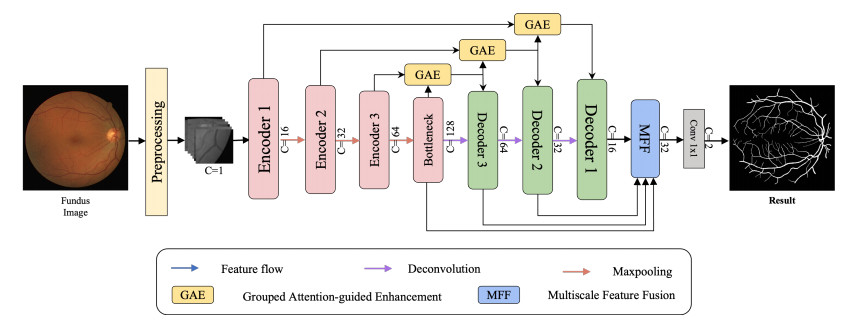
Retinal vessel segmentation plays a vital role in the clinical diagnosis of ophthalmic diseases. Despite convolutional neural networks (CNNs) excelling in this task, challenges persist, such as restricted receptive fields and information loss from downsampling. To address these issues, we propose a new multi-fusion network with grouped attention (MAG-Net). First, we introduce a hybrid convolutional fusion module instead of the original encoding block to learn more feature information by expanding the receptive field. Additionally, the grouped attention enhancement module uses high-level features to guide low-level features and facilitates detailed information transmission through skip connections. Finally, the multi-scale feature fusion module aggregates features at different scales, effectively reducing information loss during decoder upsampling. To evaluate the performance of the MAG-Net, we conducted experiments on three widely used retinal datasets: DRIVE, CHASE and STARE. The results demonstrate remarkable segmentation accuracy, specificity and Dice coefficients. Specifically, the MAG-Net achieved segmentation accuracy values of 0.9708, 0.9773 and 0.9743, specificity values of 0.9836, 0.9875 and 0.9906 and Dice coefficients of 0.8576, 0.8069 and 0.8228, respectively. The experimental results demonstrate that our method outperforms existing segmentation methods exhibiting superior performance and segmentation outcomes.
Citation: Yun Jiang, Jie Chen, Wei Yan, Zequn Zhang, Hao Qiao, Meiqi Wang. MAG-Net : Multi-fusion network with grouped attention for retinal vessel segmentation[J]. Mathematical Biosciences and Engineering, 2024, 21(2): 1938-1958. doi: 10.3934/mbe.2024086
[1] | Jinke Wang, Lubiao Zhou, Zhongzheng Yuan, Haiying Wang, Changfa Shi . MIC-Net: multi-scale integrated context network for automatic retinal vessel segmentation in fundus image. Mathematical Biosciences and Engineering, 2023, 20(4): 6912-6931. doi: 10.3934/mbe.2023298 |
[2] | Chen Yue, Mingquan Ye, Peipei Wang, Daobin Huang, Xiaojie Lu . SRV-GAN: A generative adversarial network for segmenting retinal vessels. Mathematical Biosciences and Engineering, 2022, 19(10): 9948-9965. doi: 10.3934/mbe.2022464 |
[3] | G. Prethija, Jeevaa Katiravan . EAMR-Net: A multiscale effective spatial and cross-channel attention network for retinal vessel segmentation. Mathematical Biosciences and Engineering, 2024, 21(3): 4742-4761. doi: 10.3934/mbe.2024208 |
[4] | Caixia Zheng, Huican Li, Yingying Ge, Yanlin He, Yugen Yi, Meili Zhu, Hui Sun, Jun Kong . Retinal vessel segmentation based on multi-scale feature and style transfer. Mathematical Biosciences and Engineering, 2024, 21(1): 49-74. doi: 10.3934/mbe.2024003 |
[5] | Yinlin Cheng, Mengnan Ma, Liangjun Zhang, ChenJin Jin, Li Ma, Yi Zhou . Retinal blood vessel segmentation based on Densely Connected U-Net. Mathematical Biosciences and Engineering, 2020, 17(4): 3088-3108. doi: 10.3934/mbe.2020175 |
[6] | Yanxia Sun, Xiang Li, Yuechang Liu, Zhongzheng Yuan, Jinke Wang, Changfa Shi . A lightweight dual-path cascaded network for vessel segmentation in fundus image. Mathematical Biosciences and Engineering, 2023, 20(6): 10790-10814. doi: 10.3934/mbe.2023479 |
[7] | Jinzhu Yang, Meihan Fu, Ying Hu . Liver vessel segmentation based on inter-scale V-Net. Mathematical Biosciences and Engineering, 2021, 18(4): 4327-4340. doi: 10.3934/mbe.2021217 |
[8] | Tong Shan, Jiayong Yan, Xiaoyao Cui, Lijian Xie . DSCA-Net: A depthwise separable convolutional neural network with attention mechanism for medical image segmentation. Mathematical Biosciences and Engineering, 2023, 20(1): 365-382. doi: 10.3934/mbe.2023017 |
[9] | Rafsanjany Kushol, Md. Hasanul Kabir, M. Abdullah-Al-Wadud, Md Saiful Islam . Retinal blood vessel segmentation from fundus image using an efficient multiscale directional representation technique Bendlets. Mathematical Biosciences and Engineering, 2020, 17(6): 7751-7771. doi: 10.3934/mbe.2020394 |
[10] | Jun Liu, Zhenhua Yan, Chaochao Zhou, Liren Shao, Yuanyuan Han, Yusheng Song . mfeeU-Net: A multi-scale feature extraction and enhancement U-Net for automatic liver segmentation from CT Images. Mathematical Biosciences and Engineering, 2023, 20(5): 7784-7801. doi: 10.3934/mbe.2023336 |
Retinal vessel segmentation plays a vital role in the clinical diagnosis of ophthalmic diseases. Despite convolutional neural networks (CNNs) excelling in this task, challenges persist, such as restricted receptive fields and information loss from downsampling. To address these issues, we propose a new multi-fusion network with grouped attention (MAG-Net). First, we introduce a hybrid convolutional fusion module instead of the original encoding block to learn more feature information by expanding the receptive field. Additionally, the grouped attention enhancement module uses high-level features to guide low-level features and facilitates detailed information transmission through skip connections. Finally, the multi-scale feature fusion module aggregates features at different scales, effectively reducing information loss during decoder upsampling. To evaluate the performance of the MAG-Net, we conducted experiments on three widely used retinal datasets: DRIVE, CHASE and STARE. The results demonstrate remarkable segmentation accuracy, specificity and Dice coefficients. Specifically, the MAG-Net achieved segmentation accuracy values of 0.9708, 0.9773 and 0.9743, specificity values of 0.9836, 0.9875 and 0.9906 and Dice coefficients of 0.8576, 0.8069 and 0.8228, respectively. The experimental results demonstrate that our method outperforms existing segmentation methods exhibiting superior performance and segmentation outcomes.
Globally, hundreds of millions of people suffer from eye diseases. Conditions such as diabetic retinopathy, cataracts, age-related macular degeneration, uncorrected refractive errors and glaucoma contribute significantly to visual impairment [1]. Among various fundus pathologies, changes in the morphology and structure of retinal vessels are frequently observed, carrying vital information about the overall health of the eye and the body. Hence, segmentation of the retinal vessels holds significant importance for the early screening, diagnosis and treatment of related diseases. Initially, specialists relied on the manual segmentation of retinal vessels, which is a laborious, monotonous and time-consuming task [2], particularly when dealing with a large number of segments. Therefore, the development of computer-aided diagnostic systems for the rapid and efficient automated segmentation of fundus vessels has become immensely important for ocular medical research and applications.
However, due to its own characteristics, there are a number of challenges in the retinal vessel segmentation task. First, retinal images often exhibit uneven brightness [3], resulting in low contrast between the blood vessels and the background, particularly in capillaries, which are difficult to discern visually. Second, the presence of hemorrhages, hard exudates and other interferences in the fundus retinal image, which are affected by the lesion, can cause significant noise in vessel segmentation [4]. Lastly, the retinal vasculature is highly structured. Unlike polyp and skin datasets, retinal vessels start from the fovea and gradually spread throughout the eye varying in thickness and overlapping with each other, which makes segmentation more difficult.
In the past, numerous researchers have dedicated their efforts to extracting the structure and morphology of the retina from fundus images. Currently, the primary segmentation methods can be categorized into two groups: traditional methods using hand-designed features and methods employing deep learning. Traditional segmentation methods, which extract the vascular structure directly by building algorithmic models, can be broadly classified into matched filtering methods [5], morphological [6] and mathematical modeling methods [7], vascular tracking methods [8] and variable model methods [9]. Although traditional methods have shown progress in retinal vessel segmentation, they heavily depend on manual feature extraction and parameter selection, which can be labor-intensive. Moreover, these methods yield poor segmentation accuracy for processes of capturing vessel endings and cannot meet the requirements of clinical practice.
