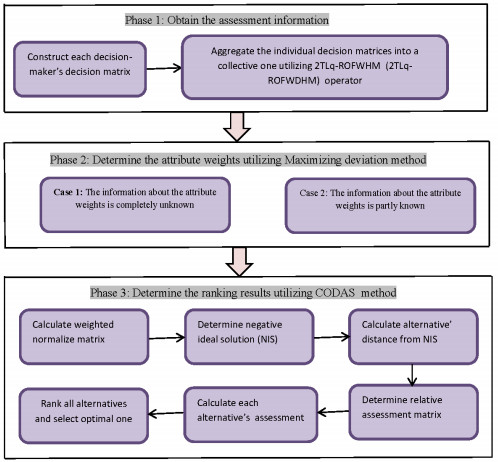
This paper investigates the practical generalized finite-time synchronization (PGFETS) of duplex networks with quantized and delayed couplings. Given that continuous transmission of signals will increase the load and cost of communication, we introduce quantized couplings in the model. Then, via the theorem of finite-time stability, the PGFETS is proposed based on the fact that PGFETS is much more extensive and practical than classical finite-time synchronization. Some sufficient criteria are formulated to achieve the goal of synchronization by utilizing quantized intermittent control schemes. Lastly, the validity of the theoretical results is illustrated by numerical simulations.
Citation: Ting Yang, Li Cao, Wanli Zhang. Practical generalized finite-time synchronization of duplex networks with quantized and delayed couplings via intermittent control[J]. AIMS Mathematics, 2024, 9(8): 20350-20366. doi: 10.3934/math.2024990
[1] | Ayesha Khan, Uzma Ahmad, Adeel Farooq, Mohammed M. Ali Al-Shamiri . Combinative distance-based assessment method for decision-making with 2-tuple linguistic q-rung picture fuzzy sets. AIMS Mathematics, 2023, 8(6): 13830-13874. doi: 10.3934/math.2023708 |
[2] | Muhammad Akram, Sumera Naz, Feng Feng, Ghada Ali, Aqsa Shafiq . Extended MABAC method based on 2-tuple linguistic T-spherical fuzzy sets and Heronian mean operators: An application to alternative fuel selection. AIMS Mathematics, 2023, 8(5): 10619-10653. doi: 10.3934/math.2023539 |
[3] | Zeeshan Ali, Tahir Mahmood, Muhammad Bilal Khan . Three-way decisions with complex q-rung orthopair 2-tuple linguistic decision-theoretic rough sets based on generalized Maclaurin symmetric mean operators. AIMS Mathematics, 2023, 8(8): 17943-17980. doi: 10.3934/math.2023913 |
[4] | Dilshad Alghazzwi, Arshad Ali, Ahmad Almutlg, E. A. Abo-Tabl, A. A. Azzam . A novel structure of q-rung orthopair fuzzy sets in ring theory. AIMS Mathematics, 2023, 8(4): 8365-8385. doi: 10.3934/math.2023422 |
[5] | Muhammad Akram, Sumera Naz, Gustavo Santos-García, Muhammad Ramzan Saeed . Extended CODAS method for MAGDM with 2-tuple linguistic T-spherical fuzzy sets. AIMS Mathematics, 2023, 8(2): 3428-3468. doi: 10.3934/math.2023176 |
[6] | Ghous Ali, Kholood Alsager, Asad Ali . Novel linguistic q-rung orthopair fuzzy Aczel-Alsina aggregation operators for group decision-making with applications. AIMS Mathematics, 2024, 9(11): 32328-32365. doi: 10.3934/math.20241551 |
[7] | Sumbal Ali, Asad Ali, Ahmad Bin Azim, Ahmad ALoqaily, Nabil Mlaiki . Averaging aggregation operators under the environment of q-rung orthopair picture fuzzy soft sets and their applications in MADM problems. AIMS Mathematics, 2023, 8(4): 9027-9053. doi: 10.3934/math.2023452 |
[8] | Attaullah, Shahzaib Ashraf, Noor Rehman, Asghar Khan, Muhammad Naeem, Choonkil Park . Improved VIKOR methodology based on q-rung orthopair hesitant fuzzy rough aggregation information: application in multi expert decision making. AIMS Mathematics, 2022, 7(5): 9524-9548. doi: 10.3934/math.2022530 |
[9] | Adel Fahad Alrasheedi, Jungeun Kim, Rukhsana Kausar . q-Rung orthopair fuzzy information aggregation and their application towards material selection. AIMS Mathematics, 2023, 8(8): 18780-18808. doi: 10.3934/math.2023956 |
[10] | Muhammad Akram, Naila Ramzan, Anam Luqman, Gustavo Santos-García . An integrated MULTIMOORA method with 2-tuple linguistic Fermatean fuzzy sets: Urban quality of life selection application. AIMS Mathematics, 2023, 8(2): 2798-2828. doi: 10.3934/math.2023147 |
This paper investigates the practical generalized finite-time synchronization (PGFETS) of duplex networks with quantized and delayed couplings. Given that continuous transmission of signals will increase the load and cost of communication, we introduce quantized couplings in the model. Then, via the theorem of finite-time stability, the PGFETS is proposed based on the fact that PGFETS is much more extensive and practical than classical finite-time synchronization. Some sufficient criteria are formulated to achieve the goal of synchronization by utilizing quantized intermittent control schemes. Lastly, the validity of the theoretical results is illustrated by numerical simulations.
Industrial robots are machines used for manufacturing. In material handling, spot welding, material removal, arc welding, inspection and testing, handling, assembly, finishing and painting, robots are used to perform repetitive, difficult and dangerous with greater precision, accuracy and precision task speed. The main reasons for industrial use of industrial robots are to reduce operating costs and increase manufacturing efficiency. An industrial robot has several parameters, including mechanical weight, payload capacity, repeatability, etc.[1]. These parameters make it a MAGDM problem. Welding is the most sought-after skill in any industrial business. Since the invention of industrial robots, there has been a high demand for industrial robots for welding applications. Arc welding, metal arc welding, carbon arc welding, metal inert gas welding, plasma arc welding, tungsten inert gas welding, electro-slag welding and submerged arc welding [2] are some of several types of arc welding. Arc welding robots are programmed to perform all forms of arc welding tasks. In arc welding [3], electricity is used to form an arc between an electrode and a conductive base metal. Arc welding is widely used in most manufacturing companies. However, as technology advances and product demand increases, manufacturing companies are turning to robot-assisted manufacturing [4]. To provide manufacturers a common configuration to let them choose between a variety of arc welding robots. The objective of this study is to investigate using MAGDM approaches to prioritize industrial arc welding robots. To choose the best robot for multiple objectives, different MAGDM techniques such as VIKOR, ELECTRE, and compromise ranking techniques were used. For robot selection, researchers used a decision model based on fuzzy linear regression. Four criteria were used to evaluate twenty-seven industrial robots [5]. The Analytic Hierarchy Process (AHP) and TOPSIS MADM techniques were used to compare and assess seven industrial robot choices based on two criterion and six sub-criteria [6]. Using a set of objective data, a PROMETHEE II approach was used to select the robot. Fourteen and seven distinct industrial robots were compared based on four and five criteria, respectively, in two numerical illustrations [7]. For solving robot selection problems with incomplete weight information, an integrated model based on hesitant 2-tuple linguistic term sets and an expanded QUALIFLEX technique was developed [8]. For robot selection, the VIKOR method was introduced, which used a type-2 fuzzy sets methodology to evaluate eight industrial robot alternatives using seven criteria [9]. The application of the COPRAS method's multi-criteria approach to solve an industrial robot selection problem was demonstrated. Seven different industrial robot models were chosen and compared based on five alternatives [10]. The WASPAS approach was proposed as an MADM tool for picking the best robot among seven different real-time industrial robot models that were assessed using five criteria [11]. To evaluate mobile robot selection for a hospital pharmacy, a fuzzy extended VIKOR method was created by combining fuzzy AHP and VIKOR-based techniques. On the basis of seven parameters, three different mobile robots were compared [12].
MAGDM is a fascinating research topic that has attracted widespread attention from scholars and scientists all over the world [13,14,15,16,17,18,19,20,21,22,23,24]. Decision-makers (DMs) use some tools in the MAGDM framework to effectively and appropriately articulate their evaluation values. Following that, several approaches or strategies are used to identify the ranking order of viable choices and make the ultimate selection. The q-ROFS, developed by Yager [25], is an effective tool for representing DM assessment data. As an extension of the intuitionistic fuzzy set [26] and Pythagorean fuzzy set [27], q-ROFS successfully models DMs' reluctance when presenting their assessment information, as it allows the membership degree (MD) and non-membership degree (NMD) sets of some values in the interval [0, 1]. The qth power of the MD and the qth power of the NMD must be ≤ 1 to satisfy the q-ROFS constraint. The preceding data can be represented as Q=(0.6,0.9), which is a q-rung orthopair fuzzy number (since (0.6)q+(0.9)q≤1). As a result, q-ROFS has been widely used in MAGDM, and several new decision-making methods have been proposed. An MAGDM technique based on the q-ROF geometric-arithmetic weighted averaging operator was proposed by Liu and Wang [28]. Liu and Liu [29] extended the traditional Bonferroni mean operator to the q-ROF set and developed an MAGDM technique based on the q-ROF Bonferroni mean operator, recognizing the correlation between many attributes may affect the decision results. The MAGDM technique proposed by Wei et al. [30] is subject to the q-ROF Maclaurin symmetric mean (MSM) operator. In light of the fact that the association between q-ROF numbers may be heterogeneous, Liu et al.[31] suggested an MAGDM approach based on the q-ROF distributed Heronian mean operator. Yang et al. [32] developed a deep learning and q-ROF interactive weighted Heronian averaging operator-based online shopping assistance model. The q-ROF power MSM operators were provided by Liu et al. [33] to develop a new MAGDM technique from the expert group's viewpoint. The MAGDM technique was developed by Hussain et al.[34] using the group-based generalized q-ROF average aggregation operations. To solve the MAGDM difficulties, He et al. [35] proposed the q-ROF power Bonferroni mean operator. The complex q-ROF MSM operators were further established by Ali and Mahmood [36]. The works cited above demonstrate the effectiveness of q-ROFSs in dealing with the difficult assessment values of DMs in the MAGDM technique.
Since Zadeh [37] proposed linguistic variation (LV) theory, in particular to solve the ensemble of linguistic MAGDM challenges, many advances have been made in the study of linguistic MAGDM challenges. Fuzzy linguistic techniques have been proven effective in various fields and applications. Several researchers have studied the problem of group decision-making, where both attributes and decision expert weights are represented as linguistic words in the recent literature. They suggested an MAGDM-based method that focuses on actual language knowledge, defined linguistic assessment operational principles, established a few new operators, and defined linguistic assessment operational principles. Originally, Herrera and Martinez[38] proposed the 2TL representation approach. It is comprised of a linguistic term and a number and represents linguistic information with a pair of values known as a 2-tuple. In linguistic information processing, the 2TL model has precise characteristics. It prevented information loss and distortion, which previously occurred during linguistic information processing. This strategy has been increasingly popular in recent years for group DM[39,40]. They also proposed the 2TL computational model and 2TL aggregation operators, as well as DM techniques. Wang[41] provided a model for determining which agile manufacturing system is best for you. Deng et al.[42] investigated novel complex T-SF 2TL Muirhead mean aggregation operators. Wei and Gao [43] developed several Pythagorean fuzzy 2TL power AOs using the power average and power geometric operations with Pythagorean fuzzy 2TL information to tackle the MAGDM challenges. To tackle the MAGDM problem using 2TLq-ROF information, Ju et al.[44] developed the 2TLq-ROF weighted AO and the 2TLq-ROF weighted geometric operator. They also propose the 2TLq-ROF Muirhead mean operator and the 2TLq-ROF dual Muirhead mean operator.
Many wide assortments of studies have been undertaken to learn more about the correlation between arguments, which is a crucial feature of aggregated data. The Hamy mean (HM) operator is one of the more comprehensive, adaptable, and dominant concepts used to operate troublesome and contradictory information in real-life challenges, and certain scholars have implemented it in the environment of numerous domains to find the relation between any number of attributes. Liang [45] also initiated the HM operators for IFSs, Li et al. [46] proposed the Dombi HM operators for IFSs, Wu et al. [47] initiated the Dombi HM operators for interval-valued IFSs, and developed the Dombi HM operators for interval-valued IFSs, Li et al. [48] investigated the HM operators for PFS, and Wang et al. [49] investigated the HM operators under the q-ROFSs. Ghorabaee et al. [50] established the CODAS technique, which is an efficient and up-to-date decision-making methodology. It is a distance-based method that employs Euclidean distance (ED) and Hamming distance (HM) measures. As a primary comparison measure, this method employs the ED. Whenever the EDs between two alternatives are relatively close, HDs are employed to compare them. A threshold parameter determines the degree of closeness of EDs. On the basis of the AHP and CODAS methods, Panchal et al.[51] developed an integrated MAGDM architecture. Badi et al. [52] used the CODAS technique to determine the ideal location for a desalination facility on Libya's northwest coast. Ghorabaee et al.[53] applied the CODAS approach to picking the most attractive providers in a fuzzy environment. Pamucar et al. [54] proposed a novel CODAS approach based on linguistic neutrosophic numbers. However, no one has utilized the HM operators' idea in the domain of 2TL-q-ROFS in terms of CODAS approach yet.
In this study, we use 2TLq-ROFS as it provides a stronger definition of fuzziness and thus more accurate evaluation of the decision making process by permitting DMs to assess a wider range due to the uncertainties in the addressed problems and the lack of information and inconsistencies among expert groups. So, we developed the 2TLq-ROFS as a new evolution in FS theory for communicating data complexities. The 2TLq-ROFS involves the integration of 2TL and q-ROF sets and expands the q-ROFS adaptability. When making a collective choice, the DMs may only have a hazy idea of how much they like one alternative over another and are unable to measure their preferences with exact numerical numbers. Rather than numerical variables, it is more appropriate to communicate their preferences through linguistic variables. We devised a technique called the maximizing deviation approach to discover the ideal relative weights of qualities under linguistic context, based on the premise that the attribute with a greater deviation value among alternatives should be considered with a greater weight. The development has the notable feature of being able to reduce the influence of DMs' subjectivity and make adequate use of decision information. Then, using the HM operator, we suggested a generic strategy for grouping multi-attribute DM issues with linguistic information, in which preference values are expressed as linguistic variables. Furthermore, we use CODAS method which is a powerful technique to solve a group DM challenge and selecting the best alternative for selection of the best arc welding robot. It has several advantages that aren't considered by other MAGDM approaches[55]. These are the main contributions of this study:
(i) We introduce 2TLq-ROFS as a new advance in FS theory to communicate the complexity of the data. 2TLq-ROFS combines the advantages of 2TL and q-ROF sets, increasing the versatility of q-ROFS.
(ii) We introduce a family of HM aggregation operators for 2TLq-ROFS, such as 2TLq-ROFHM operator, 2TLq-ROFDHM operator, 2TLq-ROFWHM operator and 2TLq-ROFWDHM. The 2TLq-ROFWDHM operator is used to deal with group decision-making problems with interrelated attributes.
(iii) Some theorems, properties, and formal definitions of the proposed information aggregation operators are inferred from existing situations.
(iv) Based on the 2TLq-ROFWHM and 2TLq-ROFWDHM operators, a 2TLq-ROF-CODAS method is proposed to rank the alternatives. A novel MAGDM model is used to fuse the evaluation preferences of DMs.
(v) A decision-making system based on 2TLq-ROF-CODAS method for evaluating and selecting arc welding robots is designed.
The following is the structure of the paper: Section 2 covers various key ideas, including the 2TL representation model, the description of q-ROFS, the HM operator, and the dual HM operator. Section 3 introduces the concept of 2TLq-ROFSs and how it works. The 2TLq-ROFHM, 2TLq-ROFDHM, 2TLq-ROFWHM and 2TLq-ROFWDHM aggregation operators with optimal properties are developed in section 4. In the section 5, the MAGDM policy is constructed by using the 2TLq-ROFWHM and 2TLq-ROFWDHM operators in the 2TLq-ROFS environment. The section 6 provides numerical examples, parameter effects, comparative analysis, and benefits to illustrate the usefulness and superiority of the established method. Finally, Section 7 summarizes the research and suggests future directions.
Definition 2.1. [56] Let there exists a linguistic term set (LTS) S={st|t=0,1,…,τ} with odd cardinality, where st indicates a possible linguistic term for a linguistic variable. If st,sȷ∈S, then the LTS meets the following characteristics:
(i) The set is ordered: st>sȷ, if and only if t>ȷ.
(ii) Max operator: max(st,sȷ)=st, if and only if t≥ȷ.
(iii) Min operator: min(st,sȷ)=st, if and only if t≤ȷ.
(iv) Negative operator: Neg(st)=sȷ such that ȷ=τ−t.
The 2TL representation model based on the idea of symbolic translation, introduced by Herrera and Martinez [57], is useful for representing the linguistic assessment information by means of a 2-tuple (st,υt), where st is a linguistic label from predefined LTS S and υt is the value of symbolic translation, and υt∈[−0.5,0.5).
Definition 2.2. [57] Let ϱ be the result of an aggregation of the indices of a set of labels assessed in a LTS S, i.e., the result of a symbolic aggregation operation, ϱ∈[0,τ], where τ is the cardinality of S. Let t=round(ϱ) and υ=ϱ−t be two values, such that, t∈[0,τ] and υ∈[−0.5,0.5) then υ is called a symbolic translation.
Definition 2.3. [57] Let S={st|t=1,…,τ} be a LTS and ϱ∈[0,τ] is a number value representing the aggregation result of linguistic symbolic. Then the function Δ used to obtain the 2TL information equivalent to ϱ is defined as:
Δ:[0,τ]→S×[−0.5,0.5), |
Δ(ϱ)={st,t=round(ϱ)υ=ϱ−t,υ∈[−0.5,0.5). | (2.1) |
Definition 2.4. [57] Let S={st|t=1,…,τ} be a LTS and (st,υt) be a 2-tuple, there exists a function Δ−1 that restore the 2-tuple to its equivalent numerical value ϱ∈[0,τ]⊂R, where
Δ−1:S×[−0.5,0.5)→[0,τ],Δ−1(st,υ)=t+υ=ϱ. | (2.2) |
Yager [25] defined the q-rung orthopair fuzzy set as an extension of intuitionistic fuzzy set and Pythagorean fuzzy set as follows.
Definition 2.5. [25] For any universal set X, a q-ROFS is of the form
T={⟨x,p(x),l(x)⟩|x∈X}, |
where p,l:X→[0,1] represent the MD and NMD, respectively, with the condition 0≤pq(ℓ)+lq(ℓ)≤1 for positive number q≥1 and r(ℓ)=q√1−(pq(ℓ)+lq(ℓ)) is known as the degree of refusal of ℓ in T. To express information conveniently, the pair (p,l) is known as a q-rung orthopair fuzzy number (q-ROFN).
A q-ROFN is a generalized form of existing fuzzy framework and it reduces to:
(i) Pythagorean fuzzy number (PFN); by taking q as 2.
(ii) Intuitionistic fuzzy number (IFN); by taking q as 1.
(iii) Fuzzy number (FN); by taking l as zero and q as 1.
