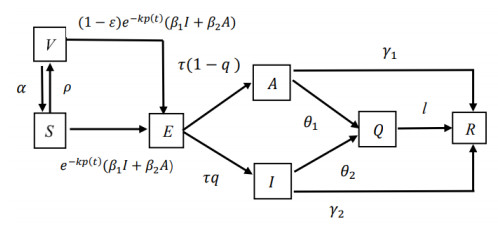
The COVID-19 pandemic has caused widespread concern around the world. In order to study the impact of media coverage and vaccination on the spread of COVID-19, we establish an SVEAIQR infectious disease model, and fit the important parameters such as transmission rate, isolation rate and vaccine efficiency based on the data from Shanghai Municipal Health Commission and the National Health Commission of the People's Republic of China. Meanwhile, the control reproduction number and the final size are derived. Moreover, through sensitivity analysis by PRCC (partial rank correlation coefficient), we discuss the effects of both the behavior change constant k according to media coverage and the vaccine efficiency ε on the transmission of COVID-19. Numerical explorations of the model suggest that during the outbreak of the epidemic, media coverage can reduce the final size by about 0.26 times. Besides that, comparing with 50% vaccine efficiency, when the vaccine efficiency reaches 90%, the peak value of infected people decreases by about 0.07 times. In addition, we simulate the impact of media coverage on the number of infected people in the case of vaccination or non-vaccination. Accordingly, the management departments should pay attention to the impact of vaccination and media coverage.
Citation: Xiaojing Wang, Yu Liang, Jiahui Li, Maoxing Liu. Modeling COVID-19 transmission dynamics incorporating media coverage and vaccination[J]. Mathematical Biosciences and Engineering, 2023, 20(6): 10392-10403. doi: 10.3934/mbe.2023456
[1] | Weike Zhou, Aili Wang, Fan Xia, Yanni Xiao, Sanyi Tang . Effects of media reporting on mitigating spread of COVID-19 in the early phase of the outbreak. Mathematical Biosciences and Engineering, 2020, 17(3): 2693-2707. doi: 10.3934/mbe.2020147 |
[2] | Yuri Amemiya, Tianwen Li, Hiroshi Nishiura . Age-dependent final size equation to anticipate mortality impact of COVID-19 in China. Mathematical Biosciences and Engineering, 2023, 20(6): 11353-11366. doi: 10.3934/mbe.2023503 |
[3] | Xinyu Liu, Zimeng Lv, Yuting Ding . Mathematical modeling and stability analysis of the time-delayed $ SAIM $ model for COVID-19 vaccination and media coverage. Mathematical Biosciences and Engineering, 2022, 19(6): 6296-6316. doi: 10.3934/mbe.2022294 |
[4] | Beatriz Machado, Liliana Antunes, Constantino Caetano, João F. Pereira, Baltazar Nunes, Paula Patrício, M. Luísa Morgado . The impact of vaccination on the evolution of COVID-19 in Portugal. Mathematical Biosciences and Engineering, 2022, 19(1): 936-952. doi: 10.3934/mbe.2022043 |
[5] | Pannathon Kreabkhontho, Watchara Teparos, Thitiya Theparod . Potential for eliminating COVID-19 in Thailand through third-dose vaccination: A modeling approach. Mathematical Biosciences and Engineering, 2024, 21(8): 6807-6828. doi: 10.3934/mbe.2024298 |
[6] | Fang Wang, Lianying Cao, Xiaoji Song . Mathematical modeling of mutated COVID-19 transmission with quarantine, isolation and vaccination. Mathematical Biosciences and Engineering, 2022, 19(8): 8035-8056. doi: 10.3934/mbe.2022376 |
[7] | Xinghua Chang, Maoxing Liu, Zhen Jin, Jianrong Wang . Studying on the impact of media coverage on the spread of COVID-19 in Hubei Province, China. Mathematical Biosciences and Engineering, 2020, 17(4): 3147-3159. doi: 10.3934/mbe.2020178 |
[8] | Tao Chen, Zhiming Li, Ge Zhang . Analysis of a COVID-19 model with media coverage and limited resources. Mathematical Biosciences and Engineering, 2024, 21(4): 5283-5307. doi: 10.3934/mbe.2024233 |
[9] | Jin Guo, Aili Wang, Weike Zhou, Yinjiao Gong, Stacey R. Smith? . Discrete epidemic modelling of COVID-19 transmission in Shaanxi Province with media reporting and imported cases. Mathematical Biosciences and Engineering, 2022, 19(2): 1388-1410. doi: 10.3934/mbe.2022064 |
[10] | Jiajia Zhang, Yuanhua Qiao, Yan Zhang . Stability analysis and optimal control of COVID-19 with quarantine and media awareness. Mathematical Biosciences and Engineering, 2022, 19(5): 4911-4932. doi: 10.3934/mbe.2022230 |
