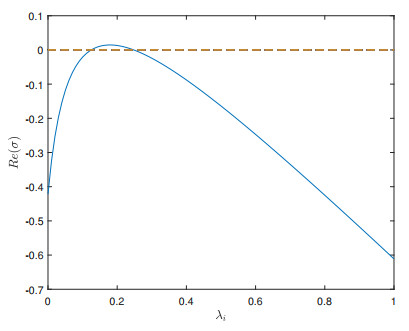
Citation: Hai-Feng Huo, Shuang-Lin Jing, Xun-Yang Wang, Hong Xiang. Modelling and analysis of an alcoholism model with treatment and effect of Twitter[J]. Mathematical Biosciences and Engineering, 2019, 16(5): 3561-3622. doi: 10.3934/mbe.2019179
[1] | Jichun Li, Gaihui Guo, Hailong Yuan . Nonlocal delay gives rise to vegetation patterns in a vegetation-sand model. Mathematical Biosciences and Engineering, 2024, 21(3): 4521-4553. doi: 10.3934/mbe.2024200 |
[2] | Hongyong Zhao, Qianjin Zhang, Linhe Zhu . The spatial dynamics of a zebrafish model with cross-diffusions. Mathematical Biosciences and Engineering, 2017, 14(4): 1035-1054. doi: 10.3934/mbe.2017054 |
[3] | Rina Su, Chunrui Zhang . The generation mechanism of Turing-pattern in a Tree-grass competition model with cross diffusion and time delay. Mathematical Biosciences and Engineering, 2022, 19(12): 12073-12103. doi: 10.3934/mbe.2022562 |
[4] | Maya Mincheva, Gheorghe Craciun . Graph-theoretic conditions for zero-eigenvalue Turing instability in general chemical reaction networks. Mathematical Biosciences and Engineering, 2013, 10(4): 1207-1226. doi: 10.3934/mbe.2013.10.1207 |
[5] | Swadesh Pal, Malay Banerjee, Vitaly Volpert . Spatio-temporal Bazykin’s model with space-time nonlocality. Mathematical Biosciences and Engineering, 2020, 17(5): 4801-4824. doi: 10.3934/mbe.2020262 |
[6] | Qianqian Zheng, Jianwei Shen, Lingli Zhou, Linan Guan . Turing pattern induced by the directed ER network and delay. Mathematical Biosciences and Engineering, 2022, 19(12): 11854-11867. doi: 10.3934/mbe.2022553 |
[7] | Yue Xing, Weihua Jiang, Xun Cao . Multi-stable and spatiotemporal staggered patterns in a predator-prey model with predator-taxis and delay. Mathematical Biosciences and Engineering, 2023, 20(10): 18413-18444. doi: 10.3934/mbe.2023818 |
[8] | P. E. Greenwood, L. M. Ward . Rapidly forming, slowly evolving, spatial patterns from quasi-cycle Mexican Hat coupling. Mathematical Biosciences and Engineering, 2019, 16(6): 6769-6793. doi: 10.3934/mbe.2019338 |
[9] | Fiona R. Macfarlane, Mark A. J. Chaplain, Tommaso Lorenzi . A hybrid discrete-continuum approach to model Turing pattern formation. Mathematical Biosciences and Engineering, 2020, 17(6): 7442-7479. doi: 10.3934/mbe.2020381 |
[10] | Van Dong Nguyen, Dinh Quoc Vo, Van Tu Duong, Huy Hung Nguyen, Tan Tien Nguyen . Reinforcement learning-based optimization of locomotion controller using multiple coupled CPG oscillators for elongated undulating fin propulsion. Mathematical Biosciences and Engineering, 2022, 19(1): 738-758. doi: 10.3934/mbe.2022033 |
The phenomenon of vegetation patterns has been found in a great many semiarid areas in recent decades [1,2,3,4]. Klausmeier [5] has proposed a semiarid vegetation model to explain the formation of striped vegetable patterns from the Turing instability and pointed out that the regular pattern would be self-organized from an irregular initial state. Klausmeier also showed that the diffusion induced by Brownian movement can act on the formation of regular patterns of plant vegetation. In addition, some more complicated spatiotemporal patterns were founded in the semiarid vegetation systems (see for example [6,7,8]). Some partial diferential equations mathematical modles were used to describe the vegetation patterns (see for example [9,10,11]). The stable steady solution were studied to demonstrate the pattern formation in [12,13]. Some frameworks of analysise were proposed to investigate the vegetation phenomena (see for example [14,15,16]). Recently, in [17,18], semiarid vegetation models have been characterized by fractional diffusion equations. In [19,20,21,22], the modified Klausmeier models were investigated by hyperbolic equations.
However, most of the research involved a reaction-diffusion system with plant seeds spreading through a continuous space. Frequent human activities have divided the space into many fine-scale habitats that form a network connected with spreading seeds in reality. The network can be depicted by a weighted graph. The graph is denoted by G=(V,E), including the vertices V={1,2,⋯,n} and the edges E. If the vertex y is adjacent to the vertex x, then we set y∼x. If every adjacent x and y is given by a weight function ω, then we get a weighed graph. Here ω:V×V→[0,∞) is a positive function satisfying ω(x,y)=ω(y,x) and ω(x,y)>0 only when x∼y. Hence, the degree of vertex x is Dω(x)=∑y∼x,y∈Vω(x,y). The weighted graph Laplacian operator is defined as follows:
Δωu(x)=∑y∼x,y∈V(u(y)−u(x))ω(x,y). |
Recent research work of pattern formation for the networked dynamics in physics illustrates that Turing patterns can occur with the large network [23]. The further recent research work exhibits that Turing-like waves can take place in a networked one-species spatiotemporal dynamics with delay [24], which is different from the convectional theory that Turing instability can only take place in the two-component spatiotemporal dynamics [25,26]. We focus on unexplored Turing patterns that occur in the weighed networked semiarid vegetation models representing fragmented habitats.