With the continuous development of deep learning, the proposal of convolutional neural networks has led to impressive achievements in medical image segmentation. Fully convolutional networks (FCNs) [10] constitute the basis of the first method to use convolutional neural networks for semantic segmentation. Fu et al. [11] introduced a network model called DeepVessel which utilizes an FCN as a framework and incorporates multi-scale features and conditional random fields. At that time DeepVessel achieved remarkable results on tasks of retinal vessel segmentation. Following this, Ronneberger et al. [12] proposed a classical network known as U-Net, which demonstrated excellent feature extraction capability and outstanding performance. Since then, U-Net and its variants [13,14,15,16] are still the most popular segmentation models in medical image processing and are widely employed for medical image segmentation tasks, such as retinal vessel segmentation. Nevertheless, these methods exhibit certain limitations in attempts to achieve high-precision retina segmentation. These include a restricted receptive domain, the loss of detailed information caused by changes in feature map size and a decline in segmentation accuracy, as attributed to the semantic gap.
To solve the above problems, we propose an efficient and flexible multi-fusion network with grouped attention (MAG-Net) for accurate retinal vessel segmentation. First, we use the classical data preprocessing scheme proposed by Jiang et al. [17] to initially alleviate the problems of uneven brightness in fundus images. Second, we adopt a U-shaped encoder-decoder structure [18], which performs well on denoising tasks, and introduce depthwise separable convolution and atrous convolution into the encoder. These operations enable enlargement of receptive field without inflation of the parameters and ensure that the feature map can learn more long-range contextual information. Additionally, we introduce a grouped attention enhancement (GAE) module for skip connections to further enhance the traditional channel attention method. By using high-level features to complement low-level features, GAE aims to reduce the semantic gap between the encoder and decoder. Finally, to further improve the model's learning capability, we propose a multi-scale feature fusion (MFF) module. This module integrates features from different decoder levels to obtain a more comprehensive and precise feature representation, helping the image restoration.
Our primary contributions can be divided into the following points:
1) We propose a new multi-fusion network with grouped attention that enhances feature extraction capability and improves model accuracy and robustness.
2) We introduce a grouped attention fusion module that facilitates information interaction between the encoder and decoder, preserving valuable information across different levels of features.
3) We have evaluated the proposed model on publicly available datasets (DRIVE, CHASE and STARE), and the results demonstrate the model's strong segmentation performance and overall effectiveness.
In recent years, U-Net and its variant models have demonstrated excellent segmentation capabilities on tasks of retinal vessel segmentation. The incorporation of innovative optimization mechanisms, including convolutional strategies [19], feature fusion techniques [20] and attention mechanisms [21], has further enhanced the segmentation performance of these models.
In traditional convolutional neural networks, the application of the receptive field plays a crucial role in segmentation [22]. How to improve the receptive field and enhance the feature extraction ability has been a popular research topic. DUNet [23] introduces deformable convolution, which adaptively adjusts the receptive field based on the scale and shape of the vessels. This adjustment allows the model to capture retinal vessels with diverse shapes and scales. SCS-Net [24] is a novel scale and context sensitive network for retinal vessel segmentation, and it includes a scale-aware feature aggregation module to dynamically adjust the receptive field to obtain multi-scale information. Zhang et al. [25] proposed a semi-isotropic model that maintains a relatively large receptive field at different stages of the convolutional network, demonstrating excellent segmentation performance. To enhance the feature extraction capability, Liu et al. proposed a ResDO-UNet [26] and applied it to retinal blood vessel segmentation by combining it with the DO-conv [27] layer. MCDAU-Net [28] was designed with a cascading dilated spatial pyramid pool module that enhances the receptive field and generates feature maps that are rich in contextual information.
In recent years, attention mechanisms have transcended their initial application of natural language processing and gained significant traction in medical image segmentation, especially on tasks of retinal vessel segmentation. For example, Guo et al. [21] proposed a lightweight network called SA-UNet, which incorporates channel attention to generate a spatial attention map of features along the spatial dimension for adaptive feature refinement. FANet [29] introduces a novel approach by integrating the current training features with the mask generated in the previous iteration to generate hard attention, which aims to suppress unwanted feature clutter. WA-Net [30] has been proposed as a width attention-based convolutional neural network that weighs the channel relevance via a width attention module, which considers the location and position correlations of feature maps. To overcome the difficulty of segmenting small blood vessels, a generator with attention augmented convolution has been proposed in [31] to highlight the region of interest in the whole image. DOLG-NeXt [32] integrates SE-Net and ConvNeXt, which overcomes the problem that the large transformer-based model performs poorly in small biomedical image environments, as well as realizes effective feature extraction and fine target segmentation.
While these methods have excelled on tasks of retinal vessel segmentation, they are not without limitations. Challenges such as the loss of fine-grained details during upsampling, significant computational overhead and the inclusion of excessive irrelevant information in attention mechanisms still persist.
The complex structure of blood vessels in fundus retinal images, which are susceptible to uneven illumination and noise, poses challenges for retinal vessel segmentation. To improve the effectiveness of retinal vessel segmentation, we propose the MAG-Net, a multi-fusion network with grouped attention.
Figure 1 illustrates the overall network structure of the MAG-Net, which consists of three components: the encoder-decoder structure, GAE module and MFF module. The backbone of our model is based on the three-layer U-Net structure, renowned for its exceptional performance and versatility on diverse medical image segmentation tasks. Initially, the input image is preprocessed and divided into greyscale images of size 48 × 48, which are then fed into the encoder. To downsample the feature maps, a maximum pooling operation is applied between the encoder blocks, reducing the dimensions by half. Similarly, a transposed convolution operation is employed between the decoder blocks to double the size of the feature maps. The bottleneck is composed of two convolution operations followed by batch normalization and ReLU activation. The letter C illustrates the changes in the feature maps for channels at each stage in Figure 1. In the MAG-Net, the hybrid convolutional fusion (HCF) module was first designed to obtain a larger receptive field and better feature extraction capability. Second, the GAE module is utilized to establish connections between corresponding encoder and decoder layers, facilitating the utilization of multi-scale features and enhancing the integration of detailed information from different locations. Finally, the MFF module combines feature maps generated at different scales in the decoder to better capture information at different levels and scales in the image. The three important modules are described in detail below.
To enhance the extraction capability of the encoder, the HCF module has been incorporated, and each original encoder module has been replaced by an HCF module. The HCF module combines depthwise separable convolutions and a spatial attention module, as illustrated in Figure 2. By employing depthwise convolutions with varying dilation rates, the HCF module encodes the fused features through element-level summation. Feature maps are then fed into the spatial attention module, making the network prioritize the vasculature. Next, a pointwise convolution is applied to perform linear transformations and nonlinear activations on individual pixels in each channel. Finally, we use a standard convolution operation, followed by DropBlock to prevent overfitting and accelerate the network convergence.
Spatial attention is a machine learning technique that is commonly used in image segmentation tasks; it emphasizes the interaction between features at different locations in spatial dimensions to help extract local features. The spatial attention module first performs global average pooling and global maximum pooling operations on the input features F∈RH×W×C along the channel dimension to obtain global information in the form of Fsavg∈RH×W×1 and Fsmax∈RH×W×1, and it subsequently generates spatial attention weights Ms∈RH×W×1 applying a 7 × 7 convolution operation and a sigmoid activation function. Finally, the spatial attention weights are multiplied element-wise with the input features and aggregated to obtain the output features Fs, thus allowing the network to focus on features at different locations for enhancement. In summary, the output features Fs can be expressed as
Fs=F⋅Ms=F⋅δ(f7×7[Fsavg;Fsmax])=F⋅δ(f7×7[avgpooling(F);maxpooling(F)]) | (3.1) |
where δ denotes a sigmoid function, f7×7 denotes a 7 × 7 convolution operation and [;] represents channel concatenation.
The integration of skip connections in U-Net facilitates information exchange between the encoder and the decoder, preventing information loss and enhancing training efficiency. However, the presence of skip connections can lead to a semantic gap problem, as caused by information distortion and loss within the encoder and the decoder. To mitigate this issue, the GAE module has been introduced into the skip connection. This module incorporates feature maps from different levels and utilizes high-level features, which contain rich category information, to guide the low-level features. The goal is to achieve more precise segmentation of intricate details.
