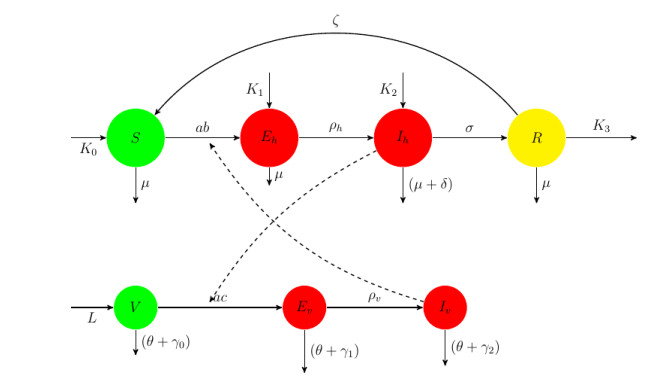
We present a compartmental model in ordinary differential equations of malaria disease transmission, accommodating the effect of indoor residual spraying on the vector population. The model allows for influx of infected migrants into the host population and for outflow of recovered migrants. The system is shown to have positive solutions. In the special case of no infected immigrants, we prove global stability of the disease-free equilibrium. Existence of a unique endemic equilibrium point is also established for the case of positive influx of infected migrants. As a case study we consider the combined South African malaria region. Using data covering 31 years, we quantify the effect of malaria infected immigrants on the South African malaria region.
Citation: Peter Witbooi, Gbenga Abiodun, Mozart Nsuami. A model of malaria population dynamics with migrants[J]. Mathematical Biosciences and Engineering, 2021, 18(6): 7301-7317. doi: 10.3934/mbe.2021361
[1] | Pride Duve, Samuel Charles, Justin Munyakazi, Renke Lühken, Peter Witbooi . A mathematical model for malaria disease dynamics with vaccination and infected immigrants. Mathematical Biosciences and Engineering, 2024, 21(1): 1082-1109. doi: 10.3934/mbe.2024045 |
[2] | Yicang Zhou, Zhien Ma . Global stability of a class of discrete age-structured SIS models with immigration. Mathematical Biosciences and Engineering, 2009, 6(2): 409-425. doi: 10.3934/mbe.2009.6.409 |
[3] | Souâd Yacheur, Ali Moussaoui, Abdessamad Tridane . Modeling the imported malaria to north Africa and the absorption effect of the immigrants. Mathematical Biosciences and Engineering, 2019, 16(2): 967-989. doi: 10.3934/mbe.2019045 |
[4] | Xia Wang, Yuming Chen . An age-structured vector-borne disease model with horizontal transmission in the host. Mathematical Biosciences and Engineering, 2018, 15(5): 1099-1116. doi: 10.3934/mbe.2018049 |
[5] | Yan-Xia Dang, Zhi-Peng Qiu, Xue-Zhi Li, Maia Martcheva . Global dynamics of a vector-host epidemic model with age of infection. Mathematical Biosciences and Engineering, 2017, 14(5&6): 1159-1186. doi: 10.3934/mbe.2017060 |
[6] | Cruz Vargas-De-León . Global analysis of a delayed vector-bias model for malaria transmission with incubation period in mosquitoes. Mathematical Biosciences and Engineering, 2012, 9(1): 165-174. doi: 10.3934/mbe.2012.9.165 |
[7] | Mugen Huang, Zifeng Wang, Zixin Nie . A stage structured model for mosquito suppression with immigration. Mathematical Biosciences and Engineering, 2024, 21(11): 7454-7479. doi: 10.3934/mbe.2024328 |
[8] | Suxia Zhang, Hongbin Guo, Robert Smith? . Dynamical analysis for a hepatitis B transmission model with immigration and infection age. Mathematical Biosciences and Engineering, 2018, 15(6): 1291-1313. doi: 10.3934/mbe.2018060 |
[9] | Maria Guadalupe Vazquez-Peña, Cruz Vargas-De-León, Jorge Velázquez-Castro . Global stability for a mosquito-borne disease model with continuous-time age structure in the susceptible and relapsed host classes. Mathematical Biosciences and Engineering, 2024, 21(11): 7582-7600. doi: 10.3934/mbe.2024333 |
[10] | Alian Li-Martín, Ramón Reyes-Carreto, Cruz Vargas-De-León . Dynamics of a dengue disease transmission model with two-stage structure in the human population. Mathematical Biosciences and Engineering, 2023, 20(1): 955-974. doi: 10.3934/mbe.2023044 |
We present a compartmental model in ordinary differential equations of malaria disease transmission, accommodating the effect of indoor residual spraying on the vector population. The model allows for influx of infected migrants into the host population and for outflow of recovered migrants. The system is shown to have positive solutions. In the special case of no infected immigrants, we prove global stability of the disease-free equilibrium. Existence of a unique endemic equilibrium point is also established for the case of positive influx of infected migrants. As a case study we consider the combined South African malaria region. Using data covering 31 years, we quantify the effect of malaria infected immigrants on the South African malaria region.
Malaria disease has been a major cause of morbidity and mortality in many regions of the world, particularly in Africa where year after year we see millions of people suffering of malaria. The World Health Organization (WHO) sets targets for control of malaria and its elimination in a number of countries [1]. The relevant authorities need to plan the most efficient strategies for malaria control and elimination [2]. Understanding the drivers of malaria disease is crucial in this regard. Over the years mathematical modelling has been an important tool in quantifying the effects of the different drivers and informing the optimal combinations of interventions [3,4,5,6,7,8,9,10]. Human lifestyle and behaviour are crucial factors which, if changed suitably, may assist in curbing the spread of malaria. The movement of people between regions with very different levels of infection, could cause new infection cases that otherwise might not have been [11]. In this article we study the effect of infected migrants into a given population for the case of malaria, using a new compartmental model in ordinary differential equations (ODEs) designed for this purpose. An SEIR model was applied to a measles problem in a similar manner in [11]. Related studies can also be found in [12,13,14], but their models are structurally very different from that in the current paper.
