2,3}, and with the assumption that f(u∗f,u∗g,u∗h,α∗)=0, by a similar analysis as in the previous case, we obtain an analogous sufficient condition for a Hopf bifurcation in ug.
Finally, if we choose uh as the free parameter, we have
f(u∗f,u∗g,uh,α∗)=d2u2h+d1uh+d0, | (4.5) |
for some constants di, i∈{0,1,2}. Once again, we assume that f(u∗f,u∗g,u∗h,α∗)=0, that is
u∗h=−d1±√d21−4d2d02d2. |
Here, ∂uhf(u∗f,u∗g,u∗h,α∗)≠0 if and only if d21−4d2d0≠0.
We now illustrate the theoretical results with the numerical simulations. To approximate values of the equilibria, we used Maple software [14], rounding all the values to 6 significant digits. For the choice of parameters given in Table 1 and
α=0.01,uf=0.5,ug=0.0006, |
it follows that the only zero of Eq (4.5) occurs for uh=0.102520. In Figure 2, we plot phase space for uh=0.05 (just before the Hopf bifurcation) using the ode15s solver from Matlab [15]. For this set of parameters, we have the following approximate values of the equilibria
(000)E=(0,0,0)(unstable),(100)E=(0.000299015,0,0)(stable),(001)E=(0,0,0.0473693)(unstable),(110)E(1)=(0.0545964,0.00534486,0)(unstable),(110)E(2)=(0.489465,0.0487183,0)(unstable),(111)E=(0.306611,0.0520205,36.2277)(unstable), |
We can see that there exists a stable periodic orbit in the system, but depending on the initial conditions, the solution might also converge to the boundary equilibrium (100)E. Thus in this case we observe bistability.
We now repeat the simulations for uh=0.3 (after the predicted Hopf bifurcation), presented in Figure 3. With all the other parameters set to the same values as in the previous case, we have the following equilibria
(000)E=(0,0,0)(unstable),(100)E=(0.00183202,0,0)(stable),(001)E=(0,0,0.297369)(unstable),(110)E(1)=(0.0528596,0.00502299,0)(unstable),(110)E(2)=(0.489467,0.0485697,0)(unstable),(111)E=(0.306611,0.0520205,36.4777)(stable). |
We can see that the Hopf bifurcation occurs between uh=0.05 and uh=0.3. The stable periodic orbit is no longer present, and the interior equilibrium is stable. Once again, the boundary equilibrium (100)E is stable, and thus we observe bistability in the system.
It is worth noticing that increasing uh had a stabilising effect on the system (actually, this type of behaviour applies also to uf and ug). This result is especially important in the context of the phenomenon modelled, since the most desirable situation happens when the production of methane is not fluctuating. Variable rates of gas production can result in decreased productivity of the biogas plant.
The notion of persistence is particularly important in modelling biological phenomena. Roughly speaking, we say that a system is persistent if all the species with positive initial populations survive. The formal definition is as follows.
Definition 4.1. The system
x′i=xifi(x1,x2,…,xn),xi(0)=xi0≥0,i=1,2,…,n, |
is said to be weakly persistent if
lim supt→∞xi(t)>0,i=1,2,…,n |
for every trajectory with positive initial conditions, and is said to be persistent if
lim inft→∞xi(t)>0,i=1,2,…,n |
for every trajectory with positive initial conditions. This system is said to be uniformly persistent if there exists a positive number ϵ such that
lim inft→∞xi(t)≥ϵ,i=1,2,…,n |
for every trajectory with positive initial conditions.
To prove that system (3.7) is persistent, we will use the Butler-McGehee Lemma [16] repeatedly.
Lemma 4.2. Assume that x∗ is a hyperbolic equilibrium point of the system
x′=f(x),x(0)=x0, |
with x∈Rn and f:Rn→Rn, where f is continuously differentiable. Assume also that x∗ is in ω(x0), the omega limit set of γ+(x0) (the positive semi-orbit through x0), but is not the entire omega limit set. Then ω(x0) has nontrivial (i.e., different from x∗) intersection with the stable and unstable manifolds of x∗.