The channel attention module, which is often used to selectively generate importance weights for each channel, is an integral component of the GAE module. The module details are depicted in Figure 3. Assuming that Xi denotes the input feature and H, W and C denote its height, width and number of input channels respectively. The channels are first compressed by using global average pooling Pavg and global maximum pooling Pmax to obtain the outputs Pavg(Xi)∈R1×1×C and Pmax(Xi)∈R1×1×C. This approach allows the pooling pixels to be taken into account and the important features to be extracted. A multi-layer perceptron (MLP) is used to stimulate the channels and adaptively recalibrate the channel relationships. The MLP consists of two fully connected layers with the first fully connected layer having an output channel number of C/r, where r=4. Subsequently, a ReLU activation function is introduced, and the output channel number of the second fully connected layer is restored to C. Subsequently, the stimulated results are fed into a sigmoid function and summed to obtain the channel attention weights βi. The exact computational procedure can be expressed as follows:
βi=δ(Ms(Pavg(Xi)))+δ(Ms(Pmax(Xi))) | (3.2) |
The specific structure of the GAE module is shown in Figure 4.
First, we use standard and transposed convolution operations to resize the low-level and high-level features in the encoder, respectively, to the same size and concatenate them into multi-scale features along the channel dimension. The multi-scale features are given as follows:
MF=[f3×3(Flow);up(Fhigh)] | (3.3) |
where Flow indicates the low-level features and Fhigh indicates the high-level features. Additionally, MF denotes the multi-scale features, f3×3 denotes a 3 × 3 standard convolution and up() means a transposed convolution operation.
Second, we employ a channel attention module to extract the channel attention weights of the feature maps at different scales, denoted as β1 and β2, respectively. The detailed calculation will be explained in the next paragraph. The multi-scale channel attention weights are obtained by cascading, and they can be expressed as follows:
β=[β1;β2] | (3.4) |
where β is the multi-scale channel attention weight. Next, a softmax function is applied to β to obtain the recalibrated soft attention atti. atti contains the complete spatial information and the attention weights on the channel dimensions that facilitate the interaction between a particular feature channel attention and another feature channel attention. This operation is defined as follows:
atti=Softmax(βi)=exp(βi)1∑i=0exp(βi),i=0,1 | (3.5) |
att is obtained by concatenating atti to denote the multi-scale channel attention weights after channel attention interactions, and it is expressed as follows:
att=[att1;att2] | (3.6) |
Subsequently, the recalibrated multi-scale channel attention weight att is multiplied by the multi-scale features to obtain the feature Fc. Finally, Fc is the output after applying a 1 × 1 convolution operation, which rescales channels and sums with adjusted low-level features.
Fc=f3×3(Flow)+f1×1(MF×att) | (3.7) |
where f1×1 denotes a 1 × 1 convolution operation.
Due to the multivariate shape and structure of fundus vessels, existing vessel segmentation methods still exhibit limitations. To overcome the problem of feature adaptation for retinal vessels at different scales, we introduced the MFF module. This module leverages bilinear interpolation upsampling to complete the semantic interaction between neighboring low-level features and high-level features as well as the aggregation of features at different scales. It merges spatial and channel information, obtaining detailed local and global information. The structure of the MFF module is shown in Figure 5.
Specifically, the MFF module initiates at the bottleneck which is positioned between the encoder and decoder. It performs a level-by-level decoding process while employing a 1 × 1 convolution operation at different scales, reducing the number of feature channels to 16 to decrease the computational effort. Next, the MFF module conducts an operation by applying bottom-up bilinear interpolation along the decoder, thus merging with the adjacent upper-level feature maps via element-by-element summation. Thereafter, a nonlinear activation function, ReLU, is applied to improve the fitting of the nonlinear model and the nonlinear transformation. After upsampling and feature merging, the feature map achieves a flexible and adaptive feature transformation and incorporates differences in information between scales, complementing the spatial and channel information. In addition, the MFF module employs upsampling and cascades the activated features from each layer through bilinear interpolation to obtain multi-scale cascaded features (MCFs). Finally, through a series of convolution operations, batch normalization and nonlinear activation, the MCFs recombine the spatial and channel information to generate multi-scale aggregated features, which are cascaded with an output feature map along the decoder to obtain the final output.
We validated our method on three standard public datasets (DRIVE, CHASE, STARE). These datasets are described in detail as follows:
1) DRIVE [33]. This dataset contains 40 digital retinal images, namely, the corresponding groundtruth images and the corresponding masked images, 20 of which are used for training and the other 20 for testing. The size of each image is 565 × 584 pixels, and each image contains annotations of retinal regions and vascular regions.
2) CHASE [34]. This dataset contains 28 digital retinal images, namely, the corresponding groundtruth images and the corresponding masked images, 14 of which are normal retinal images, and the other 14 are retinal images with lesions. The size of each image is 999 × 960 pixels. Twenty images were set as the training set, and the other eight images were set as the test set.
3) STARE [35]. This dataset contains 20 fundus images, namely, the corresponding groundtruth images and the corresponding masked images with an image size of 605 × 700 pixels. We divided the dataset with the first 10 images used for training and the last 10 images used for testing.
The segmentation performance of retinal vessels was quantitatively evaluated in this study by using several metrics: Dice coefficient, accuracy, sensitivity and specificity. These metrics were assessed by using a confusion matrix. The Dice coefficient measures the similarity between the predicted and labeled results, while the accuracy represents the ratio of correctly segmented pixels to the total pixels providing an overall assessment of segmentation accuracy. Sensitivity evaluates the model's ability to segment the vascular region by measuring the proportion of correctly segmented positive samples. Specificity quantifies the model's capability to segment non-vascular regions by measuring the proportion of correctly segmented negative samples. Accuracy reflects the accuracy of the model's prediction as a positive sample. Each metric is defined as follows:
Dice=2×TP2×TP+FN+FP | (4.1) |
Accuracy=TP+TNTP+FN+TN+FP | (4.2) |
Sensitivity=TPTP+FN | (4.3) |
Specificity=TNTN+FP | (4.4) |
Precision=TPTP+FP | (4.5) |
where true positive (TP) represents correctly segmented vessel pixels, true negative (TN) represents correctly segmented background pixels, false positive (FP) denotes the background pixels incorrectly segmented as vessel pixels and false negative (FN) denotes the vessel pixels incorrectly segmented as background pixels.
Our deep learning method, implemented through the use of a PyTorch framework, was evaluated on an Ubuntu 64-bit operating system using a QuadroRTX 6000 server. During training, we employed a random patch approach with a patch size set to 48 × 48 pixels and a total number of 104800 patches. The model underwent 100 iterations with an initial learning rate of 0.001. For the DRIVE dataset and CHASE dataset, the training batch size was 128 and the threshold was 0.48. For the STARE dataset, we set the batch size to 64 and the threshold to 0.48. The model was optimized by using the Adam optimizer, with the exponential decay rate β1 = 0.9, β2 = 0.999 and the momentum ϵ = 1 × 10−8.
The loss function we use is the cross-entropy loss function, defined as follows:
Lossce(y,ˆy)=−∑^yilog^yi+(1−yi)log(1−^yi) | (5.1) |
where yi denotes the true label and ^yi represents the predicted label.
The problem of uneven brightness in the original retinal image significantly interferes with automatic segmentation. Preprocessing of the fundus images can effectively alleviate this problem. We have processed the original images by employing the following four steps and the effect of each preprocessing step was as shown in Figure 6.
1) Three-channel fusion of the color images to convert the corresponding greyscale images;
2) Normalization of greyscale images to mean and standard deviation to improve the convergence speed of the model;
3) Adaptive histogram equalization (CLAHE) of greyscale images to increase the contrast between the blood vessels and the background and suppress noise;
4) Gamma correction for vessel images to suppress illumination unevenness and centerline reflections.
To verify the validity of each component in the MAG-Net, we conducted ablation experiments on the DRIVE, CHASE and STARE datasets. Specifically, we evaluated the impact of HCF, GAE and MFF, individually. Our baseline model was U-Net, while the standard model encompassed U-Net augmented with HCF, GAE and MFF. In the experimental setup, we also examined models without specific components, denoted as "w/o HCF", "w/o GAE" and "w/o MFF", respectively. The experimental results, displayed in Tables 1–3, highlight the most favorable outcomes for better visibility and analysis.