We consider the vegetation dynamics with the following weighted network:
{∂U∂t=A−LU−RUV2+BΔωU,(x,t)∈V×(0,∞),∂V∂t=RJUV2−MV+DΔωV,(x,t)∈V×(0,∞),U(x,0)=U0(x),V(x,0)=V0(x),x∈V, |
where U and V represent densities of water and plants, respectively. Water is supplied at the rate of A uniformly and loses at the rate of LU because of evaporation. Plants absorb water at the rate of RU2V. J is the amount of plants biomass per unit of water consumed. Plants lose at the mortal rate of MV. The diffusion of water and plants are constructed by the graph Laplacian diffusion coefficient B and D, respectively. The original Klausmeier model [5] contains the sloped terrain, while our model, a modified Klausmeier model, only studies flat terrain. Various techniques were proposed to show the existence and uniqueness of solutions for graph Laplacian equations (see for example [27,28,29,30]). In [31,32], the Lyapunov functions were constructed to show the global stability of reaction-diffusion systems where the space was confined in a graph. In [33,34,35], the method of stablity analysis were developed to deal with the networked reaction-diffusion systems. In [36,37], the pattern formation was investigated to describe the complex dynamical behavior of graph Laplacian equations.
For the sake of simplicity, scaling the variables u=R1/2L−1/2JU,v=R1/2L−1/2V,˜t=Lt and then dropping the tildes, the dimensionless, weighted networked spatiotemporal dynamics can be taken as:
{∂u∂t=a−u−uv2+cΔωu,,(x,t)∈V×(0,∞),∂v∂t=uv2−bv+dΔωv,(x,t)∈V×(0,∞),u(x,0)=u0(x),v(x,0)=v0(x),x∈V, | (1.1) |
where a=AJR1/2L−3/2, b=M/L, c=BJR1/2L−3/2 and d=DJR1/2L−3/2.
This paper is organized as follows. In Section 2, we show the global existence of solutions to the system (1.1). In Section 3, we obtain the Turing parameter space from the analytic results of the linear stability of the positive equilibrium and ensure that the Turing bifurcation takes place before Hopf bifurcation. In Section 4, an amplitude equations near the Turing instability critical point is derived by a weakly nonlinear analysis. Further, the stability of Turing patterns are considered by analyzing the amplitude equations. In Section 5, we establish the framework for deriving optimal control strategies of rainfall by deriving the adjoint equations and employing optimal control theory. In Section 6, some numerical simulations are presented to verify the theoretical analysis and explored the effects of the graph Laplacian diffusion on Turing patterns. Finally, some discussions and conclusions are given in Section 7.
In this section, we derive the existence and uniqueness result for the solution of system (1.1) by constructing the monotone iterative sequence. We need to give the definition of coupled upper and lower solutions, which was initially proposed by [38].
Definition 2.1. If ˜u,ˆu,˜v,ˆv∈C[0,T] are differentiable in (0,T], then pair of functions ˜u=(˜u,˜v) and ˆu=(ˆu,ˆv) are called coupled upper and lower solutions of (1.1) if ˜u≥ˆu≥0 and if
{∂˜u∂t−cΔω˜u≥a−˜u−˜uˆv2,(x,t)∈V×(0,∞),∂˜v∂t−dΔω˜v≥˜u˜v2−b˜v,(x,t)∈V×(0,∞),∂ˆu∂t−cΔωˆu≤a−ˆu−ˆu˜v2,(x,t)∈V×(0,∞),∂ˆv∂t−dΔωˆv≤ˆuˆv2−bˆv,(x,t)∈V×(0,∞),ˆu(x,0)≤u0(x)≤˜u(x,0),x∈V,ˆv(x,0)≤v0(x)≤˜v(x,0),x∈V. | (2.1) |
Since the reaction terms of system (1.1) are Lipschitz continuous, we denote K as the Lipschitz constant. By setting ¯u(0)=˜u and u_(0)=ˆu as the initial iterations, we construct the sequences ¯u(m) and u_(m) from the following iteration system:
{∂¯u(m)∂t−cΔω¯u(m)+K¯u(m)=K¯u(m−1)+a−¯u(m−1)−¯u(m−1)(v_(m−1))2,(x,t)∈V×(0,∞),∂¯v(m)∂t−dΔω¯v(m)+K¯v(m)=K¯v(m−1)+¯u(m−1)(¯v(m−1))2−b¯v(m−1),(x,t)∈V×(0,∞),∂u_(m)∂t−cΔωu_(m)+Ku_(m)=Ku_(m−1)+a−u_(m−1)−u_(m−1)(¯v(m−1))2,(x,t)∈V×(0,∞),∂v_(m)∂t−dΔωv_(m)+Kv_(m)=Kv_(m−1)+u_(m−1)(v_(m−1))2−bv_(m−1),(x,t)∈V×(0,∞),¯u(m)(x,0)=u_(m)(x,0)=u0(x),x∈V¯v(m)(x,0)=v_(m)(x,0)=v0(x),x∈V. | (2.2) |
Here ¯u(m)=(¯u(m),¯v(m)) and u_(m)=(u_(m),v_(m)). Since system (2.2) is a scalar, ordinary differential equation, the sequences ¯u(m) and u_(m) exist and are unique for some T. We have obtained the following monotonic properties of these sequences.
Lemma 2.1. If ¯u(m) and u_(m) are defined by (2.2), then the following holds:
ˆu≤u_(m)≤u_(m+1)≤¯u(m+1)≤¯u(m)≤˜u. | (2.3) |
Moreover, ¯u(m) and u_(m) are coupled upper and lower solutions of (1.1) for each m=1,2,⋯.
