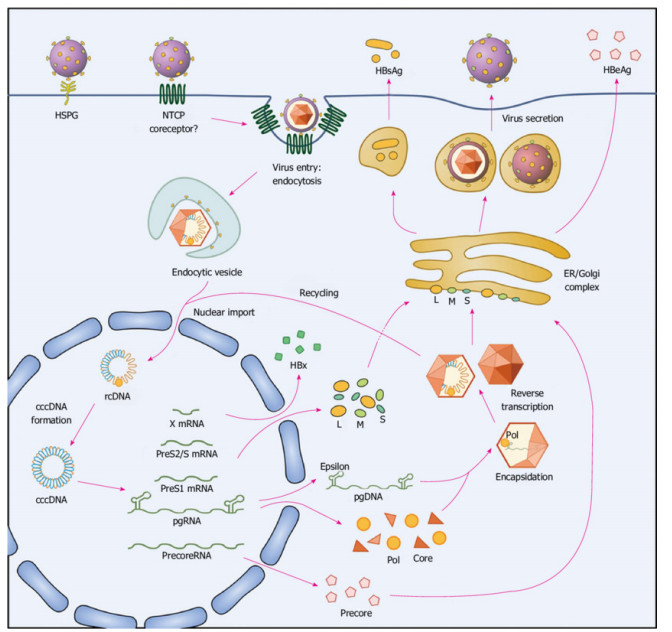
This paper mainly studies the dynamical behavior of a stochastic COVID-19 model. First, the stochastic COVID-19 model is built based on random perturbations, secondary vaccination and bilinear incidence. Second, in the proposed model, we prove the existence and uniqueness of the global positive solution using random Lyapunov function theory, and the sufficient conditions for disease extinction are obtained. It is analyzed that secondary vaccination can effectively control the spread of COVID-19 and the intensity of the random disturbance can promote the extinction of the infected population. Finally, the theoretical results are verified by numerical simulations.
Citation: Xinyu Bai, Shaojuan Ma. Stochastic dynamical behavior of COVID-19 model based on secondary vaccination[J]. Mathematical Biosciences and Engineering, 2023, 20(2): 2980-2997. doi: 10.3934/mbe.2023141
[1] | Fathalla A. Rihan, Hebatallah J. Alsakaji . Analysis of a stochastic HBV infection model with delayed immune response. Mathematical Biosciences and Engineering, 2021, 18(5): 5194-5220. doi: 10.3934/mbe.2021264 |
[2] | Saima Rashid, Rehana Ashraf, Qurat-Ul-Ain Asif, Fahd Jarad . Novel stochastic dynamics of a fractal-fractional immune effector response to viral infection via latently infectious tissues. Mathematical Biosciences and Engineering, 2022, 19(11): 11563-11594. doi: 10.3934/mbe.2022539 |
[3] | Alia M. Alzubaidi, Hakeem A. Othman, Saif Ullah, Nisar Ahmad, Mohammad Mahtab Alam . Analysis of Monkeypox viral infection with human to animal transmission via a fractional and Fractal-fractional operators with power law kernel. Mathematical Biosciences and Engineering, 2023, 20(4): 6666-6690. doi: 10.3934/mbe.2023287 |
[4] | Jiying Ma, Shasha Ma . Dynamics of a stochastic hepatitis B virus transmission model with media coverage and a case study of China. Mathematical Biosciences and Engineering, 2023, 20(2): 3070-3098. doi: 10.3934/mbe.2023145 |
[5] | Pensiri Yosyingyong, Ratchada Viriyapong . Global dynamics of multiple delays within-host model for a hepatitis B virus infection of hepatocytes with immune response and drug therapy. Mathematical Biosciences and Engineering, 2023, 20(4): 7349-7386. doi: 10.3934/mbe.2023319 |
[6] | Tingting Xue, Long Zhang, Xiaolin Fan . Dynamic modeling and analysis of Hepatitis B epidemic with general incidence. Mathematical Biosciences and Engineering, 2023, 20(6): 10883-10908. doi: 10.3934/mbe.2023483 |
[7] | Noura Laksaci, Ahmed Boudaoui, Seham Mahyoub Al-Mekhlafi, Abdon Atangana . Mathematical analysis and numerical simulation for fractal-fractional cancer model. Mathematical Biosciences and Engineering, 2023, 20(10): 18083-18103. doi: 10.3934/mbe.2023803 |
[8] | Yiping Tan, Yongli Cai, Zhihang Peng, Kaifa Wang, Ruoxia Yao, Weiming Wang . Dynamics of a stochastic HBV infection model with drug therapy and immune response. Mathematical Biosciences and Engineering, 2022, 19(8): 7570-7585. doi: 10.3934/mbe.2022356 |
[9] | Jutarat Kongson, Chatthai Thaiprayoon, Apichat Neamvonk, Jehad Alzabut, Weerawat Sudsutad . Investigation of fractal-fractional HIV infection by evaluating the drug therapy effect in the Atangana-Baleanu sense. Mathematical Biosciences and Engineering, 2022, 19(11): 10762-10808. doi: 10.3934/mbe.2022504 |
[10] | Dong-Me Li, Bing Chai, Qi Wang . A model of hepatitis B virus with random interference infection rate. Mathematical Biosciences and Engineering, 2021, 18(6): 8257-8297. doi: 10.3934/mbe.2021410 |
This paper mainly studies the dynamical behavior of a stochastic COVID-19 model. First, the stochastic COVID-19 model is built based on random perturbations, secondary vaccination and bilinear incidence. Second, in the proposed model, we prove the existence and uniqueness of the global positive solution using random Lyapunov function theory, and the sufficient conditions for disease extinction are obtained. It is analyzed that secondary vaccination can effectively control the spread of COVID-19 and the intensity of the random disturbance can promote the extinction of the infected population. Finally, the theoretical results are verified by numerical simulations.
Hepatitis B virus causes hepatitis B (HB), an extremely fatal liver disease, and is responsible for more than 2 billion chronic infections that have been discovered globally [1,2]. It is a significant issue for wellbeing promotion. It can lead to prolonged liver damage, persistent inflammation, and a significant chance of fatality through hepatocellular carcinoma and encephalopathy [3]. Hepatitis B infestations can only happen if the pathogen can get into the circulatory system and affect the liver (see Figure 1). Once inside the liver, the infection multiplies and emits a significant quantity of fresh pathogens into the interstitial fluid [4,5].
However, there are two potential stages of this infectious disease: acute and chronic. HB virus that is severe seems to last less than 6 months, typically. If the illness is severe, the defensive mechanism is expected to eradicate the organism of the pathogen, and the patient should fully recover in the next few weeks. The majority of individuals who develop HB have an active virus. The incubation period for persistent HB is 12 weeks or more. Most newborns who contract HBV during infancy, as well as several children between the ages of one and six, develop a degenerative disease. Individuals having persistent HBV disease constitute a sizable 2/3 of chronically transmitted individuals. Despite carrying and spreading germs, these individuals do not exhibit any indications of illness [6]. The surviving one-third experienced acute hepatitis, a liver condition that could potentially be incredibly dangerous (see Figure 2). Over 2.4 billion individuals worldwide suffer from recurrent liver problems. HB's severe or long-term effects cause over 600,000 deaths a year [6]. Cytotoxic T lymphocytes (CTLs) can deliberately threaten contaminated hepatocellular through severe HBV infections and contribute to the pathophysiology of liver failure by coordinating various immunological mechanisms; see [6].
It is crucial to take into account the impact of temporal constraints on the HBV pathway because intercellular propagation and disease penetration are processes that require to be accomplished within a certain environment [7,8]. Additionally, the criteria for development and contact are based on the disease's kind and phase, the defensive system's health, and the milieu of the organism wherein the association works [9]. The participant's general lack of well-being affects the environment of the body. One strategy for investigating the effects of internal environmental parameters on the evolution of HBV transmission could be to modify the determinism account of the bacteriophage association to incorporate the randomized pressure in both an incremental and random manner. Numerous studies, including [10,11,12], have investigated numerical simulations to look into the mechanisms of HBV spreading with sound intensity.
When trying to evaluate the analogous prediction systems, stochastic modelling of highly contagious infection agents has a vital influence and adds a sense of authenticity [13]. In general, various organisms respond in the equivalent habitat to virus-induced designs and highly infectious pathogens, and the consequences can vary. Because the environment is constantly changing, the system's attributes actually oscillate around certain optimum levels [14]. Wang et al. [15] created an insidiously contagious and randomized stochastic HIV infectious framework and studied the results of stationary transmission durability. The researchers [16] addressed how a randomized HBV candida transmission concept with a significant delay in the propagation factor causes regular eruptions and the stagnant distribution and elimination consequences. In addition, the pathogens will be wiped out if the stochastic procreative quantity is below one, and if the stochastic procreative index is significantly higher than one, the infectious disease will be stochastically persistent with a perturbation theory model generated. According to Sun et al. [17], the presence of a solution for the stochastic highly contagious pathogenic framework of CTL feedback and decentralized delay was investigated. Rihan and Alsakaji [18] presented an investigation of an HBV infection framework, including a delayed immune reaction. For further details on epidemic systems; see [19,20,21] and the references cited therein.
Due to their involvement in assisting representatives in exploring the concealed characteristics of the complexities of interactive structures in rheological, permeation, machine design, electromagnetic fields, control, remote sensing, and thermodynamics, implementations of deterministic fractional differential equations (DEs) and fractional stochastic DEs motivated by Brownain motion (BM) have historically attracted the majority of attention in potential implementation. The basic differential and integral operators are unable to capture imperfections, but both fractional derivative/integral operators have been recognized as powerful computing tools [22,23,24]. Furthermore, when simulating physical and experimental events, F-F formulations exhibit diverse and distinct types of variability [25,26,27]. I think it seems that there are undoubtedly many real-world problems that neither fractal nor fractional interpretations are able to accurately reproduce on an individualized dimension. Researchers realized that novel mathematical procedures were required to replicate extremely complicated formations. Despite the assertion that there is hardly anything novel or transformative, it is difficult to believe that the combination of two existing concepts may produce a groundbreaking procedure. For added complexity, a unique differential formula was first implemented in [28]. This logical statement might be understood as the result of the accumulation of the fractal differentiation of a fractional derivative of a specific model. Evidently, there are three possible readings; it all depends on the kernels [29,30,31,32]. The concept was contested and applied to other challenges such as chaotic outgrowths, outbreaks, and diffusion, among others ([33,34,35,36]), and the overwhelming majority of publications provided extremely impressive predicted findings.
In fact, random events are prevalent worldwide. Systems frequently experience random disturbances. Various studies have been conducted on stochastic dynamics; for example, a wide range of scientific theories, including meteorology, accounting, biology, and telecommunication systems, frequently exhibit randomized fluctuations with long-term dependency. In order to analyze fractional stochastic processes, fractional BM employing the Hurst index H(1/2,1) has been proposed as an alternative to classical BM [37,38]. Kerboua et al. [39] investigated the SFDEs with perturbed regulatory frameworks involving fractional BM. Pei and Xu [40] investigated the non-Lipschitz SDEs driven by fractional BM. In 2021, authors [41] presented a novel notion for analyzing and predicting the transmission of COVID-19 throughout Africa and Europe using stochastic and deterministic methods. Alkahtani and Koca [42] contemplated the fractional stochastic SIR system within the fractional calculus technique. Rashid et al. [43] expounded the stochastic F-F tuberculosis model via a nonsingular kernel with random densities.
In the research analysis, we examine the behaviour of fractional stochastic delay DEs of the HBV system involving cell-to-cell propagation and CTLs immunological responsiveness via the fractal-fractional operator based on the exponential decay kernel with random densities, which is inspired by the aforementioned clinical and mathematical concerns. This biological model governed by fractional BM has not yet been demonstrated in the mainstream. As a result of this reality and in an effort to fill this discrepancy, we commence scientific work on one of these formulae in this article. We include the impact of several time delays (TD) and randomness inside a recipient to furnish a highly authentic scenario for the virus's design phase. In the meantime, existence-uniqueness, stochastic basic reproductive number, and the local stability of disease steady states are investigated in the stochastic context. Numerical results are presented by employing the revolutionary technique proposed by Atangana and Araz [41] in the F-F derivative sense. Graphical illustrations are presented with low random densities, incorporating the fractal-dimension and fractional-order. In a nutshell, we presented the simulation findings with and without control.
Before advancing on to the formal description, it is imperative to study certain fundamental F-F operator concepts. Take into account the parameters provided in [28] as well as the functional w(t1), which is continuous and fractal differentiable on [c,d] with fractal-dimension ϖ and fractional-order δ.
Definition 2.1. ([28]) The F-F operator of w(t1) involving the index kernel in terms of Riemann–Liouville (RL) can be presented as follows for δ∈[0,1]:
FFPDδ,ϖ0,t1(w(t1))=1Γ(s−δ)ddtϖ1t1∫0(t1−u)s−δ−1w(u)du, | (2.1) |
where dw(u)duϖ=limt1↦uw(t1)−w(u)tϖ1−uϖ and s−1<δ,ϖ≤s∈N.
Definition 2.2. ([28]) The F-F operator of w(t1) involving the exponential function kernel in terms of RL can be described as follows for δ∈[0,1]:
FFEDδ,ϖ0,t1(w(t1))=M(δ)1−δddtϖ1t1∫0exp(−δ1−δ(t1−u))w(u)du, | (2.2) |
such that M(0)=M(1)=1 having δ>0,ϖ≤s∈N.