As we already noticed in Section 4.2, there are values of the parameters where one of the boundary equilibria and the interior equilibrium are both asymptotically stable, and hence system (3.7) is not persistent, even though an interior equilibrium point exists. We will thus focus on the cases for which no boundary equilibrium point of system (3.7) is stable.
Theorem 4.2. Let system (3.7) have the following equilibria configuration (as represented schematically in Figure 4):
Equilibrium | Number of eigenvalues with positive real part | Number of eigenvalues with negative real part |
(000)E | 2 | 1 |
(100)E | 1 | 2 |
(001)E | 1 | 2 |
(110)E | 1 | 2 |
Then system (3.7) is persistent.
Proof. Since planes x0x1, x0x2, and x1x2 are invariant, we know where the stable and unstable manifolds of the boundary equilibria lie. This is represented in a schematic way in Figure 4. Keeping this picture in mind should make the following argument much more transparent. Assume that a solution →x(t)=(x0(t),x1(t),x2(t)) with initial condition →x(0)=(x(0)0,x(0)1,x(0)2), where x(0)i>0, i=0,1,2, is given. We show that no point of the omega limit set has a zero coordinate using proof by contradiction.
First, assume that (000)E belongs to ω(γ+(→x(0))), the omega limit set of γ+(→x(0)). Since (000)E is a saddle point with one-dimensional stable manifold restricted to the x1-axis, it is not the entire omega limit set ω(γ+(→x(0))). Hence, by Lemma 4.2, there is a point x∗≠(000)E in both ω(γ+(→x(0))) and Ws((000)E), the stable manifold of (000)E. The entire orbit through any point in an omega limit set is also in the omega limit set. The stable manifold of (000)E is the x1-axis, and the x1-axis is unbounded. By Lemma 3.1, all orbits of system (3.7) are bounded, and hence the omega limit set of any orbit of system (3.7) is bounded. This contradicts the existence of such an x∗. Thus (000)E∉ω(γ+(→x(0))).
Next, assume that (001)E∈ω(γ+(→x(0))). Since (001)E is a saddle point with two-dimensional stable manifold restricted to the x0x1-plane, {(001)E} is not the entire omega limit set ω(γ+(→x(0))). Thus, by Lemma 4.2, there is a point x∗∈ω(γ+(→x(0)))∩Ws((001)E)∖{(001)E}. Since the stable manifold Ws((001)E) lies entirely in the x1x2-plane, and the entire orbit through x∗ is in ω(γ+(→x(0))), by the analysis in Subsection 4.1, this orbit becomes unbounded in backward time. This contradiction shows that (001)E∉ω(γ+(→x(0))).
Now, assume that (100)E∈ω(γ+(→x(0))). Similarly as in the previous cases, this implies that there exists a point x∗∈ω(γ+(→x(0)))∩Ws((100)E)∖{(100)E}. This time the stable manifold Ws((100)E) is two-dimensional and lies entirely in the x0x2-plane. By the analysis in Subsection 4.1, the entire orbit through x∗(which belongs to ω(γ+(→x(0)))) becomes unbounded in backward time or its closure contains (001)E. This contradiction proves that (100)E∉ω(γ+(→x(0))).
Now, assume that (110)E∈ω(γ+(→x(0))). Again, {(110)E} is not the entire omega limit set ω(γ+(→x(0))), so there exists a point x∗∈ω(γ+(→x(0)))∩Ws((110)E)∖{(110)E}. This point lies in the x0x1-plane, since Ws((110)E) is two-dimensional and is entirely contained in this plane. As in the previous cases, the entire orbit through x∗ is in ω(γ+(→x(0))). Since there are no periodic orbits in the x0x1 face, and since {(100)E}∉ω(γ+(→x(0))), the orbit becomes unbounded in backward time. This contradiction proves that (110)E∉ω(γ+(→x(0))).
