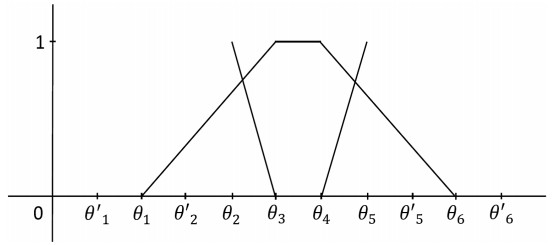
A recursive filtering problem on minimum variance is investigated for a type of two-dimensional systems incorporating noise and a random parameter matrix in the measurement equation, along with random nonlinearity. It methodically describes random variables using statistical characteristics, placing a strong emphasis on the application of random multivariate analysis and computational techniques. A bidirectional time-sequence recursive filter is designed to achieve unbiasedness and reduce error variance effectively. This involves deriving the gain matrix through a completion of squares method and solving a complex difference equation with two independent variances. To facilitate the online implementation of this filter, various formulations and an algorithm are proposed. A numerical study demonstrates the effectiveness of the design in practical applications.
Citation: Shulan Kong, Chengbin Wang, Yawen Sun. A recursive filter for a class of two-dimensional nonlinear stochastic systems[J]. AIMS Mathematics, 2025, 10(1): 1741-1756. doi: 10.3934/math.2025079
[1] | Nadiyah Hussain Alharthi, Mdi Begum Jeelani . Analyzing a SEIR-Type mathematical model of SARS-COVID-19 using piecewise fractional order operators. AIMS Mathematics, 2023, 8(11): 27009-27032. doi: 10.3934/math.20231382 |
[2] | Huda Alsaud, Muhammad Owais Kulachi, Aqeel Ahmad, Mustafa Inc, Muhammad Taimoor . Investigation of SEIR model with vaccinated effects using sustainable fractional approach for low immune individuals. AIMS Mathematics, 2024, 9(4): 10208-10234. doi: 10.3934/math.2024499 |
[3] | Maria M. Martignoni, Proton Rahman, Amy Hurford . Rotational worker vaccination provides indirect protection to vulnerable groups in regions with low COVID-19 prevalence. AIMS Mathematics, 2022, 7(3): 3988-4003. doi: 10.3934/math.2022220 |
[4] | Muhammad Farman, Ali Akgül, Kottakkaran Sooppy Nisar, Dilshad Ahmad, Aqeel Ahmad, Sarfaraz Kamangar, C Ahamed Saleel . Epidemiological analysis of fractional order COVID-19 model with Mittag-Leffler kernel. AIMS Mathematics, 2022, 7(1): 756-783. doi: 10.3934/math.2022046 |
[5] | Moh. Mashum Mujur Ihsanjaya, Nanang Susyanto . A mathematical model for policy of vaccinating recovered people in controlling the spread of COVID-19 outbreak. AIMS Mathematics, 2023, 8(6): 14508-14521. doi: 10.3934/math.2023741 |
[6] | Asma Hanif, Azhar Iqbal Kashif Butt, Tariq Ismaeel . Fractional optimal control analysis of Covid-19 and dengue fever co-infection model with Atangana-Baleanu derivative. AIMS Mathematics, 2024, 9(3): 5171-5203. doi: 10.3934/math.2024251 |
[7] | Mdi Begum Jeelani, Abeer S Alnahdi, Rahim Ud Din, Hussam Alrabaiah, Azeem Sultana . Mathematical model to investigate transmission dynamics of COVID-19 with vaccinated class. AIMS Mathematics, 2023, 8(12): 29932-29955. doi: 10.3934/math.20231531 |
[8] | Sara Salem Alzaid, Badr Saad T. Alkahtani . Real-world validation of fractional-order model for COVID-19 vaccination impact. AIMS Mathematics, 2024, 9(2): 3685-3706. doi: 10.3934/math.2024181 |
[9] | Shao-Wen Yao, Muhammad Farman, Maryam Amin, Mustafa Inc, Ali Akgül, Aqeel Ahmad . Fractional order COVID-19 model with transmission rout infected through environment. AIMS Mathematics, 2022, 7(4): 5156-5174. doi: 10.3934/math.2022288 |
[10] | Salah Boulaaras, Ziad Ur Rehman, Farah Aini Abdullah, Rashid Jan, Mohamed Abdalla, Asif Jan . Coronavirus dynamics, infections and preventive interventions using fractional-calculus analysis. AIMS Mathematics, 2023, 8(4): 8680-8701. doi: 10.3934/math.2023436 |
A recursive filtering problem on minimum variance is investigated for a type of two-dimensional systems incorporating noise and a random parameter matrix in the measurement equation, along with random nonlinearity. It methodically describes random variables using statistical characteristics, placing a strong emphasis on the application of random multivariate analysis and computational techniques. A bidirectional time-sequence recursive filter is designed to achieve unbiasedness and reduce error variance effectively. This involves deriving the gain matrix through a completion of squares method and solving a complex difference equation with two independent variances. To facilitate the online implementation of this filter, various formulations and an algorithm are proposed. A numerical study demonstrates the effectiveness of the design in practical applications.
In 1965, Zadeh [56] presented fuzzy set theory. Later, Atanasov [10] generalized the notion of a fuzzy set and added the notion of an intuitionistic fuzzy set. One of the underlying issues of fuzzy arithmetic and fuzzy decision-making is the ranking of fuzzy numbers. Before the decision-maker can act, fuzzy numbers should be ranked. Real numbers can be ordered linearly by the connection ≤ or ≥, however, and fuzzy numbers do not have this type of disparity. Because a probable distribution depicts fuzzy numbers, they could also overlap, making it challenging to determine whether one fuzzy number is either larger or smaller than another. A ranking component, which outlines each fuzzy number further into a real line in which a natural order persists, is an efficient method to order the fuzzy numbers. Ramesh [49] compares the notion of ranking function for making comparisons of normal fuzzy numbers.
Abbasbandy and Hajjari [1] developed an innovative method for classifying trapezoidal fuzzy numbers. Wang and Kerre [52] proposed ordering features for fuzzy quantities. Angelov [8] adhered the Bellman and Zadeh [12] fuzzy optimization approach to intuitionistic fuzzy optimization. Numerous authors, including Jana and Roy [30], Mahapatra et al. [35], Dubey et al. [20], Mukherjee and Basu [39] have investigated the issue of optimization in an intuitionistic fuzzy background. One initiative to model the decision-making challenge with ambiguous quantities is to treat such imprecise quantities as intuitionistic fuzzy numbers. Consequently, the analogy of fuzzy numbers is required in the intuitionistic fuzzy optimisation problem. To create ranking systems for intuitionistic fuzzy numbers, comparisons of these erroneous numbers are necessary.