Definition 2.3. ([28]) The corresponding F-F integral operator of (2.1) is stated as:
FFPJδ0,t1(w(t1))=ϖΓ(δ)t1∫0(t1−u)δ−1uϖ−1w(u)du. | (2.3) |
Definition 2.4. ([28]) The corresponding F-F integral operator of (2.2) is stated as:
FFEJδ0,t1(w(t1))=δϖM(δ)t1∫0uϖ−1w(u)du+ϖ(1−δ)tϖ−11w(t1)M(δ). | (2.4) |
In this study, we reveal a revolutionary system of stochastic delay DEs for Hepatitis B virus replication in a single recipient, despite the fact that the intracellular stage of over expression is not entirely appreciated. We surmise that throughout an HBV infestation, balanced (unexposed) metabolic enzymes can become infectious both by interaction with contaminated hepatocytes and by freshly generated complimentary pathogens. We furthermore suppose that the challenge-contaminated lymphocytes can be especially attacked by the cytotoxic T lymphocytes (CTLs). With the infiltration of a membrane and the ejection of retroviruses and the creation of viruses, there is still an unavoidably intracellular TD (significant-delay). Additionally, TD is necessary to reflect the gestation, which is the duration needed for the creation of fresh pathogens. Furthermore, we offer a delay differential framework to integrate the CTL community alongside infections predicated on the underlying framework of Nowak et al. [44]. A model is used to evaluate the structure
{˙˜H(t1)=λ−ϕ1˜H(t1)−γ1˜H(t1)˜V(t1)−γ2˜H(t1)˜I(t1),˙˜I(t1)=γ1˜H(t1−ζ1)˜V(t1−ζ1)+γ2˜H(t1−ζ1)˜I(t1−ζ1)−γ3˜I(t1)˜D(t1)−ϕ2˜I(t1),˙˜V(t1)=ω˜I(t1−ζ2)−ϕ3˜V(t1),˙˜D(t1)=ϑ−ϕ4˜D(t1)+γ4˜I(t1)˜D(t1), | (3.1) |
where ˜H(t1),˜I(t1),˜V(t1),˜D(t1), respectively, indicate the hepatocytes that are pure and productive of pathogens, infectious hepatocellular, HB infection pathogens as well as the CTLs. From the first component of the following formula, TD containing ζ1 is applied to estimate the length of period it takes from the first infections of a tissue and the generation of additional vesicles. The other component also incorporates the response time vital for proper hepatocytes to be invaded by malignant hepatocytes interactions before becoming contaminated hepatocellular, whilst ζ2 denotes the time that is required for recently generated particulates to develop before becoming contagious components. Either uncontrolled pathogens attack normal tissue at a pace of γ1˜H˜V (disease transmission mechanism), or viral proteins communicate directly normal tissues at a speed of γ2˜H˜I (cell-to-cell spread mechanism). As a result, the expression "γ1˜H˜V+γ2˜H˜I" denotes the overall disease incidence of susceptible organisms. CTLs generate at a consistent rate of η2 from the hypothalamus and at a pace of γ4˜I˜D as a consequence of stimulating invading pathogens, and they remove invading pathogens at a rate of γ3˜I˜D, where ω is the rate at which viral proteins producing free radicals infections (see Figure 3).
In addition, the complexities of HBV transmission may be impacted by unpredictable perturbations in the mechanism of transmission within the recipient, including fluctuations in climate, emotion, and other endogenous rhythms. Because of this, many researchers have included randomization to determinism in studies of biologic processes to illustrate the influence of environmental heterogeneity, as seen in [44,45].
By incorporating nonlinear disturbance on the spontaneous mortality rate using white noise into every other component of the scheme, we capture the influence of randomness in the host for a more reasonable position of the virus's progression (3.2). Although the HBV infection model's characteristics are not known in advance, the region to which they correspond can indeed be easily identified. So, we suggest a delayed probabilistic approach of the following:
{d˜H(t1)=(λ−ϕ1˜H(t1)−γ1˜H(t1)˜V(t1)−γ2˜H(t1)˜I(t1))dt1+σ1˜HB1(t1),d˜I(t1)=(γ1˜H(t1−ζ1)˜V(t1−ζ1)+γ2˜H(t1−ζ1)˜I(t1−ζ1)−γ3˜I(t1)˜D(t1)−ϕ3˜I(t1))dt1+σ2˜IB2(t1),d˜V(t1)=(ω˜I(t1−ζ2)−ϕ3˜V(t1))dt1+σ3˜VB3(t1),d˜D(t1)=(ϑ−ϕ4˜D(t1)+γ4˜I(t1)˜D(t1))dt1+σ4˜DB4(t1), | (3.2) |
depending on the ICs ˜H(δ)=μ1(δ),˜I(δ)=μ2(δ),˜V(δ)=μ3(δ),˜D(δ)=μ4(δ).
Also, δ∈[−ζ,0],ζ=max{ζ1,ζ2},μκ(δ)∈C,κ=1,...,4. C([−ζ,0],R4+) is the collection of Lebesgue integrable functions in this case including Bκ,κ=1,...,4 is a real-valued standard BM specified on a complete probability space (ω,A,P) meeting the basic requirements [46] and σκ,κ=1,...,4 denote the concentrations of the white noise.
Symbols | Explanation |
λ | Rate of viral hepatocellular development via bone marrow and various tissues |
ϑ | Rate at which CTLs are produced in the thymus |
ϕ1 | Percentage of uninfectious hepatocytes that naturally die |
ϕ2 | Percentage of infectious hepatocytes that naturally die |
ϕ3 | Frequency of harmless pathogens dying |
ϕ4 | Fatality rate of CTLs |
γ1 | Successful viral interaction incidence with healthy hepatocytes |
γ2 | Efficient proportion of interaction between healthy and diseased hepatocytes |
γ3 | CTLs' efficiency at eradicating infectious hepatocytes |
γ4 | CTL development speed as a result of contaminated cells' activation |
ω | Speed of spontaneous viral activity in affected tissues |
In previous decades, the idea of reproduction has been extensively used in epidemiological modelling since it has been recognized as a valuable mathematical tool for evaluating reproduction in a specific illness. According to the concept proposed by Atangana [48], one will identify two components F and ˜V, then
(F˜V−λ˜I)=0 |
will be analyzed to generate reproductive number [49]. The component F is particularly intriguing because it is derived from the nonlinear part of the infected classes.
∂∂˜˜I(˜IN)=[N−˜I]N2 |
and
∂2∂˜I2((N−˜I)N2)=−2[N−˜I]N3=−2(˜H+˜V+˜D)(˜H+˜I+˜V+˜D)3. |
At disease free equilibrium E0=(λϕ1,0,0,ϑϕ4), we have
∂2∂˜I2((N−˜I)N2)=−2(˜H0+˜D0)(˜H0+˜D0)3. |
Therefore, we have
FA=[−2(γ1˜H0+˜D0)(˜H0+˜D0)30]=[−2γ1(λϕ1+ϑϕ4)(λϕ1+ϑϕ4)30]=[−2γ1(ϕ1ϕ4)2(λϕ4+ϑϕ1)20] |
Then,
det(FA˜V−1−λ˜I)=0 |
gives
A=−2γ1ϕ21ϕ34(λϕ4+ϑϕ1)2(γ3ϑ+ϕ2ϕ4)<0. |
Also, A indicates that the expansion will not repeat and will consequently have a single magnitude and wipe out. A>0 indicates that there is sufficient intensity to initiate the regeneration phase, implying that the dispersion will have more than one cycle. Consequently, researchers will supply a strong insight of the aforesaid number.
In this part, we outline a few prerequisites that will ensure a non-negative solution of the stochastic delay DEs scheme presented in (3.2). This is feasible because a favourable result will exist if the system's coefficients satisfy the growth and Lipschitz assumptions.
Theorem 4.1. Suppose there is a system (3.2) (˜H(t1),˜I(t1),˜V(t1),˜D(t1)) with ICs having t1≥−ζ and the solution will stay in R4+, almost probably.
Proof. By means of the system (3.2), satisfies the Lipschitz continuous, there exists a peculiar solution (˜H(t1),˜I(t1),˜V(t1),˜D(t1)) defined on [−ζ,ζe), where ζe signifies the explosion time. In order to demonstrate the solution, we need to to illustrate that ζe=∞. Assume that Λ0>0 be a large enough, thus, we have (˜H(t1),˜I(t1),˜V(t1),˜D(t1))={(μ1(t1),μ2(t1),μ3(t1),μ4(t1)):t1∈(−ζ,0)}∈C([−ζ,0];R4+) contained in [1Λ0,Λ0]. Introducing the stopping time, so for every Λ≥Λ0, we have
ζΛ:=inf{t1∈[−ζ,ζe):min{˜H(t1),˜I(t1),˜V(t1),˜D(t1)}}≤1Λ |
or equivalently
ζΛ:=inf{t1∈[−ζ,ζe):max{˜H(t1),˜I(t1),˜V(t1),˜D(t1)}}≥Λ. |
Now letting infψ=∞ and increasing function ζΛ on Λ. Also, suppose ζ∞=limΛ↦∞ζΛ, then ζ∞≤ζe and need to prove ζ∞=∞ almost surely. We intend to find that ζe=∞ almost surely. If this claim is factually inaccurate, then ∃ some constants T>0 and ϵ∈(0,1) such that P{ζ∞≤T}>ϵ. Thus, for an integer Λ1≥Λ0 such that P{ζΛ≤T}>ϵ,∀Λ≥Λ1. Introducing a mapping U1:R4+↦R+ as:
U1(˜H(t1),˜I(t1),˜V(t1),˜D(t1)):=(˜H−1−ln˜H)+(˜I−1−ln˜I)+Λ2˜V+Λ2(˜D−1−ln˜D)+t1∫t1−ζ1(γ1˜H(s1)˜V(s1)+γ2˜H(s1)˜I(s1))ds1+ωΛ2t1∫t1−ζ1˜I(s1)ds1, |
where Λ2 refer to be a non-negative constant to be determined. In view of the Itô's formula, we have
dU1(˜H(t1),˜I(t1),˜V(t1),˜D(t1))=(1−1˜H)d˜H+(1−1˜I)d˜I+Λ2(1−1˜D)d˜D+Λ2˜V+121˜H2(d˜H)2+121˜I2(d˜I)2+Λ2121˜D2(d˜D)2+(γ1˜H˜V−γ1˜H(t1−ζ1)˜V(t1−ζ1)+γ2˜H˜I−γ2˜H(t1−ζ1)˜I(t1−ζ1)+ωΛ2˜I−ωΛ2˜I(t1−ζ1))=(˜H−1)σ1˜HdB1+(˜I−1)σ2˜IdB2+Λ2(˜D−1)σ4˜DdB4+Λ2σ3˜VdB3+{(λ−ϕ1˜H−λ˜H+ϕ1+γ1˜V+γ2˜I+12σ21)+(ϕ2+γ3˜D−γ1˜H(t1−ζ1)˜V(t1−ζ1)˜I−γ2˜H(t1−ζ1)˜I(t1−ζ1)˜I+12σ22)+Λ2(ω˜I−ϕ3˜V)+Λ2(ϑ−ϕ4˜D+γ4˜I˜D−ϑ˜D+ϕ4−γ4˜I+12σ24)}dt1. |
Simple computations yield
dU1(t1)=HU1dt1−{σ1dB1(t1)+σ2dB2(t1)+σ3dB3(t1)+σ4dB4(t1)}, |
where HU1=(λ−ϕ1˜H−λ˜H+ϕ1+γ1˜V+γ2˜I+12σ21)+(ϕ2+γ3˜D−γ1˜H(t1−ζ1)˜V(t1−ζ1)˜I−γ2˜H(t1−ζ1)˜I(t1−ζ1)˜I+12σ22)+Λ2(ω˜I−ϕ3˜V)+Λ2(ϑ−ϕ4˜D+γ4˜I˜D−ϑ˜D+ϕ4−γ4˜I+12σ24)=C.
Thus, we have
dU1(t1)≤Cdt1−{σ1dB1(t1)+σ2dB2(t1)+Λ2σ3dB3(t1)+Λ2σ4dB4(t1)}. |
Performing integration from 0toζΛ∧Ψ, it can be deduced that
∫ζΛ∧Ψ0dU1(χ(t1))≤∫ζΛ∧Ψ0Cdt1−{∫ζΛ∧Ψ0σ1dB1(t1)+∫ζΛ∧Ψ0σ2dB2(t1)+Λ2∫ζΛ∧Ψ0σ3dB3(t1)+Λ2∫ζΛ∧Ψ0σ4dB4(t1)}, |
using the fact that ζΛ∧T=min{ζn,t1}. Implementing the expectation on the aforesaid variants gives
U1(χ(ζΛ∧T))≤U1(χ(0))+C∫ζΛ∧T0dt1−{∫ζΛ∧T0σ1dB1(t1)+∫ζΛ∧T0σ2dB2(t1)+Λ2∫ζΛ∧T0σ3dB3(t1)+Λ2∫ζΛ∧T0σ4dB4(t1)}. |
This implies that
EU1(χ(ζΛ∧T))≤U1(χ(0))+CE≤U1(χ(0))+CT. | (4.1) |
As U1(χ(ζΛ∧T))>0, then
EU1(χ(ζΛ∧T))=E[U1(χ(ζΛ∧T))x(ζΛ≤T)]+E[U1(χ(ζΛ∧T))x(ζΛ>⊺)]≥E[U1(χ(ζΛ∧T))x(ζΛ≤T)]. | (4.2) |
Further, for ζΛ, since certain factors of χ(ζΛ),say(˜H(ζΛ)) including 0<˜H(ζΛ)≤1Λ<1.