In the literature up to now, a population with two distinguishable components have been approached by way of a 2-group model, see [12,15] for instance. Here we follow a much simpler single group model, which allows for migrants not only to enter into, but also to exit from a local population. To the best of the authors' knowledge, the only example of such a model is in the paper [11]. For vector-borne diseases such a model has not been published before. In conventional models with inflow of infected such as [13,16,17], the immigrants usually become part of the single population. The current model allows the recovered migrants to exit the local population. Our model of a local poulation with migrants is simpler than the two-group models such as those of [12,15] and others. This is possible since we do not study the entire group of migrants. Instead, we assume that we know the rate of influx of infected among the migrants, and then we are interested in quantifying its effect on disease transmission in the local population. Thus the model can be applied to test and quantify the effect of disease resulting from infected tourists, infected business travellers, traveling sports people, touring musicians or cultural groups, and similar traveling people from elsewhere, visiting a local population. This is important in particular when the disease in point is well under control in the local population.
We show how a steady flow of migrants into and out of a local population, keeps the number of infections in the population above a certain positive threshold level. The model also explicitly accommodates the indoor residual spraying (IRS). As an application we consider the malaria region of South Africa over the period 1971–2001. The region experienced a very significant upsurge of malaria case numbers towards the end of the said period [18]. Our model is utilized to determine the extent to which the upsurge is due to a combination of influx of malaria infected migrants into the region and the effect of IRS. We test different scenarios of infected immigrants, who leave the region subsequently to recovery, and we record the local infections caused by the infected immigrants. Even with limited data, only annual case numbers, we obtain meaningful results.
The basic form of the model of malaria disease dynamics that we introduce in this paper has been popularly used in the literature. In particular, models with inflow of infectives appear in [12,13] for instance. Our model has the distinct feature of allowing for also the outflow of migrants.
The model comprises human compartments S, Eh, Ih and R to represent (resp.) susceptibles, latently infected, infectious and recovered. The vector population has the classes V, Ev and Iv, being (resp.) the susceptibles, latently infected and infectious vectors. The flow diagram, Figure 1, depicts the movement of individuals into and out of the population, and also the transfer of individuals between the different compartments inside the population.
The parameters of the system are described in Table 1. Among these parameters we assume that:
K0 | Rate of inflow into the class of susceptible humans, |
K1 | Rate of inflow of latently infected humans, |
K2 | Rate of inflow of infectious humans, |
K3 | Rate of outflow of humans from the recovered class, |
μ | Mortality rate for humans, not including death directly due to malaria, |
δ | The rate of human deaths due to malaria, |
θ | Mortality rate of vectors, not including the effect of IRS, |
a | The probability of a specific human getting bitten by a mosquito during a one-day period, |
b | The probability that a bite by an infected mosquito will lead to a (new) human infection, |
c | The probability that a bite on an infected human will lead to a (new) mosquito infection, |
ρh | Transfer rate of humans from Eh-class to Ih-class, |
σ | Transfer rate of humans from Ih-class to R-class (recovery rate), |
ζ | Transfer rate from R-class to S-class (rate of loss of temporary immunity) |
ρv | Transfer rate of vectors from Ev-class to Iv-class, |
γ0 | Mortality rate due to IRS in class V, |
γ1 | Mortality rate due to IRS in class Ev, |
γ2 | Mortality rate due to IRS in class Iv. |
K1, K2, K3, γ0, γ1 and γ2 are non-negative,
all the other parameters are positive.
IRS is assumed to increase the mortality rates of the vector by increments γi in the various compartments (cf. [8]). We assume that
γ0≤min{γ1,γ2}. |
This is based on the assumption that the bulk of vector bites that lead to transmission of malaria, will occur indoors, and indoors is where the vectors get exposed to the IRS.
We introduce the numbers B1, B2 and K=K0+K1+K2−K3, with
B1=K1/(ρh+μ) and B2=(K2+ρhB1)/(σ+μ+δ).
We shall fix the value of K3 to be
K3=σB2.
Motivation for this choice is given in Remark 5(c). The particular form and function of the numbers B1, B2 and K3 constitute the main novelty of this model and pan out in Theorem 2 and in Remark 5.
We present the following system of ODEs.
TheODEmodel˙S=K0+ζR−abIvS−μS˙Eh=K1+abIvS−(μ+ρh)Eh˙Ih=K2+ρhEh−(μ+δ+σ)Ih˙R=σIh−(μ+ζ)R−K3˙V=L−acIhV−(θ+γ0)V˙Ev=acIhV−(θ+ρv+γ1)Ev˙Iv=ρvEv−(θ+γ2)Iv |
The initial conditions are:
S(0)>0,Eh(0)>B1,Ih(0)>B2,R(0)>0,V(0)>0,Ev(0)>0,Iv(0)>0. |
The total N(t) of the human population and the total M(t) of the mosquito population satisfy the two identities, for all t≥0:
S(t)+Eh(t)+Ih(t)+R(t)=N(t), V(t)+Ev(t)+Iv(t)=M(t).