Model | AUC_ROC | Sp | Se | Acc | Dice |
baseline | 0.9891 | 0.9856 | 0.8375 | 0.9704 | 0.8529 |
w/o HCF | 0.989 | 0.9846 | 0.8474 | 0.9705 | 0.8549 |
w/o GAE | 0.9892 | 0.9837 | 0.8544 | 0.9705 | 0.8556 |
w/o MFF | 0.9891 | 0.9842 | 0.8473 | 0.9701 | 0.8532 |
ours | 0.9895 | 0.9836 | 0.8588 | 0.9708 | 0.8576 |
Model | AUC_ROC | Sp | Se | Acc | Dice |
baseline | 0.9867 | 0.9883 | 0.7876 | 0.9765 | 0.7970 |
w/o HCF | 0.9878 | 0.9885 | 0.7957 | 0.9772 | 0.8031 |
w/o GAE | 0.9878 | 0.9872 | 0.8104 | 0.9769 | 0.8040 |
w/o MFF | 0.9880 | 0.9876 | 0.8014 | 0.9767 | 0.8009 |
ours | 0.9884 | 0.9875 | 0.8123 | 0.9773 | 0.8069 |
Model | AUC_ROC | Pr | Sp | Se | Acc | Dice |
baseline | 0.9886 | 0.9166 | 0.9932 | 0.7461 | 0.9742 | 0.8161 |
w/o HCF | 0.9895 | 0.9195 | 0.9929 | 0.7578 | 0.9748 | 0.8219 |
w/o GAE | 0.9890 | 0.9138 | 0.9915 | 0.7604 | 0.9738 | 0.8167 |
w/o MFF | 0.9882 | 0.9141 | 0.9915 | 0.7676 | 0.9743 | 0.8208 |
ours | 0.9895 | 0.9158 | 0.9906 | 0.7781 | 0.9743 | 0.8228 |
As can be seen in Tables 1–3, the MAG-Net achieved better overall performance compared than the baseline model. The area under the receiver operating characteristic curve (AUC_ROC), accuracy, sensitivity and Dice coefficient for the MAG-Net respectively reached 0.9895, 0.9708, 0.8588 and 0.8576 on the DRIVE dataset, 0.9884, 0.9773, 0.8123 and 0.8069 on the CHASE dataset and 0.9895, 0.7781, 0.9743 and 0.8228 on the STARE dataset. When we removed the HCF module from the standard model and replaced it with the standard convolution operation in U-Net, the model showed a slight decrease of 0.04 and 1.14% for the AUC_ROC and 0.06 and 1.66% for sensitivity on the DRIVE dataset and CHASE dataset, respectively, and a decrease of 2.01% for sensitivity on the STARE dataset. It demonstrates that increasing the perceptual field by using depthwise atrous convolutions with different dilation rates is effective in segmenting vascular pixels accurately. When we removed the GAE, the data in Tables 1–3 revealed that the absence of the GAE module has a small effect, as the effects were more pronounced for the accuracy and Dice coefficient, which decreased by 0.03 and 0.29%, 0.04 and 0.29% and 0.05 and 0.61%, respectively. Results emphasize the importance of the GAE module in the MAG-Net. The effectiveness of the MFF module is also well demonstrated by the fact that the three metrics, i.e., sensitivity, accuracy and the Dice coefficient, decreased by 1.15, 0.07 and 0.42% on the DRIVE dataset and 1.09, 0.06 and 0.60% on the CHASE dataset without the MFF.
In Figure 7, we show the segmentation results of various models in the ablation experiments on three datasets, aiming to visually validate the effectiveness of our module in segmenting retinal vessel details. The figure showcases six columns, (a) to (f), representing the preprocessed fundus images, ground truth annotations and the segmentation results obtained by using "w/o HCF", "w/o GAE", "w/o MFF" and the MAG-Net, respectively. The results on the DRIVE, CHASE and STARE datasets are shown from top to bottom in the figure above. Upon examination of the images, it is evident that all four models possess the ability to accurately segment the main trunk of the vessel and finer vessels across different datasets. Using capillary segmentation as a significant metric, the MAG-Net demonstrates its exceptional performance. We have highlighted the segmentation of some capillaries by marking and enlarging the same areas of each image with red boxes.
The GAE module is a very important part of the MAG-Net, and to validate the reliability of our proposed grouped attention, we conducted a series of ablation experiments for the GAE module. We selected the widely used SE module [36], CBAM [37] module and GC block [38] to substitute the attention computation method in the GAE module and conducted experiments on the DRIVE dataset. Table 4 shows the results of our experiments.
Model | AUC_ROC | Sp | Se | Acc | Dice |
MAG-Net+SE | 0.9892 | 0.9837 | 0.8517 | 0.9702 | 0.8542 |
MAG-Net+CBAM | 0.9891 | 0.9850 | 0.844 | 0.9706 | 0.8547 |
MAG-Net+GC | 0.9894 | 0.9849 | 0.8471 | 0.9708 | 0.8559 |
ours | 0.9895 | 0.9836 | 0.8588 | 0.9708 | 0.8576 |
Although the addition of the SE, CBAM and GC block improves the model's performance to a certain extent, these three methods are slightly inferior compared to the grouped attention approach. This is because the GAE module not only learns the channel weights of features at different scales, it also retains the details of low-level features and high-level features to the greatest extent possible. At the same time, the GAE module is simple in structure and achieves efficient segmentation with only a small amount of computation.
Figure 8 presents a visual comparison of our model, MAG-Net, with other competing models. We selected U-Net [12], SA-UNet [21] and Attention-UNet [39] as the competition, and we applied a test image from both the DRIVE and CHASE datasets for visual analysis, arranged in left-to-right order. Columns (a)–(f) display the preprocessed original image, the groundtruth image, U-Net segmented results, Attention-UNet segmented results, SA-UNet segmented results and MAG-Net segmented results, respectively. Under each image, we included an enlarged local area to promote the comparison of the results.
By comparing the detailed regions, it can be seen that U-Net, Attention U-Net, SA-UNet and MAG-Net already have the ability to extract the vascular backbone from the original image and achieves satisfactory segmentation for most vascular regions. However, when comparing the groudtruth images, disconnections and mis-segmentations in the capillary region are easy to observe. Comparing the details, it can be seen that the MAG-Net does well at denoising, minimizes mis-segmentation and has excellent vessel connectivity.
To further demonstrate the superiority of our method, we compared the MAG-Net with some other deep learning-based retinal vessel segmentation methods. We evaluated them by using four metrics: specificity, sensitivity, accuracy and the Dice coefficient, respectively. Tables 5–7 indicate their segmentation results on the DRIVE, CHASE, and STARE datasets, respectively. When compared to existing segmentation methods, our proposed method showed significant improvements for most metrics, especially accuracy, outperforming the other methods on all three datasets. As can be seen in Table 5, the MAG-Net exhibits competitive ability that is not exhibited by other methods, with the best accuracy and Dice coefficient, which improved by 0.04 and 2.73%, respectively. As shown in Table 6, on the CHASE dataset, the MAG-Net excelled in the cases of the specificity and accuracy metrics, achieving 98.75 and 97.73% respectively. As can be seen in Table 7, similar to the CHASE dataset, the MAG-Net performed best for specificity and accuracy metrics, with a respective improvement of 0.28 and 0.18%.
Model | Year | Sp | Se | Acc | Dice |
R2U-Net [40] | 2018 | 0.9813 | 0.7792 | 0.9556 | 0.8171 |
DUNet [23] | 2019 | 0.9800 | 0.7963 | 0.9566 | 0.8237 |
NFN+ [41] | 2020 | 0.9813 | 0.7996 | 0.9582 | 0.8295 |
SA-UNet [21] | 2020 | 0.9840 | 0.8212 | 0.9698 | 0.8263 |
MPS-Net [42] | 2021 | 0.9740 | 0.8361 | 0.9563 | 0.8278 |
SCS-Net [24] | 2021 | 0.9838 | 0.8289 | 0.9697 | 0.8189 |
AACA-MLA-D-UNet [43] | 2022 | 0.9805 | 0.8046 | 0.9581 | 0.8303 |
Bridge-Net [44] | 2022 | 0.9818 | 0.7853 | 0.9565 | 0.8203 |
CRAUNet [45] | 2022 | - | 0.7954 | 0.9586 | 0.8302 |
SDDC-Net [46] | 2023 | 0.9808 | 0.8603 | 0.9704 | 0.8289 |
ours | 2023 | 0.9836 | 0.8588 | 0.9708 | 0.8576 |
Model | Year | Sp | Se | Acc | Dice |
R2U-Net [40] | 2018 | 0.9862 | 0.8298 | 0.9712 | 0.8475 |
DUNet [23] | 2019 | 0.9752 | 0.8155 | 0.9610 | 0.7883 |
NFN+ [41] | 2020 | 0.9880 | 0.8003 | 0.9688 | 0.8369 |
SA-UNet [21] | 2020 | 0.9835 | 0.8573 | 0.9755 | 0.8153 |
SCS-Net [24] | 2021 | 0.9839 | 0.8365 | 0.9744 | - |
MPS-Net [42] | 2021 | 0.9795 | 0.8488 | 0.9668 | 0.8332 |
AACA-MLA-D-UNet [43] | 2022 | 0.9801 | 0.8402 | 0.9673 | 0.8246 |
Bridge-Net [44] | 2022 | 0.9840 | 0.8132 | 0.9667 | 0.8293 |
CRAUNet [45] | 2022 | - | 0.8259 | 0.9659 | 0.8156 |
SDDC-Net [46] | 2023 | 0.9789 | 0.8268 | 0.9669 | 0.7965 |
ours | 2023 | 0.9875 | 0.8123 | 0.9773 | 0.8069 |
Model | Year | Sp | Se | Acc | Dice |
R2U-Net [40] | 2018 | 0.9820 | 0.7756 | 0.9634 | 0.7928 |
DUNet [23] | 2019 | 0.9878 | 0.7595 | 0.9641 | 0.8143 |
NFN+ [41] | 2020 | 0.9863 | 0.7963 | 0.9672 | 0.8298 |
SCS-Net [24] | 2021 | 0.9839 | 0.8207 | 0.9736 | - |
MPS-Net [42] | 2021 | 0.9819 | 0.8566 | 0.9689 | 0.8491 |
AACA-MLA-D-UNet [43] | 2022 | 0.9870 | 0.7914 | 0.9665 | 0.8276 |
Bridge-Net [44] | 2022 | 0.9864 | 0.8002 | 0.9668 | 0.8289 |
SDDC-Net [46] | 2023 | 0.9784 | 0.7988 | 0.9642 | 0.7776 |
ours | 2023 | 0.9906 | 0.7781 | 0.9743 | 0.8228 |
Generalization ability is a very important performance evaluation metric for retinal vessel segmentation tasks. We evaluated the generalization ability of the model by performing cross-validation on different training and test sets. Table 8 gives a comparison of the generalization ability of the proposed method with three other methods, under the condition of the training being performed on the DRIVE dataset and testing performed on the STARE dataset, as well as the opposite experiment. Experiments show that our method has good overall performance and excellent performance on the Dice coefficient and sensitivity metrics, which shows that our method has excellent generalization ability in the task of vessel segmentation.
