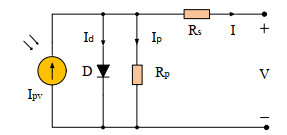
Photovoltaic (PV) parameter extraction plays a key role in establishing accurate and reliable PV models based on the manufacturer's current-voltage data. Owning to the characteristics such as implicit and nonlinear of the PV model, it remains a challenging and research-meaningful task in PV system optimization. Despite there are many methods that have been developed to solve this problem, they are often consuming a great deal of computing resources for more satisfactory results. To reduce computing resources, in this paper, an advanced differential evolution with search space decomposition is developed to effectively extract the unknown parameters of PV models. In proposed approach, a recently proposed advanced differential evolution algorithm is used as a solver. In addition, a search space decomposition technique is introduced to reduce the dimension of the problem, thereby reducing the complexity of the problem. Three different PV cell models are selected for verifying the performance of proposed approach. The experimental result is firstly compared with some representative differential evolution algorithms that do not use search space decomposition technique, which demonstrates the effectiveness of the search space decomposition. Moreover, the comparison results with some reported well-established parameter extraction methods suggest that the proposed approach not only obtains accurate and reliable parameters, but also uses the least computational resources.
Citation: Zhen Yan, Shuijia Li, Wenyin Gong. An adaptive differential evolution with decomposition for photovoltaic parameter extraction[J]. Mathematical Biosciences and Engineering, 2021, 18(6): 7363-7388. doi: 10.3934/mbe.2021364
[1] | Yujun Zhang, Yufei Wang, Shuijia Li, Fengjuan Yao, Liuwei Tao, Yuxin Yan, Juan Zhao, Zhengming Gao . An enhanced adaptive comprehensive learning hybrid algorithm of Rao-1 and JAYA algorithm for parameter extraction of photovoltaic models. Mathematical Biosciences and Engineering, 2022, 19(6): 5610-5637. doi: 10.3934/mbe.2022263 |
[2] | Juan Zhao, Yujun Zhang, Shuijia Li, Yufei Wang, Yuxin Yan, Zhengming Gao . A chaotic self-adaptive JAYA algorithm for parameter extraction of photovoltaic models. Mathematical Biosciences and Engineering, 2022, 19(6): 5638-5670. doi: 10.3934/mbe.2022264 |
[3] | Junhua Ku, Shuijia Li, Wenyin Gong . Photovoltaic models parameter estimation via an enhanced Rao-1 algorithm. Mathematical Biosciences and Engineering, 2022, 19(2): 1128-1153. doi: 10.3934/mbe.2022052 |
[4] | Lingyu Wu, Zixu Li, Wanzhen Ge, Xinchao Zhao . An adaptive differential evolution algorithm with elite gaussian mutation and bare-bones strategy. Mathematical Biosciences and Engineering, 2022, 19(8): 8537-8553. doi: 10.3934/mbe.2022396 |
[5] | Di-Wen Kang, Li-Ping Mo, Fang-Ling Wang, Yun Ou . Adaptive harmony search algorithm utilizing differential evolution and opposition-based learning. Mathematical Biosciences and Engineering, 2021, 18(4): 4226-4246. doi: 10.3934/mbe.2021212 |
[6] | Qishuo Pang, Xianyan Mi, Jixuan Sun, Huayong Qin . Solving nonlinear equation systems via clustering-based adaptive speciation differential evolution. Mathematical Biosciences and Engineering, 2021, 18(5): 6034-6065. doi: 10.3934/mbe.2021302 |
[7] | J. Ignacio Tello . On a mathematical model of tumor growth based on cancer stem cells. Mathematical Biosciences and Engineering, 2013, 10(1): 263-278. doi: 10.3934/mbe.2013.10.263 |
[8] | Ana I. Muñoz, José Ignacio Tello . Mathematical analysis and numerical simulation of a model of morphogenesis. Mathematical Biosciences and Engineering, 2011, 8(4): 1035-1059. doi: 10.3934/mbe.2011.8.1035 |
[9] | Shuaiqun Wang, Tianshun Zhang, Wei Kong, Gen Wen, Yaling Yu . An improved MOPSO approach with adaptive strategy for identifying biomarkers from gene expression dataset. Mathematical Biosciences and Engineering, 2023, 20(2): 1580-1598. doi: 10.3934/mbe.2023072 |
[10] | Xiao Chen, Zhaoyou Zeng . Bird sound recognition based on adaptive frequency cepstral coefficient and improved support vector machine using a hunter-prey optimizer. Mathematical Biosciences and Engineering, 2023, 20(11): 19438-19453. doi: 10.3934/mbe.2023860 |
Photovoltaic (PV) parameter extraction plays a key role in establishing accurate and reliable PV models based on the manufacturer's current-voltage data. Owning to the characteristics such as implicit and nonlinear of the PV model, it remains a challenging and research-meaningful task in PV system optimization. Despite there are many methods that have been developed to solve this problem, they are often consuming a great deal of computing resources for more satisfactory results. To reduce computing resources, in this paper, an advanced differential evolution with search space decomposition is developed to effectively extract the unknown parameters of PV models. In proposed approach, a recently proposed advanced differential evolution algorithm is used as a solver. In addition, a search space decomposition technique is introduced to reduce the dimension of the problem, thereby reducing the complexity of the problem. Three different PV cell models are selected for verifying the performance of proposed approach. The experimental result is firstly compared with some representative differential evolution algorithms that do not use search space decomposition technique, which demonstrates the effectiveness of the search space decomposition. Moreover, the comparison results with some reported well-established parameter extraction methods suggest that the proposed approach not only obtains accurate and reliable parameters, but also uses the least computational resources.
With the introduction of the concept of green economic development, the use of renewable energy has been paid great attention. The use of renewable energy can not only overcome the high cost of traditional fossil energy, but also reduce the negative environmental impact such as environmental pollution and global warming caused by traditional fossil energy [1,2,3]. In commonly used renewable energy, the solar energy has been considered as an option of clean energy because it is widely available, and it has no pollution and no noise [4]. In addition, an important reason is that the solar energy is able to be directly transformed into electricity by a PV device [5,6]. In this connection, it is worthwhile to mention that the performance of the PV system will be influenced by temperature and radiation. Therefore, it is fairly common to establish accurate and reliable PV models according to the manufacturer's current-voltage (I-V) data to predict and evaluate the performance of PV systems before being installed [7]. Until now, researchers have put forward to several PV models to fit the manufacturer's I-V data under different conditions. They mainly include the single diode model [8], the double diode model [9], and the triple diode model [10]. The single diode model is fairly simple and the double diode model is deemed as more accurate on accuracy when compared with the former. As for the triple diode model, it has ten unknown parameters which escalates the complexity [11]. Therefore, the first two PV models are most commonly used in practice [12]. Unfortunately, the parameter values in these PV models are not provided by manufacturers [13]. Thus, how to effectively extract the unknown parameter values of these PV models has become an urgent work.
In recent years, many efforts have been devoted to design effective parameter extraction methods. From the reported studies, these parameter extraction methods could be broadly classified into two types including deterministic methods and intelligent optimization methods. The deterministic method is a commonly used parameter extraction method in earlier years, representative as the Newton-Raphson methods [14] and Lambert W-function methods [15]. For such methods, they solve the problem mainly by analyzing the models equivalent circuit, deriving the relationship between unknown parameters. They can quickly obtain unknown parameters to be extracted, but often suffer from not high precision. Besides, it should be pointed out that the deterministic method has the following disadvantages: i) easy to trap into local optimum; ii) sensitive to provided initial solution; iii) have some extra requirements on the model equation, i.e., convexity and differentiability. Unfortunately, in this problem, the condition iii) is often not satisfied. With the rapid development of intelligent computing, more and more intelligent optimization methods inspired by nature are favored by many researchers. These methods have a simple structure and are easy to be coded. What should be pointed out is that these methods have no additional requirements for the characteristics of the model. Over the years, there are extensive intelligent optimization approaches that have been applied for extracting the parameters in PV models, such as pattern search (PS) [16], simulated annealing algorithm (SA) [17], genetic algorithm (GA) [18], harmony search (HS) [19], particle swarm optimization (PSO) [20,21], JAYA (JAYA) [22,23], differential evolution (DE) [24,25], whale optimization algorithm (WOA) [26,27], artificial bee swarm optimization (ABSO) [28], backtracking search algorithm (BSA) [29,30], cuckoo search (CS) [31], simplified swarm optimization (SSO) [32], teaching-learning-based optimization (TLBO) [33,34], fireworks algorithm (FA) [35], shuffled frog leaping algorithm (SFLA) [36,37], hybrid algorithms [38,39], and so on. It goes without saying that these methods have yielded considerable results when comparing with the deterministic methods. However, it must point out that the disadvantage of the intelligent optimization methods is that they require significant computational resources when it is employed for solving the PV parameters estimation problem. For example, in [26], the maximum consumed computing resource (the maximum number of objective function calls) is set to be 150,000; 1,500,000 in [27]; 100,000 in [40]; and 50,000 in [33,34]. Thus, it is worth further studying how to use as few computing resources as possible to obtain more accurate and reliable parameter values.
In this study, to fast, accurately, and reliably extract the parameter values in PV models with less computing resources, an advanced DE with search space decomposition is proposed. Firstly, a search space decomposition technique is presented, where the parameters of PV models have been decomposed into two parts i.e., linear part and nonlinear part. Subsequently, the advanced established adaptive differential evolution (EJADE) proposed by Li et al. [41] is employed for a general solver. EJADE is picked because it has good performance and no additional arithmetic parameters to set. The linear part parameter decomposed by the search space decomposition technique is determined by the algebraic method and the related nonlinear part parameter. While the nonlinear part parameter is solved by the intelligent optimization algorithm i.e., EJADE in this paper. Due to the proposed search space decomposition technique, the parameter to be extracted by EJADE is reduced. In consequence, significant computational resources can be saved when employing the intelligent optimization methods and thus the parameter extraction process can be greatly improved. The performance of proposed approach is tested on three PV cell models. Experimental results of proposed method are competitive with those of other state-of-the-art methods on accuracy and reliability with less computational resources.
The novelty and main contributions of this study can be listed as follows:
● An advanced differential evolution with search space decomposition algorithm is developed to effectively extract PV models parameters.
● The search space decomposition technique is proposed to reduce the search space and thus reduce the complexity of the problem.
● A lot of computing resources have been saved by adopting the proposed search space decomposition technique.
● The performance of proposed algorithm has been verified by extracting the unknown parameters in different PV cell models and a large number of reported well-established algorithms are selected as its competitors.
The structure of the rest of this paper is organized as follows. The description of PV modes is given in Section 2. Section 3 explains the proposed algorithm in detail. The experimental setting of this paper is given in Section 4, and Section 5 reports the results and gives the analysis on these results. Finally, the conclusion of this study is concluded in Section 6.
In this section, firstly, three different PV models are described. Then, the optimization objective of PV models is defined.
Figure 1 provides the equivalent circuit of the single diode model, where the output current I is defined as follows [18]:
I=Ipv−Id−Ip | (1) |
where Ipv is the photo-generated current, Id denotes the diode current, and the shunt resistor current is represented as Ip. Note that Id and Ip can be calculated as Eqs (2) and (3) by applying for Shockley equation and Kirchhoffs Voltage Law.
Id=Isd[exp((V+IRs)⋅qnkT)−1] | (2) |
Ip=V+IRsRp | (3) |
where Isd is the diode reverse saturation current, the output voltage is denoted as V, Rs and Rp are the series and shunt resistance, n denotes the non-physical diode ideality factor, q is the electron charge and its value is 1.60217646×10−19 C, k is the Boltzmann constant and its value is 1.3806503×10−23 J/K, and the temperature of junction in Kelvin is represented as T.
Based on above, the output current of this equivalent circuit can be represented as below:
I=Ipv−Isd[exp((V+IRs)⋅qnkT)−1]−V+IRsRp | (4) |
where it is straightforward to see that there are five unknown parameters i.e., Ipv, Isd, Rs, Rp, and n that need to be extracted.
From the equivalent circuit of the double diode model shown in Figure 2, it can be seen that there are two diodes in this model. The output current I is expressed as follows [18]:
I=Ipv−Id1−Id2−Ip | (5) |
where Id1 and Id2 are the first and second diode currents, respectively. Similar to Eq (2), Id1 and Id2 can be calculated using Eqs (6) and (7)
Id1=Isd1[exp((V+IRs)⋅qn1kT)−1] | (6) |
Id2=Isd2[exp((V+IRs)⋅qn2kT)−1] | (7) |
where Isd1 is the diffusion current, Isd2 is the saturation current, and the first and second non-physical diode ideality factors are denoted as n1, n2, respectively.
Thus, the output current of the equivalent circuit of the double diode model can be represented as below:
I=Ipv−Isd1[exp((V+IRs)⋅qn1kT)−1]−Isd2[exp((V+IRs)⋅qn2kT)−1]−V+IRsRp | (8) |
where it is clear that there are seven unknown parameters i.e., Ipv, Isd1, Isd2, Rs, Rp, n1, and n2 to be extracted in this model.
On the basis of the single diode model, researchers proposed a new model, namely the single based PV module. In Figure 3, the equivalent circuit of this model can be found, and the output current I can be calculated as follows [26]:
I=IpvNp−IsdNp[exp((VNp+IRsNs)⋅qnNsNpkT)−1]−VNp+IRsNsRpNs | (9) |
where Ns denotes the count of PV cells connected in series, and Np is the count of PV cells connected in parallel.
From Eq (9), five unknown parameters need to be extracted in the single based PV module like the single diode model.
To extract the parameters in different PV models in Eqs (4)–(9) according to the measured data provided by the manufacturers, an optimization objective function needs to be adopted when using intelligent optimization as solver. In this paper, like many published studies [9,16,18,19,22,26,28,29,33,34,36,42,43,44,45,46,47,48,49,50], the root mean square error (RMSE) is employed as the optimization objective, which can reflect the degree of dispersion of the measured data and the simulated data. And it is expressed as below:
minRMSE(x)=√1NN∑i=1(Ii−ˆIi)2 | (10) |
where x represents the parameter vector; for the single and the single based PV module, x=[Ipv,Isd,Rs,Rp,n]; x=[Ipv,Isd1,Isd2,Rs,Rp,n1,n2] for the double diode model. N is the total number of the measured I−V data provided by manufacturers. ˆI denotes the simulated current obtained by proposed approach.
In this section, we proposed our approach in detail. Firstly, the motivations of this study is described. Then the search space decomposition technique is proposed, and the advanced adaptive differential evolution is briefly introduced.
In recent years, more and more intelligent computing methods have been employed for parameter extraction of PV models. Although they have achieved better results to some extent, they need to consume a lot of computing resources. One obvious reason is that intelligent optimization algorithms need to extract all unknown parameters, which results in search space is very large. In consequence, a considerable number of computing resources is required for these algorithms. If we can reduce some unknown parameters, i.e., reduce the search space, and at this point the intelligent optimization algorithm is called, which will save a lot of computing resources. Taking this cue, we can observe Eqs (4), (8) and (9). We can observe that these unknown parameters can be divided into two groups: linear parameters and nonlinear parameters. For example, in the single diode model and the single based PV module, the linear parameters are Ipv, Isd, and Rp; and nonlinear parameters are Rs and n. In the double diode model, the linear parameters are Ipv, Isd1, Isd2, and Rp; and nonlinear parameters are Rs, n1, and n2. Based on the manufacturers measured data, the linear parameters can be determined by the nonlinear parameters.
Taking the above observations into consideration, the search space decomposition technique is proposed. Based on this decomposition technique, the linear parameters can be determined by the algebraic method while the intelligent optimization algorithms only extract the nonlinear parameters. As a result, a considerable number of computing resources can be saved.
By observing Eq (10), it can be reformulated as
minRMSE(x)=√1N∑Ni=1(Ii−ˆIi)T(Ii−ˆIi)subjecttof(x,Ii,Vi)−ˆIi=0i=1,⋯,N | (11) |
where
● Single diode model:
f(x,Ii,Vi)=Ipv−Isd[exp((V+IiRs)⋅qnkT)−1]−V+IiRsRp | (12) |
● Double diode model:
f(x,Ii,Vi)=Ipv−Isd1[exp((V+IiRs)⋅qn1kT)−1]−Isd2[exp((V+IiRs)⋅qn2kT)−1]−V+IiRsRp | (13) |
● Single diode based PV module:
f(x,Ii,Vi)=IpvNp−IsdNp[exp((VNp+IiRsNs)⋅qnNsNpkT)−1]−VNp+IiRsNsRpNs | (14) |
According to generalized Benders-like decomposition [51], the parameter vector x in Eq (11) can be decomposed two sub-parameter vector x1 and x2:
x1=[n,Rs]
x2=[Ipv,Isd,Rp]
Then, the nested form of Eq (11) can be defined as:
minx1,ˆIRMSE(x)=√1N∑Ni=1(Ii−ˆIi)T(Ii−ˆIi)+φ(x1,ˆI)subjecttof(x1,x2,Ii)−ˆIi=0i=1,⋯,N | (15) |
where
φ(x1,ˆI)=minx2,ˆI√1NN∑i=1(Ii−ˆIi)T(Ii−ˆIi) | (16) |
It is postulated that x1 is extracted by the intelligent optimization algorithm, then, the linear parameters x2 can be extracted by solving the corresponding normal equation, which can be expressed as follows:
B(x1)⏞[ETIMTIQTI]=A(x1)⏞[ETEETMETQMTEMTMMTQQTEQTMQTQ]x2⏞[IpvIsdRp′] | (17) |
where Rp′=1/Rp; E, M, and Q are vectors of size N, in which E is a unit vector. In addition, the elements in M and Q are calculated by the following:
● Single diode model
{Mi=Mi(x1)=−[exp((V+IiRs)⋅qnkT)−1]Qi=Qi(x1)=−(V+IiRs) | (18) |
● Single diode based PV module
{Mi(x1)=−[exp((V+IRsNs)⋅qaNskT)−1]Qi(x1)=−V+IRsNsNs | (19) |
According to Eq (17), it can be seen that once the value of the nonlinear parameter vector x1 is given, the linear parameter vector x2 can be extracted by Eq (20).
x2=A−1(x1)B(x1) | (20) |
In the double diode model, similar to the single diode model, the parameter vector x in Eq (11) can also be decomposed into nonlinear parameters x1 and linear parameters x2, which is expressed as follows:
x1=[n1,n2,Rs]
x2=[Ipv,Isd1,Isd2,Rp]
Like the single diode model and the single based PV module, assume that x1 is provided by an intelligent optimization algorithm, then the linear parameters x2 can be determined as below:
B(x1)⏞[ETIMTIQTIOTI]=A(x1)⏞[ETEETMETQETOMTEMTMMTQMTOQTEQTMQTQQTOOTEOTMOTQOTO]x2⏞[IpvIsd1Isd2Rp′] | (21) |
where the elements in M, Q, and O are calculated by the following:
{Mi=Mi(x1)=−[exp((V+IiRs)⋅qn1kT)−1]Qi=Qi(x1)=−[exp((V+IiRs)⋅qn2kT)−1]Oi=Oi(x1)=−(V+IiRs) | (22) |
It is worth mentioning that through this decomposition technique, there may be two scenarios:
1) The matrix A in Eqs (17) and (21) may not be inverse. If this happens, we will discard the values of this set of nonlinear parameters x1 to ensure that the inversion of matrix A exists.