Finally, consider any ˆx=(ˆx0,ˆx1,ˆx2), such that ˆxi=0 for at least one i=0,1,2, and assume that ˆx∈ω(γ+(→x(0))). Then, the entire orbit through ˆx is in ω(γ+(→x(0))). But since this orbit lies entirely in either x0x1, x1x2, or x0x2 face, it converges to one of the boundary equilibria. This implies that this boundary equilibrium is in ω(γ+(→x(0))), and this possibility has been eliminated in the previous part of the proof.
We have therefore proven that
lim inft→∞xi(t)>0,i=0,1,2, |
i.e., that system (3.7) is persistent.
An example satisfying the assumptions of Theorem 4.2 occurs for
α=0.0002,uf=0.6,ug=0,uh=0.1. | (4.6) |
Persistence can also be observed with the addition of phenol, i.e., with ug>0.
Theorem 4.3. Let system (3.7) have the following equilibria configuration (as represented schematically in Figure 5):
Equilibrium | Number of eigenvalues with positive real part | Number of eigenvalues with negative real part |
(000)E | 2 | 1 |
(100)E | 1 | 2 |
(001)E | 1 | 2 |
(011)E | 1 | 2 |
(110)E | 1 | 2 |
Then system (3.7) is persistent.
Proof. The idea behind this proof is very similar to the method presented in the proof of Theorem 4.2. Let →x(t)=(x0(t),x1(t),x2(t)) be a solution of system (3.7) with initial condition →x(0)=(x(0)0,x(0)1,x(0)2), where x(0)i>0, i=0,1,2. Since the stable and unstable manifolds of the all of the equilibria, except (001)E and (011)E, have the same configuration as in the hypothesis of Theorem 4.2, the argument eliminating them from the omega limit set of γ+(→x(0)) is exactly the same and we only need to focus on (001)E and (011)E.
Assume that (001)E∈ω(γ+(→x(0))). Since (001)E is a saddle point with one-dimensional stable manifold restricted to the x2-axis, we have ω(γ+(→x(0)))∖{(001)E}≠∅. Hence, by Lemma 4.2, there is a point x∗∈ω(γ+(→x(0)))∩Ws((001)E)∖{(001)E}. The entire orbit through x∗ also belongs to ω(γ+(→x(0))) and either becomes unbounded in backward time or converges to (000)E. Since all orbits of system (3.7) are bounded and (000)E∉ω(γ+(→x(0))), we obtain a contradiction. Hence, (001)E∉ω(γ+(→x(0))).
Next, assume that (011)E∈ω(γ+(→x(0))). Since (011)E is a saddle point with two-dimensional stable manifold restricted to the x1x2-plane (it is repelling into the interior), we have ω(γ+(→x(0)))∖{(011)E}≠∅. By Lemma 4.2, there exists a point x∗∈ω(γ+(→x(0)))∩Ws((011)E)∖{(011)E}. The entire orbit through x∗ belongs to ω(γ+(→x(0))) and either becomes unbounded in backward time or converges to (000)E or (001)E. We have previously shown in Subsection 4.1 that there are no periodic orbits in the x1x2 face. Since we have already proven that (000)E∉ω(γ+(→x(0))) and (001)E∉ω(γ+(→x(0))), we obtain a contradiction. This proves that (011)E∉ω(γ+(→x(0))).
Finally, consider any ˆx=(ˆx0,ˆx1,ˆx2), such that ˆxi=0 for at least one i=0,1,2, and assume that ˆx∈ω(γ+(→x(0))). Then, the entire orbit through ˆx is in ω(γ+(→x(0))). But since this orbit lies entirely in either the x0x1, x1x2, or x0x2 face, it converges to one of the boundary equilibria. This implies that this boundary equilibrium is in ω(γ+(→x(0))). But this possibility has been eliminated in the previous part of the proof.