In the case of influx only into the compartment of susceptibles, the model has a disease-free equilibrium,
Z0=(K0/μ,0,0,0,L/(θ+γ0),0,0). |
We can calculate a threshold R for local stability of the disease-free equilibrium, following the next generation method [19]. This yields an invariant
R=√a2bcρhρvK0Lμ(θ+γ0)(μ+ρh)(μ+σ+δ)(θ+ρv+γ1)(θ+γ2). |
This R has the property that if R<1, then the disease-free equilibrium is locally asymptotically stable, and if R>1, then the disease-free equilibrium is unstable. We immediately note that R<1 if and only if R2<1. We define the sets G0,G1 as below.
G0={x∈R7+: x2>B1, x3>B2}, |
G1={x∈G0: x1+x2+x3+x4≤K/μ, x5+x6+x7≤V0}, |
with
V0=Lθ+γ0. |
Theorem 1. (a) B1+B2≤(K1+K2−K3)/μ.
Consider a fixed number t1>0. Suppose that X(t) is a local solution for which X(t)∈G0 while 0<t<t1.
(b) If N(0)≤K/μ, then N(t)≤K/μ for all 0<t≤t1.
(c) If M(0)≤V0, then M(t)≤V0 for all 0<t≤t1.
Proof. (a) We prove that K1+K2−K3−μ(B2+B1)≥0.
K1+K2−K3−μ(B2+B1)=K1+K2−σB2−μB2−μB1=K1+K2−σ+μσ+μ+δ[K2+ρhB1]−μB1≥K1−[(σ+μ)ρhσ+μ+δ+μ]B1=K1−(σ+μ)ρh+μ(σ+μ+δ)(σ+μ+δ)(ρh+μ)K1=δρh(σ+μ+δ)(ρh+μ)K1≥0. |
This proves (a).
Consider any local solution with X(t)∈R7+ for all 0<t≤t1.
(b) We note that
d(N(t)−K/μ)dt=K0+K1+K2−K3−μN(t)−δIh(t)≤−μ[N(t)−K/μ]. |
Therefore N(0)<K/μ implies that N(t)<K/μ for all 0<t≤t1.
(c) Also,
d(M(t)−V0)dt=L−(θ+γ0)M(t)−(γ1−γ0)Eh(t)−(γ2−γ0)Ih(t). |
By assumption, γ1−γ0>0 and γ2−γ0>0. Therefore,
d(M(t)−V0)dt≤L−(θ+γ0)M(t)=−(θ+γ0)(M(t)−V0). |
Therefore, (c) follows.
The method of proof by contradiction for positivity of solutions that we follow in Theorem 2, is popularly used, for instance in [11,20,21].
Theorem 2 (Positive global solutions). The set G1 is a positively invariant set.
Proof. Note that in view of Theorem 1, it suffices to prove that for paths with initial values in G1, the path will stay inside G0. Consider any point y∈G1. Then there exists a solution X(t) with initial value X(0)=y, and at least for some t0>0 we shall have X(t)∈G0 while t≤t0. Suppose that, to the contrary of the statement of the theorem, there exists a time value z with X(z)∉G0. Then the set
{t>0| X(t)∉G0} |
is non-empty and has (a finite) infimum, t1. We shall show by contradiction that t1=∞. We introduce the following two auxiliary variables:
J1(t)=Eh(t)−B1 and J2(t)=Ih(t)−B2.
Let us define the following function for 0≤t<t1.
F1(X(t))=ln(KμS(t))+ln(KμJ1(t))+ln(KμJ2(t))+ln(KμR(t))+Σ7i=5ln(V0Xi(t)). |
Then each of the terms in the summation is a positive-valued function, and we know that for a constant q>0,
limu→0+ln(q/u)=+∞ |
In particular, if any of the variables S,J1,J2,R,V,Ev or Iv tends to 0 as t→t1, then F1→∞, i.e.,
limt→t−1F1(t)=+∞. |
We shall prove that the latter is impossible if t1 is finite, and this will pose a contradiction.
We first note the following:
−J′1(t)J1(t)=−1J1(t)[K1+abS(t)Iv(t)−(ρh+μ)Eh(t)]≤−1J1(t)[K1−(ρh+μ)(J1(t)+B1)]=ρh+μ, |
−J′2(t)J2(t)=−1J2(t)[K2+ρhE(t)−(σ+δ+μ)Ih(t)]≤−1J2(t)[K2+ρhB1−(σ+δ+μ)(J2(t)+B2)]=σ+δ+μ, |
−R′(t)R(t)=−1R(t)[σIh(t)−(μ+ζ)R(t)−K3]≤−1R(t)[σB2−(μ+ζ)R(t)−K3]=μ+ζ. |
We calculate the derivative:
dF1dt=−1S[(K0−μS)+ζR−abIvS]−J′1J1−J′2J2−R′R−1V[(θ+γ0)(V0−V)−acIhV]−1Ev[acIhV−(θ+ρv+γ1)Ev]−1Iv[ρvEv−(θ+γ2)Iv]. |
After cancellation of some terms which are obviously negative, we obtain an inequality:
F′1≤abIv+(ρh+μ)+(δ+σ+μ)+(μ+ζ)+acIh+(θ+ρv+γ1)+(θ+γ2).
Thus F′1≤F0, with F0 being the constant
F0=abV0+ρh+(δ+σ)+μ+ζ+acK/μ+(θ+ρv+γ1)+(θ+γ2). |
Consequently for any t∈[0,t1), 0≤F1(t)≤F0t1. This is the contradiction, completing the proof.
For Theorem 3 below, the work on [5] is relevant, as there are no inflow of infections to consider. The proof that we present here is fairly direct and elementary.
Theorem 3. Consider the special case of our ODE model, with K1=K2=0. If R<1, then the disease-free equilibrium is globally asymptotically stable.
