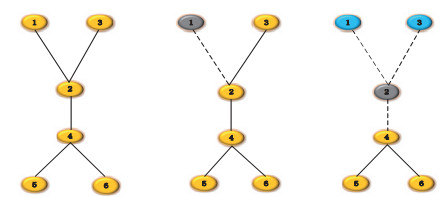
Citation: Mingli Lei, Daijun Wei. A measure of identifying influential community based on the state of critical functionality[J]. Mathematical Biosciences and Engineering, 2020, 17(6): 7167-7191. doi: 10.3934/mbe.2020368
[1] | Paula Mercurio, Di Liu . Identifying transition states of chemical kinetic systems using network embedding techniques. Mathematical Biosciences and Engineering, 2021, 18(1): 868-887. doi: 10.3934/mbe.2021046 |
[2] | Meili Tang, Qian Pan, Yurong Qian, Yuan Tian, Najla Al-Nabhan, Xin Wang . Parallel label propagation algorithm based on weight and random walk. Mathematical Biosciences and Engineering, 2021, 18(2): 1609-1628. doi: 10.3934/mbe.2021083 |
[3] | Huan-Ming Chuang, Shahab S. Band, Mehdi Sookhak, Kenneth Pinandhito . The value co-creation behavior in learning communities: Comparing conventional learning and e-learning. Mathematical Biosciences and Engineering, 2021, 18(6): 7239-7268. doi: 10.3934/mbe.2021358 |
[4] | Shaojun Zhu, Jinhui Zhao, Yating Wu, Qingshan She . Intermuscular coupling network analysis of upper limbs based on R-vine copula transfer entropy. Mathematical Biosciences and Engineering, 2022, 19(9): 9437-9456. doi: 10.3934/mbe.2022439 |
[5] | Sathyanarayanan Gopalakrishnan, Swaminathan Venkatraman . Prediction of influential proteins and enzymes of certain diseases using a directed unimodular hypergraph. Mathematical Biosciences and Engineering, 2024, 21(1): 325-345. doi: 10.3934/mbe.2024015 |
[6] | Biao Gao, Lin Huang . Toward a theory of smart media usage: The moderating role of smart media market development. Mathematical Biosciences and Engineering, 2021, 18(6): 7218-7238. doi: 10.3934/mbe.2021357 |
[7] | Hao Yuan, Qiang Chen, Hongbing Li, Die Zeng, Tianwen Wu, Yuning Wang, Wei Zhang . Improved beluga whale optimization algorithm based cluster routing in wireless sensor networks. Mathematical Biosciences and Engineering, 2024, 21(3): 4587-4625. doi: 10.3934/mbe.2024202 |
[8] | Changwei Gong, Bing Xue, Changhong Jing, Chun-Hui He, Guo-Cheng Wu, Baiying Lei, Shuqiang Wang . Time-sequential graph adversarial learning for brain modularity community detection. Mathematical Biosciences and Engineering, 2022, 19(12): 13276-13293. doi: 10.3934/mbe.2022621 |
[9] | Run Tang, Wei Zhu, Huizhu Pu . Event-triggered distributed optimization of multi-agent systems with time delay. Mathematical Biosciences and Engineering, 2023, 20(12): 20712-20726. doi: 10.3934/mbe.2023916 |
[10] | Miao Zhu, Tao Yan, Shijie Zhu, Fan Weng, Kai Zhu, Chunsheng Wang, Changfa Guo . Identification and verification of FN1, P4HA1 and CREBBP as potential biomarkers in human atrial fibrillation. Mathematical Biosciences and Engineering, 2023, 20(4): 6947-6965. doi: 10.3934/mbe.2023300 |
A community is made up of some nodes in the network, which is the subnetwork of the network. In the community, the nodes are highly agammaegated, and the internal connections are more complicated than others [1]. These communities have different sizes and topologies, and there are many uncertainty situations need to be measured [2]. The community structure has been considered in practical application, for example, the community vulnerability in critical infrastructures or natural disasters [3,4] and microbial community structures in waste water treatment plants or populations [5,6,7]. Based on the characteristics of community, community is widely studied by many researchers, such as the community vulnerability [3,8], the application of community structure [9,10], and community division [11,12,13]. With the research of complex network structure [14,15], as the subnetwork, the structure of the community plays an important role in the study of network. Thus, the community structure and community division attract the research interest of many scholars [16,17,18], especially for the community division algorithm. For example, The fast-newman algorithm (F−N) [19,20] and the label propagation algorithm (LPA) [21] are the classic two algorithms. Besides, Zhou et al. proposed a community division algorithm based on the graph clustering method [22]. Claudio et al. have given a simple Monte Carlo approach to evaluate the effects of multi-state links on community detection [23]. Based on the label propagation and fuzzy C-means, a complex network community detection algorithm is presented [13].
The community division algorithm is to study the community structure from the perspective of community division. Besides designing algorithms to study the structure of community, evaluation of the importance of the community is also very necessary for the network structure [24,25,26]. In Ref. [3], a value of relative vulnerability is defined. Community vulnerability is reflected by the number of links among communities. Recently, an improved vulnerability of community was proposed, the external and internal factors of the topology of communities are considered [27]. In Ref. [28], the weights of edges are considered by Sluban, et al. to define the internal influence of community Iin(C) and the influence of among communities Iout(C), respectively. Also, Ref. [29] renormalizing community as node to study the key community, communities are studied as nodes of networks.
On the one hand, community is the subnetwork of the network, the method of studying network is applied to research the structure of community. On the other hand, how to measure the influence of the community is not very clear. In order to understand the community structure influences on dynamical processes, Wu et al. presented a model with an adjustable cluster coefficient and degree of community [30]. Furthermore, some researches try to study the influence of community based on the k-core and designs some algorithms to search the maximum influence community. In Ref. [31], Li, et al. introduced a k-influential community based on the k-core, which is used to identify the influence of communities. In Ref. [32], based on k-core, the k-influential community, and the top-rk-influential community was introduced by Chen, et al. By removing the smallest weight node of the largest k-core, the k-influential community is obtained. The control parameter k is used to identify the influence of communities. The average weights of the community are used to measure the influence of the community (k influential community) and the index construction algorithm (ICA+) is used to search the top-r communities and their influence. Li, et al. found that the influence value of a community is defined as the minimum weight of the nodes in that community, and all of the largest k-influential communities were able to remove the node of the smallest weight in the largest k-core. Additionally, a linear threshold depth-first-search (DFS) algorithm is designed to look for top-rk-influential communities [33].
Based on the k-core, the k-influential community is found, however, it is only a local influential community. Only the communities whose k influence is found, the influence of all communities is not measured. As a part of network, studying the important nodes of network has guiding significance for the research of the community structure. There are many methods to identify influential nodes[34,35,36,37]. By extending basic centrality measures, several centrality measures are available for identifying influential nodes, such as semi-local centrality [38] and eigenvector centrality [39]. Some researchers also considered this problem based on algorithms to estimate the influence of nodes, such as the LeaderRank [40], the diffusion model [41], and so on [42,43]. Besides, Liu et al., proposed a generalized mechanic model to identify the importance nodes by considering the combination of local and global information of nodes [44]. The weighted degree decomposition had been used to identify the influence of nodes by Yang et al. [45]. In addition, on the basis of the structure similarity of nodes, the influential nodes are identified by Zhao et al. [46]. Bhatia et al., proposed a method, which names the state of critical functionality (SCF), to study the network recovery sequences and influential nodes [47]. The multi-local dimension was used to identify the influence of nodes by Wen et al. [48]. Some researchers also try to identify the influence of nodes from the perspective of community [17,49,50,51]. In Ref. [49], the community structure is considered to identify the influential nodes in social networks based on the LPA. Based on the community structure, the number and sizes of communities are considered in the community-based centrality (CbC) [50].
Although the community is a subnetwork of network, there are differences between the community and the network. The reason is that besides the internal structure of the community, there are also connections among communities. This makes it difficult to measure the influence of the community. Moreover, in real social networks, some communities are always heterogeneous. For example, the young student communicates with each other more frequently than elderly people. There is also heterogeneity in web pages, and different web pages have different clicks. The number of different species in the same region is also different and heterogeneous. It is difficult to discuss the influence of the community from the view of the topology or the size of the community. Though many scholars try to measure the influence of community, there is no uniform method to measure the influence of community. The Ref. [28] discussed the influence of the community from the view of the weights of edges within and outsider of communities, however, the structure of within community is not considered. Recently, based on the influence of nodes, the method of SCF is applied to judge the influence of the community [29]. In Ref. [29], based on the SCF method, the renormalize method is used to reveal the influence of community in complex networks, communities are considered for the network nodes.