Definition 2.6. Let aȷ(ȷ=1,2,…,n) be a set of non-negative real numbers. Some HM aggregation operators are defined as follows:
(1) Hamy mean [58]: HM(κ)(a1,a2,…,an)=∑1≤t1<…<tκ≤n(κ∏ȷ=1atȷ)1κCκn;
(2) Weighted Hamy mean [58]: WHM(κ)ϖ(a1,a2,…,an)=∑1≤t1<…<tκ≤n(κ∏ȷ=1(atȷ)ϖtȷ)1κCκn;
(3) Dual Hamy mean [59]: DHM(κ)(a1,a2,…,an)=(∏1≤t1<…<tκ≤n(κ∑ȷ=1atȷκ))1Cκn;
(4) Weighted dual Hamy mean [59]: WDHM(κ)ϖ(a1,a2,…,an)=(∏1≤t1<…<tκ≤n(κ∑ȷ=1ϖtȷatȷκ))1Cκn,
where κ is a parameter and κ=1,2,…,n,t1,t2,…,tκ are κ integer values taken from the set {1,2,…,n} of t integer values, Cκn denotes the binomial coefficient, and Cκn=n!/(κ!(n−κ)!).
For other concepts and applications, the readers are refer to [60,61,62,63].
We introduce the 2TLq-ROFS with its operational laws as a new advancement of FS theory, in this section. Inspired by the ideas of 2TL and q-ROF sets, we develop the new concept of 2TLq-ROFS by combining both the advantages of 2TL and q-ROF sets, as an extension of 2TLIFSs and 2TLPFSs. The newly proposed set has flexibility due to the qth power of MD and NMD. The mathematical representation of 2TLq-ROFS is described as follows.
Definition 3.1. Let S={st|t=0,1,…,τ} be a LTS with odd cardinality. If (sp(x),℘(x)),(sr(x),ζ(x)) is defined for sp(x),sr(x)∈S,℘(x),ζ(x)∈[−0.5,0.5), where (sp(x),℘(x)) and (sr(x),ζ(x)) represent the MD and NMD by 2TLSs, respectively. A 2TL q-rung orthopair fuzzy set is defined as:
ℵ={⟨x,((sp(x),℘(x)),(sr(x),ζ(x)))⟩|x∈X}, | (3.1) |
where 0≤Δ−1(sp(x),℘(x))≤τ,0≤Δ−1(sr(x),ζ(x))≤τ, and 0≤(Δ−1(sp(x),℘(x)))q+(Δ−1(sr(x),ζ(x)))q≤τq.
To compare any two 2TLq-ROFNs, their score value and accuracy value are defined as follows.
Definition 3.2. Let η=((sp,℘),(sr,ζ)) be a 2TLq-ROFN. Then the score function S of a 2TLq-ROFN η, can be represented as:
S(η)=Δ(τ2(1+(Δ−1(sp,℘)τ)q−(Δ−1(sr,ζ)τ)q)),S(η)∈[0,τ], | (3.2) |
and its accuracy function H is defined as:
H(η)=Δ(τ((Δ−1(sp,℘)τ)q+(Δ−1(sr,ζ)τ)q)),H(η)∈[0,τ]. | (3.3) |
Definition 3.3. Let η1=((sp1,℘1),(sr1,ζ1)) and η2=((sp2,℘2),(sr2,ζ2)) be two 2TLq-ROFNs, then these two 2TLq-ROFNs can be compared according to the following rules:
(1) If S(η1)>S(η2), then η1>η2;
(2) If S(η1)=S(η2), then
● If H(η1)>H(η2), then η1>η2;
● If H(η1)=H(η2), then η1∼η2.
Definition 3.4. Let η1=((sp1,℘1),(sl1,ζ1)) and η2=((sp2,℘2),(sl2,ζ2)) be two 2TLq-ROFNs. We define the 2TLq-ROF normalized ED and HD as:
ED(η1,η2)=Δ(τ2(|(Δ−1(sp1,℘1)τ)q−(Δ−1(sp2,℘2)τ)q|q+|(Δ−1(sη1,ζ1)τ)q−(Δ−1(sη2,ζ2)τ)q|q)1q). | (3.4) |
HD(η1,η2)=Δ(τ2(|(Δ−1(sp1,℘1)τ)q−(Δ−1(sp2,℘2)τ)q|+|(Δ−1(sr1,ζ1)τ)q−(Δ−1(sr2,ζ2)τ)q|)). | (3.5) |
We now put forward the novel operational laws based on 2TLq-ROFNs, including addition, multiplication, scalar multiplication, power and ranking rules.
Definition 3.5. Let η=((sp,℘),(sr,ζ)), η1=((sp1,℘1),(sr1,ζ1)), and η2=((sp2,℘2),(sr2,ζ2)) be three 2TLq-ROFNs, q≥1, then
(1) η1⊕η2 = (Δ(τq√1−(1−(Δ−1(sp1,℘1)τ)q)(1−(Δ−1(sp2,℘2)τ)q)),Δ(τ(Δ−1(sr1,ζ1)τ)(Δ−1(sr2,ζ2)τ)));
(2) η1⊗η2 = (Δ(τ(Δ−1(sp1,℘1)τ)(Δ−1(sp2,℘2)τ)),Δ(τq√1−(1−(Δ−1(sr1,ζ1)τ)q)(1−(Δ−1(sr2,ζ2)τ)q)));
(3) λη=(Δ(τq√1−(1−(Δ−1(sp,℘)τ)q)λ),Δ(τ(Δ−1(sr,ζ)τ)λ)),λ>0;
(4) ηλ=(Δ(τ(Δ−1(sp,℘)τ)λ),Δ(τq√1−(1−(Δ−1(sr,ζ)τ)q)λ)),λ>0.
Hara et al.[58] proposed the concept of Hamy mean operator. In this Section, the 2TLq-ROFHM, 2TLq-ROFWHM, 2TLq-ROFDHM, and 2TLq-ROFWDHM operators for aggregating the 2TLq-ROFNs are proposed to extend the HM aggregation operators to the 2TLq-ROFS environment. Since 2TLq-ROFS is a useful technique for expressing ambiguous data in a real-world decision-making context. Core features of aggregation operators are idempotency, monotonicity, and boundedness.
This subsection introduces the new concept of the 2TLq-ROFHM operator for aggregating 2TLq-ROFNs and examines its distinctive and preferred features.
Definition 4.1. Let ηȷ=((spȷ,℘ȷ),(srȷ,ζȷ))(ȷ=1,2,…,n) be a collection of 2TLq-ROFNs. The 2TLq-ROFHM operator is a mapping Tn→T such that
2TL q -ROFHM(κ)(η1,η2,…,ηn)=⊕1≤t1<…<tκ≤n(⊗κȷ=1ηtȷ)1κCκn. | (4.1) |
Theorem 4.1. Utilizing the 2TLq-ROFHM operator, the aggregated value is likewise a 2TLq-ROFN value, where
2TL q -ROFHM(κ)(η1,η2,…,ηn)=(Δ(τq√1−∏1≤t1<…<tκ≤n(1−(κ∏ȷ=1Δ−1(spȷ,℘ȷ)τ)qκ)1Cκn),Δ(τ(∏1≤t1<…<tκ≤nq√1−κ∏ȷ=1(1−(Δ−1(srȷ,ζȷ)τ)q)1κ)1Cκn)). | (4.2) |
Proof. By utilizing Definition 3.5, we get
⊗κȷ=1ηtȷ=(Δ(τκ∏ȷ=1Δ−1(spȷ,℘ȷ)τ),Δ(τq√1−κ∏ȷ=1(1−(Δ−1(srȷ,ζȷ)τ)q))). |
Thus,
(⊗κȷ=1ηtȷ)1κ=(Δ(τ(κ∏ȷ=1Δ−1(spȷ,℘ȷ)τ)1κ),Δ(τq√1−κ∏ȷ=1(1−(Δ−1(srȷ,ζȷ)τ)q)1κ)). |
Therefore,
⊕1≤t1<…<tκ≤n(⊗κȷ=1ηtȷ)1κ=(Δ(τq√1−∏1≤t1<…<tκ≤n(1−(κ∏ȷ=1Δ−1(spȷ,℘ȷ)τ)qκ)),Δ(τ∏1≤t1<…<tκ≤nq√1−κ∏ȷ=1(1−(Δ−1(srȷ,ζȷ)τ)q)1κ)). |
Furthermore,
2TL q -ROFHM(κ)(η1,η2,…,ηn)=(Δ(τq√1−∏1≤t1<…<tκ≤n(1−(κ∏ȷ=1Δ−1(spȷ,℘ȷ)τ)qκ)1Cκn),Δ(τ(∏1≤t1<…<tκ≤nq√1−κ∏ȷ=1(1−(Δ−1(srȷ,ζȷ)τ)q)1κ)1Cκn)). |
The desirable properties of the 2TLq-ROFHM operator, such as idempotency, monotonicity, and boundedness, are also described below.
Property 4.1. (Idempotency). If all ηȷ=((spȷ,℘ȷ),(srȷ,ζȷ))(ȷ=1,2,…,n) are equal, for all ȷ, then
2TLq−ROFFHM(κ)(η1,η2,…,ηn)=η. |
Proof.
2TL q -ROFHM(κ)(η1,η2,…,ηn)=(Δ(τq√1−∏1≤t1<…<tκ≤n(1−(κ∏ȷ=1Δ−1(sp,℘)τ)qκ)1Cκn),Δ(τ(∏1≤t1<…<tκ≤nq√1−κ∏ȷ=1(1−(Δ−1(sr,ζ)τ)q)1κ)1Cκn))=(Δ(τq√1−((1−((Δ−1(sp,℘)τ)κ)qκ)Cκn)1Cκn),Δ(τ(q√1−((1−(Δ−1(sr,ζ)τ)q)κ)1κ)Cκn)1Cκn)=((sp,℘),(sr,ζ))=η. |
Property 4.2. (Monotonicity). Let ηȷ=((spȷ,℘ȷ),(srȷ,ζȷ)) and η′ȷ=((s′pȷ,℘′ȷ),(s′rȷ,ζ′ȷ))(ȷ=1,2,…,n) be two sets of 2TLq-ROFNs, if ηȷ≤η′ȷ, for all ȷ, then
2TLq−ROFHM(κ)(η1,η2,…,ηn)≤2TLq−ROFHM(κ)(η′1,η′2,…,η′n). |
Proof. Let ηȷ=((spȷ,℘ȷ),(srȷ,ζȷ)) and η′ȷ=((s′pȷ,℘′ȷ),(s′rȷ,ζ′ȷ))(ȷ=1,2,…,n) be two sets of 2TLq-ROFNs, let
(sp,℘)=Δ(τq√1−∏1≤t1<…<tκ≤n(1−(κ∏ȷ=1Δ−1(spȷ,℘ȷ)τ)qκ)1Cκn),(sr,ζ)=Δ(τ(∏1≤t1<…<tκ≤nq√1−κ∏ȷ=1(1−(Δ−1(srȷ,ζȷ)τ)q)1κ)1Cκn), |
given that (spȷ,℘ȷ)≤(s′pȷ,℘′ȷ); then
(κ∏ȷ=1Δ−1(spȷ,℘ȷ)τ)qκ≤(κ∏ȷ=1Δ−1(s′pȷ,℘′ȷ)τ)qκ. |
Moreover,
∏1≤t1<…<tκ≤n(1−(κ∏ȷ=1Δ−1(spȷ,℘ȷ)τ)qκ)1Cκn≥∏1≤t1<…<tκ≤n(1−(t∏ȷ=1Δ−1(s′pȷ,℘′ȷ)τ)qκ)1Cκn. |
Furthermore,
Δ(τq√1−∏1≤t1<…<tκ≤n(1−(κ∏ȷ=1Δ−1(spȷ,℘ȷ)τ)qκ)1Cκn)≤Δ(τq√1−∏1≤t1<…<tκ≤n(1−(κ∏ȷ=1Δ−1(s′pȷ,℘′ȷ)τ)qκ)1Cκn). |
Therefore, (sp,℘)≤(s′p,℘′). Similarly, we can show that (sr,ζ)≥(s′r,ζ′).
Hence, 2TL q -ROFHM(κ)(η1,η2,…,ηn)≤2TL q -ROFHM(κ)(η′1,η′2,…,η′n).
Property 4.3. (Boundedness). Let ηȷ=((spȷ,℘ȷ),(srȷ,ζȷ))(ȷ=1,2,…,n) be a collection of 2TLq-ROFNs, and let η−=minȷ((spȷ,℘ȷ),(srȷ,ζȷ)) and η+=maxȷ((spȷ,℘ȷ),(srȷ,ζȷ)); then
η−≤2TLq−ROFHM(κ)(η1,η2,…,ηn)≤η+. |
From Property 4.1,
2TLq−ROFHM(κ)(η−1,η−2,…,η−n)=η−,2TLq−ROFHM(κ)(η+1,η+2,…,η+n)=η+. |
From Property 4.2,
η−≤2TLq−ROFHM(κ)(η1,η2,…,ηn)≤η+. |
The 2TLq-ROFHM aggregation operator does not show the weighting values of attributes in Theorem 4.1. To overcome the constraints of the 2TLq-ROFHM operator, we shall introduce the 2TLq-ROFWHM operator with certain preferred features.
Definition 4.2. Let ηȷ=((spȷ,℘ȷ),(srȷ,ζȷ))(ȷ=1,2,…,n) be a collection of 2TLq-ROFNs with weighting vector ϖȷ=(ϖ1,ϖ2,…,ϖn)T, thereby satisfying ϖȷ∈[0,1] and n∑ȷ=1ϖȷ=1. The 2TLq-ROFWHM operator is a mapping Tn→T such that
2TLq−ROFWHM(κ)ϖ(η1,η2,…,ηn)=⊕1≤t1<…<tκ≤n(⊗κȷ=1(ηtȷ)ϖtȷ)1κCκn. | (4.3) |
Theorem 4.2. Using the 2TLq-ROFWHM operator, the aggregated value is likewise a 2TLq-ROFN value, where
2TLq−ROFWHM(κ)ϖ(η1,η2,…,ηn)=(Δ(τq√1−∏1≤t1<…<tκ≤n(1−(κ∏ȷ=1(Δ−1(spȷ,℘ȷ)τ)ϖtȷ)qκ)1Cκn),Δ(τ(∏1≤t1<…<tκ≤nq√1−(κ∏ȷ=1(1−(Δ−1(srȷ,ζȷ)τ)q)ϖtȷ)1κ)1Cκn)). | (4.4) |
Proof. By utilizing Definition 3.5, we get
(ηtȷ)ϖtȷ=(Δ(τ(Δ−1(spȷ,℘ȷ)τ)ϖtȷ),Δ(τq√1−(1−(Δ−1(srȷ,ζȷ)τ)q)ϖtȷ)). |
Then,
⊗κȷ=1(ηtȷ)ϖtȷ=(Δ(τκ∏ȷ=1(Δ−1(spȷ,℘ȷ)τ)ϖtȷ),Δ(τq√1−κ∏ȷ=1(1−(Δ−1(srȷ,ζȷ)τ)q)ϖtȷ)). |
Thus,
(⊗κȷ=1(ηtȷ)ϖtȷ)1κ=(Δ(τ(κ∏ȷ=1(Δ−1(spȷ,℘ȷ)τ)ϖtȷ)1κ),Δ(τq√1−(t∏ȷ=1(1−(Δ−1(srȷ,ζȷ)τ)q)ϖtȷ)1κ)). |
Therefore,
⊕1≤t1<…<tκ≤n(⊗κȷ=1(ηtx)ϖtȷ)1κ=(Δ(τq√1−∏1≤t1<…<tκ≤n(1−(κ∏ȷ=1(Δ−1(spȷ,℘ȷ)τ)ϖtȷ)qκ)),Δ(τ∏1≤t1<…<tκ≤nq√1−(κ∏ȷ=1(1−(Δ−1(srȷ,ζȷ)τ)q)ϖtȷ)1κ)). |
Furthermore,
2TLq−ROFWHM(κ)ϖ(η1,η2,…,ηn)=(Δ(τq√1−∏1≤t1<…<tκ≤n(1−(κ∏ȷ=1(Δ−1(spȷ,℘ȷ)τ)ϖtȷ)qκ)1Cκn),Δ(τ(∏1≤t1<…<tκ≤nq√1−(κ∏ȷ=1(1−(Δ−1(srȷ,ζȷ)τ)q)ϖtȷ)1κ)1Cκn)). |
Property 4.4. (Monotonicity). Let ηȷ=((spȷ,℘ȷ),(srȷ,ζȷ)) and η′ȷ=((s′pȷ,℘′ȷ),(s′rȷ,ζ′ȷ))(ȷ=1,2,…,n) be two sets of 2TLq-ROFNs, if ηȷ≤η′ȷ, for all ȷ, then
2TLq−ROFWHM(κ)ϖ(η1,η2,…,ηn)≤2TLq−ROFWHM(κ)ϖ(η′1,η′2,…,η′n). |
Property 4.5. (Boundedness). Let ηȷ=((spȷ,℘ȷ),(srȷ,ζȷ))(ȷ=1,2,…,n) be a collection of 2TLq-ROFNs, and let η−=minȷ((spȷ,℘ȷ),(srȷ,ζȷ)) and η+=maxȷ((spȷ,℘ȷ),(srȷ,ζȷ)); then
η−≤2TLq−ROFWHM(κ)ϖ(η1,η2,…,ηn)≤η+. |
Idempotency is obviously not a feature of the 2TLq-ROFWHM operator.
In this subsection, we will augment the DHM operator with 2TLq-ROFS to propose the 2TLq-ROFDHM operator for aggregating 2TLq-ROFNs, and also examine its desirable features.
Definition 4.3. Let ηȷ=((spȷ,℘ȷ),(srȷ,ζȷ))(ȷ=1,2,…,n) be a collection of 2TLq-ROFNs. The 2TLq-ROFDHM operator is a mapping Tn→T such that
2TLq−ROFDHM(κ)(η1,η2,…,ηn)=(⊗1≤t1<…<tκ≤n(⊕κȷ=1ηtȷκ))1Cκn. | (4.5) |
Theorem 4.3. The aggregated value by utilizing 2TLq-ROFDHM operator is also a 2TLq-ROFN, where
2TLq−ROFDHM(κ)(η1,η2,…,ηn)=(Δ(τ(∏1≤t1<…<tκ≤nq√1−κ∏ȷ=1(1−(Δ−1(spȷ,℘ȷ)τ)q)1κ)1Cκn),Δ(τq√1−∏1≤t1<…<tκ<n(1−(κ∏ȷ=1Δ−1(srȷ,ζȷ)τ)qκ)1Cκn)). | (4.6) |
Property 4.6. (Idempotency). If all ηȷ=((spȷ,℘ȷ),(srȷ,ζȷ))(ȷ=1,2,…,n) are equal, for all ȷ, then
2TLq−ROFDHM(κ)(η1,η2,…,ηn)=η. |
Property 4.7. (Monotonicity). Let ηȷ=((spȷ,℘ȷ),(srȷ,ζȷ)) and η′ȷ=((s′pȷ,℘′ȷ),(s′rȷ,ζ′ȷ))(ȷ=1,2,…,n) be two sets of 2TLq-ROFNs, if ηȷ≤η′ȷ, for all ȷ, then
2TLq−ROFDHM(κ)(η1,η2,…,ηn)≤2TLq−ROFDHM(κ)(η′1,η′2,…,η′n). |
Property 4.8. (Boundedness). Let ηȷ=((spȷ,℘ȷ),(srȷ,ζȷ))(ȷ=1,2,…,n) be a collection of 2TLq-ROFNs, and let η−=minȷ((spȷ,℘ȷ),(srȷ,ζȷ)) and η+=maxȷ((spȷ,℘ȷ),(srȷ,ζȷ)); then
η−≤2TLq−ROFDHM(κ)(η1,η2,…,ηn)≤η+. |
The value of the aggregated arguments is not taken into account by the 2TLq-ROFDHM operator, as demonstrated in Theorem 4.3. However, in many real-life circumstances, particularly in MAGDM, attribute weights play an important role in the aggregation process. The attributes' values are omitted by the 2TLq-ROFDHM operator. The 2TLq-ROFWDHM operator is proposed to overcome the constraints of 2TLq-ROFDHM.