An example satisfying the assumptions of Theorem 4.3 occurs for
α=0.0002,uf=0.6,ug=0.00015,uh=0.1. | (4.7) |
Remark 1. Interestingly enough, in many cases of models describing biological phenomena, persistence already implies uniform persistence. The rigorous results were obtained in [17]. In our context, the key theorem from [17] states that if F is a dynamical system for which Rn+ and ∂Rn+ are invariant, then F is uniformly persistent provided that
1. F is dissipative (meaning that ∀x∈Rn+ω(x)≠∅ and ⋃x∈Rn+ω(x) has compact closure),
2. F is weakly persistent,
3. ∂F (the restriction of F to the boundary ∂Rn+) is "isolated",
4. ∂F is "acyclic".
These results can be easily modified [18] so that we consider the flow F on Ω and ∂Ω, which are positively invariant. The following theorem is proven using results of [17,18], by checking conditions 1–4 on Ω and ∂Ω.
Theorem 4.4. Under the hypotheses of Theorem 4.2 or Theorem 4.3, system (3.7) is uniformly persistent.
Proof. In our case, the positively invariant set Ω, on which we analyse system (3.7) is bounded. Hence, condition 1 is satisfied. Condition 2 holds by Theorem 4.2 (persistence implies weak persistence). In our context condition 3 is satisfied, because the omega limit set on ∂Ω consists of a finite number of hyperbolic equilibria. Condition 4 is satisfied because the boundary equilibria are not cyclically linked, i.e., there is no cyclic chain of heteroclinic orbits joining them. Thus, we have shown not only persistence, but also uniform persistence of system (3.7) in the cases when the hypotheses of Theorem 4.2 or Theorem 4.3 are satisfied.
We finish this subsection by extending the uniform persistence to the original six dimensional system (2.1).
Theorem 4.5. Under the hypotheses of Theorem 4.2 or Theorem 4.3, the six dimensional system (2.1) is uniformly persistent.
Proof. Notice that if →X0=(x0(0),x1(0),x2(0),s0(0),s1(0),s2(0)) with xi(0)>0, si(0)>0, i=0,1,2, then we necessarily must have ω(→X0)∈Ω. Otherwise, there would exist a point →p∈R6+∖Ω and a sequence of times (tn) with tn→∞ for which the corresponding solution converges to →p. This would mean that Ω is not globally attracting, which was proven in Lemma 3.2. Moreover, there is only a finite number of equilibria on ∂Ω, with all of their stable manifolds lying entirely in ∂Ω. Thus, if the assumptions of either Theorem 4.2 or Theorem 4.3 hold, by Theorem 4.4 and its proof, the corresponding three-dimensional system is uniformly persistent and the boundary ∂Ω is acyclic. Hence, we can apply the results of Thieme [19] to conclude that the six-dimensional system (3.1) is also uniformly persistent.
As previously stated in Section 4.2, we now study effects on the qualitative behaviour of system (3.7) numerically. We consider α as a bifurcation parameter. Throughout this section, we assume that parameters ω0, ω1, ω2, ϕ1, ϕ2, KP, and KI are fixed at the values given in Table 1.
We now fix the following parameters
uf=2,ug=0,uh=0, |
and plot a one-parameter bifurcation diagram in α, with x0 on the y-axis. All simulations were performed using [20].
