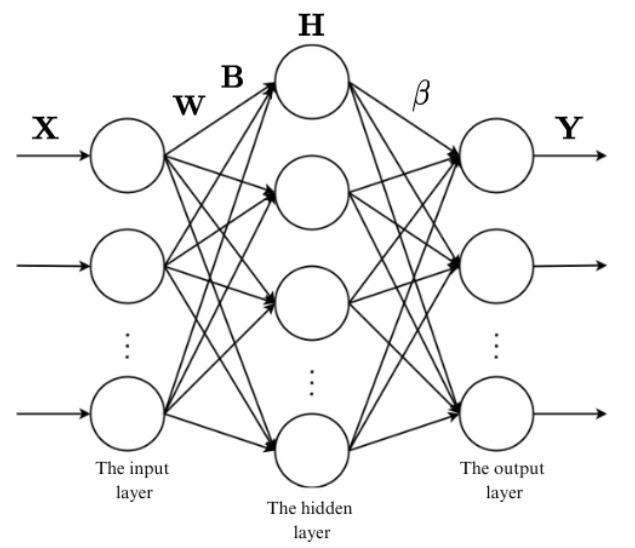
In this paper, we present a novel two-step inertial algorithm for finding a common fixed-point of a countable family of nonexpansive mappings. Under mild assumptions, we prove a weak convergence theorem for the method. We then demonstrate its versatility by applying it to convex minimization problems and extending it to data classification tasks, specifically through a multihidden-layer extreme learning machine (MELM). Numerical experiments show that our approach outperforms existing methods in both convergence speed and classification accuracy. These results highlight the potential of the proposed algorithm for broader applications in machine learning and optimization.
Citation: Kobkoon Janngam, Suthep Suantai, Rattanakorn Wattanataweekul. A novel fixed-point based two-step inertial algorithm for convex minimization in deep learning data classification[J]. AIMS Mathematics, 2025, 10(3): 6209-6232. doi: 10.3934/math.2025283
[1] | Puntita Sae-jia, Suthep Suantai . A new two-step inertial algorithm for solving convex bilevel optimization problems with application in data classification problems. AIMS Mathematics, 2024, 9(4): 8476-8496. doi: 10.3934/math.2024412 |
[2] | Adisak Hanjing, Panadda Thongpaen, Suthep Suantai . A new accelerated algorithm with a linesearch technique for convex bilevel optimization problems with applications. AIMS Mathematics, 2024, 9(8): 22366-22392. doi: 10.3934/math.20241088 |
[3] | Suparat Kesornprom, Papatsara Inkrong, Uamporn Witthayarat, Prasit Cholamjiak . A recent proximal gradient algorithm for convex minimization problem using double inertial extrapolations. AIMS Mathematics, 2024, 9(7): 18841-18859. doi: 10.3934/math.2024917 |
[4] | Kaiwich Baewnoi, Damrongsak Yambangwai, Tanakit Thianwan . A novel algorithm with an inertial technique for fixed points of nonexpansive mappings and zeros of accretive operators in Banach spaces. AIMS Mathematics, 2024, 9(3): 6424-6444. doi: 10.3934/math.2024313 |
[5] | Anjali, Seema Mehra, Renu Chugh, Salma Haque, Nabil Mlaiki . Iterative algorithm for solving monotone inclusion and fixed point problem of a finite family of demimetric mappings. AIMS Mathematics, 2023, 8(8): 19334-19352. doi: 10.3934/math.2023986 |
[6] | Meiying Wang, Luoyi Shi, Cuijuan Guo . An inertial iterative method for solving split equality problem in Banach spaces. AIMS Mathematics, 2022, 7(10): 17628-17646. doi: 10.3934/math.2022971 |
[7] | Hasanen A. Hammad, Hassan Almusawa . Modified inertial Ishikawa iterations for fixed points of nonexpansive mappings with an application. AIMS Mathematics, 2022, 7(4): 6984-7000. doi: 10.3934/math.2022388 |
[8] | Wei Xue, Pengcheng Wan, Qiao Li, Ping Zhong, Gaohang Yu, Tao Tao . An online conjugate gradient algorithm for large-scale data analysis in machine learning. AIMS Mathematics, 2021, 6(2): 1515-1537. doi: 10.3934/math.2021092 |
[9] | Bao Ma, Yanrong Ma, Jun Ma . Adaptive robust AdaBoost-based kernel-free quadratic surface support vector machine with Universum data. AIMS Mathematics, 2025, 10(4): 8036-8065. doi: 10.3934/math.2025369 |
[10] | Austine Efut Ofem, Jacob Ashiwere Abuchu, Godwin Chidi Ugwunnadi, Hossam A. Nabwey, Abubakar Adamu, Ojen Kumar Narain . Double inertial steps extragadient-type methods for solving optimal control and image restoration problems. AIMS Mathematics, 2024, 9(5): 12870-12905. doi: 10.3934/math.2024629 |
In this paper, we present a novel two-step inertial algorithm for finding a common fixed-point of a countable family of nonexpansive mappings. Under mild assumptions, we prove a weak convergence theorem for the method. We then demonstrate its versatility by applying it to convex minimization problems and extending it to data classification tasks, specifically through a multihidden-layer extreme learning machine (MELM). Numerical experiments show that our approach outperforms existing methods in both convergence speed and classification accuracy. These results highlight the potential of the proposed algorithm for broader applications in machine learning and optimization.
Convex minimization problems are widespread in optimization. They are applied in various fields such as machine learning, signal processing, economics, and engineering. These problems are important because they are often assured to have a global minimum and thus give robust and reliable solutions to complex systems [1]. Convex minimization problems are fundamental in machine learning since they play an important role in training models, in particular for the support vector machines and logistic regression, where the goal is to minimize a loss function over additional regularizing terms [2]. These problems occur in signal processing tasks such as image reconstruction and denoising, where we seek to recover signals from noisy observations [3]. In finance as well, portfolio optimization is also based upon convex optimization, where one must find the optimal asset allocation that optimizes returns while minimizing risk [4].
The general form of a convex minimization problem can be expressed as:
minx∈Rn(f(x)+g(x)), | (1.1) |
where f:Rn→R is a smooth, convex function representing data fidelity, and g:Rn→R is a proper, lower semi-continuous convex function representing regularization or constraints. This formulation is widely used because it encompasses a broad range of practical problems where balancing accuracy and simplicity (or sparsity) of the solution is necessary [5]. Problem (1.1) has been studied by many researchers, and a number of algorithms have been proposed to solve this problem; see, for example, [6,7,8,9].
A powerful class of methods for solving convex minimization problems are fixed-point algorithms. These algorithms deal with finding x∗ such that x∗=Tx∗, where T=proxλg(I–λ∇f) is a proximal operator, f and g are as in Eq (1.1), and λ>0 is a step size parameter. In general, the proximal operator is defined for a function g as:
proxg(v)=argminx(g(x)+12‖x−v‖2). | (1.2) |
This operator is used to deal efficiently with functions g that are nonsmooth, a common characteristic of regularization terms in convex optimization. It is well known that if x is a fixed-point of the forward-backward operator
x=proxλg(x−λ∇f(x)), | (1.3) |
which involves the proximal operator, then x is a minimizer of f+g. This relationship between fixed-points and minimizers is a key insight in convex minimization. It serves as the theoretical foundation for using fixed-point iterations to solve convex minimization problems [10,11].
Many researchers have proposed fixed-point algorithms that leverage the properties of the proximal operator [12,13,14,15]. Algorithms such as the proximal gradient method or forward-backward splitting (FBS) algorithm [7] update iterates using:
xn+1=proxλg(xn−λ∇f(xn)). | (1.4) |
This method combines gradient descent for the smooth function f with the proximal step for the nonsmooth function g, making it particularly useful for large-scale optimization problems. It is noted that when L is a Lipschitz constant of ∇f, then T=proxλg(I−λ∇f) is nonexpansive if λ∈(0,2/L). Solving variational inequalities and equilibrium problems is especially well suited to such an approach.