Proof. In the proof we find it convenient to use the symbol
D=(μ+ρh)(μ+σ+δ)(θ+ρv+γ1)(θ+γ2). |
Let us suppose that R<1, which is equivalent to the inequality:
a2bcρhρv(K0Lμ(θ+γ0))−D<0. |
Then there exists a positive number z<1, for which
a2bcρhρv(K0Lμ(θ+γ0))−z3D<0. |
We fix such a number z. We can also find (and take as fixed) positive numbers g3 and g4 which satisfy the inequality:
g3σ+[g4+abρhρv(K0μ)]ac(Lθ+γ0)−z3D<0. | (1) |
Now we proceed to introduce a set of numbers: {g0,g1,g2.....g6} that will be used in order to define a Lyapunov function. The candidates g3 and g4 have already been chosen. Furthermore, we let
g5=abρhρvK0/μ,g6=zg5(θ+γ1+ρv)/ρv,g1=zg6μ(θ+γ2)/(abK0),g2=zg1(μ+ρh)/ρh. |
Now since 0<z<1, we note that g1abK0/μ<g6(θ+γ2). Thus we can find a positive number g0 such that:
(g0+g1)abK0/μ−g6(θ+γ2)<0. | (2) |
All of these numbers gi are positive. Now we define the following function
F2=F2(S,Eh,Ih,R,V,Ev,Iv).
F2=g0(K0/μ−S)+g1Eh+g2Ih+g3R+g4(L/(θ+γ0)−V)+g5Ev+g6Iv.
We now show that F2 is Lyapunov at the disease-free equilibrium point. Certainly F2 is positive-definite. We can express F′2(t) as follows:
dF2dt=Q0(K0/μ−S)+Q1Eh+Q2Ih+Q3R+Q4(L/(θ+γ0)−V)+Q5Ev+Q6Iv,
where
Q0=−g0μ, Q4=−g4(θ+γ0), Q3=−g0ζ−g3(μ+ζ),
Q1=g2ρh−g1(μ+ρh), Q5=g6ρv−g5(θ+γ1+ρv),
Q2=g3σ+(g4+g5)acV−g2(μ+δ+σ), Q6=(g0+g1)abS−g6(θ+γ2).
Clearly Q0<0, Q3<0 and Q4<0. Considering the coefficient Q2, we note that
acV≤ac(Lθ+γ0) |
and g2(μ+δ+σ)=z3D. Thus from inequality (1) it follows that Q2<0. From the inequality (2) it follows that Q6<0. From the choice of g6 it follows that Q5<0 and our choice of g2 guarantees that Q1<0. Therefore F2(t) is Lyapunov at the disease-free equilibrium point.
Thus, if R<1, then in order to secure elimination of malaria from the population, it is sufficient to stop infected immigrants from entering.
Theorem 4. A unique endemic equilibrium point exists when one of the following conditions (a) or (b) holds:
(a) K1+K2>0,
(b) K1+K2=0 and R>1.
Proof. In this proof we shall use the notation:
μ1=μ+δ+σ, θ1=θ+ρv+γ1.
The coordinates of the endemic equilibrium point, when it exists, will satisfy the following equations.
R∗=σI∗h−K3μ+ζ, E∗h=μ1I∗h−K2ρh, S∗=K0+ζR∗μ+abI∗v, E∗v=(θ+γ2)I∗vρv, |
V∗=θ1E∗vacI∗h, V∗=Lθ+γ0+acI∗h, S∗=(μ+ρh)E∗h−K1abI∗v. |
Let us write
I∗h=x and I∗v=y,ζ0=ζμ+ζ and ρ0=μ+ρhρh |
Using the two different ways of expressing V∗, we derive the following identity.
y=acρvLxθ1(θ+γ2)(θ+γ0+acx) . |
Now we consider the two expressions for S∗, which we equate and we obtain an equation without S∗. From the latter equation we can eliminate all the variables other than x, ending up with a quadratic equation
Q2x2+Q1x+Q0=0, |
the coefficients of which are as follows:
Q2=a2bcρvL(ρ0μ1−ζ0σ)+acρ0μ1μθ1(θ+γ2),
Q1=−a2bcρvL(K0−ζ0K3)−(ρ0K2+K1)[μθ1(θ+γ2)ac+a2bcρvL]
+ρ0μμ1θ1(θ+γ2)(θ+γ0),
Q0=−μθ1(θ+γ2)(θ+γ0)(ρ0K2+K1).
Note that ζ0<1<ρ0 and σ<μ1, and thus, ρ0μ1−ζ0σ>0. Therefore Q2>0.
(a) If K1+K2>0, then Q0<0. Since Q2Q0<0, P has a unique positive root which is I∗h, and consequently there is a unique endemic equilibrium point.
(b) If K1+K2=0, then Q0=0 and Q1=ρ0μμ1θ1(θ+γ2)(θ+γ0)−a2bcρvLK0.
Note that Q1<0 if and only if R>1. Having R>1, we obtain Q2Q1<0. Consequently P has a unique positive root which is I∗h, and again there is a unique endemic equilibrium point.
Remark 5.. (a) The state (Emi,Imi,Rmi) of the migrant sub-population is described by the following system of ODEs:
E′mi(t)=K1−(ρh+μ)Emi(t)I′mi(t)=K2+ρhEmi(t)−(σ+δ+μ)Imi(t)R′mi(t)=σImi(t)−μRmi(t)−K3. |
with initial conditions: Emi(0)>B1, Imi(0)>B2, Rmi(0)>0.
(b) Similarly as in Theorem 2 it can be proved that any solution
(Emi(t), Imi(t), Rmi(t))
of the system in 5(a) above will be positive.
(c) If instead of taking K3=σB2 we venture to assign a higher value to K3, then we find that there will not be an equilibrium point for the system, as it would render R∗mi<0. This does not make sense biologically, and will certainly not be permissible.