2) The other is that the linear parameters in x2 determined by x1 may violate the upper and lower bounds. When this happens, then we truncate to its specified range.
Recently, a large number of differential evolution algorithms [52,53,54,55,56] have been proposed to solve various practical problems. Adaptive differential evolution algorithm (JADE) as an effective and efficient algorithm was proposed by Zhang et al in [52] to solve continuous optimization problem. In order to leverage this algorithm to deal with the PV problem. Li et al. [41] proposed an enhanced adaptive differential evolution algorithm (EJADE). From the perspective of experimental results on PV models parameter extraction, EJADE shows remarkably performance when compared with reported parameter extraction methods. Therefore, in this work, the EJADE algorithm is selected as the intelligent optimization solver. For an overview of the algorithm, the reader is referred to [41].
In this paper, the proposed search space decomposition technique is infused in the EJADE algorithm. For convenience, we refer to the algorithm simply as EJADE-D. Figure 4 provides the flowchart of the proposed EJADE-D. Its main steps are described in Table 1:
Step 1: | Firstly, load the measured I-V data of PV models provided by the manufacturers. |
Step 2: | Randomly initialize NP (population size) solutions of population, in which each solution Xi=x1 is initialized in their specified range. |
Step 3: | According to each solution, calculate the linear unknown parameters x2 by using the proposed search space decomposition technique i.e., Eqs (20) and (21). |
Step 4: | After all solution have been done, then, each solution's linear parameters x2 and nonlinear parameters x1 are substituted into Eq (10) to calculate the objective function value of each solution. |
Step 5: | Leverage the advanced differential evolution algorithm i.e., EJADE to produce NP new solution. |
Step 6: | Similar to the Step 3, calculate the linear parameters x2 according related nonlinear parameters x1 for each new solution by using search space decomposition technique. |
Step 7: | Calculate the objection function value of each solution based on its linear parameters x2 and corresponding nonlinear parameters x1. |
Step 8: | According to the objective function value, selection between the new solution and old solution to form the next generation population. If the termination condition is met, then go to Step 9; else go to the loop: Steps 5–8. |
Step 9: | Output the best solution. |
To verify the performance of proposed EJADE-D, three different PV models have been tested on four PV data. For the first two PV models, the 57 mm diameter commercial R.T.C France solar cell is selected. While for the single based PV module, two different solar cells, i.e., mono-crystalline STM6-40/36 and ploy-crystalline STP6-120/36 are selected. The measured I-V data are obtained from [14,34,57]. The detailed description about these PV data is provided in Table 2. In addition, the upper and lower bound of unknown parameters of these solar cells are given in Table 3, which is similar to several published literature [16,18,22,34,42,45].
Parameter | The single/double diode model | The single based PV module | |
Solar cell model | R.T.C. France solar cell | STM6-40/36 | STP6-120/36 |
Ns | 1 | 36 | 36 |
Np | 1 | 1 | 1 |
Number of I-V data | 26 | 20 | 24 |
Temperature | 25 ℃ | 51 ℃ | 55 ℃ |
Radiation | 1000 W/m2 | 1000 W/m2 | 1000 W/m2 |
Unknown parameters | R.T.C. France solar cell | STM6-40/36 | STP6-120/36 | |||||
Lower | Upper | Lower | Upper | Lower | Upper | |||
Ipv (A) | 0 | 1 | 0 | 2 | 0 | 8 | ||
Isd, Isd1, Isd2 (μA) | 0 | 1 | 0 | 50 | 0 | 50 | ||
Rs (Ω) | 0 | 0.5 | 0 | 0.36 | 0 | 0.36 | ||
Rp (Ω) | 0 | 100 | 0 | 1000 | 0 | 1500 | ||
n, n1, n2 | 1 | 2 | 1 | 60 | 1 | 50 |
In order to demonstrate the effectiveness of the proposed adaptive differential evolution with search space decomposition algorithm, basic differential evolution (DE) [58], Zhang's adaptive differential evolution (JADE) [52], and EJADE [41] have been selected for comparison. The arithmetic parameters setting of the selected algorithms are shown in Table 4. Additionally, what should be pointed out is that the maximum number of function evaluations (Max_NFE) is set to 2000 for the single diode model, 4000 for the double diode model, 3000, and 7000 for the STM6-40/36, and STP6-120/36, respectively. Note that all algorithms are coded in Matlab and independently executed 30 runs. Besides, the experiment environment is on a desktop PC with an Intel Core i7-9700 processor @ 3.0 GHz, 32GB RAM, under the Windows 10 64-bit OS.
Algorithm | Parameter value |
DE | NP = 50, F = 0.5, CR = 0.9 |
JADE | NP = 50 |
EJADE | NPmax = 50, NPmin = 4 |
EJADE-D | NPmax = 50, NPmin = 4 |
In this section, we first analyze the results including the extracted parameter values, statistical results and convergence speed of different DEs algorithms. Then the result achieved by proposed EJADE-D is compared with other state-of-the-art algorithms.
For the single diode model, the statistical results obtained by DE, JADE, EJADE, and proposed EJADE-D are reported in Table 5, where the best RMSE (Best), worst RMSE (Worst), mean RMSE (Mean), and standard deviation (Std) for 30 independent runs are included. From Table 5, it is clear that DE, JADE and EJADE were unable to find the best RMSE value (9.8602E-04) at 2000 function evaluations. In addition, from other statistical analysis indicators, what can be observed is that only EJADE-D algorithm is able to achieve the best performance on the Worst, Mean, and Std RMSE values. Besides, it is worth mentioning that the Std of EJADE-D is very small (3.67E-17) when compared with its competitors, which means that the proposed algorithm has an excellent robustness.
Algorithm | RMSE | |||
Best | Worst | Mean | Std | |
DE | 1.3733E-03 | 4.3933E-03 | 2.2122E-03 | 6.30E-04 |
JADE | 2.4628E-03 | 9.0467E-03 | 4.6089E-03 | 1.64E-03 |
EJADE | 9.9336E-04 | 2.1651E-03 | 1.4245E-03 | 3.03E-04 |
EJADE-D | 9.8602E-04 | 9.8602E-04 | 9.8602E-04 | 3.67E-17 |
Table 6 provides the best RMSE values and corresponding extracted parameter values achieved by compared algorithms. In order to better explain the accuracy of the extracted parameters, we put these parameter values into the objective function to calculate the experimental current called the simulated current data. Figure 5(a) shows the fitting curve of the measured current data and the simulated current data obtained from the parameters provided by EJADE-D. From Figure 5(a), what can be clearly seen is that the simulated current data can fit the measured current data well, which proves that the extracted parameter values provided by EJADE-D are very accurate. In addition, we also draw the current absolute value error* of different algorithms in Figure 5(b), where it can be observed that the current absolute value error of EJADE-D is small at each data point while DE and JADE are significantly inferior to EJADE and EJADE-D.
* which is the absolute of the measured current data and the simulated current data.
Algorithm | Ipv (A) | Isd (μA) | Rs (Ω) | Rp (Ω) | n | RMSE |
DE | 0.75946361 | 0.41068723 | 0.03569428 | 86.22084780 | 1.50561834 | 1.3733E-03 |
JADE | 0.76241894 | 0.65695921 | 0.03323643 | 80.94523627 | 1.55621196 | 2.4628E-03 |
EJADE | 0.76070190 | 0.34171649 | 0.03613743 | 55.62560314 | 1.48687849 | 9.9336E-04 |
EJADE-D | 0.76077553 | 0.32302079 | 0.03637709 | 53.71852020 | 1.48118359 | 9.8602E-04 |
Finally, in order to show that the proposed search space decomposition technique can accelerate convergence, Figure 6 plots the convergence speed of different compared algorithms on this model. What can be observed from this figure is that EJADE-D has the fastest convergence speed. Besides, DE and JADE do not converge to the best RMSE value until all function evaluations have been consumed. Based on these, it can be concluded that the search space decomposition technique can be used to save the computing resources.
For this model, we adopt the same experimental data as the single diode model. Different from the latter, the double diode model has seven parameters (Ipv, Isd1, Isd2, Rs, Rp, n1, and n2) that need to be extracted. Two more parameters complicate the problem. Table 7 gives the statistical results achieved by different algorithms, where it could be seen that EJADE-D achieves the best RMSE value (9.8248E-04), followed by EJADE (9.8497E-04), JADE (2.2919E-03), and DE (1.4793E-03). From the Worst, Mean, and Std of the RMSE value, EJADE-D exhibits remarkable performance. Additionally, the best RMSE value and corresponding extracted parameter values of different algorithms are reported in Table 8. Thereby, these extracted parameter values are put into the objective function to get the simulated current data. Figure 7 gives the comparisons between the measured current data and simulated current data on the double diode model. From Figure 7(a), it is evident that the simulated data obtained by EJADE-D is highly consistent with the measured current data, which demonstrates that the parameters extracted by EJADE-D on this model are also very accurate. Moreover, from the current absolute value error shown as Figure 7(b), EJADE-D has the smallest current absolute error, followed by EJADE, DE, and JADE. It is worthwhile to mention that DE obtains better performance than JADE on this model.
Algorithm | RMSE | |||
Best | Worst | Mean | Std | |
DE | 1.4793E-03 | 4.5821E-03 | 2.4962E-03 | 6.93E-04 |
JADE | 2.2919E-03 | 5.1370E-03 | 3.4453E-03 | 7.37E-04 |
EJADE | 9.8497E-04 | 2.0383E-03 | 1.2113E-03 | 2.83E-04 |
EJADE-D | 9.8248E-04 | 9.8248E-04 | 9.8248E-04 | 5.50E-17 |
Algorithm | Ipv (A) | Isd1 (μA) | Rs (Ω) | Rp (Ω) | n1 | Isd2 (μA) | n2 | RMSE |
DE | 0.76161938 | 0.29934314 | 0.03458265 | 62.11841082 | 1.95055472 | 0.44875729 | 1.51798488 | 1.4793E-03 |
JADE | 0.75904695 | 0.13872632 | 0.03655444 | 47.70175773 | 1.43701409 | 0.16306212 | 1.52688650 | 2.2919E-03 |
EJADE | 0.76077378 | 0.24691111 | 0.03653971 | 54.42053982 | 1.46090798 | 0.21482898 | 1.76404886 | 9.8497E-04 |
EJADE-D | 0.76078108 | 0.22597441 | 0.03674043 | 55.48543767 | 1.45101682 | 0.74934630 | 2.00000000 | 9.8248E-04 |
Figure 8 shows the convergence curves of EJADE-D and its competitors. From the convergence curves, we can clearly see that DE, JADE, and EJADE converge faster than EJADE-D before NFE=1000, but after 1000, EJADE-D quickly converges to the best RMSE value (9.8248E-04). The reason is that EJADE-D needs a certain number of function evaluation times to find the better nonlinear unknown parameters in PV models, once these better solutions are found, the use of search space decomposition technique will quickly find the linear unknown parameters. Therefore, EJADE-D will converge quickly in the later period.
For the mono-crystalline STM6-40/36, the statistical results are given in Table 9, where it can be clearly seen that EJADE-D shows remarkable performance on the Best, Worst, Mean, and Std RMSE values, especially in Std (9.85E-18) when compared with other algorithms. Table 10 reports the extracted parameters values obtained by different methods, and Figure 9 provides the comparisons between the measured current data and simulated current data. From this figure, two points can be observed: i) the simulated current data achieved by EJADE-D are consistent with the measured current data; ii) the proposed EJADE-D has the smallest current absolute value error at each measured data. Note that the current absolute value error of DE at the last point is relatively large, which may be due to inaccurate extraction parameters. In addition, the convergence curve of compared algorithms on this model is drawn in Figure 10, in which it can be obviously observed that EJADE-D exhibits very competitive performance on the convergence speed. Besides, another fact worth saying is that other DEs methods are not able to converge to the best RMSE value (1.7298E-03).
Algorithm | RMSE | |||
Best | Worst | Mean | Std | |
DE | 1.0448E-02 | 2.5021E-01 | 7.4874E-02 | 6.90E-02 |
JADE | 5.6988E-03 | 1.3783E-02 | 7.5998E-03 | 1.71E-03 |
EJADE | 2.6987E-03 | 4.0918E-03 | 3.3056E-03 | 2.78E-04 |
EJADE-D | 1.7298E-03 | 1.7298E-03 | 1.7298E-03 | 9.85E-18 |
Algorithm | Ipv (A) | Isd (μA) | Rs (Ω) | Rp (Ω) | n | RMSE |
DE | 1.65602020 | 7.78528613 | 0.00090772 | 317.05546222 | 1.70743127 | 1.0448E-02 |
JADE | 1.65376894 | 5.35917258 | 0.00130049 | 289.11110965 | 1.65342824 | 5.6988E-03 |
EJADE | 1.66220446 | 3.98041243 | 0.00125715 | 20.23972593 | 1.61727931 | 2.6987E-03 |
EJADE-D | 1.66390478 | 1.73865681 | 0.00427377 | 15.92829378 | 1.52030292 | 1.7298E-03 |
The statistical results of 30 independent runs on the ploy-crystalline STP6-120/36 PV module are shown in Table 11, where it is obvious that EJADE and EJADE-D achieve the best RMSE value (1.6602E-02), followed by DE (2.2855E-02), and JADE (3.2110E-02). However, for the other evaluation indicators, proposed EJADE-D also provides the best result. And the best RMSE value obtained by different algorithms and its corresponding parameter values are reported in Table 12. In addition, from Figure 11(a), it can be obviously observed that the simulated data obtained by EJADE-D agree well with the measured data, which also reflects the accuracy of the extracted parameter values in this model. Besides, the current absolute value error of EJADE-D fluctuates less than other algorithms shown as Figure 11(b).
Algorithm | RMSE | |||
Best | Worst | Mean | Std | |
DE | 2.2855E-02 | 2.4084E-01 | 5.7271E-02 | 5.53E-02 |
JADE | 3.2110E-02 | 2.5739E-01 | 4.8897E-02 | 3.97E-02 |
EJADE | 1.6601E-02 | 4.0248E-02 | 1.7799E-02 | 4.27E-03 |
EJADE-D | 1.6601E-02 | 1.6601E-02 | 1.6601E-02 | 1.55E-16 |
Algorithm | Ipv (A) | Isd (μA) | Rs (Ω) | Rp (Ω) | n | RMSE |
DE | 7.48185880 | 6.48830083 | 0.00406738 | 1124.25574692 | 1.35185994 | 2.2855E-02 |
JADE | 7.48402233 | 1.15549180 | 0.00369744 | 1359.20725027 | 1.41018636 | 3.2110E-02 |
EJADE | 7.47249358 | 2.33478056 | 0.00459468 | 22.26217949 | 1.26009565 | 1.6601E-02 |
EJADE-D | 7.47252992 | 2.33499494 | 0.00459463 | 22.21989617 | 1.26010347 | 1.6601E-02 |
Figure 12 gives the convergence curves obtained by different methods on the STP6-120/36, where it is evident that EJADE-D also has the fastest convergence speed, about NFE=2000. Similar to the previous three models, DE and JADE can not converge to the best RMSE value (1.6602E-02) until all function evaluations have been consumed.
