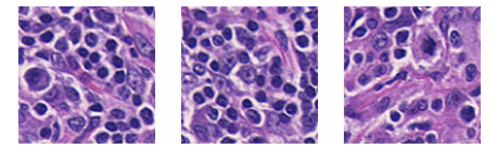
Automatic segmentation of tumor-infiltrating lymphocytes (TILs) from pathological images is essential for the prognosis and treatment of cancer. Deep learning technology has achieved great success in the segmentation task. It is still a challenge to realize accurate segmentation of TILs due to the phenomenon of blurred edges and adhesion of cells. To alleviate these problems, a squeeze-and-attention and multi-scale feature fusion network (SAMS-Net) based on codec structure, namely SAMS-Net, is proposed for the segmentation of TILs. Specifically, SAMS-Net utilizes the squeeze-and-attention module with the residual structure to fuse local and global context features and boost the spatial relevance of TILs images. Besides, a multi-scale feature fusion module is designed to capture TILs with large size differences by combining context information. The residual structure module integrates feature maps from different resolutions to strengthen the spatial resolution and offset the loss of spatial details. SAMS-Net is evaluated on the public TILs dataset and achieved dice similarity coefficient (DSC) of 87.2% and Intersection of Union (IoU) of 77.5%, which improved by 2.5% and 3.8% compared with UNet. These results demonstrate the great potential of SAMS-Net in TILs analysis and can further provide important evidence for the prognosis and treatment of cancer.
Citation: Xiaoli Zhang, Kunmeng Liu, Kuixing Zhang, Xiang Li, Zhaocai Sun, Benzheng Wei. SAMS-Net: Fusion of attention mechanism and multi-scale features network for tumor infiltrating lymphocytes segmentation[J]. Mathematical Biosciences and Engineering, 2023, 20(2): 2964-2979. doi: 10.3934/mbe.2023140
[1] | Yun Jiang, Jie Chen, Wei Yan, Zequn Zhang, Hao Qiao, Meiqi Wang . MAG-Net : Multi-fusion network with grouped attention for retinal vessel segmentation. Mathematical Biosciences and Engineering, 2024, 21(2): 1938-1958. doi: 10.3934/mbe.2024086 |
[2] | Yuqing Zhang, Yutong Han, Jianxin Zhang . MAU-Net: Mixed attention U-Net for MRI brain tumor segmentation. Mathematical Biosciences and Engineering, 2023, 20(12): 20510-20527. doi: 10.3934/mbe.2023907 |
[3] | Rongrong Bi, Chunlei Ji, Zhipeng Yang, Meixia Qiao, Peiqing Lv, Haiying Wang . Residual based attention-Unet combing DAC and RMP modules for automatic liver tumor segmentation in CT. Mathematical Biosciences and Engineering, 2022, 19(5): 4703-4718. doi: 10.3934/mbe.2022219 |
[4] | Tong Shan, Jiayong Yan, Xiaoyao Cui, Lijian Xie . DSCA-Net: A depthwise separable convolutional neural network with attention mechanism for medical image segmentation. Mathematical Biosciences and Engineering, 2023, 20(1): 365-382. doi: 10.3934/mbe.2023017 |
[5] | Dongwei Liu, Ning Sheng, Tao He, Wei Wang, Jianxia Zhang, Jianxin Zhang . SGEResU-Net for brain tumor segmentation. Mathematical Biosciences and Engineering, 2022, 19(6): 5576-5590. doi: 10.3934/mbe.2022261 |
[6] | Jun Liu, Zhenhua Yan, Chaochao Zhou, Liren Shao, Yuanyuan Han, Yusheng Song . mfeeU-Net: A multi-scale feature extraction and enhancement U-Net for automatic liver segmentation from CT Images. Mathematical Biosciences and Engineering, 2023, 20(5): 7784-7801. doi: 10.3934/mbe.2023336 |
[7] | Wencong Zhang, Yuxi Tao, Zhanyao Huang, Yue Li, Yingjia Chen, Tengfei Song, Xiangyuan Ma, Yaqin Zhang . Multi-phase features interaction transformer network for liver tumor segmentation and microvascular invasion assessment in contrast-enhanced CT. Mathematical Biosciences and Engineering, 2024, 21(4): 5735-5761. doi: 10.3934/mbe.2024253 |
[8] | Jiajun Zhu, Rui Zhang, Haifei Zhang . An MRI brain tumor segmentation method based on improved U-Net. Mathematical Biosciences and Engineering, 2024, 21(1): 778-791. doi: 10.3934/mbe.2024033 |
[9] | Qian Wu, Yuyao Pei, Zihao Cheng, Xiaopeng Hu, Changqing Wang . SDS-Net: A lightweight 3D convolutional neural network with multi-branch attention for multimodal brain tumor accurate segmentation. Mathematical Biosciences and Engineering, 2023, 20(9): 17384-17406. doi: 10.3934/mbe.2023773 |
[10] | Zhuang Zhang, Wenjie Luo . Hierarchical volumetric transformer with comprehensive attention for medical image segmentation. Mathematical Biosciences and Engineering, 2023, 20(2): 3177-3190. doi: 10.3934/mbe.2023149 |
Automatic segmentation of tumor-infiltrating lymphocytes (TILs) from pathological images is essential for the prognosis and treatment of cancer. Deep learning technology has achieved great success in the segmentation task. It is still a challenge to realize accurate segmentation of TILs due to the phenomenon of blurred edges and adhesion of cells. To alleviate these problems, a squeeze-and-attention and multi-scale feature fusion network (SAMS-Net) based on codec structure, namely SAMS-Net, is proposed for the segmentation of TILs. Specifically, SAMS-Net utilizes the squeeze-and-attention module with the residual structure to fuse local and global context features and boost the spatial relevance of TILs images. Besides, a multi-scale feature fusion module is designed to capture TILs with large size differences by combining context information. The residual structure module integrates feature maps from different resolutions to strengthen the spatial resolution and offset the loss of spatial details. SAMS-Net is evaluated on the public TILs dataset and achieved dice similarity coefficient (DSC) of 87.2% and Intersection of Union (IoU) of 77.5%, which improved by 2.5% and 3.8% compared with UNet. These results demonstrate the great potential of SAMS-Net in TILs analysis and can further provide important evidence for the prognosis and treatment of cancer.
TILs are the types of immune cells, which exist in tumor tissues and are of great significance for the diagnosis and prognosis of cancer [1]. As the gold standard for cancer diagnosis, pathological images contain a lot of information [2]. TILs can be observed in pathological images, and their role is particularly important as the main immune cells in the tumor microenvironment [3,4]. Now many studies have shown that the number and spatial characteristics of TILs on pathological images can be used as predictors of breast cancer prognosis [5,6]. Part of the pathological images of TILs are shown in Figure 1.