Thus, U1(χ(ζΛ))≥−ln(1Λ), this allow us to write U1(χ(ζΛ))=ln(˜H(ζΛ))≤ln(1Λ).
As a result, from (4.2) and the previous expression, we have
EU1(χ(ζΛ∧T))≥E[U1(χ(ζΛ∧T))x(ζΛ≤T)]≥{−ln(1Λ)}. | (4.3) |
Combining (4.1)-(4.3), we have
EU1(χ(ζΛ∧T))≥−ln(1Λ)P(ζΛ∧T). | (4.4) |
It follows that
P(ζΛ∧T)≤EU1(χ(ζΛ∧T))lnΛ≤U1(χ(0))+CTlnΛ. |
Applying limit sup Λ↦∞ on (4.4), ∀T>0, we find
P(ζΛ∧T)≤0⟹limt1↦∞P(ζΛ∧T)=0. |
This is the desired result.
The average number of subsequent viral infections that a contagious individual causes while they are still dangerous is the primary reproductive component in this scenario. We also want to show that stochastic reproduction (Rs0) is a special kind of basic reproduction number.
Initially, considering the system's (3.2) second cohort, that is
d˜I(t1)=(γ1˜H(t1−ζ1)˜V(t1−ζ1)+γ2˜H(t1−ζ1)˜I(t1−ζ1)−γ3˜I(t1)˜D(t1)−ϕ3˜I(t1))dt1+σ2˜IdB2(t1). | (4.5) |
Considering the Itô's technique for twice differentiation mapping f1(˜I)=ln(I), the Taylor series representation is
df1(t1,˜I(t1))=∂f1∂t1dt1+∂f1∂˜Id˜I+12∂2f1∂˜I2(d˜I)2+∂2f1∂˜I∂t1dt1d˜I+12∂2f1∂t21(dt1)2. |
This implies that
df1(t1,˜I(t1))=1˜I(t1){(γ1˜H(t1−ζ1)˜V(t1−ζ1)+γ2˜H(t1−ζ1)˜I(t1−ζ1)−γ3˜I(t1)˜D(t1)−ϕ3˜I(t1))dt1+σ2˜IdB2(t1)}−12˜I2(t1){(γ1˜H(t1−ζ1)˜V(t1−ζ1)+γ2˜H(t1−ζ1)˜I(t1−ζ1)−γ3˜I(t1)˜D(t1)−ϕ3˜I(t1))dt1+σ2˜IdB2(t1)}2. |
It follows that
df1(t1,˜I(t1))={(γ1˜H(t1−ζ1)˜V(t1−ζ1)˜I(t1)+γ2˜H(t1−ζ1)−γ3˜D(t1)−ϕ3)dt1+σ2dB2(t1)}−12˜I2(t1){A21(dt1)2+2A1A2dt1dB2(t1)+A22(dB2(t1))2}, |
where A1=γ1˜H(t1−ζ1)˜V(t1−ζ1)+γ2˜H(t1−ζ1)˜I(t1−ζ1)−γ3˜I(t1)˜D(t1)−ϕ3˜I(t1) and A2=σ2˜I, then (4.6) can be written as
df1(t1,˜I(t1))={(γ1˜H(t1−ζ1)˜V(t1−ζ1)˜I(t1)+γ2˜H(t1−ζ1)−γ3˜D(t1)−ϕ3)dt1+σ2dB2(t1)}−12˜I2(t1){A22(dB2(t1))2}={(γ1˜H(t1−ζ1)˜V(t1−ζ1)˜I(t1)+γ2˜H(t1−ζ1)−γ3˜D(t1)−ϕ3)dt1+σ2dB2(t1)}−12˜I2(t1){(σ2˜I)2}dt1. | (4.6) |
As dt1↦0,(dt1)2,dt1dB2(t1)↦0 and (dB2(t1))2 can be converted to dt1 (By the variance of Wiener technique), we have
df1(t1,˜I(t1))={(γ1˜H(t1−ζ1)˜V(t1−ζ1)˜I(t1)+γ2˜H(t1−ζ1)−γ3˜D(t1)−ϕ3)dt1+σ2dB2(t1)}−12(σ2)2dt1={(γ1˜H(t1−ζ1)˜V(t1−ζ1)˜I(t1)+γ2˜H(t1−ζ1)−γ3˜D(t1)−ϕ3)dt1−12σ22}dt1+σ2dB2(t1). | (4.7) |
Taking into consideration the next generation matrices [47] are as follows
F=[γ2λϕ1γ1λϕ100]and˜V=[γ3ϑϕ4+ϕ20−ωϕ3]. |
Therefore, F and ˜V at disease-free equilibrium E0=(λϕ1,0,0,ϑϕ4), we find
˜V−1=[ϕ4γ3ϑ+ϕ2ϕ40ωϕ4ϕ3(ϕ2ϕ4+γ3ϑ)1/ϕ3]. |
Thus, the basic reproduction number is Rs0=ρ(F˜V−1) is
Rs0=λϕ4(ωγ1+ϕ3γ2)ϕ1ϕ3(ϑγ3+ϕ2ϕ4), |
which is the required stochastic fundamental reproduction number.
Theorem 4.2. For a community's infection to be eradicated, then Rs0<1.
If Rs0<1, then for any provided ICs (˜H(0),˜V(0),˜I(0),˜D(0))=(˜H0,˜V0,˜I0,R0)∈R4+. Therefore, ˜I(t1) admits limt1↦∞supln(˜I(t1))t1≤ϕ3(Rs0−1) almost surely.
Proof. Taking into consideration (4.5), we have
df1(t1,˜I(t1))={(γ1˜H˜V+γ2˜H−γ3˜D−ϕ3−12σ22)dt1+σ2dB2(t1)}. |
It follows that
dln(˜I)=(γ1˜H˜V+γ2˜H−γ3˜D−ϕ3−12σ22)dt1+σ2dB2(t1). |
After performing integration, we have
ln(˜I)=ln(˜I0)+t1∫0(γ1˜H˜V+γ2˜H−γ3˜D−ϕ3−12σ22)dt1+σ2t1∫0dB2(t1)≤ln(˜I0)+(γ2λϕ1−γ3ϑϕ4−12σ22−ϕ3)t1⏟atDFEPE0+σ2t1∫0dB2(t1)≤ln(˜I0)+(γ2λϕ1−γ3ϑϕ4−12σ22−ϕ3)t1+Υ(t1), | (4.8) |
where Υ(t1)=σ3t1∫0dB3(t1) is the martingale. therefore, by the strong principal of large values for Υ(t1), see [50], we get limt1↦∞supΥ(t1)t1=0 almost probably.
After dividing by t1 and applying limit t1↦∞, then (4.8) reduces to
limt1↦∞supln(˜I)t1≤γ2λϕ1−γ3ϑϕ4−12σ22−ϕ3=ϕ3(λϕ4(ωγ1+ϕ3γ2)ϕ1ϕ3(ϑγ3+ϕ2ϕ4)−12ϕ3σ22)=ϕ3(Rs0−1)<0. |
This indicates that Rs0<1.
Finally, Rs0 should be smaller than 1 for virus elimination in a population.
Remark 4.1. Under some settings, the solution of framework (3.2) oscillates all around endemic equilibrium of the undisturbed system (3.1) if Rs0>1. This indicates that as long as the concentrations of white noise are low enough, the sickness will endure.
In what follows, the framework is further expanded to F-F derivative operators.
Firstly, we present the Caputo-Fabrizio F-F derivative for the classical derivative formulation. As a result, the stochastic F-F framework will be quantitatively determined employing the numerical technique described previously. This type of system is presented by
{FFE0Dδ,ϖt1˜H(t1)=(λ−ϕ1˜H(t1)−γ1˜H(t1)˜V(t1)−γ1˜H(t1)˜I(t1))+σ1G1(t1,˜H)B1(t1),FFE0Dδ,ϖt1˜I(t1)=(γ1˜H(t1−ζ1)˜V(t1−ζ1)+γ2˜H(t1−ζ1)˜I(t1−ζ1)−γ3˜I(t1)˜D(t1)−ϕ3˜I(t1))+σ2G2(t1,˜I)B2(t1),FFE0Dδ,ϖt1˜V(t1)=(ω˜I(t1−ζ2)−ϕ3˜V(t1))+σ3G3(t1,˜V)B3(t1),FFE0Dδ,ϖt1˜D(t1)=(ϑ−ϕ4˜D(t1)+γ4˜I(t1)˜D(t1))+σ4G4(t1,˜D)B4(t1). | (5.1) |
For tm+1=(m+1)Δt1, then we the aforementioned system can be integrated as follows
˜Hm+1=˜H0+(1−δ)δϖM(δ)ϖt1ϖ−1m+1[{˜H∗(t1m+1,˜Hqm+1,˜Iqm+1,˜Vqm+1,˜Dqm+1)+σ1G1(t1m+1,˜Hqm+1)(B1(t1m+1)−B1(t1m))]+δϖM(δ)m∑ℓ=0[t1ℓ+1∫t1ℓϱδ−1˜H∗(ϱ,˜H,˜I,˜V,˜D)dϱ+t1ℓ+1∫t1ℓϱδ−1σ1G1(ϱ,˜H)dB1ϱ], |
˜Im+1=˜I0+(1−δ)δϖM(δ)ϖt1ϖ−1m+1[{˜I∗(t1m+1,˜Hqm+1,˜Iqm+1,˜Vqm+1,˜Dqm+1)+σ2G2(t1m+1,˜Iqm+1)(B2(t1m+1)−B2(t1m))]+δϖM(δ)m∑ℓ=0[t1ℓ+1∫t1ℓϱδ−1˜I∗(ϱ,˜H,˜I,˜V,˜D)dϱ+t1ℓ+1∫t1ℓϱδ−1σ2G2(ϱ,˜I)dB2ϱ], |
˜Vm+1=˜V0+(1−δ)δϖM(δ)ϖt1ϖ−1m+1[{˜V∗(t1m+1,˜Iqm+1,˜Vqm+1)+σ3G3(t1m+1,˜Vqm+1)(B3(t1m+1)−B3(t1m))]+δϖM(δ)m∑ℓ=0[t1ℓ+1∫t1ℓϱδ−1˜V∗(ϱ,˜I,˜V)dϱ+t1ℓ+1∫t1ℓϱδ−1σ3G3(ϱ,˜V)dB3ϱ], |
˜Dm+1=˜D0+(1−δ)δϖM(δ)ϖt1ϖ−1m+1[{˜D∗(t1m+1,˜Iqm+1,˜Dqm+1)+σ4G4(t1m+1,˜Dqm+1)(B4(t1m+1)−B4(t1m))]+δϖM(δ)m∑ℓ=0[t1ℓ+1∫t1ℓϱδ−1˜D∗(ϱ,˜I,˜D)dϱ+t1ℓ+1∫t1ℓϱδ−1σ4G4(ϱ,˜D)dB4ϱ]. |
Utilizing the fact of polynomials in the aforesaid system, we find
˜Hm+1=˜H0+(1−δ)δϖM(δ)ϖt1ϖ−1m+1[{˜H∗(t1m+1,˜Hqm+1,˜Iqm+1,˜Vqm+1,˜Dqm+1)+σ1G1(t1m+1,˜Hqm+1)(B1(t1m+1)−B1(t1m))]+δϖM(δ)m−1∑ℓ=0[{˜H∗(t1ℓ+1,˜Hℓ+1,˜Iℓ+1,˜Vℓ+1,˜Dℓ+1)hϖϖ((ℓ+1)ϖ−ℓϖ)+˜H∗(t1ℓ+1,˜Hℓ+1,˜Iℓ+1,˜Vℓ+1,˜Dℓ+1)−˜H∗(t1ℓ,˜Hℓ,˜Vℓ,˜Iℓ,˜Dℓ)h×(hϖ+1(ℓϖ(ℓ+1+ϖ)−(ℓ+1)ϖ+1)ϖ(ϖ+1))+˜H∗(t1ℓ+1,˜Hℓ+1,˜Iℓ+1,˜Vℓ+1,˜Dℓ+1)−2˜H∗(t1ℓ,˜Hℓ,˜Vℓ,˜Iℓ,˜Dℓ)+˜H∗(t1ℓ−1,˜Hℓ−1,˜Iℓ−1,˜Vℓ−1,˜Dℓ−1)2h2×(2hϖ+2((ℓ−ϖ2)(ℓ+1)ϖ+1−ℓϖ+1(ℓ+1+ϖ2))ϖ(ϖ+1)(ϖ+2))]+σ1δϖM(δ)m−1∑ℓ=0[{G1(t1ℓ+1,˜Hℓ+1)(B1(t1ℓ+1)−B1(t1ℓ))hϖϖ((ℓ+1)ϖ−ℓϖ)+{G1(t1ℓ+1,˜Hℓ+1)(B1(t1ℓ+1)−B1(t1ℓ))−G1(t1ℓ,˜Hℓ)(B1(t1ℓ)−B1(t1ℓ−1))}×(hϖ+1(ℓϖ(ℓ+1+ϖ)−(ℓ+1)ϖ+1)ϖ(ϖ+1))+{G1(t1ℓ+1,˜Hℓ+1)(B1(t1ℓ+1)−B1(t1ℓ))−2G1(t1ℓ,˜Hℓ)(B1(t1ℓ+1)−B1(t1ℓ))2h+G1(t1ℓ−1,˜Hℓ−1)(B1(t1ℓ)−B1(t1ℓ−1))2h}(2hϖ+2((ℓ−ϖ2)(ℓ+1)ϖ+1−ℓϖ+1(ℓ+1+ϖ2))ϖ(ϖ+1)(ϖ+2))], |