Definition 4.4. Let ηȷ=((spȷ,℘ȷ),(srȷ,ζȷ))(ȷ=1,2,…,n) be a collection of 2TLq-ROFNs with weighting vector ϖȷ=(ϖ1,ϖ2,…,ϖn)T, thereby satisfying ϖȷ∈[0,1] and n∑ȷ=1ϖȷ=1. The 2TLq-ROFWDHM operator is a mapping Tn→T such that
2TLq−ROFWDHM(κ)ϖ(η1,η2,…,ηn)=(⊗1≤t1<…<tκ≤n(⊕κȷ=1ϖtȷηtȷκ))1Cκn. | (4.7) |
Theorem 4.4. Using the 2TLq-ROFWDHM operator, the aggregated value is likewise a 2TLq-ROFN value, where
2TLq−ROFWDHM(κ)ϖ(η1,η2,…,ηn)=(Δ(τ(∏1≤t1<…<tκ≤nq√1−(κ∏ȷ=1(1−(Δ−1(spȷ,℘ȷ)τ)q)ϖtȷ)1κ)1Cκn),Δ(τq√1−∏1≤t1<…<tκ<n(1−(κ∏ȷ=1(Δ−1(srȷ,ζȷ)τ)ϖtȷ)qκ)1Cκn)). | (4.8) |
Property 4.9. (Monotonicity). Let ηȷ=((spȷ,℘ȷ),(srȷ,ζȷ)) and η′ȷ=((s′pȷ,℘′ȷ),(s′rȷ,ζ′ȷ)),(ȷ=1,2,…,n) be two sets of 2TLq-ROFNs, if ηȷ≤η′ȷ, for all ȷ, then
2TLq−ROFWDHM(κ)ϖ(η1,η2,…,ηn)≤2TLq−ROFWDHM(κ)ϖ(η′1,η′2,…,η′n). |
Property 4.10. (Boundedness). Let ηȷ=((spȷ,℘ȷ),(srȷ,ζȷ))(ȷ=1,2,…,n) be a collection of 2TLq-ROFNs, and let η−=minȷ((spȷ,℘ȷ),(srȷ,ζȷ)) and η+=maxȷ((spȷ,℘ȷ),(srȷ,ζȷ)); then
η−≤2TLq−ROFWDHM(κ)ϖ(η1,η2,…,ηn)≤η+. |
Idempotency is obviously not a feature of the 2TLq-ROFWDHM operator.
This section gives a framework for calculating attribute weights and the ranking orders for all the alternatives with incomplete weight information under 2TLq-ROF environment.
Suppose there are e alternatives R={R1,R2,…,Re}, n attributes G={G1,G2,…,Gn}, and g experts E={Θ1,Θ2,…,Θg}, and let ϖ=(ϖ1,ϖ2,…,ϖn)T and ϖ′=(ϖ′1,ϖ′2,…,ϖ′g)T be the weighting vector of the attributes and weighting vector of the experts satisfying ϖȷ∈[0,1], ϖ′ℓ∈[0,1], ∑nȷ=1ϖȷ=1, and g∑ℓ=1ϖ′ℓ=1, respectively.
Case 1: Completely unknown information on attribute weights
To find the best relative weights for attributes Gȷ∈G, we build an optimization model based on the maximizing deviation method in a 2TLq-ROF environment. The deviation of the alternative Rt from all other alternatives for the attribute can be expressed as:
Dtȷ(ϖ)=e∑k=1d(ηtȷ,ηkȷ)(ϖȷ),t=1,2,…,e,ȷ=1,2,…,n | (5.1) |
where,
d(ηtȷ,hkȷ)=Δ(τ2(|(Δ−1(sptȷ,℘tȷ)τ)q−(Δ−1(spkȷ,℘kȷ)τ)q|q+|(Δ−1(srtȷ,ζtȷ)τ)q−(Δ−1(srkȷ,ζkȷ)τ)q|q))1q | (5.2) |
denotes the 2TLq-ROF ED between the 2TLq-ROFEs htȷ and hkȷ.
Let
Dȷ(ϖ)=e∑t=1Dtȷ(ϖ)=e∑t=1e∑k=1ϖȷd(ηtȷ,hkȷ),ȷ=1,2,…,n. | (5.3) |
Dȷ(ϖ) represents the deviation value of all alternatives to other alternatives for the attribute Gȷ∈G.
(M−1){maxD(ϖ)=n∑ȷ=1e∑t=1e∑k=1ϖȷd(ηtȷ,hkȷ)s.t.ϖȷ≥0,ȷ=1,2,…,n,n∑ȷ=1ϖ2ȷ=1. |
In order to solve the above model, we consider
L(ϖ,ℸ)=n∑ȷ=1e∑t=1e∑k=1ϖȷd(ηtȷ,hkȷ)+ℸ2(n∑ȷ=1ϖ2ȷ−1) | (5.4) |
which represents the Lagrange function of the constrained optimization problem (M-1), where ℸ is a real number, denoting the Lagrange multiplier variable. Then the partial derivatives of L are calculated as:
∂L∂ϖȷ=e∑t=1e∑k=1d(ηtȷ,hkȷ)+ℸϖȷ=0, | (5.5) |
∂L∂ℸ=12(n∑ȷ=1ϖ2ȷ−1)=0. | (5.6) |
It follows from Eq (5.5) that
ϖȷ=−e∑t=1e∑k=1d(ηtȷ,hkȷ)ℸ,ȷ=1,2,…,n. | (5.7) |
Putting Eq (5.7) into Eq (5.6), we get
ℸ=−√n∑ȷ=1(e∑t=1e∑k=1d(ηtȷ,hkȷ))2. | (5.8) |
Obviously, ℸ<0,e∑t=1e∑k=1d(ηtȷ,hkȷ) denotes the sum of all the alternatives' deviations from the jth attribute, and √n∑ȷ=1(e∑t=1e∑k=1d(ηtȷ,hkȷ))2 denotes the sum of all of the alternatives' deviations for all the attributes. Then utilizing Eqs (5.7) and (5.8), we get
ϖȷ=e∑t=1e∑k=1d(ηtȷ,hkȷ)√n∑ȷ=1(e∑t=1e∑k=1d(ηtȷ,hkȷ))2. | (5.9) |
For the sake of simplicity,
χȷ=e∑t=1e∑k=1d(ηtȷ,hkȷ)ȷ=1,2,…,n. | (5.10) |
Then the Eq (5.9) becomes
ϖȷ=χȷ√n∑ȷ=1χ2ȷ,ȷ=1,2,…,n. | (5.11) |
It is simple to verify that ϖȷ(ȷ=1,2,…,n) are positive and fulfill the constrained conditions in the model (M-1) and that the solution is unique using Eq (5.11).
By normalizing ϖȷ(ȷ=1,2,…,n), to let the sum of ϖȷ into a unit, we have
ϖ∗ȷ=ϖȷn∑ȷ=1ϖȷ=χȷn∑ȷ=1χȷ,ȷ=1,2,…,n. | (5.12) |
Case 2: Partly known information on attribute weights
In some cases, the weighting vectors' information is only partially known rather than completely unknown. In these cases, the constrained optimization model can be designed as follows, based on the set of weight's information that is known, Ψ
(M−2){maxD(ϖ)=n∑ȷ=1e∑t=1e∑k=1ϖȷd(ηtȷ,hkȷ)s.t.ϖ∈Ψ,ϖȷ≥0,ȷ=1,2,…,n,n∑ȷ=1ϖȷ=1 |
where Ψ also refers to a collection of restriction constraints that the weight value ϖȷ should satisfy in order to fulfil the requirements in real-world scenarios. A linear programming model (M−2) is used. We acquire the best solution ϖ=(ϖ1,ϖ2,…,ϖn)T, by solving this model, which can be used as the weighting vector for the attributes.
In this subsection, we present a new approch to deal with MAGDM problems, known as 2TLq-ROF-CODAS model based on 2TLq-ROFWHM and 2TLq-ROFWDHM operators by considering the flexibility of 2TLq-ROFNs. The preference of alternatives is calculated using two measures in this method. The largest and the most important measurement is the ED between alternatives and the negative-ideal solution (NIS), and the second measure is the HD. It is clear that the alternative which has greater distance from the NIS is more preferable. The ED and HD measures are used for the relative assessment (RA) of alternatives in order to construct the RA based matrix to fuse the information. The technique of implementing the 2TLq-ROF-CODAS approach is described in the following steps:
Step 1. Switch the linguistic information into 2TLq-ROFNs ηℓtȷ=((spℓtȷ,℘ℓtȷ),(srℓtȷ,ζℓtȷ))(ℓ=1,2,…g).
Step 2. According to 2TLq-ROFNs ηℓtȷ=((spℓtȷ,℘ℓtȷ),(srℓtȷ,ζℓtȷ))(ℓ=1,2,…g) and by utilizing Eqs (4.3) and (4.7), independent panel evaluations can be combined to form the fused 2TLq-ROFNs matrix ηtȷ=((sptȷ,℘tȷ),(srtȷ,ζtȷ)).
ϝ=[ηtȷ]e×n=[η11η12…η1nη21η22…η2n⋮⋮⋮⋮ηe1ηe2…ηen]. | (5.13) |
Step 3. Calculate the weighted 2TLq-ROFNs matrix as follows:
ttȷ=ϖȷ⊗ηtȷ, | (5.14) |
where ϖȷ is the attribute weight of Gȷ, and 0≤ϖȷ≤1, ∑nȷ=1ϖȷ=1.
Step 4. Calculate the NIS by using 2TLq-ROFNs' score function. If the score function is similar, the accuracy function is used to rank the 2TLq-ROFNs:
NIS=[NISȷ]1×n; | (5.15) |
NISȷ=mintS(ttȷ). | (5.16) |
Step 5. Calculate the weighted EDt and HDt as follows:
EDt=n∑ȷ=1ED(ttȷ,NISȷ); | (5.17) |
HDt=n∑ȷ=1HD(ttȷ,NISȷ). | (5.18) |
Step 6. In the following equations, build the relative assessment matrix RA:
RA=[htℓ]e×e; | (5.19) |
htℓ=(EDt−EDℓ)+(g(EDt−EDℓ)×(HDt−HDℓ)), | (5.20) |
where ℓ∈{1,2,3,…,g} and g denotes a significant function that could be designed:
g(θ)={1if |θ|≥ℑ0if|θ|<ℑ, | (5.21) |
where ℑ∈[0.01,0.05] specified by DMs. Here, ℑ=0.02.
Step 7. Derive the average solution (£t) by using:
£t=g∑ℓ=1htℓ. | (5.22) |
Step 8. On the basis of computing outcomes of £t, all the alternatives can be ranked. The best option has the highest evaluation score.
The scheme of the developed approach for MAGDM problems is shown in Figure 1.
Robotic welding is the most visible manifestation of current welding technology. The first generation robotic welding systems used a two-pass weld method, with the first pass committed to learn the seam geometry and the second pass committed to track and weld the seam. The second generation of robotic welding systems came with the technological advancements, which tracked the seam in real-time while learning and seam-tracking at the same time. Third-generation robotic welding systems are the most advanced in robotic welding technology, as they not only function in real-time but also understand the quickly changing geometry of the seam while operating in unorganized situations. Higher product quality criteria should drive it at a lower cost and generate a dependable weld, according to the selection of industrial arc welding robots. Weight density, replicability, freight capacity, maximum reach, Average power consumption, and Motion of a robot are some of the characteristics that can be used to characterize robots. All of these aspects must be taken into account when choosing robots for a certain application. The most prevalent type of robot in industrial robotic arc welding is one with a revolute (or jointed arm) arrangement, which is based on the workspace geometry. The CODAS approach is used to investigate the selection of industrial robots for arc welding operations in this study. The data for arc welding robots was gathered to apply nine distinct robots with six controllable axes and varied controllers from their manufacturers. Six attributes are assigned to these nine robots. After looking over several datasheets offered by robot manufacturers to describe their goods, the selection criteria were evaluated. The opinions of industry professionals are also taken into account. The selection criteria were decided after a discussion between the research group and an industry specialist. The final decision matrix was reviewed using the joint decision of both groups, and the significant traits possessed by each robot were used as criteria for evaluation.The following are the six important attributes shown in Table 1 to consider while choosing an arc welding robot:
Criterion | Explanation |
Weight density | This criterion takes into account the physical weight of the robot. In general, consumer chooses a lighter robot. The weight density is usually expressed in kg (G1). |
Replicability | This refers to a robot's ability to repeat a task over and over again. More replicability is often preferred. Replicability is usually measured in millimeters (G2). |
Freight capacity | The highest total weight a robot can lift in one turn is referred to as freight capacity. Being more is often preferred. The weight density is usually expressed in kg (G3). |
Maximum reach | This is the average of the maximum vertical and horizontal distances a robot's arm can extend to complete a task. It is common to want to be more. The robot's maximum reach is typically measured in millimeters (G4). |
Average power consumption | It refers to the robot's average power consumption in units of electricity. It is often desirable that a robot consumes less energy. The robot's power consumption is usually measured in kilowatts (G5). |
Motion of a robot | The motion of a robot at a reference point near the end effector's tip is referred to as robot motion. Trajectory, speed, acceleration, and acceleration derivative are commonly used to describe robot motion. The robot's motion is usually expressed in ms−1 or ms−2(G6) |
Comprehensive above, the set of nine alternatives R={R1,R2,…R9} is evaluated by four experts E={Θ1,Θ2,Θ3,Θ4} which consists of experienced engineers and customers in evaluation stage having weights ϖ′=(0.19,0.31,0.17,0.33)T. The four experts use the six attributes shown in Table 2 to select the best alternatives for additive manufacturing of linear delta robot.
Sr. No. | Criteria | Units | Symbol |
1 | Weight density | Kg | G1 |
2 | Replicability | (+/−)mm | G2 |
3 | Freight capacity | Kg | G3 |
4 | Maximum Reach | mm | G4 |
5 | Average Power Consumption | KW | G5 |
6 | Motion of a robot | ms−2 | G6 |
The linguistic variables of 2TLq-ROFNs are recorded in Table 3.
Linguistic variables | 2TLq-ROFNs |
Certainly high value (CHV) | ((s8,0),(s0,0)) |
Very high value (VHV) | ((s7,0),(s1,0)) |
High value(HV) | ((s6,0),(s2,0)) |
Above average value (AAV) | ((s5,0),(s3,0)) |
Average vlaue (AV) | ((s4,0),(s4,0)) |
Under average value (UAV) | ((s3,0),(s5,0)) |
Low value (LV) | ((s2,0),(s6,0)) |
Very low value (VLV) | ((s1,0),(s7,0)) |
Certainly low value (CLV) | ((s0,0),(s8,0)) |
Establish the 2TLq-ROF evaluation matrix ϝℓ=[ηℓtȷ]9×6(ℓ=1,2,3,4,5,6) in Table 4 based on linguistic variables listed in Table 3, which are the assessments of four DMs.
Experts | Alternatives | Attributes | |||||
G1 | G2 | G3 | G4 | G5 | G6 | ||
Θ1 | |||||||
R1 | AV | LV | VHV | VLV | CLV | CHV | |
R2 | HV | UAV | CLV | AAV | VHV | LV | |
R3 | VHV | VLV | CHV | HV | UAV | CLV | |
R4 | CLV | AAV | LV | VHV | VLV | AAV | |
R5 | CHV | HV | UAV | CLV | LV | AV | |
R6 | LV | VHV | VLV | CHV | AV | HV | |
R7 | UAV | CLV | AAV | AV | HV | VLV | |
R8 | AAV | AV | HV | UAV | CHV | VHV | |
R9 | VLV | CHV | AV | LV | AAV | UAV | |
Θ2 | |||||||
R1 | VLV | AV | HV | UAV | LV | CHV | |
R2 | LV | VHV | VLV | CHV | CLV | AAV | |
R3 | AV | HV | UAV | CLV | AAV | VHV | |
R4 | CLV | AAV | LV | VHV | VLV | UAV | |
R5 | VHV | VLV | CHV | HV | UAV | AV | |
R6 | HV | UAV | CLV | AAV | AV | LV | |
R7 | AAV | LV | VHV | AV | CHV | CLV | |
R8 | UAV | CHV | AV | LV | VHV | VLV | |
R9 | CHV | CLV | AAV | VLV | HV | AV | |
Θ3 | |||||||
R1 | AV | UAV | LV | AAV | HV | VLV | |
R2 | LV | VHV | AV | HV | UAV | AAV | |
R3 | VHV | VLV | CHV | AV | LV | UAV | |
R4 | CHV | AV | UAV | VLV | AAV | HV | |
R5 | HV | CHV | VLV | UAV | CLV | VHV | |
R6 | AAV | LV | CLV | CHV | VLV | AV | |
R7 | CLV | AAV | HV | VHV | AV | CHV | |
R8 | VLV | HV | VHV | CLV | CHV | LV | |
R9 | UAV | CLV | AAV | LV | VHV | CLV | |
Θ4 | |||||||
R1 | CHV | LV | UAV | AV | AAV | HV | |
R2 | LV | HV | CLV | UAV | CHV | AAV | |
R3 | UAV | VHV | CHV | HV | VLV | CHV | |
R4 | AV | AAV | VLV | CLV | HV | LV | |
R5 | VLV | CLV | AV | LV | VHV | UAV | |
R6 | AAV | CHV | LV | VLV | CLV | AV | |
R7 | VHV | VLV | AAV | CHV | AV | VHV | |
R8 | HV | AV | VHV | AAV | UAV | VLV | |
R9 | CLV | UAV | HV | VHV | LV | CLV |
Transformation of the linguistic decision matrix given in Table 4 into 2TLq-ROF decision matrix shown in Table 5.