Proof. Considering system (2.2) is a monotone system, we can extend the method of monotone semiflows in [38] to our graph Laplacian equations.
Let p_(1)=u_(1)−u_(0). Then, by (2.2), for (x,t)∈V×(0,T], p_(1) satisfies
∂p_(1)∂t−cΔωp_(1)+Kp_(1)=Ku_(0)+a−u_(0)−u_(0)(¯v(0))2−(∂u_(0)∂t−cΔωu_(0)+Ku_(0))=a−ˆu−ˆu˜v2−(∂ˆu∂t−cΔωˆu)≥0, |
where the last inequality comes from (2.1). Meanwhile, p_(1)(x,0)=0 for x∈V. Using the maximum principle (Lemma 2.1 in [39]), we have p_(1)(x,t)≥0 for (x,t)∈V×[0,T]. Therefore, u_(0)(x,t)≤u_(1)(x,t) for (x,t)∈V×[0,T].
Let q_(1)=v_(1)−v_(0). Then, by (2.2), for (x,t)∈V×(0,T], q_(1) satisfies
∂q_(1)∂t−dΔωq_(1)+Kq_(1)=Kv_(0)+u_(0)(v_(0))2−bv_(0)−(∂v_(0)∂t−dΔωv_(0)+Kv_(0))=ˆuˆv2−bˆv−(∂ˆv∂t−dΔωˆv)≥0, |
where the last inequality comes from (2.1). Meanwhile q_(1)(x,0)=0 for x∈V. Using the maximum principle (Lemma 2.1 in [39]), we have q_(1)(x,t)≥0 for (x,t)∈V×[0,T]. Therefore, v_(0)(x,t)≤v_(1)(x,t) for (x,t)∈V×[0,T].
Thus, we have
u_(1)(x,t)≥u_(0)(x,t), for (x,t)∈V×[0,T]. | (2.4) |
By a similar argument as above, we also have
¯u(1)(x,t)≤¯u(0)(x,t), for (x,t)∈V×[0,T]. | (2.5) |
Now, we set ϕ(1)=¯u(1)−u_(1). By (2.2), it follows that for (x,t)∈V×(0,T],
∂ϕ(1)∂t−cΔωϕ(1)+Kϕ(1)=K¯u(0)+a−¯u(0)−¯u(0)(v_(0))2−(Ku_(0)+a−u_(0)−u_(0)(¯v(0))2)≥K¯u(0)+a−¯u(0)−¯u(0)(v_(0))2−(Ku_(0)+a−u_(0)−u_(0)(v_(0))2), |
where the last inequality comes from 0≤v_(0)(x,t)≤¯v(0)(x,t). Moreover, K is the Lipschitz constant of (1.1). Hence, we have
∂ϕ(1)∂t−cΔωϕ(1)+Kϕ(1)≥0. |
The initial condition is ϕ(1)(x,0)=0 for x∈V. Applying the maximum principle (Lemma 2.1 in [39]) yields that ¯u(1)≥u_(1). By a similar argument for v, we have ¯v(1)≥v_(1). Therefore,
u_(1)(x,t)≤¯u(1)(x,t), for (x,t)∈V×[0,T]. | (2.6) |
Combining (2.4)–(2.6) yields that
u_(0)≤u_(1)≤¯u(1)≤¯u(0). | (2.7) |
Then, we show that ¯u(1) and u_(1) are coupled upper and lower solutions of (1.1). It remains to show that ¯u(1) and u_(1) satisfy (2.1). By (2.2) and (2.7), for (x,t)∈V×(0,T], we have
∂¯u(1)∂t−cΔω¯u(1)+K¯u(1)=K¯u(0)+a−¯u(0)−¯u(0)(v_(0))2≥K¯u(0)+a−¯u(0)−¯u(0)(v_(1))2≥K¯u(1)+a−¯u(1)−¯u(1)(v_(1))2, |
where the last inequality holds because K is defined in (1.1). In a similar argument, we obtain that ¯u(1) and u_(1) are coupled upper and lower solutions of (1.1).
By an induction method, we extend the above result to some arbitrary m. We choose ˜u=¯u(1) and ˆu=u_(1). After the above process, we have
u_(1)≤u_(2)≤¯u(2)≤¯u(1). |
Thus, we can obtian (2.3).
Theorem 2.1. If ˜u and ˆu are a pair of coupled upper and lower solutions of system (1.1), then there exists u∗ in <ˆu,˜u> the unique solution of system (1.1) for t∈[0,T].