The COVID-19 pandemic has caused widespread concern around the world. In order to study the impact of media coverage and vaccination on the spread of COVID-19, we establish an SVEAIQR infectious disease model, and fit the important parameters such as transmission rate, isolation rate and vaccine efficiency based on the data from Shanghai Municipal Health Commission and the National Health Commission of the People's Republic of China. Meanwhile, the control reproduction number and the final size are derived. Moreover, through sensitivity analysis by PRCC (partial rank correlation coefficient), we discuss the effects of both the behavior change constant k according to media coverage and the vaccine efficiency ε on the transmission of COVID-19. Numerical explorations of the model suggest that during the outbreak of the epidemic, media coverage can reduce the final size by about 0.26 times. Besides that, comparing with 50% vaccine efficiency, when the vaccine efficiency reaches 90%, the peak value of infected people decreases by about 0.07 times. In addition, we simulate the impact of media coverage on the number of infected people in the case of vaccination or non-vaccination. Accordingly, the management departments should pay attention to the impact of vaccination and media coverage.
Coronavirus disease 2019 (COVID-19) has spread all over the world since December 2019, causing a dramatic impact on global public health. Up to now, COVID-19 is still producing a pandemic worldwide with the emergence of new SARS-CoV-2 variants [1]. In order to control the spread of the epidemic, many mathematical models were developed and studied. Some literature focuses on the impact of media coverage and vaccination on the prevalence of COVID-19. In 2020, Yan et al. proposed a novel model to analyze the impact of media coverage on the transmission of the COVID-19 epidemic [2]. The results implied that the management departments should guide people's behavior through media coverage and apply effective quarantine strategies. Collinson et al. employed a stochastic agent based model to study the effects of mass media coverage on the 2009 H1N1 pandemic. The study indicated that reporting rates and the speed of media fatigue greatly affected the variability of important public health measures. When mass media coverage data was considered, two peaks of infection occurred [3]. Koutou et al. proposed a mathematical model with a standard incidence rate to assess the role of media such as in the mitigation of the propagation of COVID-19 [4]. Liu et al. [5] evaluated how media coverage can alleviate the prevalence of COVID-19 by constructing a provincial dataset on COVID-19. It turned out that the impact of media reports on the spread of COVID-19 in China was an inverted U-shaped curve, and it was mediated by intra-provincial and inter-provincial population mobility. In 2022, based on the transportation network among 31 provinces in China, Zou et al. established a multi-patch coupling model under the strategy of combining vaccination and quarantine, and then derived critical quarantine rate to control the spread of the pandemic and vaccination rate to achieve herd immunity [11]. In [12], Avila-Ponce de León et al. developed a mathematical model to explain the effects of two strains and vaccination on the transmission of COVID-19. The mathematical model yielded the minimum percentage of fully vaccinated individuals to reduce the spread of the variants. In [13] Song et al. investigated a mathematical model of COVID-19 transmission with vaccination and isolation delay, and analyzed the optimal control strategy. They found that the optimal isolation rate could minimize the cumulative number of infected people and the cost of disease control, and it could effectively control the propagation of disease with limited resources. In [14], Krueger et al. evaluated the impact of vaccines, other public health measures and the decline of immunity on the control of COVID-19. The results showed that the high re-vaccination rates and the reduction of the proportion of unvaccinated population increased the benefits of vaccination pass and need to remain vigilant. In order to restrain the rapid spread of the omicron variant in Europe, health professionals and researchers from across Europe clarified three measures in [15] as follows: reducing the number of infected people, protecting children from infection and buying time for more people to be vaccinated. Markovic et al. proposed and studied a social network epidemiological model considering population heterogeneity and different vaccination strategies. They explained how COVID-19 evolved and how different vaccination schemes controlled the epidemic [16]. [17,18,19] proposed a series of COVID-19 transmission dynamics models with vaccination, and proved that COVID-19 vaccination can decrease the size of infection. Additionally, there are also literatures modeling and demonstrating the positive role of media reporting in controlling the spread of the epidemic [20,21,22].