Between intuitionistic fuzzy sets, Atanassov identified four fundamental distances: "The Hamming, normalised Hamming, Euclidean, and normalised Euclidean distances". Szmidt and Kacprzyk [51] added to this principle and suggested a new definition of distance between intuitionistic fuzzy sets. Wang and Xin [53] also investigated the striking similarities and detachments between intuitionistic fuzzy sets by presenting some new axioms. Besides this, Nayagam et al. [41] and Nehi [42] also have investigated the ranking of intuitionistic fuzzy numbers. Li [34] defined and implemented a ratio methodology for triangular intuitionistic fuzzy numbers to MADM. In literature, there are additional ranking techniques that have been developed by authors like Kumar and Kaur [33], Zhang and Yu [57], Esmailzadeh and Esmailzadeh [22] and Papakostas et al. [44]. By adding the valuation and ambiguity indexes of a trapezoidal intuitionistic fuzzy number, De and Das [19] were capable of describing a ranking function.
The centroid approach of ranking intuitionistic fuzzy numbers was introduced by Nishad et al. [43]. Bharati and Singh [13,14] have explored intuitionistic fuzzy multiple objective programming and implemented it in agricultural planning and control. In a two-stage time-minimizing transportation concern, Bharati and Malhotra [15] used intuitionistic fuzzy sets. A novel algorithm for ranking intuitionistic fuzzy digits using the centroid method was put forth by Prakash et al. [45]. Mitchell [37] introduced some techniques for ranking intuitively fuzzy numbers. To use the intuitionistic fuzzy number's anticipated interval, Grzegorzewski [26] suggested a ranking and having-to-order method for intuitionistic fuzzy numbers. This is entirely predicated on the possible values for the fuzzy number first presented in Chiao [17]. An approach for ranking fuzzy numbers using the circumcenter of centroids and an indicator of modality was presented by Rao and Shankar [46]. Nasseri et al. [40] introduce an addition to using the circumcenter of centroids to rank fuzzy numbers with the aid of an area method. Roseline and Amirtharaj [50] presented intinuistic fuzzy numbers using distance methods that rely on the circumcenter of centroids.
Additionally, Yager [54,55] expanded upon the idea of PFS and introduced a new definition known as a q-rung orthopair fuzzy set (q-ROFS). Chen [16] suggested m-polar FS, while Zhang [57] established bipolar FS and its relationships. Akram [2] investigated the theory, procedures, and applications of m-polar F graphs in DM. Riaz and Hashmi [47] proposed the cutting-edge idea of a linear Diophantine fuzzy set (LDFS). The research on LDFSs has recently expanded significantly. Iampan et al. [29] researched linear Diophantine fuzzy Einstein aggregation operators, spherical linear Diophantine fuzzy, and linear Diophantine fuzzy relations concerning decision-making issues. Developed a fresh method for the COVID-19 q-linear Diophantine fuzzy emergency decision support system. Algebraic linear Diophantine fuzzy structures were researched by Kamac [31]. Khan et al. [32] use triangular linear Diophantine fuzzy numbers to solve linear and quadratic equations. There are several authors who studied different applications of generalized fuzzy set models, for instance, Ali et al. [3,4,5,6], Ashraf et al. [9], Ayub et al. [11], Das and Granados [18], Farid et al. [23], Gupta et al. [27], Hashmi et al. [28], Mahmood et al. [36], Mohammad et al. [38] and Riaz and Farid [48].
The domain principle of intuitionistic fuzzy set (IFS), interval-valued intuitionistic fuzzy set (IVIFS), Pythagorean fuzzy set (PFS), interval-valued Pythagorean fuzzy set (IVPFS) and q-rung orthopair fuzzy set (q-ROFS) have several actual applications in diverse fields. But researchers found some limitations to apply these concepts in much uncertain problems due to some issues related to membership and non-membership grades. For instance, in all these theories the researchers cannot choose 1 for membership and 1 for no-membership, if someone choose 1 for membership and 1 for no-membership the 1q+1q>1. In order to remedy the issues, firstly, Riaz and Hashmi introduce the novel idea of linear Diophantine fuzzy set (LDFS). In LDFS concept, they use the reference parameters similar to membership and non-membership grades makes it most accommodating in the direction of modeling uncertainties in actual existence issues. This research proposes a novel circumcenter-based algorithm for ranking LD fuzzy numbers. A trapezoid is initially divided into three segments in a trapezoidal LD fuzzy number, with the first, second, and third parts consecutively being a triangle, a rectangle, and a triangle. Next, the centroids of each of these three components are computed and their circumcenters. To rank LD fuzzy numbers, a ranking algorithm is lastly specified as the circumcenter position plus the original position. The centroid of the trapezoid, which serves as the trapezoid's balance point, is used as a point of reference in most ranking algorithms suggested in the literature. But since all of the centroids' vertices are fairly different from this point, the centroids' circumcenter could be considered a significantly balanced location.
This section is dedicated to reviewing some fundamental ideas essential for comprehending the dominant model.
Definition 2.1. [56] Consider a non-empty set X as the universe of discourse. Then a fuzzy set ξ in X is defined as follows:
ξ={(θ,μξ(θ))|θ∈X}, | (2.1) |
where μξ(θ):X⟶[0,1] is the membership degree.
Definition 2.2. [24] A fuzzy set ξ defined on the universe set X is said to be normal iff μξ(θ)=1.
Definition 2.3. [21] A fuzzy set ξ of universe set X is said to be convex iff
μξ(λx+(1−λ)y)≥min(μξ(x),μξ(y)) ∀x,y∈Xandλ∈[0,1]. | (2.2) |
Definition 2.4. [21] A fuzzy set ξ of universe set X is a fuzzy number iff ξ is normal and convex on X.
A real fuzzy number ξ is described as any fuzzy subset of the real line R with membership function μξ(θ) possessing the following properties:
● μξ is a continuous mapping from R to the closed interval [0,1].
● ξ is normalized : there exist t∈R such that μξ(t)=1.
● Convexity of ξ: i.e., u,w∈R, if t≤u≤w, then μξ(u)≥min{μξ(t),μξ(w)}.
● Boundness of support: i.e., ∃S∈R and ∀t∈R, if |t|≥S, then μξ(t)=0.
Definition 2.5. [10] An intuitionistic fuzzy set ϖ in X defined by
ϖ={(θ,⟨αϖ(θ),βϖ(θ)⟩):θ∈X}, | (2.3) |
where αϖ:X⟶[0,1] and βϖ:X⟶[0,1] are the membership degree and non-membership degree, respectively, with the condition:
0≤αϖ(θ)+βϖ(θ)≤1. | (2.4) |
The hesitation degree of IFS ϖ defined in X is denoted as πϖ(θ). It is determined by the following expression:
πϖ(θ)=1−αϖ(θ)−βϖ(θ). | (2.5) |
Definition 2.6. [47] Let X be the universe. A linear Diophantine fuzzy set (LDFS) £R on X is defined as follows:
£R={(θ,⟨ζτR(θ),ηυR(θ)⟩,⟨α(θ),β(θ)⟩):θ∈X}, | (2.6) |
where ζτR(θ),ηυR(θ),α(θ),β(θ)∈[0,1] such that
0≤α(θ)ζτR(θ)+β(θ)ηυR(θ)≤1, ∀θ∈X,0≤α(θ)+β(θ)≤1. | (2.7) |
The hesitation part can be written as
ϱπR=1−(α(θ)ζτR(θ)+β(θ)ηυR(θ)), | (2.8) |
where ϱ is the reference parameter.