Proof. In terms of Lemma 2.1, the sequences ¯u(m) and u_(m) are convergent for t∈[0,T]. We set
limm→∞¯u(m)=¯u,limm→∞u_(m)=u_. |
We directly solve the solution of system (2.2) as follows:
¯u(m)(x,t)=u0(x)+∫t0(cΔω¯u(m)−K¯u(m)+K¯u(m−1)+a−¯u(m−1)−¯u(m−1)(v_(m−1))2)ds,u_(m)(x,t)=u0(x)+∫t0(cΔωu_(m)−Ku_(m)+Ku_(m−1)+a−u_(m−1)−u_(m−1)(¯v(m−1))2)ds. |
Since ¯u(m) and u_(m) are bounded in <ˆu,˜u>, by the dominated convergence theorem, it follows that ¯u(x,t) and u_(x,t) satisfy
¯u(x,t)=u0(x)+∫t0(cΔω¯u+a−¯u−¯uv_2)ds,u_(x,t)=u0(x)+∫t0(cΔωu_+a−u_−u_¯v2)ds. |
Since K is the Lipschitz constant, we obtain
¯u−u_≤∫t0(cΔω(¯u−u_)+K(¯u−u_)+K(¯v−v_))ds. |
By (2.2), it follows that
¯v(m)(x,t)=v0(x)+∫t0(dΔω¯v(m)−K¯v(m)+K¯v(m−1)+¯u(m−1)(¯v(m−1))2−b¯v(m−1))ds,v_(m)(x,t)=v0(x)+∫t0(dΔωv_(m)−Kv_(m)+Kv_(m−1)+u_(m−1)(v_(m−1))2−bv_(m−1))ds. |
Since ¯v(m) are bounded in (ˆv,˜v) for (x,t)∈V×[0,T], applying the dominated convergence theorem yields that
¯v(x,t)=v0(x)+∫t0(dΔω¯v+¯u¯v2−b¯v)ds,v_(x,t)=v0(x)+∫t0(dΔωv_+u_v_2−bv_)ds. |
In term of the definition of K, we have
¯v−v_≤∫t0(dΔω(¯v−v_)+K(¯u−u_)+K(¯v−v_))ds. |
We now estimate the boundedness ofthe above inequality. We have
Δω(¯u−u_)≤2nmaxx∈VDω(x)||¯u−u_||∞, |
where n is the number of the vertices of graph. Thus, we have
||¯u−u_||∞≤tC1(||¯u−u_||∞+||¯v−v_||∞),||¯v−v_||∞≤tC2(||¯u−u_||∞+||¯v−v_||∞), | (2.8) |
where C1 and C2 only depends on K, c, n, and maxx∈VDω(x).
Thus, there exists a constant T0:=1/2(C1+C2) such that ||¯u−u_||∞=0 and ||¯v−v_||∞=0, that is ¯u≡u_ and ¯v≡v_ hold for t∈(0,T0]. Since Ci does not depend on the initial value, we can extend T0 to the time T. By setting ¯u=u∗ and ¯v=v∗, we obtain
{u∗(x,t)=u0(x)+∫t0(cΔωu∗+a−u∗−u∗(v∗)2)ds,(x,t)∈V×[0,T],v∗(x,t)=v0(x)+∫t0(dΔωv∗+u∗(v∗)2−bv∗)ds,(x,t)∈V×[0,T]. |
Hence, (u∗,v∗) is the unique solution of system (1.1) for t∈[0,T].
Theorem 2.2. The solution of system (1.1) exists and is unique for all t∈[0,∞).
Proof. In view of Theorem 2.1, system (1.1) has an unique solution u∗ for t∈[0,T]. In order to show that T=∞, we need to show a priori upped bound of the solution by the approach of upper and lower solutions. We set (ˆu,ˆv)=(0,0) and (˜u,˜v). Thus,
˜u=max{||u0(x)||∞,a},˜v=max{||v0(x)||∞,b˜u}. |
Since they satisfy
{a−˜u−˜uˆv2≤0,a−ˆu−ˆu˜v2≥0,˜u˜v2−b˜≤0,ˆuˆv2−bˆv≥0, |
(˜u,˜v) and (ˆu,ˆv) are a pair of upper and lower solutions of system (1.1). By Theorem 2.1, the solution of system (1.1) is the uniformly upper bounded. Hence, T=∞.
This section is devoted to obtaining the existence of the Turing bifurcation around the uniform equilibrium of system (1.1). System (1.1) has three equilibria: E0, E1 and E2. Here E0=(a,0), E1=(a+√a2−4b22,2ba+√a2−4b2), and E2=(a−√a2−4b22,2ba−√a2−4b2). Considering the biological significance of the system (1.1), we only focus on the case of the positive equilibrium existing. Thus, the case a>2b always holds. By performing linear stability analysis, the second positive equilibrium E1 is an unstable saddle. Therefore, we only consider the case of the third positive equilibrium E2≡(us,vs) existing. In what follows, we give the space decomposition of [L2(V)]2 with respect to graph Laplace.
Lemma 3.1. The eigenvalue problem
{−Δωϕ(x)=λϕ(x),x∈V,∫Vϕ2(x)dx=1, | (3.1) |
for
∫Vϕ2(x)dx≡∑x∈Vϕ2(x), |
admits eigenvalues
{λi}ni=1:0=λ1<λ2≤⋯≤λn, |
where the associated eigenfunctions are {ϕi}ni=1. Moreover, [L2(V)]2 space decomposition
[L2(V)]2=n⨁i=1Ei | (3.2) |
holds. Here, Ei:={c⋅ϕi: c∈R2}.
Proof. Let λ and ϕ(x) be a solution of (3.1). By multiplying ϕ(x) and integrating it over V, we have
λ∫x∈Vϕ2(x)dx=−∫x∈Vϕ(x)Δω(x)dx=∑x,y∈V(ϕ(y)−ϕ(x))2ω(x,y)dx. |
We define a bilinear functional
F(u)=∑x,y∈V(u(y)−u(x))2ω(x,y), | (3.3) |
the domain D(F) of F is D(F):={u:u∈L2(V),||u||L2(V)=1}.
Step 1: Determine λ1. In light of the definition of F in (3.3) and (1.1), F is continuous in D(F) and bounded from below, that is, there exists ϕ1∈D(F) such that
λ1:=F(ϕ1)=infu∈D(F)F(u). | (3.4) |
Here, λ1 and ϕ1 are a solution of the eigenvalue problem (3.1). By substituting λ1=0 and ϕ1(x)=1 into (3.1), it easy to see that λ1=0 is the first eigenvalue. Moreover, λ1 is simple.
Step 2: Determine λ2. In view of the continuous property of F, the second minimization problem admits a solution, that is, ϕ2(x)∈L2(V) with ||ϕ2||L2(V)=1 and ϕ2⊥ϕ1 such that
λ2:=F(ϕ2)=infu∈L2(V){F(u):||u||L2(V)=1,u⊥ϕ1}, | (3.5) |
where u⊥ϕ1 means that u and ϕ1 are orthogonal, that is, ∫Vuϕ1dx=0. By the definition of (3.5), we have λ2>λ1.