Experts | Alternatives | Attributes | |||||
G1 | G2 | G3 | G4 | G5 | G6 | ||
Θ1 | |||||||
R1 | ((s4,0),(s4,0)) | ((s2,0),(s6,0)) | ((s7,0),(s1,0)) | ((s1,0),(s7,0)) | ((s0,0),(s8,0)) | ((s8,0),(s0,0)) | |
R2 | ((s6,0),(s2,0)) | ((s3,0),(s5,0)) | ((s0,0),(s8,0)) | ((s5,0),(s3,0)) | ((s7,0),(s1,0)) | ((s2,0),(s6,0)) | |
R3 | ((s7,0),(s1,0)) | ((s1,0),(s7,0)) | ((s8,0),(s0,0)) | ((s6,0),(s2,0)) | ((s3,0),(s5,0)) | ((s0,0),(s8,0)) | |
R4 | ((s0,0),(s8,0)) | ((s5,0),(s3,0)) | ((s2,0),(s6,0)) | ((s7,0),(s1,0)) | ((s1,0),(s7,0)) | ((s5,0),(s3,0)) | |
R5 | ((s8,0),(s0,0)) | ((s6,0),(s2,0)) | ((s3,0),(s5,0)) | ((s0,0),(s8,0)) | ((s2,0),(s6,0)) | ((s4,0),(s4,0)) | |
R6 | ((s2,0),(s6,0)) | ((s7,0),(s1,0)) | ((s1,0),(s7,0)) | ((s8,0),(s0,0)) | ((s4,0),(s4,0)) | ((s6,0),(s2,0)) | |
R7 | ((s3,0),(s5,0)) | ((s0,0),(s8,0)) | ((s5,0),(s3,0)) | ((s4,0),(s4,0)) | ((s6,0),(s2,0)) | ((s1,0),(s7,0)) | |
R8 | ((s5,0),(s3,0)) | ((s4,0),(s4,0)) | ((s6,0),(s2,0)) | ((s3,0),(s5,0)) | ((s8,0),(s0,0)) | ((s7,0),(s1,0)) | |
R9 | ((s1,0),(s7,0)) | ((s8,0),(s0,0)) | ((s4,0),(s4,0)) | ((s2,0),(s6,0)) | ((s5,0),(s3,0)) | ((s3,0),(s5,0)) | |
Θ2 | |||||||
R1 | ((s1,0),(s7,0)) | ((s4,0),(s4,0)) | ((s6,0),(s2,0)) | ((s3,0),(s5,0)) | ((s2,0),(s6,0)) | ((s8,0),(s0,0)) | |
R2 | ((s2,0),(s6,0)) | ((s7,0),(s1,0)) | ((s1,0),(s7,0)) | ((s8,0),(s0,0)) | ((s0,0),(s8,0)) | ((s5,0),(s3,0)) | |
R3 | ((s4,0),(s4,0)) | ((s6,0),(s2,0)) | ((s3,0),(s5,0)) | ((s0,0),(s8,0)) | ((s5,0),(s3,0)) | ((s7,0),(s1,0)) | |
R4 | ((s0,0),(s8,0)) | ((s5,0),(s3,0)) | ((s2,0),(s6,0)) | ((s7,0),(s1,0)) | ((s1,0),(s7,0)) | ((s3,0),(s5,0)) | |
R5 | ((s7,0),(s1,0)) | ((s1,0),(s7,0)) | ((s8,0),(s0,0)) | ((s6,0),(s2,0)) | ((s3,0),(s5,0)) | ((s4,0),(s4,0)) | |
R6 | ((s6,0),(s2,0)) | ((s3,0),(s5,0)) | ((s0,0),(s8,0)) | ((s5,0),(s3,0)) | ((s4,0),(s4,0)) | ((s2,0),(s6,0)) | |
R7 | ((s5,0),(s3,0)) | ((s2,0),(s6,0)) | ((s7,0),(s1,0)) | ((s4,0),(s4,0)) | ((s8,0),(s0,0)) | ((s0,0),(s8,0)) | |
R8 | ((s3,0),(s5,0)) | ((s8,0),(s0,0)) | ((s4,0),(s4,0)) | ((s2,0),(s6,0)) | ((s7,0),(s1,0)) | ((s1,0),(s7,0)) | |
R9 | ((s8,0),(s0,0)) | ((s0,0),(s8,0)) | ((s5,0),(s3,0)) | ((s1,0),(s7,0)) | ((s6,0),(s2,0)) | ((s4,0),(s4,0)) | |
Θ3 | |||||||
R1 | ((s4,0),(s4,0)) | ((s3,0),(s5,0)) | ((s2,0),(s6,0)) | ((s5,0),(s3,0)) | ((s6,0),(s2,0)) | ((s1,0),(s7,0)) | |
R2 | ((s2,0),(s6,0)) | ((s7,0),(s1,0)) | ((s4,0),(s4,0)) | ((s6,0),(s2,0)) | ((s3,0),(s5,0)) | ((s5,0),(s3,0)) | |
R3 | ((s7,0),(s1,0)) | ((s1,0),(s7,0)) | ((s8,0),(s0,0)) | ((s4,0),(s4,0)) | ((s2,0),(s6,0)) | ((s3,0),(s5,0)) | |
R4 | ((s8,0),(s0,0)) | ((s4,0),(s4,0)) | ((s3,0),(s5,0)) | ((s1,0),(s7,0)) | ((s5,0),(s3,0)) | ((s6,0),(s2,0)) | |
R5 | ((s6,0),(s2,0)) | ((s8,0),(s0,0)) | ((s1,0),(s7,0)) | ((s3,0),(s5,0)) | ((s0,0),(s8,0)) | ((s7,0),(s1,0)) | |
R6 | ((s5,0),(s3,0)) | ((s2,0),(s6,0)) | ((s0,0),(s8,0)) | ((s8,0),(s0,0)) | ((s1,0),(s7,0)) | ((s4,0),(s4,0)) | |
R7 | ((s0,0),(s8,0)) | ((s5,0),(s3,0)) | ((s6,0),(s2,0)) | ((s7,0),(s1,0)) | ((s4,0),(s4,0)) | ((s8,0),(s0,0)) | |
R8 | ((s1,0),(s7,0)) | ((s6,0),(s2,0)) | ((s7,0),(s1,0)) | ((s0,0),(s8,0)) | ((s8,0),(s0,0)) | ((s2,0),(s6,0)) | |
R9 | ((s3,0),(s5,0)) | ((s0,0),(s8,0)) | ((s5,0),(s3,0)) | ((s2,0),(s6,0)) | ((s7,0),(s1,0)) | ((s0,0),(s8,0)) | |
Θ4 | |||||||
R1 | ((s8,0),(s0,0)) | ((s2,0),(s6,0)) | ((s3,0),(s5,0)) | ((s4,0),(s4,0)) | ((s5,0),(s3,0)) | ((s6,0),(s2,0)) | |
R2 | ((s2,0),(s6,0)) | ((s6,0),(s2,0)) | ((s0,0),(s8,0)) | ((s3,0),(s5,0)) | ((s8,0),(s0,0)) | ((s5,0),(s3,0)) | |
R3 | ((s3,0),(s5,0)) | ((s7,0),(s1,0)) | ((s8,0),(s0,0)) | ((s6,0),(s2,0)) | ((s1,0),(s7,0)) | ((s8,0),(s0,0)) | |
R4 | ((s4,0),(s4,0)) | ((s5,0),(s3,0)) | ((s1,0),(s7,0)) | ((s0,0),(s8,0)) | ((s6,0),(s2,0)) | ((s2,0),(s6,0)) | |
R5 | ((s1,0),(s7,0)) | ((s0,0),(s8,0)) | ((s4,0),(s4,0)) | ((s2,0),(s6,0)) | ((s7,0),(s1,0)) | ((s3,0),(s5,0)) | |
R6 | ((s5,0),(s3,0)) | ((s8,0),(s0,0)) | ((s2,0),(s6,0)) | ((s1,0),(s7,0)) | ((s0,0),(s8,0)) | ((s4,0),(s4,0)) | |
R7 | ((s7,0),(s1,0)) | ((s1,0),(s7,0)) | ((s5,0),(s3,0)) | ((s8,0),(s0,0)) | ((s4,0),(s4,0)) | ((s7,0),(s1,0)) | |
R8 | ((s6,0),(s2,0)) | ((s4,0),(s4,0)) | ((s7,0),(s1,0)) | ((s5,0),(s3,0)) | ((s3,0),(s5,0)) | ((s1,0),(s7,0)) | |
R9 | ((s0,0),(s8,0)) | ((s3,0),(s5,0)) | ((s6,0),(s2,0)) | ((s7,0),(s1,0)) | ((s2,0),(s6,0)) | ((s0,0),(s8,0)) |
The MAGDM technique to select best arc welding robot involves the following cases:
Case 1: Assume that the information about the attribute weights is completely unknown: Utilize the Eq (5.12) to get the optimal weight vector ϖ=(0.1574,0.1881,0.2079,0.1398,0.1449,0.1619)T.
Step 1. Individual expert assessments can be integrated into the collective assessing matrix with 2TLq-ROFNs, according to Tables 4 and 5 and Eq (4.3) (q = 4 and κ = 3) (see Table 6).
G1 | G2 | G3 | |
R1 | ((s7,−0.3891),(s4,−0.2277)) | ((s6,0.0997),(s4,−0.0756)) | ((s7,−0.1965),(s3,0.2730)) |
R2 | ((s6,0.0081),(s4,0.1537)) | ((s7,0.3642),(s2,0.1177)) | ((s0,0),(s8,0)) |
R3 | ((s7,−0.0292),(s3,−0.1356)) | ((s6,0.4762),(s4,0.1480)) | ((s8,0),(s0,0)) |
R4 | ((s0,0),(s8,0)) | ((s7,0.0485),(s2,0.2979)) | ((s5,0.4993),(s5,−0.3450)) |
R5 | ((s7,0.0101),(s3,0.0473)) | ((s5,−0.3156),(s7,−0.1305)) | ((s7,−0.3557),(s4,−0.3383)) |
R6 | ((s7,−0.0726),(s3,−0.1504)) | ((s7,0.0101),(s3,0.2390)) | ((s0,0),(s8,0)) |
R7 | ((s5,0.3996),(s6,0.0833)) | ((s4,−0.1232),(s7,0.0370)) | ((s7,0.3708),(s2,−0.1898)) |
R8 | ((s7,−0.4816),(s4,−0.3182)) | ((s7,0.2618),(s2,0.3874)) | ((s7,0.3997),(s2,−0.1125)) |
R9 | ((s5,−0.0390),(s7,−0.2683)) | ((s0,0),(s8,0)) | ((s7,0.1479),(s2,0.1867)) |
G4 | G5 | G6 | |
R1 | ((s6,0.2466),(s4,−0.1424)) | ((s5,−0.1877),(s7,−0.4491)) | ((s7,0.2954),(s3,−0.1998)) |
R2 | ((s7,0.2201),(s3,−0.4669)) | ((s6,−0.0280),(s6,−0.0258)) | ((s7,−0.1752),(s3,−0.0620)) |
R3 | ((s6,−0.2876),(s6,−0.3069)) | ((s6,−0.0466),(s4,−0.2966)) | ((s6,−0.0874),(s6,−0.0255)) |
R4 | ((s5,0.2866),(s7,−0.3760)) | ((s6,0.0168),(s4,0.4795)) | ((s6,0.4588),(s4,−0.4168)) |
R5 | ((s5,−0.3423),(s7,−0.3634)) | ((s5,−0.1427),(s7,−0.4539)) | ((s7,−0.2352),(s3,−0.0154)) |
R6 | ((s7,−0.1643),(s3,0.4871)) | ((s5,−0.3171),(s7,−0.2974)) | ((s7,−0.4421),(s3,0.3799)) |
R7 | ((s7,0.3384),(s2,0.3524)) | ((s7,0.2766),(s2,−0.3623)) | ((s5,0.2401),(s7,−0.3311)) |
R8 | ((s5,−0.4488),(s7,−0.3605)) | ((s7,0.4941,(s2,−0.0552)) | ((s5,0.4849),(s5,−0.1040)) |
R9 | ((s6,0.0488),(s4,0.3846)) | ((s7,0.0848),(s3,−0.0375)) | ((s0,0),(s8,0)) |
Step 2. Determine the weighted assessing matrix with 2TLq-ROFNs using Eq (5.14) (see Table 7).
G1 | G2 | G3 | |
R1 | ((s6,0.0539),(s5,−0.0285)) | ((s6,−0.2441),(s5,−0.3317)) | ((s7,−0.4061),(s4,−0.2107)) |
R2 | ((s5,0.4486),(s5,0.2840)) | ((s7,0.0878),(s3,−0.0722)) | ((s0,0),(s8,0)) |
R3 | ((s6,0.4378),(s4,0.1766)) | ((s6,0.1349),(s5,−0.1320)) | ((s8,0),(s0,0)) |
R4 | ((s0,0),(s8,0)) | ((s7,−0.2648),(s3,0.1142)) | ((s5,0.2848),(s5,0.0870)) |
R5 | ((s6,0.4812),(s4,0.3434)) | ((s4,0.3848),(s7,0.1293)) | ((s6,0.4298),(s4,0.1621)) |
R6 | ((s6,0.3903),(s4,0.1630)) | ((s7,−0.3064),(s4,0.0374)) | ((s0,0),(s8,0)) |
R7 | ((s5,−0.1340),(s7,0.2730)) | ((s4,−0.3785),(s7,0.2604)) | ((s7,0.1988),(s2,0.3094)) |
R8 | ((s6,−0.0415),(s5,−0.1043)) | ((s7,−0.0291),(s3,0.2056)) | ((s7,0.2309),(s2,0.3916)) |
R9 | ((s4,0.4567),(s7,0.1722)) | ((s0,0),(s8,0)) | ((s7,−0.0436),(s3,−0.2953)) |
G4 | G5 | G6 | |
R1 | ((s6,−0.4600),(s5,0.3085)) | ((s4,0.2348),(s7,0.1206)) | ((s7,−0.1581),(s4,0.0392)) |
R2 | ((s7,−0.4190),(s4,0.1904)) | ((s5,0.3148),(s7,−0.2517)) | ((s6,0.3139),(s4,0.1675)) |
R3 | ((s5,0.0255),(s7,−0.3929)) | ((s5,0.2969),(s6,−0.4309)) | ((s5,0.3897),(s7,−0.3847)) |
R4 | ((s5,−0.3703),(s7,0.1945)) | ((s5,0.3582),(s6,−0.2940)) | ((s6,−0.0676),(s,−0.2575)) |
R5 | ((s4,0.0599),(s7,0.2022)) | ((s4,0.2756),(s7,0.1176)) | ((s6,0.2501),(s4,0.2387)) |
R6 | ((s6,0.1459),(s5,0.0155)) | ((s4,0.1177),(s7,0.2162)) | ((s6,0.0339),(s5,−0.4345)) |
R7 | ((s7,−0.2744),(s4,0.0196)) | ((s7,−0.3088),(s4,−0.0700)) | ((s5,−0.2523),(s7,0.1062)) |
R8 | ((s4,−0.0354),(s7,0.2039)) | ((s7,−0.0325),(s4,−0.4910)) | ((s5,−0.0211),(s6,−0.1888)) |
R9 | ((s5,0.3467),(s6,−0.2952)) | ((s6,0.2762),(s4,0.4843)) | ((s0,0),(s8,0)) |
Step 3. Calculate the NIS by Eq (5.16).
NIS={((s0,0),(s8,0)),((s0,0),(s8,0)),((s0,0),(s8,0)),((s4,−0.0354),(s7,0.2039))((s4,0.1177),(s7,0.2162)),((s0,0),(s8,0))}. |
Step 4. Calculate the HDt and EDt:
HD1=2.9440,HD2=2.5988,HD3=3.0646,HD4=2.1374,HD5=2.2474,HD6=2.3838,HD7=2.6034,HD8=3.1897,HD9=1.7095.ED1=3.5009,ED2=2.9797,ED3=3.4908,ED4=2.6627,ED5=2.6899,ED6=2.7760,ED7=2.9501,ED8=3.6246,ED8=1.9711. |
Step 5. Determine the RA matrix (see Table 8).
R1 | R2 | R3 | R4 | R5 | R6 | R7 | R8 | R9 | |
R1 | 0 | 0.8665 | 0.0101 | 1.6448 | 1.5077 | 1.2851 | 0.8915 | −0.3694 | 2.7643 |
R2 | −0.8665 | 0 | −0.9769 | 0.7783 | 0.6413 | 0.4187 | 0.0251 | −1.2359 | 1.8979 |
R3 | −0.0101 | 0.9769 | 0 | 1.7553 | 1.6182 | 1.3956 | 1.0020 | −0.2589 | 2.8748 |
R4 | −1.6448 | −0.7783 | −1.7553 | 0 | −0.1371 | −0.3597 | −0.7533 | −2.0142 | 1.1195 |
R5 | −1.5077 | −0.6413 | −1.6182 | 0.1371 | 0 | −0.2226 | −0.6162 | −1.8771 | 1.2566 |
R6 | −1.2851 | −0.4187 | −1.3956 | 0.3597 | 0.2226 | 0 | −0.3936 | −1.6545 | 1.4792 |
R7 | −0.8915 | −0.0251 | −1.0020 | 0.7533 | 0.6162 | 0.3936 | 0 | −1.2609 | 1.8728 |
R8 | 0.3694 | 1.2359 | 0.2589 | 2.0142 | 1.8771 | 1.6545 | 1.2609 | 0 | 3.1337 |
R9 | −2.7643 | −1.8979 | −2.8748 | −1.1195 | −1.2566 | −1.4792 | −1.8728 | −3.1337 | 0 |
Step 6. Derive the £t by using Eq (5.22). The results of £t are as follows:
£1=8.6007,£2=0.6820,£3=9.3537,£4=−6.3231,£5=−5.0894,£6=−3.0861,£7=0.4563,£8=11.8046,£9=−16.39873. |
Step 7. On the basis of computing results of £t, all the alternatives can be ranked. The ranking of alternatives is as follows:
R8>R3>R1>R2>R7>R6>R5>R4>R9. |
So, R8 is the best alternative.
Case 2: The weights of attributes are partly known, and the information of known weights is as follows:
Ψ={0.15≤ϖ1≤0.2,0.16≤ϖ2≤0.18,0.05≤ϖ3≤0.15,0.25≤ϖ4≤0.35,0.3≤ϖ5≤0.45,0.09≤ϖ6≤0.13,6∑ȷ=1ϖȷ=1}. |
To construct the single-objective model, utilize the model (M-2) as follows:
(M−2){maxD(ϖ)=17.5771ϖ1+21.0079ϖ2+23.2248ϖ3+15.6182ϖ4+16.1851ϖ5+18.0836ϖ6s.t.w∈Ψ,wȷ≥0,j=1,2,…,6,6∑ȷ=1wȷ=1. |
We obtain the optimal weighting vector by solving this model ϖ=(0.1500,0.1600,0.0500,0.2500,0.3000,0.0900)T.
Step 1. Determine the weighted assessing matrix with 2TLq-ROFNs using Eq (5.14) (see Table 9).
G1 | G2 | G3 | |
R1 | ((s4,0.3805),(s7,0.1469)) | ((s4,0.0216),(s7,0.1384)) | ((s3,0.4930),(s8,−0.3496)) |
R2 | ((s4,−0.1115),(s7,0.2509)) | ((s5,0.2350),(s6,0.4675)) | ((s0,0),(s8,0)) |
R3 | ((s5,−0.2826),(s7,−0.1423)) | ((s4,0.3307),(s7,0.2019)) | ((s8,0),(s0,0)) |
R4 | ((s0,0),(s8,0)) | ((s5,−0.1305),(s7,−0.4475)) | ((s3,−0.3221),(s8,−0.2137)) |
R5 | ((s5,−0.2428),(s7,−0.0783)) | ((s3,0.0013),(s8,−0.1927)) | ((s3,0.3777),(s8,−0.3066)) |
R6 | ((s5,−0.3257),(s7,−0.1476)) | ((s5,−0.1706),(s7,−0.0775)) | ((s0,0),(s8,0)) |
R7 | ((s3,0.4426),(s8,−0.3220)) | ((s2,0.4665),(s8,−0.1625)) | ((s4,0.0118),(s7,0.4271)) |
R8 | ((s4,0.3003),(s7,0.1209)) | ((s5,0.1080),(s7,−0.4073)) | ((s4,0.0193),(s7,0.4427)) |
R9 | ((s3,0.1395),(s8,0.2045)) | ((s0,0),(s8,0)) | ((s4,−0.2276),(s7,0.4976)) |
G4 | G5 | G6 | |
R1 | ((s5,−0.3960),(s7,−0.3335)) | ((s4,−0.3952),(s8,−0.4655)) | ((s5,−0.4962),(s7,0.2788)) |
R2 | ((s6,−0.4103),(s6,0.0011)) | ((s5,−0.4401),(s7,0.3290)) | ((s4,0.0492),(s7,0.3103)) |
R3 | ((s4,0.1512),(s7,0.3477)) | ((s5,−0.4562),(s7,−0.3610)) | ((s3,0.3673),(s8,−0.2075)) |
R4 | ((s4,−0.1886),(s8,−0.3687)) | ((s5,−0.4008),(s7,−0.2775)) | ((s4,−0.2445),(s7,0.4421)) |
R5 | ((s3,0.3309),(s8,−0.3651)) | ((s4,−0.3597),(s8,−0.4672)) | ((s4,−0.0015),(s7,0.3274)) |
R6 | ((s5,0.1621),(s7,−0.4997)) | ((s4,−0.4967),(s8,−0.4136)) | ((s4,−0.1684),(s7,0.4031)) |
R7 | ((s6,−0.2606),(s6,0.1089)) | ((s6,−0.1166),(s6,−0.4517)) | ((s3,−0.0639),(s8,−0.1300)) |
R8 | ((s3,0.2513),(s8,−0.3643)) | ((s6,0.1821),(s5,0.2339)) | ((s3,0.0888),(s8,−0.3458)) |
R9 | ((s4,0.4321),(s7,−0.1166)) | ((s5,0.4627),(s6,0.0617)) | ((s0,0),(s8,0)) |
Step 3. Calculate the NIS by Eq (5.16).