Pathological image analysis relies on professional doctors, which is time-consuming and laborious, meanwhile, the specificity of pathological images will also affect the reliability of doctors' diagnosis [7]. Deep learning technology has attracted extensive attention in the medical field because of its autonomy and intelligence [8]. It has been gradually applied to many fields, such as medical image classification [9,10], detection [11,12] and segmentation [13,14], etc. Using deep learning methods to segment TILs in pathological images, and quantify the number and characteristics of TILs has become one of the hotspots of current research. However, due to the specificity of pathological images and cells, there are three challenges in the segmentation tasks of TILs: 1) The problem of cell adhesion and overlap. During the sampling process, many cells tend to cluster together because of cell movement; 2) The coexistence of multiple types of cells. There are many kinds of cells in a pathological image, it is difficult to segment a kind of cells accurately; 3) The problem of the large difference between the front and background. Compared with the background area, the cells occupy a small area and are not easy to capture in the segmentation process.
Considering the above challenges, we take advantage of deep learning technology to design a segmentation network, which is called as SAMS-Net. The proposed network model has three contributions:
● Squeeze-and-attention with the residual structure module (SAR) fuses local and global context features, which makes up for the missing spatial information in the ordinary convolution process.
● Multi-scale feature fusion module (MSFF) is integrated into the network to capture TILs of smaller size, and combine the context features to enrich the decoding stage features.
● Convolution module with residual structure (RS) merges feature maps from different scales to strengthen the fusion capability of high-level and low-level semantic information.
Early cell segmentation methods such as threshold segmentation method [15], watershed algorithm [16] etc., are mostly using local features while ignoring global features, so the segmentation accuracy needs to be improved. Cell segmentation algorithms based on deep learning have been proposed and widely used in medical image segmentation, like fully convolutional networks (FCN) [17], UNet [18] and DeepLab networks [19]. The experiment has shown that compared to traditional segmentation algorithms, these networks have high performance.
Automated cell segmentation methods have been studied extensively in the literature [20,21,22,23,24]. The literature [20] introduced a combined loss function and adopted 4 × 4 max-pooling layers instead of widely used 2 × 2 to reinforce the learning of the cell's boundary area, thereby improving the network performance. The study [21] applied a weakly supervised multi-task learning algorithm for cell's segmentation and detection, which effectively solved the problems of difficult segmentation. In addition, Zhang et al. [22] put forward a dense dual-task network (DDTNet), this network uses the pyramid network as the backbone network. The boundary sensing module and feature fusion strategy are designed to realize the automatic detection and segmentation of TILs at the same time. The results show that it is not only superior to other advanced methods in detecting and segmentation indexes, but also can complete automatic annotation of unlabeled TILs. Study [23] found a new approach for the prognosis and treatment of hepatocellular carcinoma by utilizing Mask-RCNN to segment lymphocytes and extract spatial features of images. Based on the concept of autoencoder, Budginaite et al. [24] devised a multiple-image input layer architecture to ensure the automatic segmentation of TILs, where the convolutional texture blocks can not only improve the performance of the model but also reduce the complexity. However, the cell segmentation methods proposed by the above scholars are single network models, without considering the characteristics of pathological images and cells. Improving the network model by utilizing the characteristics of images can help further increase the segmentation effect of cells.
Attention mechanism is a method to measure the importance of different features [25]. Originally, the attentional mechanism is initially used in machine translation, but has gradually been applied to semantic segmentation because of its ability to filter high-value features. The attention mechanism can be divided into soft attention and hard attention. Since the hard attention mechanism is difficult to train, the soft attention mechanism module is often used to extract key features [26].
Related researches have shown that the spatial correlation between features can be captured by integrating learning mechanism into the network. Study [27] presented the squeeze-and-excitation (SE) module by introducing channel learning to emphasize useful features and suppress useless features. Residual attention network [28] exploited a stacked attention module to generate attention-aware features, and the residual learning coupled with the attention module can make the network expansion easier. Furthermore, Yin et al. [29] employed a selective attention regularization module based on the traditional classification network to improve the interpretability and reliability of the model. This type of attention module only used channel attention to enhance the main features, while ignoring the spatial features, and is not suitable for segmentation tasks. With the transformer, architecture success has been achieved in many natural language processing tasks, Gao et al. [30] proposed UTNet, which integrated self-attention into UNet frame for enhancing network performance. In addition, the literature [31] believed that semantic segmentation included two aspects, one is pixel-wise prediction, and the other is pixel grouping. Thus, the squeeze-and-attention (SA) module is designed to generate the attention mask of pixel group to improve the segmentation effect.
Ordinary segmentation networks applied single convolution and pooling operations to extract features, which led to under-segmentation due to a lack of relevant information between images. To address this problem, a number of studies have proposed multi-scale feature fusion methods to mine context information that improve the effect of network segmentation. Feature pyramid network [32] extracted semantic feature maps at different scales by a top-down architecture with lateral connections. The atrous spatial pyramid pooling (ASPP) module capitalized on dilated convolutions with different expansion rates to obtain semantic information of multi-scale contexts. UNet++ [33] introduced nested and dense jump connections to aggregate semantic features from different scales. Moreover, UNet3+ [34] exploited full-scale jump connections to make full use of multi-scale features. which combined low-level details and high-level semantics in full-scale feature maps to improve segmentation accuracy. In addition, atrous convolution and deformable convolution obtained multi-scale semantic information by changing the size and position of the convolution kernel.
In this section, we elaborate on the proposed TILs segmentation network. First, the pathological images of TILs were labeled by labelme software, and then segmented by the SAMS-Net algorithm. The algorithm framework of SAMS-Net is shown in Figure 2. Specifically, the coding structure of the model consists of a SA module and a residual structure, this structure is named SAR modules, and the blocks are connected by down-sampling operations. SAR modules enhance the spatial features of pathological images while extracting their features. In the middle of the second layer and the third layer, multi-scale feature fusion (MSFF) modules are added to fuse the low-level and high-level features. In the decoding stage, RS modules are designed based on the residual network to enhance the feature recovery capability of the model.
As the depth of the network increases, the "gradient disappearance problem" follows. A common solution method is to add residual learning. Residual learning structure was first proposed by He [35], which mainly uses jump connections to realize the identity mapping from the upper layer features to the lower layer network. The formula is as follows:
H(x)=F(x)+x | (1) |
Where, x indicates the network input of the local layer. F(x) stands for the residual learning part. This paper applies the idea of residual network to design the residual block. Because of the short connection, the convergence speed of the network is accelerated. The research utilizes the residual idea in both the encoding and decoding stages. In the encoding stage, the function of the residual structure is to enhance the ability of feature extraction, while in the decoding stage, the purpose of the residual structure is to fuse features from different scales to enhance the feature recovery ability. As shown in Figure 3, two 3 × 3 convolutions are used to extract features in the decoding module, and a 1 × 1 convolution is used to form a residual connection, so that the network can be extended to integrate high-level and low-level features.