Motivated by the above literature, we focus on revealing the impact of media coverage and vaccination on the transmission of COVID-19 by different behavior change constant k and vaccine efficiency. Furthermore, some people will be infected after vaccination. However, as far as we know, there is no research on combining the two measures. So, we formulate an SVEAIQR model based on the data from Shanghai in April 2022, and we estimate the parameters of the force of infection, media coverage, vaccine efficiency and other related parameters using the least squares method. Through numerical simulations, we clarify the impact of media coverage and vaccination on the final size of infected people, and we verify the significance of isolating asymptomatic infected persons for the epidemic in Shanghai in April 2022.
The rest of the paper is organized as follows. In Section 2, we present the SVEAIQR model, and the final size without vaccination is derived. In Section 3, first, we fit the parameters according to the data of Shanghai and analyze the sensitivity of the parameters. Second, we simulate the changes of the number of infected people under different media coverage, vaccine efficiency and isolation rates. Then, we summarize our findings in Section 4.
Due to the transmission mechanism of COVID-19 and control measures, we divide the population into seven subclasses including susceptible (S), vaccinated (V), exposed (E), asymptomatic (A), symptomatic (I), quarantined (Q), and recovered (R). Let N denote the total number of the population. We provide the transfer flow chart in Figure 1:
Then, we construct an epidemic model as follows:
{˙S(t)=−e−kp(t)(β1I+β2A)S+αV−ρS,˙V(t)=ρS−[(1−ε)e−kp(t)(β1I+β2A)+α]V,˙E(t)=e−kp(t)(β1I+β2A)S+(1−ε)e−kp(t)(β1I+β2A)V−τE,˙A(t)=(1−q)τE−(γ1+θ1)A,˙I(t) =qτE−(γ2+θ2)I,˙Q(t)=θ1A+θ2I−lQ,˙R(t)=γ1A+γ2I+lQ. | (2.1) |
For model (2.1), we propose the following assumptions:
1. Vaccinated individuals in compartment V lose vaccine protection and become susceptible at per-capita rates α and ρ, respectively. However, the vaccine fails to protect a portion (1−ε) of individuals.
2. The force of infection is reduced by the function of mask wearing rate e−kp(t), which describes the impact of individual behavioral changes (media) on the COVID-19 epidemic.
3. Individuals in compartment E become symptomatic infected in the proportion of q, and the transmission rate is τ.
4. Asymptomatic infected persons and symptomatic infected persons are isolated at rates θ1 and θ2, respectively.
5. The average duration of infectiousness is 1/l.
The variable parameters and biological significance in model (2.1) are shown in Table 1.
β1 | The force of infection for symptomatic | 7.6453×10−12 | Fit |
β2 | The force of infection for asymptomatic | 6.36×10−8 | Fit |
ρ | Vaccination coverage rate | 0.00112 | [6] |
α | Rate of losing vaccine induced immunity | 0.0056 | [5] |
τ | Removal rate of the incubation period population | 0.3125 | [7] |
q | Proportion of symptomatic infection | 0.7 | Assumed |
ε | Vaccine efficiency | 0.7323 | Fit |
θ1 | Isolation rate of asymptomatic infection | 0.702 | Fit |
θ2 | Isolation rate of symptomatic infection | 0.8 | [8] |
γ1 | Recovery rate of asymptomatic infection | 0.0714 | Assumed |
γ2 | Recovery rate of symptomatic infection | 0.033 | Assumed |
k | The behavior change constant | 2.2073×10−9 | Fit |
p(t) | The rate of wearing masks | 1 | [1] |
l | Recovery rate for quarantined | 114 | [8] |
Let I = 0 and all the right sides equal zero in model (2.1), and we can obtain the disease-free equilibrium E0=(S0,V0,0,0,0,0,0).
The control reproduction number Rc is a threshold that reflects the transmission ability, indicating the average number of secondary infections produced by an infected person during the infection period under the implementation of certain prevention and control measures. When Rc<1, the disease can be effectively controlled, and when Rc>1, the disease will persist. The control reproduction number can be calculated by the method of the next generation matrix [9].
We write as
F=(S0+(1−ε)V0)e−kp(t)(0β2β1000000),V−1=(1τ001−qγ1+θ11γ1+θ10qγ2+θ201γ2+θ2). |
Then, we can calculate Rc=ρ(FV−1∣E0)=(1−q)β2Mγ1+θ1+qβ1Mγ2+θ2, where M=[S0+(1−ε)V0]e−kp(t).