In fact, there are two other factors for identifying the influential community. One is the strength of connection among communities, another is the topological structure within the community. By the SCF method, there is little information about community in the SCF method [29], we only know that which communities are connected. Therefore, to give a measure to identify the influence of community, inspired by the idea of studying communities as network nodes, in this paper, the community is treated as a entirety from a macro perspective, each community of complex network is treated as a node by renormalizing the original network. The WSCF method is proposed based on the SCF method. For the WSCF, not only the external connection of community but also the internal structure of community are considered. The strengths of the connection among communities are mainly reflected by the number of connected edges among them. For the topological property within the community, the cluster coefficient could well reflect the tightness of the structure, it is considered to measure the internal structure of the community. The influence of a community in complex networks can be revealed by the number of connections with other communities [3,52] and the information status within the community (cluster coefficient) [30,53]. And then, based on the WSCF, a quantitative measure is proposed to identify the influence of community. Meanwhile, the influence of the community in complex networks is judged from the values of WSCF. The SCF in Ref. [29] is a special case of WSCF. The influences of communities of three classic constructed networks (i.e., a Erodös-Rényi (ER) random network [54], a BA scale free network [55] and a small-word (SW) network [56]) and three real networks, namely the 9/11 terrorist network [57], a USAir network [58] and a PolBooks network [59], are measured by the proposed method, the influences of these communities are differentiated by the proposed method. The results reveal that the proposed method is effective in distinguishing the influence of community, which is not affected by the community division algorithms. The contributions of this paper is as follows,
● The communities are renormalized as the nodes of network.
● A weighted network is constructed by considering the cluster coefficient within community and the connection among communities.
● A quantitative measure to identify the influence of community is proposed, which is the weighted state of critical functionality (WSCF).
The paper is organized as follows: basic concepts are introduced in Section 2. In Section 3, we proposed a measure to identify influential communities in complex networks. In Section 4, the influences of the community of three constructed networks and three real networks are measured by the proposed method. The conclusion is drawn in Section 5.
Notation: N, E respectively represent the total number of nodes and edges of the network. Q stands for the modularity of community division. l signifies the number of communities, gs is the number of edges in the community s, ds denotes the degree of every node in the community s. SCFi represents the state of critical functionality of ith community. The TF represents the number of nodes in the renormalized complex network. FF stands for the number of nodes in the giant component after one node is removed from the network. Ci denotes the cluster coefficient of the node i. ⟨C⟩ represents the average cluster coefficient of the network. ki, Mi are respectively the degree of the node i and the number of edges between adjacent nodes of node i. WSCFi represents the weighted state of critical functionality of ith community. TW denotes the sum of the weights in the weighted complex network. FWi represents the sum of weights of the rest of nodes after the node i is removed from the weighted network. TC is the sum of the cluster coefficient of all communities. FCi stands for the sum of the cluster coefficients of the remaining connected nodes in the remaining network after the renormalization nodes are removed. I(α) represents the influence evaluation value of the community α.
In this section, the F−N algorithm [19,20], LPA algorithm [21], the state of critical functionality (SCF) [47] and the cluster coefficient [56] are described, respectively.
The F−N algorithm is proposed by Newman et al. to detect the community structure of complex networks [19,20]. In this method, a quantitative measure, which is denoted by Q to determine the community structure. The definition of Q is given as follows,
Q=l∑s=1(gsE−(ds2E)2). | (2.1) |
where l is the number of communities, gs is the number of edges in community s, ds is the degree of every node in community s, and E is the total number of edges in the network. All the nodes are in one single community in complex networks if the value of Q is equal to zero, i.e., the network has not community structure. Inversely, the community structure is strongly indicated when Q=1. The community structure of the network is determined by changing the value of Q, the rules are given as follows:
1) All nodes of the network are in one single community, i.e., each node is a community.
2) Communities i and j are randomly combined as a new community.
3) The value of Q and change of that (ΔQij) are recorded. Additionally the value of the highest ΔQij, the communities i and j are combined as one community.
4) The final network is obtained when ΔQij≤0. Otherwise, repeat the 2)-3) steps.
Raghavan et al. proposed a fast label propagation algorithm (LPA) based on information propagation among nodes within the community [21]. First, the unique label is given for very node. Second, the label of most frequently in the neighbor of the node is selected to update the label of the node. If the times of the label are the same, the label is selected randomly to replace the original label. Third, repeat the step of updating the label of the node until the label of each node is no longer change. Finally, all of the node which have the same label are classified as a community. The detailed algorithm steps are as follows,
1) Each node is given a label, that is, node 1 corresponds to label 1, node i corresponds to label i.
2) Each node looks for its neighbors and founds the label of the neighbors, the most frequent label in the neighbor of the node is selected to update the label of the node. When the times of the label are the same, the label is selected randomly to replace the original label.
3) Repeating step 2), until the labels of all nodes do not change. The same labels nodes are divided into the same community.
The advantage of the algorithm is that the convergence period is short, other parameters (the size and number of the community) are not needed to input in advance, no community indicators need to be calculated during the execution of the algorithm and the time complexity is nearly linear.
F−N algorithm is mainly modularized by the degree of nodes and the total number of edges of the network, and use the modularity to identify the detection of community. However, the LPA algorithm is semi-supervised learning, mainly to find the nearest neighbor of a node, making each node and most neighbors are in the same community. Therefore, there have different calculation procedures of the F−N and LPA algorithms. F−N algorithm reflected the results of community detection by the modularity Q, which made as many nodes as possible within the community, and the number of external nodes are as few as possible. When the greater the Q is, the better the community division result is, and the community is divided when the value of ΔQij is negative. While the community label is used to divide the community by LPA algorithm. The node's label is updated by finding the most frequently occurring label in the node's neighbor, and finally, the nodes of the same label are divided into a community.
The SCF method has been applied to studying errors of complex networks, identifying key players in social networks and analyzing the resilience of complex networks [47]. In this method, two variables are introduced, they are total functionality (TF) and fragmented functionality (FF), respectively. The TF represents the number of nodes in the renormalized network. FF is the number of nodes in the giant component after one node is removed from the network. According to the values of TF and FF, the value of SCF of the ith node is defined as follows,
SCFi=FFiTF. | (2.2) |
The value of SCFi is smaller, the influence of the corresponding community is greater.
For example, a network with 6 nodes and 5 edges is given and shown in Figure 1.
From Figure 1, the number of nodes of the original network is 6, we have, TF = 6. From the middle of Figure 1, the number of nodes in the structure of the remaining networks is 5 when node 1 is removed. According to the definition of FF and Eq (2.2), we have:
SCF1=56,SCF2=SCF4=12,SCF3=SCF5=SCF6=56. |
According to the SCF method, the results indicate that the influence of nodes 2 and 4 are equal and more important than other nodes.
The cluster coefficient of node i reflects the correlation between node i and its adjacent nodes, indicates the degree of cluster of the nodes. In an undirected network, the local cluster coefficient of a node reflects the closeness of its formation with adjacent nodes. Watts and Strogatz introduced the cluster coefficient to measure whether a network is the small world network [56]. The definition of cluster coefficient is given as follows,
Ci=MiC2ki=2Miki(ki−1). | (2.3) |
where ki, Mi are the degree of the node i and the number of edges of adjacent nodes of node i, respectively.
The degree of cluster of a network is always reflected by the average cluster coefficient, which is given as follows,
⟨C⟩=1NN∑i=1Ci. | (2.4) |
where N is the total number of nodes.
From Eqs (2.3) and (2.4), the cluster coefficient is a global index and it reflects the overall structure and viewpoint. More importantly, The cluster coefficient reflects the connection relationship among adjacent nodes of the network. For the density, it is a measure to reflect the sparseness of the number of edges and does not express the complete connection relationship among nodes. Comparing with other indexes, the cluster coefficient not only reflects the global index but also expresses the degree of node-to-node connection, which is very consistent with the goal of this paper to measure the influence of the community from the perspective of considering the connection. In this paper, the cluster coefficient is applied to measure the structure of within communities.
In this section, revealing the information within and among communities is ignored in the classical renormalization methods. Besides, an improved method is proposed based on the SCF, which is the weighted state of critical functionality (WSCF). The influence of the community could be measured by the WSCF.