SA module and residual structure are used to extract image features simultaneously. In the encoding module, two 3 × 3 convolutions are parallel with SA module and residual structure. Each SA module includes two parts: compression and attention extraction. Compression part uses global average pooling to obtain feature vectors. Attention extraction part realizes multi-scale feature aggregation through two attention convolutions channels and up-sampling operations, and generates a global soft attention mask at the same time. In addition, For the input image whose feature maps is X∈RH×W×C, a 1 × 1 convolution operation is used to match the output feature maps. Finally, the attention mask obtained from SA module and the feature map generated by trunk convolution are added to capture the key features. Among them, the role of the SA module is to enhance the attention feature of pixel-grouping. Encoding module is shown in Figure 4.
Figure 4 shows that the output characteristic graph is obtained by adding three input values, and its formula is as follows:
Xa = Up(Fa(Apl(Xin),C)) | (2) |
Xout=Xin+F(Xin,C)∗Xa+Xa | (3) |
Where, Xin∈RH×W×C, Xout∈RH′×W′×C′ are input and output feature maps, F(⋅) is the residual function, and C stands for two 3 × 3 convolutions. Up(⋅) represents the up-sampled operation, which is used to expand the number of channels of the output feature maps. Apl(⋅) represents the average pooling layer, which implements the compression operation of SA modules.
Receptive field is often regarded as the mapping region of the input image that can be seen by convolutional neural network (CNN). Receptive field size increases as the number of network layers deepen [36]. A large number of studies show that there are great differences in the characteristics of different scales. Small receptive field has lower detailed information, and large receptive field has stronger semantic information. The calculation formula of receptive field is shown in the formula:
RFi+1=RFi+(Ki+1−1)×i∏j=1Sj | (4) |
Among them, i represents the current number of network layers; K stands for the size of the convolution kernel of a certain layer of the network; S denotes the step size of a certain layer of the network. When i=0, RF0 is the input layer receptive field, and RF0=1.
Using features of different scales for segmentation tasks can obtain richer semantic information, which is conducive to improving the segmentation effect. The feature fusion method of the early network model is the jump connection between the same layers. This method only employs single-scale features and does not apply multi-scale features. After experimental verification, the characteristics of the receptive fields in the second and third layers of the SAMS-Net network are suitable for TILs that capture pathological images. Therefore, this study uses the second and third layers of the encoding part as the multi-scale feature fusion layer. To effectively combine shallow detail information with deep semantic information, feature maps of different scales are connected to each layer of the decoding module through up-sampling or pooling operation. The specific implementation is shown in Figure 5.
D4 is taken as an example to represent the implementation process of the multi-scale feature fusion module. When the image passes through the coding module, the features from the E2 layer and E3 layer are fused with the features of the E4 layer through the maximum pooling operation of different sizes, and the E5 features from the decoding part after the upsampling operation to obtain the rich information of the joint context.
Assuming that E0 and D0 are the input feature maps of the encoding part and the output feature maps of the decoding part, respectively. i indicates the number of current network layers. H(∗) is used to represent the nonlinear transformation of layer i, which can be realized by a series of operations, such as ReLu, Batch Normalization, and Pooling etc. The formula of the MSFF module is as follows:
Di=H([E2,E3,Ei,Di+1]) | (5) |
where [⋅] is concatenate operation, E2 and E3 stand for the feature maps of the 2 and 3 layers in the encoding stage, respectively. Di is the feature map of the current layer in the decoding stage. Ei is the feature map of the current layer in the encoding stage.
The experiment uses the HER2-positive breast cancer tumor infiltrating lymphocyte data set in the literature [37], which is marked by a professional pathologist, and the image size is 100 × 100 pixels. There is a risk of overfitting when the data set is too small. The data enhancement methods such as clipping, mirror transformation and flipping are used to prevent overfitting. According to the ratio of 8:1:1, the dataset was divided into a training set, validation set, and test set. This research uses a ten-fold cross-validation method to evaluate the generalization performance of the model.
The SAMS-Net algorithm is written using the Pytorch1.8.1 deep learning framework, and is trained on the experimental platform of Intel(R) Core (TM) i5-1135G7 CPU and NVIDIA Tesla V100 32 GB GPU. The initial learning rate of the algorithm is set to 0.0025. In this network, adaptive moment estimation (Adam) is used as the optimizer, DiceLoss is employed as the loss function, and L2 regularization operation is used to prevent overfitting.
To verify the effectiveness of the algorithm proposed in this study, we use IoU, DSC, positive prediction value (PPV), F1 score, pixel accuracy (PA), recall, Hausdorff distance (Hd) indicators to evaluate the performance of the algorithm. The IoU is used to measure the coincidence of the predicted graph with the ground-truth, the DSC is used to calculate the similarity between the predicted map and the ground truth, the closer the value is to 1, the better the segmentation effect. On the contrary, Hausdorff distance is a distance defined between any two sets in the metric space, the closer the value is to 0, the better the splitting effect. The calculation formulas are:
IoU=P∩GP∪G | (6) |
DSC=2|P∩G||P|+|G| | (7) |
PPV=TPTP+FP | (8) |
F1=2TP2TP+FP+FN | (9) |
PA=TP+TNTP+TN+FP+FN | (10) |
Recall=TPTP+FN | (11) |
Hd=max{h(P,G),(G,P)} | (12) |
Among them, in Eqs (6), (7) and (12), P represents the area of TILs predicted in the segmentation result, G represents the area of TILs in the ground truth image. In Eqs (8)–(11), TP is a true example, FP is a false positive example, TN is a true negative example, and FN is a false negative example.
In order to use multi-scale features more effectively, the fusion strategy between different layers of the algorithm is experimentally studied. The experimental results show that using different layers of information to integrate multi-scale features in TILs segmentation has a certain effect on improving the segmentation accuracy. However, the second and third layers of SAMS-Net can retain the semantic information of TILs to the maximum extent, improve the overall segmentation effect, and perform the best in TILs segmentation task. The experimental results are shown in Table 1. E1, E2, E3 and E4 represent the first, second, third, and fourth layers of the coding part respectively. It can be seen from the table that using E2 and E3 joint feature vectors have the best effect for the SAMS-Net algorithm.
Model | IoU (%) ↑ | DSC (%) ↑ | PPV (%) ↑ | F1 (%) ↑ | PA (%) ↑ | Recall (%) ↑ | Hd↓ |
E1 + E2 | 77.2 | 87.0 | 92.7 | 92.4 | 96.1 | 92.2 | 3.40 |
E1 + E3 | 76.1 | 86.3 | 92.0 | 91.9 | 96.2 | 92.1 | 3.503 |
E1 + E4 | 76.7 | 86.8 | 92.4 | 91.7 | 94.9 | 91.3 | 3.781 |
E2 + E4 | 76.2 | 86.4 | 92.3 | 92.0 | 96.1 | 91.8 | 3.450 |
E3 + E4 | 75.8 | 86.1 | 92.0 | 91.8 | 95.8 | 91.9 | 3.443 |
E2+E3 | 77.5 | 87.2 | 93.0 | 92.6 | 96.4 | 92.1 | 3.354 |
Note: Different metrics between the automated and ground truth for evaluating segmentation performance. Where ↑ means that the larger the value, the better the effect, ↓ means that the smaller the value, the better the effect. The best results are highlighted in bold. |
In order to verify the effectiveness of the proposed algorithm, the proposed SAMS-Net algorithm is compared with other classical segmentation algorithms in Table 1 (such as FCN network, DeepLab V3+ network, and UNet network, etc.) on the same experimental platform. The experimental results are shown in Table 2. It can be seen from the experimental results that SAMS-Net performs best in the TILs segmentation task, and its IoU, DSC and other indicators are optimal among the eight segmentation algorithms.