The biological interpretation of Rc is as follows: The component 1 represents the numbers of secondary cases produced by an asymptomatic infected case with (1−q) ratio in the 1γ1+θ1 infection period. The component 2 indicates the number of new cases of symptomatic infected persons with q ratio during the infection period 1γ2+θ2. Based on the fitted data in Table 1, we calculate the control reproduction number Rc=0.5266.
Let V=0 in model (2.1), then model (2.1) reduces to the following model (2.2):
{˙S(t)=−e−kp(t)(β1I+β2A)S,˙E(t)=e−kp(t)(β1I+β2A)S−τE,˙A(t)=(1−q)τE−(γ1+θ1)A,˙I(t) =qτE−(γ2+θ2)I,˙Q(t)=θ1A+θ2I−lQ,˙R(t)=γ1A+γ2I+lQ, | (2.2) |
with initial conditions S(0)=N−E0−A0−I0−Q0−R0, E(0)=E0, A(0)=A0, I(0)=I0, Q(0)=Q0, R(0)=R0. Similar to the method used in model (2.1), we can get the control reproduction number of model (2.2):
Rc0=(1−q)e−kp(t)β2S(0)γ1+θ1+qe−kp(t)β1S(0)γ2+θ2. |
Adding the first two equations of model (2.2), we obtain
˙S(t)+˙E(t)=−τE. | (2.3) |
We call ˙S(t)+˙E(t) uniformly continuous, and then limt→∞(˙S(t)+˙E(t))=0, so for any ε>0, there exists a δ=δ(ε)>0 satisfying
|(˙S+˙E)(t1)−(˙S+˙E)(t2)|<ε2, if |t1−t2|<δ . |
Hence, the limit of S(t)+E(t) exists. By convergence principle, there is a T=T(ε)>0, such that when t>T we have
|(S+E)(t+δ)−(S+E)(t)|<εδ2. |
So, the following formula holds:
|˙S(t)+˙E(t)|=|˙S(t)+˙E(t)−(S+E)(t+δ)−(S+E)(t)δ+(S+E)(t+δ)−(S+E)(t)δ|≤|(˙S+˙E)(t)−(˙S+˙E)(α)|+1δ|(S+E)(t+δ)−(S+E)(t)|<ε2+1δεδ2=ε, |
where α∈(t,t+δ). Thus,
limt→∞E(t)=E(∞)=0. |
According to the third and fourth equations of model (2.2), we can gain limt→∞ sup I(t)≤0 and limt→∞ sup A(t)≤0. Since A(t)>0,I(t)>0, limt→∞I(t)=limt→∞A(t)=0 holds. For the sixth equation of model (2.2), similarly, we have limt→∞Q(t)=0. Therefore, S(∞) exists.
In model (2.2), it follows that
∫∞0˙S(t)+˙E(t)dt=−τ∫∞0E(t)dt. |
Furthermore, we have
∫∞0E(t)dt=S(0)+E(0)−S(∞)−E(∞)τ=S(0)+E(0)−S(∞)τ. | (2.4) |
Adding the first three equations of model (2.2), we have
˙S(t)+˙E(t)+˙A(t)=−qτE−(γ1+θ1)A, |
and then integrating both sides yields
S(0)+E(0)+A(0)−S(∞)−E(∞)−A(∞)=qτ∫∞0E(t)dt+(γ1+θ1)∫∞0A(t)dt. |
Substituting (2.4) into the above equation gives
∫∞0A(t)dt=(1−q)S(0)+(1−q)E(0)+A(0)−(1−q)S(∞)γ1+θ1. | (2.5) |
Similarly, adding the first four equations of model (2.2), and we have
S(0)+E(0)+A(0)+I(0)−S(∞)−E(∞)−A(∞)−I(∞)=(γ1+θ1)∫∞0A(t)dt+(γ2+θ2)∫∞0I(t)dt. |
Combining (2.5) gives
∫∞0I(t)dt=qS(0)+qE(0)+qI(0)−qS(∞)γ2+θ2. | (2.6) |
From (2.5) and (2.6), we can conclude that
∫∞0β1e−kp(t)I(t)dt+∫∞0β2e−kp(t)A(t)dt |
is convergent. Therefore,
S(∞)=S(0)e−∫∞0β1e−kp(t)I(t)dt+∫∞0β2e−kp(t)A(t)dt>0. |
Divide the first equation of model (2.2) by S and then integrate, we have
lnS(0)S(∞)=β1e−kp(t)∫∞0I(t)dt+β2e−kp(t)∫∞0A(t)dt. |
That is,
lnS(0)S(∞)=β1e−kp(t)qS(0)+qE(0)+I(0)−qS(∞)γ2+θ2+β2e−kp(t)(1−q)S(0)+(1−q)E(0)+A(0)−(1−q)S(∞)γ1+θ1. |
Furthermore, we have
lnS(0)S(∞)=Rc0(1−E(0)S(0)−S(∞)S(0))+β1e−kp(t)I(0)γ2+θ2+β2e−kp(t)A(0)γ1+θ1. |
Based on [10], we take E(0)=A(0)=I(0)=0, and S(0)=N. Then,
lnS(0)S(∞)=Rc0(1−S(∞)N). |
By the above formula, we can combine Rc0 to infer the influence of parameters on the final size of the epidemic, the number of the population who are infected over the course of the epidemic, is N−S(∞)=S(0)−S(∞) [23].