One of the most prominent forward-backward type algorithms with inertial step and design is the fast iterative shrinkage-thresholding algorithm (FISTA) introduced by Beck et al. [16]. The authors proved the algorithm's convergence and showed its practical use by applying it to image restoration problems, demonstrating its efficacy in real-world tasks. The FISTA algorithm is defined as follows:
{yn=Txn,tn+1=1+√1+4t2n2,θn=tn−1tn+1,xn+1=yn+θn(yn−yn−1), | (1.5) |
where n≥1, T:=prox1Lg(I−1L∇f), x1=y0∈Rn, t1=1, and θn represents the inertial step size introduced by Nesterov [17].
Bussaban et al. [12] introduced the parallel inertial S-iteration forward-backward algorithm (PISFBA), a new method designed to solve convex minimization problems efficiently. The algorithm is defined as:
{yn=xn+θn(xn−xn−1),zn=(1−βn)xn+βnTnxn,xn+1=(1−αn)Tnyn+αnTnzn, | (1.6) |
where n≥1, Tn=proxcng(I−cn∇f), x0=x1∈H, 0<q<αn≤1, 0<s<βn<r<1, and ∑∞n=1θn‖xn−xn−1‖<∞. The authors proved a weak convergence theorem for PISFBA under the assumption of Lipschit continuity of ∇f. In addition, they showed their method's practical applicability by applying it to regression and data classification problems, showing that PISFBA can effectively solve real-world machine learning problems. After that, various fixed-point algorithms with a one-step inertial technique were introduced for solving the convex minimization problem (1.1) and employed to solving the data classification problem and the image restoration problem; see [18,19,20,21].
To relax the continuity assumption of ∇f, D. Reem et al. [22] introduced a new variant of the proximal gradient method that does not impose the above-mentioned global Lipschitz continuity assumption.
The FISTA and PISFBA algorithms both employ a single inertial parameter, θ. Such single-step algorithms are most effective when the problems are high-dimensional, and under more general conditions can be unstable and slow to converge. Two-step inertial algorithms incorporate two inertial parameters, resulting in improved convergence and stability. The basic idea is to update iterates using a combination of current and previous step information, formalized as:
yn=xn+θ(xn−xn−1)+δ(xn−1−xn−2), | (1.7) |
where θ>0 and δ<0 are inertial parameters that incorporate momentum from previous iterates. This approach enhances motion modeling, improves stability, and increases redundancy, making it suitable for a broader range of applications. The incorporation of two inertial parameters also provides flexibility and adaptability in algorithm design, allowing fine-tuning for specific optimization problems. Recent research showcases the improved stability of two-step inertial algorithms over their one-step counterparts. Izuchukwu et al. (2023) [23] introduced a two-step inertial forward-reflected-anchored-backward splitting algorithm for monotone inclusion problems, demonstrating strong convergence with fewer computational steps compared to one-step methods. Iyiola and Shehu (2022) [24] proposed a two-step inertial proximal point algorithm for convex minimization, establishing a non-asymptotic O(1/n) convergence rate. Recently, Thong et al. (2025) [25] introduced a double-inertial-step algorithm for split common fixed-point problems, demonstrating strong convergence with an application to signal processing.
Motivated by the literature discussed above, we propose a new two-step inertial algorithm that incorporates two inertial parameters and exhibits enhanced convergence. The proposed method is versatile and can be applied to various complex hierarchical optimization tasks. The remainder of the paper is organized as follows: In Section 2, we recall some basic definitions and results that are crucial for understanding the proposed method. In Section 3, we present our algorithm and the relevant convergence analysis, and we discuss the algorithm's application to solving convex minimization problems. Section 4 delves into the underlying principles of the machine learning models used for data classification and demonstrates the application of our algorithm by reformulating these models as convex minimization problems. Section 5 presents numerical experiments that demonstrate the performance of the proposed method. Finally, Section 6 concludes the paper with a summary of findings and potential future research directions.
In this section, we introduce the notation and give some fundamental definitions and lemmas that are used in the following sections. Let H be a real Hilbert space with an inner product ⟨⋅,⋅⟩ and the induced norm ‖⋅‖. The set of positive integers, the set of real numbers, the set of non-negative real numbers, and the set of positive real numbers are denoted by N, R, R+, and R>0, respectively. For a mapping T:H→H, the set of fixed-points of T is denoted by F(T) i.e., F(T)={x∈H:Tx=x}. Let ψ be a family of mappings from H into itself; F(ψ) denotes the set of all common fixed-points of ψ, that is, F(ψ)={x∈H:T(x)=xfor allT∈ψ}. A mapping T:H→H is said to be nonexpansive if ‖Tx−Ty‖≤‖x−y‖ for all x,y∈H.
Definition 2.1. [26] Let {Tn} and ψ be countable families of nonexpansive mappings of H into itself such that ∅≠F(ψ)⊂∩∞n=1F(Tn). We say that the sequence {Tn} satisfies the NST-condition (I) with respect to ψ if for any bounded sequence {un} in C and all T∈ψ, the following holds:
limn→∞‖un−Tnun‖=0 implies limn→∞‖un−Tun‖=0. |
In the case that ψ={T}, {Tn} is said to satisfy the NST-condition (I) with respect to T.
Definition 2.2. Let f:Rn→R be a smooth convex function and let g:Rn→R be a proper, lower semi-continuous, convex function. Following Moreau [10], define, for λ>0, the proximity operator with respect to λ and g by
proxλg(x)=argminy∈H{g(y)+12λ‖y−x‖2}, |
see [11,27]. Define the forward-backward operator T as
T:=proxλg(I−λ∇f) |
with λ>0, where ∇f represents the gradient of the function f. If λ∈(0,2/L), where L is a Lipschitz constant of ∇f, then T is nonexpansive. More related results on nonexpansive projections and resolvents of accretive operators in Banach spaces are discussed in [28].
The lemmas stated below play a significant role in proving our main result.
Lemma 2.1. [29] Suppose that {an} and {bn} are two sequences of nonnegative numbers such that an+1≤an+bn for all n≥1. If ∑∞n=1bn converges, then limn→∞an exists.
Lemma 2.2. [30] For a real Hilbert space H, the following results hold:
(i) For any vectors x,y∈H and any scalar γ∈[0,1],
‖γx+(1−γ)y‖2=γ‖x‖2+(1−γ)‖y‖2−γ(1−γ)‖x−y‖2. |
(ii) For any vectors x,y∈H,
‖x±y‖2=‖x‖2±2⟨x,y⟩+‖y‖2. |
Lemma 2.3. [31] Let H be a Hilbert space and {un} be a sequence in H such that for some nonempty subset Υ⊂H, the following conditions are satisfied:
(i) For every p∈Υ, limn→∞‖un−p‖ exists.
(ii) Any weak-cluster point of the sequence {un} belongs in Υ.
Then, there exists v∈Υ such that {un} weakly converges to v.
Lemma 2.4. [12] Let H be a real Hilbert space. Consider a proper, lower semi-continuous convex function g:H→R∪{∞} and a convex differentiable function f:H→R whose gradient ∇f is Lipschitz continuous with constant L>0. Let T denote the forward-backward operator associated with f and g. Suppose that {Tn} is a sequence of forward-backward operators corresponding to step sizes {cn} such that cn→c with cn,c∈(0,2/L). Then, the sequence {Tn} satisfies the NST-condition (I) with respect to T.
In what follows, T:H→H is a nonexpansive mapping with F(T)≠∅, and {Tn:H→H} is family of nonexpansive mappings such that F(T)⊂Γ, where Γ:=∩∞n=1F(Tn).
We now present Algorithm 1 and demonstrate its weak convergence.