Considered a given complex network G(N,E), where N=(1,2,⋯,n) is the set of nodes, E=(1,2,⋯,m) is the set of edges. m, n are the number of edges and nodes in network G, respectively. Supposing complex network G(N,E) is divided into k communities by the F−N algorithm. In the process of renormalization, each community is treated as a node. An example is given and shown in Figure 2. On the left of Figure 2, the original network has 25 nodes. By F−N algorithm, the original network is divided into 6 communities. According to the classical method to renormalize network, each community is treated as a node [29]. All edges connected with two communities are combined as one edge, a new renormalized network is obtained which is shown in the right of Figure 2.
As above described, each community is treated as a node. And there is only one edge between two communities, that is, these edges among communities have been merged, even if there are many edges. However, from the left of Figure 2, the number of edges between communities are different. For example, only one edge connected with community 5 and community 4, but community 2 and community 4 are connected by two edges.
Moreover, the topological property within the community is different. For example, communities 1 and 5 are a triangle, they are different from other communities. That is, the topological property within the community is not considered in the classical renormalization method [29]. Thus, some information of community is lost in the process of renormalization.
As above described, Ref. [29] only considered the node was removed, there are some drawbacks, especially when the network is renormalized. After all, the number of edges among communities and the edges within the communities are not taken into account, which causes the information of the original network to be lost after renormalizing. In this paper, based on the SCF, the number of links among communities is regarded as the weight of the edges in the renormalization progress, the topological property within the community is reflected by the local structure index of cluster coefficient of community. That is, information of the original network is included as much as possible. The WSCF method is proposed, based on the SCF. TW stands for the sum of information. FW represents the rest of the information. TC denotes the sum of structure stability. FC represents the rest of the structure stability. Therefore, the WSCF is given as follows,
WSCFi=FFiTF∙FWiTW∙FCiTC. | (3.1) |
where TW is the sum of weights in the weighted complex network. FWi represents the sum of weights of the rest of the nodes after node i is removed from the weighted network. TC is the sum of the cluster coefficient of all communities. FCi is the sum of the cluster coefficients of the remaining connected nodes in the remaining network after the renormalization nodes are removed. The WSCFi represents the value of WSCF of the corresponding node i.
For example, the original network in Figure 2 is renormalized and shown in the right of Figure 4. From Figure 4, the number of edges between communities 2 and 3 is two, thus, the weight of the edges between nodes 2 and 3 is two in the renormalized network.
For the cluster coefficient, there is a special case: If there is only one node in the community, the cluster coefficient of the corresponding community is supposed that the reciprocal of the sum of all the nodes of the network.
According to the renormalization method, a community can be regarded as one node. Meanwhile, the influence of the node in complex networks can be revealed by the SCF method [29]. From Eq (3.1), in WSCF, more information of community is considered. Inspired by the SCF method, a quantitative measure of the influence of the community is given as follows,
I(α)=1WSCFα, |
where I(α) represents the influence evaluation value of the community α, WSCFα is a function about the community α. The result is that the smaller the value of WSCFi, the greater the influence of the corresponding community. That is, the greater the value of the I(α), the influence of the community is bigger. To summarize, the whole steps of the proposed method are shown in Figure 3.
For the right of Figure 4, the renormalized network is a weighted network. The influence of the node of the renormalized network is measured by the WSCF. Nodes 1 and 2 are removed, which are shown in the left and right of Figure 5, respectively. The changes of weight and internal connection of each community are shown in Tables 1 and 2 after the community structure determined by the F−N and LPA algorithms. And the results of the detection community are shown in the left and right of Figure 6, respectively.
F−N | Community | FW/TW | FC/TC | SCF | Rank | WSCF | Rank |
1 | 0.86 | 0.78 | 0.83 | 2 | 0.56 | 4 | |
2 | 0.86 | 0.43 | 0.50 | 1 | 0.06 | 1 | |
3 | 0.71 | 0.81 | 0.83 | 2 | 0.48 | 3 | |
4 | 0.43 | 0.57 | 0.50 | 1 | 0.12 | 2 | |
5 | 0.43 | 0.86 | 0.83 | 2 | 0.61 | 5 | |
6 | 0.86 | 0.78 | 0.83 | 2 | 0.56 | 4 |
LPA | Community | FW/TW | FC/TC | SCF | Rank | WSCF | Rank |
1′ | 0.86 | 0.82 | 0.83 | 2 | 0.58 | 4 | |
2′ | 0.71 | 0.84 | 0.83 | 2 | 0.50 | 3 | |
3′ | 0.29 | 0.52 | 0.50 | 1 | 0.07 | 1 | |
4′ | 0.43 | 0.48 | 0.50 | 1 | 0.10 | 2 | |
5′ | 0.86 | 0.82 | 0.83 | 2 | 0.58 | 4 | |
6′ | 0.86 | 0.82 | 0.83 | 2 | 0.58 | 4 |
From the left of Figure 5, the sum of nodes is 6, that is, TF=6. The sum of links among communities is 7, we have TW=7. According to the Eq (2.4), the TC=23/5. Node 2 is removed from the renormalized network, node 2 and node 4 not connected, node 4 and node 5, node 4 and node 6 are connected, that is, FF2=3, and the sum of the rest of the weights of edges is 2, i.e., FW2=2, from Eqs (2.4) and (3.1), the FC2=2. According to Eq (3.1), the values of WSCF of nodes 1 and 2 are respectively given as follows,
WSCF1=56×67×1823=90161;WSCF2=12×27×1023=10161. |
Similarly, the values of WSCF for other nodes can be calculated, and the influences of these communities are shown in the last column of Tables 1 and 2. And that, the rank of community influential is measured by the SCF and WSCF, the results are shown in Table 1.
From Table 1, for F−N algorithm and SCF method, the six communities are divided into only two levels, i.e., SCF2=SCF4<SCF1=SCF3=SCF5=SCF6. The results reveal that the influence of community 2 and 4 is bigger than other communities, that is, the influence of community is 2=4>1=3=5=6. However, for the WSCF method, the six communities are divided into five levels. According to the value of WSCF, we have, WSCF2<WSCF4<WSCF3<WSCF1=WSCF6<WSCF5, which shows that the community 2 has the greatest influence, the influence of community 5 is the smallest.
From Table 2, using the LPA algorithm, the network is also divided into 6 communities too, but which is different from the F−N algorithm. From Figure 6, the yellow nodes originally located in communities 4 and 5, now they are respectively divided into communities 2′ and 4′. The original node in community 2, it makes the structure of community 2 of a triangle. After the node is divided into community 4′, although the structure of communities is changed, the connections between community 4′ and 5′ have increased. Similarly, for the nodes in community 4 are divided into community 2′, the connections between community 2′ and 4′ have increase and there's an extra a triangle structure in community 2′.
Using the proposed method to measure the influence of community, the 6 communities are divided into 4 levels. The results of SCF and WSCF are different. The value of WSCF is WSCF3′<WSCF4′<WSCF2′<WSCF1′=WSCF5′=WSCF6′. That is, the influence of community is 3′>4′>2′>1′=5′=6′, which shows that community 3′ has great influence than others. Compared with SCF and WSCF, the proposed method can be used to identify the influence of community even if different community detection algorithms are used. The results of F−N and LPA algorithm showed that the WSCF method has more detail and better discrimination than the SCF method. influence of that the node corresponds community is smaller.
In this section, the influences of the communities of three constructed networks (i.e., a Erodös-Rényi (ER) random network [54], a BA scale free network [55] and a small-word (SW) network [56]) and three real networks, including the 9/11 terrorist network [57], a USAir network [58] and a PolBooks network [59] are measured by the proposed method.
As the ER random network model had been proposed, it provides a method to study the network structure [54]. In addition, the networks of real world are usually random. BA scale free network is used to explain the generation mechanism of the power law, the network is a classic constructed network and it has the priority connection mechanism in the process of network generation [55]. Moreover, when the small-world characteristics of the network were discovered, many networks have been found that they have the small-world properties [56]. In summary, the three classic networks are applied to test the proposed method.
In this subsection, in order to simply test the proposed method, the communities structures of networks are only divided by the F−N algorithm, the influences of communities of three classic constructed networks including a Erodös-Rényi (ER) random network with 1000 nodes, a BA scale free network with 1825 nodes and a small-word (SW) network with 2000 nodes, are measured. The results are respectively listed in the Tables 3, 4 and 5.