Based on above, the efficiency of proposed EJADE-D has been verified. In this section, in order to further illustrate the superiority of the EJADE-D algorithm, the result of EJADE-D has been compared with other reported well-established algorithms. Note that these algorithms are chosen because they have achieved better results and are recently proposed. These compared algorithms are generalized oppositional TLBO (GOTLBO) [47], self-adaptive TLBO (SATLBO) [33], improved JAYA (IJAYA) [45], hybrid TLBO and ABC (TLABC) [48], multiple learning BSA (MLBSA) [29], hybrid DE and WOA (DE/WOA) [59], opposition-based WOA (OBWOA) [27], improved TLBO (ITLBO) [34], performance-guided JAYA (PGJAYA) [22], hybrid BH and CS (BHCS) [38], flexible PSO (FPSO) [60], improved Lozi map based COA (ILCOA) [61], BSA with reusing differential vectors (BSARDVs) [30], enhanced Lˊevy flight bat algorithm (ELBA) [62], either-or TLBO (ELTLBO) [63], comprehensive learning JAYA (CLJAYA) [4], BSA with competitive learning (CBSA) [64], hybrid adaptive TLBO and DE (ATLDE) [39], enhanced JAYA (EJAYA) [23], improved gaining-sharing knowledge algorithm (IGSK) [65], enhanced adaptive butterfly optimization algorithm (EABOA) [66], shuffled frog leaping with memory pool (SFLBS) [67], reinforcement learning-based DE (RLDE) [68], chaotic WOA (CWOA) [26], modified simplified swarm optimization (MSSO) [32], improved WOA (IWOA) [40], hybrid FA and PS (HFAPS) [35], similarity-guided DE (SGDE) [24], classified perturbation mutation PSO (CPMPSO) [20], niche-based PSO with parallel computing (NPSOPC) [21]. The comparison results on different PV models are reported in Tables 13–16. Note that "NA" in these tables means that the result is not available in the reported literature. From the results, it can be observed that:
Algorithm | RMSE | NFEs | |||
Best | Worst | Mean | Std | ||
GOTLBO (2016) | 9.8744E-04 | 1.9824E-03 | 1.3349E-03 | 2.09E-04 | 10,000 |
SATLBO (2017) | 9.8602E-04 | 9.9494E-03 | 9.8780E-04 | 2.30E-06 | 50,000 |
IJAYA (2017) | 9.8603E-04 | 1.0622E-03 | 9.9204E-04 | 1.40E-05 | 50,000 |
TLABC (2018) | 9.8602E-04 | 1.0397E-03 | 9.9852E-04 | 1.86E-05 | 50,000 |
MLBSA (2018) | 9.8602E-04 | 9.8602E-04 | 9.8602E-04 | 9.15E-12 | 50,000 |
DE/WOA (2018) | 9.8602E-04 | 9.8602E-04 | 9.8602E-04 | 3.55E-17 | 50,000 |
OBWOA (2018) | 9.8602E-04 | NA | 9.8603E-03 | 1.02E-08 | 1,500,000 |
ITLBO (2019) | 9.8602E-04 | 9.8602E-04 | 9.8602E-04 | 2.19E-17 | 50,000 |
PGJAYA (2019) | 9.8602E-04 | 9.8603E-04 | 9.8602E-04 | 1.45E-09 | 50,000 |
BHCS (2019) | 9.8602E-04 | 9.8602E-04 | 9.8602E-04 | 2.61E-17 | 50,000 |
FPSO (2019) | 9.8602E-04 | NA | NA | NA | NA |
ILCOA (2019) | 9.8602E-04 | NA | NA | 1.01E-08 | 10,000*NP |
BSARDVs (2020) | 9.8602E-04 | NA | NA | NA | 25,000 |
ELBA (2020) | 9.8602E-04 | 9.8602E-04 | 9.8602E-04 | 1.97E-17 | 50,000 |
EOTLBO (2020) | 9.8602E-04 | 9.8602E-04 | 9.8602E-04 | 4.13E-17 | 20,000 |
CLJAYA (2020) | 9.8602E-04 | NA | NA | NA | 20,000 |
CBSA (2020) | 9.8602E-04 | NA | NA | NA | 25,000 |
IEO (2020) | 9.8602E-04 | NA | NA | NA | 1,500,000 |
ATLDE (2020) | 9.8602E-04 | 9.8602E-04 | 9.8602E-04 | 2.44E-17 | 30,000 |
EJAYA (2021) | 9.8602E-04 | 9.8602E-04 | 9.8602E-04 | 6.80E-17 | 30,000 |
IGSK (2021) | 9.8602E-04 | 9.8602E-04 | 9.8602E-04 | 3.58E-17 | 10,000 |
EABOA (2021) | 9.8602E-04 | 9.8784E-04 | 9.8678E-04 | 9.30E-07 | 50,000 |
SFLBS (2021) | 9.8602E-04 | 9.8602E-04 | 9.8602E-04 | 1.43E-14 | 60,000 |
RLDE (2021) | 9.8602E-04 | 9.8602E-04 | 9.8602E-04 | 3.48E-17 | 30,000 |
EJADE-D | 9.8602E-04 | 9.8602E-04 | 9.8602E-04 | 3.67E-17 | 2000 |
Algorithm | RMSE | NFEs | |||
Best | Worst | Mean | Std | ||
GOTLBO (2016) | 9.8318E-04 | 1.7877E-03 | 1.2436E-03 | 2.09E-04 | 20,000 |
SATLBO (2017) | 9.8280E-04 | 1.0470E-03 | 9.9811E-04 | 1.95E-05 | 50,000 |
IJAYA (2017) | 9.8293E-04 | 1.4055E-03 | 1.0269E-03 | 9.83E-05 | 50,000 |
TLABC (2018) | 9.8415E-04 | 1.5048E-03 | 1.0555E-03 | 1.55E-04 | 50,000 |
MLBSA (2018) | 9.8249E-04 | 9.8798E-04 | 9.8518E-04 | 1.35E-06 | 50,000 |
DE/WOA (2018) | 9.8248E-04 | 9.8604E-04 | 9.8297E-04 | 9.15E-07 | 50,000 |
OBWOA (2018) | 9.8251E-04 | NA | 9.8294E-04 | 1.13E-07 | 1,500,000 |
PGJAYA (2019) | 9.8263E-04 | 9.9499E-04 | 9.8582E-04 | 2.54E-06 | 50,000 |
BHCS (2019) | 9.8249E-04 | 9.8687E-04 | 9.8380E-04 | 1.54E-06 | 50,000 |
FPSO (2019) | 9.8253E-04 | NA | NA | NA | NA |
ILCOA (2019) | 8.8257E-04 | NA | NA | 6.25E-07 | 10,000*NP |
ITLBO (2019) | 9.8248E-04 | 9.8812E-04 | 9.8497E-04 | 1.54E-06 | 50,000 |
BSARDVs (2020) | 9.8248E-04 | NA | NA | NA | 45,000 |
ELBA (2020) | 9.8248E-04 | 9.8615E-04 | 9.8349E-04 | 1.43E-06 | 50,000 |
EOTLBO (2020) | 9.8248E-04 | 9.8942E-04 | 9.8473E-04 | 1.69E-06 | 20,000 |
CLJAYA (2020) | 9.8249E-04 | NA | NA | NA | 48,000 |
CBSA (2020) | 9.8248E-04 | NA | NA | NA | 50,000 |
IEO (2020) | 9.8248E-04 | NA | NA | NA | 1,500,000 |
ATLDE (2020) | 9.8248E-04 | 9.8603E-04 | 9.8372E-04 | 1.37E-06 | 30,000 |
EJAYA (2021) | 9.8248E-04 | 9.8602E-04 | 9.8448E-04 | 1.51E-06 | 30,000 |
IGSK (2021) | 9.8248E-04 | 9.8602E-04 | 9.8273E-04 | 8.96E-07 | 20,000 |
EABOA (2021) | 9.8607E-04 | 1.0012E-03 | 9.9190E-04 | 6.62E-06 | 50,000 |
SFLBS (2021) | 9.8249E-04 | 9.8787E-04 | 9.8541E-04 | 1.79E-06 | 60,000 |
RLDE (2021) | 9.8248E-04 | 9.8695E-04 | 9.8457E-04 | 1.75E-06 | 30,000 |
EJADE-D | 9.8248E-04 | 9.8248E-04 | 9.8248E-04 | 5.50E-17 | 4,000 |
Algorithm | RMSE | NFEs | |||
Best | Worst | Mean | Std | ||
BHCS (2019) | 1.7298E-03 | 3.3299E-03 | 1.8365E-03 | 4.06E-04 | 50,000 |
ITLBO (2019) | 1.7298E-03 | 1.7298E-03 | 1.7298E-03 | 4.75E-18 | 50,000 |
ELBA (2020) | 1.7298E-03 | 1.7298E-03 | 1.7298E-03 | 6.16E-18 | 50,000 |
IEO (2020) | 1.7298E-03 | NA | NA | NA | 1,500,000 |
ATLDE (2020) | 1.7298E-03 | 1.7298E-03 | 1.7298E-03 | 8.22E-18 | 30,000 |
EJAYA (2021) | 1.7298E-03 | 1.7298E-03 | 1.7298E-03 | 1.47E-17 | 30,000 |
IGSK (2021) | 1.7298E-03 | 1.7298E-03 | 1.7298E-03 | 7.02E-18 | 15,000 |
RLDE (2021) | 1.7298E-03 | 1.7298E-03 | 1.7298E-03 | 1.58E-17 | 30,000 |
EJADE-D | 1.7298E-03 | 1.7298E-03 | 1.7298E-03 | 9.85E-18 | 3,000 |
Algorithm | RMSE | NFEs | |||
Best | Worst | Mean | Std | ||
BHCS (2019) | 1.6601E-02 | 1.3482E-01 | 2.4360E-02 | 2.61E-02 | 50,000 |
ITLBO (2019) | 1.6601E-02 | 1.6601E-02 | 1.6601E-02 | 7.22E-17 | 50,000 |
IEO (2020) | 1.6601E-02 | NA | NA | NA | 1,500,000 |
ATLDE (2020) | 1.6601E-02 | 1.6601E-02 | 1.6601E-02 | 1.02E-16 | 30,000 |
EJAYA (2021) | 1.6601E-02 | 1.6601E-02 | 1.6601E-02 | 2.68E-16 | 30,000 |
IGSK (2021) | 1.6601E-02 | 1.6601E-02 | 1.6601E-02 | 1.71E-16 | 15,000 |
RLDE (2021) | 1.6601E-02 | 1.6601E-02 | 1.6601E-02 | 1.98E-16 | 30,000 |
EJADE-D | 1.6601E-02 | 1.6601E-02 | 1.6601E-02 | 1.55E-16 | 7000 |
● For the single diode model, most reported algorithms can achieve the best RMSE value (9.8602E-04). However, for the Worst, Mean, and Std, only DE/WOA, ITLBO, BHCS, ELBA, EOTLBO, ATLDE, EJAYA, IGSK, RLDE, and EJADE-D are able to obtain the best result. Besides, from the perspective of the computing resources consumed, it is straightforward to see that the proposed EJADE-D only consumes 2000 objective function evaluations, while 10,000 for IGSK, 20,000 for EOTLBO, 30,000 for ATLDE, EJAYA, and RLDE, 50,000 for DE/WOA, ITLBO, BHCS, and ELBA.
● For the double diode model, it is clear that DE/WOA, ITLBO, BSARDVs, ELBA, EOTLBO, CBSA, IEO, ATLDE, EJAYA, IGSK, and RLDE provide the same RMSE value (9.8248E-04). Unlike the single diode model, in this model, only proposed EJADE-D can achieve the best result in terms of the Worst, Mean, and Std metrics, which shows that EJADE-D has great advantages in accuracy and reliability on this model. In addition, EJADE-D also consumes the fewest NFEs, using only 4000.
● For the single based PV model, almost all recently proposed algorithms can get the best RMSE values, i.e., 1.7298E-03 for STM6-40/36 and 1.6601E-02 for STP6-120/36. Moreover, except the BHCS and IEO, the proposed EJADE-D and other algorithms can obtain a very good statistical results. However, EJADE-D consumes less computing resources, only 3000 NFEs for the STM6-40/36 and 7000 NFEs for the STP6-120/36.
In summary, the EJADE-D algorithm proposed in this paper can provide better, or at least comparably results, in terms of the accuracy and reliability of the extracted parameter values when compared with most reported well-established parameter extraction algorithms. However, it is worthwhile to mention that among all the compared algorithms, only the proposed EJADE-D uses the least computational resources. In addition, the best RMSE value obtained by different compared algorithms and its corresponding parameter values on different PV models are reported in Tables 17–20, which makes it easier for researchers to find some relevant extracted parameters data.
Algorithm | Ipv (A) | Isd (μA) | Rs (Ω) | Rp (Ω) | n | RMSE |
GOTLBO (2016) | 0.7608 | 0.3316 | 0.0363 | 54.1154 | 1.4838 | 9.8744E-04 |
IJAYA (2017) | 0.7608 | 0.3228 | 0.0364 | 53.7595 | 1.4811 | 9.8603E-04 |
SATLBO (2017) | 0.7608 | 0.3232 | 0.0363 | 53.7256 | 1.4812 | 9.8602E-04 |
CWOA (2017) | 0.76077 | 0.3239 | 0.03636 | 53.7987 | 1.4812 | 9.8602E-04 |
MSSO (2017) | 0.760777 | 0.323564 | 0.036370 | 53.742465 | 1.481244 | 9.8607E-04 |
IWOA (2018) | 0.7608 | 0.3232 | 0.0364 | 53.7317 | 1.4812 | 9.8602E-04 |
HFAPS (2018) | 0.760777 | 0.322622 | 0.0363819 | 53.6784 | 1.48106 | 9.8602E-04 |
TLABC (2018) | 0.76078 | 0.32302 | 0.03638 | 53.71636 | 1.48118 | 9.8602E-04 |
MLBSA (2018) | 0.7608 | 0.32302 | 0.0364 | 53.7185 | 1.4812 | 9.8602E-04 |
DE/WOA (2018) | 0.760776 | 0.323021 | 0.036377 | 53.718524 | 1.481184 | 9.8602E-04 |
OBWOA (2018) | 0.76077 | 0. 3232 | 0. 0363 | 53.6836 | 1.5208 | 9.8602E-04 |
PGJAYA (2019) | 0.7608 | 0.3230 | 0.0364 | 53.7185 | 1.4812 | 9.8602E-04 |
BHCS (2019) | 0.76078 | 0.32302 | 0.03638 | 53.71852 | 1.48118 | 9.8602E-04 |
FPSO (2019) | 0.76077552 | 0.323020 | 0.036370 | 53.718520 | 1.48110817 | 9.8602E-04 |
ILCOA (2019) | 0.760775 | 0.323021 | 0.036377 | 53.718679 | 1.481108 | 9.8602E-04 |
ITLBO (2019) | 0.7608 | 0.3230 | 0.0364 | 53.7185 | 1.4812 | 9.8602E-04 |
BSARDVs (2020) | 0.760776 | 0.323021 | 0.036377 | 53.718520 | 1.481184 | 9.8602E-04 |
ELBA (2020) | 0.760776 | 0.323021 | 0.036377 | 53.718523 | 1.481185 | 9.8602E-04 |
EOTLBO (2020) | 0.76077553 | 0.32302083 | 0.03637709 | 53.71852514 | 1.48118359 | 9.8602E-04 |
SGDE (2020) | 0.76078 | 0.32302 | 0.036377 | 53.71853 | 1.481184 | 9.8602E-04 |
CLJAYA (2020) | 0.76078 | 0.3230208 | 0.0363771 | 53.718521 | 1.481184 | 9.8602E-04 |
CPMPSO (2020) | 0.760776 | 0.323021 | 0.036377 | 53.71852 | 1.481184 | 9.8602E-04 |
NPSOPC (2020) | 0.7608 | 0.3325 | 0.03639 | 53.7583 | 1.4814 | 9.8856E-04 |
CBSA (2020) | 0.760776 | 0.323021 | 0.036377 | 53.71852 | 1.481184 | 9.8602E-04 |
LFBSA (2020) | 0.760776 | 0.323021 | 0.036377 | 53.71852 | 1.481184 | 9.8602E-04 |
IEO (2020) | 0.760775529 | 0.323 | 0.036377 | 53.71852 | 1.481183 | 9.8602E-04 |
ATLDE (2020) | 0.76077553 | 0.32302082 | 0.03637712 | 53.71852699 | 1.48118359 | 9.8602E-04 |
EJAYA (2021) | 0.76078 | 0.32302 | 0.03638 | 53.71852 | 1.48118 | 9.8602E-04 |
IGSK (2021) | 0.76077553 | 0.323 | 0.036377093 | 53.71852532 | 1.481183592 | 9.8602E-04 |
EABOA (2021) | 0.760771077 | 0.322929 | 0.036379593 | 53.76600144 | 1.481153457 | 9.8602E-04 |
SFLBS (2021) | 0.76078 | 0.323021 | 0.03638 | 53.7185 | 1.481184 | 9.8602E-04 |
RLDE (2021) | 0.7608 | 0.3231 | 0.0364 | 53.7185 | 1.4812 | 9.8602E-04 |
EJADE-D | 0.76077553 | 0.32302079 | 0.03637709 | 53.71852020 | 1.48118359 | 9.8602E-04 |
Algorithm | Ipv (A) | Isd1 (μA) | Rs (Ω) | Rp (Ω) | n1 | Isd2 (μA) | n2 | RMSE |
GOTLBO (2016) | 0.7608 | 0.8002 | 0.0368 | 56.0753 | 2 | 0.2205 | 1.4490 | 9.8318E-04 |
IJAYA (2017) | 0.7601 | 0.0050445 | 0.0376 | 77.8519 | 1.2186 | 0.75904 | 1.6247 | 9.8293E-04 |
SATLBO (2017) | 0.7608 | 0.2509 | 0.0366 | 55.1170 | 1.4598 | 0.5454 | 1.9994 | 9.8280E-04 |
CWOA (2017) | 0.76077 | 0.24150 | 0.03666 | 55.20160 | 1.45651 | 0.60000 | 1.98990 | 9.8272E-04 |