If instead of taking K3=σB2 we choose a lower value to K3, then R∗mi>0. This means that some of the migrants will stay in the local population permanently. However, the aim of this model is to only consider short-term visitors rather than immigrants staying permanently in the local population. This is the motivation for our assumption: K3=σB2. Moreover, this results in a stable equilibrium point for the system of Remark 5(a), see Proposition 6.
The following proposition assures us that the outflow rate K3 will keep the migrant population size stable.
Proposition 6. The equilibrium point (B1,B2,0) of the system in 5(a) is globally asymptotically stable.
Proof. Let us consider the function
F2(t)=μ1(Emi(t)−B1)+(Imi(t)−B2)+Rmi(t).
We can calculate its time derivative and simplify, eventually to obtain
F′2(t)=−μ[Emi(t)−B1]−(μ1+δ)[Imi(t)−B2]−μRmi(t).
Thus F2(t) is a Lyapunov function, and it follows that (B1,B2,0) is globally asymptotically stable.
In this section we apply the theory to South Africa. Over the period 1971–1995, South Africa had an annual malaria prevalence which was at a level of well below 14000 cases p.a. on the average [18]. The annual prevalence shows a very clear spike in the period 1996–2001. Since malaria is closely correlated with certain climatic variables, such climatic anomalies could have been the reason for this upsurge of malaria, see [22] for instance. Another possible reason for the spike is that the IRS might have lost its effect due to mosquitos having become resistant to the insecticide [23]. Yet another possibility is that during the period of upsurge of malaria cases, there might have been a heavy influx of people who are symptomatically or asymptomatically infected with malaria. In this section of the paper we show how our model can assist in explaining the upsurge in terms of malaria imports and the waning of the effect of the insecticide. We utilise the model for estimating the average inflow over the period under the assumption that without the inflow, the relevant model parameters (all those other than the Ki-parameters) would have the same values as in the foregoing period. In the absence of data or sufficient information we tried a value for L that we hoped would work well. We have worked on an average density of 15 vectors per human.
Note that the parameters K1 and K2 are not listed in Table 2, because computing values for them is part of the computational exercise that follows.
Parameter | Description | Numerical value | Reference/comment |
δ | The rate of human deaths due to malaria | 0.00004858 per day | estimated from [23] |
μ | Mortality rate for humans, not including malaria-induced deaths | 0.017365 per day | [9] |
θ | Mortality rate for (vector) mosquitos | 0.04 per day | [3,24] |
K0 | Human birth rate | 3994200μ | [25] |
L | Birth rate of vector mosquitos | 60000000θ | Nominal |
a | The probability of a specific human getting bitten by a mosquito during a one-day period | 0.00000567 per person per day | estimated from [18] |
b | The probability of a bite by an infected mosquito leading to a new human infection | 0.075 | [26,27] |
c | The probability of a bite by an infected human leading to a new mosquito infection | 0.0375 | cf. [26,27] |
ρh | Transfer rate from Eh-class to Ih-class | 112 per day | [27,28] |
ρv | Transfer rate from Ev-class to Iv-class | 18 per day | [27,28] |
σ | Transfer rate from Ih-class to R-class (recovery rate) | 1180 per day | [27,28] |
γ1, γ2, γ3 | Rate of vector mortalities due to IRS | 0, 0.01, 0.01 per day | Estimates |
ζ | Transfer rate from R-class to S-class (rate of loss of temporary immunity) | 12×365 per day | [27,28] |
For the purposes of computation we use parameters in line with the paper [24]. This excludes of course the parameters pertaining to the population sizes. The essential computations cover two periods:
Period A: 01 January 1971 - 31 December 1995,
Period B: 01 January 1996 - 31 December 2001.
We assume that over the first period, Period A, the inflow of infected were insignificantly low whereas over the period B there was a significant inflow. During the 25 years of Period A, the annual number of cases were well below 14000. During Period B the number of malaria cases were well above 20000 p.a. and in one case even over 40000 p.a. Generally with compartmental models of ordinary differential equations it is assumed that the human population has a maximum size which is constant over time. For this reason we pick the end of 1995 as our reference date, and all the numbers referring to human population will be converted to the 1995 equivalents. We do these conversions before we calculate mean values to feed into the model. The South African population numbers over the entire period is obtained from Worldometer [29]. We assume that population growth over the malaria region was proportional to the national population growth of South Africa. In this manner, from [18] we calculate the mean number of malaria cases over Period A to be 5773 p.a. and over Period B it is 33451 p.a. The population at risk is calculated in a similar manner starting with its year-2000 value obtained from [25]. The 1995-converted value of the population at risk is 3.9942 million. The proportion of mortalities among the malaria cases is estimated from [23]. Using the data on Period A, through simulation we determine values of the parameters a and δ and we calculate numerical values of an equilibrium point that corresponds to an annual number of cases and mortalities as per the data. This equilibrium point is:
S∗=3973600; E∗h=190; I∗h=2800; R∗=10987;
V∗=59120000; E∗v=80440; I∗v=201150,
and it serves as the initial state for our computations over Period B.
Now we perform some computational analysis on Period B. We test the effect of varying the γi values, which are the additional rate of deaths in the vector population, induced by the IRS. The graphs are shown in Figures 2 and 3. Note that these two graphs are only exploring the effect of IRS on the malaria case numbers. We do not consider the inflow of infected migrants yet. The baseline values of γ1 and γ2 were taken as 0.01 of the compartment per day, and resulted in 5772 cases per year. Reduction of the γi values to 0.008, 0.005 or 0, respectively, yields 6203, 6940 or 8466 cases per year. The relevant Ih-trajectories appear in Figure 2. The Ih-trajectories over a longer period are shown in Figure 3, in order to give a view of how the curves will stabilize over time.