Definition 2.7. [47] An absolute LDFS on X can be written as
1£R={(θ,⟨1,0⟩,⟨1,0⟩:θ∈X}, | (2.9) |
and empty or null LDFS can be expressed as
0£R={(θ,⟨0,1⟩,⟨0,1⟩:θ∈X}. | (2.10) |
Definition 2.8. [47] Let £R={(θ,⟨ζτR(θ),ηυR(θ)⟩,⟨α(θ),β(θ)⟩):θ∈X} be an LDFS. For any constants s,t,u,v∈[0,1] such that 0≤su+tv≤1 with 0≤u+v≤1, define the (⟨s,t⟩,⟨u,v⟩)-cut of £R as follows:
£⟨s,t⟩R⟨u,v⟩={θ∈X:ζτR(θ)≥s, ηυR(θ)≤t, α(θ)≥u, β(θ)≤v}. | (2.11) |
Definition 2.9. [32] A LDF number £R is
● a LDF subset of the real line R,
● normal, i.e., there is any θ0∈R such that ζτR(θ0)=1, ηυR(θ0)=0, α(θ0)=1, β(θ0)=0,
● convex for the membership functions ζτR and α, i.e.,
ζτR(λθ1+(1−λ)θ2)≥min{ζτR(θ1),ζτR(θ2)} ∀θ1,θ2∈R, λ∈[0,1],α(λθ1+(1−λ)θ2)≥min{α(θ1),α(θ2)} ∀θ1,θ2∈R, λ∈[0,1], | (2.12) |
● concave for the nonmembership functions ηυR and β, i.e.,
ηυR(λθ1+(1−λ)θ2)≤max{ηυR(θ1),ηυR(θ2)} ∀θ1,θ2∈R, λ∈[0,1],β(λθ1+(1−λ)θ2)≤max{β(θ1),β(θ2)} ∀θ1,θ2∈R, λ∈[0,1]. | (2.13) |
Definition 2.10. Let £R be a trapezoidal LDFN (TrapLDFN) on R with the following membership functions (ζτR and α) and non-membership functions (ηυR and β):
ζτR(x)={0x<θ1x−θ1θ3−θ1θ1≤x≤θ31θ3≤x≤θ4θ6−xθ6−θ4θ4≤x≤θ60θ6<x, | (2.14) |
ηυR(x)={0x<θ2θ3−xθ3−θ2θ2≤x≤θ30θ3≤x≤θ4x−θ4θ5−θ4θ4≤x≤θ50θ5<x, | (2.15) |
where θ1≤θ2≤θ3≤θ4≤θ5≤θ6 for all x∈R. The figure of (θ1,θ2,θ3,θ4,θ5,θ6) is shown in Figure 1.
α(x)={0x<θ′2x−θ′2θ3−θ′2θ′2≤x≤θ31θ3≤x≤θ4θ′5−xθ′5−θ4θ4≤x≤θ′50θ′5<x, | (2.16) |
β(x)={0x<θ′1θ3−xθ3−θ′1θ′1≤x≤θ30θ3≤x≤θ4x−θ4θ′6−θ4θ4≤x≤θ′60θ′6<x, | (2.17) |
where θ′1≤θ′2≤θ3≤θ4≤θ′5≤θ′6 for all x∈R. The figure of (θ′1,θ′2,θ3,θ4,θ′5,θ′6) is shown in Figure 2.
The figure of £RTrapLDFN is shown in Figure 3.
Definition 2.11. Consider a TrapLDFN £RTrapLDFN={(θ1,θ2,θ3,θ4,θ5,θ6)(θ′1,θ′2,θ3,θ4,θ′5,θ′6). Then
(i) s-cut set of £RTrapLDFN is a crisp subset of R, which is defined as follows
£sRTrapLDFN={x∈X:ζτR(x)≥s}=[ζτR(s)_,¯ζτR(s)]=[θ1+s(θ3−θ1),θ6−s(θ6−θ4)], | (2.18) |
(ii) t-cut set of £RTrapLDFN is a crisp subset of R, which is defined as follows
£tRTrapLDFN={x∈X:ηνR(x)≤t}=[ηνR(t)_,¯ηνR(t)]=[θ3−t(θ3−θ2),θ4+t(θ5−θ4)], | (2.19) |
(iii) u-cut set of £RTrapLDFN is a crisp subset of R, which is defined as follows
£uRTrapLDFN={x∈X:α(x)≥u}=[α(u)_,¯α(u)]=[θ′2+u(θ3−θ′2),θ′5−u(θ′5−θ4)], | (2.20) |
(iv) v-cut set of £RTrapLDFN is a crisp subset of R, which is defined as follows
£vRTrapLDFN={x∈X:β(x)≤v}=[β(v)_,¯β(v)]=[θ3−v(θ3−θ′1),θ4+v(θ′6−θ4)]. | (2.21) |
We can denote the (⟨s,t⟩,⟨u,v⟩)-cut of £RTrapLDFN={(θ1,θ2,θ3,θ4,θ5,θ6)(θ′1,θ′2,θ3,θ4,θ′5,θ′6) by
(£RTrapLDFN)⟨s,t⟩⟨u,v⟩={([ζτR(s)_,¯ζτR(s)],[ηνR(t)_,¯ηνR(t)]),([α(u)_,¯α(u)],[β(v)_,¯β(v)]). |
We denote the set of all TrapLDFN on R by £RTrapLDFN(R).
In this part, we determine the centroid location of the trapezoidal linear Diaphontine fuzzy number (TrapLDFN). The geometric core of a trapezoidal Linear diophantine fuzzy number is used in the process of ranking TrapLDFNs with a centroid index. Values on the horizontal and vertical axes correlate to the geometric centre.
Consider a TrapLDFN £RTrapLDFN={(θ1,θ2,θ3,θ4,θ5,θ6)(θ′1,θ′2,θ3,θ4,θ′5,θ′6), whose membership function can be defined as follows:
ζτR(x)={0x<θ1fLA(x)θ1≤x≤θ31θ3≤x≤θ4fRA(x)θ4≤x≤θ60θ6≤x, | (3.1) |
ηυR(x)={0x<θ2gLA(x)θ2≤x≤θ30θ3≤x≤θ4gRA(x)θ4≤x≤θ50θ5≤x, | (3.2) |
α(x)={0x<θ′2f′LA(x)θ′2≤x≤θ31θ3≤x≤θ4f′RA(x)θ4≤x≤θ′50θ′5≤x, | (3.3) |
β(x)={0x<θ′1g′LA(x)θ′1≤x≤θ30θ3≤x≤θ4g′RA(x)θ4≤x≤θ′60θ′6≤x. | (3.4) |
Where
fLA:R⟶[0,1], fRA:R⟶[0,1],gLA:R⟶[0,1], gRA:R⟶[0,1],f′LA:R⟶[0,1], f′RA:R⟶[0,1],g′LA:R⟶[0,1] and g′RA:R⟶[0,1], | (3.5) |
are called the sides of TrapLDFN, where fLA, gRA, f′LA and g′RA are non-decreasing and fRA, gLA, f′RA and g′LA are non-increasing functions. Therefore the inverse functions of fLA, fRA, gLA, gRA, f′LA, f′RA, g′LA and g′RA exist which are also of the same nature. Let
hLA:[0,1]⟶R, hRA:[0,1]⟶R,kLA:[0,1]⟶R, kRA:[0,1]⟶R,h′LA:[0,1]⟶R, h′RA:[0,1]⟶R,k′LA:[0,1]⟶R and k′RA:[0,1]⟶R, | (3.6) |
be the inverse functions of fLA, fRA, gLA, gRA, f′LA, f′RA, g′LA and g′RA respectively. Then, hLA, hRA, kLA, kRA, h′LA, h′RA, k′LA and k′RA should be integrable on R. In the case of the above defined TrapLDFN, the above inverse functions can be analytically expressed as follows:
hLA(y)=θ1+(θ3−θ1)y0≤y≤1,hRA(y)=θ6+(θ4−θ6)y0≤y≤1,kLA(y)=θ3+(θ2−θ3)y0≤y≤1,kRA(y)=θ4+(θ5−θ4)y0≤y≤1,h′LA(y)=θ′2+(θ3−θ′2)y0≤y≤1,h′RA(y)=θ′5+(θ4−θ′5)y0≤y≤1,k′LA(y)=θ3+(θ′1−θ3)y0≤y≤1,k′RA(y)=θ4+(θ′6−θ4)y0≤y≤1. | (3.7) |
The centroid point of the TrapLDFN is determined as follows. First we find ζτR(x) and ηυR(x), also see the Figure 4.