In this study, the COVID-19 data comes from the Shanghai Municipal Health Commission [6] and National Health Commission of the People's Republic of China [24]. The total population of Shanghai is around N = 24894300, and thus we choose the initial values as S(0)=20000000,V(0)=5000000,E(0)=7000,A(0)=8000,I(0)=2000,Q(0)=3600,R(0)=10000.
In Figure 2, we draw the actual number of COVID-19 cases from Shanghai and the fitted curve by model (2.1), respectively. Simultaneously, the fitted parameter values are presented in Table 1.
The LHS / PRCC method, namely, latin hypercube sampling / partial rank correlation coefficient method, is a global parameter sensitivity analysis method, which can quickly and effectively identify the sensitive parameters to Rc in the model, and is applicable to the correlation analysis among multiple variables. The greater the absolute value of partial rank correlation coefficient (PRCC), the stronger the correlation is. A positive (negative) value represents a positive (negative) correlation.
As shown in Figure 3 (a), the isolation rate of asymptomatic infection θ1 and recovery rate of asymptomatic infection γ1 are negatively correlated with the control reproduction number Rc. In addition, the force of infection for asymptomatic β2, the proportion of symptomatic infection q and the force of infection for symptomatic β1 are positively correlated with Rc, where β2 is the strongest negative partial rank correlation coefficient, which is because the asymptomatic infections accounted for a large proportion of infections in Shanghai. Therefore, the isolation rate of infected people should be strengthened, and people should be called for nucleic acid testing, so as to effectively prevent and control the disease transmission during the epidemic in Shanghai in April 2022.
To study the impact of media coverage and vaccination, we conducted a sensitivity analysis on k and ε. From Figure 3 (b), we know that with the increase of behavior change constant k and vaccine efficiency ε, the control reproduction number Rc decreases. Therefore, it is necessary to reinforce the impact of media coverage and vaccine efficiency for COVID-19.
Figure 4 describes how different measures affect the final size of COVID-19 epidemic in Shanghai in April 2022. First of all, without any measures, the final size of infectious diseases is the largest. Also, the final size with only vaccination is 1.08 times that with only media coverage, and media coverage can reduce the final size by about 0.26 times. So, the outcome suggests that media coverage is more effective than vaccination.
In case of only considering the influence of media coverage, Figure 5(a) shows that the peak value of the epidemic decreases by 5000 when the behavior change constant k from 0.01 to 0.5. Figure 5 (b) indicates that under the combined effect of vaccination and media coverage, the peak value of the epidemic cuts down by about 4000 with the increase of the behavior change constant k changes from 0.01 to 0.5. Comparing Figure 5(a) and 5(b), we can see that, when k=0.01, vaccination can reduce the peak of infection by more than 2000 during the outbreak of COVID-19 epidemic in 2022 in Shanghai. Therefore, public health departments should encourage people to take vaccine actively, and timely provide the information about the dynamic situation of the epidemic and the effective prevention strategy by media coverage.
In Figure 6, we examine the effect of vaccine efficiency ε on the number of infected people. One of the purposes is to observe whether increasing vaccination efficiency will always be beneficial in terms of reducing the infection level. Compared with 50% vaccine efficiency, when the vaccine efficiency reaches 90%, the peak value of infected people decreases by about 0.07 times. Furthermore, with the improvement of vaccine efficiency ε, the peak time for COVID-19 can be advanced.