NIS={((s0,0),(s8,0)),((s0,0),(s8,0)),((s0,0),(s8,0)),((s4,−0.0354),(s7,0.2039))((s4,0.1177),(s7,0.2162)),((s0,0),(s8,0))}. |
Step 4. Calculate the HDt and EDt.
HD1=2.9440,HD2=2.5988,HD3=3.0646,HD4=2.1374,HD5=2.2474,HD6=2.3838,HD7=2.6034,HD8=3.1897,HD9=1.7095.ED1=3.5009,ED2=2.9797,ED3=3.4908,ED4=2.6627,ED5=2.6899,ED6=2.7760,ED7=2.9501,ED8=3.6246,ED9=1.9711. |
Step5. Determine the RA matrix (see Table 10).
R1 | R2 | R3 | R4 | R5 | R6 | R7 | R8 | R9 | |
R1 | 0 | 0.8665 | 0.0101 | 1.6448 | 1.5077 | 1.2851 | 0.8915 | −0.3694 | 2.7643 |
R2 | −0.8665 | 0 | −0.9769 | 0.7783 | 0.6413 | 0.4187 | 0.0251 | −1.2359 | 1.8979 |
R3 | −0.0101 | 0.9769 | 0 | 1.7553 | 1.6182 | 1.3956 | 1.0020 | −0.2589 | 2.8748 |
R4 | −1.6448 | -0.7783 | -1.7553 | 0 | -0.1371 | -0.3597 | -0.7533 | -2.0142 | 1.1195 |
{{R}}_{5} | -1.5077 | -0.6413 | -1.6182 | 0.1371 | 0 | -0.2226 | -0.6162 | -1.8771 | 1.2566 |
{{R}}_{6} | -1.2851 | -0.4187 | -1.3956 | 0.3597 | 0.2226 | 0 | -0.3936 | -1.6545 | 1.4792 |
{{R}}_{7} | -0.8915 | -0.0251 | -1.0020 | 0.7533 | 0.6162 | 0.3936 | 0 | -1.2609 | 1.8728 |
{{R}}_{8} | 0.3694 | 1.2359 | 0.2589 | 2.0142 | 1.8771 | 1.6545 | 1.2609 | 0 | 3.1337 |
{{R}}_{9} | -2.7643 | -1.8979 | -2.8748 | -1.1195 | -1.2566 | -1.4792 | -1.8728 | -3.1337 | 0 |
Step 6. Derive the £_{\mathfrak{t}} by using Eq (5.22). The results of £_{\mathfrak{t}} are as follows:
\begin{eqnarray*} &&£_{1} = 8.6007, \; £_{2} = 0.6820, \; £_{3} = 9.3537, \; £_{4} = -6.3231, \; £_{5} = -5.0894, \\ &&£_{6} = -3.0861, \; £_{7} = 0.4563 , \; £_{8} = 11.8046 , \; £_{9} = -16.3987. \end{eqnarray*} |
Step 7. On the basis of computing results of £_{\mathfrak{t}} , all the alternatives can be ranked. The ranking of alternatives is as follows:
{{R}}_{8} > {{R}}_{3} > {{R}}_{1} > {{R}}_{2} > {{R}}_{7} > {{R}}_{6} > {{R}}_{5} > {{R}}_{4} > {{R}}_{9}. |
So, {{R}}_8 is the best alternative.
The MAGDM technique to select best arc welding robot involves the following cases:
Case 1: Assume that the information about the attribute weights is completely unknown: Utilize the Eq (5.12) to get the optimal weight vector \varpi = (0.1574, 0.1881, 0.2079, 0.1398, 0.1449, 0.1619)^{T} .
Step 1. Individual expert assessments can be integrated into the collective assessing matrix with 2TL q -ROFNs, according to Tables 4 and 5 and Eq (4.7) ( q = 4 and \kappa = 3) (see Table 11).
{{G}}_{1} | {{G}}_{2} | {{G}}_{3} | |
{{R}}_{1} | ((s_6, -0.0961), (s_6, -0.3603)) | ((s_2, 0.1718), (s_7, 0.1783)) | ((s_4, 0.0624), (s_6, 0.2396)) |
{{R}}_{2} | ((s_3, -0.3998), (s_7, 0.0990)) | ((s_5, -0.2385), (s_5, 0.4972)) | ((s_1, 0.4422), (s_8, -0.2737)) |
{{R}}_{3} | ((s_4, 0.2422), (s_6, 0.0676)) | ((s_4, 0.3482), (s_6, 0.1491)) | ((s_8, 0), (s_0, 0)) |
{{R}}_{4} | ((s_6, -0.1292), (s_6, -0.1601)) | ((s_3, 0.4843), (s_6, 0.3538)) | ((s_2, -0.4878), (s_7, 0.4843)) |
{{R}}_{5} | ((s_7, -0.0444), (s_4, 0.2884)) | ((s_6, 0.3337), (s_6, -0.4017)) | ((s_6, -0.0684), (s_5, -0.4954)) |
{{R}}_{6} | ((s_4, -0.2807), (s_6, 0.3164)) | ((s_7, -0.3151), (s_5, -0.0977)) | ((s_1, -0.0599), (s_8, -0.2005)) |
{{R}}_{7} | ((s_4, 0.0689), (s_6, 0.2841)) | ((s_2, 0.0635, (s_7, 0.4490)) | ((s_4, 0.4322), (s_6, -0.2882)) |
{{R}}_{8} | ((s_3, 0.4526), (s_7, -0.3928)) | ((s_6, 0.4542), (s_5, -0.110)) | ((s_5, -0.2896), (s_6, -0.4666)) |
{{R}}_{9} | ((s_5, 0.2397), (s_6, 0.3559)) | ((s_5, 0.3566), (s_6, 0.1203)) | ((s_4, -0.2063), (s_6, 0.1743)) |
{{G}}_{4} | {{G}}_{5} | {{G}}_{6} | |
{{R}}_{1} | ((s_3, -0.2832), (s_7, -0.0381)) | ((s_3, 0.2726), (s_7, -0.1462)) | ((s_8, 0), (s_0, 0)) |
{{R}}_{2} | ((s_6, 0.4719), (s_5, -0.0714)) | ((s_7, -0.3232), (s_5, -0.1548)) | ((s_3, 0.3950), (s_6, 0.4988)) |
{{R}}_{3} | ((s_4, -0.2593), (s_7, -0.4324)) | ((s_3, -0.4913), (s_7, 0.1456)) | ((s_7, -0.1266), (s_5, -0.3632)) |
{{R}}_{4} | ((s_4, 0.4649), (s_6, 0.3020)) | ((s_3, 0.3650), (s_7, -0.1510)) | ((s_3, 0.1467), (s_7, -0.2130)) |
{{R}}_{5} | ((s_3, -0.0851), (s_7, 0.0082)) | ((s_4, -0.4871), (s_7, -0.3113)) | ((s_4, -0.4392), (s_6, 0.4802)) |
{{R}}_{6} | ((s_8, 0), (s_0, 0) | ((s_2, 0.3524), (s_7, 0.3384)) | ((s_3, 0.1466), (s_7, -0.2670)) |
{{R}}_{7} | ((s_7, -0.2974), (s_5, -0.3171)) | ((s_6, 0.4789), (s_5, -0.1396)) | ((s_7, -0.1059), (s_5, -0.3389)) |
{{R}}_{8} | ((s_3, -0.4455), (s_7, 0.1303)) | ((s_8, 0, (s_0, 0) | ((s_3, -0.2232), (s_7, 0.1423)) |
{{R}}_{9} | ((s_3, 0.1835), (s_7, 0.1663)) | ((s_4, 0.1105), (s_6, 0.1671)) | ((s_2, 0.1042), (s_7, 0.4790)) |
Step 2. Determine the weighted assessing matrix with 2TL q -ROFNs (see Table 12).
{{G}}_{1} | {{G}}_{2} | {{G}}_{3} | |
{{R}}_{1} | ((s_7, -0.3993), (s_5, 0.0934)) | ((s_3, -0.0159), (s_7, -0.1225)) | ((s_5, -0.4604), (s_6, 0.0193)) |
{{R}}_{2} | ((s_4, -0.0714), (s_7, -0.4190)) | ((s_5, 0.4034), (s_5, 0.1645)) | ((s_2, -0.0902), (s_8, -0.3910)) |
{{R}}_{3} | ((s_5, 0.3549), (s_6, -0.4932)) | ((s_5, 0.0447), (s_6, -0.1950)) | ((s_8, 0), (s_0, 0)) |
{{R}}_{4} | ((s_7, -0.4227), (s_5, 0.2854)) | ((s_4, 0.2667), (s_6, 0.0106)) | ((s_2, -0.0130), (s_7, 0.3257)) |
{{R}}_{5} | ((s_7, 0.3222), (s_4, -0.1602)) | ((s_7, -0.2953), (s_5, 0.2628)) | ((s_6, 0.2297), (s_5, 0.2811)) |
{{R}}_{6} | ((s_5, -0.0728), (s_6, -0.2464)) | ((s_7, -0.0160), (s_5, -0.4076)) | ((s_1, 0.3354), (s_8, -0.2997)) |
{{R}}_{7} | ((s_5, 0.2154), (s_6, -0.2788)) | ((s_3, -0.1290), (s_7, 0.1869)) | ((s_5, -0.1174), (s_5, 0.4940)) |
{{R}}_{8} | ((s_5, -0.2994), (s_6, 0.0500)) | ((s_7, -0.1991), (s_5, -0.4203)) | ((s_5, 0.1376), (s_5, 0.3183)) |
{{R}}_{9} | ((s_6, 0.1205), (s_6, -0.2067)) | ((s_6, -0.0101), (s_6, -0.2236)) | ((s_4, 0.2872), (s_6, -0.0462)) |
{{G}}_{4} | {{G}}_{5} | {{G}}_{6} | |
{{R}}_{1} | ((s_4, 0.3587), (s_6, 0.2841)) | ((s_5, -0.2479), (s_6, 0.2093)) | ((s_8, 0), (s_0, 0)) |
{{R}}_{2} | ((s_7, 0.1011), (s_4, 0.3037)) | ((s_7, 0.2000), (s_5, -0.4528)) | ((s_5, -0.4211), (s_6, -0.0267)) |
{{R}}_{3} | ((s_5, 0.2174), (s_6, -0.1365)) | ((s_4, 0.0702), (s_7, -0.4642)) | ((s_7, 0.2473), (s_4, 0.0.1865)) |
{{R}}_{4} | ((s_6, -0.2367), (s_6, 0.4050)) | ((s_5, -0.1702), (s_6, 0.2042)) | ((s_4, 0.3580), (s_6, 0.2737)) |
{{R}}_{5} | ((s_5, -0.4653), (s_6, 0.3357)) | ((s_5, -0.0475), (s_6, 0.0333)) | ((s_5, -0.2768), (s_6, -0.0457)) |
{{R}}_{6} | ((s_8, 0), (s_0, 0) | ((s_4, -0.0795), (s_7, -0.2330)) | ((s_4, 0.3579), (s_6, 0.2165)) |
{{R}}_{7} | ((s_7, 0.2423), (s_4, 0.0825)) | ((s_7, 0.0749), (s_4, 0.2785)) | ((s_7, 0.2615), (s_4, 0.2088)) |
{{R}}_{8} | ((s_4, 0.2103), (s_6, 0.4752)) | ((s_8, 0), (s_0, 0)) | ((s_4, 0.0172), (s_7, -0.3362)) |
{{R}}_{9} | ((s_5, -0.2350), (s_6, 0.1438)) | ((s_5, 0.4272), (s_6, -0.4952)) | ((s_3, 0.3536), (s_7, 0.0678)) |
Step 3. Calculate the NIS by Eq (5.16).
\begin{eqnarray*} NIS & = & \{((s_4, -0.0714), (s_7, -0.4190), (s_3, -0.1290)), ((s_7, 0.1869), (s_1, 0.3354), (s_8, -0.2997)), \\ && ((s_4, 0.2103), (s_6, 0.4752), (s_4, -0.0795)), ((s_7, -0.2330), (s_3, 0.3536), (s_7, 0.0678))\}. \end{eqnarray*} |
Step 4. Calculate the HD_{\mathfrak{t}} and ED_{\mathfrak{t}} .
\begin{eqnarray*} &&HD_{1} = 1.6508, \; HD_{2} = 1.4910, \; HD_{3} = 2.1233, \; HD_{4} = 1.0588 , \; HD_{5} = 1.8788, \; \\ &&HD_{6} = 1.5187, \; HD_{7} = 2.1069, \; HD_{8} = 1.8443, \; HD_{9} = 1.1751.\\ &&ED_{1} = 1.9154, \; ED_{2} = 1.6925, \; ED_{3} = 2.2336, \; ED_{4} = 1.2755 , \; ED_{5} = 2.0536, \; \\ &&ED_{6} = 1.7067, \; ED_{7} = 2.3246, \; ED_{8} = 2.1120, \; ED_{9} = 1.3759. \end{eqnarray*} |
Step 5. Determine the RA matrix (see Table 13).
{{R}}_{1} | {{R}}_{2} | {{R}}_{3} | {{R}}_{4} | {{R}}_{5} | {{R}}_{6} | {{R}}_{7} | {{R}}_{8} | {{R}}_{9} | |
{{R}}_{1} | 0 | 0.3827 | -0.7907 | 1.2319 | -0.3662 | 0.3409 | -0.8652 | -0.3901 | 1.0152 |
{{R}}_{2} | -0.3827 | 0 | -1.1735 | 0.8492 | -0.7489 | -0.0142 | -1.2480 | -0.7729 | 0.6325 |
{{R}}_{3} | 0.7907 | 1.1735 | 0 | 2.0226 | 0.4245 | 1.1316 | -0.0745 | 0.4006 | 1.8059 |
{{R}}_{4} | -1.2319 | -0.8492 | -2.0226 | 0 | -1.5981 | -0.8910 | -2.0972 | -1.6220 | -0.2167 |
{{R}}_{5} | 0.3662 | 0.7489 | -0.4245 | 1.5981 | 0 | 0.7071 | -0.4990 | -0.0239 | 1.3814 |
{{R}}_{6} | -0.3409 | 0.0142 | -1.1316 | 0.8910 | -0.7071 | 0 | -1.2061 | -0.7310 | 0.6743 |
{{R}}_{7} | 0.8652 | 1.2480 | 0.0745 | 2.0972 | 0.4990 | 1.2061 | 0 | 0.4751 | 1.8804 |
{{R}}_{8} | 0.3901 | 0.7729 | -0.4006 | 1.6220 | 0.0239 | 0.7310 | -0.4751 | 0 | 1.4053 |
{{R}}_{9} | -1.0152 | -0.6325 | -1.8059 | 0.2167 | -1.3814 | -0.6743 | -1.8804 | -1.4053 | 0 |
Step 6. Derive the £_{\mathfrak{t}} by using Eq (5.22). The results of £_{\mathfrak{t}} are as follows:
\begin{eqnarray*} && £_{1} = 0.5583, \; £_{2} = -2.8586, \; £_{3} = 7.6750 , \; £_{4} = -10.5288, \; £_{5} = 3.8543, \\ && £_{6} = -2.5370, \; £_{7} = 8.3456, \; £_{8} = 4.0695, \; £_{9} = -8.5783. \end{eqnarray*} |
Step 7. On the basis of computing results of £_{\mathfrak{t}} , all the alternatives can be ranked. The ranking of alternatives is as follows:
{{R}}_{7} > {{R}}_{3} > {{R}}_{8} > {{R}}_{5} > {{R}}_{1} > {{R}}_{6} > {{R}}_{2} > {{R}}_{9} > {{R}}_{4}. |
So, {{R}}_7 is the best alternative.
Case 2: The weights of attributes are partly known, and the information of known weights is as follows:
\begin{eqnarray*} \Psi & = & \{0.15 \leq \varpi_1 \leq 0.2, \; 0.16 \leq \varpi_2 \leq 0.18, \; 0.05 \leq \varpi_3 \leq 0.15, \; 0.25 \leq \varpi_4 \leq 0.35, \\ &&0.3 \leq \varpi_5 \leq 0.45, 0.09 \leq \varpi_6 \leq 0.13, \sum\limits_{\jmath = 1}^6 \varpi_\jmath = 1\}. \end{eqnarray*} |
To construct the single-objective model, utilize the model (M-2) as follows:
(M-2)\\ \left\{\begin{array}{ll} \max D(\varpi) = 17.5771\varpi_{1}+21.0079\varpi_{2}+23.2248\varpi_{3}+15.6182\varpi_{4}+16.1851\varpi_{5}+18.0836\varpi_{6}\\ s.t.\; \; w\in \Im, w_{\jmath}\geq0, j = 1, 2, \ldots, 6, \; \sum\limits_{\jmath = 1}^{6}w_{\jmath} = 1. \end{array} \right. |
We obtain the optimal weighting vector by solving this model \varpi = \left(0.1500, 0.1600, 0.0500, 0.2500, 0.3000, 0.0900\right)^T .
Step 1. Determine the weighted assessing matrix with 2TL q -ROFNs using Eq (5.14) (see Table 14).