Model | IoU (%) ↑ | DSC (%) ↑ | PPV (%) ↑ | F1 (%) ↑ | PA (%) ↑ | Recall (%) ↑ | Hd↓ |
FCN [17] | 74.5 | 85.1 | 91.8 | 91.3 | 95.6 | 91.0 | 3.460 |
DeepLabV3+ [19] | 70.1 | 82.3 | 90.5 | 89.7 | 95.0 | 89.2 | 4.177 |
SegNet [38] | 73.2 | 84.4 | 90.9 | 90.8 | 95.6 | 91.0 | 3.729 |
ENet [39] | 51.5 | 67.9 | 81.9 | 81.0 | 91.2 | 81.1 | 4.465 |
UNet [18] | 73.7 | 84.7 | 90.1 | 91.1 | 95.7 | 90.8 | 3.498 |
R2UNet [40] | 74.1 | 85.1 | 92.0 | 91.2 | 95.8 | 90.7 | 3.574 |
UNet++ [33] | 75.6 | 85.8 | 92.3 | 91.7 | 96.0 | 91.3 | 3.368 |
SAMS-Net(ours) | 77.5 | 87.2 | 93.0 | 92.6 | 96.4 | 92.1 | 3.354 |
Note: Different metrics between the automated and ground truth for evaluating segmentation performance. Where ↑ means that the larger the value, the better the effect, ↓ means that the smaller the value, the better the effect. The best results are highlighted in bold. |
The experimental results show that the SAMS-Net has a good effect in the TILs segmentation task, and its IoU, DSC and other indicators have achieved the best results among the eight segmentation algorithms. Compared with UNet, IoU increased by 3.8%, DSC promoted by 2.5%, compared with FCN, DeepLabV3+, SegNet, R2UNet and UNet+, IoU increased by 3, 7.4, 4.3, 3.1 and 1.9%, respectively. DSC is improved by 2.1, 4.9, 2.8, 2.1 and 1.4% respectively, which proves the effectiveness of SAMS-NET in segmentation. The analysis shows that the FCN and SegNet networks have the problem of long training time due to a large number of parameters, and the failure to consider the global information is easy to lose the image details, which leads to the segmentation is not fine enough. In order to reduce the number of model parameters, ENet algorithm carries out a down-sampling operation in advance, which leads to the serious loss of image spatial information and poor segmentation ability. DeepLabV3+ algorithm adds a variety of modules to reduce model parameters and enhance feature extraction ability, which leads to feature information redundancy and makes the network unable to learn key information, thus making the network segmentation effect low. Although the UNet, UNet++ and R2UNet networks consider the relationship between pixels, they fail to fully relate the context information to obtain richer features and thus lose part of the edge information, resulting in a slightly lower segmentation ability.
In view of the residual attention module and multi-scale feature fusion module designed by our proposed SAMS-NET algorithm, the network not only pays attention to the key information in the image but also considers the context connection, so the image segmentation results are better and can achieve better segmentation. In order to better analyze the segmentation effect, this study conducts a visual analysis on SAMS-NET and its comparison algorithm, and the comparison results are shown in Figure 6.
According to the segmentation results, SegNet, UNet and UNet++algorithms mistakenly divide normal cells into TILs cells. FCN and DeepLabV3+show the problem of cell segmentation edge adhesion in the segmentation process, and ENet shows unclear segmentation edges and burrs. Compared with other segmentation networks, the overall segmentation effect of SAMS-Net is improved, which effectively avoids under segmentation and over-segmentation, and the overall segmentation effect is better. However, although the SAMS-Net has a certain improvement effect on the segmentation ability of TILs, there are still some unclear edges and segmentation errors in some segmented regions, which may be caused by the small dataset and unbalanced front and background pixels. Adding more training samples to enhance the feature learning ability of the network can further improve the segmentation effect.
To measure the generalization performance of the algorithm and explore the influence of different modules on the algorithm, multiple improved modules were split and ablation experiments were used to validate the contribution of each module to SAMS-Net. The verification results are shown in Table 3. It can be seen from the table that compared to the basic network, each module of SAMS-Net contributes to the segmentation task of this paper, moreover, the combination of multiple modules can achieve the best effect.
SA | MSFF | RS | IoU (%) ↑ | DSC (%) ↑ | PPV (%) ↑ | F1 (%) ↑ | PA (%) ↑ | Recall (%) ↑ | Hd↓ |
74.8 | 85.3 | 91.2 | 91.4 | 96.0 | 91.7 | 3.610 | |||
✔ | 76.2 | 86.4 | 92.4 | 92.0 | 96.2 | 91.9 | 3.477 | ||
✔ | 76.3 | 86.4 | 92.5 | 92.0 | 96.2 | 91.7 | 3.388 | ||
✔ | 75.6 | 85.9 | 91.4 | 91.7 | 95.4 | 91.3 | 3.512 | ||
✔ | ✔ | 75.9 | 86.2 | 92.5 | 91.9 | 96.1 | 91.5 | 3.506 | |
✔ | ✔ | 76.1 | 86.3 | 92.4 | 92.0 | 96.1 | 91.7 | 3.454 | |
✔ | ✔ | 75.7 | 86.0 | 92.5 | 91.8 | 96.1 | 91.4 | 3.498 | |
✔ | ✔ | ✔ | 77.5 | 87.2 | 93.0 | 92.6 | 96.4 | 92.1 | 3.354 |
Note: Ablation results of different components. Where ↑ means that the larger the value, the better the effect, ↓ means that the smaller the value, the better the effect. The best results are highlighted in bold. |
As can be seen from the table, compared with the basic network, each module of SAMS-NET has contributed to the segmentation task of this research, and the best effect can be achieved through the combination of multiple modules.
In order to verify the effectiveness of the data enhancement operation and L2 regularization [41] method on the algorithm, the benchmark algorithm is compared with the algorithm after adding data enhancement and L2 regularization, and the comparison results are shown in Figure 7.
Where, Base is the algorithm without data enhancement and L2 regularization, Aug stands for data enhancement operation, and L2 stands for L2 regularization method. As can be seen, compared with the Base network, the IoU index of the algorithm is increased by 4.4% and DSC index is improved by 3% after adding the data enhancement operation and L2 regularization method. The results show that these two operations play a certain role in improving the segmentation effect.