We now reach the highlight of this application. In what follows, we apply the model for estimating what was the average daily influx of infected migrants. Since the values of the γ-parameters are not known, we repeat this estimation process for different values of γ1 and γ2. In Figure 4 we show the results of combining a reduction in the γ values with increasing influx of infected in each case to obtain an average annual number of cases equal to 33451 as was observed in the data. The graphs of the Ih-compartments are shown in Figure 4 and the combinations are as in Table 2. For the same parameters as for Figure 4, in Figure 5 the Ih-trajectories are shown over a period longer than Period B, in order to illustrate how the curves split and eventually stabilize over time. These graphs reveal the dramatic effect that inflow of infectives have on a region where malaria is reasonably well under control. Our attention is now focused on Figure 4 and comparison with the observed data.
Let us consider the first scenario presented in Table 2, having γ1=γ2=0.01 per week. Then the model tells us that there must have been an average infux of less than 16 malaria infected migrants per day into the malaria region of South Africa. The precise number per year is 15.75×365=5749. The average annual number of observed cases during Period A was 5773 and during Period B it was 33451. This means that the inflow of 5749 migrants resulted in an additional number of cases equal to 33451−5773=27686 p.a. on average.
The common value of γ1 and γ2 | Daily inflow of imported cases |
0.01 | 15.75 |
0.0075 | 14.87 |
0.005 | 13.9 |
Now we calculate 27686/5749=4.816. This means that every infected immigrant caused 4 extra local infections (naively interpreted). This is valuable information revealed by the model.
For smaller values of γ1 and γ2, the corresponding influx of infected immigrants was also smaller. This is well expected, since the infected mosquitos now have a smaller mortality rate. Consequently the number of local cases per infected immigrant will now increase.
These graphs reveal the dramatic effect that inflow of infectives have on a region where malaria is reasonably well under control.
The model that we present in this study is of a completely new class. The model accommodates both inflow of infected migrants and outflow of the surviving recovered migrants. Application of such a model is versatile and the application in this paper is relatively simple. We have illustrated with an example, the process of estimating the rate of influx that was responsible for an observed number of malaria cases in the South African malaria region during the late 1990's. It is likewise possible to compute the expected number of cases that will arise from a given influx of infected migrants. One can equally well make future projections of local cases if the rate of importation of cases is known. Already there is an application to measles [11] in this regard. Our application gives fairly clear results on the effects of infective immigrants on malaria epidemiology in South Africa, even though the input date was limited only to annual case numbers. Accuracy of the results can be improved if the initial state of the system is known. In this case we simply use equilibrium values of the foregoing period as our initial state. Further improvements in the accuracy can be achieved by using a climate-driven model such as [9,10]. However in the latter case the mathematics around inflow and outflow of migrants will be much more complex, and climatic data or predictions will be required.
We are grateful to the Reviewers for all the constructive comments, which resulted in a much improved paper.
The authors have no competing interests.
[1] | World Health Organization, 2015. Global technical strategy for malaria 2016–2030, Available from: http://www.who.int/malaria/publications/atoz/9789241564991/en/. |
[2] |
N. Chitnis, A. Schapira, C. Schindler, M. A. Penny, T. A. Smith, Mathematical analysis to prioritise strategies for malaria elimination, J. Theor. Biol., 455 (2018), 118–130. doi: 10.1016/j.jtbi.2018.07.007
![]() |
[3] |
N. Chitnis, J. M. Hyman, J. M. Cushing, Determining important parameters in the spread of malaria through the sensitivity analysis of a mathematical model, Bull. Math. Bio., 70 (2008), 1272–1296. doi: 10.1007/s11538-008-9299-0
![]() |
[4] |
C. N. Ngonghala, M. I. Teboh-Ewungkem, G. A. Ngwa, Persistent oscillations and backward bifurcation in a malaria model with varying human and mosquito populations: implications for control, J. Math. Biol., 70 (2015), 1581–1622. doi: 10.1007/s00285-014-0804-9
![]() |
[5] |
G. A. Ngwa, W. S. Shu, A mathematical model for endemic malaria with variable human and mosquito populations, Math. Comput. Modelling, 32 (2000), 747–763. doi: 10.1016/S0895-7177(00)00169-2
![]() |
[6] |
I. Enahoro, S. Eikenberry, A. B. Gumel, S. Huijben, K. Paaijmans, Long-lasting insecticidal nets and the quest for malaria eradication: a mathematical modeling approach, J. Math. Biol., 81 (2020), 113–158. doi: 10.1007/s00285-020-01503-z
![]() |
[7] |