ζτR(x)=∫θ3θ1xfLA(x)dx+∫θ4θ3xdx+∫θ6θ4xfRA(x)dx∫θ3θ1fLA(x)dx+∫θ4θ3dx+∫θ6θ4fRA(x)dx,=∫θ3θ1x2−xθ1θ3−θ1dx+∫θ4θ3xdx+∫θ6θ4θ6x−x2θ6−θ4dx∫θ3θ1x−θ1θ3−θ1dx+∫θ4θ3dx+∫θ6θ4θ6−xθ6−θ4dx,=1θ3−θ1[x33−x22θ1]θ3θ1+[x22]θ4θ3+1θ6−θ4[θ6x22−x33]θ6θ41θ3−θ1[x22−θ1x]θ3θ1+[x]θ4θ3+1θ6−θ4[θ6x−x22]θ6θ4,ζτR(x)=13[θ26+θ24−θ23−θ21−θ1θ3+θ6θ4θ6+θ4−θ3−θ1], | (3.8) |
ηυR(x)=∫θ3θ2xgLA(x)dx+∫θ4θ3xdx+∫θ5θ4xgRA(x)∫θ3θ2gLA(x)dx+∫θ4θ3dx+∫θ5θ4gRA(x),=∫θ3θ2θ3x−x2θ3−θ2dx+∫θ4θ3xdx+∫θ5θ4x2−θ4xθ5−θ4dx∫θ3θ2θ3−xθ3−θ2+∫θ4θ3dx+∫θ5θ4x−θ4θ5−θ4dx,=1θ3−θ2[θ3x22−x33]θ3θ2+[x22]θ4θ3+1θ5−θ4[x33−θ4x22]θ5θ41θ3−θ2[θ3x−x22]θ3θ2+[x]θ4θ3+1θ5−θ4[x22−θ4x]θ5θ4,ηυR(x)=13[2θ25+2θ24−2θ23−2θ22+θ3θ2−θ5θ4θ5+θ4−θ3−θ2]. | (3.9) |
Similarly, we find α(x) and β(x), also see the Figure 5.
α(x)=∫θ3θ′2xf′LA(x)dx+∫θ4θ3xdx+∫θ′5θ4xf′RA(x)dx∫θ3θ′2f′LA(x)dx+∫θ4θ3dx+∫θ′5θ4f′RA(x)dx,=∫θ3θ′2x2−θ′2xθ3−θ′2dx+∫θ4θ3xdx+∫θ′5θ4θ′5x−x2θ′5−θ4dx∫θ3θ′2x−θ′2θ3−θ′2dx+∫θ4θ3dx+∫θ′5θ4θ′5−xθ′5−θ4dx,=1θ3−θ′2[x33−θ′2x22]θ3θ′2+[x22]θ4θ3+1θ′5−θ4[θ′5x22−x33]θ′5θ41θ3−θ′2[x22−θ′2x]θ3θ′2+[x]θ4θ3+1θ′5−θ4[θ′5x−x23]θ′5θ4,α(x)=13[θ′25+θ24−θ23−θ′22−θ′2θ3+θ4θ′5θ′5+θ4−θ3−θ′2], | (3.10) |
β(x)=∫θ3θ′1xg′LA(x)dx+∫θ4θ3xdx+∫θ′6θ4g′RA(x)dx∫θ3θ′1g′LA(x)dx+∫θ4θ3dx+∫θ′6θ4g′RA(x)dx,=∫θ3θ′1θ3x−x2θ3−θ′1dx+∫θ4θ3xdx+∫θ′6θ4x2−θ4xθ′6−θ4dx∫θ3θ′1θ3−xθ3−θ′1dx+∫θ4θ3dx+∫θ′6θ4x−θ4θ′6−θ4dx,=1θ3−θ′1[θ3x22−x33]θ3θ′1+[x22]θ4θ3+1θ′6−θ4[x33−θ4x22]θ′6θ41θ3−θ′1[θ3x−x22]θ3θ′1+[x]θ4θ3++1θ′6−θ4[x22−θ4x]θ′6θ4,β(x)=13[2θ′26+2θ24−2θ23−2θ′21+θ3θ′1−θ′6θ4θ′6+θ4−θ3−θ′1]. | (3.11) |
Next, we find ζτR(y) and ηυR(y), also see the Figure 6.
ζτR(y)=∫10yhRA(y)dy−∫10yhLA(y)dy∫10hRA(y)dy−∫10hLA(y)dy=∫10(θ6y+θ4y2−θ6y2)dy−∫10(θ1y+θ3y2−θ1y2)dy∫10(θ6+θ4y−θ6y)dy−∫10(θ1+θ3y−θ1y)dy=13[θ6+2θ4−θ1−2θ3θ6+θ4−θ1−θ3]. | (3.12) |
ηυR(y)=∫10ykRAdy−∫10ykLA(y)dy∫10kRAdy−∫10kLA(y)dy,=∫10(θ4y+θ5y2−θ4y2)dy−∫10(θ3y+θ2y2−θ3y2)dy∫10(θ4+θ5y−θ4y)dy−∫10(θ3+θ2y−θ3y)dy,ηυR(y)=13[2θ5+θ4−θ3−2θ2θ5+θ4−θ3−θ2]. | (3.13) |
Similarly, we find α(y) and β(y), also see the Figure 7.