Database | Methods | Sp | Se | Acc | Dice |
STARE (trained on DRIVE) | MS-CANet [47] | - | - | 0.9673 | 0.7826 |
GDF-Net [48] | 0.9795 | 0.7089 | 0.9588 | - | |
AA-WGAN [31] | 0.9883 | 0.7839 | 0.9647 | - | |
ours | 0.9745 | 0.8471 | 0.9648 | 0.7855 | |
DRIVE (trained on STARE) | MS-CANet [47] | - | - | 0.9701 | 0.8035 |
GDF-Net [48] | 0.9902 | 0.7289 | 0.9593 | - | |
AA-WGAN [31] | 0.9928 | 0.8250 | 0.9635 | - | |
ours | 0.9828 | 0.8255 | 0.9690 | 0.8236 |
In addition, we present the Receiver operating characteristic (ROC) curves for the different models in the ablation experiment for the three datasets. As can be seen in Figure 9, the values of the area under the curve for all four models were close to 1, indicating the models' superior performance for retinal vessel segmentation. Of these, the MAG-Net had the largest area of the ROC curve among all four models for all three datasets, further emphasizing the superiority of the MAG-Net over the other models in the three ablation experiments.
Furthermore, we compared our work with other state of the art models in terms of the number of model parameters on the DRIVE dataset. The comparison results are shown in Table 9. It can be seen that our model demonstrated lower complexity than the other methods. In other words, our model performs well in terms of efficiency.
U-Net | ResUnet | R2U-Net | AACA-MLA-D-UNet | MRC-Net [49] | ours | |
# Param. | 4.32M | 32.61M | 39.09M | 2.03M | 0.9M | 0.6M |
This paper presents a new retinal vessel segmentation framework, the MAG-Net, which combines a multi-scale technique and an attention mechanism to realize improvements. The HCF module was introduced, and it utilizes spatial attention and multiple convolutions to expand the receptive field of the encoder, minimize noise and optimize the performance of the feature extractor at the source. The GAE module uses high-level features to guide low-level features on skip connections, optimizing segmentation details and alleviating the semantic gap between the encoder and decoder. The MFF module addresses the information loss problem during upsampling in the decoder by integrating features of multiple scales, which serves to supplement the detailed information. We validated our method on three datasets, i.e., the DRIVE, the CHASE and the STARE datasets. The experimental results show that our method achieves superior retinal vessel segmentation performance, as compared to U-Net, Attention-UNet and SA-UNet.
However, there are certain limitations of our work. First, the current implementation relies on annotated datasets, which can be expensive and restricts the availability of a larger number of annotated datasets. Second, our network was specifically designed for retinal vessel segmentation, while fundus images contain a wealth of information related to various fundus diseases. A single-target segmentation architecture is temporarily unable to meet the diagnostic needs of multiple fundus diseases.
Regarding future research, our research goals can be divided into two main points. First, we will focus on the improvement and optimization of the model to make it more adaptable to the specificity of fundus images and to increase its robustness for applicability to different pathological situations. Second, transfer learning and self-supervised learning are effective means to address data scarcity and labeling difficulties. In the absence of large-scale labeled data, we will consider exploring how to learn knowledge from other medical image tasks through transfer learning and use self-supervised learning to improve the performance of the model.
The authors declare they have not used Artificial Intelligence (AI) tools in the creation of this article.
Our work was supported in part by the National Natural Science Foundation of China (No. 61962054), the National Natural Science Foundation of China (No. 61163036), the Cultivation Plan of Major Scientific Research Projects of Northwest Normal University (No. NWNU-LKZD2021-06).
We declare that there is no conflict of interest.
[1] |
T. Xu, B. Wang, H. Liu, H. Wang, P. Yin, W. Dong, et al., Prevalence and causes of vision loss in China from 1990 to 2019: findings from the Global Burden of Disease Study 2019, Lancet Public Health, 5 (2020), e682–e691. https://doi.org/10.1016/S2468-2667(20)30254-1 doi: 10.1016/S2468-2667(20)30254-1
![]() |
[2] |
M. Mookiah, S. Hogg, T. J. MacGillivray, V. Prathiba, R. Pradeepa, V. Mohan, et al., A review of machine learning methods for retinal blood vessel segmentation and artery/vein classification, Med. Image Anal., 68 (2021), 101905. https://doi.org/10.1016/j.media.2020.101905 doi: 10.1016/j.media.2020.101905
![]() |
[3] |
C. Chen, J. H. Chuah, R. Ali, Y. Wang, Retinal vessel segmentation using deep learning: a review, IEEE Access, 9 (2021), 111985–112004. https://doi.org/10.1109/ACCESS.2021.310217 doi: 10.1109/ACCESS.2021.310217
![]() |
[4] |
C. L. Srinidhi, P. Aparna, J. Rajan, Recent advancements in retinal vessel segmentation, J. Med. Syst., 41 (2017), 1–22. https://doi.org/10.1007/s10916-017-0719-2 doi: 10.1007/s10916-017-0719-2
![]() |
[5] |
B. Zhang, L. Zhang, L. Zhang, F. Karray, Retinal vessel extraction by matched filter with first-order derivative of Gaussian, Comput. Biol. Med., 40 (2010), 438–445. https://doi.org/10.1016/j.compbiomed.2010.02.008 doi: 10.1016/j.compbiomed.2010.02.008
![]() |
[6] |
G. Hassan, N. El-Bendary, A. E. Hassanien, A. Fahmy, A. M. Shoeb, V. Snasel, Retinal blood vessel segmentation approach based on mathematical morphology, Procedia Comput. Sci., 65 (2015), 612–622. https://doi.org/10.1016/j.procs.2015.09.005 doi: 10.1016/j.procs.2015.09.005
![]() |
[7] |
F. Zana, J. C. Klein, Segmentation of vessel-like patterns using mathematical morphology and curvature evaluation, IEEE Trans. Image Process., 10 (2001), 1010–1019. https://doi.org/10.1109/83.931095 doi: 10.1109/83.931095
![]() |
[8] |