Related research shows that TILs can predict cancer chemotherapy response and survival outcome [42], and can provide a basis for precise treatment of cancer. This paper proposes a segmentation network based on the squeeze attention mechanism and multi-scale feature fusion to segment TILs in breast cancer pathological images. SAMS-Net has three modules: SAR module, MSFF module, and RS module. Different from the traditional attention mechanism, the interdependence between spatial channels is effectively taken into consideration by the SAR module, which can enhance the dense prediction at the pixel level. MSFF module effectively combines low-level and high-level semantic features in different scale feature maps on the basis of enhancing context features. RS module can enhance the ability of gradient return to speed up training.
Lacking the spatial information of the image and the pixel difference of the segmentation target are common problems in traditional segmentation networks, which cause the unsuitability for the task of cell segmentation. Based on the traditional network, the segmentation effect of different receptive fields on the cell area was taken into account in this paper, and a MSFF module combining multiple receptive fields were proposed to solve the problem of difficulty in capturing the segmentation process due to small cell pixels. SAMS-Net uses the attention mechanism combined with the residual structure to extract richer semantic information. A large number of experiments have proved that among the state-of-the-art methods, SAMS-Net has a better segmentation effect and can further provide important evidence for the prognosis and treatment of cancer. In addition, this study can also be applied to the diagnosis of various diseases by optical imaging (optical coherence tomography), such as age-related macular degeneration and Stargardt's disease [43,44,45]. Due to the uses of multiple modules to improve the segmentation effect, which increases the number of parameters and calculations of the model. In the future, the network model needs to be further improved to reduce the scores of parameters and calculations.
This work is supported by the National Nature Science Foundation of China (No. 61872225), the Natural Science Foundation of Shandong Province (No. ZR2020KF013, No. ZR2020ZD44, No. ZR2019ZD04, No. ZR2020QF043) and Introduction and Cultivation Program for Young Creative Talents in Colleges and Universities of Shandong Province(No.2019–173), the Special fund of Qilu Health and Health Leading Talents Training Project.
We declare that we do not have any commercial or associative interest that represents a conflict of interest in connection with the work submitted.
[1] |
C. Kolberg-Liedtke, F. Feuerhake, M. Garke, M. Christgen, R. Kates, E. M. Grischke, et al., Impact of stromal tumor-infiltrating lymphocytes (sTILs) on response to neoadjuvant chemotherapy in triple-negative early breast cancer in the WSG-ADAPT TN trial, Breast Cancer Res., 24 (2022), 1–13. https://doi.org/10.1186/s13058-022-01552-w doi: 10.1186/s13058-022-01552-w
![]() |
[2] | T. Nguyen, M. V. Ngo, V. P. Nguyen, Histopathological imaging classification of breast tissue for cancer diagnosis support using deep learning models, in International Conference on Industrial Networks and Intelligent Systems, 444 (2022), 152–164. https://doi.org/10.1007/978-3-031-08878-0_11 |
[3] | G. Floris, G. Broeckx, A. Antoranz, M. D. Schepper, R. Salgado, C. Desmedt, et al., Tumor infiltrating lymphocytes in breast cancer: Implementation of a new histopathological biomarker, in Biomarkers of the Tumor Microenvironment, Springer, (2022), 207–243. https://doi.org/10.1007/978-3-030-98950-7_13 |
[4] |
H. Kuroda, T. Jamiyan, R. Yamaguchi, A. Kakumoto, A. Abe, O. Harada, et al., Tumor microenvironment in triple-negative breast cancer: The correlation of tumor-associated macrophages and tumor-infiltrating lymphocytes, Clin. Transl. Oncol., 23 (2021), 2513–2525. https://doi.org/10.1007/s12094-021-02652-3 doi: 10.1007/s12094-021-02652-3
![]() |
[5] |
T. Odate, M. K. Le, M. Kawai, M. Kubota, Y. Yamaguchi, T. Kondo, Tumor-infiltrating lymphocytes in breast FNA biopsy cytology: A predictor of tumor-infiltrating lymphocytes in histologic evaluation, Cancer Cytopathol., 130 (2022), 336–343. https://doi.org/10.1002/cncy.22551 doi: 10.1002/cncy.22551
![]() |
[6] |
S. Wang, J. Sun, K. Chen, P. Ma, N. Li, Perspectives of tumor-infiltrating lymphocyte treatment in solid tumors, BMC Med., 140 (2021), 1–7. https://doi.org/10.1186/s12916-021-02006-4 doi: 10.1186/s12916-021-02006-4
![]() |
[7] |
Y. Li, Z. Yang, Y. Wang, X. Cao, X. Xu, A neural network approach to analyze cross-sections of muscle fibers in pathological images, Comput. Biol. Med., 104 (2019), 97–104. https://doi.org/10.1016/j.compbiomed.2018.11.007 doi: 10.1016/j.compbiomed.2018.11.007
![]() |
[8] |
X. Wu, Y. Zheng, C. H. Chu, L. Cheng, J. Kim, Applying deep learning technology for automatic fall detection using mobile sensors, Biomed. Signal Process. Control, 72 (2022), 103355. https://doi.org/10.1016/j.bspc.2021.103355 doi: 10.1016/j.bspc.2021.103355
![]() |
[9] |
J. Cheng, S. Tian, L. Yu, C. Gao, X. Kang, X. Ma, et al., ResGANet: Residual group attention network for medical image classification and segmentation, Med. Image Anal., 76 (2022), 102313. https://doi.org/10.1016/j.media.2021.102313 doi: 10.1016/j.media.2021.102313
![]() |
[10] |