ERnetwork | Community | SCF | FW/TW | FC/TC | WSCF | Rank |
1 | 0.86 | 0.58 | 0.97 | 0.48 | 3 | |
2 | 0.86 | 0.65 | 0.92 | 0.51 | 4 | |
3 | 0.86 | 0.56 | 0.96 | 0.462 | 2 | |
4 | 0.86 | 0.56 | 0.96 | 0.460 | 1 | |
5 | 0.86 | 0.86 | 0.76 | 0.56 | 6 | |
6 | 0.86 | 0.83 | 0.81 | 0.58 | 7 | |
7 | 0.86 | 0.96 | 0.63 | 0.52 | 5 |
BAnetwork | Community | SCF | FW/TW | FC/TC | WSCF | Rank |
1 | 0.94 | 0.62 | 0.98 | 0.57 | 1 | |
2 | 0.94 | 0.90 | 0.94 | 0.801 | 7 | |
3 | 0.94 | 0.71 | 0.97 | 0.65 | 2 | |
4 | 0.94 | 0.72 | 0.97 | 0.66 | 3 | |
5 | 0.94 | 0.87 | 0.95 | 0.78 | 6 | |
6 | 0.94 | 0.78 | 0.98 | 0.71 | 4 | |
7 | 0.94 | 0.95 | 0.95 | 0.85 | 15 | |
8 | 0.94 | 0.94 | 0.91 | 0.806 | 9 | |
9 | 0.94 | 0.92 | 0.93 | 0.803 | 8 | |
10 | 0.94 | 0.83 | 0.96 | 0.75 | 5 | |
11 | 0.94 | 0.94 | 0.93 | 0.82 | 11 | |
12 | 0.94 | 0.93 | 0.92 | 0.813 | 10 | |
13 | 0.94 | 0.98 | 0.93 | 0.86 | 16 | |
14 | 0.94 | 0.98 | 0.94 | 0.87 | 17 | |
15 | 0.94 | 0.97 | 0.91 | 0.83 | 12 | |
16 | 0.94 | 0.99 | 0.91 | 0.844 | 14 | |
17 | 0.94 | 0.98 | 0.91 | 0.839 | 13 |
SWnetwork | Community | SCF | FW/TW | FC/TC | WSCF | Rank |
1 | 0.75 | 0.26 | 0.77 | 0.15 | 1 | |
2 | 0.75 | 0.77 | 0.73 | 0.42 | 4 | |
3 | 0.75 | 0.27 | 0.76 | 0.16 | 2 | |
4 | 0.75 | 0.70 | 0.74 | 0.39 | 3 |
For the ER random networks with 1000 nodes, it is divided into 7 communities by the F−N algorithm. From Table 3, the influences of 7 communities are identified as only one degree by the SCF method. When using the proposed method, the communities are divided into 7 stages, the values of WSCF of these communities are different. That is, the influences of the 7 communities are different, the rank of 7 communities are shown in the last column.
From Table 4, the BA scale free network with 1825 nodes is divided into 17 communities by the F−N algorithm. The cluster coefficients and connections among communities are different. Using the SCF method to measure the influences of the 17 community, the influence of them is no different. However, when the constructions within and among communities are considered, the influences of these communities are distinguished. From the values of WSCF of Table 4, the values of the 17 communities are different, that is the influences of the 17 communities are separate by the proposed method. The results show that the proposed method is more effective than SCF in measuring the influence of community.
According to the F−N algorithm, the constructed 2000 nodes SW network is divided into 4 communities. From Table 5, the values of SCF of the 4 communities are the same, it is shown that the SCF method is not clearly separated the influences of the 4 communities. For the proposed method, the values of WSCF of the 4 communities are different, that is the influences of the 4 communities are separated, the rank of the influences of the 4 communities is shown in the last column of Table 5.
From the results of the three constructed networks, the proposed method has a better effect than SCF in measuring the influence of the community, and the discrimination is higher. When the community structure is divided, the influence of the community could be measured by the WSCF method. To further verify the effectiveness of the proposed method, the community influential of the three real networks was measured, which will be introduced in the next subsections
The 9/11 terrorist network with 62 nodes and 153 links is described by Krebs [57]. These nodes represent persons, edges represent the existence of acquaintance. The 9/11 terrorist network is verified as 5 communities by the F−N algorithm (Q=0.52) and shown in Figure 7.
Using Eq (3.1), the values of WSCF of communities are calculated as shown in column 5 of Table 6. According to the values of WSCF, these communities have been divided into five levels by using the F−N algorithm to detect community. The results are shown in column 6 of Table 6.
F−N | Community | SCF | FW/TW | FC/TC | WSCF | Rank |
1 | 0.8 | 0.90 | 0.79 | 0.57 | 5 | |
2 | 0.8 | 0.88 | 0.80 | 0.56 | 4 | |
3 | 0.8 | 0.40 | 0.78 | 0.25 | 2 | |
4 | 0.8 | 0.50 | 0.85 | 0.34 | 3 | |
5 | 0.8 | 0.31 | 0.78 | 0.19 | 1 |
Form Table 6, the values of SCF of 5 communities are the same, that is the influences of community 1, 2, 3, 4 are the same as 5, the method of SCF cannot distinguish the influence of each community. However, when using the WSCF method the influence of community 5 is bigger than other communities. The influences of these communities are different. The ranking of influence of communities is 5>3>4>2>1, that is, community 5 is more important than others. In fact, from Figure 8, the weight of node 5 is 29, which is the biggest within the renormalization network. And from 4th column of Table 6, the cluster coefficient of community 5 is bigger than others, community 5 is the greatest influence than others.
However, the network is only divided into 3 communities when using the LPA algorithm, the results are shown in Table 7. Using the SCF method, the values of each community are the same. According to Eq (3.1), the values of WSCF and the influence of the community are shown in Table 8. From Table 8, the values of WSCF are divided into three levels, detailed results are shown in 6th column of Table 8. From Table 8, the influence of community is 2′>3′>1′, that is, community 2′ have great influence than others.
Communities | Nodes |
1′ | 1, 2, 3, 4, 5, 6, 7, 8, 9, 10, 11, 12 |
2′ | 13, 14, 15, 16, 17, 18, 19, 20, 21, 22 |
3′ | 23, 24, 25, 26, 27, 28, 29, ..., 60, 61, 62 |
LPA | Community | SCF | TW/TW | FC/TC | WSCF | Rank |
1′ | 0.67 | 0.43 | 0.64 | 0.18 | 3 | |
2′ | 0.67 | 0.29 | 0.66 | 0.126 | 1 | |
3′ | 0.67 | 0.29 | 0.69 | 0.131 | 2 |
In fact, node 32 is a hub node and the closeness is biggest, node 57 has the greatest betweenness [57]. In Ref. [57], many terrorists are associated with node 32 MohamedAtta which was the ring leader of this conspiracy, and many terrorist organizations communicate with each other must through node 57 NawafAlhazmi. From figure 8, node 32 in community 3 which located in the center of five communities. By using the proposed method to measure the influence of community, the ranking of influence of community 3 is the second. Node 57 is located in community 5, and community 5 has the greatest influence. When using the LPA algorithm to divide the community, the network is divided into 3 communities, node 57 is divided into community 3. Using the proposed method to measure the influence of communities, the ranking of influence of community 3 is the second. Node 32 is located in community 3, and community 3 was identified as the second most influential. MohamedAtta and NawafAlhazmiIn are the most harmful in terrorist organizations [57]. In summary, the proposed method is a feasible and effective measure to identify influential communities, the recognition effect is better than SCF method.
The USAir network has 332 nodes and 2126 links. These nodes represent positions and edges represent the airlines of every two positions [58]. The USAir network is divided into 7 communities by the F−N algorithm (Q=0.3190) as shown in Figure 9.
These communities of the USAir network are renormalized as nodes and are shown in Figure 10. Using WSCF, the changes of weight and cluster coefficient are calculated, respectively, which are shown in Table 9.