α(y)=∫10yh′RA(y)dy−∫10yh′LA(y)dy∫10h′RA(y)dy−∫10h′LA(y)dy,=∫10(θ′5y+θ4y2−θ′5y2)dy−∫10(θ′2y+θ3y2−θ′2y2)dy∫10(θ′5+θ4y−θ′5y)dy−∫10(θ′2+θ3y−θ′2y)dy,α(y)=13[θ′5+2θ4−2θ3−θ′2θ′5+θ4−θ3−θ′2]. | (3.14) |
β(y)=∫10yk′RA(y)dy−∫10yk′LA(y)dy∫10k′RA(y)dy−∫10k′LA(y)dy,=∫10(θ4y+θ′6y2−θ4y2)dy−∫10(θ3y+θ′1y2−θ3y2)dy∫10(θ4+θ′6y−θ4y)dy−∫10(θ3+θ′1y−θ3y)dy,=13[2θ′6+θ4−θ3−2θ′1θ′6+θ4−θ3−θ′1]. | (3.15) |
Then (⟨ζτR(x),ζτR(y)⟩,⟨α(x),α(y)⟩;⟨ηυR(x),ηυR(y)⟩,⟨β(x),β(y)⟩) gives the centroid of the TrapLDFN.
Definition 3.1. The ranking function of the TrapLDFN A is defined by
ℜ(A)=√[ζτR(x)−ζτR(y)]2+[α(x)−α(y)]2+[ηυR(x)−ηυR(y)]2+[β(x)−β(y)]2 | (3.16) |
which is the Eculidean distance.
As a special case, if in a TrapLDFN, we let θ3=θ4, then we will get a triangular LDFN with parameters θ1≤θ2≤θ3≤θ4≤θ5≤θ6 and θ′1≤θ′2≤θ3≤θ4≤θ′5≤θ′6. It is denoted by £RTriLDFN={(θ1,θ2,θ3,θ5,θ6)(θ′1,θ′2,θ3,θ′5,θ′6). The centroids of the membership functions and non-membership functions of the triangular LDFN respectively are defined as
ζτR(x)=13[θ1+θ3+θ6],ηυR(x)=13[2θ2−θ3+2θ5],α(x)=13[θ′2+θ3+θ′5],β(x)=13[2θ′1−θ3+2θ′6], | (3.17) |
and
ζτR(y)=13,ηυR(y)=23,α(y)=13,β(y)=23. | (3.18) |
Definition 3.2. The ranking function of the triangular LDFN A is defined by
ℜ(A)=√[ζτR(x)−ζτR(y)]2+[α(x)−α(y)]2+[ηυR(x)−ηυR(y)]2+[β(x)−β(y)]2, | (3.19) |
which is the Eculidean distance.
Example 3.3. Consider two TriLDFNs A={(3,5,7,8,13)(1,4,7,10,14) and B={(1,3,9,10,13)(0,4,9,13,15). Then using the proposed method we find ℜ(A),
ζτR(x)=13[θ1+θ3+θ6]=13[3+7+13]=7.67 | (3.20) |
ηυR(x)=13[2θ2−θ3+2θ5]=13[10−7+16]=6.33 | (3.21) |
α(x)=13[θ′2+θ3+θ′5]=13[4+7+10]=7 | (3.22) |
β(x)=13[2θ′1−θ3+2θ′6]=13[2−7+28]=7.67. | (3.23) |
Also
ζτR(y)=0.33, ηυR(y)=0.67, α(y)=0.33, β(y)=0.67. | (3.24) |
Now,
ℜ(A)=√[ζτR(x)−ζτR(y)]2+[α(x)−α(y)]2+[ηυR(x)−ηυR(y)]2+[β(x)−β(y)]2=13.394. | (3.25) |
Now, by using the proposed method we find ℜ(B),
ζτR(x)=13[θ1+θ3+θ6]=13[1+9+13]=7.67 | (3.26) |
ηυR(x)=13[2θ2−θ3+2θ5]=13[6−9+20]=5.67 | (3.27) |
α(x)=13[θ′2+θ3+θ′5]=13[4+9+13]=8.67 | (3.28) |
β(x)=13[2θ′1−θ3+2θ′6]=13[0−9+30]=7. | (3.29) |
Also
ζτR(y)=0.33, ηυR(y)=0.67, α(y)=0.33, β(y)=0.67. | (3.30) |
Now,
ℜ(B)=√[ζτR(x)−ζτR(y)]2+[α(x)−α(y)]2+[ηυR(x)−ηυR(y)]2+[β(x)−β(y)]2=13.729. | (3.31) |
As ℜ(A)<ℜ(B)⟹A<B.
A trapezoid's centroid is regarded as the shape's equilibrium position. The linear Diophantine fuzzy number's membership function trapezoid is divided into three planar figures. These three plane figures are in order, a triangle, a rectangle, and another triangle. The point of reference for defining the ordering of linear Diophantine fuzzy numbers is the circumcenter of the centroids of these three plane figures. Each centroid point (G1 of a triangle, G2 of a rectangle, and G3 of a triangle) is a balancing point for each unique planar figure, and the circumcenter of these centroid points is equidistant from each vertex, which is why this point was chosen as a point of reference (which are centroids). As a result, this point would serve as a more accurate reference point than the trapezoid's centroid.
Take into consideration the trapezoidal linear Diophantine fuzzy number
£RTrapLDFN={(θ1,θ2,θ3,θ4,θ5,θ6)(θ′1,θ′2,θ3,θ4,θ′5,θ′6). | (4.1) |
The centroids of the three plane figures that make up the ζτR(x) are G1=(θ1+2θ33,13),G2=(θ3+θ42,12) and G3=(2θ4+θ63,13) and membership function are G1=(θ1+2θ33,13),G2=(θ3+θ42,12) and G3=(2θ4+θ63,13)) are non-collinear and form a triangle. Since the equation of line G1G3 is y=13 and G2 does not lie on line G1G3. Figure 8 displays the circumcenter of the centroids of ζτR(x).
Likewise, the centroids of the three plane figures that make up the membership function of α(x) are, in a similar manner, G′1=(θ′2+2θ33,13),G′2=(θ3+θ42,12) and G′3=(2θ4+θ′53,13). G′2 does not fall on the line G′1G′3, and its equation is y=13. G′1,G′2and G′3 are therefore non-collinear and form a triangle. Figure 9 displays the circumcenter of the centroids of α(x).
Finding the triangle's circumcenter is our next task. The general equation for a triangle's circumcentre with the coordinates (x1,y1), (x2,y2) and (x3,y3) is
x=−(y1−y2)u+(y1−y3)v2K, | (4.2) |
y=(x1−x2)u−(x1−x3)v2K, | (4.3) |
where
v=x21+y21−x22−y22, | (4.4) |
u=x21+y21−x23−y23, | (4.5) |
K=(x1−x2)(y1−y3)−(x1−x3)(y1−y2). | (4.6) |
The circumcenter ˆSA(ζτR(x))(¯x0,¯y0) of the triangle with vertices G1, G2 and G3 (as shown in Figure 8) of the membership function of the trapezoidal LDFN A={(θ1,θ2,θ3,θ4,θ5,θ6)(θ′1,θ′2,θ3,θ4,θ′5,θ′6) is
ˆSA(ζτR(x))(¯x0,¯y0)=(θ1+2θ3+2θ4+θ66,(2θ1+θ3−3θ4)(2θ6+θ4−3θ3)+512), | (4.7) |
Also, the circumcenter ˆSA(α(x))(¯x′0,¯y′0) of the triangle with vertices G′1, G′2and G′3 (as shown in Figure 9) is
ˆSA(α(x))(¯x′0,¯y′0)=(θ′2+2θ3+2θ4+θ′56,(2θ′2+θ3−3θ4)(2θ′5+θ4−3θ3)+512). | (4.8) |
Separate the TrapLDFN trapezoid of non-membership functions into three plane figures as well. Again, a triangle, a rectangle, and a triangle successively make up these three plane figures. Additionally, the centroids of the three plane figures that make up the non-membership function ηνR(x) are G11=(θ2+2θ33,23), G12=((θ3+θ4)2,12) and G13=((2θ4+θ53,23) correspondingly. The line G11G13 is has the equation y=23, and G12 does not fall on this line.