D. Müller, I. Soto-Rey, F. Kramer, An analysis on ensemble learning optimized medical image classification with deep convolutional neural networks, IEEE Access, 10 (2022), 66467–66480. https://doi.org/10.1109/ACCESS.2022.3182399 doi: 10.1109/ACCESS.2022.3182399
![]() |
[11] |
W. Pinaya, P. D. Tudosiu, R. Gray, G. Rees, P. Nachev, S. Ourselin, et al., Unsupervised brain imaging 3d anomaly detection and segmentation with transformers, Med. Image Anal., 79 (2022), 102475. https://doi.org/10.1016/j.media.2022.102475 doi: 10.1016/j.media.2022.102475
![]() |
[12] |
S. Javed, A. Mahmood, J. Dias, N. Werghi, N. Rajpoot, Spatially constrained context-aware hierarchical deep correlation filters for nucleus detection in histology images, Med. Image Anal., 72 (2021), 102104. https://doi.org/10.1016/j.media.2021.102104 doi: 10.1016/j.media.2021.102104
![]() |
[13] | Z. Tan, J. Feng, J. Zhou, SGNet: Structure-aware graph-based network for airway semantic segmentation, in International Conference on Medical Image Computing and Computer-Assisted Intervention, (2021), 153–163. https://doi.org/10.1007/978-3-030-87193-2_15 |
[14] |
Mehdi, S. Örjan, W. Chunliang, Prior-aware autoencoders for lung pathology segmentation, Med. Image Anal., 80 (2022), 102491. https://doi.org/10.1016/j.media.2022.102491 doi: 10.1016/j.media.2022.102491
![]() |
[15] | T. Vicar, J. Chmelik, R. Kolar, Cell segmentation in quantitative phase images with improved iterative thresholding method, in European Medical and Biological Engineering Conference, (2020), 233–239. https://doi.org/10.1007/978-3-030-64610-3_27 |
[16] |
M. Gamarra, E. Zurek, H. J. Escalante, L. Hurtado, H. San-Juan-Vergara, Split and merge watershed: A two-step method for cell segmentation in fluorescence microscopy images, Biomed. Signal Process. Control, 53 (2019), 101575. https://doi.org/10.1016/j.bspc.2019.101575 doi: 10.1016/j.bspc.2019.101575
![]() |
[17] |
E. Shelhamer, J. Long, T. Darrell, Fully convolutional networks for semantic segmentation, IEEE Trans. Pattern Anal. Mach. Intell., 39 (2017), 640–651. https://doi.org/10.1109/TPAMI.2016.2572683 doi: 10.1109/TPAMI.2016.2572683
![]() |
[18] | O. Ronneberger, P. Fischer, T. Brox, U-Net: Convolutional networks for biomedical image segmentation, in Proceedings of the International Conference on Medical Image Computing and Computer-Assisted Intervention, (2015), 234–241. https://doi.org/10.1007/978-3-319-24574-4_28 |
[19] | L. C. Chen, Y. Zhu, G. Papandreou, F. Schroff, H. Adam, Encoder-decoder with atrous separable convolution for semantic image segmentation, in Proceedings of the European Conference on Computer Vision, 11211 (2018), 833–851. https://doi.org/10.1007/978-3-030-01234-2_49 |
[20] | C. E. Akbas, M. Kozubek, Condensed U-Net (Cu-Net): An improved u-net architecture for cell segmentation powered by 4×4 max-pooling layers, in Proceedings of the 2020 IEEE 17th International Symposium on Biomedical Imaging (ISBI), (2020), 446–450. https://doi.org/10.1109/ISBI45749.2020.9098351 |
[21] | C. E. Akbaş, M. Kozubek, Weakly supervised multi-task learning for cell detection and segmentation, in Proceedings of the 2020 IEEE 17th International Symposium on Biomedical Imaging (ISBI), (2020), 513–516. https://doi.org/10.1109/ISBI45749.2020.9098518 |
[22] |
X. Zhang, X. Zhu, K. Tang, Y. Zhao, Z. Lu, Q. Feng, DDTNet: A dense dual-task network for tumor-infiltrating lymphocyte detection and segmentation in histopathological images of breast cancer, Med. Image Anal., 78 (2022), 102415. https://doi.org/10.1016/j.media.2022.102415 doi: 10.1016/j.media.2022.102415
![]() |
[23] |
H. Wang, Y. Jiang, B. Li, Y. Cui, R. Li, Single-cell spatial analysis of tumor and immune microenvironment on whole-slide image reveals hepatocellular carcinoma subtypes, Cancers, 12 (2020), 3562. https://doi.org/10.3390/cancers12123562 doi: 10.3390/cancers12123562
![]() |
[24] |
E. Budginait, M. A. Morkūnas, Laurinaviius, P. Treigys, Deep learning model for cell nuclei segmentation and lymphocyte identification in whole slide histology images, Informatica, 1 (2021), 1–18. https://doi.org/10.15388/20-INFOR442 doi: 10.15388/20-INFOR442
![]() |
[25] |
J. Li, K. Jin, D. Zhou, N. Kubota, Z. Ju, Attention mechanism-based cnn for facial expression recognition, Neurocomputing, 411 (2020). https://doi.org/10.1016/j.neucom.2020.06.014 doi: 10.1016/j.neucom.2020.06.014
![]() |
[26] |
Z. Li, Z. Peng, S. Tang, C. Zhang, H. Ma, Text summarization method based on double attention pointer network, IEEE Access, 8 (2020). 11279–11288. https://doi.org/10.1109/ACCESS.2020.2965575 doi: 10.1109/ACCESS.2020.2965575
![]() |
[27] | H. Jie, S. Li, S. Gang, Squeeze-and-excitation networks, in Proceedings of the 2018 IEEE/CVF Conference on Computer Vision and Pattern Recognition (CVPR), (2018), 7132–7141. https://doi.org/10.1109/CVPR.2018.00745 |
[28] | W. Fei, M. Jiang, Q. Chen, S. Yang, X. Tang, Residual attention network for image classification, in Proceedings of the IEEE Conference on Computer Vision and Pattern Recognition (CVPR), (2017), 6450–6458. https://doi.org/10.1109/CVPR.2017.683 |
[29] | C. Yin, S. Liu, R. Shao, P. C. Yuen, Focusing on clinically interpretable features: selective attention regularization for liver biopsy image classification, in Proceedings of the International Conference on Medical Image Computing and Computer-Assisted Intervention (MICCAI), 12905 (2021), 153–162. https://doi.org/10.1007/978-3-030-87240-3_15 |