˜Im+1=˜I0+(1−δ)δϖM(δ)ϖt1ϖ−1m+1[{˜I∗(t1m+1,˜Hqm+1,˜Iqm+1,˜Vqm+1,˜Dqm+1)+σ2G2(t1m+1,˜Iqm+1)(B2(t1m+1)−B2(t1m))]+δϖM(δ)m−1∑ℓ=0[{˜I∗(t1ℓ+1,˜Hℓ+1,˜Iℓ+1,˜Vℓ+1,Rℓ+1)hϖϖ((ℓ+1)ϖ−ℓϖ)+˜I∗(t1ℓ+1,˜Hℓ+1,˜Iℓ+1,˜Vℓ+1,˜Dℓ+1)−˜I∗(t1ℓ,˜Hℓ,˜Iℓ,˜Vℓ,˜Dℓ)h×(hϖ+1(ℓϖ(ℓ+1+ϖ)−(ℓ+1)ϖ+1)ϖ(ϖ+1))+˜I∗(t1ℓ+1,˜Hℓ+1,˜Iℓ+1,˜Vℓ+1,˜Dℓ+1)−2˜I∗(t1ℓ,˜Hℓ,˜Iℓ,˜Vℓ,˜Dℓ)+˜I∗(t1ℓ−1,˜Hℓ−1,˜Iℓ−1,˜Vℓ−1,˜Dℓ−1)2h2×(2hϖ+2((ℓ−ϖ2)(ℓ+1)ϖ+1−ℓϖ+1(ℓ+1+ϖ2))ϖ(ϖ+1)(ϖ+2))]+σ2δϖM(δ)m−1∑ℓ=0[{G2(t1ℓ+1,˜Iℓ+1)(B2(t1ℓ+1)−B2(t1ℓ))hϖϖ((ℓ+1)ϖ−ℓϖ)+{G2(t1ℓ+1,˜Iℓ+1)(B2(t1ℓ+1)−B2(t1ℓ))−G2(t1ℓ,˜Iℓ)(B2(t1ℓ)−B2(t1ℓ−1))}×(hϖ+1(ℓϖ(ℓ+1+ϖ)−(ℓ+1)ϖ+1)ϖ(ϖ+1))+{G2(t1ℓ+1,˜Iℓ+1)(B2(t1ℓ+1)−B2(t1ℓ))−2G2(t1ℓ,˜Iℓ)(B2(t1ℓ+1)−B2(t1ℓ))2h+G2(t1ℓ−1,˜Iℓ−1)(B2(t1ℓ)−B2(t1ℓ−1))2h}(2hϖ+2((ℓ−ϖ2)(ℓ+1)ϖ+1−ℓϖ+1(ℓ+1+ϖ2))ϖ(ϖ+1)(ϖ+2))], |
˜Vm+1=˜V0+(1−δ)δϖM(δ)ϖt1ϖ−1m+1[{˜V∗(t1m+1,˜Iqm+1,˜Vqm+1)+σ2G2(t1m+1,˜Vqm+1)(B3(t1m+1)−B3(t1m))]+δϖM(δ)m−1∑ℓ=0[{˜V∗(t1ℓ+1,˜Iℓ+1,˜Vℓ+1)hϖϖ((ℓ+1)ϖ−ℓϖ)+˜V∗(t1ℓ+1,˜Iℓ+1,˜Vℓ+1)−˜V∗(t1ℓ,˜Iℓ,˜Vℓ)h×(hϖ+1(ℓϖ(ℓ+1+ϖ)−(ℓ+1)ϖ+1)ϖ(ϖ+1))+˜V∗(t1ℓ+1,˜Iℓ+1,˜Vℓ+1)−2˜V∗(t1ℓ,˜Iℓ,˜Vℓ)+˜V∗(t1ℓ−1,˜Iℓ−1,˜Vℓ−1)2h2×(2hϖ+2((ℓ−ϖ2)(ℓ+1)ϖ+1−ℓϖ+1(ℓ+1+ϖ2))ϖ(ϖ+1)(ϖ+2))]+σ2δϖM(δ)m−1∑ℓ=0[{G2(t1ℓ+1,˜Vℓ+1)(B3(t1ℓ+1)−B3(t1ℓ))hϖϖ((ℓ+1)ϖ−ℓϖ)+{G3(t1ℓ+1,˜Vℓ+1)(B3(t1ℓ+1)−B3(t1ℓ))−G3(t1ℓ,˜Vℓ)(B3(t1ℓ)−B3(t1ℓ−1))}×(hϖ+1(ℓϖ(ℓ+1+ϖ)−(ℓ+1)ϖ+1)ϖ(ϖ+1))+{G3(t1ℓ+1,˜Vℓ+1)(B3(t1ℓ+1)−B3(t1ℓ))−2G3(t1ℓ,˜Vℓ)(B3(t1ℓ+1)−B3(t1ℓ))2h+G3(t1ℓ−1,˜Vℓ−1)(B3(t1ℓ)−B3(t1ℓ−1))2h}(2hϖ+2((ℓ−ϖ2)(ℓ+1)ϖ+1−ℓϖ+1(ℓ+1+ϖ2))ϖ(ϖ+1)(ϖ+2))], |
˜Dm+1=˜D0+(1−δ)δϖM(δ)ϖt1ϖ−1m+1[{˜D∗(t1m+1,˜Iqm+1,˜Dqm+1)+σ4G4(t1m+1,˜Dqm+1)(B4(t1m+1)−B4(t1m))]+δϖM(δ)m−1∑ℓ=0[{˜D∗(t1ℓ+1,˜Iℓ+1,˜Dℓ+1)hϖϖ((ℓ+1)ϖ−ℓϖ)+˜D∗(t1ℓ+1,˜Iℓ+1,˜Dℓ+1)−˜D∗(t1ℓ,˜Iℓ,˜Dℓ)h×(hϖ+1(ℓϖ(ℓ+1+ϖ)−(ℓ+1)ϖ+1)ϖ(ϖ+1))+˜D∗(t1ℓ+1,˜Iℓ+1,˜Dℓ+1)−2˜D∗(t1ℓ,˜Iℓ,˜Dℓ)+˜D∗(t1ℓ−1,˜Iℓ−1,˜Dℓ−1)2h2×(2hϖ+2((ℓ−ϖ2)(ℓ+1)ϖ+1−ℓϖ+1(ℓ+1+ϖ2))ϖ(ϖ+1)(ϖ+2))]+σ1δϖM(δ)m−1∑ℓ=0[{G4(t1ℓ+1,˜Dℓ+1)(B4(t1ℓ+1)−B4(t1ℓ))hϖϖ((ℓ+1)ϖ−ℓϖ)+{G4(t1ℓ+1,˜Dℓ+1)(B4(t1ℓ+1)−B4(t1ℓ))−G4(t1ℓ,˜Dℓ)(B4(t1ℓ)−B4(t1ℓ−1))}×(hϖ+1(ℓϖ(ℓ+1+ϖ)−(ℓ+1)ϖ+1)ϖ(ϖ+1))+{G4(t1ℓ+1,˜Dℓ+1)(B4(t1ℓ+1)−B4(t1ℓ))−2G4(t1ℓ,˜Dℓ)(B4(t1ℓ+1)−B4(t1ℓ))2h+G4(t1ℓ−1,˜Dℓ−1)(B4(t1ℓ)−B4(t1ℓ−1))2h}(2hϖ+2((ℓ−ϖ2)(ℓ+1)ϖ+1−ℓϖ+1(ℓ+1+ϖ2))ϖ(ϖ+1)(ϖ+2))]. |
Furthermore, we can write
˜Hm+1=˜H0+(1−δ)δϖM(δ)ϖt1ϖ−1m+1[{˜H∗(t1m+1,˜Hqm+1,˜Iqm+1,˜Vqm+1,˜Dqm+1)+σ1G1(t1m+1,˜Hqm+1)(B1(t1m+1)−B1(t1m))]+δϖM(δ)m−1∑ℓ=0[{˜H∗(t1ℓ+1,˜Hℓ+1,˜Iℓ+1,˜Vℓ+1,˜Dℓ+1)hϖϖ((ℓ+1)ϖ−ℓϖ)+˜H∗(t1ℓ+1,˜Hℓ+1,˜Iℓ+1,˜Vℓ+1,˜Dℓ+1)−˜H∗(t1ℓ,˜Hℓ,˜Iℓ,˜Vℓ,˜Dℓ)h×(hϖ+1(ℓϖ(ℓ+1+ϖ)−(ℓ+1)ϖ+1)ϖ(ϖ+1))+˜H∗(t1ℓ+1,˜Hℓ+1,˜Iℓ+1,˜Vℓ+1,˜Dℓ+1)−2˜H∗(t1ℓ,˜Hℓ,˜Iℓ,˜Vℓ,˜Dℓ)+˜H∗(t1ℓ−1,˜Hℓ−1,˜Iℓ−1,˜Vℓ−1,˜Dℓ−1)2h2×(2hϖ+2((ℓ−ϖ2)(ℓ+1)ϖ+1−ℓϖ+1(ℓ+1+ϖ2))ϖ(ϖ+1)(ϖ+2))]+σ1δϖM(δ)m−1∑ℓ=0[{G1(t1ℓ+1,˜Hℓ+1)(B1(t1ℓ+1)−B1(t1ℓ))hϖϖ((ℓ+1)ϖ−ℓϖ)+{G1(t1ℓ+1,˜Hℓ+1)(B1(t1ℓ+1)−B1(t1ℓ))−G1(t1ℓ,˜Hℓ)(B1(t1ℓ)−B1(t1ℓ−1))}×(hϖ+1(ℓϖ(ℓ+1+ϖ)−(ℓ+1)ϖ+1)ϖ(ϖ+1))+{G1(t1ℓ+1,˜Hℓ+1)(B1(t1ℓ+1)−B1(t1ℓ))−2G1(t1ℓ,˜Hℓ)(B1(t1ℓ+1)−B1(t1ℓ))2h+G1(t1ℓ−1,˜Hℓ−1)(B1(t1ℓ)−B1(t1ℓ−1))2h}(2hϖ+2((ℓ−ϖ2)(ℓ+1)ϖ+1−ℓϖ+1(ℓ+1+ϖ2))ϖ(ϖ+1)(ϖ+2))] |
+δϖM(δ)[{{˜H∗(t1m+1,˜Hqm+1,˜Iqm+1,˜Vqm+1,˜Dqm+1)+G1(t1m+1,˜Hqm+1)(B1(t1m+1)−B1(t1m))}hϖϖ((m+1)ϖ−mϖ)+˜H∗(t1m+1,˜Hm+1,˜Im+1,˜Vm+1,˜Dm+1)−˜H∗(t1m,˜Hm,˜Im,˜Vm,˜Dm)h+{G1(t1m+1,˜Hm+1)(B1(t1m+1)−B1(t1m))−G1(t1m,˜Hm)(B1(t1m)−B1(t1m−1))}×(hϖ+1(ℓϖ(ℓ+1+ϖ)−(ℓ+1)ϖ+1)ϖ(ϖ+1))+˜H∗(t1m+1,˜Hm+1,˜Im+1,˜Vm+1,˜Dm+1)−2˜H∗(t1m,˜Hm,˜Im,˜Vm,˜Dm)+˜H∗(t1m−1,˜Hm−1,˜Im−1,˜Vm−1,˜Dm−1)2h2+{G1(t1m+1,˜Hm+1)(B1(t1m+1)−B1(t1m))−2G1(t1m,˜Hm)(B1(t1m)−B1(t1m−1))2h+G1(t1m−1,˜Hm−1)(B1(t1m−1)−B1(t1m−2))2h}(2hϖ+2((ℓ−ϖ2)(ℓ+1)ϖ+1−ℓϖ+1(ℓ+1+ϖ2))ϖ(ϖ+1)(ϖ+2))], |
˜Im+1=˜I0+(1−δ)δϖM(δ)ϖt1ϖ−1m+1[{˜I∗(t1m+1,˜Hqm+1,˜Iqm+1,˜Vqm+1,˜Dqm+1)+σ2G2(t1m+1,˜Iqm+1)(B3(t1m+1)−B2(t1m))]+δϖM(δ)m−1∑ℓ=0[{˜I∗(t1ℓ+1,˜Hℓ+1,˜Iℓ+1,˜Vℓ+1,˜Dℓ+1)hϖϖ((ℓ+1)ϖ−ℓϖ)+˜I∗(t1ℓ+1,˜Hℓ+1,˜Iℓ+1,˜Vℓ+1,˜Dℓ+1)−˜I∗(t1ℓ,˜Hℓ,˜Iℓ,˜Vℓ,˜Dℓ)h×(hϖ+1(ℓϖ(ℓ+1+ϖ)−(ℓ+1)ϖ+1)ϖ(ϖ+1))+˜I∗(t1ℓ+1,˜Hℓ+1,˜Iℓ+1,˜Vℓ+1,˜Dℓ+1)−2˜V∗(t1ℓ,˜Hℓ,˜Iℓ,˜Vℓ,˜Dℓ)+˜V∗(t1ℓ−1,˜Hℓ−1,˜Iℓ−1,˜Vℓ−1,˜Dℓ−1)2h2×(2hϖ+2((ℓ−ϖ2)(ℓ+1)ϖ+1−ℓϖ+1(ℓ+1+ϖ2))ϖ(ϖ+1)(ϖ+2))]+σ3δϖM(δ)m−1∑ℓ=0[{G2(t1ℓ+1,˜Iℓ+1)(B2(t1ℓ+1)−B2(t1ℓ))hϖϖ((ℓ+1)ϖ−ℓϖ)+{G2(t1ℓ+1,˜Iℓ+1)(B2(t1ℓ+1)−B2(t1ℓ))−G2(t1ℓ,˜Iℓ)(B2(t1ℓ)−B2(t1ℓ−1))}×(hϖ+1(ℓϖ(ℓ+1+ϖ)−(ℓ+1)ϖ+1)ϖ(ϖ+1))+{G2(t1ℓ+1,˜Iℓ+1)(B2(t1ℓ+1)−B2(t1ℓ))−2G2(t1ℓ,˜Iℓ)(B2(t1ℓ+1)−B2(t1ℓ))2h+G2(t1ℓ−1,˜Iℓ−1)(B2(t1ℓ)−B2(t1ℓ−1))2h}(2hϖ+2((ℓ−ϖ2)(ℓ+1)ϖ+1−ℓϖ+1(ℓ+1+ϖ2))ϖ(ϖ+1)(ϖ+2))] |
+δϖM(δ)[{{˜I∗(t1m+1,˜Hqm+1,˜Iqm+1,˜Vqm+1,˜Dqm+1)+G2(t1m+1,˜Iqm+1)(B2(t1m+1)−B2(t1m))}hϖϖ((m+1)ϖ−mϖ)+˜I∗(t1m+1,˜Hm+1,˜Im+1,˜Vm+1,˜Dm+1)−˜I∗(t1m,˜Hm,˜Im,˜Vm,dm)h+{G2(t1m+1,˜Im+1)(B2(t1m+1)−B2(t1m))−G2(t1m,˜Im)(B2(t1m)−B2(t1m−1))}×(hϖ+1(ℓϖ(ℓ+1+ϖ)−(ℓ+1)ϖ+1)ϖ(ϖ+1))+˜I∗(t1m+1,˜Hm+1,˜Im+1,˜Vm+1,˜Dm+1)−2˜I∗(t1m,˜Hm,˜Im,˜Vm,˜Dm)+˜I∗(t1m−1,˜Hm−1,˜Im−1,˜Vm−1,˜Dm−1)2h2+{G2(t1m+1,˜Im+1)(B2(t1m+1)−B2(t1m))−2G2(t1m,˜Im)(B2(t1m)−B2(t1m−1))2h+G2(t1m−1,˜Im−1)(B2(t1m−1)−B2(t1m−2))2h}(2hϖ+2((ℓ−ϖ2)(ℓ+1)ϖ+1−ℓϖ+1(ℓ+1+ϖ2))ϖ(ϖ+1)(ϖ+2))], |
˜Vm+1=˜V0+(1−δ)δϖM(δ)ϖt1ϖ−1m+1[{˜V∗(t1m+1,˜Iqm+1,˜Vqm+1)+σ3G3(t1m+1,˜Vqm+1)(B3(t1m+1)−B3(t1m))]+δϖM(δ)m−1∑ℓ=0[{˜V∗(t1ℓ+1,˜Iℓ+1,˜Vℓ+1)hϖϖ((ℓ+1)ϖ−ℓϖ)+˜V∗(t1ℓ+1,˜Iℓ+1,˜Vℓ+1)−˜V∗(t1ℓ,˜Iℓ,˜Vℓ)h×(hϖ+1(ℓϖ(ℓ+1+ϖ)−(ℓ+1)ϖ+1)ϖ(ϖ+1))+˜V∗(t1ℓ+1,˜Iℓ+1,˜Vℓ+1)−2˜V∗(t1ℓ,˜Iℓ,˜Vℓ)+˜V∗(t1ℓ−1,˜Iℓ−1,˜Vℓ−1)2h2×(2hϖ+2((ℓ−ϖ2)(ℓ+1)ϖ+1−ℓϖ+1(ℓ+1+ϖ2))ϖ(ϖ+1)(ϖ+2))]+σ1δϖM(δ)m−1∑ℓ=0[{G3(t1ℓ+1,˜Vℓ+1)(B3(t1ℓ+1)−B3(t1ℓ))hϖϖ((ℓ+1)ϖ−ℓϖ)+{G3(t1ℓ+1,˜Vℓ+1)(B1(t1ℓ+1)−B3(t1ℓ))−G3(t1ℓ,˜Vℓ)(B3(t1ℓ)−B3(t1ℓ−1))}×(hϖ+1(ℓϖ(ℓ+1+ϖ)−(ℓ+1)ϖ+1)ϖ(ϖ+1))+{G3(t1ℓ+1,˜Vℓ+1)(B3(t1ℓ+1)−B3(t1ℓ))−2G3(t1ℓ,˜Vℓ)(B3(t1ℓ+1)−B3(t1ℓ))2h+G3(t1ℓ−1,˜Vℓ−1)(B3(t1ℓ)−B3(t1ℓ−1))2h}(2hϖ+2((ℓ−ϖ2)(ℓ+1)ϖ+1−ℓϖ+1(ℓ+1+ϖ2))ϖ(ϖ+1)(ϖ+2))] |