G11 ,G12 and G13 are therefore not collinear and form a triangle. Figure 10 displays the circumcenter of the centroids of ηνR(x). And the circumcenter ˆSA(ηνR(x)) of the triangle formed by the vertices G11 ,G12 and G13 of the non-membership function of the trapezoidal LDFN £RTrapLDFN={(θ1,θ2,θ3,θ4,θ5,θ6)(θ′1,θ′2,θ3,θ4,θ′5,θ′6) is
ˆSA(ηνR(x))(¯x1,¯y1)=(θ2+2θ3+2θ4+θ56,(2θ2+θ3−3θ4)(−2θ5−θ4+3θ3)+712). | (4.9) |
Similarly, the centroids of the three plane figures of nonmembership function β(x) are G1′1=(θ′1+2θ33,23), G1′2=((θ3+θ4)2,12) and G1′3=((2θ4+θ′63,23) respectively. Equation of the line G1′1G1′3 is y=23 and G1′2 does not lie on the line G1′1G1′3. Therefore G1′1,G1′2 and G1′3 (as shown in Figure 11) are non-collinear and they form a triangle. The circumcenter of centroids of β(x) is
ˆSA(β(x))(¯x′1,¯y′1)=(θ′1+2θ3+2θ4+θ′66,(2θ′1+θ3−3θ4)(−2θ′6−θ4+3θ3)+712). | (4.10) |
Definition 4.1. The ranking function of the trapezoidal LDFN A={(θ1,θ2,θ3,θ4,θ5,θ6)(θ′1,θ′2,θ3,θ4,θ′5,θ′6) for membership function and non-membership function are defined as RA(ζτR(x))=√¯x20+¯y20 , RA(α(x))=√¯x′20+¯y′20 and RA(ηνR(x))=√¯x12+¯y12, RA(β(x))=√¯x′21¯+y′21, then
RA=14(RA(ζτR(x))+RA(α(x))+RA(ηνR(x))+RA(β(x))). | (4.11) |
As an exception, if we allow θ3=θ4 in a TrapLDFN, we will obtain a triangular LDFN with the parameters θ1≤θ2≤θ3≤θ4≤θ5≤θ6 and θ′1≤θ′2≤θ3≤θ4≤θ′5≤θ′6. It is indicated by £RTriLDFN={(θ1,θ2,θ3,θ5,θ6)(θ′1,θ′2,θ3,θ′5,θ′6). The circumcenters of the centroids for the triangular LDFN's membership function and nonmembership function are defined as follows.
ˆSA(ζτR(x))(¯x0,¯y0)=(θ1+4θ3+θ66,4(θ1−θ3)(θ6−θ3)+512), | (4.12) |
ˆSA(α(x))(¯x′0,¯y′0)=(θ′2+4θ3+θ′56,4(θ′2−θ3)(θ′5−θ3)+512), | (4.13) |
and
ˆSA(ηνR(x))(¯x1,¯y1)=(θ2+4θ3+θ56,4(θ2−θ3)(−θ5+θ3)+712), | (4.14) |
ˆSA(β(x))(¯x′1,¯y′1)=(θ′1+4θ3+θ′66,4(θ′1−θ3)(−θ′6+θ3)+712). | (4.15) |
Example 4.2. Consider two TriLDFN A= {(2,4,5,7,9)(1,3,5,8,10) and B={(3,5,7,8,9)(2,4,7,9,9). Then using the proposed method we find ℜ(A),
ˆSA(ζτR(x))(¯x0,¯y0)=(θ1+4θ3+θ66,4(θ1−θ3)(θ6−θ3)+512)=(5.16,−3.58), | (4.16) |
ˆSA(α(x))(¯x′0,¯y′0)=(θ′2+4θ3+θ′56,4(θ′2−θ3)(θ′5−θ3)+512)=(5.16,−1.58), | (4.17) |
ˆSA(ηνR(x))(¯x1,¯y1)=(θ2+4θ3+θ56,4(θ2−θ3)(−θ5+θ3)+712)=(5.33,1.25), | (4.18) |
ˆSA(β(x))(¯x′1,¯y′1)=(θ′1+4θ3+θ′66,4(θ′1−θ3)(−θ′6+θ3)+712)=(5.16,7.25). | (4.19) |
Also,
ℜA(ζτR(x))=√¯x20+¯y20=6.28,ℜA(α(x))=√¯x′20+¯y′20=5.39,ℜA(ηνR(x))=√¯x12+¯y12=5.47,ℜA(β(x))=√¯x′21¯+y′21=8.89. | (4.20) |
Now,
\begin{equation} \Re _{A} = \frac{1}{4}\left( R_{A_{(\zeta _{R}^{\tau }(x))}}+R_{A_{(\alpha (x))}}+R_{A_{(\eta _{\mathfrak{R}}^{\nu }(x))}}+R_{A_{(\beta (x))}}\right) = 6.50. \end{equation} | (4.21) |
Now, using the proposed method we find \Re (B),
\begin{eqnarray} \hat{S}_{B_{(\zeta _{R}^{\tau }(x))}}(\overline{x_{0}}, \overline{y_{0}}) & = &\left( \frac{\theta _{1}+4\theta _{3}+\theta _{6}}{6}, \frac{4\left( \theta _{1}-\theta _{3}\right) \left( \theta _{6}-\theta _{3}\right) +5}{12} \right) = (6.66, -2.25), \end{eqnarray} | (4.22) |
\begin{eqnarray} \hat{S}_{B_{(\alpha (x))}}(\overline{x_{0}^{\prime }}, \overline{ y_{0}^{\prime }}) & = &\left( \frac{\theta _{2}^{^{\prime }}+4\theta _{3}+\theta _{5}^{^{\prime }}}{6}, \frac{4\left( \theta _{2}^{^{\prime }}-\theta _{3}\right) \left( \theta _{5}^{^{\prime }}-\theta _{3}\right) +5}{ 12}\right) = (6.83, -1.58), \end{eqnarray} | (4.23) |
\begin{eqnarray} \hat{S}_{B_{(\eta _{\mathfrak{R}}^{\nu }(x))}}(\overline{x_{1}}, \overline{ y_{1}}) & = &\left( \frac{\theta _{2}+4\theta _{3}+\theta _{5}}{6}, \frac{ 4(\theta _{2}-\theta _{3})(-\theta _{5}+\theta _{3})+7}{12}\right) = (6.83, 1.25), \end{eqnarray} | (4.24) |
\begin{eqnarray} \hat{S}_{B_{(\beta (x))}}(\overline{x_{1}^{\prime }}, \overline{y_{1}^{\prime }}) & = &\left( \frac{\theta _{1}^{^{\prime }}+4\theta _{3}+\theta _{6}^{\prime }}{6}, \frac{4(\theta _{1}^{^{\prime }}-\theta _{3})(-\theta _{6}^{\prime }+\theta _{3})+7}{12}\right) = (6.5, 3.91). \end{eqnarray} | (4.25) |
Also,
\begin{equation} \begin{array}{ccccc} \Re _{B_{(\zeta _{R}^{\tau }(x))}} = \sqrt{\overline{x_{0}^{2}}+\overline{ y_{0}^{2}}} = 6.97, & & & & \Re _{B_{(\alpha (x))}} = \sqrt{\overline{ x_{0}^{\prime ^{2}}}+\overline{y_{0}^{\prime ^{2}}}} = 7.01, \\ \Re _{B_{(\eta _{\mathfrak{R}}^{\nu }(x))}} = \sqrt{\overline{x_{1}}^{2}+ \overline{y_{1}}^{2}} = 6.94, & & & & \Re _{B_{(\beta (x))}} = \sqrt{ \overline{x_{1}^{\prime ^{2}}}\overline{+y_{1}^{\prime ^{2}}}} = 7.58. \end{array} \end{equation} | (4.26) |
Now,
\begin{equation} \Re _{B} = \frac{1}{4}\left( R_{B_{(\zeta _{R}^{\tau }(x))}}+R_{B_{(\alpha (x))}}+R_{B_{(\eta _{\mathfrak{R}}^{\nu }(x))}}+R_{B_{(\beta (x))}}\right) = 7.12. \end{equation} | (4.27) |
As \Re (A) < \Re (B)\Longrightarrow A < B.