Algorithm 1 Two-Step Inertial Modified SP-Algorithm (TIMSPA) |
Initialization: Let {βn}, {αn} ⊂[0,1], {τn}⊂R+ and let {μn}, {ρn}⊂R>0 be bounded sequences. Take z−1,z0,x1∈H arbitrarily. For n∈N, do the following steps. |
Step 1. Compute yn and zn: |
yn=(1−βn)xn+βnTnxn,zn=(1−αn)yn+αnTnyn. |
Step 2. Compute the inertial step: |
θn={min{μn,τn‖zn−zn−1‖},ifzn≠zn−1,μn,otherwise, |
and |
δn={max{−ρn,−τn‖zn−1−zn−2‖},ifzn−1≠zn−2,−ρn,otherwise. |
Step 3. Compute xn+1: |
xn+1=zn+θn(zn−zn−1)+δn(zn−1−zn−2). |
Remark 3.1. Allowing δn to take nonpositive values provides a damping or "pull-back" effect that helps to stabilize the forward inertial term. Empirically, combining a positive θn and a negative δn strikes an effective balance between acceleration and stability in a two-step inertial algorithm; see [23,24,25] for more details.
Assume that the control sequences {τn}, {θn}, {δn}, and {βn} in Algorithm 1 satisfy the following conditions:
(C1) ∑∞n=1τn<∞;
(C2) 0<a1≤βn≤a2<1 for some a1,a2∈R.
The following lemmas provide a crucial estimate in the proof of Theorem 3.1.
Lemma 3.1. Let {xn} be a sequence generated by Algorithm 1. Then, for any x∗∈Γ, we have
(i) ‖xn+1−x∗‖≤‖xn−x∗‖+2τn,
(ii) limn→∞‖xn−x∗‖ exists.
Proof. Let x∗∈Γ. By Algorithm 1, we obtain
‖yn−x∗‖=‖(1−βn)xn+βnTnxn−x∗‖≤‖xn−x∗‖ | (3.1) |
and
‖zn−x∗‖=‖(1−αn)yn+αnTnyn−x∗‖≤‖yn−x∗‖. | (3.2) |
From the choice of θn and δn, we note that
θn‖zn−zn−1‖≤τnand |δn|‖zn−1−zn−2‖≤τn. | (3.3) |
By (3.1)–(3.3), we obtain
‖xn+1−x∗‖≤‖zn−x∗‖+θn‖zn−zn−1‖+|δn|‖zn−1−zn−2‖≤‖xn−x∗‖+2τn. | (3.4) |
Hence, by Lemma 2.1, it follows that limn→∞‖xn−x∗‖ exists, as required.
Lemma 3.2. Let {xn} be a sequence generated by Algorithm 1. Then limn→∞‖Tnxn−xn‖=0.
Proof. By the definition of yn and Lemma 2.2, we obtain
‖yn−x∗‖2=‖(1−βn)(xn−x∗)+βn(Tnxn−x∗)‖2=(1−βn)‖xn−x∗‖2+βn‖Tnxn−x∗‖2−βn(1−βn)‖Tnxn−xn‖2≤‖xn−x∗‖2−βn(1−βn)‖Tnxn−xn‖2. |
This implies that, for n≥1,
βn(1−βn)‖Tnxn−xn‖2≤‖xn−x∗‖2−‖yn−x∗‖2. | (3.5) |
By Lemma 3.1, we let
limn→∞‖xn−x∗‖=a. | (3.6) |
From (3.1), we obtain
lim supn→∞‖yn−x∗‖≤lim supn→∞‖xn−x∗‖. |
It follows that
lim supn→∞‖yn−x∗‖≤a. | (3.7) |
Using (3.2) and (3.4), we obtain
‖xn+1−x∗‖≤‖yn−x∗‖+θn‖zn−zn−1‖+|δn|‖zn−1−zn−2‖. | (3.8) |
Since (C1) and the definitions of θn and |δn|, we obtain
limn→∞θn‖zn−zn−1‖=0=limn→∞|δn|‖zn−1−zn−2‖. |
From (3.6) and (3.8), we obtain
a≤lim infn→∞‖yn−x∗‖. | (3.9) |
It follows from (3.7) and (3.9) that
limn→∞‖yn−x∗‖=a. | (3.10) |
So, we get from (C3), (3.5), (3.6) and (3.10) that
limn→∞‖Tnxn−xn‖=0 |
as required.
We now establish the main convergence property of Algorithm 1.
Theorem 3.1. Let {xn} be a sequence generated by Algorithm 1. Suppose that {Tn} satisfies the NST-condition (I) with respect to T. Then, the sequence {xn} converges weakly to an element x∗∈Γ, where Γ:=∩∞n=1F(Tn)≠∅.
Proof. Let x∗∈Γ. Using Lemma 3.1, we obtain limn→∞‖xn−x∗‖ exists. From Lemma 3.2 and {Tn} satisfying the NST-condition (I) with respect to T, we obtain
limn→∞‖Txn−xn‖=0. |
Since I−T is demiclosed at 0, we obtain ωw(xn)⊂F(T), where ωw(xn) is the set of all weak cluster points of {xn}. So, based on Lemma 2.3, we can conclude that {xn} converges weakly to x∗∈F(T)⊂Γ.
Remark 3.2. From Lemma 3.1 and Theorem 3.1, we know that the sequence {xn} generated by Algorithm 1 converges weakly to x∗∈⋂∞n=1F(Tn) and
‖xn+1−x∗‖≤‖xn−x∗‖+νn, |
where νn=2τn.For each n∈N, put en=‖xn−x∗‖. From the condition (C2), we can choose νn in such a way that limn→∞νnen=0. One can show that lim supn→∞en+1en≤1. In this case, the sequence ‖xn−x∗‖ seems to be linear or sublinear convergence.
Next, we present Algorithm 2, which adapts Algorithm 1 by setting T:=proxcg(I−c∇f) and Tn:=proxcng(I−cn∇f). This modification allows us to solve the convex minimization problem and establish the weak convergence of the generated sequence to a minimizer of f+g.
Algorithm 2 Two-Step Inertial forward-backward Modified SP-Algorithm (TIFBSPA) |
Initialization: Let {βn}, {αn}⊂[0,1], {τn}⊂R+, and let {μn}, {ρn}⊂R>0 be bounded sequences. |
Let cn∈(0,2/L) with {cn}→c as n→∞. |
Take z−1,z0,x1∈Rn arbitrarily. For n≥1, do the following steps: |
Step 1. Compute yn and zn: |
yn=(1−βn)xn+βnproxcng(I−cn∇f)xn,zn=(1−αn)yn+αnproxcng(I−cn∇f)yn. |
Step 2. Compute the inertial steps: |
θn={min{μn,τn‖zn−zn−1‖},ifzn≠zn−1,μn,otherwise,(3.11) |
and |
δn={max{−ρn,−τn‖zn−1−zn−2‖},ifzn−1≠zn−2,−ρn,otherwise.(3.12) |
Step 3. Compute xn+1: |
xn+1=zn+θn(zn−zn−1)+δn(zn−1−zn−2).(3.13) |
Theorem 3.2. Let f,g:Rn→R∪{∞} be functions such that f is convex and differentiable, having Lipschitz continuous ∇f with constant L>0, and g is a proper, lower semicontinuous, convex function. Consider the sequence {xn} generated by Algorithm 2, with parameters {τn}, {θn}, {δn}, and {βn} as defined in Algorithm 1. Then, the sequence {xn} converges to an element of Argmin(f+g).
Proof. We begin by noting that both T and each Tn are nonexpansive operators. For all n, the fixed-points of Tn=proxcng(I−cn∇f) are exactly the minimizers of f+g. Therefore,
F(T)=∞⋂n=1F(Tn)=Argmin(f+g). |
By Lemma 2.4, this means that the sequence {Tn} satisfies the NST-condition (I) with respect to T. Applying Theorem 3.1, we conclude that the sequence {xn} generated by Algorithm 2 converges to a point in F(T)=Argmin(f+g).
Artificial intelligence (AI) and its subfields, like machine learning (ML) and deep learning (DL), have been applied to many domains, such as image processing and data classification. The adoption of these technologies has made possible huge advances in solving complex problems that were previously intractable. AI, for example, has played a key role in the analysis of images for making medical diagnoses, helping diagnose diseases earlier. In image processing, DL programs have remarkably advanced object detection and increased the accuracy of recognition tasks. Numerous additional examples could be given from a variety of industries, where ML and DL models have improved predictive capabilities and helped solve difficult classification problems [32,33].