+δϖM(δ)[{{˜V∗(t1m+1,˜Iqm+1,˜Vqm+1)+G3(t1m+1,˜Vqm+1)(B3(t1m+1)−B3(t1m))}hϖϖ((m+1)ϖ−mϖ)+˜V∗(t1m+1,˜Im+1,˜Vm+1)−˜V∗(t1m,˜Im,˜Vm)h+{G3(t1m+1,˜Vm+1)(B3(t1m+1)−B3(t1m))−G3(t1m,˜Vm)(B3(t1m)−B3(t1m−1))}×(hϖ+1(ℓϖ(ℓ+1+ϖ)−(ℓ+1)ϖ+1)ϖ(ϖ+1))+˜V∗(t1m+1,˜Im+1,˜Vm+1)−2˜V∗(t1m,˜Im,˜Vm)+˜V∗(t1m−1,˜Im−1,˜Vm−1)2h2+{G3(t1m+1,˜Vm+1)(B3(t1m+1)−B3(t1m))−2G3(t1m,˜Vm)(B3(t1m)−B3(t1m−1))2h+G3(t1m−1,˜Vm−1)(B3(t1m−1)−B3(t1m−2))2h}(2hϖ+2((ℓ−ϖ2)(ℓ+1)ϖ+1−ℓϖ+1(ℓ+1+ϖ2))ϖ(ϖ+1)(ϖ+2))], |
\begin{array}{l} \tilde{{\bf D}}_{\mathfrak{m}+1} = \tilde{{\bf D}}_{0}+\frac{(1-\delta)\delta\varpi}{\mathbb{M}(\delta)}\varpi{{\bf t}_{1}}_{\mathfrak{m}+1}^{\varpi-1}\left[ \begin{cases}\tilde{{\bf D}}^{\ast}\big({{\bf t}_{1}}_{\mathfrak{m}+1}, \tilde{{\bf I}}_{\mathfrak{m}+1}^{{\bf q}}, \tilde{{\bf D}}_{\mathfrak{m}+1}^{{\bf q}}\big)\cr+\sigma_{4}\mathbb{G}_{4}\big({{\bf t}_{1}}_{\mathfrak{m}+1}, \tilde{{\bf D}}_{\mathfrak{m}+1}^{{\bf q}}\big)\big(\mathbb{B}_{4}({{\bf t}_{1}}_{\mathfrak{m}+1})-\mathbb{B}_{4}({{\bf t}_{1}}_{\mathfrak{m}})\big)\end{cases}\right]\nonumber\\ + \frac{\delta\varpi}{\mathbb{M}(\delta)}\sum\limits_{\ell = 0}^{\mathfrak{m}-1}\left[\begin{cases}\tilde{{\bf D}}^{\ast}\big({{\bf t}_{1}}_{\ell+1}, \tilde{{\bf I}}_{\ell+1}, \tilde{{\bf D}}_{\ell+1}\big)\frac{{\bf h}^{\varpi}}{\varpi}\big((\ell+1)^{\varpi}-\ell^{\varpi}\big)\cr+\frac{\tilde{{\bf D}}^{\ast}\big({{\bf t}_{1}}_{\ell+1}, \tilde{{\bf I}}_{\ell+1}, \tilde{{\bf D}}_{\ell+1}\big)-\tilde{{\bf D}}^{\ast}\big({{\bf t}_{1}}_{\ell}, \tilde{{\bf I}}_{\ell}, \tilde{{\bf D}}_{\ell}\big)}{{\bf h}}\cr\times\bigg(\frac{{\bf h}_{\varpi+1}(\ell^{\varpi}(\ell+1+\varpi)-(\ell+1)^{\varpi+1})}{\varpi(\varpi+1)}\bigg)\cr+ \frac{\tilde{{\bf D}}^{\ast}\big({{\bf t}_{1}}_{\ell+1}, \tilde{{\bf I}}_{\ell+1}, \tilde{{\bf D}}_{\ell+1}\big)-2\tilde{{\bf D}}^{\ast}\big({{\bf t}_{1}}_{\ell}, \tilde{{\bf I}}_{\ell}, \tilde{{\bf D}}_{\ell}\big)+\tilde{{\bf D}}^{\ast}\big({{\bf t}_{1}}_{\ell-1}, \tilde{{\bf I}}_{\ell-1}, \tilde{{\bf D}}_{\ell-1}\big)}{2{\bf h}^{2}}\cr\times\bigg(\frac{2{\bf h}^{\varpi+2}\big((\ell-\frac{\varpi}{2})(\ell+1)^{\varpi+1}-\ell^{\varpi+1}(\ell+1+\frac{\varpi}{2})\big)}{\varpi(\varpi+1)(\varpi+2) }\bigg)\end{cases}\right]\nonumber\\ + \frac{\sigma_{4}\delta\varpi}{\mathbb{M}(\delta)}\sum\limits_{\ell = 0}^{\mathfrak{m}-1}\\ \left[\begin{cases}\mathbb{G}_{4}\big({{\bf t}_{1}}_{\ell+1}, \tilde{{\bf D}}_{\ell+1}\big)\big(\mathbb{B}_{4}({{\bf t}_{1}}_{\ell+1})-\mathbb{B}_{4}({{\bf t}_{1}}_{\ell})\big)\frac{{\bf h}^{\varpi}}{\varpi}\big((\ell+1)^{\varpi}-\ell^{\varpi}\big)\cr+\Big\{\mathbb{G}_{4}\big({{\bf t}_{1}}_{\ell+1}, \tilde{{\bf D}}_{\ell+1}\big)\big(\mathbb{B}_{4}({{\bf t}_{1}}_{\ell+1})-\mathbb{B}_{4}({{\bf t}_{1}}_{\ell})\big)-\mathbb{G}_{4}\big({{\bf t}_{1}}_{\ell}, \tilde{{\bf D}}_{\ell}\big)\big(\mathbb{B}_{4}({{\bf t}_{1}}_{\ell})-\mathbb{B}_{4}({{\bf t}_{1}}_{\ell-1})\big)\Big\}\cr\times\bigg(\frac{{\bf h}_{\varpi+1}(\ell^{\varpi}(\ell+1+\varpi)-(\ell+1)^{\varpi+1})}{\varpi(\varpi+1)}\bigg)\cr+ \Big\{\frac{\mathbb{G}_{4}\big({{\bf t}_{1}}_{\ell+1}, \tilde{{\bf D}}_{\ell+1}\big)\big(\mathbb{B}_{4}({{\bf t}_{1}}_{\ell+1})-\mathbb{B}_{4}({{\bf t}_{1}}_{\ell})\big)-2\mathbb{G}_{4}\big({{\bf t}_{1}}_{\ell}, \tilde{{\bf D}}_{\ell}\big)\big(\mathbb{B}_{4}({{\bf t}_{1}}_{\ell+1})-\mathbb{B}_{4}({{\bf t}_{1}}_{\ell})\big)}{2{\bf h}}\cr+\frac{\mathbb{G}_{4}\big({{\bf t}_{1}}_{\ell-1}, \tilde{{\bf D}}_{\ell-1}\big)\big(\mathbb{B}_{4}({{\bf t}_{1}}_{\ell})-\mathbb{B}_{4}({{\bf t}_{1}}_{\ell-1})\big)}{2{\bf h}}\Big\}\bigg(\frac{2{\bf h}^{\varpi+2}\big((\ell-\frac{\varpi}{2})(\ell+1)^{\varpi+1}-\ell^{\varpi+1}(\ell+1+\frac{\varpi}{2})\big)}{\varpi(\varpi+1)(\varpi+2) }\bigg)\end{cases}\right] \end{array} |
\quad+\frac{\delta\varpi}{\mathbb{M}(\delta)}\left[\begin{cases} \Big\{\tilde{{\bf D}}^{\ast}\big({{\bf t}_{1}}_{\mathfrak{m}+1}, \tilde{{\bf I}}_{\mathfrak{m}+1}^{{\bf q}}, \tilde{{\bf D}}_{\mathfrak{m}+1}^{{\bf q}}\big)\cr+\mathbb{G}_{4}\big({{\bf t}_{1}}_{\mathfrak{m}+1}, \tilde{{\bf D}}_{\mathfrak{m}+1}^{{\bf q}}\big)\big(\mathbb{B}_{4}({{\bf t}_{1}}_{\mathfrak{m}+1})-\mathbb{B}_{4}({{\bf t}_{1}}_{\mathfrak{m}})\big)\Big\}\frac{{\bf h}^{\varpi}}{\varpi}\big((\mathfrak{m}+1)^{\varpi}-\mathfrak{m}^{\varpi}\big)\cr+\frac{\tilde{{\bf D}}^{\ast}\big({{\bf t}_{1}}_{\mathfrak{m}+1}, \tilde{{\bf I}}_{\mathfrak{m}+1}, \tilde{{\bf D}}_{\mathfrak{m}+1}\big)-\tilde{{\bf D}}^{\ast}\big({{\bf t}_{1}}_{\mathfrak{m}}, \tilde{{\bf I}}_{\mathfrak{m}}, \tilde{{\bf D}}_{\mathfrak{m}}\big)}{{\bf h}}\cr+\Big\{\mathbb{G}_{4}\big({{\bf t}_{1}}_{\mathfrak{m}+1}, \tilde{{\bf D}}_{\mathfrak{m}+1}\big)\big(\mathbb{B}_{4}({{\bf t}_{1}}_{\mathfrak{m}+1})-\mathbb{B}_{4}({{\bf t}_{1}}_{\mathfrak{m}})\big)\cr-\mathbb{G}_{4}\big({{\bf t}_{1}}_{\mathfrak{m}}, \tilde{{\bf D}}_{\mathfrak{m}}\big)\big(\mathbb{B}_{4}({{\bf t}_{1}}_{\mathfrak{m}})-\mathbb{B}_{4}({{\bf t}_{1}}_{\mathfrak{m}-1})\big)\Big\}\cr\times\bigg(\frac{{\bf h}_{\varpi+1}(\ell^{\varpi}(\ell+1+\varpi)-(\ell+1)^{\varpi+1})}{\varpi(\varpi+1)}\bigg)\cr+ \frac{\tilde{{\bf D}}^{\ast}\big({{\bf t}_{1}}_{\mathfrak{m}+1}, \tilde{{\bf I}}_{\mathfrak{m}+1}, \tilde{{\bf D}}_{\mathfrak{m}+1}\big)-2\tilde{{\bf D}}^{\ast}\big({{\bf t}_{1}}_{\mathfrak{m}}, \tilde{{\bf I}}_{\mathfrak{m}}, \tilde{{\bf D}}_{\mathfrak{m}}\big)+\tilde{{\bf D}}^{\ast}\big({{\bf t}_{1}}_{\mathfrak{m}-1}, \tilde{{\bf I}}_{\mathfrak{m}-1}, \tilde{{\bf D}}_{\mathfrak{m}-1}\big)}{2{\bf h}^{2}}\cr+ \bigg\{\frac{\mathbb{G}_{4}\big({{\bf t}_{1}}_{\mathfrak{m}+1}, \tilde{{\bf D}}_{\mathfrak{m}+1}\big)\big(\mathbb{B}_{4}({{\bf t}_{1}}_{\mathfrak{m}+1})-\mathbb{B}_{4}({{\bf t}_{1}}_{\mathfrak{m}})\big)-2\mathbb{G}_{4}\big({{\bf t}_{1}}_{\mathfrak{m}}, \tilde{{\bf D}}_{\mathfrak{m}}\big)\big(\mathbb{B}_{4}({{\bf t}_{1}}_{\mathfrak{m}})-\mathbb{B}_{4}({{\bf t}_{1}}_{\mathfrak{m}-1})\big)}{2{\bf h}}\cr+\frac{\mathbb{G}_{4}\big({{\bf t}_{1}}_{\mathfrak{m}-1}, \tilde{{\bf D}}_{\mathfrak{m}-1}\big)\big(\mathbb{B}_{4}({{\bf t}_{1}}_{\mathfrak{m}-1})-\mathbb{B}_{4}({{\bf t}_{1}}_{\mathfrak{m}-2})\big)}{2{\bf h}}\bigg\} \bigg(\frac{2{\bf h}^{\varpi+2}\big((\ell-\frac{\varpi}{2})(\ell+1)^{\varpi+1}-\ell^{\varpi+1}(\ell+1+\frac{\varpi}{2})\big)}{\varpi(\varpi+1)(\varpi+2) }\bigg) \end{cases}\right], |