MSSO (2017) | 0.760748 | 0.234925 | 0.036688 | 55.714662 | 1.454255 | 0.671593 | 1.995305 | 9.8281E-04 |
IWOA (2018) | 0.7608 | 0.6771 | 0.0367 | 55.4082 | 2 | 0.2355 | 1.4545 | 9.8255E-04 |
HFAPS (2018) | 0.760781 | 0.225974 | 0.0367404 | 55.4855 | 1.45101 | 0.7493580 | 2.000000 | 9.8248E-04 |
TLABC (2018) | 0.76081 | 0.42394 | 0.03667 | 54.66797 | 1.90750 | 0.24011 | 1.45671 | 9.8415E-04 |
MLBSA (2018) | 0.7608 | 0.22728 | 0.0670 | 55.4612 | 1.4515 | 0.73835 | 2.0000 | 9.8249E-04 |
DE/WOA (2018) | 0.760781 | 0.225974 | 0.036740 | 55.485437 | 1.451017 | 0.749346 | 2.000000 | 9.8248E-04 |
OBWOA (2018) | 0.76076 | 0.22990 | 0.03671 | 55.3990 | 1.49154 | 0.61956 | 2.000000 | 9.8251E-04 |
PGJAYA (2019) | 0.7608 | 0.21031 | 0.0368 | 55.8135 | 1.4450 | 0.88534 | 2.0000 | 9.8263E-04 |
BHCS (2019) | 0.76078 | 0.74935 | 0.03674 | 55.48544 | 2.00000 | 0.22597 | 1.45102 | 9.8249E-04 |
FPSO (2019) | 0.76078 | 0.22731 | 0.036737 | 55.39230 | 1.45160 | 0.72786 | 1.99969 | 9.8253E-04 |
ILCOA (2019) | 0.76078 | 0.22601 | 0.036739 | 55.5320 | 1.45101 | 0.74921 | 2.00000 | 9.8257E-04 |
ITLBO (2019) | 0.7608 | 0.2260 | 0.0367 | 55.4854 | 1.4510 | 0.7493 | 2.0000 | 9.8248E-04 |
SGDE (2020) | 0.76079 | 0.28070 | 0.036480 | 54.3667 | 1.46966 | 0.24996 | 1.93228 | 9.8441E-04 |
BSARDVs (2020) | 0.760781 | 0.225808 | 0.036741 | 55.4878 | 1.45096 | 0.750861 | 2 | 9.8248E-04 |
ELBA (2020) | 0.760781 | 0.749338 | 0.03674 | 55.48544 | 2 | 0.225975 | 1.451018 | 9.8248E-04 |
EOTLBO (2020) | 0.76078108 | 0.22597468 | 0.03674043 | 55.48543568 | 1.45101692 | 0.74934431 | 2 | 9.8248E-04 |
CLJAYA (2020) | 0.76078 | 0.226051 | 0.03674 | 55.48599 | 1.45105 | 0.74876 | 1.99999 | 9.8249E-04 |
CPMPSO (2020) | 0.76078 | 0.74935 | 0.03674 | 55.48544 | 2 | 0.22597 | 1.45102 | 9.8248E-04 |
NPSOPC (2020) | 0.76078 | 0.25093 | 0.03663 | 55.117 | 1.45982 | 0.545418 | 1.99941 | 9.8208E-04 |
CBSA (2020) | 0.76078 | 0.2259739 | 0.03674 | 55.48544 | 1.451017 | 0.74935 | 2 | 9.8248E-04 |
LFBSA (2020) | 0.760781 | 0.225974 | 0.03674 | 55.48543 | 1.451017 | 0.749345 | 2 | 9.8249E-04 |
IEO (2020) | 0.760781 | 0.749 | 0.03674 | 55.48544 | 1.451016 | 0.226 | 1.999999 | 9.8248E-04 |
ATLDE (2020) | 0.76078108 | 0.22597412 | 0.03674043 | 55.48544744 | 1.45101671 | 0.74934885 | 2.00000000 | 9.8248E-04 |
EJAYA (2021) | 0.76078 | 0.22597 | 0.03674 | 55.48509 | 1.45102 | 0.74934 | 2 | 9.8248E-04 |
IGSK (2021) | 0.760781079 | 0.7493 | 0.036740429 | 55.48543425 | 2 | 0.226 | 1.451016893 | 9.8248E-04 |
EABOA (2021) | 0.76082865 | 0.25072 | 0.0366266 | 55.3660129 | 1.45988481 | 0.72069 | 1.99997318 | 9.8607E-04 |
SFLBS (2021) | 0.76077 | 0.775995 | 0.036755 | 55.5496 | 2 | 1.449857 | 9.8249E-04 | |
RLDE (2021) | 0.7608 | 0.226 | 0.0367 | 55.4847 | 2 | 0.7492 | 1.451 | 9.8248E-04 |
EJADE-D | 0.76078108 | 0.22597441 | 0.03674043 | 55.48543767 | 1.45101682 | 0.74934630 | 2.00000000 | 9.8248E-04 |
Algorithm | Ipv (A) | Isd (μA) | Rs (Ω) | Rp (Ω) | n | RMSE |
CWOA (2017) | 1.7 | 1.6338 | 0.0050 | 15.4 | 1.5 | 1.8000E-03 |
HFAPS (2018) | 1.6663 | 1.0703 | 0.24849 | 490.03 | 53.016 | 1.9700E-03 |
OBWOA (2018) | 1.6642 | 1.65025 | 0.0044 | 15.5299 | 1.51424 | 1.7530E-03 |
BHCS (2019) | 1.66390 | 1.73866 | 0.00427 | 15.92829 | 1.52030 | 1.7298E-03 |
FPSO (2019) | 1.2323 | 7.4732 | 0.0049 | 9.6889 | 1.2086 | 1.3000E-03 |
ILCOA (2019) | 1.2001 | 7.4812 | 0.0049 | 9.6991 | 1.2067 | 1.6932E-02 |
ITLBO (2019) | 1.6639 | 1.7387 | 0.0043 | 15.9283 | 1.5203 | 1.7298E-03 |
ELBA (2020) | 1.663905 | 1.738657 | 0.004274 | 15.928294 | 1.520305 | 1.7298E-03 |
IEO (2020) | 1.663904802 | 1.74 | 0.004274 | 15.92827 | 1.520303 | 1.7298E-03 |
ATLDE (2020) | 1.66390478 | 1.73865697 | 0.00427377 | 15.92829439 | 1.52030293 | 1.7298E-03 |
EJAYA (2021) | 1.6639 | 1.73866 | 0.00427 | 15.92829 | 1.5203 | 1.7298E-03 |
IGSK (2021) | 1.663904777 | 1.7387 | 0.004273771 | 15.92829435 | 1.520302921 | 1.7298E-03 |
RLDE (2021) | 1.6639 | 1.7387 | 0.00427 | 15.9283 | 1.5203 | 1.7298E-03 |
EJADE-D | 1.66390478 | 1.73865681 | 0.00427377 | 15.92829378 | 1.52030292 | 1.7298E-03 |
Algorithm | Ipv (A) | Isd (μA) | Rs (Ω) | Rp (Ω) | n | RMSE |
CWOA (2017) | 7.4760 | 1.2 | 0.00000490 | 9.7942 | 1.2069 | 1.7601E-02 |
ITLBO (2019) | 7.4725 | 2.335 | 0.0046 | 22.2199 | 1.2601 | 1.6601E-02 |
BHCS (2019) | 7.47253 | 2.33499 | 0.00459 | 22.21990 | 1.26010 | 1.6601E-02 |
IEO (2020) | 7.472531264 | 2.23 | 0.004595 | 22.21679 | 1.260101 | 1.6601E-02 |
ATLDE (2020) | 7.47252992 | 2.33499485 | 0.00459463 | 22.21989607 | 1.26010347 | 1.6601E-02 |
EJAYA (2021) | 7.47253 | 2.33499 | 0.00459 | 22.21989 | 1.2601 | 1.6601E-02 |
IGSK (2021) | 7.47252992 | 2.335 | 0.004594635 | 22.21989406 | 1.260103467 | 1.6601E-02 |
RLDE (2021) | 7.4725 | 2.335 | 0.0046 | 22.2199 | 1.2601 | 1.6601E-02 |
EJADE-D | 7.47252992 | 2.33499494 | 0.00459463 | 22.21989617 | 1.26010347 | 1.6601E-02 |
In order to use less computing resources to accurately and reliably extract unknown parameters in PV models, in this paper, an advanced differential evolution with search space decomposition (EJADE-D) is developed. In EJADE-D, the search space decomposition technique is proposed to reduce the dimension of the PV models parameter extraction problem. Benefit from this technique, the advanced adaptive differential evolution is employed as a solver. The proposed EJADE-D has been used to extract the unknown parameters of different PV models. Experimental results achieved by EJADE-D are firstly compared with DE, JADE, and EJADE, and the comparison results demonstrate the superiority of the EJADE-D. In addition, many recently reported well-established parameter extraction algorithms are selected for comparison. When compared with these advanced algorithms, proposed EJADE-D can not only obtain accurate and reliable results, especially for the double diode model, but also consume the least computing resources. Therefore, it can be leveraged as an effective approach to extracting the unknown parameters in PV models.
In future works, we will use the proposed search space decomposition technique for more complex optimization, such as pollution isolation [69], supplier selection [70], nonlinear equations [71].
This work was partly supported by the National Natural Science Foundation of China under Grant Nos. 62076225 and 62073300, and the Natural Science Foundation for Distinguished Young Scholars of Hubei under Grant No. 2019CFA081.
All authors declare no conflicts of interest in this paper.
[1] | T. Teo, T. Logenthiran, W. Woo, Forecasting of photovoltaic power using extreme learning machine, in 2015 IEEE Innovative Smart Grid Technologies - Asia (ISGT ASIA), (2015), 1–6. |
[2] | V. De, T. Teo, W. Woo, T. Logenthiran, Photovoltaic power forecasting using lstm on limited dataset, in 2018 IEEE Innovative Smart Grid Technologies - Asia (ISGT Asia), (2018), 710–715. |
[3] |
I. Ibrahim, M. Hossain, B. Duck, M. Nadarajah, An improved wind driven optimization algorithm for parameters identification of a triple-diode photovoltaic cell model, Energy Convers. Manage., 213 (2020), 112872. doi: 10.1016/j.enconman.2020.112872
![]() |
[4] |
Y. Zhang, M. Ma, Z. Jin, Comprehensive learning jaya algorithm for parameter extraction of photovoltaic models, Energy, 211 (2020), 118644. doi: 10.1016/j.energy.2020.118644
![]() |
[5] |
B. Dong, A. Luzin, D. Gura, The hybrid method based on ant colony optimization algorithm in multiple factor analysis of the environmental impact of solar cell technologies, Math. Biosci. Eng., 17 (2020), 6342–6354. doi: 10.3934/mbe.2020334
![]() |
[6] |
S. Li, W Gong, Q. Gu, A comprehensive survey on meta-heuristic algorithms for parameter extraction of photovoltaic models, Renew. Sustain. Energy Rev., 141 (2021), 110828. doi: 10.1016/j.rser.2021.110828
![]() |
[7] |
S. Bana, R. Saini, Identification of unknown parameters of a single diode photovoltaic model using particle swarm optimization with binary constraints, Renew. Energy, 101 (2017), 1299–1310. doi: 10.1016/j.renene.2016.10.010
![]() |
[8] | T. Ayodele, A. Ogunjuyigbe, E. Ekoh, Evaluation of numerical algorithms used in extracting the parameters of a single-diode photovoltaic model, Sustain. Energy Technol. Assess., 13 (2016), 51–59. |
[9] |
T. Babu, J. Ram, K. Sangeetha, A. Laudani, N. Rajasekar. Parameter extraction of two diode solar pv model using fireworks algorithm, Sol. Energy, 140 (2016), 265–276. doi: 10.1016/j.solener.2016.10.044
![]() |
[10] |
V. Khanna, B. Das, D. Bisht, Vandana, P. Singh, A three diode model for industrial solar cells and estimation of solar cell parameters using pso algorithm, Renew. Energy, 78 (2015), 105–113. doi: 10.1016/j.renene.2014.12.072
![]() |
[11] | A. Jordehi. Parameter estimation of solar photovoltaic (pv) cells: A review, Renew. Sustain. Energy Rev., 61 (2016), 354–371. |
[12] |
W. Gong, Z. Cai, Parameter extraction of solar cell models using repaired adaptive differential evolution, Sol. Energy, 94 (2013), 209–220. doi: 10.1016/j.solener.2013.05.007
![]() |
[13] |
D. Kler, P. Sharma, A. Banerjee, K. Rana, V. Kumar, Pv cell and module efficient parameters estimation using evaporation rate based water cycle algorithm, Swarm Evol. Comput., 35 (2017), 93–110. doi: 10.1016/j.swevo.2017.02.005
![]() |
[14] |
T. Easwarakhanthan, J. Bottin, I. Bouhouch, C. Boutrit, Nonlinear minimization algorithm for determining the solar cell parameters with microcomputers, Int. J. Sol. Energy, 4 (1986), 1–12. doi: 10.1080/01425918608909835
![]() |
[15] |
A. Ortiz-Conde, F. Sˊanchez, J. Muci, New method to extract the model parameters of solar cells from the explicit analytic solutions of their illuminated characteristics, Sol. Energy Mater Sol. Cells, 90 (2006), 352–361. doi: 10.1016/j.solmat.2005.04.023
![]() |
[16] |
M. AlHajri, K. El-Naggar, M. AlRashidi, A. Al-Othman, Optimal extraction of solar cell parameters using pattern search, Renew. Energy, 44 (2012), 238–245. doi: 10.1016/j.renene.2012.01.082
![]() |
[17] |
R. Ben-Messaoud, Extraction of uncertain parameters of single-diode model of a photovoltaic panel using simulated annealing optimization, Energy Rep., 6 (2020), 350–357. doi: 10.1016/j.egyr.2020.01.016
![]() |
[18] |
M. Alrashidi, M. Alhajri, K. Elnaggar, A. Alothman, A new estimation approach for determining the i-v characteristics of solar cells, Sol. Energy, 85 (2011), 1543–1550. doi: 10.1016/j.solener.2011.04.013
![]() |
[19] |
A. Askarzadeh, A. Rezazadeh, Parameter identification for solar cell models using harmony search-based algorithms, Sol. Energy, 86 (2012), 3241–3249. doi: 10.1016/j.solener.2012.08.018
![]() |
[20] |
J. Liang, S. Ge, B. Qu, K. Yu, F. Liu, H. Yang, et al., Classified perturbation mutation based particle swarm optimization algorithm for parameters extraction of photovoltaic models, Energy Convers. Manage., 203 (2020), 112138. doi: 10.1016/j.enconman.2019.112138
![]() |
[21] |
X. Lin, Y. Wu, Parameters identification of photovoltaic models using niche-based particle swarm optimization in parallel computing architecture, Energy, 196 (2020), 117054. doi: 10.1016/j.energy.2020.117054
![]() |
[22] |
K. Yu, B. Qu, C. Yue, S. Ge, X. Chen, J. Liang, A performance-guided jaya algorithm for parameters identification of photovoltaic cell and module, Appl. Energy, 237 (2019), 241–257. doi: 10.1016/j.apenergy.2019.01.008
![]() |
[23] |
X. Yang, W. Gong, Opposition-based jaya with population reduction for parameter estimation of photovoltaic solar cells and modules, Appl. Soft Comput., 104 (2021), 107218. doi: 10.1016/j.asoc.2021.107218
![]() |
[24] |
J. Liang, K. Qiao, M. Yuan, K. Yu, B. Qu, S. Ge, et al., Evolutionary multi-task optimization for parameters extraction of photovoltaic models, Energy Convers. Manage., 207 (2020), 112509. doi: 10.1016/j.enconman.2020.112509
![]() |
[25] |
W. Li, W. Gong, Differential evolution with quasi-reflection-based mutation, Math. Biosci. Eng., 18 (2021), 2425–2441. doi: 10.3934/mbe.2021123
![]() |
[26] |