Since there are many asymptomatic infections during the outbreak of COVID-19 in Shanghai in April 2022, isolation of asymptomatic infected persons is very significant to control COVID-19. Figure 7 indicates that the number of infected people always increases when the isolation rate of asymptomatic infection θ1 is 0.3, and when the isolation rate is not less than 50%, the number of infected people eventually decreases. Hence, the number of infected people declines only if the isolation rate reaches a certain threshold. This is consistent with the previous sensitivity analysis (in section 3). Therefore, during the COVID-19 outbreak in Shanghai, it is necessary to enhance the isolation of asymptomatic infections.
On the basis of the available, we investigate the impact of media coverage and vaccination on the spread of COVID-19. Concurrently, the efficacy of the vaccine will continue to decline over time, and then some people will be infected again. Thus, we put forward a class of SVEAIQR infectious disease model. The main intention of this study is to examine the joint impact of vaccination and media coverage on the prevalence of COVID-19. The control reproduction number is calculated, and there is formula derivation for the final size of the outbreak in case of non-vaccine.
According to the COVID-19 data of Shanghai in April 2022, we fitted the relevant parameters through the least squares method and then performed control measures related numerical experiments. Sensitivity analysis makes clear that the behavior change constant k and vaccine efficiency ε are negatively correlated with Rc. Under the combined effect of vaccination and media coverage, the peak value of the epidemic cuts down by about 4000 with the increase of the behavior change constant k. When the vaccine efficiency ε increases from 0.5 to 0.9, the peak value of infected people decreases by about 2000. This indicates that increasing vaccine efficiency and media coverage intensity will reduce the control reproduction number and consequently will alleviate the risk of disease transmission. At the same time, our simulations have revealed the number of infected people always increases when the isolation rate of asymptomatic infection θ1 is 0.3. It declines only if the isolation rate reaches a certain threshold.
Our model contains seven essential compartments, but it still has some deficiencies. First of all, this paper simulates the epidemic data only from Shanghai in April 2022, and the COVID-19 data of other countries and regions were not used for fitting. If you want to apply this model to fit the COVID-19 transmission of other countries and regions, you should consider different strategies. Second, although media coverage can change people's behaviors, our research depicts this aspect in a very simple form, that is, the effect of media education on the propagation of COVID-19 is reflected in the rate function of wearing masks. In addition, the media broadcast will also have saturation or retroaction, and we feel impelled to investigate further. Finally, we only consider the fixed vaccination rate. In fact, vaccination strategy is a very complex process. Generally, our study reveals that vaccination and media coverage have significant effects on alleviating the spread of COVID-19. So, the authorities should stress precise and differentiated strategies in the prevention and control of the novel coronavirus epidemic.
This research is supported by the National Nature Science Foundation of China (Grant No. 12071445), the BUCEA Post Graduate Education Teaching Quality Improvement Project (J2023021) and the BUCEA Post Graduate Innovation Project (No. PG2022142, PG2023145). We thank all the individuals who provided support and guidance to this study.
The authors declare there is no conflict of interest.
[1] |
G. Vattiatio, A. Lustig, O. J. Maclaren, M. J. Plank, Modelling the dynamics of infection, waning of immunity and re-infection with the Omicron variant of SARS-CoV-2 in Aotearoa New Zealandr, Epidemics, 41 (2022), 100657. https://doi.org/10.1016/j.epidem.2022.100657 doi: 10.1016/j.epidem.2022.100657