J. Zhao, J. Yang, D. Ai, H. Song, Y. Jiang, Y. Huang, et al., Automatic retinal vessel segmentation using multi-scale superpixel chain tracking, Digital Signal Process., 81 (2018), 26–42. https://doi.org/10.1016/j.dsp.2018.06.006 doi: 10.1016/j.dsp.2018.06.006
![]() |
[9] |
J. Yang, C. Lou, J. Fu, C. Feng, Vessel segmentation using multiscale vessel enhancement and a region based level set model, Comput. Med. Imaging Graphics, 85 (2020), 101783. https://doi.org/10.1016/j.compmedimag.2020.101783 doi: 10.1016/j.compmedimag.2020.101783
![]() |
[10] | J. Long, E. Shelhamer, T. Darrell, Fully convolutional networks for semantic segmentation, in Proceedings of the IEEE conference on computer vision and pattern recognition, (2015), 3431–3440. https://doi.org/10.1109/cvpr.2015.7298965 |
[11] | H. Fu, Y. Xu, S. Lin, D. W. Wong, J. Liu, Deepvessel: Retinal vessel segmentation via deep learning and conditional random fiel, in Medical Image Computing and Computer-Assisted Intervention–MICCAI 2016: 19th International Conference, (2016), 132–139. https://doi.org/10.1007/978-3-319-46723-8_16 |
[12] | O. Ronneberger, P. Fischer, T. Brox, U-net: Convolutional networks for biomedical image segmentation, in Medical Image Computing and Computer-Assisted Intervention–MICCAI 2015: 18th International Conference, (2015), 234–241. https://doi.org/10.1007/978-3-319-24574-4_28 |
[13] | M. Entov, L. Polterovich, F. Zapolsky, Transunet: Transformers make strong encoders for medical image segmentation, preprint, arXiv: 2102.04306. |
[14] | H. Cao, Y. Wang, J. Chen, D. Jiang, X. Zhang, Q. Tian, et al., Swin-unet: Unet-like pure transformer for medical image segmentation, in European Conference on Computer Vision, (2022), 205–218. https://doi.org/10.1007/978-3-031-25066-8_9 |
[15] | S. Roy, G. Koehler, C. Ulrich, M. Baumgartner, J. Petersen, F. Isensee, et al., MedNeXt: Transformer-driven scaling of convNets for medical image segmentation, preprint, arXiv: 2303.09975. |
[16] | A. Tragakis, C. Kaul, R. Murray-Smith, D. Husmeier, The fully convolutional transformer for medical image segmentation, in Proceedings of the IEEE/CVF Winter Conference on Applications of Computer Vision, (2023), 3660–3669. https://doi.org/10.1109/wacv56688.2023.00365 |
[17] |
Y. Jiang, H. Zhang, N. Tan, L. Chen, Automatic retinal blood vessel segmentation based on fully convolutional neural networks, Symmetry, 11 (2019), 1112. https://doi.org/10.3390/sym11091112 doi: 10.3390/sym11091112
![]() |
[18] | A. Zhao, Image denoising with deep convolutional neural networks, Comput. Sci., 2016 (2016), 1–5. |
[19] |
M. Z. Alom, C. Yakopcic, M. Hasan, T. M. Taha, V. K. Asari, Recurrent residual U-Net for medical image segmentation, J. Med. Imaging, 6 (2019), 014006. https://doi.org/10.1117/1.JMI.6.1.014006 doi: 10.1117/1.JMI.6.1.014006
![]() |
[20] | M. Zhang, F. Yu, J. Zhao, L. Zhang, Q. Li, BEFD: Boundary enhancement and feature denoising for vessel segmentation, in Medical Image Computing and Computer Assisted Intervention–MICCAI 2020: 23rd International Conference, (2020), 775–785. https://doi.org/10.1007/978-3-030-59722-1_75 |
[21] | C. Guo, M. Szemenyei, Y. Yi, W. Wang, B. Chen, C. Fan, Sa-unet: Spatial attention u-net for retinal vessel segmentation, in 2020 25th international conference on pattern recognition (ICPR), (2021), 1236–1242. https://doi.org/10.1109/ICPR48806.2021.9413346 |
[22] | W. Luo, Y. Li, R. Urtasun, R. Zemel, Understanding the effective receptive field in deep convolutional neural networks, Adv. Neural Inform. Process. Syst., 29 (2016). |
[23] |
Q. Jin, Z. Meng, T. D. Pham, Q. Chen, L. Wei, DUNet: A deformable network for retinal vessel segmentation, Knowl. Based Syst., 178 (2019), 149–162. https://doi.org/10.1016/j.knosys.2019.04.025 doi: 10.1016/j.knosys.2019.04.025
![]() |
[24] |
H. Wu, W. Wang, J. Zhong, B. Lei, Z. Wen, J. Qin, Scs-net: A scale and context sensitive network for retinal vessel segmentation, Med. Image Anal., 70 (2021), 102025. https://doi.org/10.1016/j.media.2021.102025 doi: 10.1016/j.media.2021.102025
![]() |
[25] |
Z. Zhang, Y. Jiang, H. Qiao, M. Wang, W. Yan, SIL-Net: A Semi-Isotropic L-shaped network for dermoscopic image segmentation, Comput. Biol. Med., 150 (2022), 106146. https://doi.org/10.1016/j.compbiomed.2022.106146 doi: 10.1016/j.compbiomed.2022.106146
![]() |
[26] |
Y. Liu, J. Shen, L. Yang, G. Bian, H. Yu, ResDO-UNet: A deep residual network for accurate retinal vessel segmentation from fundus images, Biomed. Signal Process. Control, 79 (2023), 104087. https://doi.org/10.1016/j.bspc.2022.104087 doi: 10.1016/j.bspc.2022.104087
![]() |
[27] |
J. Cao, Y. Li, M. Sun, Y. Chen, D. Lischinski, D. Cohen-Or, et al., Do-conv: Depthwise over-parameterized convolutional layer, IEEE Trans. Image Process., 31 (2022), 3726–3736. https://doi.org/10.1109/TIP.2022.3175432 doi: 10.1109/TIP.2022.3175432
![]() |
[28] |
W. Zhou, W. Bai, J. Ji, Y. Yi, N. Zhang, W. Cui, Dual-path multi-scale context dense aggregation network for retinal vessel segmentation, Comput. Biol. Med., 164 (2023), 107269. https://doi.org/10.1016/j.compbiomed.2023.107269 doi: 10.1016/j.compbiomed.2023.107269
![]() |
[29] |
N. K. Tomar, D. Jha, M. A. Riegler, Fanet: A feedback attention network for improved biomedical image segmentation, IEEE Trans. Neural Networks Learn. Syst., 2022 (2022). https://doi.org/10.1109/TNNLS.2022.3159394 doi: 10.1109/TNNLS.2022.3159394
![]() |
[30] |
D. E. Alvarado-Carrillo, O. S. Dalmau-Cedeño, Width attention based convolutional neural network for retinal vessel segmentation, Expert Syst. Appl., 209 (2022), 118313. https://doi.org/10.1016/j.eswa.2022.118313 doi: 10.1016/j.eswa.2022.118313
![]() |
[31] |
M. Liu, Z. Wang, H. Li, P. Wu, F. E. Alsaadi, AA-WGAN: Attention augmented Wasserstein generative adversarial network with application to fundus retinal vessel segmentation, Comput. Biol. Med., 158 (2023), 106874. https://doi.org/10.1016/j.compbiomed.2023.106874 doi: 10.1016/j.compbiomed.2023.106874
![]() |
[32] |