One of the major building blocks for the development of AI is a type of ML algorithm called a neural network, which is a model inspired by the structure of the human brain, consisting of interconnected layers of nodes (or 'neurons') that process data. In the very early days of neural networks, it was found that multiple-layered neural networks, called deep neural networks, could be used to model complex nonlinear relationships in data. However, traditional training algorithms, such as backpropagation, were computationally intensive and prone to overfitting and local minima [34].
To address these challenges, Huang et al. (2006) [35] introduced the extreme learning machine (ELM), simplifying the training of single hidden-layer, feed-forward neural networks (SLFNs) significantly. ELM randomly initializes the weights and biases that exist between the input and hidden layers and then, using a least squares solution, figures out the output weights. Training time is reduced, and generalization performance is improved using this approach. After their success with ELM, the researchers studied the deeper architectures in order to improve the learning capabilities further. To allow ELM to capture more complex data patterns, Qu et al. (2016) [36] proposed the two-hidden-layer ELM (TELM). The TELM algorithm exhibited improved performance over traditional ELM when working with nonlinear and high-dimensional data.
Xiao et al. (2017) [37] take this concept and expand it by developing the multihidden-layer extreme learning machine (MELM), which uses multiple hidden layers. For the first hidden (random) layer, MELM follows the characteristic random initialization of ELM, whereas the parameters of subsequent hidden layers are iteratively adjusted. This multilayer structure helps the network to better model important data relationships. Thus, it is an excellent network algorithm for regression and classification problems.
Let {(X,T):X∈RN×n,T∈RN×m} be a training set of N distinct samples, where X is the training data matrix, n is the number of input nodes (features), T is the target matrix, and m is the number of output nodes (classes).
In the context of ELM (see Figure 1 for the ELM structure), the training set consists of N samples. The training data matrix X, the target matrix T, the input weight matrix W, and the bias matrix B are defined as follows:
X=[x11⋯x1n⋮⋱⋮xN1⋯xNn]∈RN×n,T=[t11⋯t1m⋮⋱⋮tN1⋯tNm]∈RN×m, |
W=[w11⋯w1L⋮⋱⋮wn1⋯wnL]∈Rn×L,B=[b11⋯b1L⋮⋱⋮bN1⋯bNL]∈RN×L. |
First, the input data matrix X passes through the model, where it is multiplied by the weight matrix W that connects the input layer to the hidden layer. After multiplying by W, the bias matrix B is added, where L is the number of hidden nodes. The core principle of ELM is that with a sufficiently large number of hidden neurons, the random initialization of the weight matrix W and bias vector B has minimal impact on the model's accuracy. As such, in ELM, W and B are typically initialized randomly without significant consequences on performance [35].
The output of this operation is passed through an activation function g(⋅) at the hidden layer. The purpose of the activation function is to introduce nonlinearity into the model, enabling it to learn complex patterns in the data. Common activation functions include the sigmoid, ReLU, and hyperbolic tangent functions. The choice of an activation function depends on the specific application and the nature of the data.
The hidden layer output matrix H∈RN×L is computed as:
H=g(XW+B)=[g(∑nj=1x1jwj1+b11)⋯g(∑nj=1x1jwjL+b1L)g(∑nj=1x2jwj1+b21)⋯g(∑nj=1x2jwjL+b2L)⋮⋱⋮g(∑nj=1xNjwj1+bN1)⋯g(∑nj=1xNjwjL+bNL)]. |
The ELM model uses the equation Hβ=T, where the weight matrix between the hidden and output layers (output weights) β∈RL×m is defined by
β=[β11⋯β1m⋮⋱⋮βL1⋯βLm]. |
To determine the output weights β, the ELM model minimizes the least-squares error between the predicted output Y and the target output T. This is achieved using the Moore-Penrose pseudoinverse:
β=H†T, |
where H† is the Moore-Penrose pseudoinverse of H. The pseudoinverse H† is computed as:
H†=(HTH)−1HT, |
assuming HTH is invertible. The output of the network is then given by:
Y=Hβ, |
where Y∈RN×m is the predicted output matrix.
The TELM algorithm tries to calculate and update the weights and biases between the first and second hidden layers, as well as between the second hidden layer and the output layer (see Figure 2 for the TELM structure). Initially, the computation is simplified by merging the two hidden layers into one equivalent hidden layer. Output weights are calculated for the combined hidden layer, and then the combined layer is separated, and the model is refined further by updating the weights and biases via additional steps.
The TELM algorithm starts by treating the two hidden layers as one hidden layer to simplify the initialization. The output of this combined hidden layer, denoted by H∈RN×L, is expressed as:
H=g(XW+B), | (4.1) |
where X∈RN×n is the input matrix, W∈Rn×L is the weight matrix of the first hidden layer, B∈RN×L is the bias matrix of the first hidden layer (broadcasted to match the dimensions), L is the number of hidden nodes, and g(⋅) is the activation function applied element-wise. The weight matrix W and bias matrix B are initialized randomly.
Once H is obtained, the output weight matrix β between the second hidden layer and the output layer is determined using:
β=H†T, | (4.2) |
where H† is the Moore-Penrose pseudoinverse of H, and T∈RN×m is the target matrix.
After the initial output weight matrix β is determined, the TELM algorithm separates the two hidden layers, which were previously merged, so that the network now contains two distinct hidden layers. To refine the model, the expected output, H1, of the second hidden layer is computed in a way that minimizes error, with the previously calculated output weight matrix beta acting as a constraint. This is done using β and the target matrix T:
H1=Tβ†, | (4.3) |
where β† is the Moore-Penrose pseudoinverse of the matrix β.
Next, the algorithm updates the weights between the first and second hidden layers in a way that minimizes error, with H1 and H acting as constraints. Observe that, according to the typical algorithm, the expected output of the second hidden layer would be calculated using
H1=g(HW1+B1), | (4.4) |
where W1∈RL×L is the weight matrix between the first and second hidden layers, and B1∈RN×L is the bias matrix for the second hidden layer. Using the expected output H1 and the inverse function of the activation function g(·), the updated weight matrix WHE is calculated as:
WHE=H†Eg−1(H1), | (4.5) |
where WHE=[B1W1]T∈R(L+1)×L, H†E is the Moore-Penrose pseudoinverse of HE=[1H]∈RN×(L+1), and g−1(⋅) is the inverse of the activation function g(⋅).
With the updated weight matrix WHE, the actual output of the second hidden layer H2∈RN×L is updated as follows:
H2=g(HEWHE), | (4.6) |
where H2 represents the final output of the second hidden layer after weight and bias adjustments.
Finally, the output weight matrix βnew∈RL×m between the second hidden layer and the output layer is updated as follows:
βnew=H†2T, | (4.7) |
where H†2 is the Moore-Penrose pseudoinverse of H2. The final output (predicted output matrix) Y∈RN×m of the TELM network is then computed by:
Y=H2βnew. | (4.8) |
Briefly summarized, the TELM first simplifies the network by merging the hidden layers, calculates the output weight matrix as in the one-hidden layer case, and then separates the layers, iteratively refining the weight and bias by a series of updates. This process guarantees that the network can replicate complex relationships in the data by using multiple hidden layers and fine-tuning their parameters.
The multihidden-layer extreme learning machine (MELM) architecture extends ELM and TELM by adding additional hidden layers, improving the learning capabilities of the model. The incorporation of the additional layers enables the network to leverage the data in finer detail, making the model especially effective for complicated tasks. An examination of a model with three hidden layers, as shown in Figure 3, serves to illustrate how MELM works. The strategy is to reduce the three-layer network by replacing the second and third hidden layers with a single combined equivalent hidden layer, thus reducing it to a TELM framework. In this way, the TELM framework is extended for use on more complex network structures.
A 3-layer MELM begins by combining the second and third hidden layers into one. The TELM methodology is then used to derive the output weight matrix, βnew, between the combined second hidden layer and the output layer. After obtaining the initial output weight matrix, βnew, the MELM algorithm then separates the merged hidden layers, resulting in three distinct hidden layers. To further refine the model, the expected output of the third hidden layer, H3∈RN×L, is computed using the updated weight matrix βnew as a constraint:
H3=Tβ†new, | (4.9) |
where β†new∈RL×m is the Moore-Penrose pseudoinverse of the weight matrix βnew.