![]() |
[2] |
Q. Yan, Y. Tang, D. Yan, J. Wang, L. Yang, X. Yang, et al., Impact of media reports on the early spread of COVID-19 epidemic, J. Theor. Biol., 502 (2020), 110385. https://doi.org/10.1016/j.jtbi.2020.110385 doi: 10.1016/j.jtbi.2020.110385
![]() |
[3] |
S. Collinson, J. M. Heffernan, Modelling the effects of media during an influenza epidemic, BMC Public Health, 14 (2014), 376. https://doi.org/10.1186/1471-2458-14-376 doi: 10.1186/1471-2458-14-376
![]() |
[4] |
O. Koutou, A. B. Diabaté, B. Sangaré, Mathematical analysis of the impact of the media coverage in mitigating the outbreak of COVID-19, Math. Comput. Simul., 205 (2023), 600–618. https://doi.org/10.1016/j.matcom.2022.10.017 doi: 10.1016/j.matcom.2022.10.017
![]() |
[5] |
N. Liu, Z. Chen, G. Bao, Role of media coverage in mitigating COVID-19 transmission: Evidence from China, Technol. Forecast Soc. Change, 163 (2021), 120435. https://doi.org/10.1016/j.techfore.2020.120435 doi: 10.1016/j.techfore.2020.120435
![]() |
[6] | Shanghai Municipal Health Commission, News center [EB/OL], (2022). Available from: https://wsjkw.sh.gov.cn/xwfb/index.html (accessed March 15, 2023). |
[7] |
T. Hu, M. Zhang, A. Deng, Y. Zhang, B. Li, Y. Li, et al., Comparison of Omicron and Delta Variant Infection COVID-19 Cases — Guangdong Province, China, 2022, China CDC Weekly, 4 (2022), 385–388. https://doi.org/10.46234/ccdcw2022.087 doi: 10.46234/ccdcw2022.087
![]() |
[8] |
C. Xu, Z. Zhang, X. Huang, J. Cui, X. Han, The Dynamic Effects of Different Quarantine Measures on the Spread of COVID-19, JAAC, 12 (2022), 1532–1543. https://doi.org/10.11948/20210326 doi: 10.11948/20210326
![]() |
[9] | F. Brauer, C. Castillo-Chavez, Mathematical Models in Population Biology and Epidemiology, Springer-Verlag New York, New York, (2010). https://doi.org/10.1007/978-1-4614-1686-9 |
[10] |
J. Arino, F. Brauer, P. van den Driessche, J. Watmough, J. Wu, Simple models for containment of a pandemic, J. R Soc. Interface, 3 (2006), 453–457. https://doi.org/10.1098/rsif.2006.0112 doi: 10.1098/rsif.2006.0112
![]() |
[11] |
Y. Zou, W. Yang, J. Lai, J. Hou, W. Lin, Vaccination and quarantine effect on COVID-19 transmission dynamics incorporating Chinese-Spring-Festival travel rush: Modeling and simulations, B Math. Biol., 84 (2022), 30. https://doi.org/10.1007/s11538-021-00958-5 doi: 10.1007/s11538-021-00958-5
![]() |
[12] |
U. A. de León, E. Avila-Vales, K. L. Huang, Modeling COVID-19 dynamic using a two-strain model with vaccination, Chaos Soliton. Fract., 157 (2022), 111927. https://doi.org/10.1016/j.chaos.2022.111927 doi: 10.1016/j.chaos.2022.111927
![]() |
[13] |
H. Song, R Wang, S. Liu, Z. Jin, D. He, Global stability and optimal control for a COVID-19 model with vaccination and isolation delays, Results Phys., 42 (2022), 106011. https://doi.org/10.1016/j.rinp.2022.106011 doi: 10.1016/j.rinp.2022.106011
![]() |
[14] |
T. Krueger, K. Gogolewski, M. Bodych, A. Gambin, G. Giordano, S. Cuschieri, et al., Risk assessment of COVID-19 epidemic resurgence in relation to SARS-CoV-2 variants and vaccination passes, Commun. Med. (Lond), 2 (2022), 23. https://doi.org/10.1038/s43856-022-00084-w doi: 10.1038/s43856-022-00084-w
![]() |
[15] |
Europe must come together to confront omicron, Health professionals and researchers from across Europe, BMJ, 376 (2022), o90. http://dx.doi.org/10.1136/bmj.o90 doi: 10.1136/bmj.o90
![]() |
[16] |
R. Markovič, M. Šterk, M. Marhl, M. Perc, M. Gosak, Socio-demographic and health factors drive the epidemic progression and should guide vaccination strategies for best COVID-19 containment, Results Phys., 26 (2021), 104433. https://doi.org/10.1016/j.rinp.2021.104433 doi: 10.1016/j.rinp.2021.104433
![]() |
[17] |
E. Rashed, S. Kodera, A. Hirata, COVID-19 forecasting using new viral variants and vaccination effectiveness models, Int. J. Biomath., 2 (2022), 23. https://doi.org/10.1016/j.compbiomed.2022.105986 doi: 10.1016/j.compbiomed.2022.105986