By performing the above steps n times, we can construct a sequence {λk}nk=1 whose associated eigenfunction is {ϕk}nk=1. Owing to the finite nature of V, we find that n is finite.
Step 3: [L2(V)]2 space decomposition. We denote Ei as Ei:={c⋅ϕi: c∈R2}. Owing to the orthogonality of F(ϕi) for each i=1,2,⋯,n, we obtain [L2(V)]2=⨁ni=1Ei.
Theorem 3.1. If
b<min{a/2,1+v2s} | (3.6) |
holds, and (us,vs) is locally asymptotically stable.
Proof. The linearization of system (1.1) at the equilibrium (us,vs) is
∂U∂t=(J+DΔω)U,U=(u−usv−vs) | (3.7) |
with
J=(−1−v2s−2usvsv2s2usvs−b),D=(c00d). |
In view of Lemma 3.1, the eigenvalue of (J+DΔω) is equilivalent to the matrix (J−λiD). Then, we can write the solution of (3.7) as follows:
U=n∑i=1(c1ic2i)eσtϕi,(i=1,2,⋯,n), | (3.8) |
Substituting (3.8) into (3.7), we obtain
σeσtϕi(c1ic2i)=Jeσtϕi(c1ic2i)+Deσtλiϕi(c1ic2i). |
Since ϕi and eσt are nonzero vectors, we can obtain
(σI−λiD−J)(c1ic2i)=0. | (3.9) |
Hence, σ is sastified
det(σI−λiD−J)=0. | (3.10) |
This leads to the characteristic polynomials of (1.1)
σ2+giσ+hi=0, |
where gi=1+b+v2s−2usvs−cλi−dλi and hi=(1+v2s−cλi)(b−2usvs−dλi)+2usv3s.
In view of (3.6), we obtain that gi>0 and hi>0. Hence, σ<0. We conclude that (us,vs) is locally asymptotically stable.
Theorem 3.2. System (1.1) presents a Turing bifurcation at b=2usvs+dλi−2usv3s1+v2s−cλi.
Proof. According to Theorem 3.1, the characteristic polynomial of (1.1) is
σ2+giσ+hi=0, |
where gi=1+b+v2s−2usvs−cλi−dλi and hi=(1+v2s−cλi)(b−2usvs−dλi)+2usv3s.
Turing bifurcation point refers to gi>0 and hi=0. Thus, the Turing bifurcation critical value bc is
bc=2usvs+dλi−2usv3s1+v2s−cλi. | (3.11) |
Then, system (1.1) has a Turing bifurcation at b=2usvs+dλi−2usv3s1+v2s−cλi.
Remark 3.1. Although there were many standard techniques for Turing bifurcation of reaction-diffusion equations in the past, our method is aimed at network diffusion equations, which is a major advancement in bifurcation dynamics in network equations.
In this section, we investigate the bifurcation behavior in the area of the Turing bifurcation point bc by using a weakly nonlinear analysis of system (1.1).
Theorem 4.1. If (3.6) and Γ+4vsρ2+2usρ22>0 hold, the Turing bifurcation of system (1.1) is stable.
Proof. By transforming (us,vs) into (0,0), we first rewrite the system (1.1) into the following form:
∂U∂t=(J+DΔω)U+R(U), | (4.1) |
where
R(U)=(−2vsuv−usv2−uv22vsuv+usv2+uv2). |
We introduce the parameter ε satisfying ε2=b−bcbc by the method of perturbation technique. Let T=ε2t be the slow time of the Turing pattern bifurcation and expand U with respect to the parameter ε as follows:
(uv)=ε(u1v1)+ε2(u2v2)+ε3(u3v3)+⋯. | (4.2) |
Therefore, J is
J=Jc−(b−bc)M, | (4.3) |
where
Jc=(−1−v2s−2usvsv2s2usvs−bc),M=(0001). |
Expanding (4.1) with respect to different orders of ε, we can get three equations at orders εj(j=1,2,3):
O(ε):(Jc+DΔω)(u1v1)=0,O(ε2):(Jc+DΔω)(u2v2)=R1,O(ε3):(Jc+DΔω)(u3v3)=∂∂T(u1v1)+R2, | (4.4) |
where
R1=(2vsu1v1+usv21−2vsu1v1−usv21),R2=(2vsu1v2+2vsu2v1+2usv1v2+u1v21−2vsu1v2−2vsu2v1−2usv1v2−u1v21+bcv1). |
Since (u1,v1)T is the linear combination of the eigenvector of system (3.9) associated σ=0. Thus, the solution at O(ε) is (u1,v1)T=ρA(T)ϕi, and ρ=(ρ1,ρ2)T=(1,−1+v2s−cλi2usvs)T. Here, ϕi is the eigenvector corresponding to λi and ϕi is the spatial wave function of Turing pattern. A(T) is the amplitude of the solution determined by the higher order perturbation term of ε and is still unknown.
Next, we have the equation associated O(ε2)
(Jc+DΔω)(u2v2)=A2ϕ2i(2vsρ2+usρ22)(1−1). |
As a result, we can obtain that
(u2v2)=A2(ρ1ρ2)+A2ϕ2i(2vsρ2+usρ22)(ρ3ρ4), |
where ρ3=bc−λidΘ and ρ4=λic−1Θ, Θ=(1+v2s−λic)(bc−2usvs−λid)+2usv3s.