D. Oliva, M. Aziz, A. Hassanien, Parameter estimation of photovoltaic cells using an improved chaotic whale optimization algorithm, Appl. Energy, 200 (2017), 141–154. doi: 10.1016/j.apenergy.2017.05.029
![]() |
[27] |
M. Elaziz, D. Oliva, Parameter estimation of solar cells diode models by an improved opposition-based whale optimization algorithm, Energy Convers. Manage., 171 (2018), 1843–1859. doi: 10.1016/j.enconman.2018.05.062
![]() |
[28] |
A. Askarzadeh, A. Rezazadeh, Artificial bee swarm optimization algorithm for parameters identification of solar cell models, Appl. Energy, 102 (2013), 943–949. doi: 10.1016/j.apenergy.2012.09.052
![]() |
[29] |
K. Yu, J. Liang, B. Qu, Z. Cheng, H. Wang, Multiple learning backtracking search algorithm for estimating parameters of photovoltaic models, Appl. Energy, 226 (2018), 408–422. doi: 10.1016/j.apenergy.2018.06.010
![]() |
[30] |
Y. Zhang, C. Huang, Z. Jin, Backtracking search algorithm with reusing differential vectors for parameter identification of photovoltaic models, Energy Convers. Manage., 223 (2020), 113266. doi: 10.1016/j.enconman.2020.113266
![]() |
[31] | T. Kang, J. Yao, M. Jin, S. Yang, T. Duong, A novel improved cuckoo search algorithm for parameter estimation of photovoltaic (pv) models, Energies, 11 (2018), 1–31. |
[32] |
P. Lin, S. Cheng, W. Yeh, Z. Chen, L. Wu, Parameters extraction of solar cell models using a modified simplified swarm optimization algorithm, Sol. Energy, 144 (2017), 594–603. doi: 10.1016/j.solener.2017.01.064
![]() |
[33] |
K. Yu, X. Chen, X. Wang, Z. Wang, Parameters identification of photovoltaic models using self-adaptive teaching-learning-based optimization, Energy Convers. Manage., 145 (2017), 233–246. doi: 10.1016/j.enconman.2017.04.054
![]() |
[34] | S. Li, W. Gong, X. Yan, C. Hu, D. Bai, L. Wang, et al., Parameter extraction of photovoltaic models using an improved teaching-learning-based optimization, Energy Convers. Manage., 189 (2019), 293–305. |
[35] |
A. Beigi, A. Maroosi, Parameter identification for solar cells and module using a hybrid firefly and pattern search algorithms, Sol. Energy, 171 (2018), 435–446. doi: 10.1016/j.solener.2018.06.092
![]() |
[36] |
H. Hasanien, Shuffled frog leaping algorithm for photovoltaic model identification, IEEE Trans. Sustain. Energy, 6 (2015), 509–515. doi: 10.1109/TSTE.2015.2389858
![]() |
[37] |
Y. Fan, P. Wang, A. Heidari, X. Zhao, H. Turabieh, H. Chen, Delayed dynamic step shuffling frog-leaping algorithm for optimal design of photovoltaic models, Energy Rep., 7 (2021), 228–246. doi: 10.1016/j.egyr.2020.12.013
![]() |
[38] |
X. Chen, K. Yu, Hybridizing cuckoo search algorithm with biogeography-based optimization for estimating photovoltaic model parameters, Sol. Energy, 180 (2019), 192–206. doi: 10.1016/j.solener.2019.01.025
![]() |
[39] |
S. Li, W. Gong, L. Wang, X. Yan, C. Hu, A hybrid adaptive teaching-learning-based optimization and differential evolution for parameter identification of photovoltaic models, Energy Convers. Manage., 225 (2020), 113474. doi: 10.1016/j.enconman.2020.113474
![]() |
[40] |
G. Xiong, J. Zhang, D. Shi, Y. He, Parameter extraction of solar photovoltaic models using an improved whale optimization algorithm, Energy Convers. Manage., 174 (2018), 388–405. doi: 10.1016/j.enconman.2018.08.053
![]() |
[41] |
S. Li, Q. Gu, W. Gong, B. Ning, An enhanced adaptive differential evolution algorithm for parameter extraction of photovoltaic models, Energy Convers. Manage., 205 (2020), 112443. doi: 10.1016/j.enconman.2019.112443
![]() |
[42] |
K. El-Naggar, M. AlRashidi, M. AlHajri, A. Al-Othman, Simulated annealing algorithm for photovoltaic parameters identification, Sol. Energy, 86 (2012), 266–274. doi: 10.1016/j.solener.2011.09.032
![]() |
[43] | W. Huang, C. Jiang, L. Xue, D. Song, Extracting solar cell model parameters based on chaos particle swarm algorithm, in 2011 International Conference on Electric Information and Control Engineering, (2011), 398–402. |
[44] |
K. Ishaque, Z. Salam, S. Mekhilef, A. Shamsudin, Parameter extraction of solar photovoltaic modules using penalty-based differential evolution, Appl. Energy, 99 (2012), 297–308. doi: 10.1016/j.apenergy.2012.05.017
![]() |
[45] |
K. Yu, J. Liang, B. Qu, X. Chen, H. Wang, Parameters identification of photovoltaic models using an improved jaya optimization algorithm, Energy Convers. Manage., 150 (2017), 742–753. doi: 10.1016/j.enconman.2017.08.063
![]() |
[46] |
J. Ram, T. Babu, T. Dragicevic, N. Rajasekar, A new hybrid bee pollinator flower pollination algorithm for solar pv parameter estimation, Energy Convers. Manage., 135 (2017), 463–476. doi: 10.1016/j.enconman.2016.12.082
![]() |
[47] |
X. Chen, K. Yu, W. Du, W. Zhao, G. Liu, Parameters identification of solar cell models using generalized oppositional teaching learning based optimization, Energy, 99 (2016), 170–180. doi: 10.1016/j.energy.2016.01.052
![]() |
[48] |
X. Chen, B. Xu, C. Mei, Y. Ding, K. Li, Teaching-learning-based artificial bee colony for solar photovoltaic parameter estimation, Appl. Energy, 212 (2018), 1578–1588. doi: 10.1016/j.apenergy.2017.12.115
![]() |
[49] |
F. Zeng, H. Shu, J. Wang, Y. Chen, B. Yang, Parameter identification of pv cell via adaptive compass search algorithm, Energy Rep., 7 (2021), 275–282. doi: 10.1016/j.egyr.2021.01.069
![]() |
[50] |
G. Xiong, L. Li, A. Mohamed, X. Yuan, J. Zhang, A new method for parameter extraction of solar photovoltaic models using gaining–sharing knowledge based algorithm, Energy Rep., 7 (2021), 3286–3301. doi: 10.1016/j.egyr.2021.05.030
![]() |
[51] |
R. Rahmaniani, T. Crainic, M. Gendreau, W. Rei, The benders decomposition algorithm: A literature review, Eur. J. Oper. Res., 259 (2017), 801–817. doi: 10.1016/j.ejor.2016.12.005
![]() |
[52] |
J. Zhang, A. Sanderson, Jade: Adaptive differential evolution with optional external archive, IEEE Trans. Evol. Comput., 13 (2009), 945–958. doi: 10.1109/TEVC.2009.2014613
![]() |
[53] |
S. Li, W. Gong, L. Wang, X. Yan, C. Hu, Optimal power flow by means of improved adaptive differential evolution, Energy, 198 (2020), 117314. doi: 10.1016/j.energy.2020.117314
![]() |
[54] | K. Yu, J. Liang, B. Qu, Y. Luo, C. Yue, Dynamic selection preference-assisted constrained multiobjective differential evolution, IEEE Trans. Syst. Man Cybern. Syst., (2021), 1–12. |
[55] |
K. Yu, J. Liang, B. Qu, C. Yue, Purpose-directed two-phase multiobjective differential evolution for constrained multiobjective optimization, Swarm Evol. Comput., 60 (2021), 100799. doi: 10.1016/j.swevo.2020.100799
![]() |
[56] |
S. Li, W. Gong, C. Hu, X. Yan, L. Wang, Q. Gu, Adaptive constraint differential evolution for optimal power flow, Energy, 235 (2021), 121362. doi: 10.1016/j.energy.2021.121362
![]() |
[57] |
N. Tong, W. Pora, A parameter extraction technique exploiting intrinsic properties of solar cells, Appl. Energy, 176 (2016), 104–115. doi: 10.1016/j.apenergy.2016.05.064
![]() |
[58] |
R. Storn, K. Price, Differential evolution-a simple and efficient heuristic for global optimization over continuous spaces, J. Global Optim., 11 (1997), 341–359. doi: 10.1023/A:1008202821328
![]() |
[59] |
G. Xiong, J. Zhang, X. Yuan, D. Shi, Y. He, . Yao, Parameter extraction of solar photovoltaic models by means of a hybrid differential evolution with whale optimization algorithm, Sol. Energy, 176 (2018), 742–761. doi: 10.1016/j.solener.2018.10.050
![]() |
[60] |
S. Ebrahimi, E. Salahshour, M. Malekzadeh, F. Gordillo, Parameters identification of pv solar cells and modules using flexible particle swarm optimization algorithm, Energy, 179 (2019), 358–372. doi: 10.1016/j.energy.2019.04.218
![]() |
[61] |
N. Pourmousa, S. Ebrahimi, M. Malekzadeh, M. Alizadeh, Parameter estimation of photovoltaic cells using improved lozi map based chaotic optimization algorithm, Sol. Energy, 180 (2019), 180–191. doi: 10.1016/j.solener.2019.01.026
![]() |
[62] |
L. Deotti, J. Pereira, I. Jˊenior, Parameter extraction of photovoltaic models using an enhanced lˊevy flight bat algorithm, Energy Convers. Manage., 221 (2020), 113114. doi: 10.1016/j.enconman.2020.113114
![]() |
[63] |
G. Xiong, J. Zhang, D. Shi, L. Zhu, X. Yuan, Parameter extraction of solar photovoltaic models with an either-or teaching learning based algorithm, Energy Convers. Manage., 224 (2020), 113395. doi: 10.1016/j.enconman.2020.113395
![]() |
[64] |
Y. Zhang, M. Ma, Z. Jin, Backtracking search algorithm with competitive learning for identification of unknown parameters of photovoltaic systems, Expert Syst. Appl., 160 (2020), 113750. doi: 10.1016/j.eswa.2020.113750
![]() |
[65] |
K. Sallam, M. Hossain, R. Chakrabortty, M. Ryan, An improved gaining-sharing knowledge algorithm for parameter extraction of photovoltaic models, Energy Convers. Manage., 237 (2021), 114030. doi: 10.1016/j.enconman.2021.114030
![]() |
[66] |
W. Long, T. Wu, M. Xu, M. Tang, S. Cai, Parameters identification of photovoltaic models by using an enhanced adaptive butterfly optimization algorithm, Energy, 229 (2021), 120750. doi: 10.1016/j.energy.2021.120750
![]() |
[67] |
Y. Liu, A. Heidari, X. Ye, C. Chi, X. Zhao, C. Ma, et al., Evolutionary shuffled frog leaping with memory pool for parameter optimization, Energy Rep., 7 (2021), 584–606. doi: 10.1016/j.egyr.2021.01.001
![]() |
[68] |
Z. Hu, W. Gong, S. Li, Reinforcement learning-based differential evolution for parameters extraction of photovoltaic models, Energy Rep., 7 (2021), 916–928. doi: 10.1016/j.egyr.2021.01.096
![]() |
[69] |
C. Hu, J. Cai, D. Zeng, X. Yan, W. Gong, L. Wang, Deep reinforcement learning based valve scheduling for pollution isolation in water distribution network, Math. Biosci. Eng., 17 (2020), 105–121. doi: 10.3934/mbe.2020006
![]() |
[70] |
A. Alejo-Reyes, E. Olivares-Benitez, A. Mendoza, A. Rodriguez, Inventory replenishment decision model for the supplier selection problem using metaheuristic algorithms, Math. Biosci. Eng., 17 (2020), 2016–2036. doi: 10.3934/mbe.2020107
![]() |
[71] |
W. Gong, Z. Liao, X. Mi, L. Wang, Y. Guo, Nonlinear equations solving with intelligent optimization algorithms: A survey, Complex Syst. Model. Simul., 1 (2021), 15–32. doi: 10.23919/CSMS.2021.0002
![]() |
1. | Boyang Xu, Ali Asghar Heidari, Fangjun Kuang, Siyang Zhang, Huiling Chen, Zhennao Cai, Quantum Nelder‐Mead Hunger Games Search for optimizing photovoltaic solar cells , 2022, 46, 0363-907X, 12417, 10.1002/er.8011 | |
2. | Zhiqiang Zeng, Huanhuan Zhang, An evolutionary-state-based selection strategy for enhancing differential evolution algorithm, 2022, 617, 00200255, 373, 10.1016/j.ins.2022.10.111 | |
3. | Junhua Ku, Shuijia Li, Wenyin Gong, Photovoltaic models parameter estimation via an enhanced Rao-1 algorithm, 2021, 19, 1551-0018, 1128, 10.3934/mbe.2022052 | |
4. | Xiaobing Yu, Zhengpeng Hu, Xuming Wang, Wenguan Luo, Ranking teaching–learning-based optimization algorithm to estimate the parameters of solar models, 2023, 123, 09521976, 106225, 10.1016/j.engappai.2023.106225 | |
5. | Yixin Zhuo, Ling Li, Jian Tang, Wenchuan Meng, Zhanhong Huang, Kui Huang, Jiaqiu Hu, Yiming Qin, Houjian Zhan, Zhencheng Liang, Optimal real-time power dispatch of power grid with wind energy forecasting under extreme weather, 2023, 20, 1551-0018, 14353, 10.3934/mbe.2023642 | |
6. | Yujun Zhang, Shuijia Li, Yufei Wang, Yuxin Yan, Juan Zhao, Zhengming Gao, Self-adaptive enhanced learning differential evolution with surprisingly efficient decomposition approach for parameter identification of photovoltaic models, 2024, 308, 01968904, 118387, 10.1016/j.enconman.2024.118387 | |
7. | Xiaoyun Yang, Gang Zeng, Zan Cao, Xuefei Huang, Juan Zhao, Parameters estimation of complex solar photovoltaic models using bi-parameter coordinated updating L-SHADE with parameter decomposition method, 2024, 61, 2214157X, 104917, 10.1016/j.csite.2024.104917 | |
8. | Tianyu Gao, Yajun Zhang, Juan Zhao, Dynamic diversity capture differential evolution to accurately design complex nonlinear photovoltaic system: A heuristic case, 2025, 290, 0038092X, 113332, 10.1016/j.solener.2025.113332 |