In Figure 6, we can see that as α decreases, there is a saddle-node bifurcation, resulting in two equilibria (110)E(1) and (110)E(2) appearing (both unstable). Next, there is a transcritical bifurcation with the (110)E(1) equilibrium. This results in the positive equilibrium moving into the interior of the admissible region, Ω. After that, a saddle-node of limit cycles bifurcation occurs that gives birth to stable and unstable periodic orbits. The (111)E equilibrium (unstable), undergoes a Hopf bifurcation, and as a consequence it becomes asymptotically stable, and the stable periodic orbit disappear. Since these bifurcations occur for a narrow range of α, a close-up is presented in Figure 7. Existence of a stable periodic orbit represents a case in which all three populations oscillate indefinitely, and hence the production of methane fluctuates. As already mentioned in Section 4.2, this situation is not a desirable one, because it might result it decreased productivity by the biogas plant. The unstable periodic orbit acts as a separatrix, giving the border of the basin of attraction of two asymptotically stable equilibria in the case of bistability.
Since by the conservation principles (3.6), s0=uf−x0, the bifurcation diagram in α with s0 on the y-axis is similar to the one presented in Figure 6. The amount of chlorophenol in the system is inversely proportional to the concentration of the phenol degrader. As the dilution rate α decreases, the concentration of the chlorophenol degrader in the interior equilibrium increases, and as a consequence, the amount of chlorophenol decreases. It thus suggests that operating on lower dilution rates results in the most desirable dynamics, i.e., an asymptotically stable interior equilibrium and fast chlorophenol removal.
To extend the previous analysis, we now fix the following parameters:
ug=0,uh=0.1, |
and plot a two-parameter bifurcation diagram of system (3.7), choosing α and uf as the bifurcation parameters. Each region of the diagram is labeled and the corresponding dynamics are represented schematically in the surrounding figures in phase space. Black dashed curves correspond to saddle-node of equilibria bifurcations (LP), black solid curves represent saddle-node of limit cycles bifurcations (SNLC), black dotted curves depict Hopf bifurcations (HB), and grey solid curves represent transcritical bifurcations (BP). We also depict the predicted heteroclinic bifurcation by a grey dashed curve which lies very close to the BP curve.
We can see in Figures 8–10, that varying two parameters at the same time can demonstrate much more complicated dynamics than in the case of one-parameter bifurcation diagrams. There is a generalised Hopf bifurcation, at the point at which the Hopf curve intersects the saddle-node of limit cycles curve. This is the point where the criticality of the Hopf bifurcation changes from supercritical to subcritical, moving from left to right. The unstable periodic orbit disappears through a heteroclinic bifurcation. There are two heteroclinic orbits that form a cycle that joins the two equilibria in the x0x1 face, then passes into the interior, and then goes back to the boundary in the x0x1 face. The point at which the Hopf, homoclinic, and saddle-node of limits cycles curves intersect, represents the Bogdanov-Takens bifurcation.
From the biological viewpoint, the most interesting dynamics occurs in regions 5 and iii. There, the interior equilibrium is asymptotically stable. In the case of region 5 we also observe bistability with the (100)E equilibrium. In region iii, there is uniform persistence, and thus an interior compact attractor is present. As was previously anticipated by the analysis of the one-parameter bifurcation diagram, operating at low dilution rates is the most desirable approach. If α is small enough, it is possible to remain in region iii, even for high inflow rate uf.
In this work we have generalised the approach presented in [9] by including multiple substrate inflow in the chemostat, while maintaining generality (in most cases) with respect to the exact form of the growth functions. We observed that allowing the inflow of multiple substrates resulted in much more complex dynamics of the system. For example, eight steady states are possible. We also observed that external addition of substrates (phenol and hydrogen) can result in bistability---two equilibria can simultaneously be asymptotically stable. As well, there can be an orbitally asymptotically stable periodic orbit with all of the populations surviving and an asymptotically stable equilibrium with only the chlorophenol degrader population surviving.