where
\begin{eqnarray*} \tilde{{\bf H}}_{\mathfrak{m}+1}^{{\bf q}}&& = \tilde{{\bf H}}_{0}+\frac{(1-\delta)\delta\varpi}{\mathbb{M}(\delta)}{{\bf t}_{1}}_{\mathfrak{m}+1}^{\varpi-1}\left[ \begin{cases}\tilde{{\bf H}}^{\ast}\big({{\bf t}_{1}}_{\mathfrak{m}}, \tilde{{\bf H}}_{\mathfrak{m}}, \tilde{{\bf I}}_{\mathfrak{m}}, \tilde{{\bf V}}_{\mathfrak{m}}, \tilde{{\bf D}}_{\mathfrak{m}}\big)\cr+\varpi\sigma_{1}\mathbb{G}_{1}\big({{\bf t}_{1}}_{\mathfrak{m}+1}, \tilde{{\bf H}}_{\mathfrak{m}+1}\big)\big(\mathbb{B}_{1}({{\bf t}_{1}}_{\mathfrak{m}+1})-\mathbb{B}_{1}({{\bf t}_{1}}_{\mathfrak{m}})\big)\end{cases}\right]\nonumber\\&&\quad+ \frac{\delta\varpi}{\mathbb{M}(\delta)}\sum\limits_{\ell = 0}^{\mathfrak{m}-1}\left[\begin{cases}\tilde{{\bf H}}^{\ast}\big({{\bf t}_{1}}_{\mathfrak{m}}, \tilde{{\bf H}}_{\mathfrak{m}}, \tilde{{\bf I}}_{\mathfrak{m}}, \tilde{{\bf V}}_{\mathfrak{m}}, \tilde{{\bf D}}_{\mathfrak{m}}\big)\frac{{\bf h}^{\varpi}}{\varpi}\big((\ell+1)^{\varpi}-\ell^{\varpi}\big)\cr+\sigma_{1}\mathbb{G}_{1}\big({{\bf t}_{1}}_{\mathfrak{m}}, \tilde{{\bf H}}_{\mathfrak{m}}\big)\big(\mathbb{B}_{1}({{\bf t}_{1}}_{\mathfrak{m}+1})-\mathbb{B}_{1}({{\bf t}_{1}}_{\mathfrak{m}})\big)\frac{{\bf h}^{\varpi}}{\varpi}\big((\ell+1)^{\varpi}-\ell^{\varpi}\big)\end{cases}\right], \end{eqnarray*} |
\begin{eqnarray*} \tilde{{\bf I}}_{\mathfrak{m}+1}^{{\bf q}}&& = \tilde{{\bf I}}_{0}+\frac{(1-\delta)\delta\varpi}{\mathbb{M}(\delta)}{{\bf t}_{1}}_{\mathfrak{m}+1}^{\varpi-1}\left[ \begin{cases}\tilde{{\bf I}}^{\ast}\big({{\bf t}_{1}}_{\mathfrak{m}}, \tilde{{\bf H}}_{\mathfrak{m}}, \tilde{{\bf I}}_{\mathfrak{m}}, \tilde{{\bf V}}_{\mathfrak{m}}, \tilde{{\bf D}}_{\mathfrak{m}}\big)\cr+\varpi\sigma_{2}\mathbb{G}_{2}\big({{\bf t}_{1}}_{\mathfrak{m}+1}, \tilde{{\bf I}}_{\mathfrak{m}+1}\big)\big(\mathbb{B}_{2}({{\bf t}_{1}}_{\mathfrak{m}+1})-\mathbb{B}_{2}({{\bf t}_{1}}_{\mathfrak{m}})\big)\end{cases}\right]\nonumber\\&&\quad+ \frac{\delta\varpi}{\mathbb{M}(\delta)}\sum\limits_{\ell = 0}^{\mathfrak{m}-1}\left[\begin{cases}\tilde{{\bf I}}^{\ast}\big({{\bf t}_{1}}_{\mathfrak{m}}, \tilde{{\bf H}}_{\mathfrak{m}}, \tilde{{\bf I}}_{\mathfrak{m}}, \tilde{{\bf V}}_{\mathfrak{m}}, \tilde{{\bf D}}_{\mathfrak{m}}\big)\frac{{\bf h}^{\varpi}}{\varpi}\big((\ell+1)^{\varpi}-\ell^{\varpi}\big)\cr+\sigma_{2}\mathbb{G}_{2}\big({{\bf t}_{1}}_{\mathfrak{m}}, \tilde{{\bf I}}_{\mathfrak{m}}\big)\big(\mathbb{B}_{2}({{\bf t}_{1}}_{\mathfrak{m}+1})-\mathbb{B}_{2}({{\bf t}_{1}}_{\mathfrak{m}})\big)\frac{{\bf h}^{\varpi}}{\varpi}\big((\ell+1)^{\varpi}-\ell^{\varpi}\big)\end{cases}\right], \end{eqnarray*} |
\begin{eqnarray*} \tilde{{\bf V}}_{\mathfrak{m}+1}^{{\bf q}}&& = \tilde{{\bf V}}_{0}+\frac{(1-\delta)\delta\varpi}{\mathbb{M}(\delta)}{{\bf t}_{1}}_{\mathfrak{m}+1}^{\varpi-1}\left[ \begin{cases}\tilde{{\bf V}}^{\ast}\big({{\bf t}_{1}}_{\mathfrak{m}}, \tilde{{\bf I}}_{\mathfrak{m}}, \tilde{{\bf V}}_{\mathfrak{m}}\big)\cr+\varpi\sigma_{3}\mathbb{G}_{3}\big({{\bf t}_{1}}_{\mathfrak{m}+1}, \tilde{{\bf V}}_{\mathfrak{m}+1}\big)\big(\mathbb{B}_{3}({{\bf t}_{1}}_{\mathfrak{m}+1})-\mathbb{B}_{3}({{\bf t}_{1}}_{\mathfrak{m}})\big)\end{cases}\right]\nonumber\\&&\quad+ \frac{\delta\varpi}{\mathbb{M}(\delta)}\sum\limits_{\ell = 0}^{\mathfrak{m}-1}\left[\begin{cases}\tilde{{\bf V}}^{\ast}\big({{\bf t}_{1}}_{\mathfrak{m}}, \tilde{{\bf I}}_{\mathfrak{m}}, \tilde{{\bf V}}_{\mathfrak{m}}\big)\frac{{\bf h}^{\varpi}}{\varpi}\big((\ell+1)^{\varpi}-\ell^{\varpi}\big)\cr+\sigma_{3}\mathbb{G}_{3}\big({{\bf t}_{1}}_{\mathfrak{m}}, \tilde{{\bf V}}_{\mathfrak{m}}\big)\big(\mathbb{B}_{3}({{\bf t}_{1}}_{\mathfrak{m}+1})-\mathbb{B}_{3}({{\bf t}_{1}}_{\mathfrak{m}})\big)\frac{{\bf h}^{\varpi}}{\varpi}\big((\ell+1)^{\varpi}-\ell^{\varpi}\big)\end{cases}\right], \end{eqnarray*} |
\begin{eqnarray*} \tilde{{\bf D}}_{\mathfrak{m}+1}^{{\bf q}}&& = \tilde{{\bf D}}_{0}+\frac{(1-\delta)\delta\varpi}{\mathbb{M}(\delta)}{{\bf t}_{1}}_{\mathfrak{m}+1}^{\varpi-1}\left[ \begin{cases}\tilde{{\bf D}}^{\ast}\big({{\bf t}_{1}}_{\mathfrak{m}}, \tilde{{\bf I}}_{\mathfrak{m}}, \tilde{{\bf D}}_{\mathfrak{m}}\big)\cr+\varpi\sigma_{4}\mathbb{G}_{4}\big({{\bf t}_{1}}_{\mathfrak{m}+1}, \tilde{{\bf D}}_{\mathfrak{m}+1}\big)\big(\mathbb{B}_{4}({{\bf t}_{1}}_{\mathfrak{m}+1})-\mathbb{B}_{4}({{\bf t}_{1}}_{\mathfrak{m}})\big)\end{cases}\right]\nonumber\\&&\quad+ \frac{\delta\varpi}{\mathbb{M}(\delta)}\sum\limits_{\ell = 0}^{\mathfrak{m}-1}\left[\begin{cases}\tilde{{\bf D}}^{\ast}\big({{\bf t}_{1}}_{\mathfrak{m}}, \tilde{{\bf I}}_{\mathfrak{m}}, \tilde{{\bf D}}_{\mathfrak{m}}\big)\frac{{\bf h}^{\varpi}}{\varpi}\big((\ell+1)^{\varpi}-\ell^{\varpi}\big)\cr+\sigma_{4}\mathbb{G}_{4}\big({{\bf t}_{1}}_{\mathfrak{m}}, \tilde{{\bf D}}_{\mathfrak{m}}\big)\big(\mathbb{B}_{4}({{\bf t}_{1}}_{\mathfrak{m}+1})-\mathbb{B}_{4}({{\bf t}_{1}}_{\mathfrak{m}})\big)\frac{{\bf h}^{\varpi}}{\varpi}\big((\ell+1)^{\varpi}-\ell^{\varpi}\big)\end{cases}\right]. \end{eqnarray*} |
To illustrate the aforementioned mathematical findings, we will provide a few simulation studies in this part. We find the system's stochastic F-F derivative in the Caputo-Fabrizio context using a revolutionary numerical approach introduced in [41]. An evolutionary algorithm was developed to numerically predict outcomes since state formulas possess ICs. Table 2 is an overview of the primary attribute settings. The outcomes with no controls, just vaccine restrictions, just therapy regulation, and both vaccination and medication regulations are compared.