{{G}}_{1} | {{G}}_{2} | {{G}}_{3} | |
{{R}}_{1} | ((s_8, -0.3564), (s_4, -0.3858)) | ((s_6, 0.4936), (s_5, 0.0110)) | ((s_8, -0.2665), (s_3, 0.1101)) |
{{R}}_{2} | ((s_7, -0.2411), (s_5, -0.1498)) | ((s_7, 0.3627), (s_4, -0.4322)) | ((s_7, 0.3432), (s_4, 0.4644)) |
{{R}}_{3} | ((s_7, 0.2739), (s_4, 0.0657)) | ((s_7, 0.2565), (s_4, 0.0607)) | ((s_8, 0), (s_0, 0)) |
{{R}}_{4} | ((s_8, -0.3629), (s_4, -0.2386)) | ((s_7, 0.0038), (s_4, 0.2273)) | ((s_7, 0.3607), (s_4, 0.1157)) |
{{R}}_{5} | ((s_8, -0.1661), (s_3, -0.3069)) | ((s_8, -0.2934), (s_4, -0.3587)) | ((s_8, -0.1188), (s_3, -0.3242)) |
{{R}}_{6} | ((s_7, 0.1317), (s_4, 0.1317)) | ((s_8, -0.2266), (s_3, 0.1496)) | ((s_7, 0.1878), (s_5, -0.3895)) |
{{R}}_{7} | ((s_7, -0.2285), (s_4, 0.1055)) | ((s_6, 0.4407), (s_5, 0.3486)) | ((s_8, -0.2328), (s_3, -0.2034)) |
{{R}}_{8} | ((s_7, 0.0526), (s_4, 0.3772)) | ((s_8, -0.2702), (s_3, 0.1405)) | ((s_8, -0.2091), (s_3, -0.3033)) |
{{R}}_{9} | ((s_8, -0.4920), (s_4, 0.1640)) | ((s_8, -0.4750), (s_4, 0.0379)) | ((s_8, -0.2929), (s_3, 0.0695)) |
{{G}}_{4} | {{G}}_{5} | {{G}}_{6} | |
{{R}}_{1} | ((s_6, 0.1071), (s_5, 0.2954)) | ((s_6, 0.1183), (s_5, 0.3971)) | ((s_8, 0), (s_0, 0)) |
{{R}}_{2} | ((s_8, -0.4129), (s_4, 0.4646)) | ((s_8, 0.4224), (s_4, -0.1227)) | ((s_7, 0.4061), (s_4, -0.2140)) |
{{R}}_{3} | ((s_7, 0.3846), (s_5, -0.1023)) | ((s_6, -0.3507), (s_6, -0.2773)) | ((s_8, -0.1085), (s_3, -0.4259)) |
{{R}}_{4} | ((s_7, -0.0854), (s_5, 0.3467)) | ((s_6, 0.1696), (s_5, 0.3921)) | ((s_7, 0.3556), (s_4, 0.0171)) |
{{R}}_{5} | ((s_6, 0.2155), (s_5, 0.3451)) | ((s_6, 0.2498), (s_5, 0.2271)) | ((s_7, 0.4379), (s_4, -0.2282)) |
{{R}}_{6} | ((s_8, 0), (s_0, 0)) | ((s_6, -0.4586), (s_6, -0.0364)) | ((s_7, 0.3556), (s_4, -0.0279)) |
{{R}}_{7} | ((s_8, -0.3462), (s_3, 0.3498)) | ((s_8, -0.4904), (s_4, -0.3572)) | ((s_8, -0.1064), (s_3, -0.4116)) |
{{R}}_{8} | ((s_6, 0.0137), (s_5, 0.4829)) | ((s_8, 0), (s_0, 0)) | ((s_7, 0.2733), (s_4, 0.3421)) |
{{R}}_{9} | ((s_6, 0.3539), (s_5, 0.1601)) | ((s_7, -0.4486), (s_5, 0.2670)) | ((s_7, 0.0940), (s_5, -0.2737)) |
Step 2. Calculate the NIS by Eq (5.16).
\begin{eqnarray*} NIS & = & \{((s_7, -0.2411), (s_5, -0.1498)), ((s_6, 0.4407), (s_5, 0.3486)), ((s_7, 0.1878), (s_5, -0.3895)), \\&&((s_6, 0.0137), (s_5, 0.4829)) ((s_6, -0.4586), (s_6, -0.0364)) , ((s_7, 0.0940), (s_5, -0.2737))\}. \end{eqnarray*} |
Step 3. Calculate the HD_{\mathfrak{t}} and ED_{\mathfrak{t}} .
\begin{eqnarray*} &&HD_{1} = 0.7762 , \; HD_{2} = 1.1088, \; HD_{3} = 0.9165, \; HD_{4} = 0.7636, \; HD_{5} = 1.0274, \; \\ && HD_{6} = 0.9459, \; HD_{7} = 1.2580, \; HD_{8} = 1.1456, \; HD_{9} = 0.8280.\\ &&ED_{1} = 0.9534, \; ED_{2} = 1.3068, \; ED_{3} = 1.1052, \; ED_{4} = 0.8774, \; ED_{5} = 1.2471, \; \\ && ED_{6} = 1.1608, \; ED_{7} = 1.5152, \; ED_{8} = 1.3791, \; ED_{9} = 0.9814. \end{eqnarray*} |
Step 4. Determine the RA matrix (see Table 15).
{{R}}_{1} | {{R}}_{2} | {{R}}_{3} | {{R}}_{4} | {{R}}_{5} | {{R}}_{6} | {{R}}_{7} | {{R}}_{8} | {{R}}_{9} | |
{{R}}_{1} | 0 | -0.6860 | -0.2921 | 0.0886 | -0.5449 | -0.3772 | -1.0436 | -0.7952 | -0.0798 |
{{R}}_{2} | 0.6860 | 0 | 0.3939 | 0.7745 | 0.1410 | 0.3088 | -0.3576 | -0.1093 | 0.6062 |
{{R}}_{3} | 0.2921 | -0.3939 | 0 | 0.3807 | -0.2529 | -0.0851 | -0.7515 | -0.5032 | 0.2123 |
{{R}}_{4} | -0.0886 | -0.7745 | -0.3807 | 0 | -0.6335 | -0.4658 | -1.1322 | -0.8838 | -0.1684 |
{{R}}_{5} | 0.5449 | -0.1410 | 0.2529 | 0.6335 | 0 | 0.1677 | -0.4987 | -0.2503 | 0.4652 |
{{R}}_{6} | 0.3772 | -0.3088 | 0.0851 | 0.4658 | -0.1677 | 0 | -0.6664 | -0.4180 | 0.2974 |
{{R}}_{7} | 1.0436 | 0.3576 | 0.7515 | 1.1322 | 0.4987 | 0.6664 | 0 | 0.2484 | 0.9638 |
{{R}}_{8} | 0.7952 | 0.1093 | 0.5032 | 0.8838 | 0.2503 | 0.4180 | -0.2484 | 0 | 0.7155 |
{{R}}_{9} | 0.0798 | -0.6062 | -0.2123 | 0.1684 | -0.4652 | -0.2974 | -0.9638 | -0.7155 | 0 |
Step 5. Derive the £_{\mathfrak{t}} by using Eq (5.22). The results of £_{\mathfrak{t}} are as follows:
\begin{eqnarray*} &&£_{1} = -3.7303, \; £_{2} = 2.4435, \; £_{3} = -1.1015, \; £_{4} = -4.5274, \; £_{5} = 1.1742, \; \\ &&£_{6} = -0.3353, \; £_{7} = 5.6622, \; £_{8} = 3.4269, \; £_{9} = -3.0122. \end{eqnarray*} |
Step 6. On the basis of computing results of £_{\mathfrak{t}} , all the alternatives can be ranked. The ranking of alternatives is as follows:
{{R}}_{7} > {{R}}_{8} > {{R}}_{2} > {{R}}_{5} > {{R}}_{6} > {{R}}_{3} > {{R}}_{9} > {{R}}_{1} > {{R}}_{4}. |
So, {{R}}_7 is the best alternative.
The impact of q and {\kappa} on arc welding robot selection is investigated in this section. First, as indicated in Tables 16 and 17, we find the average solutions of the arc welding robots as q values vary (from 1 to 8) ( {\kappa} = 3) in the 2TL q -ROFWHM operator. After altering q , Tables 18 and 19 show how the average solutions of the alternatives differ and ranking on the basis of the average solutions shown in Tables 16 and 17. If the DM wants to make a judgement based on complicated data, just increase q to enlarge the information representation space of 2TL q -ROFS. Effect of variation of q and {\kappa} on the 2TL q -ROFWHM operator is shown in Figures 2 and 3, respectively.
Parameter | £_{1} | £_{2} | £_{3} | £_{4} | £_{5} | £_{6} | £_{7} | £_{8} | £_{9} |
q=1 | 9.2419 | 0.4522 | 8.0325 | -5.5686 | -5.6983 | -2.6495 | -0.7889 | 12.5685 | -15.5897 |
q=2 | 8.3757 | 0.8741 | 8.8384 | -6.4119 | -5.5053 | -2.9184 | 0.4589 | 12.1618 | -15.8735 |
q=3 | 8.6811 | 0.4570 | 9.9984 | -6.2013 | -4.6643 | -3.2330 | 0.3786 | 11.4614 | -16.8780 |
q=4 | 9.2018 | 0.1342 | 10.9091 | -6.9945 | -3.6352 | -3.6145 | 0.4292 | 11.1241 | -17.5541 |
q=5 | 9.0140 | -0.0512 | 11.6425 | -7.1200 | -3.0797 | -4.0100 | 0.5400 | 11.0921 | -18.0277 |
q=6 | 9.4112 | -0.4079 | 12.0041 | -7.2024 | -2.5031 | -4.4406 | 0.7780 | 10.7475 | -18.3869 |
q=7 | 9.0445 | -0.8170 | 12.9281 | -7.2637 | -1.8192 | -4.8432 | 0.9374 | 10.5033 | -18.6703 |
q=8 | 9.0544 | -0.9737 | 13.3726 | -7.3163 | -1.3976 | -5.2068 | 1.1006 | 10.2916 | -18.9248 |
Parameter | £_{1} | £_{2} | £_{3} | £_{4} | £_{5} | £_{6} | £_{7} | £_{8} | £_{9} |
{\kappa}=1 | -1.2161 | -0.3893 | 1.6164 | -6.7267 | 5.3976 | -7.5968 | 8.8638 | 0.7522 | -0.7012 |
{\kappa}=2 | 6.3461 | -1.8035 | 8.3705 | -7.0144 | -3.2918 | 0.3788 | 2.6722 | 9.5008 | -15.1586 |
{\kappa}=3 | 8.6007 | 0.6820 | 9.3537 | -6.3231 | -5.0894 | -3.0861 | 0.4563 | 11.8046 | -16.3987 |
{\kappa}=4 | 11.5855 | 2.7295 | 1.3611 | -2.0245 | -11.4463 | 0.5527 | -6.1084 | 15.4832 | -12.1329 |
Parameter | Ranking |
q=1 | {{R}}_{8} > {{R}}_{1} > {{R}}_{3} > {{R}}_{2} > {{R}}_{7} > {{R}}_{6} > {{R}}_{5} > {{R}}_{4} > {{R}}_{9} |
q=2 | {{R}}_{8} > {{R}}_{3} > {{R}}_{1} > {{R}}_{2} > {{R}}_{7} > {{R}}_{6} > {{R}}_{5} > {{R}}_{4} > {{R}}_{9} |
q=3 | {{R}}_{8} > {{R}}_{3} > {{R}}_{1} > {{R}}_{2} > {{R}}_{7} > {{R}}_{6} > {{R}}_{5} > {{R}}_{4} > {{R}}_{9} |
q=4 | {{R}}_{8} > {{R}}_{3} > {{R}}_{1} > {{R}}_{7} > {{R}}_{2} > {{R}}_{6} > {{R}}_{5} > {{R}}_{4} > {{R}}_{9} |
q=5 | {{R}}_{8} > {{R}}_{3} > {{R}}_{1} > {{R}}_{7} > {{R}}_{2} > {{R}}_{5} > {{R}}_{6} > {{R}}_{4} > {{R}}_{9} |
q=6 | {{R}}_{8} > {{R}}_{3} > {{R}}_{1} > {{R}}_{7} > {{R}}_{2} > {{R}}_{5} > {{R}}_{6} > {{R}}_{4} > {{R}}_{9} |
q=7 | {{R}}_{8} > {{R}}_{3} > {{R}}_{1} > {{R}}_{7} > {{R}}_{2} > {{R}}_{5} > {{R}}_{6} > {{R}}_{4} > {{R}}_{9} |
q=8 | {{R}}_{8} > {{R}}_{3} > {{R}}_{1} > {{R}}_{7} > {{R}}_{2} > {{R}}_{5} > {{R}}_{6} > {{R}}_{4} > {{R}}_{9} |
Parameter | Ranking |
{\kappa} =1 | {{R}}_{7} > {{R}}_{5} > {{R}}_{3} > {{R}}_{8} > {{R}}_{2} > {{R}}_{9} > {{R}}_{1} > {{R}}_{4} > {{R}}_{6} |
{\kappa} =2 | {{R}}_{8} > {{R}}_{3} > {{R}}_{1} > {{R}}_{7} > {{R}}_{6} > {{R}}_{2} > {{R}}_{5} > {{R}}_{4} > {{R}}_{9} |
{\kappa} =3 | {{R}}_{8} > {{R}}_{3} > {{R}}_{1} > {{R}}_{2} > {{R}}_{7} > {{R}}_{6} > {{R}}_{5} > {{R}}_{4} > {{R}}_{9} |
{\kappa} =4 | {{R}}_{8} > {{R}}_{1} > {{R}}_{2} > {{R}}_{3} > {{R}}_{6} > {{R}}_{4} > {{R}}_{7} > {{R}}_{5} > {{R}}_{9} |
To investigate the effects of the parameter {\kappa} on arc welding robot selection and decision outcomes in depth. Tables 20 and 21 ( q = 4) show the average solutions obtained after adjusting the values of {\kappa} in both the 2TL q -ROFWHM and 2TL q -ROFWDHM operators. The values of average solutions differ when the parameter {\kappa} in the 2TL q -ROFWDHM operator is changed, as shown in Tables 20 and 21, although the ranking orders are essentially the same in most cases shown in Tables 22 and 23. Effect of variation of q and {\kappa} on the 2TL q -ROFWDHM is shown in Figures 4 and 5, respectively. When the parameters based on the 2TL q -ROFWHM and 2TL q -ROFWDHM operators are changed, both the score values and alternative ranking change, indicating that the parameter \kappa influences the arc welding robot selection assessment process.
Parameter | £_{1} | £_{2} | £_{3} | £_{4} | £_{5} | £_{6} | £_{7} | £_{8} | £_{9} |
q=1 | 0.1530 | -3.1792 | 7.1507 | -12.0965 | 5.5735 | -1.8540 | 8.0698 | 3.1966 | -7.0140 |
q=2 | 0.1057 | -2.9132 | 7.8509 | -11.0315 | 4.3727 | -2.5697 | 8.5268 | 3.7767 | -8.1183 |
q=3 | 1.0370 | -2.8208 | 7.4580 | -10.1699 | 3.4083 | -2.4021 | 8.1027 | 4.3676 | -8.9809 |
q=4 | 2.0292 | -2.8761 | 6.8321 | -9.6699 | 2.5850 | -1.9598 | 7.6085 | 5.0272 | -9.5762 |
q=5 | 2.6374 | -2.9743 | 6.9033 | -9.3430 | 2.0591 | -1.4359 | 7.1377 | 4.9781 | -9.9625 |
q=6 | 3.1882 | -3.1053 | 6.7752 | -9.1052 | 1.5023 | -0.8554 | 6.6762 | 5.1364 | -10.2125 |
q=7 | 3.9542 | -3.2434 | 6.3435 | -8.9285 | 1.0057 | -0.2839 | 6.2217 | 5.2570 | -10.3264 |
q=8 | 4.2588 | -3.3906 | 6.2811 | -8.8002 | 0.5206 | 0.2603 | 5.7110 | 5.4004 | -10.2414 |
Parameter | £_{1} | £_{2} | £_{3} | £_{4} | £_{5} | £_{6} | £_{7} | £_{8} | £_{9} |
{\kappa}=1 | 3.4005 | 2.5863 | 1.4628 | -5.4874 | -8.1530 | 2.2088 | -0.6670 | 12.0452 | -7.3961 |
{\kappa}=2 | 3.7906 | -0.9149 | 11.8515 | -14.4767 | 1.8085 | -0.3653 | 6.5394 | 9.4213 | -17.6544 |
{\kappa}=3 | 0.5583 | -2.8586 | 7.6750 | -10.5288 | 3.8543 | -2.5370 | 8.3456 | 4.0695 | -8.5783 |
{\kappa}=4 | -2.6107 | -3.5564 | 1.8935 | -13.2726 | 12.1627 | -7.5384 | 11.5950 | 0.5158 | 0.8112 |
Parameter | Ranking |
q=1 | {{R}}_{7} > {{R}}_{3} > {{R}}_{5} > {{R}}_{8} > {{R}}_{1} > {{R}}_{6} > {{R}}_{2} > {{R}}_{9} > {{R}}_{4} |
q=2 | {{R}}_{7} > {{R}}_{3} > {{R}}_{5} > {{R}}_{8} > {{R}}_{1} > {{R}}_{6} > {{R}}_{2} > {{R}}_{9} > {{R}}_{4} |
q=3 | {{R}}_{7} > {{R}}_{3} > {{R}}_{8} > {{R}}_{5} > {{R}}_{1} > {{R}}_{6} > {{R}}_{2} > {{R}}_{9} > {{R}}_{4} |
q=4 | {{R}}_{7} > {{R}}_{3} > {{R}}_{8} > {{R}}_{5} > {{R}}_{1} > {{R}}_{6} > {{R}}_{2} > {{R}}_{9} > {{R}}_{4} |
q=5 | {{R}}_{7} > {{R}}_{3} > {{R}}_{8} > {{R}}_{1} > {{R}}_{5} > {{R}}_{6} > {{R}}_{2} > {{R}}_{4} > {{R}}_{9} |
q=6 | {{R}}_{3} > {{R}}_{7} > {{R}}_{8} > {{R}}_{1} > {{R}}_{5} > {{R}}_{6} > {{R}}_{2} > {{R}}_{4} > {{R}}_{9} |
q=7 | {{R}}_{3} > {{R}}_{7} > {{R}}_{8} > {{R}}_{1} > {{R}}_{5} > {{R}}_{6} > {{R}}_{2} > {{R}}_{4} > {{R}}_{9} |
q=8 | {{R}}_{3} > {{R}}_{7} > {{R}}_{8} > {{R}}_{1} > {{R}}_{5} > {{R}}_{6} > {{R}}_{2} > {{R}}_{4} > {{R}}_{9} |
Parameter | Ranking |
{\kappa} =1 | {{R}}_{8} > {{R}}_{1} > {{R}}_{2} > {{R}}_{6} > {{R}}_{3} > {{R}}_{7} > {{R}}_{4} > {{R}}_{9} > {{R}}_{5} |
{\kappa} =2 | {{R}}_{3} > {{R}}_{8} > {{R}}_{7} > {{R}}_{1} > {{R}}_{5} > {{R}}_{6} > {{R}}_{2} > {{R}}_{4} > {{R}}_{9} |
{\kappa} =3 | {{R}}_{7} > {{R}}_{3} > {{R}}_{8} > {{R}}_{5} > {{R}}_{1} > {{R}}_{6} > {{R}}_{2} > {{R}}_{9} > {{R}}_{4} |
{\kappa} =4 | {{R}}_{5} > {{R}}_{7} > {{R}}_{3} > {{R}}_{9} > {{R}}_{8} > {{R}}_{1} > {{R}}_{2} > {{R}}_{6} > {{R}}_{4} |
In this subsection, we use certain validated approaches to cope with the proposed MAGDM problem and analyze the outcomes with our developed framework to check its feasibility and effectiveness. We carefully compute the evaluation outcomes for the selection of arc welding robots utilizing these strategies. Tables 24 and 25 illustrated by Figures 6 and 7, respectively, summarize the output of the comparisons among the developed CODAS method and existing EDAS and TOPSIS methods.