Observe that, according to the typical algorithm, the expected output of the third hidden layer, H3, would be calculated using
H3=g(H2W2+B2), | (4.10) |
where W2∈RL×L is the weight matrix between the second and third hidden layers, H2 is the output from the second hidden layer, and B2∈RN×L is the bias term for the third hidden layer. The algorithm updates the weights between the second and third hidden layers using the inverse function of the activation function g(·) and the expected output H3. The weight matrix WHE1 is calculated as:
WHE1=H†E1g−1(H3), | (4.11) |
where WHE1=[B2W2]T∈R(L+1)×L, H†E1 is the Moore-Penrose pseudoinverse of HE1=[1H2]∈RN×(L+1), and g−1(⋅) is the inverse of the activation function g(⋅).
With the updated weight matrix WHE1, the actual output of the third hidden layer, H4∈RN×L, is calculated as follows:
H4=g(HE1WHE1), | (4.12) |
where H4 represents the final output of the third hidden layer before reaching the output layer.
Finally, the output weight matrix βfinal∈RL×m between the second hidden layer and the output layer is updated as follows:
βfinal=H†4T. | (4.13) |
The final output (predicted output matrix) Y∈RN×m of the three-hidden-layer ELM network is given by:
Y=H4βfinal. | (4.14) |
This process can be generalized to networks with more than three hidden layers in a natural inductive way. For a network with n hidden layers, the network structure is simplified by merging the last two layers, applying the algorithm for n−1 layers to obtain an output weight matrix, then separating the merged layers, updating the weights between layers n−1 and n, and completing the calculation for the last layer, as shown above in the discussions of TELM and MELM. This approach guarantees that the network can handle still more complicated data patterns with little additional computational cost. Specifically, the computational complexity of this process is O(n), as each additional layer contributes a fixed computational cost, ensuring scalability for deeper networks.
When the Moore-Penrose pseudoinverse matrices in expressions (4.2)–(4.5) and (4.7) exist, the computation of such matrices is usually straightforward. Unfortunately, in cases in which the Moore-Penrose pseudoinverse does not exist, computing the actual inverse directly is impractical or numerically unstable, making the usual approach nontrivial to apply. To overcome this problem, we employ regularization techniques using the least absolute shrinkage and selection operator (lasso) [38], which adds a regularization term to perform stable inverse calculations. This reformulates the calculation as a convex minimization problem. The resulting computation is more robust, and the solution exists.
We can reformulate Eqs (4.2)–(4.5) and (4.7) as follows:
minβ‖Hβ−T‖22+λ‖β‖1, | (4.15) |
minH1‖H1β−T‖22+λ‖H1‖1, | (4.16) |
minWHE‖HEWHE−g−1(H1)‖22+λ‖WHE‖1, | (4.17) |
minβnew‖H2βnew−T‖22+λ‖βnew‖1, | (4.18) |
where λ is the regularization parameter controlling the trade-off between fitting the data and penalizing the complexity of the patterns that the model is able to exploit (i.e., the L1 norm of the coefficients). This regularization ensures that even when the Moore-Penrose pseudoinverse does not exist or the problem is ill-posed, a solution can still be obtained by minimizing the above convex objective functions.
We note that Eqs (4.15)–(4.18) are each in the form of a sum of two convex functions.
minx∈Rn(f(x)+g(x)). |
We employ our algorithm (TIFBSPA) to solve these convex minimization problems.
We present numerical experiments to illustrate the performance of TIFBSPA when applied to convex minimization problems arising from standard classification tasks. In these experiments, the main goal is to demonstrate the performance of our algorithm as compared to the FISTA and PISFBA methods from the literature. The experimental setup, datasets utilized, parameter settings for the algorithms, and the results are discussed in detail.
The specific convex minimization problems we deal with in our experiments are presented in Table 1. Each row of the table shows the functions f(⋅) and g(⋅) that arise in the MELM algorithm (see Section 4.4). In managing the trade-off between data fitting and model complexity, the value λ=10−5 is used. In solving these problems with TIFBSPA, we show how TIFBSPA can address both the accuracy and complexity of the problem we are solving.
Problems | f(⋅) | g(⋅) |
(4.15) | f(β)=‖Hβ−T‖22 | g(β)=λ‖β‖1 |
(4.16) | f(H1)=‖H1β−T‖22 | g(H1)=λ‖H1‖1 |
(4.17) | f(WHE)=‖HEWHE−g−1(H1)‖22 | g(WHE)=λ‖WHE‖1 |
(4.18) | f(βnew)=‖H2βnew−T‖22 | g(βnew)=λ‖βnew‖1 |
We test the performance of our algorithm by applying it to several datasets that vary in their dimensions (i.e., number of features), number of samples, and class distributions. Applying the algorithm to data sets with diverse characteristics allows for a comprehensive study of the algorithm's capability to handle different kinds and amounts of data. Each dataset is divided into training and testing sets. Table 2 provides an overview.
Datasets | Training samples | Testing samples | Features | Classes |
Iris | 105 | 45 | 4 | 3 |
Ionosphere | 245 | 106 | 34 | 2 |
Hypertension | 4453 | 1909 | 9 | 2 |
Weather Type | 9240 | 3960 | 10 | 4 |
The datasets were chosen for their relevance to common classification tasks. Each dataset is briefly described below.
● Iris [39]: A well-known dataset in the ML community, this dataset is used for basic classification algorithm testing. It has 4 features and 3 classes.
● Ionosphere [40]: This is a binary classification using 34 features on a radar signal classification dataset.
● Hypertension: The healthcare related classification task obtained with 9 features and binary outcomes, which is particularly relevant for real-world applications, is presented in this dataset. The data was collected by Sripat Medical Center, Faculty of Medicine, Chiang Mai University, so it was real-world relevant and authentic.
● Weather Type [41]: Test the performance of the algorithm on multi-class classification problems with a more complex dataset containing 10 features and 4 classes. Because this dataset contains outliers, it can be used to test a machine learning model's accuracy and resiliency and provides a good platform to test different types of models.
In the first experiment, we measured the accuracy of each model in solving data classification, defining accuracy as follows:
accuracy=100× correct predictiontotal cases. |
To assess the effectiveness of different machine learning models, we conduct a series of experiments comparing the performance of the MELM (3-hidden-layer ELM), TELM, and ELM. For each dataset, the models are trained and tested across a range of hidden nodes, varying from 50 to 300, to examine how the number of hidden nodes influences mean accuracy. Our proposed fixed-point algorithm (TIFBSPA) is applied to each model, running 500 iterations across 10 executions. The mean accuracy from these executions is calculated and presented to highlight the algorithm's performance consistency. The variation in accuracy of classification results from the randomness of the initial weights and biases in each execution. Hence, it is more accurate to average the above results to give a better view of the model performance.
The results of the first experiment, summarized in Figure 4, demonstrate that in most of the tested datasets, the MELM performs better than both the traditional ELM and the TELM models. The most transparently observed advantage is in the Weather Type dataset (Figure 4d). Here, the MELM's better performance reflects that model's increased ability to capture complicated data trends. High variability and the presence of outliers in the weather data pose problems for models with fewer hidden layers. The three hidden layers of MELM enable the flowability of the model among the data and the acceptance of a greater number of features. This allows modeling of more complicated relationships, increasing the algorithm's accuracy. However, for the Hypertension dataset (Figure 4c), the performance of MELM, TELM, and ELM is more similar. This is likely because the Hypertension dataset is well-cleaned and preprocessed, reducing the need for a deeper model like MELM to handle the data's complexity. As a result, the simpler models perform comparably well. Because it exhibited the best performance in most datasets and offered the possibility to deal with more complex data, MELM was chosen as the model for the second experiment of the study: a comparison of TIFBSPA with FISTA and PISFBA.