Step 1: | Firstly, load the measured I-V data of PV models provided by the manufacturers. |
Step 2: | Randomly initialize NP (population size) solutions of population, in which each solution Xi=x1 is initialized in their specified range. |
Step 3: | According to each solution, calculate the linear unknown parameters x2 by using the proposed search space decomposition technique i.e., Eqs (20) and (21). |
Step 4: | After all solution have been done, then, each solution's linear parameters x2 and nonlinear parameters x1 are substituted into Eq (10) to calculate the objective function value of each solution. |
Step 5: | Leverage the advanced differential evolution algorithm i.e., EJADE to produce NP new solution. |
Step 6: | Similar to the Step 3, calculate the linear parameters x2 according related nonlinear parameters x1 for each new solution by using search space decomposition technique. |
Step 7: | Calculate the objection function value of each solution based on its linear parameters x2 and corresponding nonlinear parameters x1. |
Step 8: | According to the objective function value, selection between the new solution and old solution to form the next generation population. If the termination condition is met, then go to Step 9; else go to the loop: Steps 5–8. |
Step 9: | Output the best solution. |
Parameter | The single/double diode model | The single based PV module | |
Solar cell model | R.T.C. France solar cell | STM6-40/36 | STP6-120/36 |
Ns | 1 | 36 | 36 |
Np | 1 | 1 | 1 |
Number of I-V data | 26 | 20 | 24 |
Temperature | 25 ℃ | 51 ℃ | 55 ℃ |
Radiation | 1000 W/m2 | 1000 W/m2 | 1000 W/m2 |
Unknown parameters | R.T.C. France solar cell | STM6-40/36 | STP6-120/36 | |||||
Lower | Upper | Lower | Upper | Lower | Upper | |||
Ipv (A) | 0 | 1 | 0 | 2 | 0 | 8 | ||
Isd, Isd1, Isd2 (μA) | 0 | 1 | 0 | 50 | 0 | 50 | ||
Rs (Ω) | 0 | 0.5 | 0 | 0.36 | 0 | 0.36 | ||
Rp (Ω) | 0 | 100 | 0 | 1000 | 0 | 1500 | ||
n, n1, n2 | 1 | 2 | 1 | 60 | 1 | 50 |
Algorithm | Parameter value |
DE | NP = 50, F = 0.5, CR = 0.9 |
JADE | NP = 50 |
EJADE | NPmax = 50, NPmin = 4 |
EJADE-D | NPmax = 50, NPmin = 4 |
Algorithm | RMSE | |||
Best | Worst | Mean | Std | |
DE | 1.3733E-03 | 4.3933E-03 | 2.2122E-03 | 6.30E-04 |
JADE | 2.4628E-03 | 9.0467E-03 | 4.6089E-03 | 1.64E-03 |
EJADE | 9.9336E-04 | 2.1651E-03 | 1.4245E-03 | 3.03E-04 |
EJADE-D | 9.8602E-04 | 9.8602E-04 | 9.8602E-04 | 3.67E-17 |
Algorithm | Ipv (A) | Isd (μA) | Rs (Ω) | Rp (Ω) | n | RMSE |
DE | 0.75946361 | 0.41068723 | 0.03569428 | 86.22084780 | 1.50561834 | 1.3733E-03 |
JADE | 0.76241894 | 0.65695921 | 0.03323643 | 80.94523627 | 1.55621196 | 2.4628E-03 |
EJADE | 0.76070190 | 0.34171649 | 0.03613743 | 55.62560314 | 1.48687849 | 9.9336E-04 |
EJADE-D | 0.76077553 | 0.32302079 | 0.03637709 | 53.71852020 | 1.48118359 | 9.8602E-04 |
Algorithm | RMSE | |||
Best | Worst | Mean | Std | |
DE | 1.4793E-03 | 4.5821E-03 | 2.4962E-03 | 6.93E-04 |
JADE | 2.2919E-03 | 5.1370E-03 | 3.4453E-03 | 7.37E-04 |
EJADE | 9.8497E-04 | 2.0383E-03 | 1.2113E-03 | 2.83E-04 |
EJADE-D | 9.8248E-04 | 9.8248E-04 | 9.8248E-04 | 5.50E-17 |
Algorithm | Ipv (A) | Isd1 (μA) | Rs (Ω) | Rp (Ω) | n1 | Isd2 (μA) | n2 | RMSE |
DE | 0.76161938 | 0.29934314 | 0.03458265 | 62.11841082 | 1.95055472 | 0.44875729 | 1.51798488 | 1.4793E-03 |
JADE | 0.75904695 | 0.13872632 | 0.03655444 | 47.70175773 | 1.43701409 | 0.16306212 | 1.52688650 | 2.2919E-03 |
EJADE | 0.76077378 | 0.24691111 | 0.03653971 | 54.42053982 | 1.46090798 | 0.21482898 | 1.76404886 | 9.8497E-04 |
EJADE-D | 0.76078108 | 0.22597441 | 0.03674043 | 55.48543767 | 1.45101682 | 0.74934630 | 2.00000000 | 9.8248E-04 |
Algorithm | RMSE | |||
Best | Worst | Mean | Std | |
DE | 1.0448E-02 | 2.5021E-01 | 7.4874E-02 | 6.90E-02 |
JADE | 5.6988E-03 | 1.3783E-02 | 7.5998E-03 | 1.71E-03 |
EJADE | 2.6987E-03 | 4.0918E-03 | 3.3056E-03 | 2.78E-04 |
EJADE-D | 1.7298E-03 | 1.7298E-03 | 1.7298E-03 | 9.85E-18 |
Algorithm | Ipv (A) | Isd (μA) | Rs (Ω) | Rp (Ω) | n | RMSE |
DE | 1.65602020 | 7.78528613 | 0.00090772 | 317.05546222 | 1.70743127 | 1.0448E-02 |
JADE | 1.65376894 | 5.35917258 | 0.00130049 | 289.11110965 | 1.65342824 | 5.6988E-03 |
EJADE | 1.66220446 | 3.98041243 | 0.00125715 | 20.23972593 | 1.61727931 | 2.6987E-03 |
EJADE-D | 1.66390478 | 1.73865681 | 0.00427377 | 15.92829378 | 1.52030292 | 1.7298E-03 |
Algorithm | RMSE | |||
Best | Worst | Mean | Std | |
DE | 2.2855E-02 | 2.4084E-01 | 5.7271E-02 | 5.53E-02 |
JADE | 3.2110E-02 | 2.5739E-01 | 4.8897E-02 | 3.97E-02 |
EJADE | 1.6601E-02 | 4.0248E-02 | 1.7799E-02 | 4.27E-03 |
EJADE-D | 1.6601E-02 | 1.6601E-02 | 1.6601E-02 | 1.55E-16 |
Algorithm | Ipv (A) | Isd (μA) | Rs (Ω) | Rp (Ω) | n | RMSE |
DE | 7.48185880 | 6.48830083 | 0.00406738 | 1124.25574692 | 1.35185994 | 2.2855E-02 |
JADE | 7.48402233 | 1.15549180 | 0.00369744 | 1359.20725027 | 1.41018636 | 3.2110E-02 |
EJADE | 7.47249358 | 2.33478056 | 0.00459468 | 22.26217949 | 1.26009565 | 1.6601E-02 |
EJADE-D | 7.47252992 | 2.33499494 | 0.00459463 | 22.21989617 | 1.26010347 | 1.6601E-02 |
Algorithm | RMSE | NFEs | |||
Best | Worst | Mean | Std | ||
GOTLBO (2016) | 9.8744E-04 | 1.9824E-03 | 1.3349E-03 | 2.09E-04 | 10,000 |
SATLBO (2017) | 9.8602E-04 | 9.9494E-03 | 9.8780E-04 | 2.30E-06 | 50,000 |
IJAYA (2017) | 9.8603E-04 | 1.0622E-03 | 9.9204E-04 | 1.40E-05 | 50,000 |
TLABC (2018) | 9.8602E-04 | 1.0397E-03 | 9.9852E-04 | 1.86E-05 | 50,000 |
MLBSA (2018) | 9.8602E-04 | 9.8602E-04 | 9.8602E-04 | 9.15E-12 | 50,000 |
DE/WOA (2018) | 9.8602E-04 | 9.8602E-04 | 9.8602E-04 | 3.55E-17 | 50,000 |
OBWOA (2018) | 9.8602E-04 | NA | 9.8603E-03 | 1.02E-08 | 1,500,000 |
ITLBO (2019) | 9.8602E-04 | 9.8602E-04 | 9.8602E-04 | 2.19E-17 | 50,000 |
PGJAYA (2019) | 9.8602E-04 | 9.8603E-04 | 9.8602E-04 | 1.45E-09 | 50,000 |
BHCS (2019) | 9.8602E-04 | 9.8602E-04 | 9.8602E-04 | 2.61E-17 | 50,000 |
FPSO (2019) | 9.8602E-04 | NA | NA | NA | NA |
ILCOA (2019) | 9.8602E-04 | NA | NA | 1.01E-08 | 10,000*NP |
BSARDVs (2020) | 9.8602E-04 | NA | NA | NA | 25,000 |
ELBA (2020) | 9.8602E-04 | 9.8602E-04 | 9.8602E-04 | 1.97E-17 | 50,000 |
EOTLBO (2020) | 9.8602E-04 | 9.8602E-04 | 9.8602E-04 | 4.13E-17 | 20,000 |
CLJAYA (2020) | 9.8602E-04 | NA | NA | NA | 20,000 |
CBSA (2020) | 9.8602E-04 | NA | NA | NA | 25,000 |
IEO (2020) | 9.8602E-04 | NA | NA | NA | 1,500,000 |
ATLDE (2020) | 9.8602E-04 | 9.8602E-04 | 9.8602E-04 | 2.44E-17 | 30,000 |
EJAYA (2021) | 9.8602E-04 | 9.8602E-04 | 9.8602E-04 | 6.80E-17 | 30,000 |
IGSK (2021) | 9.8602E-04 | 9.8602E-04 | 9.8602E-04 | 3.58E-17 | 10,000 |
EABOA (2021) | 9.8602E-04 | 9.8784E-04 | 9.8678E-04 | 9.30E-07 | 50,000 |
SFLBS (2021) | 9.8602E-04 | 9.8602E-04 | 9.8602E-04 | 1.43E-14 | 60,000 |
RLDE (2021) | 9.8602E-04 | 9.8602E-04 | 9.8602E-04 | 3.48E-17 | 30,000 |
EJADE-D | 9.8602E-04 | 9.8602E-04 | 9.8602E-04 | 3.67E-17 | 2000 |
Algorithm | RMSE | NFEs | |||
Best | Worst | Mean | Std | ||
GOTLBO (2016) | 9.8318E-04 | 1.7877E-03 | 1.2436E-03 | 2.09E-04 | 20,000 |
SATLBO (2017) | 9.8280E-04 | 1.0470E-03 | 9.9811E-04 | 1.95E-05 | 50,000 |
IJAYA (2017) | 9.8293E-04 | 1.4055E-03 | 1.0269E-03 | 9.83E-05 | 50,000 |
TLABC (2018) | 9.8415E-04 | 1.5048E-03 | 1.0555E-03 | 1.55E-04 | 50,000 |
MLBSA (2018) | 9.8249E-04 | 9.8798E-04 | 9.8518E-04 | 1.35E-06 | 50,000 |
DE/WOA (2018) | 9.8248E-04 | 9.8604E-04 | 9.8297E-04 | 9.15E-07 | 50,000 |
OBWOA (2018) | 9.8251E-04 | NA | 9.8294E-04 | 1.13E-07 | 1,500,000 |
PGJAYA (2019) | 9.8263E-04 | 9.9499E-04 | 9.8582E-04 | 2.54E-06 | 50,000 |
BHCS (2019) | 9.8249E-04 | 9.8687E-04 | 9.8380E-04 | 1.54E-06 | 50,000 |
FPSO (2019) | 9.8253E-04 | NA | NA | NA | NA |
ILCOA (2019) | 8.8257E-04 | NA | NA | 6.25E-07 | 10,000*NP |
ITLBO (2019) | 9.8248E-04 | 9.8812E-04 | 9.8497E-04 | 1.54E-06 | 50,000 |
BSARDVs (2020) | 9.8248E-04 | NA | NA | NA | 45,000 |
ELBA (2020) | 9.8248E-04 | 9.8615E-04 | 9.8349E-04 | 1.43E-06 | 50,000 |
EOTLBO (2020) | 9.8248E-04 | 9.8942E-04 | 9.8473E-04 | 1.69E-06 | 20,000 |
CLJAYA (2020) | 9.8249E-04 | NA | NA | NA | 48,000 |
CBSA (2020) | 9.8248E-04 | NA | NA | NA | 50,000 |
IEO (2020) | 9.8248E-04 | NA | NA | NA | 1,500,000 |
ATLDE (2020) | 9.8248E-04 | 9.8603E-04 | 9.8372E-04 | 1.37E-06 | 30,000 |
EJAYA (2021) | 9.8248E-04 | 9.8602E-04 | 9.8448E-04 | 1.51E-06 | 30,000 |
IGSK (2021) | 9.8248E-04 | 9.8602E-04 | 9.8273E-04 | 8.96E-07 | 20,000 |
EABOA (2021) | 9.8607E-04 | 1.0012E-03 | 9.9190E-04 | 6.62E-06 | 50,000 |
SFLBS (2021) | 9.8249E-04 | 9.8787E-04 | 9.8541E-04 | 1.79E-06 | 60,000 |
RLDE (2021) | 9.8248E-04 | 9.8695E-04 | 9.8457E-04 | 1.75E-06 | 30,000 |
EJADE-D | 9.8248E-04 | 9.8248E-04 | 9.8248E-04 | 5.50E-17 | 4,000 |
Algorithm | RMSE | NFEs | |||
Best | Worst | Mean | Std | ||
BHCS (2019) | 1.7298E-03 | 3.3299E-03 | 1.8365E-03 | 4.06E-04 | 50,000 |
ITLBO (2019) | 1.7298E-03 | 1.7298E-03 | 1.7298E-03 | 4.75E-18 | 50,000 |
ELBA (2020) | 1.7298E-03 | 1.7298E-03 | 1.7298E-03 | 6.16E-18 | 50,000 |
IEO (2020) | 1.7298E-03 | NA | NA | NA | 1,500,000 |
ATLDE (2020) | 1.7298E-03 | 1.7298E-03 | 1.7298E-03 | 8.22E-18 | 30,000 |
EJAYA (2021) | 1.7298E-03 | 1.7298E-03 | 1.7298E-03 | 1.47E-17 | 30,000 |
IGSK (2021) | 1.7298E-03 | 1.7298E-03 | 1.7298E-03 | 7.02E-18 | 15,000 |
RLDE (2021) | 1.7298E-03 | 1.7298E-03 | 1.7298E-03 | 1.58E-17 | 30,000 |
EJADE-D | 1.7298E-03 | 1.7298E-03 | 1.7298E-03 | 9.85E-18 | 3,000 |
Algorithm | RMSE | NFEs | |||
Best | Worst | Mean | Std | ||
BHCS (2019) | 1.6601E-02 | 1.3482E-01 | 2.4360E-02 | 2.61E-02 | 50,000 |
ITLBO (2019) | 1.6601E-02 | 1.6601E-02 | 1.6601E-02 | 7.22E-17 | 50,000 |
IEO (2020) | 1.6601E-02 | NA | NA | NA | 1,500,000 |
ATLDE (2020) | 1.6601E-02 | 1.6601E-02 | 1.6601E-02 | 1.02E-16 | 30,000 |
EJAYA (2021) | 1.6601E-02 | 1.6601E-02 | 1.6601E-02 | 2.68E-16 | 30,000 |
IGSK (2021) | 1.6601E-02 | 1.6601E-02 | 1.6601E-02 | 1.71E-16 | 15,000 |
RLDE (2021) | 1.6601E-02 | 1.6601E-02 | 1.6601E-02 | 1.98E-16 | 30,000 |
EJADE-D | 1.6601E-02 | 1.6601E-02 | 1.6601E-02 | 1.55E-16 | 7000 |
Algorithm | Ipv (A) | Isd (μA) | Rs (Ω) | Rp (Ω) | n | RMSE |
GOTLBO (2016) | 0.7608 | 0.3316 | 0.0363 | 54.1154 | 1.4838 | 9.8744E-04 |
IJAYA (2017) | 0.7608 | 0.3228 | 0.0364 | 53.7595 | 1.4811 | 9.8603E-04 |
SATLBO (2017) | 0.7608 | 0.3232 | 0.0363 | 53.7256 | 1.4812 | 9.8602E-04 |
CWOA (2017) | 0.76077 | 0.3239 | 0.03636 | 53.7987 | 1.4812 | 9.8602E-04 |
MSSO (2017) | 0.760777 | 0.323564 | 0.036370 | 53.742465 | 1.481244 | 9.8607E-04 |
IWOA (2018) | 0.7608 | 0.3232 | 0.0364 | 53.7317 | 1.4812 | 9.8602E-04 |
HFAPS (2018) | 0.760777 | 0.322622 | 0.0363819 | 53.6784 | 1.48106 | 9.8602E-04 |
TLABC (2018) | 0.76078 | 0.32302 | 0.03638 | 53.71636 | 1.48118 | 9.8602E-04 |
MLBSA (2018) | 0.7608 | 0.32302 | 0.0364 | 53.7185 | 1.4812 | 9.8602E-04 |
DE/WOA (2018) | 0.760776 | 0.323021 | 0.036377 | 53.718524 | 1.481184 | 9.8602E-04 |
OBWOA (2018) | 0.76077 | 0. 3232 | 0. 0363 | 53.6836 | 1.5208 | 9.8602E-04 |
PGJAYA (2019) | 0.7608 | 0.3230 | 0.0364 | 53.7185 | 1.4812 | 9.8602E-04 |
BHCS (2019) | 0.76078 | 0.32302 | 0.03638 | 53.71852 | 1.48118 | 9.8602E-04 |
FPSO (2019) | 0.76077552 | 0.323020 | 0.036370 | 53.718520 | 1.48110817 | 9.8602E-04 |
ILCOA (2019) | 0.760775 | 0.323021 | 0.036377 | 53.718679 | 1.481108 | 9.8602E-04 |
ITLBO (2019) | 0.7608 | 0.3230 | 0.0364 | 53.7185 | 1.4812 | 9.8602E-04 |
BSARDVs (2020) | 0.760776 | 0.323021 | 0.036377 | 53.718520 | 1.481184 | 9.8602E-04 |
ELBA (2020) | 0.760776 | 0.323021 | 0.036377 | 53.718523 | 1.481185 | 9.8602E-04 |
EOTLBO (2020) | 0.76077553 | 0.32302083 | 0.03637709 | 53.71852514 | 1.48118359 | 9.8602E-04 |
SGDE (2020) | 0.76078 | 0.32302 | 0.036377 | 53.71853 | 1.481184 | 9.8602E-04 |
CLJAYA (2020) | 0.76078 | 0.3230208 | 0.0363771 | 53.718521 | 1.481184 | 9.8602E-04 |
CPMPSO (2020) | 0.760776 | 0.323021 | 0.036377 | 53.71852 | 1.481184 | 9.8602E-04 |
NPSOPC (2020) | 0.7608 | 0.3325 | 0.03639 | 53.7583 | 1.4814 | 9.8856E-04 |
CBSA (2020) | 0.760776 | 0.323021 | 0.036377 | 53.71852 | 1.481184 | 9.8602E-04 |