Alternatives | EDAS | Ranking | CODAS | Ranking | TOPSIS | Ranking |
{{R}}_{1} | 0.6255 | III | 8.6007 | III | 0.6850 | III |
{{R}}_{2} | 0.7340 | II | 0.6820 | IV | 0.6278 | IV |
{{R}}_{3} | 0.5452 | VI | 9.3537 | II | 0.7280 | II |
{{R}}_{4} | 0.3315 | VIII | -6.3231 | VIII | 0.5059 | VIII |
{{R}}_{5} | 0.4210 | VII | -5.0894 | VII | 0.5399 | VII |
{{R}}_{6} | 0.5998 | IV | -3.0861 | VI | 0.5812 | VI |
{{R}}_{7} | 0.5728 | V | 0.4563 | V | 0.6136 | V |
{{R}}_{8} | 0.8143 | I | 11.8046 | I | 0.7400 | I |
{{R}}_{9} | 0.1015 | IX | -16.3987 | IX | 0.4010 | IX |
Alternatives | EDAS | Ranking | CODAS | Ranking | TOPSIS | Ranking |
{{R}}_{1} | 0.4897 | VI | 0.5583 | V | 0.3909 | V |
{{R}}_{2} | 0.2840 | VII | -2.8586 | VII | 0.3490 | VII |
{{R}}_{3} | 0.8716 | I | 7.6750 | II | 0.4678 | II |
{{R}}_{4} | 0.2296 | IX | -10.5288 | IX | 0.2593 | IX |
{{R}}_{5} | 0.6853 | II | 3.8543 | IV | 0.4197 | IV |
{{R}}_{6} | 0.2521 | VIII | -2.5370 | VI | 0.3634 | VI |
{{R}}_{7} | 0.5000 | IV | 8.3456 | I | 0.4727 | I |
{{R}}_{8} | 0.5000 | III | 4.0695 | III | 0.4274 | III |
{{R}}_{9} | 0.5000 | V | -8.5783 | VIII | 0.2784 | VIII |
The CODAS ranking method is very useful and efficient when dealing with complex MAGDM difficulties. Some experts use it to evaluate a handful of alternatives by using various properties. In this paper, we have proposed AO and extended the CODAS method to MAGDM with 2TL q -ROFS, based on two different types of distance measurements. The main advantage of the proposed technique compared to techniques already in use is that it not only addresses 2TL q -ROFS, but also has a strong ability to identify the best alternatives. We have developed 2TL q -ROFS as a new advance in FS theory for conveying data complexity. 2TL q -ROFS has involved the integration of 2TL terms and q -ROF sets, increasing the adaptability of q -ROFS. Inspired by traditional AO, we have proposed two aggregations (2TL q -ROFHM and 2TL q -ROFWHM operators) to aggregate 2TL q -ROFS, and further have explored their basic features. We have devised a technique called the maximizing deviation method to discover ideal relative weights for attributes in linguistic contexts, with the premise that attributes with larger deviation values among the alternatives should be considered to have larger weights. The distinctive feature of this development is that it can reduce the influence of the subjectivity of decision makers and make full use of decision information. Furthermore, the CODAS method is extended to solve the MAGDM challenge using 2TL q -ROFS, which can fully consider both ED and HD. Finally, a practical example is given to demonstrate the suggested method for evaluating and selecting an arc welding robot. We have also examined the influence of different parameters on the selection of the arc welding robot. The proposed method is also compared with the EDAS and TOPSIS methods to demonstrate their advantages and efficacy. The four main contributions of this study are as follows: (1) The development of 2TL q -ROFS; (2) the extension of the classical CODAS method to 2TL q -ROFS; (3) the CODAS of the 2TL q -ROFS MAGDM problem method design to provide DM with an effective way to solve MAGDM problems; and (4) present a case study on the evaluation and selection of arc welding robots to demonstrate the applicability, feasibility and effectiveness of the proposed MAGDM method. We will continue to extend our proposed model to other ambiguous cases and application domains for the next study.
The fourth author extends his appreciation to the Deanship of Scientific Research at King Khalid University for funding this work through the General Research Project under grant number (R.G.P.2/48/43).
The authors declare no conflicts of interest.
[1] |
S. B. Zhu, J. Zhou, J. H. Lü, J. A. Lu, Finite-time synchronization of impulsive dynamical networks with strong nonlinearity, IEEE Trans. Autom. Control, 66 (2021), 3550–3561. https://doi.org/10.1109/TAC.2020.3022532 doi: 10.1109/TAC.2020.3022532
![]() |
[2] |
A. L. Li, X. L. Ye, Finite-time anti-synchronization for delayed inertial neural networks via the fractional and polynomial controllers of time variable, AIMS Math., 6 (2021), 8173–8190. https://doi.org/10.3934/math.2021473 doi: 10.3934/math.2021473
![]() |
[3] |
T. H. Yu, J. D. Cao, L. Rutkowski, Y. P. Luo, Finite-time synchronization of complex-valued memristive-based neural networks via hybrid control, IEEE Trans. Neural Netw. Learn. Syst., 33 (2022), 3938–3947. https://doi.org/10.1109/TNNLS.2021.3054967 doi: 10.1109/TNNLS.2021.3054967
![]() |
[4] |
E. G. Tian, Y. Zou, H. T. Chen, Finite-time synchronization of complex networks with intermittent couplings and neutral-type delays, IEEE/CAA J. Autom. Sinica, 10 (2023), 2026–2028. https://doi.org/10.1109/JAS.2023.123171 doi: 10.1109/JAS.2023.123171
![]() |
[5] |
Y. H. Xu, X. Q. Wu, B. Mao, J. H. Lü, C. R. Xie, Finite-time intra-layer and inter-layer quasi-synchronization of two-layer multi-weighted networks, IEEE Trans. Circuits Syst. I, 68 (2021), 1589–1598. https://doi.org/10.1109/TCSI.2021.3050988 doi: 10.1109/TCSI.2021.3050988
![]() |
[6] |
C. J. Song, J. Zhou, J. S. Wang, Finite time inter-layer synchronization of duplex networks via event-dependent intermittent control, IEEE Trans. Circuits Syst. II, 69 (2022), 4889–4893. https://doi.org/10.1109/TCSII.2022.3187269 doi: 10.1109/TCSII.2022.3187269
![]() |
[7] |
T. Liang, W. L. Zhang, D. G. Yang, Fixed-time synchronization of switched duplex networks with stochastic disturbances and limited communication, Commun. Nonlinear Sci. Numer. Simul., 121 (2023), 107227. https://doi.org/10.1016/j.cnsns.2023.107227 doi: 10.1016/j.cnsns.2023.107227
![]() |
[8] |
X. F. Wu, H. B. Bao, J. D. Cao, Fixed-time synchronization of multiplex networks by sliding mode control, J. Frankl. Inst., 360 (2023), 5504–5523. https://doi.org/10.1016/j.jfranklin.2023.03.057 doi: 10.1016/j.jfranklin.2023.03.057
![]() |
[9] |
X. S. Yang, Q. X. Zhu, C. X. Huang, Generalized lag-synchronization of chaotic mix-delayed systems with uncertain parameters and unknown perturbations, Nonlinear Anal.-Real, 12 (2011), 93–105. https://doi.org/10.1016/j.nonrwa.2010.05.037 doi: 10.1016/j.nonrwa.2010.05.037
![]() |
[10] |
M. W. Zheng, Z. M. Wang, L. X. Li, H. P. Peng, J. H. Xiao, Y. X. Yang, et al., Finite-time generalized projective lag synchronization criteria for neutral-type neural networks with delay, Chaos Soliton. Fract., 107 (2018), 195–203. https://doi.org/10.1016/j.chaos.2018.01.009 doi: 10.1016/j.chaos.2018.01.009
![]() |
[11] |
Y. B. Wu, C. D. Wang, W. X. Li, Generalized quantized intermittent control with adaptive strategy on finite-time synchronization of delayed coupled systems and applications, Nonlinear Dyn., 95 (2019), 1361–1377. https://doi.org/10.1007/s11071-018-4633-z doi: 10.1007/s11071-018-4633-z
![]() |
[12] |
P. Anand, B. B. Sharma, Generalized finite-time synchronization scheme for a class of nonlinear systems using backstepping like control strategy, Int. J. Dynam. Control, 11 (2023), 258–270. https://doi.org/10.1007/s40435-022-00948-y doi: 10.1007/s40435-022-00948-y
![]() |
[13] |
D. S. Xu, Y. Liu, M. Liu, Finite-time synchronization of multi-coupling stochastic fuzzy neural networks with mixed delays via feedback control, Fuzzy Set. Syst., 411 (2021), 85–104. https://doi.org/10.1016/j.fss.2020.07.015 doi: 10.1016/j.fss.2020.07.015
![]() |
[14] |
H. W. Ren, Z. P. Peng, Y. Gu, Fixed-time synchronization of stochastic memristor-based neural networks with adaptive control, Neural Networks, 130 (2020), 165–175. https://doi.org/10.1016/j.neunet.2020.07.002 doi: 10.1016/j.neunet.2020.07.002
![]() |
[15] |
X. Wang, N. Pang, Y. W. Xu, T. W. Huang, J. Kurths, On state-constrained containment control for nonlinear multiagent systems using event-triggered input, IEEE Trans. Syst. Man Cybern.: Syst., 54 (2024), 2530–2538. https://doi.org/10.1109/TSMC.2023.3345365 doi: 10.1109/TSMC.2023.3345365
![]() |
[16] |
T. Y. Jing, D. Y. Zhang, T. L. Jing, Finite-time synchronization of hybrid-coupled delayed dynamic networks via aperiodically intermittent control, Neural Process. Lett., 52 (2020), 291–311. https://doi.org/10.1007/s11063-020-10245-4 doi: 10.1007/s11063-020-10245-4
![]() |
[17] |
Y. B. Wu, Y. X. Gao, W. X. Li, Finite-time synchronization of switched neural networks with state-dependent switching via intermittent control, Neurocomputing, 384 (2020), 325–334. https://doi.org/10.1016/j.neucom.2019.12.031 doi: 10.1016/j.neucom.2019.12.031
![]() |
[18] |
X. L. Xiong, X. S. Yang, J. D. Cao, R. Q. Tang, Finite-time control for a class of hybrid systems via quantized intermittent control, Sci. China Inf. Sci., 63 (2020), 192201. https://doi.org/10.1007/s11432-018-2727-5 doi: 10.1007/s11432-018-2727-5
![]() |
[19] |
Y. Ren, H. J. Jiang, J. R. Li, B. L. Lu, Finite-time synchronization of stochastic complex networks with random coupling delay via quantized aperiodically intermittent control, Neurocomputing, 420 (2021), 337–348. https://doi.org/10.1016/j.neucom.2020.05.103 doi: 10.1016/j.neucom.2020.05.103
![]() |
[20] |
R. Q. Tang, H. S. Su, Y. Zou, X. S. Yang, Finite-time synchronization of Markovian coupled neural networks with delays via intermittent quantized control: linear programming approach, IEEE Trans. Neural Netw. Learn. Syst., 33 (2022), 5268–5278. https://doi.org/10.1109/TNNLS.2021.3069926 doi: 10.1109/TNNLS.2021.3069926
![]() |
[21] |
C. Xu, X. S. Yang, J. Q. Lu, J. W. Feng, F. E. Alsaadi, T. Hayat, Finite-time synchronization of networks via quantized intermittent pinning control, IEEE Trans. Cybern., 48 (2018), 3021–3027. https://doi.org/10.1109/TCYB.2017.2749248 doi: 10.1109/TCYB.2017.2749248
![]() |
[22] | S. Boyd, L. El Ghaoui, E. Feron, V. Balakrishnan, Linear matrix inequalities in system and control theory, SIAM, 1994. https://doi.org/10.1137/1.9781611970777.bm |
[23] |
Z. Y. Zuo, Nonsingular fixed-time consensus tracking for second-order multi-agent networks, Automatica, 54 (2015), 305–309. https://doi.org/10.1016/j.automatica.2015.01.021 doi: 10.1016/j.automatica.2015.01.021
![]() |
[24] |
J. Liu, G. T. Ran, Y. B. Wu, L. Xue, C. Y. Sun, Dynamic event-triggered practical fixed-time consensus for nonlinear multiagent systems, IEEE Trans. Circuits Syst. II, 69 (2022), 2156–2160. https://doi.org/10.1109/TCSII.2021.3128624 doi: 10.1109/TCSII.2021.3128624
![]() |
[25] |
J. Mei, M. H. Jiang, W. M. Xu, B. Wang, Finite-time synchronization control of complex dynamical networks with time delay, Commun. Nonlinear Sci. Numer. Simul., 18 (2013), 2462–2478. https://doi.org/10.1016/j.cnsns.2012.11.009 doi: 10.1016/j.cnsns.2012.11.009
![]() |
[26] | F. H. Clarke, Optimization and nonsmooth analysis, Hoboken, NJ, USA: Wiley, 1983. |
[27] | L. Cao, W. L. Zhang, Practical finite-time synchronization of T-S fuzzy complex networks with different couplings via semi-intermittent control, Int. J. Fuzzy Syst., in press. https://doi.org/10.1007/s40815-024-01686-3 |
1. | Muhammad Akram, Sumera Naz, Gustavo Santos-García, Muhammad Ramzan Saeed, Extended CODAS method for MAGDM with 2 -tuple linguistic T -spherical fuzzy sets, 2023, 8, 2473-6988, 3428, 10.3934/math.2023176 | |
2. | Tao Li, Liyuan Zhang, Zhenglong Zhang, Incomplete linguistic q-rung orthopair fuzzy preference relations and their application to multi-criteria decision making, 2023, 2199-4536, 10.1007/s40747-022-00961-w | |
3. | Ayesha Khan, Uzma Ahmad, Adeel Farooq, Mohammed M. Ali Al-Shamiri, Combinative distance-based assessment method for decision-making with 2 -tuple linguistic q -rung picture fuzzy sets, 2023, 8, 2473-6988, 13830, 10.3934/math.2023708 | |
4. | Hassan Soltan, Khaled Janada, Mohamed Omar, FAQT-2: A Customer-Oriented Method for MCDM with Statistical Verification Applied to Industrial Robot Selection, 2023, 09574174, 120106, 10.1016/j.eswa.2023.120106 | |
5. | Sumera Naz, Aqsa Shafiq, Shariq Aziz Butt, Rabia Tasneem, Dragan Pamucar, Zhoe Comas Gonzalez, Decision-making model for selecting products through online product reviews utilizing natural language processing techniques, 2025, 611, 09252312, 128593, 10.1016/j.neucom.2024.128593 | |
6. | Jawad Ali, Muhammad Naeem, r, s, t-Spherical Fuzzy VIKOR Method and Its Application in Multiple Criteria Group Decision Making, 2023, 11, 2169-3536, 46454, 10.1109/ACCESS.2023.3271141 | |
7. | Sumera Naz, Aqsa Shafiq, Shariq Aziz Butt, Shahzra Mazhar, Diaz Jorge Martinez, Emiro De la Hoz Franco, Decision analysis with IDOCRIW-QUALIFLEX approach in the 2TLq-ROF environment: An application of accident prediction models in Pakistan, 2024, 10, 24058440, e27669, 10.1016/j.heliyon.2024.e27669 | |
8. | Sumera Naz, Syeda Saba Fatima, Shariq Aziz Butt, Nadia Tabassum, A MAGDM model based on 2-tuple linguistic variables and power Hamacher aggregation operators for optimal selection of digital marketing strategies, 2023, 8, 2364-4966, 1955, 10.1007/s41066-023-00408-8 | |
9. | Sumera Naz, Aqsa Tasawar, Areej Fatima, Shariq Aziz Butt, Zhoe Comas Gonzalez, An efficient 2-tuple linguistic cubic q-rung orthopair fuzzy CILOS-TOPSIS method: evaluating the hydrological geographical regions for watershed management in Pakistan, 2025, 81, 0920-8542, 10.1007/s11227-024-06505-y | |
10. | Sumera Naz, Muhammad Ramzan Saeed, Shariq Aziz Butt, Multi-attribute group decision-making based on 2-tuple linguistic cubic q-rung orthopair fuzzy DEMATEL analysis, 2024, 9, 2364-4966, 10.1007/s41066-023-00433-7 | |
11. | Maliha Rashid, Lariab Shahid, Fatima Dar, Irshad Ayoob, Nabil Mlaiki, Existence of solution of a system of non-linear differential inclusions with non-local, integral boundary conditions via fixed points of hybrid contractions, 2024, 2024, 1687-2770, 10.1186/s13661-024-01902-x | |
12. | Sumera Naz, Muhammad Akram, Muhammad Muneeb ul Hassan, Areej Fatima, A Hybrid DEMATEL-TOPSIS Approach Using 2-Tuple Linguistic q-Rung Orthopair Fuzzy Information and Its Application in Renewable Energy Resource Selection, 2024, 23, 0219-6220, 1099, 10.1142/S0219622023500323 | |