Further, we consider O(ε3). The equation associated O(ε3) is written as follows:
(Jc+DΔω)(u3v3)=dAdT(ρ1ρ2)ϕi+((4vsρ2+2usρ22)A3bcA−(4vsρ2+2usρ22)A3)ϕi+Γ(1−1)A3ϕ3i, | (4.5) |
where Γ=ρ22+2(2vsρ2+usρ22)(usρ2ρ4+vsρ2ρ3+vsρ4).
According to Fredholm solubility condition, (Jc+DΔω) has the adjoint operator L+c, and the operator L+c has the nontrivial kernel (1,(v2s+1−cλi)/v2s)Tϕi. Multiplying (4.5) by (1,(v2s+1−cλi)/v2s)Tϕi and integrateing it on V, we can induce Turing bifurcation's amplitude equation
dAdT=σA−LA3, | (4.6) |
where σ=−bcA(v2s+1−cλi)v2s, and L=1−cλiv2s(Γ+4vsρ2+2usρ22).
Therefore, the Turing bifurcation is stable if
Γ+4vsρ2+2usρ22>0 | (4.7) |
holds, which is called supercritical bifurcation.
This section discusses the effect of rainfall on the networked system (1.1). We will study how rainfall determine the dynamics of the networked structure. Hence, we involve the decreasing rainfall due to climate change in system (1.1). We use the term W(t) to represent the probablity of decrease in rainfall. When including the probablity of decreasing in rainfall W(t), the vegetation system takes the following form:
{∂u∂t=a−u−uv2−W(t)u+cΔωu,,(x,t)∈V×(0,∞),∂v∂t=uv2−bv+dΔωv,(x,t)∈V×(0,∞),u(x,0)=u0(x),v(x,0)=v0(x),x∈V, | (5.1) |
thus, the objective functional is written as
J(W)=∫T0∫V(Au(x,t)+W2(t))dxdt, | (5.2) |
over the control set:
V={W:[0,∞)→R+,0≤W(t)≤1,W∈C1(0,T)}. | (5.3) |
Here, we define the positive constant A to assign weight to the probablity of rainfall in the objective function. The optimal rainfall control problem can be expressed as follows:
Find W∗∈V such that
J(W∗)=infW∈VJ(W) |
subject to system (5.1).
For the above optimal control problem, after a similar argument, it is easy to show the solution of system (5.1) exists. To apply Pontryagin's maximum principle [40], we need to establish the existence of an optimal control.
Theorem 5.1. There exists an optimal control W∗, and corresponding solution (u∗,v∗) of system (5.1) that minimizes J(W) defined by (5.2) over V.
Proof. We refer to the conditions described in Theorem III.4.1 by Fleming and Rishel [41]. The requirements on the set of admissible controls V and on the set of end conditions are clearly satisfied here. Moreover, for any function W(t) in V given in (5.3), using a similar argument in Theorem 2.2, there exists a unique solution (u,v) of system (5.1). It remains to show that (u,v) and W(t) are globally existing.
After an argument similar to the proof of 2.1, we have
¯u(m)(x,t)=u0(x)+∫t0(cΔω¯u(m)−K¯u(m)+K¯u(m−1)+a−¯u(m−1)−¯u(m−1)(v_(m−1))2−W(t)¯u(m−1))ds,u_(m)(x,t)=u0(x)+∫t0(cΔωu_(m)−Ku_(m)+Ku_(m−1)+a−u_(m−1)−u_(m−1)(¯v(m−1))2−W(t)u_(m−1))ds. |
By using the dominated convergence theorem, it follows that for t∈[0,T] the limits ¯u(x,t) and u_(x,t) satisfy
¯u(x,t)=u0(x)+∫t0(cΔω¯u+a−¯u−¯uv_2−W(t)¯u)ds,u_(x,t)=u0(x)+∫t0(cΔωu_+a−u_−u_¯v2−W(t)u_)ds. |
Since K satisfies the Lipschitz condition, we have
¯u−u_≤∫t0(cΔω(¯u−u_)+K(¯u−u_)+K(¯v−v_)−W(t)(¯u−u_))ds. |
In a similar way, we have
¯v−v_≤∫t0(dΔω(¯v−v_)+K(¯u−u_)+K(¯v−v_))ds. |
In terms of the definition of weighed Laplacian operator, we have
Δω(¯u−u_)≤2nmaxx∈VDω(x)||¯u−u_||∞. |
Since 0≤W(t)≤1, we have
||¯u−u_||∞≤tC1(||¯u−u_||∞+||¯v−v_||∞),||¯v−v_||∞≤tC2(||¯u−u_||∞+||¯v−v_||∞), | (5.4) |
where C1 and C2 only depends on K, c, n, and maxx∈VDω(x).
Thus, there exists a constant T0:=1/2(C1+C2) such that ||¯u−u_||∞=0 and ||¯v−v_||∞=0, that is, ¯u≡u_ and ¯v≡v_ hold for t∈(0,T0]. Since Ci is independent on the initial value, we can extend T0 to T. Thus, (u,v) and W(t) are globally existing.
Theorem 5.2. If the optimal control W∗ and corresponding solution (u∗,v∗) of system (5.1) minimize the objective functional (5.2), then there eixsts adjoint variables λ1 and λ2 satisfying
{∂λ1∂t=−A+λ1(1+v2+W(t))−λ2v2,x∈V,t∈[0,T),∂λ2∂t=2λ1uv−2λ2uv+bλ2,x∈V,t∈[0,T),∂λ1∂x(t,0)=∂λ2∂x(t,0)=0,t∈[0,T),λ1(t,h(t))=λ2(t,h(t))=0,t∈[0,T),λ1(T)=λ2(T)=0. | (5.5) |
Furthermore, this optimal control is characterized by
W∗=max(min(1,λ1u2),0). | (5.6) |
Proof. We shall use Pontryagin's minimum principle [40] to complete the proof. We define the following Hamiltonian:
H(u,v,λ1,λ2)=Au+W2(t)+λ1[a−u−uv2−W(t)u+cΔωu]+λ2[uv2−bv+dΔωv]. | (5.7) |
It is easy to check that ∂2H∂W2=2>0. Thus, the critical point W∗ is indeed a minimum. By applying Pontryagin's minimum principle [40], the adjoint equations are given by
∂λ1∂t=−∂H∂u,∂λ2∂t=−∂H∂v, | (5.8) |
and must satisfy transversality condition λi(T)=0 for i=1,2. Hence, (5.5) holds.