F−N | Community | SCF | FW/TW | FC/TC | WSCF | Rank |
1 | 0.86 | 0.97 | 0.86 | 0.72 | 6 | |
2 | 0.71 | 0.07 | 0.72 | 0.04 | 1 | |
3 | 0.86 | 0.96 | 0.84 | 0.69 | 3 | |
4 | 0.86 | 0.08 | 0.86 | 0.06 | 2 | |
5 | 0.86 | 0.99 | 0.82 | 0.70 | 4 | |
6 | 0.86 | 0.93 | 0.89 | 0.71 | 5 | |
7 | 0.86 | 1.00 | 0.86 | 0.73 | 7 |
From Table 9, the SCF method divides the 7 communities into 2 levels. According to Eq (3.1), the values of WSCF are obtained and shown in 5th column of Table 9 by the F−N algorithm. In order to identify the influence of every community, the values of WSCF are sorted, the result is shown in 6th column of Table 9.
From Table 9, these communities are divided into 7 levels, which are as shown in the 6th column of Table 9, and the influence of community 2 is the biggest. The value of WSCF is WSCF2<WSCF4<WSCF3<WSCF5<WSCF6<WSCF1<WSCF7, i.e., the influence of community is 2>4>3>5>6>1>7, that is, the influence of community 2 is biggest, community 7 is minimum. From Figure 10, the degree of node 2 is the biggest, and from the 4th column of Table 9, the cluster coefficient of community 2 is also greatest, community 2 is greater influence than others.
However, using the LPA algorithm, the network is divided into 8 communities 3 levels by the SCF method, the results are shown in the 3rd column of Table 10. According to Eq (3.1), the value of WSCF is calculated, which is shown in Table 10. From Table 10, the value of WSCF is WSCF7′<WSCF5′<WSCF3′<WSCF2′<WSCF1′<WSCF4′<WSCF6′<WSCF8′, that is, the influence of community is 7′>5′>3′>2′>1′>4′>6′>8′, community 7′ is more important than others. From the 4th and 5th columns of Table 10, the weight and cluster coefficient of community 7 is the biggest, the community 8 is contrary to community 7, the influence of community 7 is biggest, community 8 is the smallest.
LPA | Community | SCF | FW/TW | FC/TC | WSCF | Rank |
1′ | 0.875 | 0.89 | 0.85 | 0.66 | 5 | |
2′ | 0.875 | 0.63 | 0.88 | 0.48 | 4 | |
3′ | 0.75 | 0.87 | 0.72 | 0.47 | 3 | |
4′ | 0.875 | 0.93 | 0.85 | 0.69 | 6 | |
5′ | 0.875 | 0.57 | 0.87 | 0.43 | 2 | |
6′ | 0.875 | 0.87 | 0.93 | 0.71 | 7 | |
7′ | 0.875 | 0.26 | 0.63 | 0.10 | 1 | |
8′ | 0.63 | 0.98 | 0.88 | 0.75 | 8 |
For the USAir network, in the results of community division, some communities may have many nodes within the community. When the USAir network is divided by the F−N algorithm, the community 4 has 150 nodes, which indicated that the airport in community 4 is closer contact with other airports. Such as node 118 (“Chicago O'Hare Intl”), it has 40 links with other nodes. In fact, until 1998, “Chicago O'Hare Intl” was the busiest airport in the world in terms of the number of passengers https://en.wikipedia.org/wiki/O%5C04527Hare_International_Airport. In Ref. [27], community 4 has the greatest influence. For the proposed method, the influential rank of community 4 is the second. Using the LPA algorithm to divide the community, the USAir network is divided into 8 communities, node 118 located in community 7′, the influence of community 7′ is biggest by the proposed method to measure the influence of community. The results show that our method is effective and feasible, it has higher recognition and better recognition effect than SCF method.
PolBooks network was constructed by Valdis Krebs [59]. Nodes represent the books of US politics which sold by the online bookseller Amazon.com, and edges are the frequent co-purchasing of books by the same buyers, who bought this book also bought other books feature on Amazon [59]. It is constructed by 105 nodes and 441 edges, which is shown in Figure 11. While the network community structure is divided by the F−N algorithm, the renormalized network is shown in Figure 12. By the F−N and LPA algorithms, the network is divided into some communities, and some variables of WSCF are calculated, they are shown in Tables 11 and 12, respectively.
F−N | Community | SCF | FW/TW | FC/TC | WSCF | Rank |
1 | 0.75 | 0.36 | 0.81 | 0.22 | 1 | |
2 | 0.75 | 0.44 | 0.80 | 0.27 | 2 | |
3 | 0.75 | 0.69 | 0.64 | 0.28 | 3 | |
4 | 0.75 | 0.50 | 0.75 | 0.33 | 4 |
LPA | Community | SCF | FW/TW | FC/TC | WSCF | Rank |
1′ | 0.75 | 0.55 | 0.73 | 0.30 | 4 | |
2′ | 0.75 | 0.43 | 0.79 | 0.25 | 1 | |
3′ | 0.75 | 0.55 | 0.69 | 0.29 | 3 | |
4′ | 0.75 | 0.48 | 0.79 | 0.28 | 2 |
For the F−N algorithm, by Eq (3.1), the values of WSCF are obtained, which are shown in Table 11. From Table 11, the value of WSCF is WSCF1<WSCF2<WSCF3<WSCF4, i.e., the influence of community is 1>2>3>4, that is the influence of community 1 is biggest. When using the SCF method, the influences of the 4 communities are the same, they are not distinguished.
However, for the LPA algorithm, the values of SCF of each community are the same, that is, the influences of these communities are not distinguished. Using Eq (3.1), the value of WSCF is obtained, which is shown in Table 12. From Table 12, the value of WSCF is WSCF2′<WSCF4′<WSCF3′<WSCF1′, that is, the influence of community is 2′>4′>3′>1′, i.e., the influence of community 2′ is bigger than others.
By analyzing the PolBooks network, we find that nodes 8 (“A National Party No More”) and 12 (“Off with Their Heads”) have the most links with other nodes and they are hub nodes. It shows that these two books were read by many people during the US presidential election. Both of the two books are about politics. From Figure 12, the degree of the renormalized network is the biggest. Moreover, through Figure 10, the cluster coefficients of nodes 56, 49, 9, 19 are large. Two algorithms divide the network into four communities. After the community is divided by the F−N algorithm, the nodes 8, 12, 19, 49 and 56 are located in community 1, using the proposed method to measure the influence of community, find that community 1 has the greatest influence. After dividing community by the LPA algorithm, nodes 8, 12, 19, 49 and 56 are located in community 2, and it is found that community 2 is also the most influential.
Different community detection algorithm has different results, although the community is different, the values of WSCF always could be applied to measure the influence of the community and the recognition effect is better than SCF method.
In this paper, the weighted state of critical functionality (WSCF) method is proposed and a quantitative measure I(α) is proposed to identify the influence of community. For the WSCF method, not only the edges among communities but also the cluster coefficient within the communities are taken into account in the process of renormalization. Based on the WSCF method, each community has a value of I(α). The bigger the value of I(α), the greater the influence of the corresponding community. To test the proposed method, the communities influences of three constructed networks (i.e., a Erodös-Rényi (ER) random network, a BA scale free network and a small-word (SW) network) and three real networks, including the 9/11 terrorist network, a USAir network, and a PolBooks network, are measured by the proposed method. The results show that the WSCF method has better recognition than SCF method, and the SCF is a special case of WSCF, when the weights of edges and cluster coefficients are equal to 1.
The proposed method has the practical application value for measuring the influences of communities. For example, for a terrorist organization network, identifying key communities in the network and attacking the corresponding terrorist organization, it is a useful method to reduce the risk of terrorist attacks. For the economic network, by measuring the influences of various communities and monitoring the high-risk communities among them, it is beneficial to economic regulation. For the power network, measuring the influences of communities, find out the more influential community, and monitoring this part will reduce the occurrence of power failure.
Because the proposed method considers a few factors, the final value of WSCF is relatively close. When the number of the community is large, the recognition of the proposed method may be affected. However, the proposed method is not affected by the community division algorithm. Once the community structure of the network is determined, the influence of the corresponding community could be measured by the proposed method and the recognition is higher than the SCF method.
Although the proposed method could be used to measure the influences of communities, this paper also has theoretical defects. On the one hand, we selected several important indicators to reflect the structures inside and outside the community. However, only a few indexes are considered in the method, many other indicators could be combined to measure the community structure, such as the similarity among communities, the strength of links within the community, etc. On the other hand, the method of considering additional factors is not comprehensive, and it is impossible to consider all circumstances. Therefore, in the next work, we will learn from the method of identifying influential nodes, design the algorithm, and use the Susceptible-Infected-Recovered (SIR) model to verify it from the perspective of numerical simulation.