In this experiment, we implemented the MELM model using TIFBSPA, FISTA, and PISFBA in order to compare the algorithms' performance across four datasets. All algorithms are run for a maximum of 1000 iterations, and the results report the best performance achieved during this process. The datasets used, Iris (L = 50), Ionosphere (L = 80), Hypertension (L = 100), and Weather Type (L = 60), are configured with optimal hidden nodes based on Figure 4. In particular, the number of hidden nodes for each dataset was selected to balance accuracy, computational efficiency, and the risk of overfitting, as larger numbers of nodes provide diminishing returns in accuracy while increasing complexity.
For the numerical experiments, it is crucial to carefully select the algorithm parameters, as they directly influence convergence behavior and the quality of the solution. Table 3 summarizes the parameters used for TIFBSPA, FISTA, and PISFBA. Careful selection of the parameters ensures that each algorithm performs optimally under the same conditions, allowing for fair comparison. The parameters for TIFBSPA are chosen to ensure a balance between convergence speed and solution accuracy. The choices for FISTA and PISFBA are based on recommendations from the literature.
Methods | Setting |
TIFBSPA | βn=αn=1n+1, cn=1Lf, τn=1014n2, μn=nn+1, ρn=1n+1 |
FISTA | t1=1, tn+1=(1+√1+4t2n)2, θn=(tt−1)tn+1 |
PISFBA | αn=βn=0.9nn+1, c=1/Lf, |
θn={min{12n‖xn−xn−1‖},ifxn≠xn−1,0,otherwise. |
The measures being used are accuracy, precision, recall, and F1 score. Since accuracy was already defined in the first experiment, the focus here is on the additional metrics.
● Recall: The proportion of actual positive cases that are correctly predicted.
Recall=TPTP+FN. |
Recall is all about identifying all the positive cases and therefore is important in circumstances where false negatives are costly.
● Precision: The proportion of predicted positive cases that are actually positive.
Precision=TPTP+FP. |
Precision focuses on minimizing false positives, which is essential when false alarms are undesirable.
● F1 Score: The harmonic mean of Precision and Recall, balancing the trade-off between them.
F1 Score=2×Precision×RecallPrecision+Recall. |
F1-Score takes both Precision and Recall and is useful where there is an unequal distribution of data and both false positives and false negatives are costly.
TP, TN, FP, and FN stands for true positives, true negatives, false positives, and false negatives, respectively. The performance of these algorithms, measured using these metrics, is summarized in Tables 4 and 5.
Dataset | Algorithm | Iteration (Best) |
Accuracy (Train) |
Accuracy (Test) |
Recall (Train) |
Recall (Test) |
Iris | TIFBSPA | 69 | 92.38 | 100.00 | 92.38 | 100.00 |
FISTA | 97 | 94.28 | 97.79 | 94.29 | 97.78 | |
PISFBA | 556 | 94.28 | 97.79 | 94.29 | 97.78 | |
Ionosphere | TIFBSPA | 44 | 93.46 | 95.28 | 98.72 | 97.10 |
FISTA | 250 | 91.42 | 94.33 | 100 | 100 | |
PISFBA | 437 | 91.42 | 94.33 | 100 | 100 | |
Hypertension | TIFBSPA | 40 | 89.12 | 89.57 | 90.43 | 90.93 |
FISTA | 967 | 81.47 | 82.21 | 64.84 | 65.04 | |
PISFBA | 107 | 89.38 | 89.52 | 91.14 | 91.41 | |
Weather Type | TIFBSPA | 250 | 86.68 | 86.31 | 86.69 | 86.31 |
FISTA | 997 | 82.15 | 81.33 | 82.15 | 81.34 | |
PISFBA | 254 | 86.16 | 86.08 | 86.17 | 86.09 |
Dataset | Algorithm | Iteration (Best) |
Pre. (Train) |
Pre. (Test) |
F1-Score (Train) |
F1-Score (Test) |
Iris | TIFBSPA | 69 | 92.44 | 100 | 92.36 | 100 |
FISTA | 97 | 94.39 | 97.90 | 94.29 | 97.77 | |
PISFBA | 556 | 95.43 | 97.94 | 95.22 | 97.79 | |
Ionosphere | TIFBSPA | 44 | 93.46 | 95.28 | 95.06 | 96.40 |
FISTA | 250 | 91.42 | 94.33 | 93.69 | 95.83 | |
PISFBA | 437 | 91.42 | 94.33 | 93.69 | 95.83 | |
Hypertension | TIFBSPA | 40 | 86.64 | 86.89 | 88.48 | 88.86 |
FISTA | 967 | 92.96 | 94.29 | 76.37 | 76.98 | |
PISFBA | 107 | 86.58 | 86.46 | 88.80 | 88.86 | |
Weather Type | TIFBSPA | 250 | 86.65 | 86.28 | 86.66 | 86.28 |
FISTA | 997 | 82.16 | 82.15 | 82.05 | 81.23 | |
PISFBA | 254 | 86.16 | 86.09 | 86.16 | 86.08 |
The results show that TIFBSPA performs better than the other methods in terms of test accuracy, with fewer iterations required to achieve an optimal solution. For example, with the Iris dataset, TIFBSPA achieved an optimal solution in 69 iterations, as compared to 97 iterations for FISTA and 556 iterations for PISFBA. Although TIFBSPA does not outperform the other algorithms in every single metric across all cases, it offers a reasonable compromise between speed and accuracy. In some cases, measures like precision and F1 score are a little lower, but they are still reasonable. These results demonstrate that TIFBSPA converges faster and performs comparably or better than popular, existing methods.
In this paper, we proposed a novel two-step inertial algorithm (TIFBSPA) to tackle convex minimization problems when they arise in hierarchical optimization tasks. We proved a weak convergence theorem that the fixed-points of the algorithm are indeed minimizers of the associated convex minimization problems. This algorithm was then applied to a series of data classification tasks using the ELM, TELM and MELM models. All models were reformulated in the form of a series of convex minimization problems, which enabled effective use of the TIFBSPA algorithm. Numerical experiments performed on multiple datasets showed that the MELM model plus our proposed algorithm achieved the best classification accuracy and required fewer iterations to converge compared to FISTA and PISFBA. These results highlight the superior efficiency and performance of TIFBSPA.
We demonstrated that the proposed TIFBSPA algorithm is both theoretically robust and practically effective, making it a valuable tool for addressing machine learning and optimization challenges. This method can be extended to other optimization problems, and future research may investigate how this method can be integrated with other advanced machine learning approaches.
Conceptualization, R. W.; Formal analysis, K. J. and R. W.; Investigation, K. J. and R. W.; Methodology, R. W.; Software, K. J.; Supervision, S. S.; Validation, S. S.; Visualization, R. W.; Writing-original draft, K. J.; Writing-review and editing, R. W. and S. S.
The authors declare they have not used Artificial Intelligence (AI) tools in the creation of this article.
The authors thank the referees for their helpful comments and valuable suggestions. K. Janngam was supported by the CMU Proactive Researcher Program, Chiang Mai University, under Contract No. 568/2567. S. Suantai would like to thank Chiang Mai University, Fundamental Fund 2025, Chiang Mai University. R. Wattanataweekul would like to thank Ubon Ratchathani University.
The authors declare no conflict of interest.