We have also confirmed the findings of the previous analysis in [9], where numerical evidence of the occurrence of a supercritical Hopf bifurcation was given. Theoretical conditions for the existence of a Hopf bifurcation were provided in the case of specific forms of the growth functions. Varying any one of the three parameters: chlorophenol, phenol, and hydrogen inflow rates, was shown to result in a Hopf bifurcation. Theoretical results for varying the dilution rate as the bifurcation parameter has been left for future work. However, we have observed numerically, that varying this parameter can result in a Hopf bifurcation and a saddle-node of limit cycles bifurcation. Our numerical investigations also showed that increasing the inflow rate of the substrates has a stabilising effect on the entire system. From a biological engineering point of view, in a bioreactor treating a monochlorophenol rich waste stream, instability would typically be undesirable in terms of process performance. Therefore, identification of control strategies to avoid periodic behaviour is an important aspect of this work.
Another result, particularly important for engineering applications, concerns the persistence of the system for a range of parameter sets. Knowing when the microbial populations survive is again crucial from a process control perspective, and it is one of the main theoretical results of this work. We have proven that in two configurations of equilibria (in both cases all the boundary equilibria are saddle points) we observe not only persistence, but also uniform persistence, a much stronger result. These situations occur when there is an inflow of all three substrates, but also when phenol addition is not considered (i.e., when ug=0).
Although we now know much more about the dynamics of the system, it is not fully understood. This follows from the numerical results provided by the two-parameter bifurcation diagrams. The analyses reveals that varying the dilution rate and the chlorophenol inflow simultaneously, can lead to a Bogdanov-Takens and a Bautin (generalised Hopf) bifurcations. Also, for the cases of bistability, where both a boundary and the interior equilibrium are asymptotically stable, it is of great importance to empiricists to have an estimation of the basins of attraction of these equilibria. This result is usually difficult to obtain theoretically, however numerical estimations are possible. Another factor that is of interest would be the inclusion of stochasticity in the model. In practice, even if the interior equilibrium is globally asymptotically stable, one of the microorganisms may become extinct. This might occur when a population is very small, and the stochastic noise effects result in the solution curve reaching one of the invariant faces of the admissible region.
Anecdotally, there has been some resistance to using reduced order models, of the type described here. Some researchers consider them too removed from the systems they represent to be of practical use. Without experimental results to compare against model predictions, they remain mostly theoretical constructs limited by the underlying assumptions and simplifications imposed. However, we can look to emerging disciplines such as synthetic biology to help bridge the theoretical and the applied [21]. Recent studies have shown that synthetically derived anaerobic communities are able to confirm model predictions and provide insight into the ecology and dynamics of microbial communities that are relevant in practice [22]. For example, we have shown that, in a region of unstable dynamics for a given set of operational parameters and initial conditions, addition of extraneous hydrogen results in an asymptotically stable equilibrium by ensuring survival of the two hydrogen consumers. Hydrogen is a key substrate in methanogenic systems, but process stabilisation by its addition has only recently been suggested [6,7]. We believe this work provides a basis from which experimental studies describing microbial food-webs with complex, high-order interactions can be conducted with a posteriori knowledge of the anticipated behaviour.
Matthew J. Wade acknowledges the support from the European Union's Horizon 2020 research and innovation programme under the Marie Skłodowska-Curie Grant Agreement No. 702408 (DRAMATIC). Gail S.K. Wolkowicz acknowledges support from the Natural Sciences and Engineering Council of Canada (NSERC) Discovery Grant with accelerator supplement.
The Authors declare no conflict of interest in this work.