The work described in this paper is partially supported by the National Natural Science Foundation of China (Grant Nos.61763009 and 61364030).
The authors declare there is no conflict of interest in this paper.
[1] | A. Clauset, M. E. J. Newman, C. Moore, Finding community structure in very large networks, Phys. Rev. E, 70 (2004), 066111. |
[2] | Y. Deng, Uncertainty measure in evidence theory, Sci. China Inf. Sci., 64 (2021), http://10.1007/s11432--020--3006--9. |
[3] | J. Ramirez Marquez, M. Claudio, Vulnerability metrics and analysis for communities in complex networks, Reliab. Eng. Syst. Saf., 96 (2011), 1360-1366. |
[4] | Y. Zhang, M. K. Lindell, C. S. Prater, Vulnerability of community businesses to environmental disasters, Disasters, 33 (2009), 38-57. |
[5] | M. Hu, X. Wang, X. Wen, Y. Xia, Microbial community structures in different wastewater treatment plants as revealed by 454-pyrosequencing analysis, Bioresour. Technol., 117 (2012), 72-79. |
[6] | A. Tyakht, E. Kostryukova, A. Popenko, M. Belenikin, A. Pavlenko, A. Larin, et al., Human gut microbiota community structures in urban and rural populations in russia, Nat. Commun., 4 (2014), 1-9. |
[7] | O. Koren, D. Knights, A. Gonzalez, L. Waldron, N. Segata, R. Knight, et al., A guide to enterotypes across the human body: meta-analysis of microbial community structures in human microbiome datasets, PLOS Comput. Biol., 9 (2013), e1002863. |
[8] | B. H. Morrow, Identifying and mapping community vulnerability, Disasters, 23 (1999), 1-18. |
[9] | M. Girvan, M. E. J. Newman, Community structure in social and biological networks, Proc. Natl. Acad. Sci. USA, 99 (2002), 7821-7826. |
[10] | P. M. Gleiser, L. Danon, Community structure in jazz, Adv. Complex Syst., 6 (2003), 565-573. |
[11] | M. E. J. Newman, M. Girvan, Finding and evaluating community structure in networks, Phys. Rev. E, 69 (2004), 026113. |
[12] | S. Srinivas, C. Rajendran, Community detection and influential node identification in complex networks using mathematical programming, Expert Syst. Appl., 135 (2019), 296-312. |
[13] | Z. H. Deng, H. H. Qiao, Q. Song, L. Gao, A complex network community detection algorithm based on label propagation and fuzzy c-means, Physica A, 519 (2019), 217-226. |
[14] | F. Liu, Y. Deng, A fast algorithm for network forecasting time series, IEEE Access, 7 (2019), 102554-102560. |
[15] | J. Zhao, H. Mo, Y. Deng, An efficient network method for time series forecasting based on the DC algorithm and visibility relation, IEEE Access, 8 (2020), http://10.1109/ACCESS.2020.2964067. |
[16] | L. C. Freeman, A set of measures of centrality based on betweenness, Sociometry, 40 (1977), 35-41. |
[17] | Z. Ghalmane, C. Cherifi, H. Cherifi, M. E. Hassouni, Centrality in complex networks with overlapping community structure, Sci. Rep., 9 (2019), 10133. |
[18] | H. Cherifi, G. Palla, B. K. Szymanski, X. Lu, On community structure in complex networks: challenges and opportunities, Appl. Netw. Sci., 4 (2019), https://doi.org/10.1007/s41109-019-0238-9. |
[19] | M. E. J. Newman, Fast algorithm for detecting community structure in networks, Phys. Rev. E, 69 (2004), 066133. |
[20] | M. E. J. Newman, Modularityand community structure in networks, Proc. Natl. Acad. Sci. USA, 103 (2006), 8577-8582. |
[21] | U. N. Raghavan, R. Albert, S. Kumara, Near linear time algorithm to detect community structures in large-scale networks, Phys. Rev. E, 76 (2007), 036106. |
[22] | H. F. Zhou, J. Li, J. H. Li, F. C. Zhang, Y. A. Cui, A graph clustering method for community detection in complex networks, Physica A, 469 (2017), 551-562. |
[23] | C. M. Rocco, J. Moronta, J. E. Ramirez-Marquez, K. Barker, Effects of multi-state links in network community detection, Reliab. Eng. Syst. Saf., 163 (2017), 46-56. |
[24] | G. Cantin, C. J. Silva, Influence of the topology on the dynamics of a complex network of hiv/aids epidemic models, Math. Biosci. Eng., 4 (2019), 1145-1169. |
[25] | M. Liu, S. He, Y. Sun, The impact of media converge on complex networks on disease transmission, Math. Biosci. Eng., 16 (2019), 6335-6349. |
[26] | Z. Zhuang, Z. Lu, Z. Huang, C. Liu, W. Qin, A novel complex network based dynamic rule selection approach for open shop scheduling problem with release dates, Math. Biosci. Eng., 16 (2019), 4491-4505. |
[27] | D. Wei, X. Zhang, S. Mahadevan, Measuring the vulnerability of community structure in complex networks, Reliab. Eng. Syst. Saf., 174 (2018), 41-52. |
[28] | B. Sluban, J. Smailović, S. Battiston, I. Mozetič, Sentiment leaning of influential communities in social networks, Comput. Soc. Networks, 2 (2015), 9-29. |
[29] | M. Lei, D. Wei, Identifying influence for community in complex networks, IEEE 30th CCDC, (2018), 5346-5349. |
[30] | X. Wu, Z. Liu, How community structure influences epidemic spread in social networks, Physica A, 387 (2008), 623-630. |
[31] | R. H. Li, L. Qin, J. X. Yu, R. Mao, Influential community search in large networks, Proc. VlDB Endow., 8 (2015), 509-520. |
[32] | W. Chen, J. Liu, Z. Chen, J. Chen, A top-r k influential community search algorithm., Int. J. Performability Eng., 14 (2018), 2652-2662. |
[33] | R. H. Li, L. Qin, J. X. Yu, R. Mao, Finding influential communities in massive networks, VLDB J., 26 (2017), 751-776. |
[34] | J. Zhao, Y. Song, Y. Deng, A novel model to identify the influential nodes: Evidence Theory Centrality, IEEE Access, 8 (2020), 46773-46780. |