[1] | S. Boyd, L. Vandenberghe, Convex optimization, Cambridge University Press, 2004. |
[2] | C. M. Bishop, Pattern recognition and machine learning, New York: Springer, 2006. |
[3] | P. L. Combettes, J. C. Pesquet, Proximal splitting methods in signal processing, In: Fixed-point algorithms for inverse problems in science and engineering, New York: Springer, 2011. https://doi.org/10.1007/978-1-4419-9569-8_10 |
[4] | H. Markowitz, Portfolio selection, J. Financ., 7 (1952), 77–91. https://doi.org/10.1111/j.1540-6261.1952.tb01525.x |
[5] | Y. Nesterov, Introductory lectures on convex optimization: A basic course, New York: Springer, 2013. https://doi.org/10.1007/978-1-4419-8853-9 |
[6] |
R. E. Bruck Jr., On the weak convergence of an ergodic iteration for the solution of variational inequalities for monotone operators in Hilbert space, J. Math. Anal. Appl., 61 (1977), 159–164. https://doi.org/10.1016/0022-247X(77)90152-4 doi: 10.1016/0022-247X(77)90152-4
![]() |
[7] |
P. L. Lions, B. Mercier, Splitting algorithms for the sum of two nonlinear operators, SIAM J. Numer. Anal., 16 (1979), 964–979. https://doi.org/10.1137/0716071 doi: 10.1137/0716071
![]() |
[8] |
A. Cabot, Proximal point algorithm controlled by a slowly vanishing term: applications to hierarchical minimization, SIAM J. Optim., 15 (2005), 555–572. https://doi.org/10.1137/S105262340343467X doi: 10.1137/S105262340343467X
![]() |
[9] |
H. K. Xu, Averaged mappings and the gradient-projection algorithm, J. Optim. Theory Appl., 150 (2011), 360–378. https://doi.org/10.1007/s10957-011-9837-z doi: 10.1007/s10957-011-9837-z
![]() |
[10] | J. J. Moreau, Fonctions convexes duales et points proximaux dans un espace hilbertien, C. R. Acad. Sci. Paris Ser. A Math., 255 (1962), 2897–2899. |
[11] |
P. L. Combettes, V. R. Wajs, Signal recovery by proximal forward-backward splitting, Multiscale Model. Sim., 4 (2005), 1168–1200. https://doi.org/10.1137/050626090 doi: 10.1137/050626090
![]() |
[12] |
L. Bussaban, S. Suantai, A. Kaewkhao, A parallel inertial S-iteration forward-backward algorithm for regression and classification problems, Carpathian J. Math., 36 (2020), 35–44. https://doi.org/10.37193/CJM.2020.01.04 doi: 10.37193/CJM.2020.01.04
![]() |
[13] |
M. Bačák, S. Reich, The asymptotic behavior of a class of nonlinear semigroups in Hadamard spaces, J. Fixed Point Theory Appl., 16 (2014), 189–202. https://doi.org/10.1007/s11784-014-0202-3 doi: 10.1007/s11784-014-0202-3
![]() |
[14] |
K. Janngam, S. Suantai, Y. J. Cho, A. Kaewkhao, R. Wattanataweekul, A novel inertial viscosity algorithm for bilevel optimization problems applied to classification problems, Mathematics, 11 (2023), 3241. https://doi.org/10.3390/math11143241 doi: 10.3390/math11143241
![]() |
[15] |
R. Wattanataweekul, K. Janngam, S. Suantai, A novel two-step inertial viscosity algorithm for bilevel optimization problems applied to image recovery, Mathematics, 11 (2023), 3518. https://doi.org/10.3390/math11163518 doi: 10.3390/math11163518
![]() |
[16] |
A. Beck, M. Teboulle, A fast iterative shrinkage-thresholding algorithm for linear inverse problems, SIAM J. Imaging Sci., 2 (2009), 183–202. https://doi.org/10.1137/080716542 doi: 10.1137/080716542
![]() |
[17] | Y. Nesterov, A method of solving a convex programming problem with convergence rate O(1/k2), Dokl. Akad. Nauk SSSR, 269 (1983), 543–547. |
[18] | A. Kaewkhao, L. Bussaban, S. Suantai, Convergence theorem of inertial P-iteration method for a family of nonexpansive mappings with applications, Thai J. Math., 18 (2020), 1743–1751. |
[19] | P. Thongsri, S. Suantai, New accelerated fixed-point algorithms with applications to regression and classification problems, Thai J. Math., 18 (2020), 2001–2011. |
[20] | K. Janngam, S. Suantai, An accelerated forward-backward algorithm with applications to image restoration problems, Thai J. Math., 19 (2021), 325–339. |
[21] | P. Sae-jia, S. Suantai, A novel algorithm for convex bi-level optimization problems in Hilbert spaces with applications, Thai J. Math., 21 (2023), 625–645. |
[22] |
D. Reem, S. Reich, A. De Pierro, A telescopic Bregmanian proximal gradient method without the global Lipschitz continuity assumption, J. Optim. Theory Appl., 182 (2019), 851–884. https://doi.org/10.48550/arXiv.1804.10273 doi: 10.48550/arXiv.1804.10273
![]() |
[23] |
C. Izuchukwu, M. Aphane, K. O. Aremu, Two-step inertial forward-reflected-anchored-backward splitting algorithm for solving monotone inclusion problems, Comp. Appl. Math., 42 (2023), 351. https://doi.org/10.1007/s40314-023-02485-6 doi: 10.1007/s40314-023-02485-6
![]() |
[24] |
O. S. Iyiola, Y. Shehu, Convergence results of two-step inertial proximal point algorithm, Appl. Numer. Math., 182 (2022), 57–75. https://doi.org/10.1016/j.apnum.2022.07.013 doi: 10.1016/j.apnum.2022.07.013
![]() |
[25] |
D. V. Thong, S. Reich, X. H. Li, P. T. H. Tham, An efficient algorithm with double inertial steps for solving split common fixed-point problems and an application to signal processing, Comp. Appl. Math., 44 (2025), 102 https://doi.org/10.1007/s40314-024-03058-x doi: 10.1007/s40314-024-03058-x
![]() |
[26] | K. Nakajo, K. Shimoji, W. Takahashi, Strong convergence to a common fixed-point of families of nonexpansive mappings in Banach spaces, J. Nonlinear Convex A., 8 (2007), 11. |
[27] | H. H. Bauschke, P. L. Combettes, Convex analysis and monotone operator theory in Hilbert spaces, Cham: Springer, 2017. https://doi.org/10.1007/978-3-319-48311-5 |
[28] | R. E. Bruck, S. Reich, Nonexpansive projections and resolvents of accretive operators in Banach spaces, Houston J. Math., 3 (1977), 459–470. |
[29] |
K. Tan, H. K. Xu, Approximating fixed-points of nonexpansive mappings by the Ishikawa iteration process, J. Math. Anal. Appl., 178 (1993), 301–308. https://doi.org/10.1006/jmaa.1993.1309 doi: 10.1006/jmaa.1993.1309
![]() |
[30] | W. Takahashi, Introduction to nonlinear and convex analysis, Yokohama Publishers, 2009. |
[31] | A. Moudafi, E. Al-Shemas, Simultaneous iterative methods for split equality problem, Trans. Math. Program. Appl., 1 (2013), 1–11. |