LFBSA (2020) | 0.760776 | 0.323021 | 0.036377 | 53.71852 | 1.481184 | 9.8602E-04 |
IEO (2020) | 0.760775529 | 0.323 | 0.036377 | 53.71852 | 1.481183 | 9.8602E-04 |
ATLDE (2020) | 0.76077553 | 0.32302082 | 0.03637712 | 53.71852699 | 1.48118359 | 9.8602E-04 |
EJAYA (2021) | 0.76078 | 0.32302 | 0.03638 | 53.71852 | 1.48118 | 9.8602E-04 |
IGSK (2021) | 0.76077553 | 0.323 | 0.036377093 | 53.71852532 | 1.481183592 | 9.8602E-04 |
EABOA (2021) | 0.760771077 | 0.322929 | 0.036379593 | 53.76600144 | 1.481153457 | 9.8602E-04 |
SFLBS (2021) | 0.76078 | 0.323021 | 0.03638 | 53.7185 | 1.481184 | 9.8602E-04 |
RLDE (2021) | 0.7608 | 0.3231 | 0.0364 | 53.7185 | 1.4812 | 9.8602E-04 |
EJADE-D | 0.76077553 | 0.32302079 | 0.03637709 | 53.71852020 | 1.48118359 | 9.8602E-04 |
Algorithm | Ipv (A) | Isd1 (μA) | Rs (Ω) | Rp (Ω) | n1 | Isd2 (μA) | n2 | RMSE |
GOTLBO (2016) | 0.7608 | 0.8002 | 0.0368 | 56.0753 | 2 | 0.2205 | 1.4490 | 9.8318E-04 |
IJAYA (2017) | 0.7601 | 0.0050445 | 0.0376 | 77.8519 | 1.2186 | 0.75904 | 1.6247 | 9.8293E-04 |
SATLBO (2017) | 0.7608 | 0.2509 | 0.0366 | 55.1170 | 1.4598 | 0.5454 | 1.9994 | 9.8280E-04 |
CWOA (2017) | 0.76077 | 0.24150 | 0.03666 | 55.20160 | 1.45651 | 0.60000 | 1.98990 | 9.8272E-04 |
MSSO (2017) | 0.760748 | 0.234925 | 0.036688 | 55.714662 | 1.454255 | 0.671593 | 1.995305 | 9.8281E-04 |
IWOA (2018) | 0.7608 | 0.6771 | 0.0367 | 55.4082 | 2 | 0.2355 | 1.4545 | 9.8255E-04 |
HFAPS (2018) | 0.760781 | 0.225974 | 0.0367404 | 55.4855 | 1.45101 | 0.7493580 | 2.000000 | 9.8248E-04 |
TLABC (2018) | 0.76081 | 0.42394 | 0.03667 | 54.66797 | 1.90750 | 0.24011 | 1.45671 | 9.8415E-04 |
MLBSA (2018) | 0.7608 | 0.22728 | 0.0670 | 55.4612 | 1.4515 | 0.73835 | 2.0000 | 9.8249E-04 |
DE/WOA (2018) | 0.760781 | 0.225974 | 0.036740 | 55.485437 | 1.451017 | 0.749346 | 2.000000 | 9.8248E-04 |
OBWOA (2018) | 0.76076 | 0.22990 | 0.03671 | 55.3990 | 1.49154 | 0.61956 | 2.000000 | 9.8251E-04 |
PGJAYA (2019) | 0.7608 | 0.21031 | 0.0368 | 55.8135 | 1.4450 | 0.88534 | 2.0000 | 9.8263E-04 |
BHCS (2019) | 0.76078 | 0.74935 | 0.03674 | 55.48544 | 2.00000 | 0.22597 | 1.45102 | 9.8249E-04 |
FPSO (2019) | 0.76078 | 0.22731 | 0.036737 | 55.39230 | 1.45160 | 0.72786 | 1.99969 | 9.8253E-04 |
ILCOA (2019) | 0.76078 | 0.22601 | 0.036739 | 55.5320 | 1.45101 | 0.74921 | 2.00000 | 9.8257E-04 |
ITLBO (2019) | 0.7608 | 0.2260 | 0.0367 | 55.4854 | 1.4510 | 0.7493 | 2.0000 | 9.8248E-04 |
SGDE (2020) | 0.76079 | 0.28070 | 0.036480 | 54.3667 | 1.46966 | 0.24996 | 1.93228 | 9.8441E-04 |
BSARDVs (2020) | 0.760781 | 0.225808 | 0.036741 | 55.4878 | 1.45096 | 0.750861 | 2 | 9.8248E-04 |
ELBA (2020) | 0.760781 | 0.749338 | 0.03674 | 55.48544 | 2 | 0.225975 | 1.451018 | 9.8248E-04 |
EOTLBO (2020) | 0.76078108 | 0.22597468 | 0.03674043 | 55.48543568 | 1.45101692 | 0.74934431 | 2 | 9.8248E-04 |
CLJAYA (2020) | 0.76078 | 0.226051 | 0.03674 | 55.48599 | 1.45105 | 0.74876 | 1.99999 | 9.8249E-04 |
CPMPSO (2020) | 0.76078 | 0.74935 | 0.03674 | 55.48544 | 2 | 0.22597 | 1.45102 | 9.8248E-04 |
NPSOPC (2020) | 0.76078 | 0.25093 | 0.03663 | 55.117 | 1.45982 | 0.545418 | 1.99941 | 9.8208E-04 |
CBSA (2020) | 0.76078 | 0.2259739 | 0.03674 | 55.48544 | 1.451017 | 0.74935 | 2 | 9.8248E-04 |
LFBSA (2020) | 0.760781 | 0.225974 | 0.03674 | 55.48543 | 1.451017 | 0.749345 | 2 | 9.8249E-04 |
IEO (2020) | 0.760781 | 0.749 | 0.03674 | 55.48544 | 1.451016 | 0.226 | 1.999999 | 9.8248E-04 |
ATLDE (2020) | 0.76078108 | 0.22597412 | 0.03674043 | 55.48544744 | 1.45101671 | 0.74934885 | 2.00000000 | 9.8248E-04 |
EJAYA (2021) | 0.76078 | 0.22597 | 0.03674 | 55.48509 | 1.45102 | 0.74934 | 2 | 9.8248E-04 |
IGSK (2021) | 0.760781079 | 0.7493 | 0.036740429 | 55.48543425 | 2 | 0.226 | 1.451016893 | 9.8248E-04 |
EABOA (2021) | 0.76082865 | 0.25072 | 0.0366266 | 55.3660129 | 1.45988481 | 0.72069 | 1.99997318 | 9.8607E-04 |
SFLBS (2021) | 0.76077 | 0.775995 | 0.036755 | 55.5496 | 2 | 1.449857 | 9.8249E-04 | |
RLDE (2021) | 0.7608 | 0.226 | 0.0367 | 55.4847 | 2 | 0.7492 | 1.451 | 9.8248E-04 |
EJADE-D | 0.76078108 | 0.22597441 | 0.03674043 | 55.48543767 | 1.45101682 | 0.74934630 | 2.00000000 | 9.8248E-04 |
Algorithm | Ipv (A) | Isd (μA) | Rs (Ω) | Rp (Ω) | n | RMSE |
CWOA (2017) | 1.7 | 1.6338 | 0.0050 | 15.4 | 1.5 | 1.8000E-03 |
HFAPS (2018) | 1.6663 | 1.0703 | 0.24849 | 490.03 | 53.016 | 1.9700E-03 |
OBWOA (2018) | 1.6642 | 1.65025 | 0.0044 | 15.5299 | 1.51424 | 1.7530E-03 |
BHCS (2019) | 1.66390 | 1.73866 | 0.00427 | 15.92829 | 1.52030 | 1.7298E-03 |
FPSO (2019) | 1.2323 | 7.4732 | 0.0049 | 9.6889 | 1.2086 | 1.3000E-03 |
ILCOA (2019) | 1.2001 | 7.4812 | 0.0049 | 9.6991 | 1.2067 | 1.6932E-02 |
ITLBO (2019) | 1.6639 | 1.7387 | 0.0043 | 15.9283 | 1.5203 | 1.7298E-03 |
ELBA (2020) | 1.663905 | 1.738657 | 0.004274 | 15.928294 | 1.520305 | 1.7298E-03 |
IEO (2020) | 1.663904802 | 1.74 | 0.004274 | 15.92827 | 1.520303 | 1.7298E-03 |
ATLDE (2020) | 1.66390478 | 1.73865697 | 0.00427377 | 15.92829439 | 1.52030293 | 1.7298E-03 |
EJAYA (2021) | 1.6639 | 1.73866 | 0.00427 | 15.92829 | 1.5203 | 1.7298E-03 |
IGSK (2021) | 1.663904777 | 1.7387 | 0.004273771 | 15.92829435 | 1.520302921 | 1.7298E-03 |
RLDE (2021) | 1.6639 | 1.7387 | 0.00427 | 15.9283 | 1.5203 | 1.7298E-03 |
EJADE-D | 1.66390478 | 1.73865681 | 0.00427377 | 15.92829378 | 1.52030292 | 1.7298E-03 |
Algorithm | Ipv (A) | Isd (μA) | Rs (Ω) | Rp (Ω) | n | RMSE |
CWOA (2017) | 7.4760 | 1.2 | 0.00000490 | 9.7942 | 1.2069 | 1.7601E-02 |
ITLBO (2019) | 7.4725 | 2.335 | 0.0046 | 22.2199 | 1.2601 | 1.6601E-02 |
BHCS (2019) | 7.47253 | 2.33499 | 0.00459 | 22.21990 | 1.26010 | 1.6601E-02 |
IEO (2020) | 7.472531264 | 2.23 | 0.004595 | 22.21679 | 1.260101 | 1.6601E-02 |
ATLDE (2020) | 7.47252992 | 2.33499485 | 0.00459463 | 22.21989607 | 1.26010347 | 1.6601E-02 |
EJAYA (2021) | 7.47253 | 2.33499 | 0.00459 | 22.21989 | 1.2601 | 1.6601E-02 |
IGSK (2021) | 7.47252992 | 2.335 | 0.004594635 | 22.21989406 | 1.260103467 | 1.6601E-02 |
RLDE (2021) | 7.4725 | 2.335 | 0.0046 | 22.2199 | 1.2601 | 1.6601E-02 |
EJADE-D | 7.47252992 | 2.33499494 | 0.00459463 | 22.21989617 | 1.26010347 | 1.6601E-02 |
Step 1: | Firstly, load the measured I-V data of PV models provided by the manufacturers. |
Step 2: | Randomly initialize NP (population size) solutions of population, in which each solution Xi=x1 is initialized in their specified range. |
Step 3: | According to each solution, calculate the linear unknown parameters x2 by using the proposed search space decomposition technique i.e., Eqs (20) and (21). |
Step 4: | After all solution have been done, then, each solution's linear parameters x2 and nonlinear parameters x1 are substituted into Eq (10) to calculate the objective function value of each solution. |
Step 5: | Leverage the advanced differential evolution algorithm i.e., EJADE to produce NP new solution. |
Step 6: | Similar to the Step 3, calculate the linear parameters x2 according related nonlinear parameters x1 for each new solution by using search space decomposition technique. |
Step 7: | Calculate the objection function value of each solution based on its linear parameters x2 and corresponding nonlinear parameters x1. |
Step 8: | According to the objective function value, selection between the new solution and old solution to form the next generation population. If the termination condition is met, then go to Step 9; else go to the loop: Steps 5–8. |
Step 9: | Output the best solution. |
Parameter | The single/double diode model | The single based PV module | |
Solar cell model | R.T.C. France solar cell | STM6-40/36 | STP6-120/36 |
Ns | 1 | 36 | 36 |
Np | 1 | 1 | 1 |
Number of I-V data | 26 | 20 | 24 |
Temperature | 25 ℃ | 51 ℃ | 55 ℃ |
Radiation | 1000 W/m2 | 1000 W/m2 | 1000 W/m2 |
Unknown parameters | R.T.C. France solar cell | STM6-40/36 | STP6-120/36 | |||||
Lower | Upper | Lower | Upper | Lower | Upper | |||
Ipv (A) | 0 | 1 | 0 | 2 | 0 | 8 | ||
Isd, Isd1, Isd2 (μA) | 0 | 1 | 0 | 50 | 0 | 50 | ||
Rs (Ω) | 0 | 0.5 | 0 | 0.36 | 0 | 0.36 | ||
Rp (Ω) | 0 | 100 | 0 | 1000 | 0 | 1500 | ||
n, n1, n2 | 1 | 2 | 1 | 60 | 1 | 50 |
Algorithm | Parameter value |
DE | NP = 50, F = 0.5, CR = 0.9 |
JADE | NP = 50 |
EJADE | NPmax = 50, NPmin = 4 |
EJADE-D | NPmax = 50, NPmin = 4 |
Algorithm | RMSE | |||
Best | Worst | Mean | Std | |
DE | 1.3733E-03 | 4.3933E-03 | 2.2122E-03 | 6.30E-04 |
JADE | 2.4628E-03 | 9.0467E-03 | 4.6089E-03 | 1.64E-03 |
EJADE | 9.9336E-04 | 2.1651E-03 | 1.4245E-03 | 3.03E-04 |
EJADE-D | 9.8602E-04 | 9.8602E-04 | 9.8602E-04 | 3.67E-17 |
Algorithm | Ipv (A) | Isd (μA) | Rs (Ω) | Rp (Ω) | n | RMSE |
DE | 0.75946361 | 0.41068723 | 0.03569428 | 86.22084780 | 1.50561834 | 1.3733E-03 |
JADE | 0.76241894 | 0.65695921 | 0.03323643 | 80.94523627 | 1.55621196 | 2.4628E-03 |
EJADE | 0.76070190 | 0.34171649 | 0.03613743 | 55.62560314 | 1.48687849 | 9.9336E-04 |
EJADE-D | 0.76077553 | 0.32302079 | 0.03637709 | 53.71852020 | 1.48118359 | 9.8602E-04 |
Algorithm | RMSE | |||
Best | Worst | Mean | Std | |
DE | 1.4793E-03 | 4.5821E-03 | 2.4962E-03 | 6.93E-04 |
JADE | 2.2919E-03 | 5.1370E-03 | 3.4453E-03 | 7.37E-04 |
EJADE | 9.8497E-04 | 2.0383E-03 | 1.2113E-03 | 2.83E-04 |
EJADE-D | 9.8248E-04 | 9.8248E-04 | 9.8248E-04 | 5.50E-17 |
Algorithm | Ipv (A) | Isd1 (μA) | Rs (Ω) | Rp (Ω) | n1 | Isd2 (μA) | n2 | RMSE |
DE | 0.76161938 | 0.29934314 | 0.03458265 | 62.11841082 | 1.95055472 | 0.44875729 | 1.51798488 | 1.4793E-03 |
JADE | 0.75904695 | 0.13872632 | 0.03655444 | 47.70175773 | 1.43701409 | 0.16306212 | 1.52688650 | 2.2919E-03 |
EJADE | 0.76077378 | 0.24691111 | 0.03653971 | 54.42053982 | 1.46090798 | 0.21482898 | 1.76404886 | 9.8497E-04 |
EJADE-D | 0.76078108 | 0.22597441 | 0.03674043 | 55.48543767 | 1.45101682 | 0.74934630 | 2.00000000 | 9.8248E-04 |
Algorithm | RMSE | |||
Best | Worst | Mean | Std | |
DE | 1.0448E-02 | 2.5021E-01 | 7.4874E-02 | 6.90E-02 |
JADE | 5.6988E-03 | 1.3783E-02 | 7.5998E-03 | 1.71E-03 |
EJADE | 2.6987E-03 | 4.0918E-03 | 3.3056E-03 | 2.78E-04 |
EJADE-D | 1.7298E-03 | 1.7298E-03 | 1.7298E-03 | 9.85E-18 |
Algorithm | Ipv (A) | Isd (μA) | Rs (Ω) | Rp (Ω) | n | RMSE |
DE | 1.65602020 | 7.78528613 | 0.00090772 | 317.05546222 | 1.70743127 | 1.0448E-02 |
JADE | 1.65376894 | 5.35917258 | 0.00130049 | 289.11110965 | 1.65342824 | 5.6988E-03 |
EJADE | 1.66220446 | 3.98041243 | 0.00125715 | 20.23972593 | 1.61727931 | 2.6987E-03 |
EJADE-D | 1.66390478 | 1.73865681 | 0.00427377 | 15.92829378 | 1.52030292 | 1.7298E-03 |
Algorithm | RMSE | |||
Best | Worst | Mean | Std | |
DE | 2.2855E-02 | 2.4084E-01 | 5.7271E-02 | 5.53E-02 |
JADE | 3.2110E-02 | 2.5739E-01 | 4.8897E-02 | 3.97E-02 |
EJADE | 1.6601E-02 | 4.0248E-02 | 1.7799E-02 | 4.27E-03 |
EJADE-D | 1.6601E-02 | 1.6601E-02 | 1.6601E-02 | 1.55E-16 |
Algorithm | Ipv (A) | Isd (μA) | Rs (Ω) | Rp (Ω) | n | RMSE |
DE | 7.48185880 | 6.48830083 | 0.00406738 | 1124.25574692 | 1.35185994 | 2.2855E-02 |
JADE | 7.48402233 | 1.15549180 | 0.00369744 | 1359.20725027 | 1.41018636 | 3.2110E-02 |
EJADE | 7.47249358 | 2.33478056 | 0.00459468 | 22.26217949 | 1.26009565 | 1.6601E-02 |
EJADE-D | 7.47252992 | 2.33499494 | 0.00459463 | 22.21989617 | 1.26010347 | 1.6601E-02 |
Algorithm | RMSE | NFEs | |||
Best | Worst | Mean | Std | ||
GOTLBO (2016) | 9.8744E-04 | 1.9824E-03 | 1.3349E-03 | 2.09E-04 | 10,000 |
SATLBO (2017) | 9.8602E-04 | 9.9494E-03 | 9.8780E-04 | 2.30E-06 | 50,000 |
IJAYA (2017) | 9.8603E-04 | 1.0622E-03 | 9.9204E-04 | 1.40E-05 | 50,000 |
TLABC (2018) | 9.8602E-04 | 1.0397E-03 | 9.9852E-04 | 1.86E-05 | 50,000 |
MLBSA (2018) | 9.8602E-04 | 9.8602E-04 | 9.8602E-04 | 9.15E-12 | 50,000 |
DE/WOA (2018) | 9.8602E-04 | 9.8602E-04 | 9.8602E-04 | 3.55E-17 | 50,000 |
OBWOA (2018) | 9.8602E-04 | NA | 9.8603E-03 | 1.02E-08 | 1,500,000 |
ITLBO (2019) | 9.8602E-04 | 9.8602E-04 | 9.8602E-04 | 2.19E-17 | 50,000 |
PGJAYA (2019) | 9.8602E-04 | 9.8603E-04 | 9.8602E-04 | 1.45E-09 | 50,000 |
BHCS (2019) | 9.8602E-04 | 9.8602E-04 | 9.8602E-04 | 2.61E-17 | 50,000 |
FPSO (2019) | 9.8602E-04 | NA | NA | NA | NA |
ILCOA (2019) | 9.8602E-04 | NA | NA | 1.01E-08 | 10,000*NP |
BSARDVs (2020) | 9.8602E-04 | NA | NA | NA | 25,000 |