[35] | S. Oldham, B. Fulcher, L. Parkes, A. Arnatkevicit, A. Fornito, Consistency and differences between centrality measures across distinct classes of networks, PloS One, 14 (2019), 1-23. |
[36] | J. Zhao, Y. Wang, Y. Deng, Identifying influential nodes in complex networks from global perspective, Chaos Solitons Fract., 133 (2020), 109637. |
[37] | M. Perc, O. Peek, S. M. Kamal, Impact of density and interconnectedness of influential players on social welfare, Appl. Math. Comput., 249 (2014), 19-23. |
[38] | D. Chen, L. Lü, M. Shang, Y. Zhang, T. Zhou, Identifying influential nodes in complex networks, Physica A, 391 (2012), 1777-1787. |
[39] | P. Bonacich, P. Lloyd, Eigenvector-like measures of centrality for asymmetric relations, Soc. Networks, 23 (2001), 191-201. |
[40] | L. Lü, Y. Zhang, C. Yeung, T. Zhou, Leaders in social networks, the delicious case, PloS One, 6 (2011), 1-9. |
[41] | D. Kempe, J. Kleinberg, E. Tardos, Influential nodes in a diffusion model for social networks, Lect. Notes Comput. Sci., 3580 (2005), 1127-1138. |
[42] | M. Kimura, K. Saito, Tractable models for information diffusion in social networks, ECML PKDD, 4231 (2006), 259-271. |
[43] | M. Kimura, K. Saito, R. Nakano, H. Motoda, Extracting influential nodes on a social network for information diffusion, Data Min. Knowl. Discov., 20 (2010), 70-97. |
[44] | F. Liu, Z. Wang, Y. Deng, Gmm: A generalized mechanics model for identifying the importance of nodes in complex networks, Knowl. Based Syst., 193 (2020), 105464. |
[45] | G. Yang, T. P. Benko, M. Cavaliere, J. Huang, M. Perc, Identification of influential invaders in evolutionary populations, Sci. Rep., 9 (2019), 7305-7317. |
[46] | J. Zhao, Y. Song, F. Liu, Y. Deng, The identification of influential nodes based on structure similarity, Conn. Sci., 32 (2020), 1-18. |
[47] | U. Bhatia, D. Kumar, E. Kodra, A. R. Ganguly, Network science based quantification of resilience demonstrated on the indian railways network, Plos One, 10 (2015), 1-17. |
[48] | T. Wen, D. Pelusi, Y. Deng, Vital spreaders identification in complex networks with multi-local dimension, Knowl.Based Syst., 195 (2020), 105717. |
[49] | Y. Zhao, S. Li, F. Jin, Identification of influential nodes in social networks with community structure based on label propagation, Neurocomput., 210 (2016), 33-44. |
[50] | Z. Zhao, X. Wang, W. Zhang, Z. Zhu, A community-based approach to identifying influential spreaders, Entropy, 17 (2015), 2228-2252. |
[51] | Z. Ghalmane, M. E. Hassouni, C. Cherifi, H. Cherifi, Centrality in modular networks, EPJ Data Sci., 8 (2019), 15. |
[52] | J. E. Ramirez-Marquez, C. M. Rocco, K. Barker, J. Moronta, Quantifying the resilience of community structures in networks, Reliab. Eng. Syst. Saf., 169 (2018), 466-474. |
[53] | P. Zhang, J. Wang, X. Li, M. Li, Z. Di, Y. Fan, Clustering coefficient and community structure of bipartite networks, Physica A, 387 (2008), 6869-6875. |
[54] | R. A. Erdös P, On the evolution of random graphs, Publ. Math. Inst. Hung. Acad. Sci., 5 (1960), 15-27. |
[55] | A. L. Barabási, R. Albert, Emergence of scaling in random networks, Science, 286 (1999), 509-512. |
[56] | D. J. Watts, S. H. Strogatz, Collective dynamics of small-world networks, Nature, 393 (1998), 440-442. |
[57] | V. E. Krebs, Mapping networks of terrorist cells, Connections, 24 (2002), 43-52. |
[58] | V. Batagelj, A. Mrvar, Pajek datasets, 2006. Available from: http://vlado.fmf.uni-lj.si/pub/networks/data/mix/USAir97.net. |
[59] | V. E. Krebs, Unpublished, 2007. Available from: http://www.orgnet.com/. |
1. | Fu Tan, Bing Wang, Daijun Wei, A new structural entropy measurement of networks based on the nonextensive statistical mechanics and hub repulsion, 2021, 18, 1551-0018, 9253, 10.3934/mbe.2021455 |
F−N | Community | FW/TW | FC/TC | SCF | Rank | WSCF | Rank |
1 | 0.86 | 0.78 | 0.83 | 2 | 0.56 | 4 | |
2 | 0.86 | 0.43 | 0.50 | 1 | 0.06 | 1 | |
3 | 0.71 | 0.81 | 0.83 | 2 | 0.48 | 3 | |
4 | 0.43 | 0.57 | 0.50 | 1 | 0.12 | 2 | |
5 | 0.43 | 0.86 | 0.83 | 2 | 0.61 | 5 | |
6 | 0.86 | 0.78 | 0.83 | 2 | 0.56 | 4 |
LPA | Community | FW/TW | FC/TC | SCF | Rank | WSCF | Rank |
1′ | 0.86 | 0.82 | 0.83 | 2 | 0.58 | 4 | |
2′ | 0.71 | 0.84 | 0.83 | 2 | 0.50 | 3 | |
3′ | 0.29 | 0.52 | 0.50 | 1 | 0.07 | 1 | |
4′ | 0.43 | 0.48 | 0.50 | 1 | 0.10 | 2 | |
5′ | 0.86 | 0.82 | 0.83 | 2 | 0.58 | 4 | |
6′ | 0.86 | 0.82 | 0.83 | 2 | 0.58 | 4 |
ERnetwork | Community | SCF | FW/TW | FC/TC | WSCF | Rank |
1 | 0.86 | 0.58 | 0.97 | 0.48 | 3 | |
2 | 0.86 | 0.65 | 0.92 | 0.51 | 4 | |
3 | 0.86 | 0.56 | 0.96 | 0.462 | 2 | |
4 | 0.86 | 0.56 | 0.96 | 0.460 | 1 | |
5 | 0.86 | 0.86 | 0.76 | 0.56 | 6 | |
6 | 0.86 | 0.83 | 0.81 | 0.58 | 7 | |
7 | 0.86 | 0.96 | 0.63 | 0.52 | 5 |
BAnetwork | Community | SCF | FW/TW | FC/TC | WSCF | Rank |
1 | 0.94 | 0.62 | 0.98 | 0.57 | 1 | |
2 | 0.94 | 0.90 | 0.94 | 0.801 | 7 | |
3 | 0.94 | 0.71 | 0.97 | 0.65 | 2 | |
4 | 0.94 | 0.72 | 0.97 | 0.66 | 3 | |
5 | 0.94 | 0.87 | 0.95 | 0.78 | 6 | |
6 | 0.94 | 0.78 | 0.98 | 0.71 | 4 | |
7 | 0.94 | 0.95 | 0.95 | 0.85 | 15 | |
8 | 0.94 | 0.94 | 0.91 | 0.806 | 9 | |
9 | 0.94 | 0.92 | 0.93 | 0.803 | 8 | |
10 | 0.94 | 0.83 | 0.96 | 0.75 | 5 | |
11 | 0.94 | 0.94 | 0.93 | 0.82 | 11 | |
12 | 0.94 | 0.93 | 0.92 | 0.813 | 10 | |
13 | 0.94 | 0.98 | 0.93 | 0.86 | 16 | |
14 | 0.94 | 0.98 | 0.94 | 0.87 | 17 | |
15 | 0.94 | 0.97 | 0.91 | 0.83 | 12 | |
16 | 0.94 | 0.99 | 0.91 | 0.844 | 14 | |
17 | 0.94 | 0.98 | 0.91 | 0.839 | 13 |