![]() |
[18] |
L. Childs, D. W. Dick, Z. Feng, J. M. Heffernan, J. Li, G. Röst, Modeling waning and boosting of COVID-19 in Canada with vaccination, Epidemics, 39 (2022), 100583. https://doi.org/10.1016/j.epidem.2022.100583 doi: 10.1016/j.epidem.2022.100583
![]() |
[19] |
S. Y. Tchoumi, H. Rwezaura, J. M. Tchuenche, Dynamic of a two-strain COVID-19 model with vaccination, Results Phys., 39 (2022), 105777. https://doi.org/10.1016/j.rinp.2022.105777 doi: 10.1016/j.rinp.2022.105777
![]() |
[20] |
J. Zhang, Y. Qiao, Y. Zhang, Bifurcation analysis and optimal control of COVID-19 with exogenous reinfection and media coverages, Comput. Biol. Med., 16 (2023), 2250086. https://doi.org/10.1142/S1793524522500863 doi: 10.1142/S1793524522500863
![]() |
[21] |
Y. Xiao, S. Tang, J. Wu, Media impact switching surface during an infectious disease outbreak, Sci. Rep., 5 (2015), 7838. https://doi.org/10.1038/srep07838 doi: 10.1038/srep07838
![]() |
[22] |
K. Lata, A. K. Misra, Y. Takeuchi, Modeling the effectiveness of TV and social media advertisements on the dynamics of water-borne diseases, Int. J. Biomath., 15 (2022), 2150069. https://doi.org/10.1142/S1793524521500698 doi: 10.1142/S1793524521500698
![]() |
[23] |
J. Lv, S. Guo, J. Cui, J. P. Tian, Asymptomatic transmission shifts epidemic dynamics, Math. Biosci. Eng., 18 (2020), 92–111. https://doi.org/10.3934/mbe.2021005 doi: 10.3934/mbe.2021005
![]() |
[24] | National Health Commission of the People's Republic of China, [EB/OL], (2022), Available from: http://www.nhc.gov.cn/ (accessed March 15, 2023). |
1. | Shenxing Li, Wenhe Li, Onder Tutsoy, Dynamical behaviors of a stochastic SIVS epidemic model with the Ornstein-Uhlenbeck process and vaccination of newborns, 2024, 19, 1932-6203, e0310175, 10.1371/journal.pone.0310175 | |
2. | Lihua Dai, Xianning Liu, Yuming Chen, Global dynamics of a fractional-order SIS epidemic model with media coverage, 2023, 111, 0924-090X, 19513, 10.1007/s11071-023-08838-4 | |
3. | 江波 郝, Dynamic Analysis of Epidemic Models with Self-Protection and Isolation Measures, 2023, 12, 2324-7991, 4998, 10.12677/AAM.2023.1212491 | |
4. | Xinghua Chang, Jianrong Wang, Maoxing Liu, Xue Yan, An SIS infectious disease model with nonlinear incidence and disease awareness on complex networks, 2025, 196, 09600779, 116349, 10.1016/j.chaos.2025.116349 |
β1 | The force of infection for symptomatic | 7.6453×10−12 | Fit |
β2 | The force of infection for asymptomatic | 6.36×10−8 | Fit |
ρ | Vaccination coverage rate | 0.00112 | [6] |
α | Rate of losing vaccine induced immunity | 0.0056 | [5] |
τ | Removal rate of the incubation period population | 0.3125 | [7] |
q | Proportion of symptomatic infection | 0.7 | Assumed |
ε | Vaccine efficiency | 0.7323 | Fit |
θ1 | Isolation rate of asymptomatic infection | 0.702 | Fit |
θ2 | Isolation rate of symptomatic infection | 0.8 | [8] |
γ1 | Recovery rate of asymptomatic infection | 0.0714 | Assumed |
γ2 | Recovery rate of symptomatic infection | 0.033 | Assumed |
k | The behavior change constant | 2.2073×10−9 | Fit |
p(t) | The rate of wearing masks | 1 | [1] |
l | Recovery rate for quarantined | 114 | [8] |
β1 | The force of infection for symptomatic | 7.6453×10−12 | Fit |
β2 | The force of infection for asymptomatic | 6.36×10−8 | Fit |
ρ | Vaccination coverage rate | 0.00112 | [6] |
α | Rate of losing vaccine induced immunity | 0.0056 | [5] |
τ | Removal rate of the incubation period population | 0.3125 | [7] |
q | Proportion of symptomatic infection | 0.7 | Assumed |
ε | Vaccine efficiency | 0.7323 | Fit |
θ1 | Isolation rate of asymptomatic infection | 0.702 | Fit |
θ2 | Isolation rate of symptomatic infection | 0.8 | [8] |
γ1 | Recovery rate of asymptomatic infection | 0.0714 | Assumed |
γ2 | Recovery rate of symptomatic infection | 0.033 | Assumed |
k | The behavior change constant | 2.2073×10−9 | Fit |
p(t) | The rate of wearing masks | 1 | [1] |
l | Recovery rate for quarantined | 114 | [8] |