[1] | J. Zhang, W. J. Xu, G. Wang, Analysis of lamivudine-resistant mutations in 268 patients with chronic hepatitis B during lamivudine therapy(In Chinese), Chin. J. Health Lab. Technol., 18 (2008), 2343–2344. |
[2] | Y. Su, G. F. Gao, G. M. Li, Research progress on hepatitis B virus mutations and resistance to nucleosides, 2011. Available from: https://www.doc88.com/p-1963143564969.html. |
[3] | J. Deng, X. X. Zhang, Nucleoside (acid) drug resistance mechanism and its countermeasures, Chin. J. Prac. Int. Med., 27 (2007), 1402–1404. |
[4] | H. Y. Pang, W. D. Wang, Deterministic and stochastic analysis of a cell-to-cell virus dynamics model with immune impairment, J. Southwest Chin. Norm. Univ. (Nat. Sci. Ed.), 31 (2006), 26–30. |
[5] | H. Y. Pang, W. D. Wang, Global properties of virus dynamics with CTL immune response, J. Southwest Chin. Norm. Univ. (Nat. Sci. Ed.), 30 (2005), 38–41. |
[6] | F. L. Xie, M. J. Shan, X. Z. Lian, Periodic solution of a stochastic HBV infection model with logistic hepatocyte growth, Appl. Math. Comput., 293 (2017), 630–641. |
[7] | Y. Wang, X. N. Liu, An HBV infection model with logistic growth, cure rate and CTL immune response, J. Southwest Chin. Norm. Univ. (Nat. Sci. Ed.), 38 (2016), 1–6. |
[8] | H. W. Hui, The Analysis of Virus Model and Population Model with Stochastic Perturbation, MA. Thesis, Xinjiang University, 2018. |
[9] |
K. B. Bao, Q. M. Zhang, X. N. Li, A model of HBV infection with intervention strategies: dynamics analysis and numerical simulations, Math. Biosci. Eng., 16 (2019), 2562–2586. doi: 10.3934/mbe.2019129
![]() |
[10] |
Y. L. Cai, Y. Kang, M. Banerjee, W. M. Wang, A stochastic SIRS epidemic model with infectious force under intervention strategies, J. Differ. Equations, 259 (2015), 7463–7502. doi: 10.1016/j.jde.2015.08.024
![]() |
[11] |
Q. Liu, D. Q. Jiang, N. Z. Shi, T. Hayat, A. Alsaedi, Dynamical behavior of a stochastic HBV infection model with logistic hepatocyte growth, Acta Math. Sci., 37 (2017), 927–940. doi: 10.1016/S0252-9602(17)30048-6
![]() |
[12] | M. Zhao, X. Y. Wang, Asymptotic behavior of global positive solution to stochastic SIQR epidemic model, J. Univ. Jinan (Sci. Technol.), 33 (2019), 88–94. |
[13] | F. Y. Wei, Y. H. Cai. Y. H. Zhao, The asymptotic behavior of a stochastic SIQS epidemic model with nonlinear incidence, J. Biomath., 31 (2016), 109–117. |
[14] | P. Y. Xia, The Dynamic Behavior of Several Stochastic Virus Models, Ph.D thesis, Northeast Normal University, 2018. |
[15] |
Y. N. Zhao, D. Q. Jiang, The extinction and persistence of the stochastic SIS epidemic model with vaccination, Phys. A Stat. Mech. Appl., 392 (2013), 4916–4927. doi: 10.1016/j.physa.2013.06.009
![]() |
[16] | Y. Muroya, Y. Enatsu, H. Li, Global stability of a delayed HTLV–I infection model with a class of nonlinear incidence rates and CTLs immune response, Appl. Math. Comput., 219 (2013), 10559–10573. |
[17] | X. W. Cui, Dynamic Behavior Analysis of Virus Infection Model Induced by Random Noise, MA. Thesis, Taiyuan University of Technology, 2018. |
[18] |
H. Srivastava, K. Saad, J. Gómez-Aguilar, A. A. Almadiy, Some new mathematical models of the fractional-order system of human immune against IAV infection, Math. Biosci. Eng., 17 (2020), 4942–4969. doi: 10.3934/mbe.2020268
![]() |