[30] | Y. Gao, M. Zhou, D. Metaxas, UTNet: A hybrid transformer architecture for medical image segmentation, in Proceedings of the International Conference on Medical Image Computing and Computer-Assisted Intervention (MICCAI), 12903 (2021), 61–71. https://doi.org/10.1007/978-3-030-87199-4_6 |
[31] | Z. Zhong, Z. Q. Lin, R. Bidart, X. Hu, A. Wong, Squeeze-and-attention networks for semantic segmentation, in Proceedings of the 2020 IEEE/CVF Conference on Computer Vision and Pattern Recognition (CVPR), (2020), 13062–13071. https://doi.org/10.1109/CVPR42600.2020.01308 |
[32] | T. Y. Lin, P. Dollar, R. Girshick, K. He, B. Hariharan, S. Belongie, Feature pyramid networks for object detection, in Proceedings of the IEEE Conference on Computer Vision and Pattern Recognition (CVPR), (2017), 936–944. https://doi.org/10.1109/CVPR.2017.106 |
[33] |
Z. Zhou, M. Siddiquee, N. Tajbakhsh, J. Liang, Unet++: Redesigning skip connections to exploit multiscale features in image segmentation, IEEE Trans. Med. Imaging, 39 (2020), 1856–1867. https://doi.org/10.1109/TMI.2019.2959609 doi: 10.1109/TMI.2019.2959609
![]() |
[34] | H. Huang, L. Lin, R. Tong, H. Hu, J. Wu, UNet 3+: A full-scale connected unet for medical image segmentation, in Proceedings of the ICASSP 2020-2020 IEEE International Conference on Acoustics, (2020), 1055–1059. https://doi.org/10.1109/ICASSP40776.2020.9053405 |
[35] | K. M. He, X. Y. Zhang, S. Q. Ren, J. Sun, Deep residual learning for image recognition, in Proceedings of the IEEE/CVF Conference on Computer Vision and Pattern Recognition (CVPR), (2016), 770–778. https://doi.org/10.1109/CVPR.2016.90 |
[36] |
C. Zhao, M. Hu, F. Ju, Z. Chen, Y. Li, Y. Feng, Convolutional neural network with spatio-temporal-channel attention for remote heart rate estimation, Visual Comput., 2022 (2022), 1–19. https://doi.org/10.1007/s00371-022-02624-w doi: 10.1007/s00371-022-02624-w
![]() |
[37] |
A. Janowczyk, A. Madabhushi, Deep learning for digital pathology image analysis: A comprehensive tutorial with selected use cases, J. Pathol. Inf., 7 (2016), 1–18. https://doi.org/10.4103/2153-3539.186902 doi: 10.4103/2153-3539.186902
![]() |
[38] |
V. Badrinarayanan, A. Kendall, R. Cipolla, Segnet: A deep convolutional encoder-decoder architecture for image segmentation, IEEE Trans. Pattern Anal. Mach. Intel., 39 (2017), 2481–2495. https://doi.org/10.1109/TPAMI.2016.2644615 doi: 10.1109/TPAMI.2016.2644615
![]() |
[39] | A. Paszke, A. Chaurasia, S. Kim, E. Culurciello, Enet: A deep neural network architecture for real-time semantic segmentation, preprint, arXiv: 1606.02147. https://doi.org/10.48550/arXiv.1606.02147 |
[40] |
M. Z. Alom, C. Yakopcic, M. Hasan, T. M. Taha, V. K. Asari, Recurrent residual u-net for medical image segmentation, J. Med. Imaging, 6 (2019), 1–16. https://doi.org/10.1117/1.JMI.6.1.014006 doi: 10.1117/1.JMI.6.1.014006
![]() |
[41] | Y. Wu, W. Cao, Y. Liu, Z. Ming, J. Li, B. Lu, Semantic auto-encoder with l2-norm constraint for zero-shot learning, in 2021 13th International Conference on Machine Learning and Computing, (2021), 101–105. https://doi.org/10.1145/3457682.3457699 |
[42] |
F. Li, Y. Zhao, Y. Wei, Y. Xi, H. Bu, Tumor-infiltrating lymphocytes improve magee equation–based prediction of pathologic complete response in HR-Positive/HER2-Negative breast cancer, Am. J. Clin. Oncol., 158 (2022), 291–299. https://doi.org/10.1093/ajcp/aqac041 doi: 10.1093/ajcp/aqac041
![]() |
[43] |
K. M. Ratheesh, L. K. Seah, V. M Murukeshan, Spectral phase-based automatic calibration scheme for swept source-based optical coherence tomography systems, Phys. Med. Biol., 61 (2016) 7652–7663. https://doi.org/10.1088/0031-9155/61/21/7652 doi: 10.1088/0031-9155/61/21/7652
![]() |
[44] |
R. K. Meleppat, C. R. Fortenbach, Y. Jian, K. Wagner, B. S. Modjtahedi, M. J. Motta, et al., In Vivo imaging of retinal and choroidal morphology and vascular plexuses of vertebrates using swept-source optical coherence tomography, Transl. Vision Sci. Technol., 11 (2022), 1–21. https://doi.org/10.1167/tvst.11.8.11 doi: 10.1167/tvst.11.8.11
![]() |
[45] |
P. Udayaraju, P. Jeyanthi, Early diagnosis of age-related macular degeneration (ARMD) using deep learning, Intell. Syst. Sustainable Comput., 289 (2022), 657–663. https://doi.org/10.1007/978-981-19-0011-2_59. doi: 10.1007/978-981-19-0011-2_59
![]() |
1. | Alessio Fiorin, Carlos López Pablo, Marylène Lejeune, Ameer Hamza Siraj, Vincenzo Della Mea, Enhancing AI Research for Breast Cancer: A Comprehensive Review of Tumor-Infiltrating Lymphocyte Datasets, 2024, 2948-2933, 10.1007/s10278-024-01043-8 | |
2. | D. P. Yadav, Turki Aljrees, Deepak Kumar, Ankit Kumar, Kamred Udham Singh, Teekam Singh, Spatial attention-based residual network for human burn identification and classification, 2023, 13, 2045-2322, 10.1038/s41598-023-39618-0 | |
3. | Haiyan Song, Cuihong Liu, Shengnan Li, Peixiao Zhang, TS-GCN: A novel tumor segmentation method integrating transformer and GCN, 2023, 20, 1551-0018, 18173, 10.3934/mbe.2023807 | |
4. | Lei Yuan, Jianhua Song, Yazhuo Fan, FM-Unet: Biomedical image segmentation based on feedback mechanism Unet, 2023, 20, 1551-0018, 12039, 10.3934/mbe.2023 | |
5. | Nurkhairul Bariyah Baharun, Afzan Adam, Mohamed Afiq Hidayat Zailani, Nasir M. Rajpoot, Qiaoyi XU, Reena Rahayu Md Zin, Automated scoring methods for quantitative interpretation of Tumour infiltrating lymphocytes (TILs) in breast cancer: a systematic review, 2024, 24, 1471-2407, 10.1186/s12885-024-12962-8 | |