The linear Diophantine fuzzy numbers have been identified in this research. In this study, we discovered the circumcenter of centroids of the membership function and non-membership function of a linear Diophantine fuzzy number. We also suggested a distance approach for ranking the linear Diophantine fuzzy number depending on the circumcenter of centroids. The suggested method gives the precise organization of linear Diophantine fuzzy numbers. It may be used to rank the linear Diophantine fuzzy numbers in order to deal with various fuzzy optimization issues. This method can be implemented to rank trapezoidal in addition to triangular fuzzy numbers and their counterparts. The following areas may be covered by our future projects:
(ⅰ) Linear programming problems;
(ⅱ) Differential equations;
(ⅲ) Game theory;
(ⅳ) Transportation problems;
(ⅴ) Differential games.
The authors would like to express their sincere thanks to the anonymous reviewers for their careful reading and constructive comments.
The authors of this paper declare that they have no conflict of interest.
[1] | A. Habibi, Two-dimensional Bayesian estimate of images, Proc. IEEE, 60 (1972) 877–883. https://doi.org/10.1109/PROC.1972.8787 |
[2] |
R. P. Roesser, A discrete state-space model for linear image processing, IEEE Trans. Autom. Contr., 20 (1975), 1–10. https://doi.org/10.1109/TAC.1975.1100844 doi: 10.1109/TAC.1975.1100844
![]() |
[3] |
E. Fornasini, G. Marchesini, State-space realization theory of two-dimensional filters, IEEE Trans. Autom. Control, 21 (1976), 484–492. https://doi.org/10.1109/TAC.1976.1101305 doi: 10.1109/TAC.1976.1101305
![]() |
[4] |
J. W. Woods, C. H. Radewan, Kalman filtering in two dimensions, IEEE Trans. Inf. Theory, 23 (1977), 473–482. https://doi.org/10.1109/TIT.1977.1055750 doi: 10.1109/TIT.1977.1055750
![]() |
[5] |
J. W. Woods, V. K. Ingle, Kalman filtering in two dimensions: Further results, IEEE Trans. Acoust., Speech, Signal Process., 29 (1981), 188–197. https://doi.org/10.1109/TASSP.1981.1163533 doi: 10.1109/TASSP.1981.1163533
![]() |
[6] |
T. Katayama, M. Kosana, Recursive filtering algorithm for a two-dimensional system, IEEE Trans. Autom. Control, 24 (1979), 130–132. https://doi.org/10.1109/TAC.1979.1101956 doi: 10.1109/TAC.1979.1101956
![]() |
[7] |
M. \breve{S}ebek, Polynomial solution of 2D Kalman Bucy filtering problem, IEEE Trans. Autom. Control, 37 (1992), 1530–1533. https://doi.org/10.1109/9.256417 doi: 10.1109/9.256417
![]() |
[8] |
A. Concetti, L. Jetto, Two-dimensional recursive filtering algorithm with edge preserving properties and reduced numerical complexity, IEEE Trans. Circuits Syst. II-Analog Digital Signal Process., 44 (1997), 587–591. https://doi.org/10.1109/82.598429 doi: 10.1109/82.598429
![]() |
[9] | X. Chen, C. Yang, The state estimation of the stochastic 2D FMII models, Acta Autom. Sin., 27 (2001), 131–135. |
[10] |
Y. Zou, M. Sheng, N. Zhong, S. Xu, A generalized Kalman filter for 2D discrete systems, Circuits Syst. Signal Process., 23 (2004), 351–364. https://doi.org/10.1007/s00034-004-0804-x doi: 10.1007/s00034-004-0804-x
![]() |
[11] |
J. Liang, F. Wang, Z. Wang, Minimum-variance recursive filtering for two-dimensional systems with degraded measurements: Boundedness and Monotonicity, IEEE Trans. Autom. Control, 64 (2019), 4153–4166. https://doi.org/10.1109/TAC.2019.2895245 doi: 10.1109/TAC.2019.2895245
![]() |
[12] |
F. Wang, Z. Wang, J. Liang, X. Liu, Robust finite horizon filtering for 2-D systems with randomly varying sensor delays, IEEE Trans. Syst., Man, Cybern., Syst., 50 (2020), 220–232. https://doi.org/10.1109/TSMC.2017.2788503 doi: 10.1109/TSMC.2017.2788503
![]() |
[13] |
F. Wang, Z. Wang, J. Liang, C. Silvestre, Recursive locally minimum-variance filtering for two-dimensional systems: When dynamic quantization effect meets random sensor failure, Automatica, 148 (2023), 110762. https://doi.org/10.1016/j.automatica.2022.110762 doi: 10.1016/j.automatica.2022.110762
![]() |
[14] |
F. Wang, J. Liang, J. Lam, J. Yang, C. Zhao, Robust filtering for 2-D systems with uncertain-variance noises and weighted try-once-discard protocols, IEEE Trans. Syst., Man, Cybern., Syst., 53 (2023), 2914–2924. https://doi.org/10.1109/TSMC.2022.3219919 doi: 10.1109/TSMC.2022.3219919
![]() |
[15] |
F. Wang, Z. Wang, J. Liang, Q. Ge, S. X. Ding, Recursive filtering for two-dimensional systems with amplify-and-forward relays: Handling degraded measurements and dynamic biases, Inform. Fusion, 108 (2024), 102368. https://doi.org/10.1016/j.inffus.2024.102368 doi: 10.1016/j.inffus.2024.102368
![]() |
[16] |
P. Zhang, C. Zhu, B. Yang, Z. Wang, M. Hao, Event-triggered ultimately bounded filtering for two-dimensional discrete-time systems under hybrid cyber attacks, J. Franklin Inst., 361 (2024), 683–711. https://doi.org/10.1016/j.jfranklin.2023.12.019 doi: 10.1016/j.jfranklin.2023.12.019
![]() |
[17] |
S. Kong, Y. Sun, H. Zhang, Optimal Kalman-like filtering for a class of nonlinear stochastic systems, J. Ocean Eng. Sci., 8 (2023), 500–507. https://doi.org/10.1016/j.joes.2022.03.002 doi: 10.1016/j.joes.2022.03.002