[19] |
K. Saad, J. Gómez–Aguilar, A. Almadiyd, A fractional numerical study on a chronic hepatitis C virus infection model with immune response, Chaos Solitons Fractals, 139 (2020), 110062. doi: 10.1016/j.chaos.2020.110062
![]() |
[20] |
H. Srivastava, K. Saad, Numerical simulation of the fractal-fractional Ebola virus, Fractal Fractional, 4 (2020), 49. doi: 10.3390/fractalfract4040049
![]() |
[21] |
D. M. Li, B. Chai, A dynamic model of hepatitis B virus with drug-resistant treatment, AIMS Math., 5 (2020), 4734–4753. doi: 10.3934/math.2020303
![]() |
[22] | N. Wang, The research status of hepatitis B Virus cccDNA, Immunol. Studies, 3 (2015), 15–18. |
[23] | X. Y. Liu, Q. H. Shang, X. S. Liu, Relationship between the serum HBV DNA and the change of the intrahepatic HBV cceDNA levels in antiviral therapy for chronic hepatitis B, Chin. Hepatol., 20 (2015), 591–596. |
[24] | L. Min, Y. Su, Y. Kuang, Mathematical analysis of a basic virus infection model with application to HBV infection, Rocky Mt. J. Math., 38 (2008), 1573–1585. |
1. | Sarra Nouaoura, Radhouane Fekih-Salem, Nahla Abdellatif, Tewfik Sari, Mathematical analysis of a three-tiered food-web in the chemostat, 2020, 0, 1553-524X, 0, 10.3934/dcdsb.2020369 | |
2. | Mohamed Dellala, Bachir Bar, Mustapha Lakrib, A competition model in the chemostat with allelopathy and substrate inhibition, 2021, 0, 1553-524X, 0, 10.3934/dcdsb.2021120 | |
3. | Tahani Mtar, Radhouane Fekih-Salem, Tewfik Sari, Mortality can produce limit cycles in density-dependent models with a predator-prey relationship, 2022, 27, 1531-3492, 7445, 10.3934/dcdsb.2022049 | |
4. | Sarra Nouaoura, Nahla Abdellatif, Radhouane Fekih-Salem, Tewfik Sari, Mathematical Analysis of a Three-Tiered Model of Anaerobic Digestion, 2021, 81, 0036-1399, 1264, 10.1137/20M1353897 | |
5. | Sarra Nouaoura, Radhouane Fekih-Salem, Nahla Abdellatif, Tewfik Sari, Operating diagrams for a three-tiered microbial food web in the chemostat, 2022, 85, 0303-6812, 10.1007/s00285-022-01812-5 | |
6. | Abdulrahman Ali Alsolami, Miled El Hajji, Mathematical Analysis of a Bacterial Competition in a Continuous Reactor in the Presence of a Virus, 2023, 11, 2227-7390, 883, 10.3390/math11040883 | |
7. | Nour El Houda Zitouni, Mohamed Dellal, Mustapha Lakrib, Substrate inhibition can produce coexistence and limit cycles in the chemostat model with allelopathy, 2023, 87, 0303-6812, 10.1007/s00285-023-01943-3 | |
8. | Radhouane Fekih-Salem, Analysis of an intra- and interspecific interference model with allelopathic competition, 2025, 542, 0022247X, 128801, 10.1016/j.jmaa.2024.128801 | |
9. | Miled El Hajji, Mathematical modeling for anaerobic digestion under the influence of leachate recirculation, 2023, 8, 2473-6988, 30287, 10.3934/math.20231547 | |
10. | Amer Hassan Albargi, Miled El Hajji, Bacterial Competition in the Presence of a Virus in a Chemostat, 2023, 11, 2227-7390, 3530, 10.3390/math11163530 | |
11. | Grazia Policastro, Vincenzo Luongo, Luigi Frunzo, Nick Cogan, Massimiliano Fabbricino, A mechanistic mathematical model for photo fermentative hydrogen and polyhydroxybutyrate production, 2023, 20, 1551-0018, 7407, 10.3934/mbe.2023321 |
Parameters | Value |
ω0 | 0.1854 |
ω1 | 1656.69 |
ω2 | 163.08 |
ϕ1 | 1.8875 |
ϕ2 | 3.8113 |
KP | 0.04 |
KI | 7.1429 |