G. J. Abiodun, O. S. Makinde, A. M. Adeola, K. Y. Njabo, P. J. Witbooi, R. Djidjou-Demasse, et al., A Dynamical and Zero-Inflated Negative Binomial Regression Modelling of Malaria Incidence in Limpopo Province, South Africa, Int. J. Environ. Res. Public Health, 16 (2019), 2000. doi: 10.3390/ijerph16112000
![]() |
[8] | F. B. Agusto, N. Marcus, K. O. Okosun, Application of optimal control to the epidemiology of malaria, Electron. J. Differ. Equ., 81 (2012), 1–22. |
[9] | G. J. Abiodun, P. Witbooi, K. O. Okosun, Modelling the impact of climatic variables on malaria transmission, Hacettepe J. Math. Stat., 47 (2018), 219–235. |
[10] | M. Arquam, A. Singh, H. Cherifi, Impact of Seasonal Conditions on Vector-Borne Epidemiological Dynamics, IEEE Access, 8 (2020), 94510–94525. |
[11] | P. J. Witbooi, An SEIR model with infected immigrants and recovered emigrants, Adv. Differ. Equ., 1, (2021) 1–15. |
[12] | L. F. Lopez, M. Amaku, F. Antonio, B. Coutinho, M. Quam, M. N. Burattini, et al., Modeling Importations and Exportations of Infectious Diseases via Travelers, Bull. Math. Biol., 78 (2016), 185209. |
[13] |
A. Traore, Analysis of a vector-borne disease model with human and vectors immigration, J. Appl. Math. Comput., 64 (2020), 411–428. doi: 10.1007/s12190-020-01361-4
![]() |
[14] |
B. Dembele, A. Yakubu, Controlling imported malaria cases in the United States of America, Math. Biosci. Eng., 14 (2017), 95–109. doi: 10.3934/mbe.2017007
![]() |
[15] |
M. A. Khan, E. Bonyah, Z. Hammouch, E. Mifta Shaiful, A mathematical model of tuberculosis (TB) transmission with children and adults groups: a fractional model, AIMS Math., 5 (2020), 2813–2842. doi: 10.3934/math.2020181
![]() |
[16] |
F. Brauer, P. van den Driessche, Models for transmission of disease with immigration of infectives, Math. Biosci., 171 (2001), 143–154. doi: 10.1016/S0025-5564(01)00057-8
![]() |
[17] | R. P. Sigdel, P. Ram, C. McCluskey, Global stability for an SEI model of infectious disease with immigration, Appl. Math. Comput., 243 (2014), 684–689. |
[18] |
M. Coetzee, P. Kruger, R. H. Hunt, D. N. Durrheim, J. Urbach, C. F. Hansford, Malaria in South Africa: 110 years of learning to control the disease, S. Afr. Med. J., 103 (2013), 770–778. doi: 10.7196/SAMJ.7446
![]() |
[19] |
P. van den Driessche, J. Watmough, Reproduction numbers and sub-threshold endemic equilibria for compartmental models of disease transmission, Math. Biosci., 180 (2002), 29–48. doi: 10.1016/S0025-5564(02)00108-6
![]() |
[20] | X. Mao, Stochastic Differential Equations and Applications, Horwood, Chichester, 1997. |
[21] |
E. Tornatore, P. Vetro, S. M. Buccellato, SIVR epidemic model with stochastic perturbation, Neural Comput. Appl., 24 (2014), 309–315. doi: 10.1007/s00521-012-1225-6
![]() |
[22] | G. J. Abiodun, B. O. Adebiyi, R. O. Abiodun, O. Oladimeji, K. E. Oladimeji, A. M. Adeola, et al., Investigating the resurgence of malaria prevalence in South Africa between 2015 and 2018: A scoping review, Open Public Health J., 13 (2020), 119125. |
[23] | R. Maharaj, J. Raman, N. Morris, D. Moonasar, D. N. Durrheim, I. Seocharan, et al., Epidemiology of malaria in South Africa from control to elimination, S. Afr. Med. J., 203 (2013), 779–783. |
[24] | P. J. Witbooi, G. J. Abiodun, G. J. van Schalkwyk, I. H. I. Ahmed, Stochastic modeling of a mosquito-borne disease, Adv. Differ. Equ., 1 (2020), 1–15. |
[25] | World malaria report 2020. WHO. 2020 https://www.who.int/teams/global-malaria-programme/reports/world-malaria-report-2020/ |
[26] | P. M. Mwamtobe, S. Abelman, J. M. Tchuenche, A. Kasambara, Optimal control of intervention strategies for malaria epidemic in Karonga District, Malawi, Abstr. Appl. Anal., 30 (2014), ID 594256. |
[27] | G. Otieno, J. K. Koske, J. M. Mutiso, Transmission Dynamics and Optimal Control of Malaria in Kenya, Discrete Dyn. Nat. Soc., (2016), 8013574. |
[28] |
J. A. N. Filipe, E. M. Riley, C. J. Drakeley, C. J. Sutherland, A. C. Ghani, Determination of the processes driving the acquisition of immunity to malaria using a mathematical transmission model, PLoS Comput. Biol., 3 (2007), e255. doi: 10.1371/journal.pcbi.0030255
![]() |
[29] | Worldometer, South Africa Population (2021), available from: https://www.worldometers.info/world-population/south-africa-population/. |
1. | Juan Pablo Gutiérrez-Jara, Katia Vogt-Geisse, Maritza Cabrera, Collateral Effects of Insecticide-Treated Nets on Human and Environmental Safety in an Epidemiological Model for Malaria with Human Risk Perception, 2022, 19, 1660-4601, 16327, 10.3390/ijerph192316327 | |
2. | Pride Duve, Samuel Charles, Justin Munyakazi, Renke Lühken, Peter Witbooi, A mathematical model for malaria disease dynamics with vaccination and infected immigrants, 2023, 21, 1551-0018, 1082, 10.3934/mbe.2024045 | |
3. | Peter Joseph Witbooi, Gbenga Jacob Abiodun, Rajendra Maharaj, Modeling the effect of imported malaria on the elimination programme in KwaZulu-Natal province of South Africa, 2024, 47, 1937-8688, 10.11604/pamj.2024.47.80.35882 | |
4. | Akerejola, R. F., Elakhe, O. A., Isere, A. O., A Non-Standard Finite Difference Discretization Scheme Applied to a Malaria Model, 2024, 7, 2689-5323, 226, 10.52589/AJMSS-QRLVVI9E | |