M. R. Ahmed, M. Fahim, A. Islam, S. Islam, S. Shatabda, DOLG-NeXt: Convolutional neural network with deep orthogonal fusion of local and global features for biomedical image segmentation, Neurocomputing, 546 (2023), 126362. https://doi.org/10.1016/j.neucom.2023.126362 doi: 10.1016/j.neucom.2023.126362
![]() |
[33] |
J. Staal, M. D. Abràmoff, M. Niemeijer, M. A. Viergever, B. Van Ginneken, Ridge-based vessel segmentation in color images of the retina, IEEE Trans. Med. Imaging, 23 (20004), 501–509. https://doi.org/10.1109/TMI.2004.825627 doi: 10.1109/TMI.2004.825627
![]() |
[34] |
C. G. Owen, A. R. Rudnicka, R. Mullen, S. A. Barman, D. Monekosso, P. H. Whincup, et al., Measuring retinal vessel tortuosity in 10-year-old children: validation of the computer-assisted image analysis of the retina (CAIAR) program, Invest. Ophthalmol. Visual Sci., 50 (2009), 004–2010. https://doi.org/10.1167/iovs.08-3018 doi: 10.1167/iovs.08-3018
![]() |
[35] |
A. D. Hoover, V. Kouznetsova, M. Goldbaum, Locating blood vessels in retinal images by piecewise threshold probing of a matched filter response, IEEE Trans. Med. imaging, 19 (2000), 203–210. https://doi.org/10.1109/42.845178 doi: 10.1109/42.845178
![]() |
[36] | J. Hu, L. Shen, G. Sun, Squeeze-and-excitation networks, in Proceedings of the IEEE conference on computer vision and pattern recognition, (2018), 7132–7141. https://doi.org/10.1109/cvpr.2018.00745 |
[37] | S. Woo, J. Park, J. Y. Lee, I. S. Kweon, Cbam: Convolutional block attention module, in Proceedings of the European conference on computer vision (ECCV), (2018), 3–19. https://doi.org/10.1007/978-3-030-01234-2_1 |
[38] | Y. Cao, J. Xu, S. Lin, F. Wei, H. Hu, Gcnet: Non-local networks meet squeeze-excitation networks and beyond, in Proceedings of the IEEE/CVF international conference on computer vision workshops, 2019. https://doi.org/10.1109/iccvw.2019.00246 |
[39] | O. Oktay, J. Schlemper, L. L. Folgoc, M. Lee, M. Heinrich, K. Misawa, et al., Attention u-net: Learning where to look for the pancreas, preprint, arXiv: 1804.03999. |
[40] | M. Z. Alom, M. Hasan, C. Yakopcic, T. M. Taha, V. K. Asari, Recurrent residual convolutional neural network based on u-net (r2u-net) for medical image segmentation, preprint, arXiv: 1802.06955. |
[41] |
Y. Wu, Y. Xia, Y. Song, Y. Zhang, W. Cai, NFN+: A novel network followed network for retinal vessel segmentation, Neural Networks, 126 (2020), 153–162. https://doi.org/10.1016/j.neunet.2020.02.018 doi: 10.1016/j.neunet.2020.02.018
![]() |
[42] |
Z. Lin, J. Huang, Y. Chen, X. Zhang, W. Zhao, Y. Li, A high resolution representation network with multi-path scale for retinal vessel segmentation, Comput. Methods Programs Biomed., 208 (2021), 106206. https://doi.org/10.1016/j.cmpb.2021.106206 doi: 10.1016/j.cmpb.2021.106206
![]() |
[43] |
Y. Yuan, L. Zhang, L. Wang, H. Huang, Multi-level attention network for retinal vessel segmentation, IEEE J. Biomed. Health Inform., 26 (2021), 312–323. https://doi.org/10.1109/JBHI.2021.3089201 doi: 10.1109/JBHI.2021.3089201
![]() |
[44] |
Y. Zhang, M. He, Z. Chen, K. Hu, X. Li, X. Gao, Bridge-Net: Context-involved U-net with patch-based loss weight mapping for retinal blood vessel segmentation, Expert Syst. Appl., 195 (2022), 116526. https://doi.org/10.1016/j.eswa.2022.116526 doi: 10.1016/j.eswa.2022.116526
![]() |
[45] |
F. Dong, D. Wu, C. Guo, S. Zhang, B. Yang, X. Gong, CRAUNet: A cascaded residual attention U-Net for retinal vessel segmentation, Comput. Biol. Med., 147 (2022), 105651. https://doi.org/10.1016/j.compbiomed.2022.105651 doi: 10.1016/j.compbiomed.2022.105651
![]() |
[46] |
B. Yang, L. Qin, H. Peng, C. Guo, X. Luo, J. Wang, SDDC-Net: A U-shaped deep spiking neural P convolutional network for retinal vessel segmentation, Digital Signal Process., 136 (2023), 104002. https://doi.org/10.1016/j.dsp.2023.104002 doi: 10.1016/j.dsp.2023.104002
![]() |
[47] |
Y. Jiang, W. Yan, J. Chen, H. Qiao, Z. Zhang, M. Wang, MS-CANet: Multi-Scale subtraction network with coordinate attention for retinal vessel segmentation, Symmetry, 15 (2023), 835. https://doi.org/10.3390/sym15040835 doi: 10.3390/sym15040835
![]() |
[48] |
J. Li, G. Gao, L. Yang, Y. Liu, GDF-Net: A multi-task symmetrical network for retinal vessel segmentation, Biomed. Signal Process. Control, 81 (2023), 104426. https://doi.org/10.1016/j.bspc.2022.104426 doi: 10.1016/j.bspc.2022.104426
![]() |
[49] |
T. M. Khan, S. S. Naqvi, A. Robles-Kelly, I. Razzak, Retinal vessel segmentation via a Multi-resolution Contextual Network and adversarial learning, Neural Networks, 2023 (2023). https://doi.org/10.1016/j.neunet.2023.05.029 doi: 10.1016/j.neunet.2023.05.029
![]() |
Model | AUC_ROC | Sp | Se | Acc | Dice |
baseline | 0.9891 | 0.9856 | 0.8375 | 0.9704 | 0.8529 |
w/o HCF | 0.989 | 0.9846 | 0.8474 | 0.9705 | 0.8549 |
w/o GAE | 0.9892 | 0.9837 | 0.8544 | 0.9705 | 0.8556 |
w/o MFF | 0.9891 | 0.9842 | 0.8473 | 0.9701 | 0.8532 |
ours | 0.9895 | 0.9836 | 0.8588 | 0.9708 | 0.8576 |
Model | AUC_ROC | Sp | Se | Acc | Dice |
baseline | 0.9867 | 0.9883 | 0.7876 | 0.9765 | 0.7970 |
w/o HCF | 0.9878 | 0.9885 | 0.7957 | 0.9772 | 0.8031 |
w/o GAE | 0.9878 | 0.9872 | 0.8104 | 0.9769 | 0.8040 |
w/o MFF | 0.9880 | 0.9876 | 0.8014 | 0.9767 | 0.8009 |
ours | 0.9884 | 0.9875 | 0.8123 | 0.9773 | 0.8069 |
Model | AUC_ROC | Pr | Sp | Se | Acc | Dice |
baseline | 0.9886 | 0.9166 | 0.9932 | 0.7461 | 0.9742 | 0.8161 |
w/o HCF | 0.9895 | 0.9195 | 0.9929 | 0.7578 | 0.9748 | 0.8219 |
w/o GAE | 0.9890 | 0.9138 | 0.9915 | 0.7604 | 0.9738 | 0.8167 |
w/o MFF | 0.9882 | 0.9141 | 0.9915 | 0.7676 | 0.9743 | 0.8208 |
ours | 0.9895 | 0.9158 | 0.9906 | 0.7781 | 0.9743 | 0.8228 |
Model | AUC_ROC | Sp | Se | Acc | Dice |
MAG-Net+SE | 0.9892 | 0.9837 | 0.8517 | 0.9702 | 0.8542 |
MAG-Net+CBAM | 0.9891 | 0.9850 | 0.844 | 0.9706 | 0.8547 |
MAG-Net+GC | 0.9894 | 0.9849 | 0.8471 | 0.9708 | 0.8559 |
ours | 0.9895 | 0.9836 | 0.8588 | 0.9708 | 0.8576 |
Model | Year | Sp | Se | Acc | Dice |
R2U-Net [40] | 2018 | 0.9813 | 0.7792 | 0.9556 | 0.8171 |
DUNet [23] | 2019 | 0.9800 | 0.7963 | 0.9566 | 0.8237 |
NFN+ [41] | 2020 | 0.9813 | 0.7996 | 0.9582 | 0.8295 |