Symbols | Values | References | Units |
\lambda | 6 | [18] | cell ml ^{-1} day^{-1} |
\vartheta | 0.2 | [9] | cell ml ^{-1} day^{-1} |
\phi_{1} | 0.01 | [55] | day^{-1} |
\phi_{2} | 0.1 | [51] | day^{-1} |
\phi_{3} | 0.1 | [18] | day^{-1} |
\phi_{4} | 0.3 | [9] | day^{-1} |
\gamma_{1} | 0.01 | [57] | virions^{-1} day^{-1} |
\gamma_{2} | 0.1 | [18] | cell^{-1} day^{-1} |
\gamma_{3} | 0.2 | [56] | cell^{-1} day^{-1} |
\gamma_{4} | 0.015 | [18] | cell^{-1} day^{-1} |
\omega | 0.4 | [56] | cell^{-1} day^{-1} |
Figures 4–5 presents the dynamics of the F-F HBV model (5.1) with attributed values of Table 2 involving TDs \zeta_{1} = 1, \; \zeta_{2} = 2, assuming \sigma_{1} = 0.003, \sigma_{2} = 0.004, \; \sigma_{3} = 0.006 and \sigma_{4} = 0.004. Straightforward computations result in \mathbb{R}_{0}^{{\bf s}} < 1 , satisfying the requirements of Theorem 4.2. Figures 4-5 demonstrate that there are fewer individuals who have been treated by untreated adults and that the proportion of acutely and chronically contaminated youngsters is declining, respectively. Therefore, we observe that controlling the preponderance of individuals who are HBV-positive may significantly lower or reduce the amount of contaminated neonates who are released during the distribution process when fractional-order \delta decreasing and fractal-dimension \varpi remains fixed. It is concluded that the F-F HBV model (5.1) generated in this research is perfectly accurate. It is thought that it can highlight several important characteristics that are also true in more simulation designs of HBV infection.
Figures 6–7 define the complexities of the F-F HBV model (5.1) with aforementioned parametric descriptions considering TDs \zeta_{1} = 1, \; \zeta_{2} = 2, assuming \sigma_{1} = 0.003, \sigma_{2} = 0.004, \; \sigma_{3} = 0.006 and \sigma_{4} = 0.004. This also predicts that, through cytolytic and non-cytolytic processes, CTL cells are essential for the prevention and treatment of HBV infection. Infectious colonies are killed by cytolytic control by manipulating, while noncytolytic regulatory processes "treat" the intracellular pathogens [51]. According to F-F investigations, the infectious equilibrium state is robust and the pace at which pathogens produce free viral infection is low. If a medicine with a profound impact is discovered, the value of the rate of the viral bacteria's item will decrease, and other immunization collaborators should step up their efforts to reduce interaction rates to a meaningful scale when \delta is fixed and \varpi declines. The HBV infection can be treated.
Figures 8–9 illustrate the complexities of the F-F HBV model, (5.1) with aforementioned parametric descriptions, considering TDs \zeta_{1} = 1, \; \zeta_{2} = 2, assuming \sigma_{1} = 0.1, \sigma_{2} = 0.2, \; \sigma_{3} = 0.3 and \sigma_{4} = 0.4. The likelihood that the sickness will disappear is, therefore, one. It has been demonstrated that a white noise setting with a higher intensity may aid in curing the illness more quickly than a simulation sans noise when \delta decreases and \varpi increased. Our analysis's clear and fundamental goal is to minimize the harm inflicted by HBV by reducing the population's infection rate while increasing the population's rate of recovery. The authorities have a mission to ensure that people are notified of the proliferation of the HBV.
Figures 10–11 show the intricacies of the F-F HBV model, (5.1) utilizing the parameterized representations stated above and TDs \zeta_{1} = 1, \; \zeta_{2} = 2, assuming \sigma_{1} = 0.1, \sigma_{2} = 0.2, \; \sigma_{3} = 0.3 and \sigma_{4} = 0.4. The aforementioned configurations 10-11 makes it evident that the basic reproduction ratio \mathbb{R}_{0}^{{\bf s}} in framework (3.1) does not incorporate the index of CTL cells, which means that \mathbb{R}_{0}^{{\bf s}} is unable to accurately represent the function of immunogenicity. However, formula (5.1) includes the \delta and \varpi , which helps illustrate the function of the CTL cells. As a result, the model (5.1) ought to be more feasible.
Figures 12–13 presents the solution for low densities of \sigma_{\kappa}, \; \kappa = 1, 2, 3, 4 using the Matlab package. Therefore, if a treatment with a huge influence can be discovered, the HBV infection can be treated provided that the value of \vartheta is sufficiently diminished. According to the above mechanism, since many people are uneducated and unaware of viral diseases associated with them, particularly HBV, it indicates that the current regime should encourage good hygiene precautions in the general populace. Both the recycling of syringes on minors in rural areas and inadequate sanitary standards in public health sectors, which are major causes of HBV, should be prohibited. Blood donations must follow a specified protocol and must first be tested and approved by an officially recognized lab.
In a nutshell, the presence of an F-F derivative operator with stochastic disturbance (noise) and intracellular TDs in the framework (3.1) is hypothesized to deliver a better awareness in the presentation of the presented data, which has serious repercussions for treating alternatives and targeted therapies.
In the current study, we examined the effects of high-order stochastic disturbances on the complexities of the delay differential framework of HBV disease, which includes internalized latency, immunotherapy, disease-to-organ and their transmissions via the F-F derivative within the exponential decay kernel. We also presented the non-negative solution pertaining to the unit probability, stochastic reproduction number, and local stability, which is calculated for the steady states. Under certain assumptions, the sickness may eventually go away under certain circumstances, with probability 1. A revolutionary approach is used in a handful of simulated findings to demonstrate the viability of the outcomes. In the therapy of HBV and other viral disorders, the volume of Gaussian white noise is crucial. The presence of random disturbances (noise) and biochemical TDs in the framework is predicated to provide a greater understanding of the quantitative results, which has significant consequences for antibiotic compounds and regenerative medicine. Several additional intriguing subjects need to be looked into more thoroughly. Other types of environmental noise, including Lévy noise, may be taken into consideration [52]. Additionally, the deterministic system can be expanded to incorporate fractional derivatives in the framework to be able to take into account long-run memory of the bacteria's behavior, which is suggested by the research in [53,54].
All sources of funding of the study must be disclosed
The authors declare there is no conflict of interest.
[1] |
M. Mandal, S. Jana, S. K. Nandi, A. Khatua, S. Adak, T. K. Kar, A model based study on the dynamics of COVID-19: Prediction and control, Chaos Solitons Fractals, 136 (2020), 109889. https://doi.org/10.1016/j.chaos.2020.109889 doi: 10.1016/j.chaos.2020.109889
![]() |
[2] |
S. Muhammad, M. A. Z. Raja, M. T. Sabir, A. H. Bukhari, H. Alrabaiah, Z. Shah, et al., A stochastic numerical analysis based on hybrid NAR-RBFs networks nonlinear SITR model for novel COVID-19 dynamics, Comput. Methods Programs Biomed., 202 (2021), 105973. https://doi.org/10.1016/j.cmpb.2021.105973 doi: 10.1016/j.cmpb.2021.105973
![]() |
[3] |
O. M. Otunuga, Estimation of epidemiological parameters for COVID-19 cases using a stochastic SEIRS epidemic model with vital dynamics, Results Phys., 28 (2021), 104664. https://doi.org/10.1016/j.rinp.2021.104664 doi: 10.1016/j.rinp.2021.104664
![]() |
[4] |
X. Zhu, B. Gao, Y. Zhong, C. Gu, K. Choi, Extended Kalman filter based on stochastic epidemiological model for COVID-19 modelling, Comput. Biol. Med., 137 (2021), 104810. https://doi.org/10.1016/j.compbiomed.2021.104810 doi: 10.1016/j.compbiomed.2021.104810
![]() |
[5] |
J. P. Hespanha, C. Raphael, R. R. Costa, M. K. Erdal, G. Yang, Forecasting COVID-19 cases based on a parameter-varying stochastic SIR model, Annu. Rev. Control, 51 (2021), 460–476. https://doi.org/10.1016/j.arcontrol.2021.03.008 doi: 10.1016/j.arcontrol.2021.03.008
![]() |
[6] |
O. E. Deeb, M. Jalloul, The dynamics of COVID-19 spread: evidence from Lebanon, Math. Biosci. Eng., 17 (2020), 5618–5632. https://doi.org/10.3934/mbe.2020302 doi: 10.3934/mbe.2020302
![]() |
[7] |
B. Machado, L. Antunes, C. Caetano, et al., The impact of vaccination on the evolution of COVID-19 in Portugal, Math. Biosci. Eng., 19 (2022), 936–952. https://doi.org/10.3934/mbe.2022043 doi: 10.3934/mbe.2022043
![]() |
[8] |
S. He, S. Tang, L. Rong, A discrete stochastic model of the COVID-19 outbreak: Forecast and control, Math. Biosci. Eng., 17 (2020), 2792–2804. https://doi.org/10.3934/mbe.2020153 doi: 10.3934/mbe.2020153
![]() |
[9] |
W. O. Kermack, A. G. McKendrick, Contributions to the mathematical theory of epidemics-III. Further studies of the problem of endemicity, Bull. Math. Biol., 53 (1991), 89–118. https://doi.org/10.1016/S0092-8240(05)80042-4 doi: 10.1016/S0092-8240(05)80042-4
![]() |
[10] |
S. Djilali, A. Zeb, T. Saeed, Effect of Occasional heroin consumers on the spread of heroin addiction, Fractals, 5 (2022), 2240164. https://doi.org/10.1142/S0218348X22401648 doi: 10.1142/S0218348X22401648
![]() |
[11] |
E. A. Iboi, O. Sharomi, C. N. Ngonghala, A. B. Gumel, Mathematical modeling and analysis of COVID-19 pandemic in Nigeria, Math. Biosci. Eng., 17 (2020), 7192–7220. https://doi.org/10.3934/mbe.2020369 doi: 10.3934/mbe.2020369
![]() |
[12] |
S. Djilali, S. Bentout, T. M. Touaoula, A. Tridanee, S. Kumarf, Global behavior of Heroin epidemic model with time distributed delay and nonlinear incidence function, Results Phys., 31 (2021), 104953. https://doi.org/10.1016/j.rinp.2021.104953 doi: 10.1016/j.rinp.2021.104953
![]() |
[13] |
V. Piccirillo, COVID-19 pandemic control using restrictions and vaccination, Math. Biosci. Eng., 19 (2022), 1355–1372. https://doi.org/10.3934/mbe.2022062 doi: 10.3934/mbe.2022062
![]() |
[14] |
V. Piccirillo, Nonlinear control of infection spread based on a deterministic SEIR model, Chaos Solitons Fractals, 149 (2021), 111051. https://doi.org/10.1016/j.chaos.2021.111051 doi: 10.1016/j.chaos.2021.111051
![]() |
[15] |
S. Djilali, S. Bentout, T. M. Touaoula, A. Tridane, Global dynamics of alcoholism epidemic model with distributed delays, Math. Biosci. Eng., 18 (2021), 8245–8256. https://doi.org/10.3934/mbe.2021409 doi: 10.3934/mbe.2021409
![]() |
[16] |
S. Batabyal, COVID-19: Perturbation dynamics resulting chaos to stable with seasonality transmission, Chaos Solitons Fractals, 145 (2021), 110772. https://doi.org/10.1016/j.chaos.2021.110772 doi: 10.1016/j.chaos.2021.110772
![]() |
[17] |
A. B. Gumel, E. A. Iboi, C. N. Ngonghala, E. H. Elbasha, A primer on using mathematics to understand COVID-19 dynamics: Modeling, analysis and simulations, Infect. Dis. Model., 6 (2021), 148–168. https://doi.org/10.1016/j.idm.2020.11.005 doi: 10.1016/j.idm.2020.11.005
![]() |
[18] |
A. Zeb, S. Djilali, T. Saeed, M. Sh. Alhodalyd, N. Gule, Global proprieties of an SIR epidemic model with nonlocal diffusion and immigration, Results Phys., 39 (2022), 105758. https://doi.org/10.1016/j.rinp.2022.105758 doi: 10.1016/j.rinp.2022.105758