5. | Rahat Zarin, Usa Wannasingha Humphries, Analyzing spatial diffusion and vaccination strategies in malaria epidemics: a numerical approach, 2025, 11, 2363-6203, 10.1007/s40808-025-02315-0 | |
6. | Arifa Samreen, Dumitru Baleanu, Souhaila Messaoudi, Salah Boulaaras, Sonia Akram, Mati ur Rahman, Modeling the dynamics of malaria with infected immigrants using fractal–fractional techniques with deep neural networks, 2025, 1561-8625, 10.1002/asjc.3641 |
K0 | Rate of inflow into the class of susceptible humans, |
K1 | Rate of inflow of latently infected humans, |
K2 | Rate of inflow of infectious humans, |
K3 | Rate of outflow of humans from the recovered class, |
μ | Mortality rate for humans, not including death directly due to malaria, |
δ | The rate of human deaths due to malaria, |
θ | Mortality rate of vectors, not including the effect of IRS, |
a | The probability of a specific human getting bitten by a mosquito during a one-day period, |
b | The probability that a bite by an infected mosquito will lead to a (new) human infection, |
c | The probability that a bite on an infected human will lead to a (new) mosquito infection, |
ρh | Transfer rate of humans from Eh-class to Ih-class, |
σ | Transfer rate of humans from Ih-class to R-class (recovery rate), |
ζ | Transfer rate from R-class to S-class (rate of loss of temporary immunity) |
ρv | Transfer rate of vectors from Ev-class to Iv-class, |
γ0 | Mortality rate due to IRS in class V, |
γ1 | Mortality rate due to IRS in class Ev, |
γ2 | Mortality rate due to IRS in class Iv. |
Parameter | Description | Numerical value | Reference/comment |
δ | The rate of human deaths due to malaria | 0.00004858 per day | estimated from [23] |
μ | Mortality rate for humans, not including malaria-induced deaths | 0.017365 per day | [9] |
θ | Mortality rate for (vector) mosquitos | 0.04 per day | [3,24] |
K0 | Human birth rate | 3994200μ | [25] |
L | Birth rate of vector mosquitos | 60000000θ | Nominal |
a | The probability of a specific human getting bitten by a mosquito during a one-day period | 0.00000567 per person per day | estimated from [18] |
b | The probability of a bite by an infected mosquito leading to a new human infection | 0.075 | [26,27] |
c | The probability of a bite by an infected human leading to a new mosquito infection | 0.0375 | cf. [26,27] |
ρh | Transfer rate from Eh-class to Ih-class | 112 per day | [27,28] |
ρv | Transfer rate from Ev-class to Iv-class | 18 per day | [27,28] |
σ | Transfer rate from Ih-class to R-class (recovery rate) | 1180 per day | [27,28] |
γ1, γ2, γ3 | Rate of vector mortalities due to IRS | 0, 0.01, 0.01 per day | Estimates |
ζ | Transfer rate from R-class to S-class (rate of loss of temporary immunity) | 12×365 per day | [27,28] |
The common value of γ1 and γ2 | Daily inflow of imported cases |
0.01 | 15.75 |
0.0075 | 14.87 |
0.005 | 13.9 |
K0 | Rate of inflow into the class of susceptible humans, |
K1 | Rate of inflow of latently infected humans, |
K2 | Rate of inflow of infectious humans, |
K3 | Rate of outflow of humans from the recovered class, |
μ | Mortality rate for humans, not including death directly due to malaria, |
δ | The rate of human deaths due to malaria, |
θ | Mortality rate of vectors, not including the effect of IRS, |
a | The probability of a specific human getting bitten by a mosquito during a one-day period, |
b | The probability that a bite by an infected mosquito will lead to a (new) human infection, |
c | The probability that a bite on an infected human will lead to a (new) mosquito infection, |
ρh | Transfer rate of humans from Eh-class to Ih-class, |
σ | Transfer rate of humans from Ih-class to R-class (recovery rate), |
ζ | Transfer rate from R-class to S-class (rate of loss of temporary immunity) |
ρv | Transfer rate of vectors from Ev-class to Iv-class, |
γ0 | Mortality rate due to IRS in class V, |
γ1 | Mortality rate due to IRS in class Ev, |
γ2 | Mortality rate due to IRS in class Iv. |
Parameter | Description | Numerical value | Reference/comment |
δ | The rate of human deaths due to malaria | 0.00004858 per day | estimated from [23] |
μ | Mortality rate for humans, not including malaria-induced deaths | 0.017365 per day | [9] |
θ | Mortality rate for (vector) mosquitos | 0.04 per day | [3,24] |
K0 | Human birth rate | 3994200μ | [25] |
L | Birth rate of vector mosquitos | 60000000θ | Nominal |
a | The probability of a specific human getting bitten by a mosquito during a one-day period | 0.00000567 per person per day | estimated from [18] |
b | The probability of a bite by an infected mosquito leading to a new human infection | 0.075 | [26,27] |
c | The probability of a bite by an infected human leading to a new mosquito infection | 0.0375 | cf. [26,27] |
ρh | Transfer rate from Eh-class to Ih-class | 112 per day | [27,28] |
ρv | Transfer rate from Ev-class to Iv-class | 18 per day | [27,28] |
σ | Transfer rate from Ih-class to R-class (recovery rate) | 1180 per day | [27,28] |
γ1, γ2, γ3 | Rate of vector mortalities due to IRS | 0, 0.01, 0.01 per day | Estimates |
ζ | Transfer rate from R-class to S-class (rate of loss of temporary immunity) | 12×365 per day | [27,28] |
The common value of γ1 and γ2 | Daily inflow of imported cases |
0.01 | 15.75 |
0.0075 | 14.87 |
0.005 | 13.9 |