SWnetwork | Community | SCF | FW/TW | FC/TC | WSCF | Rank |
1 | 0.75 | 0.26 | 0.77 | 0.15 | 1 | |
2 | 0.75 | 0.77 | 0.73 | 0.42 | 4 | |
3 | 0.75 | 0.27 | 0.76 | 0.16 | 2 | |
4 | 0.75 | 0.70 | 0.74 | 0.39 | 3 |
F−N | Community | SCF | FW/TW | FC/TC | WSCF | Rank |
1 | 0.8 | 0.90 | 0.79 | 0.57 | 5 | |
2 | 0.8 | 0.88 | 0.80 | 0.56 | 4 | |
3 | 0.8 | 0.40 | 0.78 | 0.25 | 2 | |
4 | 0.8 | 0.50 | 0.85 | 0.34 | 3 | |
5 | 0.8 | 0.31 | 0.78 | 0.19 | 1 |
Communities | Nodes |
1′ | 1, 2, 3, 4, 5, 6, 7, 8, 9, 10, 11, 12 |
2′ | 13, 14, 15, 16, 17, 18, 19, 20, 21, 22 |
3′ | 23, 24, 25, 26, 27, 28, 29, ..., 60, 61, 62 |
LPA | Community | SCF | TW/TW | FC/TC | WSCF | Rank |
1′ | 0.67 | 0.43 | 0.64 | 0.18 | 3 | |
2′ | 0.67 | 0.29 | 0.66 | 0.126 | 1 | |
3′ | 0.67 | 0.29 | 0.69 | 0.131 | 2 |
F−N | Community | SCF | FW/TW | FC/TC | WSCF | Rank |
1 | 0.86 | 0.97 | 0.86 | 0.72 | 6 | |
2 | 0.71 | 0.07 | 0.72 | 0.04 | 1 | |
3 | 0.86 | 0.96 | 0.84 | 0.69 | 3 | |
4 | 0.86 | 0.08 | 0.86 | 0.06 | 2 | |
5 | 0.86 | 0.99 | 0.82 | 0.70 | 4 | |
6 | 0.86 | 0.93 | 0.89 | 0.71 | 5 | |
7 | 0.86 | 1.00 | 0.86 | 0.73 | 7 |
LPA | Community | SCF | FW/TW | FC/TC | WSCF | Rank |
1′ | 0.875 | 0.89 | 0.85 | 0.66 | 5 | |
2′ | 0.875 | 0.63 | 0.88 | 0.48 | 4 | |
3′ | 0.75 | 0.87 | 0.72 | 0.47 | 3 | |
4′ | 0.875 | 0.93 | 0.85 | 0.69 | 6 | |
5′ | 0.875 | 0.57 | 0.87 | 0.43 | 2 | |
6′ | 0.875 | 0.87 | 0.93 | 0.71 | 7 | |
7′ | 0.875 | 0.26 | 0.63 | 0.10 | 1 | |
8′ | 0.63 | 0.98 | 0.88 | 0.75 | 8 |
F−N | Community | SCF | FW/TW | FC/TC | WSCF | Rank |
1 | 0.75 | 0.36 | 0.81 | 0.22 | 1 | |
2 | 0.75 | 0.44 | 0.80 | 0.27 | 2 | |
3 | 0.75 | 0.69 | 0.64 | 0.28 | 3 | |
4 | 0.75 | 0.50 | 0.75 | 0.33 | 4 |
LPA | Community | SCF | FW/TW | FC/TC | WSCF | Rank |
1′ | 0.75 | 0.55 | 0.73 | 0.30 | 4 | |
2′ | 0.75 | 0.43 | 0.79 | 0.25 | 1 | |
3′ | 0.75 | 0.55 | 0.69 | 0.29 | 3 | |
4′ | 0.75 | 0.48 | 0.79 | 0.28 | 2 |
F−N | Community | FW/TW | FC/TC | SCF | Rank | WSCF | Rank |
1 | 0.86 | 0.78 | 0.83 | 2 | 0.56 | 4 | |
2 | 0.86 | 0.43 | 0.50 | 1 | 0.06 | 1 | |
3 | 0.71 | 0.81 | 0.83 | 2 | 0.48 | 3 | |
4 | 0.43 | 0.57 | 0.50 | 1 | 0.12 | 2 | |
5 | 0.43 | 0.86 | 0.83 | 2 | 0.61 | 5 | |
6 | 0.86 | 0.78 | 0.83 | 2 | 0.56 | 4 |
LPA | Community | FW/TW | FC/TC | SCF | Rank | WSCF | Rank |
1′ | 0.86 | 0.82 | 0.83 | 2 | 0.58 | 4 | |
2′ | 0.71 | 0.84 | 0.83 | 2 | 0.50 | 3 | |
3′ | 0.29 | 0.52 | 0.50 | 1 | 0.07 | 1 | |
4′ | 0.43 | 0.48 | 0.50 | 1 | 0.10 | 2 | |
5′ | 0.86 | 0.82 | 0.83 | 2 | 0.58 | 4 | |
6′ | 0.86 | 0.82 | 0.83 | 2 | 0.58 | 4 |
ERnetwork | Community | SCF | FW/TW | FC/TC | WSCF | Rank |
1 | 0.86 | 0.58 | 0.97 | 0.48 | 3 | |
2 | 0.86 | 0.65 | 0.92 | 0.51 | 4 | |
3 | 0.86 | 0.56 | 0.96 | 0.462 | 2 | |
4 | 0.86 | 0.56 | 0.96 | 0.460 | 1 | |
5 | 0.86 | 0.86 | 0.76 | 0.56 | 6 | |
6 | 0.86 | 0.83 | 0.81 | 0.58 | 7 | |
7 | 0.86 | 0.96 | 0.63 | 0.52 | 5 |
BAnetwork | Community | SCF | FW/TW | FC/TC | WSCF | Rank |
1 | 0.94 | 0.62 | 0.98 | 0.57 | 1 | |
2 | 0.94 | 0.90 | 0.94 | 0.801 | 7 | |
3 | 0.94 | 0.71 | 0.97 | 0.65 | 2 | |
4 | 0.94 | 0.72 | 0.97 | 0.66 | 3 | |
5 | 0.94 | 0.87 | 0.95 | 0.78 | 6 | |
6 | 0.94 | 0.78 | 0.98 | 0.71 | 4 | |
7 | 0.94 | 0.95 | 0.95 | 0.85 | 15 | |
8 | 0.94 | 0.94 | 0.91 | 0.806 | 9 | |
9 | 0.94 | 0.92 | 0.93 | 0.803 | 8 | |
10 | 0.94 | 0.83 | 0.96 | 0.75 | 5 | |
11 | 0.94 | 0.94 | 0.93 | 0.82 | 11 | |
12 | 0.94 | 0.93 | 0.92 | 0.813 | 10 | |
13 | 0.94 | 0.98 | 0.93 | 0.86 | 16 | |
14 | 0.94 | 0.98 | 0.94 | 0.87 | 17 | |
15 | 0.94 | 0.97 | 0.91 | 0.83 | 12 | |
16 | 0.94 | 0.99 | 0.91 | 0.844 | 14 | |
17 | 0.94 | 0.98 | 0.91 | 0.839 | 13 |
SWnetwork | Community | SCF | FW/TW | FC/TC | WSCF | Rank |
1 | 0.75 | 0.26 | 0.77 | 0.15 | 1 | |
2 | 0.75 | 0.77 | 0.73 | 0.42 | 4 | |
3 | 0.75 | 0.27 | 0.76 | 0.16 | 2 | |
4 | 0.75 | 0.70 | 0.74 | 0.39 | 3 |
F−N | Community | SCF | FW/TW | FC/TC | WSCF | Rank |
1 | 0.8 | 0.90 | 0.79 | 0.57 | 5 | |
2 | 0.8 | 0.88 | 0.80 | 0.56 | 4 | |
3 | 0.8 | 0.40 | 0.78 | 0.25 | 2 | |
4 | 0.8 | 0.50 | 0.85 | 0.34 | 3 | |
5 | 0.8 | 0.31 | 0.78 | 0.19 | 1 |
Communities | Nodes |
1′ | 1, 2, 3, 4, 5, 6, 7, 8, 9, 10, 11, 12 |
2′ | 13, 14, 15, 16, 17, 18, 19, 20, 21, 22 |
3′ | 23, 24, 25, 26, 27, 28, 29, ..., 60, 61, 62 |
LPA | Community | SCF | TW/TW | FC/TC | WSCF | Rank |
1′ | 0.67 | 0.43 | 0.64 | 0.18 | 3 | |
2′ | 0.67 | 0.29 | 0.66 | 0.126 | 1 | |
3′ | 0.67 | 0.29 | 0.69 | 0.131 | 2 |
F−N | Community | SCF | FW/TW | FC/TC | WSCF | Rank |
1 | 0.86 | 0.97 | 0.86 | 0.72 | 6 | |
2 | 0.71 | 0.07 | 0.72 | 0.04 | 1 | |
3 | 0.86 | 0.96 | 0.84 | 0.69 | 3 | |
4 | 0.86 | 0.08 | 0.86 | 0.06 | 2 | |
5 | 0.86 | 0.99 | 0.82 | 0.70 | 4 | |
6 | 0.86 | 0.93 | 0.89 | 0.71 | 5 | |
7 | 0.86 | 1.00 | 0.86 | 0.73 | 7 |
LPA | Community | SCF | FW/TW | FC/TC | WSCF | Rank |
1′ | 0.875 | 0.89 | 0.85 | 0.66 | 5 | |
2′ | 0.875 | 0.63 | 0.88 | 0.48 | 4 | |
3′ | 0.75 | 0.87 | 0.72 | 0.47 | 3 | |
4′ | 0.875 | 0.93 | 0.85 | 0.69 | 6 | |
5′ | 0.875 | 0.57 | 0.87 | 0.43 | 2 | |
6′ | 0.875 | 0.87 | 0.93 | 0.71 | 7 | |
7′ | 0.875 | 0.26 | 0.63 | 0.10 | 1 | |
8′ | 0.63 | 0.98 | 0.88 | 0.75 | 8 |
F−N | Community | SCF | FW/TW | FC/TC | WSCF | Rank |
1 | 0.75 | 0.36 | 0.81 | 0.22 | 1 | |
2 | 0.75 | 0.44 | 0.80 | 0.27 | 2 | |
3 | 0.75 | 0.69 | 0.64 | 0.28 | 3 | |
4 | 0.75 | 0.50 | 0.75 | 0.33 | 4 |
LPA | Community | SCF | FW/TW | FC/TC | WSCF | Rank |
1′ | 0.75 | 0.55 | 0.73 | 0.30 | 4 | |
2′ | 0.75 | 0.43 | 0.79 | 0.25 | 1 | |
3′ | 0.75 | 0.55 | 0.69 | 0.29 | 3 | |
4′ | 0.75 | 0.48 | 0.79 | 0.28 | 2 |