Next, since W∗ is a critical point of the Hamiltonian, it follows that ∂H∂W=0 at W∗. This yields the following condition on the optimal control:
W∗=λ1u2. |
Since W∗ must belong to W, we have W∗=max(min(1,λ1u2),0).
Remark 5.1. The biological interpretation of the optimal rainfall W∗ in (5.6) is read as: When W(t) satisfies 0<W(t)<1, then the optimal rainfall W∗=λ1u2. The optimal rainfall probability is determined only by the density of water, not by the density of plants.
In this section, we shall carry out some numerical simulations to validate and extend analytical results of supercritical Turing patterns. In view of the conditions (3.6) and (4.7) determining the stability of the Turing bifurcation, we take the following set of parameters coming from the semiarid vegetation model [5]:
a=2,b=0.45,c=1,d=242.5. | (6.1) |
We show the real part of the eigenvalue characteristic polynomials (3.9) corresponding to the wave number λi in Figure 1. When the solid line is above the dashed line, Turing bifurcation occurs. In addition, we describe that the behavior of system (1.1) is determined by the input rate of water a and the death rate of plants b. By using bc as the critical value of the Turing bifurcation, when the plant Laplacian diffusion coefficient d=242.5, the Turing parameter space is shown in Figure 2. Considering the decrease of water input or the increase of plants loss, a transition from homogeneous vegetation to no vegetation is predicted in Figure 2.
Furthermore, we construct a Watts-Strogatz network consisting of a ring grid with 100 vertices connected to the nearest 4 neighbors from the left and right directions. In view of the fact that the motion of water usually obeys random walk, we add edges to the graph to satisfy the edge with a rewired probability of 0.15 for each pair of vertices. Through the use of numerical approximation, we obtain the solution of u and v. Figure 3 displays the plant density with network at different time. We describe the initial plant density with a small perturbation near the equilibrium, which can not effect the formation of spatiotemporal patterns as shown on the first panel of Figure 3. By means of the time integration of Runge-Kutta scheme, we exhibit the evolution of the spatial density of the plant with network at t=50,75, and 100 as shown in the 2nd, 3rd and 4th panels of Figure 3, respectively. From Figure 3, we can see that as the time t increases, the density of plant exhibits different value for each fixed vertex, that is, the system shows that a Turing pattern occurs under Turing parameter space a=2 and b=0.45. Though spatial patterns for different time are illustrated in these figures, we can not get the asymptotic behavior of the plant density for each vertex. Therefore, we describe that the asymptotic behavior converges to the temporal solution of system (1.1) for each vertex, where each node is arranged in a vertical column without considering the network structure. In Figure 4, the Turing pattern is stable and the solution converges to the temporally homogeneous behavior for each node in the left panel, the average density of plant converges to a constant with time in the middle panel, and the average density of plant over time is inhomogenous with respect to each node in the right panel. Hencen Figure 4 illustrates that the Turing pattern has a stable dynamical behavior under a large diffusion rate d=242.5.
In Figure 5, we plot the 2-dimensional continuous spatial Turing pattern for the plant density, the solution of which has generated a spatial regular pattern based on a self-organizational continuous diffusion mechanism. In its right panel, the average density of plants over continuous space converges to a constant with time. Comparing to the different spatial type, we find that the speed of the Turing self-organizational process is not different. The 2-dimensional continuous spatial system (middle panel of Figure 4) is faster than the networked system (right panel of Figure 5).
The reaction-diffusion system of a semiarid vegetation model is studied in this paper with a network considering the fragmented habitats due to the dispersion of plant seeds. The existence and uniqueness of solutions are shown by the monotone iterative technique. The stability of the Turing bifurcation is studied by the technique of weakly nonlinear analysis, which brought about the occurrence of the stable Turing patterns. We also propose a nonstandard finite difference method for the numerical solution. Furthermore, we demonstrate that the graph Lapacian diffusion plays an important role in the pattern formation.
Our results indicate that both the plant loss and rainfall have an impact on the Turing parameter space of a semiarid vegetation model with weighted networks. By Theorem 2.2, we have shown that the system with weighted networks admits an unique global solution. Theorems 3.1 and 3.2 indicate that if the plant loss b crosses to bc, the positive equilibrium of the system has a different dynamical behavior, which generates Turing instability. Theorem 4.1 indicates that when the Turing instability occurs, the Turing pattern is asymptotic stable.
It is shown that optimal rainfall probability is determined only by the density of water, not by the density of plants. Moreover, we have performed the numerical simulations to find the difference between these two self-organizational Turing patterns. The reaction-diffusion system has a self-organizational speed faster than that of the graph Laplacian diffusion model. In summary, this paper has proposed a new way to depict the movement of surface water and biomass with the graph Laplacian diffusion term defined in the semiarid vegetable model.
The authors declare they have not used Artificial Intelligence (AI) tools in the creation of this article.
Research was partially supported by NSF of China (No. 12471476) and the NSF of Jiangsu Province (No. BK20231357).
The authors declare there is no conflict of interest.