![]() |
[19] |
F. A. Rihan, H. J. Alsakaji, Dynamics of a stochastic delay differential model for COVID-19 infection with asymptomatic infected and interacting people: Case study in the UAE, Results Phys., 28 (2021), 104658. https://doi.org/10.1016/j.rinp.2021.104658 doi: 10.1016/j.rinp.2021.104658
![]() |
[20] |
Z. Zhang, A. Zeb, S. Hussain, E. Alzahrani, Dynamics of COVID-19 mathematical model with stochastic perturbation, Adv. Differ. Equations, 1 (2020), 451. https://doi.org/10.1186/s13662-020-02909 doi: 10.1186/s13662-020-02909
![]() |
[21] |
J. Danane, K. Allali, Z. Hammouch, K. S. Nisar, Mathematical analysis and simulation of a stochastic COVID-19 L\acute{e}vy jump model with isolation strategy, Results Phys., 23 (2021), 103994. https://doi.org/10.1016/j.rinp.2021.103994 doi: 10.1016/j.rinp.2021.103994
![]() |
[22] |
D. Adak, A. Majumder, N. Bairagi, Mathematical perspective of Covid-19 pandemic: Disease extinction criteria in deterministic and stochastic models, Chaos Solitons Fractals, 142 (2020), 110381. https://doi.org/10.1016/j.chaos.2020.110381 doi: 10.1016/j.chaos.2020.110381
![]() |
[23] |
B. Boukanjime, T. Caraballo, M. El Fatini, M. El Khalifi, Dynamics of a stochastic coronavirus (COVID-19) epidemic model with Markovian switching, Chaos Solitons Fractals, 141 (2020), 110361. https://doi.org/10.1016/j.chaos.2020.110361 doi: 10.1016/j.chaos.2020.110361
![]() |
[24] |
N. H. Sweilam, S. M. AL-Mekhlafi, D. Baleanu, A hybrid stochastic fractional order Coronavirus (2019-nCov) mathematical model, Chaos Solitons Fractals, 145 (2021), 110762. https://doi.org/10.1016/j.chaos.2021.110762 doi: 10.1016/j.chaos.2021.110762
![]() |
[25] |
A. Din, A. Khan, D. Baleanuc, Stationary distribution and extinction of stochastic coronavirus (COVID-19) epidemic model, Chaos Solitons Fractals, 139 (2020), 110036. https://doi.org/10.1016/j.chaos.2020.110036 doi: 10.1016/j.chaos.2020.110036
![]() |
[26] |
T. Khan, G. Zaman, Y. El-Khatib, Modeling the dynamics of novel coronavirus (COVID-19) via stochastic epidemic model, Results Phys., 24 (2020), 104004. https://doi.org/10.1016/j.rinp.2021.104004 doi: 10.1016/j.rinp.2021.104004
![]() |
[27] |
A. Tesfay, T. Saeed, A. Zeb, D. Tesfay, A. Khalaf, J. Brannan, Dynamics of a stochastic COVID-19 epidemic model with jump-diffusion, Adv. Differ. Equations, 1 (2021), 228. https://doi.org/10.1186/s13662-021-03396-8 doi: 10.1186/s13662-021-03396-8
![]() |
[28] |
K. Zhao, S. Ma, Qualitative analysis of a two-group SVIR epidemic model with random effect, Adv. Differ. Equations, 1 (2021), 172. https://doi.org/10.1186/s13662-021-03332-w doi: 10.1186/s13662-021-03332-w
![]() |
[29] |
Q. Liu, D. Jiang, T. Hayat, A. Alsaedi, Stationary distribution of a stochastic delayed SVEIR epidemic model with vaccination and saturation incidence, Phys. A, 512 (2018), 849–863. https://doi.org/10.1016/j.physa.2018.08.054 doi: 10.1016/j.physa.2018.08.054
![]() |
[30] |
Z. Zhang, R. K. Upadhyay, Dynamical analysis for a deterministic SVIRS epidemic model with Holling type II incidence rate and multiple delays, Results Phys., 24 (2021), 104181. https://doi.org/10.1016/j.rinp.2021.104181 doi: 10.1016/j.rinp.2021.104181
![]() |
[31] |
Y. Xing, H. Li, Almost periodic solutions for a SVIR epidemic model with relapse, Math. Biosci. Eng., 18 (2021), 7191–7217. https://doi.org/10.3934/mbe.2021356 doi: 10.3934/mbe.2021356
![]() |
[32] |
S. Djilali, S. Bentout, Global dynamics of SVIR epidemic model with distributed delay and imperfect vaccine, Results Phys., 25 (2021), 104245. https://doi.org/10.1016/j.rinp.2021.104245 doi: 10.1016/j.rinp.2021.104245
![]() |
[33] |
X. Zhang, D. Jiang, T. Hayat, B. Ahmad, Dynamical behavior of a stochastic SVIR epidemic model with vaccination, Phys. A, 483 (2017), 94–108. https://doi.org/10.1016/j.physa.2017.04.173 doi: 10.1016/j.physa.2017.04.173
![]() |
[34] |
A. W. Tesfaye, T. S. Satana, Stochastic model of the transmission dynamics of COVID-19 pandemic, Adv. Differ. Equations, 1 (2021), 457–457. https://doi.org/10.1186/s13662-021-03597-1 doi: 10.1186/s13662-021-03597-1
![]() |
[35] |
F. Wang, L. Cao, X. Song, Mathematical modeling of mutated COVID-19 transmission with quarantine, isolation and vaccination, Math. Biosci. Eng., 19 (2022), 8035–8056. https://doi.org/10.3934/mbe.2022376 doi: 10.3934/mbe.2022376
![]() |
[36] |
O. A. M. Omar, Y. Alnafisah, R. A. Elbarkouky, H. M. Ahmed, COVID-19 deterministic and stochastic modelling with optimized daily vaccinations in Saudi Arabia, Results Phys., 28 (2021), 104629. https://doi.org/10.1016/j.rinp.2021.104629 doi: 10.1016/j.rinp.2021.104629
![]() |
[37] |
M. A. Alshaikh, Stability of discrete-time delayed influenza model with two-strain and two vaccinations, Results Phys., 28 (2021), 104563. https://doi.org/10.1016/j.rinp.2021.104629 doi: 10.1016/j.rinp.2021.104629
![]() |
[38] |
D. J. Higham, An Algorithmic Introduction to Numerical Simulation of Stochastic Differential Equations, SIAM Rev., 43 (2001), 525–546. https://doi.org/10.1137/S0036144500378302 doi: 10.1137/S0036144500378302
![]() |
[39] |
P. Agarwal, R. Singh, A. ul Rehmanc, Numerical solution of hybrid mathematical model of dengue transmission with relapse and memory via Adam-Bashforth-Moulton predictor-corrector scheme, Chaos Solitons Fractals, 143 (2021), 110564. https://doi.org/10.1016/j.chaos.2020.110564 doi: 10.1016/j.chaos.2020.110564
![]() |
[40] |
N. Anggriani, M.Z. Ndii, R. Ameliaa, W. Suryaningrata, M. A. AjiPratamaa, A mathematical COVID-19 model considering asymptomatic and symptomatic classes with waning immunity, Alexandria Eng. J., 61(2022), 113–124. https://doi.org/10.1016/j.aej.2021.04.104 doi: 10.1016/j.aej.2021.04.104
![]() |
[41] |
X. Zhang, X. Zhang, The threshold of a deterministic and a stochastic SIQS epidemic model with varying total population size, Appl. Math. Model., 91 (2021), 749–767. https://doi.org/10.1016/j.apm.2020.09.050 doi: 10.1016/j.apm.2020.09.050
![]() |
1. | Maysaa Al-Qureshi, Saima Rashid, Fahd Jarad, Mohammed Shaaf Alharthi, Dynamical behavior of a stochastic highly pathogenic avian influenza A (HPAI) epidemic model via piecewise fractional differential technique, 2023, 8, 2473-6988, 1737, 10.3934/math.2023089 | |
2. | Abdon Atangana, Saima Rashid, Analysis of a deterministic-stochastic oncolytic M1 model involving immune response via crossover behaviour: ergodic stationary distribution and extinction, 2023, 8, 2473-6988, 3236, 10.3934/math.2023167 | |
3. | Saowaluck Chasreechai, Muhammad Aamir Ali, Surapol Naowarat, Thanin Sitthiwirattham, Kamsing Nonlaopon, On some Simpson's and Newton's type of inequalities in multiplicative calculus with applications, 2023, 8, 2473-6988, 3885, 10.3934/math.2023193 | |
4. | Maysaa Al Qurashi, Saima Rashid, Ahmed M. Alshehri, Fahd Jarad, Farhat Safdar, New numerical dynamics of the fractional monkeypox virus model transmission pertaining to nonsingular kernels, 2022, 20, 1551-0018, 402, 10.3934/mbe.2023019 | |
5. | Farhat Safdar, Muhammad Attique, Extended weighted Simpson-like type inequalities for preinvex functions and their use in physical system, 2022, 1016-2526, 621, 10.52280/pujm.2022.541001 | |
6. | Hanan S. Gafel, Saima Rashid, Sayed K. Elagan, Novel codynamics of the HIV-1/HTLV-Ⅰ model involving humoral immune response and cellular outbreak: A new approach to probability density functions and fractional operators, 2023, 8, 2473-6988, 28246, 10.3934/math.20231446 | |
7. | Fabio Grizzi, Marco Spadaccini, Maurizio Chiriva-Internati, Mohamed A A A Hegazi, Robert S Bresalier, Cesare Hassan, Alessandro Repici, Silvia Carrara, Fractal nature of human gastrointestinal system: Exploring a new era, 2023, 29, 1007-9327, 4036, 10.3748/wjg.v29.i25.4036 | |
8. | XUEJUAN LI, RUI ZHAO, PHYSICS-INFORMED DEEP AI SIMULATION FOR FRACTAL INTEGRO-DIFFERENTIAL EQUATION, 2024, 32, 0218-348X, 10.1142/S0218348X24500221 | |
9. | Saima Rashid, Sher Zaman Hamidi, Saima Akram, Muhammad Aon Raza, S. K. Elagan, Beida Mohsen Tami Alsubei, Theoretical and mathematical codynamics of nonlinear tuberculosis and COVID-19 model pertaining to fractional calculus and probabilistic approach, 2024, 14, 2045-2322, 10.1038/s41598-024-59261-7 | |
10. | Saima Rashid, Sher Zaman Hamidi, Muhammad Aon Raza, Rafia Shafique, Assayel Sultan Alsubaie, Sayed K. Elagan, Robustness and exploration between the interplay of the nonlinear co-dynamics HIV/AIDS and pneumonia model via fractional differential operators and a probabilistic approach, 2024, 14, 2045-2322, 10.1038/s41598-024-65329-1 |
Symbols | Explanation |
\lambda | Rate of viral hepatocellular development via bone marrow and various tissues |
\vartheta | Rate at which CTLs are produced in the thymus |
\phi_{1} | Percentage of uninfectious hepatocytes that naturally die |
\phi_{2} | Percentage of infectious hepatocytes that naturally die |
\phi_{3} | Frequency of harmless pathogens dying |
\phi_{4} | Fatality rate of CTLs |
\gamma_{1} | Successful viral interaction incidence with healthy hepatocytes |
\gamma_{2} | Efficient proportion of interaction between healthy and diseased hepatocytes |
\gamma_{3} | CTLs' efficiency at eradicating infectious hepatocytes |
\gamma_{4} | CTL development speed as a result of contaminated cells' activation |
\omega | Speed of spontaneous viral activity in affected tissues |
Symbols | Values | References | Units |
\lambda | 6 | [18] | cell ml ^{-1} day^{-1} |
\vartheta | 0.2 | [9] | cell ml ^{-1} day^{-1} |
\phi_{1} | 0.01 | [55] | day^{-1} |
\phi_{2} | 0.1 | [51] | day^{-1} |
\phi_{3} | 0.1 | [18] | day^{-1} |
\phi_{4} | 0.3 | [9] | day^{-1} |
\gamma_{1} | 0.01 | [57] | virions^{-1} day^{-1} |
\gamma_{2} | 0.1 | [18] | cell^{-1} day^{-1} |
\gamma_{3} | 0.2 | [56] | cell^{-1} day^{-1} |
\gamma_{4} | 0.015 | [18] | cell^{-1} day^{-1} |
\omega | 0.4 | [56] | cell^{-1} day^{-1} |
Symbols | Explanation |
\lambda | Rate of viral hepatocellular development via bone marrow and various tissues |
\vartheta | Rate at which CTLs are produced in the thymus |
\phi_{1} | Percentage of uninfectious hepatocytes that naturally die |
\phi_{2} | Percentage of infectious hepatocytes that naturally die |
\phi_{3} | Frequency of harmless pathogens dying |
\phi_{4} | Fatality rate of CTLs |
\gamma_{1} | Successful viral interaction incidence with healthy hepatocytes |
\gamma_{2} | Efficient proportion of interaction between healthy and diseased hepatocytes |
\gamma_{3} | CTLs' efficiency at eradicating infectious hepatocytes |
\gamma_{4} | CTL development speed as a result of contaminated cells' activation |
\omega | Speed of spontaneous viral activity in affected tissues |
Symbols | Values | References | Units |
\lambda | 6 | [18] | cell ml ^{-1} day^{-1} |
\vartheta | 0.2 | [9] | cell ml ^{-1} day^{-1} |
\phi_{1} | 0.01 | [55] | day^{-1} |
\phi_{2} | 0.1 | [51] | day^{-1} |
\phi_{3} | 0.1 | [18] | day^{-1} |
\phi_{4} | 0.3 | [9] | day^{-1} |
\gamma_{1} | 0.01 | [57] | virions^{-1} day^{-1} |
\gamma_{2} | 0.1 | [18] | cell^{-1} day^{-1} |
\gamma_{3} | 0.2 | [56] | cell^{-1} day^{-1} |
\gamma_{4} | 0.015 | [18] | cell^{-1} day^{-1} |
\omega | 0.4 | [56] | cell^{-1} day^{-1} |