[32] |
Y. LeCun, Y. Bengio, G. Hinton, Deep learning, Nature, 521 (2015), 436–444. https://doi.org/10.1038/nature14539 doi: 10.1038/nature14539
![]() |
[33] |
J. Schmidhuber, Deep learning in neural networks: An overview, Neural Netw., 61 (2015), 85–117. https://doi.org/10.1016/j.neunet.2014.09.003 doi: 10.1016/j.neunet.2014.09.003
![]() |
[34] |
D. E. Rumelhart, G. E. Hinton, R. J. Williams, Learning representations by back-propagating errors, Nature, 323 (1986), 533–536. https://doi.org/10.1038/323533a0 doi: 10.1038/323533a0
![]() |
[35] |
G. B. Huang, Q. Y. Zhu, C. K. Siew, Extreme learning machine: Theory and applications, Neurocomputing, 70 (2006), 489–501. https://doi.org/10.1016/j.neucom.2005.12.126 doi: 10.1016/j.neucom.2005.12.126
![]() |
[36] |
B. Y. Qu, B. F. Lang, J. J. Liang, A. K. Qin, O. D. Crisalle, Two-hidden-layer extreme learning machine for regression and classification, Neurocomputing, 175 (2016), 826–834. https://doi.org/10.1016/j.neucom.2015.11.009 doi: 10.1016/j.neucom.2015.11.009
![]() |
[37] |
D. Xiao, B. Li, Y. Mao, A multiple hidden layers extreme learning machine method and its application, Math. Probl. Eng., 2017 (2017), 4670187. https://doi.org/10.1155/2017/4670187 doi: 10.1155/2017/4670187
![]() |
[38] |
R. Tibshirani, Regression shrinkage and selection via the lasso, J. R. Stat. Soc. B, 58 (1996), 267–288. https://doi.org/10.1111/j.2517-6161.1996.tb02080.x doi: 10.1111/j.2517-6161.1996.tb02080.x
![]() |
[39] | R. A. Fisher, Iris, UCI machine learning repository, 1988. https://doi.org/10.24432/C56C76 |
[40] | V. Sigillito, S. Wing, L. Hutton, K. Baker, Ionosphere, UCI machine learning repository, 1989. |
[41] | Nikhil7280, Weather type classification dataset, Kaggle, 2021. Available from: https://www.kaggle.com/datasets/nikhil7280/weather-type-classification/data. |
Problems | f(⋅) | g(⋅) |
(4.15) | f(β)=‖Hβ−T‖22 | g(β)=λ‖β‖1 |
(4.16) | f(H1)=‖H1β−T‖22 | g(H1)=λ‖H1‖1 |
(4.17) | f(WHE)=‖HEWHE−g−1(H1)‖22 | g(WHE)=λ‖WHE‖1 |
(4.18) | f(βnew)=‖H2βnew−T‖22 | g(βnew)=λ‖βnew‖1 |
Datasets | Training samples | Testing samples | Features | Classes |
Iris | 105 | 45 | 4 | 3 |
Ionosphere | 245 | 106 | 34 | 2 |
Hypertension | 4453 | 1909 | 9 | 2 |
Weather Type | 9240 | 3960 | 10 | 4 |
Methods | Setting |
TIFBSPA | βn=αn=1n+1, cn=1Lf, τn=1014n2, μn=nn+1, ρn=1n+1 |
FISTA | t1=1, tn+1=(1+√1+4t2n)2, θn=(tt−1)tn+1 |
PISFBA | αn=βn=0.9nn+1, c=1/Lf, |
θn={min{12n‖xn−xn−1‖},ifxn≠xn−1,0,otherwise. |
Dataset | Algorithm | Iteration (Best) |
Accuracy (Train) |
Accuracy (Test) |
Recall (Train) |
Recall (Test) |
Iris | TIFBSPA | 69 | 92.38 | 100.00 | 92.38 | 100.00 |
FISTA | 97 | 94.28 | 97.79 | 94.29 | 97.78 | |
PISFBA | 556 | 94.28 | 97.79 | 94.29 | 97.78 | |
Ionosphere | TIFBSPA | 44 | 93.46 | 95.28 | 98.72 | 97.10 |
FISTA | 250 | 91.42 | 94.33 | 100 | 100 | |
PISFBA | 437 | 91.42 | 94.33 | 100 | 100 | |
Hypertension | TIFBSPA | 40 | 89.12 | 89.57 | 90.43 | 90.93 |
FISTA | 967 | 81.47 | 82.21 | 64.84 | 65.04 | |
PISFBA | 107 | 89.38 | 89.52 | 91.14 | 91.41 | |
Weather Type | TIFBSPA | 250 | 86.68 | 86.31 | 86.69 | 86.31 |
FISTA | 997 | 82.15 | 81.33 | 82.15 | 81.34 | |
PISFBA | 254 | 86.16 | 86.08 | 86.17 | 86.09 |
Dataset | Algorithm | Iteration (Best) |
Pre. (Train) |
Pre. (Test) |
F1-Score (Train) |
F1-Score (Test) |
Iris | TIFBSPA | 69 | 92.44 | 100 | 92.36 | 100 |
FISTA | 97 | 94.39 | 97.90 | 94.29 | 97.77 | |
PISFBA | 556 | 95.43 | 97.94 | 95.22 | 97.79 | |
Ionosphere | TIFBSPA | 44 | 93.46 | 95.28 | 95.06 | 96.40 |
FISTA | 250 | 91.42 | 94.33 | 93.69 | 95.83 | |
PISFBA | 437 | 91.42 | 94.33 | 93.69 | 95.83 | |
Hypertension | TIFBSPA | 40 | 86.64 | 86.89 | 88.48 | 88.86 |
FISTA | 967 | 92.96 | 94.29 | 76.37 | 76.98 | |
PISFBA | 107 | 86.58 | 86.46 | 88.80 | 88.86 | |
Weather Type | TIFBSPA | 250 | 86.65 | 86.28 | 86.66 | 86.28 |
FISTA | 997 | 82.16 | 82.15 | 82.05 | 81.23 | |
PISFBA | 254 | 86.16 | 86.09 | 86.16 | 86.08 |
Problems | f(⋅) | g(⋅) |
(4.15) | f(β)=‖Hβ−T‖22 | g(β)=λ‖β‖1 |
(4.16) | f(H1)=‖H1β−T‖22 | g(H1)=λ‖H1‖1 |
(4.17) | f(WHE)=‖HEWHE−g−1(H1)‖22 | g(WHE)=λ‖WHE‖1 |
(4.18) | f(βnew)=‖H2βnew−T‖22 | g(βnew)=λ‖βnew‖1 |
Datasets | Training samples | Testing samples | Features | Classes |
Iris | 105 | 45 | 4 | 3 |
Ionosphere | 245 | 106 | 34 | 2 |
Hypertension | 4453 | 1909 | 9 | 2 |
Weather Type | 9240 | 3960 | 10 | 4 |
Methods | Setting |
TIFBSPA | βn=αn=1n+1, cn=1Lf, τn=1014n2, μn=nn+1, ρn=1n+1 |
FISTA | t1=1, tn+1=(1+√1+4t2n)2, θn=(tt−1)tn+1 |
PISFBA | αn=βn=0.9nn+1, c=1/Lf, |
θn={min{12n‖xn−xn−1‖},ifxn≠xn−1,0,otherwise. |
Dataset | Algorithm | Iteration (Best) |
Accuracy (Train) |
Accuracy (Test) |
Recall (Train) |
Recall (Test) |
Iris | TIFBSPA | 69 | 92.38 | 100.00 | 92.38 | 100.00 |
FISTA | 97 | 94.28 | 97.79 | 94.29 | 97.78 | |
PISFBA | 556 | 94.28 | 97.79 | 94.29 | 97.78 | |
Ionosphere | TIFBSPA | 44 | 93.46 | 95.28 | 98.72 | 97.10 |
FISTA | 250 | 91.42 | 94.33 | 100 | 100 | |
PISFBA | 437 | 91.42 | 94.33 | 100 | 100 | |
Hypertension | TIFBSPA | 40 | 89.12 | 89.57 | 90.43 | 90.93 |
FISTA | 967 | 81.47 | 82.21 | 64.84 | 65.04 | |
PISFBA | 107 | 89.38 | 89.52 | 91.14 | 91.41 | |
Weather Type | TIFBSPA | 250 | 86.68 | 86.31 | 86.69 | 86.31 |
FISTA | 997 | 82.15 | 81.33 | 82.15 | 81.34 | |
PISFBA | 254 | 86.16 | 86.08 | 86.17 | 86.09 |
Dataset | Algorithm | Iteration (Best) |
Pre. (Train) |
Pre. (Test) |
F1-Score (Train) |
F1-Score (Test) |
Iris | TIFBSPA | 69 | 92.44 | 100 | 92.36 | 100 |
FISTA | 97 | 94.39 | 97.90 | 94.29 | 97.77 | |
PISFBA | 556 | 95.43 | 97.94 | 95.22 | 97.79 | |
Ionosphere | TIFBSPA | 44 | 93.46 | 95.28 | 95.06 | 96.40 |
FISTA | 250 | 91.42 | 94.33 | 93.69 | 95.83 | |
PISFBA | 437 | 91.42 | 94.33 | 93.69 | 95.83 | |
Hypertension | TIFBSPA | 40 | 86.64 | 86.89 | 88.48 | 88.86 |
FISTA | 967 | 92.96 | 94.29 | 76.37 | 76.98 | |
PISFBA | 107 | 86.58 | 86.46 | 88.80 | 88.86 | |
Weather Type | TIFBSPA | 250 | 86.65 | 86.28 | 86.66 | 86.28 |
FISTA | 997 | 82.16 | 82.15 | 82.05 | 81.23 | |
PISFBA | 254 | 86.16 | 86.09 | 86.16 | 86.08 |