ELBA (2020) | 9.8602E-04 | 9.8602E-04 | 9.8602E-04 | 1.97E-17 | 50,000 |
EOTLBO (2020) | 9.8602E-04 | 9.8602E-04 | 9.8602E-04 | 4.13E-17 | 20,000 |
CLJAYA (2020) | 9.8602E-04 | NA | NA | NA | 20,000 |
CBSA (2020) | 9.8602E-04 | NA | NA | NA | 25,000 |
IEO (2020) | 9.8602E-04 | NA | NA | NA | 1,500,000 |
ATLDE (2020) | 9.8602E-04 | 9.8602E-04 | 9.8602E-04 | 2.44E-17 | 30,000 |
EJAYA (2021) | 9.8602E-04 | 9.8602E-04 | 9.8602E-04 | 6.80E-17 | 30,000 |
IGSK (2021) | 9.8602E-04 | 9.8602E-04 | 9.8602E-04 | 3.58E-17 | 10,000 |
EABOA (2021) | 9.8602E-04 | 9.8784E-04 | 9.8678E-04 | 9.30E-07 | 50,000 |
SFLBS (2021) | 9.8602E-04 | 9.8602E-04 | 9.8602E-04 | 1.43E-14 | 60,000 |
RLDE (2021) | 9.8602E-04 | 9.8602E-04 | 9.8602E-04 | 3.48E-17 | 30,000 |
EJADE-D | 9.8602E-04 | 9.8602E-04 | 9.8602E-04 | 3.67E-17 | 2000 |
Algorithm | RMSE | NFEs | |||
Best | Worst | Mean | Std | ||
GOTLBO (2016) | 9.8318E-04 | 1.7877E-03 | 1.2436E-03 | 2.09E-04 | 20,000 |
SATLBO (2017) | 9.8280E-04 | 1.0470E-03 | 9.9811E-04 | 1.95E-05 | 50,000 |
IJAYA (2017) | 9.8293E-04 | 1.4055E-03 | 1.0269E-03 | 9.83E-05 | 50,000 |
TLABC (2018) | 9.8415E-04 | 1.5048E-03 | 1.0555E-03 | 1.55E-04 | 50,000 |
MLBSA (2018) | 9.8249E-04 | 9.8798E-04 | 9.8518E-04 | 1.35E-06 | 50,000 |
DE/WOA (2018) | 9.8248E-04 | 9.8604E-04 | 9.8297E-04 | 9.15E-07 | 50,000 |
OBWOA (2018) | 9.8251E-04 | NA | 9.8294E-04 | 1.13E-07 | 1,500,000 |
PGJAYA (2019) | 9.8263E-04 | 9.9499E-04 | 9.8582E-04 | 2.54E-06 | 50,000 |
BHCS (2019) | 9.8249E-04 | 9.8687E-04 | 9.8380E-04 | 1.54E-06 | 50,000 |
FPSO (2019) | 9.8253E-04 | NA | NA | NA | NA |
ILCOA (2019) | 8.8257E-04 | NA | NA | 6.25E-07 | 10,000*NP |
ITLBO (2019) | 9.8248E-04 | 9.8812E-04 | 9.8497E-04 | 1.54E-06 | 50,000 |
BSARDVs (2020) | 9.8248E-04 | NA | NA | NA | 45,000 |
ELBA (2020) | 9.8248E-04 | 9.8615E-04 | 9.8349E-04 | 1.43E-06 | 50,000 |
EOTLBO (2020) | 9.8248E-04 | 9.8942E-04 | 9.8473E-04 | 1.69E-06 | 20,000 |
CLJAYA (2020) | 9.8249E-04 | NA | NA | NA | 48,000 |
CBSA (2020) | 9.8248E-04 | NA | NA | NA | 50,000 |
IEO (2020) | 9.8248E-04 | NA | NA | NA | 1,500,000 |
ATLDE (2020) | 9.8248E-04 | 9.8603E-04 | 9.8372E-04 | 1.37E-06 | 30,000 |
EJAYA (2021) | 9.8248E-04 | 9.8602E-04 | 9.8448E-04 | 1.51E-06 | 30,000 |
IGSK (2021) | 9.8248E-04 | 9.8602E-04 | 9.8273E-04 | 8.96E-07 | 20,000 |
EABOA (2021) | 9.8607E-04 | 1.0012E-03 | 9.9190E-04 | 6.62E-06 | 50,000 |
SFLBS (2021) | 9.8249E-04 | 9.8787E-04 | 9.8541E-04 | 1.79E-06 | 60,000 |
RLDE (2021) | 9.8248E-04 | 9.8695E-04 | 9.8457E-04 | 1.75E-06 | 30,000 |
EJADE-D | 9.8248E-04 | 9.8248E-04 | 9.8248E-04 | 5.50E-17 | 4,000 |
Algorithm | RMSE | NFEs | |||
Best | Worst | Mean | Std | ||
BHCS (2019) | 1.7298E-03 | 3.3299E-03 | 1.8365E-03 | 4.06E-04 | 50,000 |
ITLBO (2019) | 1.7298E-03 | 1.7298E-03 | 1.7298E-03 | 4.75E-18 | 50,000 |
ELBA (2020) | 1.7298E-03 | 1.7298E-03 | 1.7298E-03 | 6.16E-18 | 50,000 |
IEO (2020) | 1.7298E-03 | NA | NA | NA | 1,500,000 |
ATLDE (2020) | 1.7298E-03 | 1.7298E-03 | 1.7298E-03 | 8.22E-18 | 30,000 |
EJAYA (2021) | 1.7298E-03 | 1.7298E-03 | 1.7298E-03 | 1.47E-17 | 30,000 |
IGSK (2021) | 1.7298E-03 | 1.7298E-03 | 1.7298E-03 | 7.02E-18 | 15,000 |
RLDE (2021) | 1.7298E-03 | 1.7298E-03 | 1.7298E-03 | 1.58E-17 | 30,000 |
EJADE-D | 1.7298E-03 | 1.7298E-03 | 1.7298E-03 | 9.85E-18 | 3,000 |
Algorithm | RMSE | NFEs | |||
Best | Worst | Mean | Std | ||
BHCS (2019) | 1.6601E-02 | 1.3482E-01 | 2.4360E-02 | 2.61E-02 | 50,000 |
ITLBO (2019) | 1.6601E-02 | 1.6601E-02 | 1.6601E-02 | 7.22E-17 | 50,000 |
IEO (2020) | 1.6601E-02 | NA | NA | NA | 1,500,000 |
ATLDE (2020) | 1.6601E-02 | 1.6601E-02 | 1.6601E-02 | 1.02E-16 | 30,000 |
EJAYA (2021) | 1.6601E-02 | 1.6601E-02 | 1.6601E-02 | 2.68E-16 | 30,000 |
IGSK (2021) | 1.6601E-02 | 1.6601E-02 | 1.6601E-02 | 1.71E-16 | 15,000 |
RLDE (2021) | 1.6601E-02 | 1.6601E-02 | 1.6601E-02 | 1.98E-16 | 30,000 |
EJADE-D | 1.6601E-02 | 1.6601E-02 | 1.6601E-02 | 1.55E-16 | 7000 |
Algorithm | Ipv (A) | Isd (μA) | Rs (Ω) | Rp (Ω) | n | RMSE |
GOTLBO (2016) | 0.7608 | 0.3316 | 0.0363 | 54.1154 | 1.4838 | 9.8744E-04 |
IJAYA (2017) | 0.7608 | 0.3228 | 0.0364 | 53.7595 | 1.4811 | 9.8603E-04 |
SATLBO (2017) | 0.7608 | 0.3232 | 0.0363 | 53.7256 | 1.4812 | 9.8602E-04 |
CWOA (2017) | 0.76077 | 0.3239 | 0.03636 | 53.7987 | 1.4812 | 9.8602E-04 |
MSSO (2017) | 0.760777 | 0.323564 | 0.036370 | 53.742465 | 1.481244 | 9.8607E-04 |
IWOA (2018) | 0.7608 | 0.3232 | 0.0364 | 53.7317 | 1.4812 | 9.8602E-04 |
HFAPS (2018) | 0.760777 | 0.322622 | 0.0363819 | 53.6784 | 1.48106 | 9.8602E-04 |
TLABC (2018) | 0.76078 | 0.32302 | 0.03638 | 53.71636 | 1.48118 | 9.8602E-04 |
MLBSA (2018) | 0.7608 | 0.32302 | 0.0364 | 53.7185 | 1.4812 | 9.8602E-04 |
DE/WOA (2018) | 0.760776 | 0.323021 | 0.036377 | 53.718524 | 1.481184 | 9.8602E-04 |
OBWOA (2018) | 0.76077 | 0. 3232 | 0. 0363 | 53.6836 | 1.5208 | 9.8602E-04 |
PGJAYA (2019) | 0.7608 | 0.3230 | 0.0364 | 53.7185 | 1.4812 | 9.8602E-04 |
BHCS (2019) | 0.76078 | 0.32302 | 0.03638 | 53.71852 | 1.48118 | 9.8602E-04 |
FPSO (2019) | 0.76077552 | 0.323020 | 0.036370 | 53.718520 | 1.48110817 | 9.8602E-04 |
ILCOA (2019) | 0.760775 | 0.323021 | 0.036377 | 53.718679 | 1.481108 | 9.8602E-04 |
ITLBO (2019) | 0.7608 | 0.3230 | 0.0364 | 53.7185 | 1.4812 | 9.8602E-04 |
BSARDVs (2020) | 0.760776 | 0.323021 | 0.036377 | 53.718520 | 1.481184 | 9.8602E-04 |
ELBA (2020) | 0.760776 | 0.323021 | 0.036377 | 53.718523 | 1.481185 | 9.8602E-04 |
EOTLBO (2020) | 0.76077553 | 0.32302083 | 0.03637709 | 53.71852514 | 1.48118359 | 9.8602E-04 |
SGDE (2020) | 0.76078 | 0.32302 | 0.036377 | 53.71853 | 1.481184 | 9.8602E-04 |
CLJAYA (2020) | 0.76078 | 0.3230208 | 0.0363771 | 53.718521 | 1.481184 | 9.8602E-04 |
CPMPSO (2020) | 0.760776 | 0.323021 | 0.036377 | 53.71852 | 1.481184 | 9.8602E-04 |
NPSOPC (2020) | 0.7608 | 0.3325 | 0.03639 | 53.7583 | 1.4814 | 9.8856E-04 |
CBSA (2020) | 0.760776 | 0.323021 | 0.036377 | 53.71852 | 1.481184 | 9.8602E-04 |
LFBSA (2020) | 0.760776 | 0.323021 | 0.036377 | 53.71852 | 1.481184 | 9.8602E-04 |
IEO (2020) | 0.760775529 | 0.323 | 0.036377 | 53.71852 | 1.481183 | 9.8602E-04 |
ATLDE (2020) | 0.76077553 | 0.32302082 | 0.03637712 | 53.71852699 | 1.48118359 | 9.8602E-04 |
EJAYA (2021) | 0.76078 | 0.32302 | 0.03638 | 53.71852 | 1.48118 | 9.8602E-04 |
IGSK (2021) | 0.76077553 | 0.323 | 0.036377093 | 53.71852532 | 1.481183592 | 9.8602E-04 |
EABOA (2021) | 0.760771077 | 0.322929 | 0.036379593 | 53.76600144 | 1.481153457 | 9.8602E-04 |
SFLBS (2021) | 0.76078 | 0.323021 | 0.03638 | 53.7185 | 1.481184 | 9.8602E-04 |
RLDE (2021) | 0.7608 | 0.3231 | 0.0364 | 53.7185 | 1.4812 | 9.8602E-04 |
EJADE-D | 0.76077553 | 0.32302079 | 0.03637709 | 53.71852020 | 1.48118359 | 9.8602E-04 |
Algorithm | Ipv (A) | Isd1 (μA) | Rs (Ω) | Rp (Ω) | n1 | Isd2 (μA) | n2 | RMSE |
GOTLBO (2016) | 0.7608 | 0.8002 | 0.0368 | 56.0753 | 2 | 0.2205 | 1.4490 | 9.8318E-04 |
IJAYA (2017) | 0.7601 | 0.0050445 | 0.0376 | 77.8519 | 1.2186 | 0.75904 | 1.6247 | 9.8293E-04 |
SATLBO (2017) | 0.7608 | 0.2509 | 0.0366 | 55.1170 | 1.4598 | 0.5454 | 1.9994 | 9.8280E-04 |
CWOA (2017) | 0.76077 | 0.24150 | 0.03666 | 55.20160 | 1.45651 | 0.60000 | 1.98990 | 9.8272E-04 |
MSSO (2017) | 0.760748 | 0.234925 | 0.036688 | 55.714662 | 1.454255 | 0.671593 | 1.995305 | 9.8281E-04 |
IWOA (2018) | 0.7608 | 0.6771 | 0.0367 | 55.4082 | 2 | 0.2355 | 1.4545 | 9.8255E-04 |
HFAPS (2018) | 0.760781 | 0.225974 | 0.0367404 | 55.4855 | 1.45101 | 0.7493580 | 2.000000 | 9.8248E-04 |
TLABC (2018) | 0.76081 | 0.42394 | 0.03667 | 54.66797 | 1.90750 | 0.24011 | 1.45671 | 9.8415E-04 |
MLBSA (2018) | 0.7608 | 0.22728 | 0.0670 | 55.4612 | 1.4515 | 0.73835 | 2.0000 | 9.8249E-04 |
DE/WOA (2018) | 0.760781 | 0.225974 | 0.036740 | 55.485437 | 1.451017 | 0.749346 | 2.000000 | 9.8248E-04 |
OBWOA (2018) | 0.76076 | 0.22990 | 0.03671 | 55.3990 | 1.49154 | 0.61956 | 2.000000 | 9.8251E-04 |
PGJAYA (2019) | 0.7608 | 0.21031 | 0.0368 | 55.8135 | 1.4450 | 0.88534 | 2.0000 | 9.8263E-04 |
BHCS (2019) | 0.76078 | 0.74935 | 0.03674 | 55.48544 | 2.00000 | 0.22597 | 1.45102 | 9.8249E-04 |
FPSO (2019) | 0.76078 | 0.22731 | 0.036737 | 55.39230 | 1.45160 | 0.72786 | 1.99969 | 9.8253E-04 |
ILCOA (2019) | 0.76078 | 0.22601 | 0.036739 | 55.5320 | 1.45101 | 0.74921 | 2.00000 | 9.8257E-04 |
ITLBO (2019) | 0.7608 | 0.2260 | 0.0367 | 55.4854 | 1.4510 | 0.7493 | 2.0000 | 9.8248E-04 |
SGDE (2020) | 0.76079 | 0.28070 | 0.036480 | 54.3667 | 1.46966 | 0.24996 | 1.93228 | 9.8441E-04 |
BSARDVs (2020) | 0.760781 | 0.225808 | 0.036741 | 55.4878 | 1.45096 | 0.750861 | 2 | 9.8248E-04 |
ELBA (2020) | 0.760781 | 0.749338 | 0.03674 | 55.48544 | 2 | 0.225975 | 1.451018 | 9.8248E-04 |
EOTLBO (2020) | 0.76078108 | 0.22597468 | 0.03674043 | 55.48543568 | 1.45101692 | 0.74934431 | 2 | 9.8248E-04 |
CLJAYA (2020) | 0.76078 | 0.226051 | 0.03674 | 55.48599 | 1.45105 | 0.74876 | 1.99999 | 9.8249E-04 |
CPMPSO (2020) | 0.76078 | 0.74935 | 0.03674 | 55.48544 | 2 | 0.22597 | 1.45102 | 9.8248E-04 |
NPSOPC (2020) | 0.76078 | 0.25093 | 0.03663 | 55.117 | 1.45982 | 0.545418 | 1.99941 | 9.8208E-04 |
CBSA (2020) | 0.76078 | 0.2259739 | 0.03674 | 55.48544 | 1.451017 | 0.74935 | 2 | 9.8248E-04 |
LFBSA (2020) | 0.760781 | 0.225974 | 0.03674 | 55.48543 | 1.451017 | 0.749345 | 2 | 9.8249E-04 |
IEO (2020) | 0.760781 | 0.749 | 0.03674 | 55.48544 | 1.451016 | 0.226 | 1.999999 | 9.8248E-04 |
ATLDE (2020) | 0.76078108 | 0.22597412 | 0.03674043 | 55.48544744 | 1.45101671 | 0.74934885 | 2.00000000 | 9.8248E-04 |
EJAYA (2021) | 0.76078 | 0.22597 | 0.03674 | 55.48509 | 1.45102 | 0.74934 | 2 | 9.8248E-04 |
IGSK (2021) | 0.760781079 | 0.7493 | 0.036740429 | 55.48543425 | 2 | 0.226 | 1.451016893 | 9.8248E-04 |
EABOA (2021) | 0.76082865 | 0.25072 | 0.0366266 | 55.3660129 | 1.45988481 | 0.72069 | 1.99997318 | 9.8607E-04 |
SFLBS (2021) | 0.76077 | 0.775995 | 0.036755 | 55.5496 | 2 | 1.449857 | 9.8249E-04 | |
RLDE (2021) | 0.7608 | 0.226 | 0.0367 | 55.4847 | 2 | 0.7492 | 1.451 | 9.8248E-04 |
EJADE-D | 0.76078108 | 0.22597441 | 0.03674043 | 55.48543767 | 1.45101682 | 0.74934630 | 2.00000000 | 9.8248E-04 |
Algorithm | Ipv (A) | Isd (μA) | Rs (Ω) | Rp (Ω) | n | RMSE |
CWOA (2017) | 1.7 | 1.6338 | 0.0050 | 15.4 | 1.5 | 1.8000E-03 |
HFAPS (2018) | 1.6663 | 1.0703 | 0.24849 | 490.03 | 53.016 | 1.9700E-03 |
OBWOA (2018) | 1.6642 | 1.65025 | 0.0044 | 15.5299 | 1.51424 | 1.7530E-03 |
BHCS (2019) | 1.66390 | 1.73866 | 0.00427 | 15.92829 | 1.52030 | 1.7298E-03 |
FPSO (2019) | 1.2323 | 7.4732 | 0.0049 | 9.6889 | 1.2086 | 1.3000E-03 |
ILCOA (2019) | 1.2001 | 7.4812 | 0.0049 | 9.6991 | 1.2067 | 1.6932E-02 |
ITLBO (2019) | 1.6639 | 1.7387 | 0.0043 | 15.9283 | 1.5203 | 1.7298E-03 |
ELBA (2020) | 1.663905 | 1.738657 | 0.004274 | 15.928294 | 1.520305 | 1.7298E-03 |
IEO (2020) | 1.663904802 | 1.74 | 0.004274 | 15.92827 | 1.520303 | 1.7298E-03 |
ATLDE (2020) | 1.66390478 | 1.73865697 | 0.00427377 | 15.92829439 | 1.52030293 | 1.7298E-03 |
EJAYA (2021) | 1.6639 | 1.73866 | 0.00427 | 15.92829 | 1.5203 | 1.7298E-03 |
IGSK (2021) | 1.663904777 | 1.7387 | 0.004273771 | 15.92829435 | 1.520302921 | 1.7298E-03 |
RLDE (2021) | 1.6639 | 1.7387 | 0.00427 | 15.9283 | 1.5203 | 1.7298E-03 |
EJADE-D | 1.66390478 | 1.73865681 | 0.00427377 | 15.92829378 | 1.52030292 | 1.7298E-03 |
Algorithm | Ipv (A) | Isd (μA) | Rs (Ω) | Rp (Ω) | n | RMSE |
CWOA (2017) | 7.4760 | 1.2 | 0.00000490 | 9.7942 | 1.2069 | 1.7601E-02 |
ITLBO (2019) | 7.4725 | 2.335 | 0.0046 | 22.2199 | 1.2601 | 1.6601E-02 |
BHCS (2019) | 7.47253 | 2.33499 | 0.00459 | 22.21990 | 1.26010 | 1.6601E-02 |
IEO (2020) | 7.472531264 | 2.23 | 0.004595 | 22.21679 | 1.260101 | 1.6601E-02 |
ATLDE (2020) | 7.47252992 | 2.33499485 | 0.00459463 | 22.21989607 | 1.26010347 | 1.6601E-02 |
EJAYA (2021) | 7.47253 | 2.33499 | 0.00459 | 22.21989 | 1.2601 | 1.6601E-02 |
IGSK (2021) | 7.47252992 | 2.335 | 0.004594635 | 22.21989406 | 1.260103467 | 1.6601E-02 |
RLDE (2021) | 7.4725 | 2.335 | 0.0046 | 22.2199 | 1.2601 | 1.6601E-02 |
EJADE-D | 7.47252992 | 2.33499494 | 0.00459463 | 22.21989617 | 1.26010347 | 1.6601E-02 |