[1] | World Health Organization, Global status report on alcohol and health, 2014. Avail- able from: https://www.who.int/substance_abuse/publications/global_alcohol_ report/msb_gsr_2014_1.pdf. |
[2] | Shanghai Institute of Environmental Economics Disaster Prevention Laboratory, China, 2018. Available from: http://www.saes.sh.cn/cn/index.asp. |
[3] | R. Bani, R. Hameed and S. Szymanowski, Influence of environmental factors on college alcohol drinking patterns, Math. Biosci. Eng., 10(2013), 1281–1300. |
[4] | G. Mulone and B. Straughan, Modeling binge drinking, Int. J. Biomath. 5(2012), 57–70. |
[5] | S. Mushayabasa and C. P. Bhunu, Modelling the effects of heavy alcohol consumption on the transmission dynamics of gonorrhea, Nonlinear Dynamics, 66(2011), 695–706. |
[6] | S. Lee, E. Jung and C. Castillo-Chavez, Optimal control intervention strategies in low- and high- risk problem drinking populations, Socio-economic Plan. Sci., 44(2010), 258–265. |
[7] | A. Mubayi, P. Greenwood and C. Castillo-Chavez, The impact of relative residence times on the distribution of heavy drinkers in highly distinct environments, Socio-economic Plan. Sci., 44(2010), 45–56. |
[8] | H. F. Huo, Y. L. Chen and H. Xiang, Stability of a binge drinking model with delay, J. Biol. Dynam., 11(2017), 210–225. |
[9] | H. Xiang, Y. P. Liu and H. F. Huo, Stability of an sairs alcoholism model on scale-free networks, Physica A Statist. Mechan., 473(2017), 276–292. |
[10] | J. Cui, Y. Sun and H. Zhu, The impact of media on the control of infectious diseases, J. Dynam. Differ. Equat., 20(2008), 31–53. |
[11] | K. A. Pawelek, A. Oeldorf-Hirsch and L. Rong, Modeling the impact of twitter on influenza epi- demics, Math. Biosci. Eng. 11(2014), 1337–1356. |
[12] | H. F. Huo and X. M. Zhang, Modeling the Influence of Twitter in Reducing and Increasing the Spread of Influenza Epidemics, SpringerPlus, 5(2016), 88. |
[13] | H. F. Huo and X. M. Zhang, Complex dynamics in an alcoholism model with the impact of twitter, Math. Biosci., 281(2016), 24–35. |
[14] | H. F. Huo, P. Yang and H. Xiang, Stability and bifurcation for an seis epidemic model with the impact of media, Physica A Statist. Mechan. Appl., 490(2018), 702–720. |
[15] | X. Y. Meng and J. G. Wang, Analysis of a delayed diffusive model with Beddington-DeAngelis functional response, Int. J. Biomath., doi:10.1142/S1793524519500475. |
[16] | H. Xiang, M. X. Zou and H. F. Huo, Modeling the Effects of Health Education and Early Ther- apy on Tuberculosis Transmission Dynamics, International Journal of Nonlinear Sciences and Numerical Simulation, DOI:https://doi.org/10.1515/ijnsns-2016-0084. |
[17] | H. Xiang, Y. Y. Wang and H. F. Huo, Analysis of the binge drinking models with demographics and nonlinear infectivity on networks, J. Appl. Anal. Comput. 8(2018), 1535–1554. |
[18] | Y. L. Cai, J. J. Jiao and Z. J. Gui, Environmental variability in a stochastic epidemic model, Appl. Math. Comput., 329(2018), 210–226. |
[19] | Z. Du and Z. Feng, Existence and asymptotic behaviors of traveling waves of a modified vector- disease model, Commu. Pure Appl. Anal., 17(2018), 1899–1920. |
[20] | X. B. Zhang, Q. H. Shi and S. H. Ma, Dynamic behavior of a stochastic SIQS epidemic model with levy jumps, Nonlinear Dynam., 93(2018), 1481–1493. |
[21] | W. M. Wang, Y. l. Cai and Z. Q. Ding, A stochastic differential equation SIS epidemic model incorporating Ornstein-Uhlenbeck process, Physica A Statist. Mechan. Appl., 509(2018), 921– 936. |
[22] | X. Y. Meng and Y. Q. Wu, Bifurcation and control in a singular phytoplankton-zooplankton-fish model with nonlinear fish harvesting and taxation, Int. J. Bifurc. Chaos, 28(2018), 1850042. |
[23] | National Institute on Alcohol Abuse and Alcoholism, United States of America, 2018. Available from: https://www.niaaa.nih.gov/. |
[24] | H. F. Huo, F. F. Cui and H. Xiang, Dynamics of an saits alcoholism model on unweighted and weighted network, Physica A Statist. Mechan., 496(2018), 321–335. |
[25] | H. F. Huo, R. Chen and X. Y. Wang, Modelling and stability of HIV/AIDS epidemic model with treatment, Appl. Math. Modell., 40(2016), 6550–6559. |
[26] | H. F. Huo and M. X. Zou, Modelling effects of treatment at home on tuberculosis transmission dynamics, Appl. Math. Modell., 40(2016), 9474–9484. |
[27] | P. van den Driessche and J. Watmough, Reproduction numbers and sub-threshold endemic equi- libria for compartmental models of disease transmission, Math. Biosci., 180(2002), 29–48. |
[28] | L. Salle and P. Joseph, The stability of dynamical systems, Society for Industrial and Applied Mathematics, Philadelphia, Pa., 1976. |
[29] | C. Castillo-Chavez and B. Song, Dynamical models of tuberculosis and their applications, Math. Biosci. Eng., 1(2004), 361–401. |
[30] | A. D. Lê, D. Funk, S. Lo, et al., Operant self-administration of alcohol and nicotine in a preclinical model of co-abuse, Psychopharmacology, 231(2014), 4019–4029. |