6. | Xiang Li, Jian Wang, Haifeng Wei, Jinyu Cong, Hongfu Sun, Pingping Wang, Benzheng Wei, MH2AFormer: An Efficient Multiscale Hierarchical Hybrid Attention With a Transformer for Bladder Wall and Tumor Segmentation, 2024, 28, 2168-2194, 4772, 10.1109/JBHI.2024.3397698 | |
7. | Lei Yuan, Jianhua Song, Yazhuo Fan, FM-Unet: Biomedical image segmentation based on feedback mechanism Unet, 2023, 20, 1551-0018, 12039, 10.3934/mbe.2023535 | |
8. | Jie Huang, Yangsheng Hu, Yuanchao Xue, Yu Yao, Haitao Wang, Jianfeng He, A Deep Learning Model for Assessing Ki-67 and Tumor-Infiltrating Lymphocytes Using Multiscale Attention and Pixel Channel Fusion, 2024, 12, 2169-3536, 167856, 10.1109/ACCESS.2024.3494241 |
Model | IoU (%) ↑ | DSC (%) ↑ | PPV (%) ↑ | F1 (%) ↑ | PA (%) ↑ | Recall (%) ↑ | Hd↓ |
E1 + E2 | 77.2 | 87.0 | 92.7 | 92.4 | 96.1 | 92.2 | 3.40 |
E1 + E3 | 76.1 | 86.3 | 92.0 | 91.9 | 96.2 | 92.1 | 3.503 |
E1 + E4 | 76.7 | 86.8 | 92.4 | 91.7 | 94.9 | 91.3 | 3.781 |
E2 + E4 | 76.2 | 86.4 | 92.3 | 92.0 | 96.1 | 91.8 | 3.450 |
E3 + E4 | 75.8 | 86.1 | 92.0 | 91.8 | 95.8 | 91.9 | 3.443 |
E2+E3 | 77.5 | 87.2 | 93.0 | 92.6 | 96.4 | 92.1 | 3.354 |
Note: Different metrics between the automated and ground truth for evaluating segmentation performance. Where ↑ means that the larger the value, the better the effect, ↓ means that the smaller the value, the better the effect. The best results are highlighted in bold. |
Model | IoU (%) ↑ | DSC (%) ↑ | PPV (%) ↑ | F1 (%) ↑ | PA (%) ↑ | Recall (%) ↑ | Hd↓ |
FCN [17] | 74.5 | 85.1 | 91.8 | 91.3 | 95.6 | 91.0 | 3.460 |
DeepLabV3+ [19] | 70.1 | 82.3 | 90.5 | 89.7 | 95.0 | 89.2 | 4.177 |
SegNet [38] | 73.2 | 84.4 | 90.9 | 90.8 | 95.6 | 91.0 | 3.729 |
ENet [39] | 51.5 | 67.9 | 81.9 | 81.0 | 91.2 | 81.1 | 4.465 |
UNet [18] | 73.7 | 84.7 | 90.1 | 91.1 | 95.7 | 90.8 | 3.498 |
R2UNet [40] | 74.1 | 85.1 | 92.0 | 91.2 | 95.8 | 90.7 | 3.574 |
UNet++ [33] | 75.6 | 85.8 | 92.3 | 91.7 | 96.0 | 91.3 | 3.368 |
SAMS-Net(ours) | 77.5 | 87.2 | 93.0 | 92.6 | 96.4 | 92.1 | 3.354 |
Note: Different metrics between the automated and ground truth for evaluating segmentation performance. Where ↑ means that the larger the value, the better the effect, ↓ means that the smaller the value, the better the effect. The best results are highlighted in bold. |
SA | MSFF | RS | IoU (%) ↑ | DSC (%) ↑ | PPV (%) ↑ | F1 (%) ↑ | PA (%) ↑ | Recall (%) ↑ | Hd↓ |
74.8 | 85.3 | 91.2 | 91.4 | 96.0 | 91.7 | 3.610 | |||
✔ | 76.2 | 86.4 | 92.4 | 92.0 | 96.2 | 91.9 | 3.477 | ||
✔ | 76.3 | 86.4 | 92.5 | 92.0 | 96.2 | 91.7 | 3.388 | ||
✔ | 75.6 | 85.9 | 91.4 | 91.7 | 95.4 | 91.3 | 3.512 | ||
✔ | ✔ | 75.9 | 86.2 | 92.5 | 91.9 | 96.1 | 91.5 | 3.506 | |
✔ | ✔ | 76.1 | 86.3 | 92.4 | 92.0 | 96.1 | 91.7 | 3.454 | |
✔ | ✔ | 75.7 | 86.0 | 92.5 | 91.8 | 96.1 | 91.4 | 3.498 | |
✔ | ✔ | ✔ | 77.5 | 87.2 | 93.0 | 92.6 | 96.4 | 92.1 | 3.354 |
Note: Ablation results of different components. Where ↑ means that the larger the value, the better the effect, ↓ means that the smaller the value, the better the effect. The best results are highlighted in bold. |
Model | IoU (%) ↑ | DSC (%) ↑ | PPV (%) ↑ | F1 (%) ↑ | PA (%) ↑ | Recall (%) ↑ | Hd↓ |
E1 + E2 | 77.2 | 87.0 | 92.7 | 92.4 | 96.1 | 92.2 | 3.40 |
E1 + E3 | 76.1 | 86.3 | 92.0 | 91.9 | 96.2 | 92.1 | 3.503 |
E1 + E4 | 76.7 | 86.8 | 92.4 | 91.7 | 94.9 | 91.3 | 3.781 |
E2 + E4 | 76.2 | 86.4 | 92.3 | 92.0 | 96.1 | 91.8 | 3.450 |
E3 + E4 | 75.8 | 86.1 | 92.0 | 91.8 | 95.8 | 91.9 | 3.443 |
E2+E3 | 77.5 | 87.2 | 93.0 | 92.6 | 96.4 | 92.1 | 3.354 |
Note: Different metrics between the automated and ground truth for evaluating segmentation performance. Where ↑ means that the larger the value, the better the effect, ↓ means that the smaller the value, the better the effect. The best results are highlighted in bold. |
Model | IoU (%) ↑ | DSC (%) ↑ | PPV (%) ↑ | F1 (%) ↑ | PA (%) ↑ | Recall (%) ↑ | Hd↓ |
FCN [17] | 74.5 | 85.1 | 91.8 | 91.3 | 95.6 | 91.0 | 3.460 |
DeepLabV3+ [19] | 70.1 | 82.3 | 90.5 | 89.7 | 95.0 | 89.2 | 4.177 |
SegNet [38] | 73.2 | 84.4 | 90.9 | 90.8 | 95.6 | 91.0 | 3.729 |
ENet [39] | 51.5 | 67.9 | 81.9 | 81.0 | 91.2 | 81.1 | 4.465 |
UNet [18] | 73.7 | 84.7 | 90.1 | 91.1 | 95.7 | 90.8 | 3.498 |
R2UNet [40] | 74.1 | 85.1 | 92.0 | 91.2 | 95.8 | 90.7 | 3.574 |
UNet++ [33] | 75.6 | 85.8 | 92.3 | 91.7 | 96.0 | 91.3 | 3.368 |
SAMS-Net(ours) | 77.5 | 87.2 | 93.0 | 92.6 | 96.4 | 92.1 | 3.354 |
Note: Different metrics between the automated and ground truth for evaluating segmentation performance. Where ↑ means that the larger the value, the better the effect, ↓ means that the smaller the value, the better the effect. The best results are highlighted in bold. |
SA | MSFF | RS | IoU (%) ↑ | DSC (%) ↑ | PPV (%) ↑ | F1 (%) ↑ | PA (%) ↑ | Recall (%) ↑ | Hd↓ |
74.8 | 85.3 | 91.2 | 91.4 | 96.0 | 91.7 | 3.610 | |||
✔ | 76.2 | 86.4 | 92.4 | 92.0 | 96.2 | 91.9 | 3.477 | ||
✔ | 76.3 | 86.4 | 92.5 | 92.0 | 96.2 | 91.7 | 3.388 | ||
✔ | 75.6 | 85.9 | 91.4 | 91.7 | 95.4 | 91.3 | 3.512 | ||
✔ | ✔ | 75.9 | 86.2 | 92.5 | 91.9 | 96.1 | 91.5 | 3.506 | |
✔ | ✔ | 76.1 | 86.3 | 92.4 | 92.0 | 96.1 | 91.7 | 3.454 | |
✔ | ✔ | 75.7 | 86.0 | 92.5 | 91.8 | 96.1 | 91.4 | 3.498 | |
✔ | ✔ | ✔ | 77.5 | 87.2 | 93.0 | 92.6 | 96.4 | 92.1 | 3.354 |
Note: Ablation results of different components. Where ↑ means that the larger the value, the better the effect, ↓ means that the smaller the value, the better the effect. The best results are highlighted in bold. |