![]() |
[18] |
W. D. Koning, Optimal estimation of linear discrete-time systems with stochastic parameters, Automatica, 20 (1984), 113–115. https://doi.org/10.1016/0005-1098(84)90071-2 doi: 10.1016/0005-1098(84)90071-2
![]() |
[19] |
J. Hu, Z. Wang, H. Gao, Recursive filtering with random parameter matrices, multiple fading measurements and correlated noise, Automatica, 49 (2013), 3440–3448. https://doi.org/10.1016/j.automatica.2013.08.021 doi: 10.1016/j.automatica.2013.08.021
![]() |
[20] |
W. Wang, J. Zhou, Optimal linear filtering design for discrete-time systems with cross-correlated stochastic parameter matrices and noises, IET Control Theory Appl., 11 (2017), 3353–3362. https://doi.org/10.1049/iet-cta.2017.0425 doi: 10.1049/iet-cta.2017.0425
![]() |
1. | Muhammad Abdurrahman Rois, Cicik Alfiniyah, Chidozie W. Chukwu, Dynamic analysis and optimal control of COVID-19 with comorbidity: A modeling study of Indonesia, 2023, 8, 2297-4687, 10.3389/fams.2022.1096141 | |
2. | Le Cui, Diyi Chen, Chun Li, Xiaodong Tan, Xunfeng Yuan, A General Study on 3D Fractional Order Hexagon × n RLɑ Cβ Circuit Network, 2022, 10, 2169-3536, 55889, 10.1109/ACCESS.2022.3168720 | |
3. | Xiao-Ping Li, Mahmoud H. DarAssi, Muhammad Altaf Khan, C.W. Chukwu, Mohammad Y. Alshahrani, Mesfer Al Shahrani, Muhammad Bilal Riaz, Assessing the potential impact of COVID-19 Omicron variant: Insight through a fractional piecewise model, 2022, 38, 22113797, 105652, 10.1016/j.rinp.2022.105652 | |
4. | Hengki Tasman, Dipo Aldila, Putri A. Dumbela, Meksianis Z. Ndii, Faishal F. Herdicho, Chidozie W. Chukwu, Assessing the Impact of Relapse, Reinfection and Recrudescence on Malaria Eradication Policy: A Bifurcation and Optimal Control Analysis, 2022, 7, 2414-6366, 263, 10.3390/tropicalmed7100263 | |
5. | Bevina D. Handari, Rossi A. Ramadhani, Chidozie W. Chukwu, Sarbaz H. A. Khoshnaw, Dipo Aldila, An Optimal Control Model to Understand the Potential Impact of the New Vaccine and Transmission-Blocking Drugs for Malaria: A Case Study in Papua and West Papua, Indonesia, 2022, 10, 2076-393X, 1174, 10.3390/vaccines10081174 | |
6. | Endang Yuliani, Cicik Alfiniyah, Maureen L. Juga, Chidozie W. Chukwu, On the Modeling of COVID-19 Transmission Dynamics with Two Strains: Insight through Caputo Fractional Derivative, 2022, 6, 2504-3110, 346, 10.3390/fractalfract6070346 | |
7. | E. Bonyah, M. L. Juga, L. M. Matsebula, C. W. Chukwu, On the Modeling of COVID-19 Spread via Fractional Derivative: A Stochastic Approach, 2023, 15, 2070-0482, 338, 10.1134/S2070048223020023 | |
8. | Muhammad Abdurrahman Rois, Cicik Alfiniyah, Santi Martini, Dipo Aldila, Farai Nyabadza, Modeling and optimal control of COVID-19 with comorbidity and three-dose vaccination in Indonesia, 2024, 6, 25889338, 181, 10.1016/j.jobb.2024.06.004 | |
9. | Olumuyiwa James Peter, Nadhira Dwi Fahrani, C.W. Chukwu, A fractional derivative modeling study for measles infection with double dose vaccination, 2023, 4, 27724425, 100231, 10.1016/j.health.2023.100231 | |
10. | C.W. Chukwu, R.T. Alqahtani, C. Alfiniyah, F.F. Herdicho, , A Pontryagin’s maximum principle and optimal control model with cost-effectiveness analysis of the COVID-19 epidemic, 2023, 8, 27726622, 100273, 10.1016/j.dajour.2023.100273 | |
11. | Dipo Aldila, Nadya Awdinda, Faishal F. Herdicho, Meksianis Z. Ndii, Chidozie W. Chukwu, Optimal control of pneumonia transmission model with seasonal factor: Learning from Jakarta incidence data, 2023, 9, 24058440, e18096, 10.1016/j.heliyon.2023.e18096 | |
12. | G. M. Vijayalakshmi, P. Roselyn Besi, Ali Akgül, Fractional commensurate model on COVID‐19 with microbial co‐infection: An optimal control analysis, 2024, 45, 0143-2087, 1108, 10.1002/oca.3093 | |
13. | Gaohui Fan, Ning Li, Application and analysis of a model with environmental transmission in a periodic environment, 2023, 31, 2688-1594, 5815, 10.3934/era.2023296 | |
14. | G. M. Vijayalakshmi, P. Roselyn Besi, A. Kalaivani, G. Infant Sujitha, S. Mahesh, Microbial coinfections in COVID-19: mathematical analysis using Atangana–Baleanu–Caputo type, 2024, 7, 2520-8160, 4097, 10.1007/s41939-024-00418-2 | |
15. | C.W. Chukwu, E. Bonyah, M.L. Juga, , On mathematical modeling of fractional-order stochastic for tuberculosis transmission dynamics, 2023, 11, 26667207, 100238, 10.1016/j.rico.2023.100238 | |
16. | C.W. Chukwu, M.I. Utoyo, A. Setiawan, J.O. Akanni, Fractional model of HIV transmission on workplace productivity using real data from Indonesia, 2024, 225, 03784754, 1089, 10.1016/j.matcom.2023.11.014 | |
17. | CW Chukwu, S. Y. Tchoumi, Z. Chazuka, M. L. Juga, G. Obaido, Assessing the impact of human behavior towards preventative measures on COVID-19 dynamics for Gauteng, South Africa: a simulation and forecasting approach, 2024, 9, 2473-6988, 10511, 10.3934/math.2024514 | |
18. | Puntipa Pongsumpun, Puntani Pongsumpun, I-Ming Tang, Jiraporn Lamwong, The role of a vaccine booster for a fractional order model of the dynamic of COVID-19: a case study in Thailand, 2025, 15, 2045-2322, 10.1038/s41598-024-80390-6 |