SA-UNet [21] | 2020 | 0.9840 | 0.8212 | 0.9698 | 0.8263 |
MPS-Net [42] | 2021 | 0.9740 | 0.8361 | 0.9563 | 0.8278 |
SCS-Net [24] | 2021 | 0.9838 | 0.8289 | 0.9697 | 0.8189 |
AACA-MLA-D-UNet [43] | 2022 | 0.9805 | 0.8046 | 0.9581 | 0.8303 |
Bridge-Net [44] | 2022 | 0.9818 | 0.7853 | 0.9565 | 0.8203 |
CRAUNet [45] | 2022 | - | 0.7954 | 0.9586 | 0.8302 |
SDDC-Net [46] | 2023 | 0.9808 | 0.8603 | 0.9704 | 0.8289 |
ours | 2023 | 0.9836 | 0.8588 | 0.9708 | 0.8576 |
Model | Year | Sp | Se | Acc | Dice |
R2U-Net [40] | 2018 | 0.9862 | 0.8298 | 0.9712 | 0.8475 |
DUNet [23] | 2019 | 0.9752 | 0.8155 | 0.9610 | 0.7883 |
NFN+ [41] | 2020 | 0.9880 | 0.8003 | 0.9688 | 0.8369 |
SA-UNet [21] | 2020 | 0.9835 | 0.8573 | 0.9755 | 0.8153 |
SCS-Net [24] | 2021 | 0.9839 | 0.8365 | 0.9744 | - |
MPS-Net [42] | 2021 | 0.9795 | 0.8488 | 0.9668 | 0.8332 |
AACA-MLA-D-UNet [43] | 2022 | 0.9801 | 0.8402 | 0.9673 | 0.8246 |
Bridge-Net [44] | 2022 | 0.9840 | 0.8132 | 0.9667 | 0.8293 |
CRAUNet [45] | 2022 | - | 0.8259 | 0.9659 | 0.8156 |
SDDC-Net [46] | 2023 | 0.9789 | 0.8268 | 0.9669 | 0.7965 |
ours | 2023 | 0.9875 | 0.8123 | 0.9773 | 0.8069 |
Model | Year | Sp | Se | Acc | Dice |
R2U-Net [40] | 2018 | 0.9820 | 0.7756 | 0.9634 | 0.7928 |
DUNet [23] | 2019 | 0.9878 | 0.7595 | 0.9641 | 0.8143 |
NFN+ [41] | 2020 | 0.9863 | 0.7963 | 0.9672 | 0.8298 |
SCS-Net [24] | 2021 | 0.9839 | 0.8207 | 0.9736 | - |
MPS-Net [42] | 2021 | 0.9819 | 0.8566 | 0.9689 | 0.8491 |
AACA-MLA-D-UNet [43] | 2022 | 0.9870 | 0.7914 | 0.9665 | 0.8276 |
Bridge-Net [44] | 2022 | 0.9864 | 0.8002 | 0.9668 | 0.8289 |
SDDC-Net [46] | 2023 | 0.9784 | 0.7988 | 0.9642 | 0.7776 |
ours | 2023 | 0.9906 | 0.7781 | 0.9743 | 0.8228 |
Database | Methods | Sp | Se | Acc | Dice |
STARE (trained on DRIVE) | MS-CANet [47] | - | - | 0.9673 | 0.7826 |
GDF-Net [48] | 0.9795 | 0.7089 | 0.9588 | - | |
AA-WGAN [31] | 0.9883 | 0.7839 | 0.9647 | - | |
ours | 0.9745 | 0.8471 | 0.9648 | 0.7855 | |
DRIVE (trained on STARE) | MS-CANet [47] | - | - | 0.9701 | 0.8035 |
GDF-Net [48] | 0.9902 | 0.7289 | 0.9593 | - | |
AA-WGAN [31] | 0.9928 | 0.8250 | 0.9635 | - | |
ours | 0.9828 | 0.8255 | 0.9690 | 0.8236 |
U-Net | ResUnet | R2U-Net | AACA-MLA-D-UNet | MRC-Net [49] | ours | |
# Param. | 4.32M | 32.61M | 39.09M | 2.03M | 0.9M | 0.6M |
Model | AUC_ROC | Sp | Se | Acc | Dice |
baseline | 0.9891 | 0.9856 | 0.8375 | 0.9704 | 0.8529 |
w/o HCF | 0.989 | 0.9846 | 0.8474 | 0.9705 | 0.8549 |
w/o GAE | 0.9892 | 0.9837 | 0.8544 | 0.9705 | 0.8556 |
w/o MFF | 0.9891 | 0.9842 | 0.8473 | 0.9701 | 0.8532 |
ours | 0.9895 | 0.9836 | 0.8588 | 0.9708 | 0.8576 |
Model | AUC_ROC | Sp | Se | Acc | Dice |
baseline | 0.9867 | 0.9883 | 0.7876 | 0.9765 | 0.7970 |
w/o HCF | 0.9878 | 0.9885 | 0.7957 | 0.9772 | 0.8031 |
w/o GAE | 0.9878 | 0.9872 | 0.8104 | 0.9769 | 0.8040 |
w/o MFF | 0.9880 | 0.9876 | 0.8014 | 0.9767 | 0.8009 |
ours | 0.9884 | 0.9875 | 0.8123 | 0.9773 | 0.8069 |
Model | AUC_ROC | Pr | Sp | Se | Acc | Dice |
baseline | 0.9886 | 0.9166 | 0.9932 | 0.7461 | 0.9742 | 0.8161 |
w/o HCF | 0.9895 | 0.9195 | 0.9929 | 0.7578 | 0.9748 | 0.8219 |
w/o GAE | 0.9890 | 0.9138 | 0.9915 | 0.7604 | 0.9738 | 0.8167 |
w/o MFF | 0.9882 | 0.9141 | 0.9915 | 0.7676 | 0.9743 | 0.8208 |
ours | 0.9895 | 0.9158 | 0.9906 | 0.7781 | 0.9743 | 0.8228 |
Model | AUC_ROC | Sp | Se | Acc | Dice |
MAG-Net+SE | 0.9892 | 0.9837 | 0.8517 | 0.9702 | 0.8542 |
MAG-Net+CBAM | 0.9891 | 0.9850 | 0.844 | 0.9706 | 0.8547 |
MAG-Net+GC | 0.9894 | 0.9849 | 0.8471 | 0.9708 | 0.8559 |
ours | 0.9895 | 0.9836 | 0.8588 | 0.9708 | 0.8576 |
Model | Year | Sp | Se | Acc | Dice |
R2U-Net [40] | 2018 | 0.9813 | 0.7792 | 0.9556 | 0.8171 |
DUNet [23] | 2019 | 0.9800 | 0.7963 | 0.9566 | 0.8237 |
NFN+ [41] | 2020 | 0.9813 | 0.7996 | 0.9582 | 0.8295 |
SA-UNet [21] | 2020 | 0.9840 | 0.8212 | 0.9698 | 0.8263 |
MPS-Net [42] | 2021 | 0.9740 | 0.8361 | 0.9563 | 0.8278 |
SCS-Net [24] | 2021 | 0.9838 | 0.8289 | 0.9697 | 0.8189 |
AACA-MLA-D-UNet [43] | 2022 | 0.9805 | 0.8046 | 0.9581 | 0.8303 |
Bridge-Net [44] | 2022 | 0.9818 | 0.7853 | 0.9565 | 0.8203 |
CRAUNet [45] | 2022 | - | 0.7954 | 0.9586 | 0.8302 |
SDDC-Net [46] | 2023 | 0.9808 | 0.8603 | 0.9704 | 0.8289 |
ours | 2023 | 0.9836 | 0.8588 | 0.9708 | 0.8576 |
Model | Year | Sp | Se | Acc | Dice |
R2U-Net [40] | 2018 | 0.9862 | 0.8298 | 0.9712 | 0.8475 |
DUNet [23] | 2019 | 0.9752 | 0.8155 | 0.9610 | 0.7883 |
NFN+ [41] | 2020 | 0.9880 | 0.8003 | 0.9688 | 0.8369 |
SA-UNet [21] | 2020 | 0.9835 | 0.8573 | 0.9755 | 0.8153 |
SCS-Net [24] | 2021 | 0.9839 | 0.8365 | 0.9744 | - |
MPS-Net [42] | 2021 | 0.9795 | 0.8488 | 0.9668 | 0.8332 |
AACA-MLA-D-UNet [43] | 2022 | 0.9801 | 0.8402 | 0.9673 | 0.8246 |
Bridge-Net [44] | 2022 | 0.9840 | 0.8132 | 0.9667 | 0.8293 |
CRAUNet [45] | 2022 | - | 0.8259 | 0.9659 | 0.8156 |
SDDC-Net [46] | 2023 | 0.9789 | 0.8268 | 0.9669 | 0.7965 |
ours | 2023 | 0.9875 | 0.8123 | 0.9773 | 0.8069 |
Model | Year | Sp | Se | Acc | Dice |
R2U-Net [40] | 2018 | 0.9820 | 0.7756 | 0.9634 | 0.7928 |
DUNet [23] | 2019 | 0.9878 | 0.7595 | 0.9641 | 0.8143 |
NFN+ [41] | 2020 | 0.9863 | 0.7963 | 0.9672 | 0.8298 |
SCS-Net [24] | 2021 | 0.9839 | 0.8207 | 0.9736 | - |
MPS-Net [42] | 2021 | 0.9819 | 0.8566 | 0.9689 | 0.8491 |
AACA-MLA-D-UNet [43] | 2022 | 0.9870 | 0.7914 | 0.9665 | 0.8276 |
Bridge-Net [44] | 2022 | 0.9864 | 0.8002 | 0.9668 | 0.8289 |
SDDC-Net [46] | 2023 | 0.9784 | 0.7988 | 0.9642 | 0.7776 |
ours | 2023 | 0.9906 | 0.7781 | 0.9743 | 0.8228 |
Database | Methods | Sp | Se | Acc | Dice |
STARE (trained on DRIVE) | MS-CANet [47] | - | - | 0.9673 | 0.7826 |
GDF-Net [48] | 0.9795 | 0.7089 | 0.9588 | - | |
AA-WGAN [31] | 0.9883 | 0.7839 | 0.9647 | - | |
ours | 0.9745 | 0.8471 | 0.9648 | 0.7855 | |
DRIVE (trained on STARE) | MS-CANet [47] | - | - | 0.9701 | 0.8035 |
GDF-Net [48] | 0.9902 | 0.7289 | 0.9593 | - | |
AA-WGAN [31] | 0.9928 | 0.8250 | 0.9635 | - | |
ours | 0.9828 | 0.8255 | 0.9690 | 0.8236 |
U-Net | ResUnet | R2U-Net | AACA-MLA-D-UNet | MRC-Net [49] | ours | |
# Param. | 4.32M | 32.61M | 39.09M | 2.03M | 0.9M | 0.6M |