13. | Sumera Naz, Aqsa Shafiq, Shariq Aziz Butt, Rabia Ijaz, A new approach to sentiment analysis algorithms: Extended SWARA-MABAC method with 2-tuple linguistic q-rung orthopair fuzzy information, 2023, 126, 09521976, 106943, 10.1016/j.engappai.2023.106943 | |
14. | Sumera Naz, Aqsa Shafiq, Maheen Abbas, An approach for 2-tuple linguistic q-rung orthopair fuzzy MAGDM for the evaluation of historical sites with power Heronian mean, 2024, 80, 0920-8542, 6435, 10.1007/s11227-023-05678-2 | |
15. | Sumera Naz, Muhammad Akram, Aqsa Shafiq, Kiran Akhtar, Optimal airport selection utilizing power Muirhead mean based group decision model with 2-tuple linguistic q-rung orthopair fuzzy information, 2024, 15, 1868-8071, 303, 10.1007/s13042-023-01911-9 | |
16. | M.A. Alsalem, A.H. Alamoodi, O.S. Albahri, A.S. Albahri, Luis Martínez, R. Yera, Ali M. Duhaim, Iman Mohamad Sharaf, Evaluation of trustworthy artificial intelligent healthcare applications using multi-criteria decision-making approach, 2024, 246, 09574174, 123066, 10.1016/j.eswa.2023.123066 | |
17. | Sumera Naz, Shariq Aziz Butt, Muhammad Muneeb ul Hassan, José Escorcia-Gutierrez, Areej Fatima, Farhat ul Ain, A hybrid novel SWARA-ELECTRE-I method using probabilistic uncertain linguistic information for feature selection in image recognition, 2025, 612, 09252312, 128615, 10.1016/j.neucom.2024.128615 | |
18. | Sumera Naz, Muhammad Muneeb ul Hassan, Areej Fatima, Diaz Jorge Martinez, Elisa Ospino Mendoza, Shariq Aziz Butt, A decision-making mechanism for multi-attribute group decision-making using 2-tuple linguistic T-spherical fuzzy maximizing deviation method, 2023, 8, 2364-4966, 1659, 10.1007/s41066-023-00388-9 | |
19. | Abid Mahboob, Zafar Ullah, Ali Ovais, Muhammad Waheed Rasheed, S. A. Edalatpanah, Kainat Yasin, A MAGDM approach for evaluating the impact of artificial intelligence on education using 2-tuple linguistic q-rung orthopair fuzzy sets and Schweizer-Sklar weighted power average operator, 2024, 7, 2624-8212, 10.3389/frai.2024.1347626 | |
20. | Sumera Naz, Areej Fatima, Shariq Aziz But, Arooj Ashiq, 2024, Chapter 17, 978-981-97-6971-1, 353, 10.1007/978-981-97-6972-8_17 | |
21. | Pairote Yiarayong, Bipolar Linguistic q-Rung Orthopair Fuzzy Modeling for Sustainable Tourism Development and Decision-Making in Tourism Cities, 2024, 1562-2479, 10.1007/s40815-024-01886-x | |
22. | Sumera Naz, Muhammad Muneeb ul Hassan, Atif Mehmood, Gabriel Piñeres Espitia, Shariq Aziz Butt, Enhancing industrial robot selection through a hybrid novel approach: integrating CRITIC-VIKOR method with probabilistic uncertain linguistic q-rung orthopair fuzzy, 2024, 58, 1573-7462, 10.1007/s10462-024-11001-z | |
23. | Sumera Naz, Syeda Saba Fatima, Shariq Aziz Butt, Mehwish Majeed, Areej Fatima, 2025, Chapter 21, 978-3-031-78504-7, 403, 10.1007/978-3-031-78505-4_21 | |
24. | Anirban Tarafdar, Azharuddin Shaikh, Md Niamot Ali, Anupam Haldar, An integrated fuzzy decision-making framework for autonomous mobile robot selection: balancing subjective and objective measures with fuzzy TOPSIS and picture fuzzy CoCoSo approach, 2025, 0160-5682, 1, 10.1080/01605682.2025.2486705 |
Criterion | Explanation |
Weight density | This criterion takes into account the physical weight of the robot. In general, consumer chooses a lighter robot. The weight density is usually expressed in kg ({{G}}_{1}) . |
Replicability | This refers to a robot's ability to repeat a task over and over again. More replicability is often preferred. Replicability is usually measured in millimeters ({{G}}_{2}) . |
Freight capacity | The highest total weight a robot can lift in one turn is referred to as freight capacity. Being more is often preferred. The weight density is usually expressed in kg ({{G}}_{3}) . |
Maximum reach | This is the average of the maximum vertical and horizontal distances a robot's arm can extend to complete a task. It is common to want to be more. The robot's maximum reach is typically measured in millimeters ({{G}}_{4}) . |
Average power consumption | It refers to the robot's average power consumption in units of electricity. It is often desirable that a robot consumes less energy. The robot's power consumption is usually measured in kilowatts ({{G}}_{5}) . |
Motion of a robot | The motion of a robot at a reference point near the end effector's tip is referred to as robot motion. Trajectory, speed, acceleration, and acceleration derivative are commonly used to describe robot motion. The robot's motion is usually expressed in ms^{-1} or ms^{-2} \; ({{G}}_{6}) |
Sr. No. | Criteria | Units | Symbol |
1 | Weight density | Kg | {{G}}_{1} |
2 | Replicability | (+/-) mm | {{G}}_{2} |
3 | Freight capacity | Kg | {{G}}_{3} |
4 | Maximum Reach | mm | {{G}}_{4} |
5 | Average Power Consumption | KW | {{G}}_{5} |
6 | Motion of a robot | ms^{-2} | {{G}}_{6} |
Linguistic variables | 2TL q -ROFNs |
Certainly high value (CHV) | ((s_{8}, 0), (s_{0}, 0)) |
Very high value (VHV) | ((s_{7}, 0), (s_{1}, 0)) |
High value(HV) | ((s_{6}, 0), (s_{2}, 0)) |
Above average value (AAV) | ((s_{5}, 0), (s_{3}, 0)) |
Average vlaue (AV) | ((s_{4}, 0), (s_{4}, 0)) |
Under average value (UAV) | ((s_{3}, 0), (s_{5}, 0)) |
Low value (LV) | ((s_{2}, 0), (s_{6}, 0)) |
Very low value (VLV) | ((s_{1}, 0), (s_{7}, 0)) |
Certainly low value (CLV) | ((s_{0}, 0), (s_{8}, 0)) |
Experts | Alternatives | Attributes | |||||
{{G}}_{1} | {{G}}_{2} | {{G}}_{3} | {{G}}_{4} | {{G}}_{5} | {{G}}_{6} | ||
\Theta_{1} | |||||||
{{R}}_{1} | AV | LV | VHV | VLV | CLV | CHV | |
{{R}}_{2} | HV | UAV | CLV | AAV | VHV | LV | |
{{R}}_{3} | VHV | VLV | CHV | HV | UAV | CLV | |
{{R}}_{4} | CLV | AAV | LV | VHV | VLV | AAV | |
{{R}}_{5} | CHV | HV | UAV | CLV | LV | AV | |
{{R}}_{6} | LV | VHV | VLV | CHV | AV | HV | |
{{R}}_{7} | UAV | CLV | AAV | AV | HV | VLV | |
{{R}}_{8} | AAV | AV | HV | UAV | CHV | VHV | |
{{R}}_{9} | VLV | CHV | AV | LV | AAV | UAV | |
\Theta_{2} | |||||||
{{R}}_{1} | VLV | AV | HV | UAV | LV | CHV | |
{{R}}_{2} | LV | VHV | VLV | CHV | CLV | AAV | |
{{R}}_{3} | AV | HV | UAV | CLV | AAV | VHV | |
{{R}}_{4} | CLV | AAV | LV | VHV | VLV | UAV | |
{{R}}_{5} | VHV | VLV | CHV | HV | UAV | AV | |
{{R}}_{6} | HV | UAV | CLV | AAV | AV | LV | |
{{R}}_{7} | AAV | LV | VHV | AV | CHV | CLV | |
{{R}}_{8} | UAV | CHV | AV | LV | VHV | VLV | |
{{R}}_{9} | CHV | CLV | AAV | VLV | HV | AV | |
\Theta_{3} | |||||||
{{R}}_{1} | AV | UAV | LV | AAV | HV | VLV | |
{{R}}_{2} | LV | VHV | AV | HV | UAV | AAV | |
{{R}}_{3} | VHV | VLV | CHV | AV | LV | UAV | |
{{R}}_{4} | CHV | AV | UAV | VLV | AAV | HV | |
{{R}}_{5} | HV | CHV | VLV | UAV | CLV | VHV | |
{{R}}_{6} | AAV | LV | CLV | CHV | VLV | AV | |
{{R}}_{7} | CLV | AAV | HV | VHV | AV | CHV | |
{{R}}_{8} | VLV | HV | VHV | CLV | CHV | LV | |
{{R}}_{9} | UAV | CLV | AAV | LV | VHV | CLV | |
\Theta_{4} | |||||||
{{R}}_{1} | CHV | LV | UAV | AV | AAV | HV | |
{{R}}_{2} | LV | HV | CLV | UAV | CHV | AAV | |
{{R}}_{3} | UAV | VHV | CHV | HV | VLV | CHV | |
{{R}}_{4} | AV | AAV | VLV | CLV | HV | LV | |
{{R}}_{5} | VLV | CLV | AV | LV | VHV | UAV | |
{{R}}_{6} | AAV | CHV | LV | VLV | CLV | AV | |
{{R}}_{7} | VHV | VLV | AAV | CHV | AV | VHV | |
{{R}}_{8} | HV | AV | VHV | AAV | UAV | VLV | |
{{R}}_{9} | CLV | UAV | HV | VHV | LV | CLV |
Experts | Alternatives | Attributes | |||||
{{G}}_{1} | {{G}}_{2} | {{G}}_{3} | {{G}}_{4} | {{G}}_{5} | {{G}}_{6} | ||
\Theta_{1} | |||||||
{{R}}_{1} | ((s_{4}, 0), (s_{4}, 0)) | ((s_{2}, 0), (s_{6}, 0)) | ((s_{7}, 0), (s_{1}, 0)) | ((s_{1}, 0), (s_{7}, 0)) | ((s_{0}, 0), (s_{8}, 0)) | ((s_{8}, 0), (s_{0}, 0)) | |
{{R}}_{2} | ((s_{6}, 0), (s_{2}, 0)) | ((s_{3}, 0), (s_{5}, 0)) | ((s_{0}, 0), (s_{8}, 0)) | ((s_{5}, 0), (s_{3}, 0)) | ((s_{7}, 0), (s_{1}, 0)) | ((s_{2}, 0), (s_{6}, 0)) | |
{{R}}_{3} | ((s_{7}, 0), (s_{1}, 0)) | ((s_{1}, 0), (s_{7}, 0)) | ((s_{8}, 0), (s_{0}, 0)) | ((s_{6}, 0), (s_{2}, 0)) | ((s_{3}, 0), (s_{5}, 0)) | ((s_{0}, 0), (s_{8}, 0)) | |
{{R}}_{4} | ((s_{0}, 0), (s_{8}, 0)) | ((s_{5}, 0), (s_{3}, 0)) | ((s_{2}, 0), (s_{6}, 0)) | ((s_{7}, 0), (s_{1}, 0)) | ((s_{1}, 0), (s_{7}, 0)) | ((s_{5}, 0), (s_{3}, 0)) | |
{{R}}_{5} | ((s_{8}, 0), (s_{0}, 0)) | ((s_{6}, 0), (s_{2}, 0)) | ((s_{3}, 0), (s_{5}, 0)) | ((s_{0}, 0), (s_{8}, 0)) | ((s_{2}, 0), (s_{6}, 0)) | ((s_{4}, 0), (s_{4}, 0)) | |
{{R}}_{6} | ((s_{2}, 0), (s_{6}, 0)) | ((s_{7}, 0), (s_{1}, 0)) | ((s_{1}, 0), (s_{7}, 0)) | ((s_{8}, 0), (s_{0}, 0)) | ((s_{4}, 0), (s_{4}, 0)) | ((s_{6}, 0), (s_{2}, 0)) | |
{{R}}_{7} | ((s_{3}, 0), (s_{5}, 0)) | ((s_{0}, 0), (s_{8}, 0)) | ((s_{5}, 0), (s_{3}, 0)) | ((s_{4}, 0), (s_{4}, 0)) | ((s_{6}, 0), (s_{2}, 0)) | ((s_{1}, 0), (s_{7}, 0)) | |
{{R}}_{8} | ((s_{5}, 0), (s_{3}, 0)) | ((s_{4}, 0), (s_{4}, 0)) | ((s_{6}, 0), (s_{2}, 0)) | ((s_{3}, 0), (s_{5}, 0)) | ((s_{8}, 0), (s_{0}, 0)) | ((s_{7}, 0), (s_{1}, 0)) | |
{{R}}_{9} | ((s_{1}, 0), (s_{7}, 0)) | ((s_{8}, 0), (s_{0}, 0)) | ((s_{4}, 0), (s_{4}, 0)) | ((s_{2}, 0), (s_{6}, 0)) | ((s_{5}, 0), (s_{3}, 0)) | ((s_{3}, 0), (s_{5}, 0)) | |
\Theta_{2} | |||||||
{{R}}_{1} | ((s_{1}, 0), (s_{7}, 0)) | ((s_{4}, 0), (s_{4}, 0)) | ((s_{6}, 0), (s_{2}, 0)) | ((s_{3}, 0), (s_{5}, 0)) | ((s_{2}, 0), (s_{6}, 0)) | ((s_{8}, 0), (s_{0}, 0)) | |
{{R}}_{2} | ((s_{2}, 0), (s_{6}, 0)) | ((s_{7}, 0), (s_{1}, 0)) | ((s_{1}, 0), (s_{7}, 0)) | ((s_{8}, 0), (s_{0}, 0)) | ((s_{0}, 0), (s_{8}, 0)) | ((s_{5}, 0), (s_{3}, 0)) | |
{{R}}_{3} | ((s_{4}, 0), (s_{4}, 0)) | ((s_{6}, 0), (s_{2}, 0)) | ((s_{3}, 0), (s_{5}, 0)) | ((s_{0}, 0), (s_{8}, 0)) | ((s_{5}, 0), (s_{3}, 0)) | ((s_{7}, 0), (s_{1}, 0)) | |
{{R}}_{4} | ((s_{0}, 0), (s_{8}, 0)) | ((s_{5}, 0), (s_{3}, 0)) | ((s_{2}, 0), (s_{6}, 0)) | ((s_{7}, 0), (s_{1}, 0)) | ((s_{1}, 0), (s_{7}, 0)) | ((s_{3}, 0), (s_{5}, 0)) | |
{{R}}_{5} | ((s_{7}, 0), (s_{1}, 0)) | ((s_{1}, 0), (s_{7}, 0)) | ((s_{8}, 0), (s_{0}, 0)) | ((s_{6}, 0), (s_{2}, 0)) | ((s_{3}, 0), (s_{5}, 0)) | ((s_{4}, 0), (s_{4}, 0)) | |
{{R}}_{6} | ((s_{6}, 0), (s_{2}, 0)) | ((s_{3}, 0), (s_{5}, 0)) | ((s_{0}, 0), (s_{8}, 0)) | ((s_{5}, 0), (s_{3}, 0)) | ((s_{4}, 0), (s_{4}, 0)) | ((s_{2}, 0), (s_{6}, 0)) | |
{{R}}_{7} | ((s_{5}, 0), (s_{3}, 0)) | ((s_{2}, 0), (s_{6}, 0)) | ((s_{7}, 0), (s_{1}, 0)) | ((s_{4}, 0), (s_{4}, 0)) | ((s_{8}, 0), (s_{0}, 0)) | ((s_{0}, 0), (s_{8}, 0)) | |
{{R}}_{8} | ((s_{3}, 0), (s_{5}, 0)) | ((s_{8}, 0), (s_{0}, 0)) | ((s_{4}, 0), (s_{4}, 0)) | ((s_{2}, 0), (s_{6}, 0)) | ((s_{7}, 0), (s_{1}, 0)) | ((s_{1}, 0), (s_{7}, 0)) | |
{{R}}_{9} | ((s_{8}, 0), (s_{0}, 0)) | ((s_{0}, 0), (s_{8}, 0)) | ((s_{5}, 0), (s_{3}, 0)) | ((s_{1}, 0), (s_{7}, 0)) | ((s_{6}, 0), (s_{2}, 0)) | ((s_{4}, 0), (s_{4}, 0)) | |
\Theta_{3} | |||||||
{{R}}_{1} | ((s_{4}, 0), (s_{4}, 0)) | ((s_{3}, 0), (s_{5}, 0)) | ((s_{2}, 0), (s_{6}, 0)) | ((s_{5}, 0), (s_{3}, 0)) | ((s_{6}, 0), (s_{2}, 0)) | ((s_{1}, 0), (s_{7}, 0)) | |
{{R}}_{2} | ((s_{2}, 0), (s_{6}, 0)) | ((s_{7}, 0), (s_{1}, 0)) | ((s_{4}, 0), (s_{4}, 0)) | ((s_{6}, 0), (s_{2}, 0)) | ((s_{3}, 0), (s_{5}, 0)) | ((s_{5}, 0), (s_{3}, 0)) | |
{{R}}_{3} | ((s_{7}, 0), (s_{1}, 0)) | ((s_{1}, 0), (s_{7}, 0)) | ((s_{8}, 0), (s_{0}, 0)) | ((s_{4}, 0), (s_{4}, 0)) | ((s_{2}, 0), (s_{6}, 0)) | ((s_{3}, 0), (s_{5}, 0)) | |
{{R}}_{4} | ((s_{8}, 0), (s_{0}, 0)) | ((s_{4}, 0), (s_{4}, 0)) | ((s_{3}, 0), (s_{5}, 0)) | ((s_{1}, 0), (s_{7}, 0)) | ((s_{5}, 0), (s_{3}, 0)) | ((s_{6}, 0), (s_{2}, 0)) | |
{{R}}_{5} | ((s_{6}, 0), (s_{2}, 0)) | ((s_{8}, 0), (s_{0}, 0)) | ((s_{1}, 0), (s_{7}, 0)) | ((s_{3}, 0), (s_{5}, 0)) | ((s_{0}, 0), (s_{8}, 0)) | ((s_{7}, 0), (s_{1}, 0)) | |
{{R}}_{6} | ((s_{5}, 0), (s_{3}, 0)) | ((s_{2}, 0), (s_{6}, 0)) | ((s_{0}, 0), (s_{8}, 0)) | ((s_{8}, 0), (s_{0}, 0)) | ((s_{1}, 0), (s_{7}, 0)) | ((s_{4}, 0), (s_{4}, 0)) | |
{{R}}_{7} | ((s_{0}, 0), (s_{8}, 0)) | ((s_{5}, 0), (s_{3}, 0)) | ((s_{6}, 0), (s_{2}, 0)) | ((s_{7}, 0), (s_{1}, 0)) | ((s_{4}, 0), (s_{4}, 0)) | ((s_{8}, 0), (s_{0}, 0)) | |
{{R}}_{8} | ((s_{1}, 0), (s_{7}, 0)) | ((s_{6}, 0), (s_{2}, 0)) | ((s_{7}, 0), (s_{1}, 0)) | ((s_{0}, 0), (s_{8}, 0)) | ((s_{8}, 0), (s_{0}, 0)) | ((s_{2}, 0), (s_{6}, 0)) | |
{{R}}_{9} | ((s_{3}, 0), (s_{5}, 0)) | ((s_{0}, 0), (s_{8}, 0)) | ((s_{5}, 0), (s_{3}, 0)) | ((s_{2}, 0), (s_{6}, 0)) | ((s_{7}, 0), (s_{1}, 0)) | ((s_{0}, 0), (s_{8}, 0)) | |
\Theta_{4} | |||||||
{{R}}_{1} | ((s_{8}, 0), (s_{0}, 0)) | ((s_{2}, 0), (s_{6}, 0)) | ((s_{3}, 0), (s_{5}, 0)) | ((s_{4}, 0), (s_{4}, 0)) | ((s_{5}, 0), (s_{3}, 0)) | ((s_{6}, 0), (s_{2}, 0)) | |
{{R}}_{2} | ((s_{2}, 0), (s_{6}, 0)) | ((s_{6}, 0), (s_{2}, 0)) | ((s_{0}, 0), (s_{8}, 0)) | ((s_{3}, 0), (s_{5}, 0)) | ((s_{8}, 0), (s_{0}, 0)) | ((s_{5}, 0), (s_{3}, 0)) | |
{{R}}_{3} | ((s_{3}, 0), (s_{5}, 0)) | ((s_{7}, 0), (s_{1}, 0)) | ((s_{8}, 0), (s_{0}, 0)) | ((s_{6}, 0), (s_{2}, 0)) | ((s_{1}, 0), (s_{7}, 0)) | ((s_{8}, 0), (s_{0}, 0)) | |
{{R}}_{4} | ((s_{4}, 0), (s_{4}, 0)) | ((s_{5}, 0), (s_{3}, 0)) | ((s_{1}, 0), (s_{7}, 0)) | ((s_{0}, 0), (s_{8}, 0)) | ((s_{6}, 0), (s_{2}, 0)) | ((s_{2}, 0), (s_{6}, 0)) | |
{{R}}_{5} | ((s_{1}, 0), (s_{7}, 0)) | ((s_{0}, 0), (s_{8}, 0)) | ((s_{4}, 0), (s_{4}, 0)) | ((s_{2}, 0), (s_{6}, 0)) | ((s_{7}, 0), (s_{1}, 0)) | ((s_{3}, 0), (s_{5}, 0)) | |
{{R}}_{6} | ((s_{5}, 0), (s_{3}, 0)) | ((s_{8}, 0), (s_{0}, 0)) | ((s_{2}, 0), (s_{6}, 0)) | ((s_{1}, 0), (s_{7}, 0)) | ((s_{0}, 0), (s_{8}, 0)) | ((s_{4}, 0), (s_{4}, 0)) | |
{{R}}_{7} | ((s_{7}, 0), (s_{1}, 0)) | ((s_{1}, 0), (s_{7}, 0)) | ((s_{5}, 0), (s_{3}, 0)) | ((s_{8}, 0), (s_{0}, 0)) | ((s_{4}, 0), (s_{4}, 0)) | ((s_{7}, 0), (s_{1}, 0)) | |
{{R}}_{8} | ((s_{6}, 0), (s_{2}, 0)) | ((s_{4}, 0), (s_{4}, 0)) | ((s_{7}, 0), (s_{1}, 0)) | ((s_{5}, 0), (s_{3}, 0)) | ((s_{3}, 0), (s_{5}, 0)) | ((s_{1}, 0), (s_{7}, 0)) | |
{{R}}_{9} | ((s_{0}, 0), (s_{8}, 0)) | ((s_{3}, 0), (s_{5}, 0)) | ((s_{6}, 0), (s_{2}, 0)) | ((s_{7}, 0), (s_{1}, 0)) | ((s_{2}, 0), (s_{6}, 0)) | ((s_{0}, 0), (s_{8}, 0)) |
{{G}}_{1} | {{G}}_{2} | {{G}}_{3} | |
{{R}}_{1} | ((s_7, -0.3891), (s_4, -0.2277)) | ((s_6, 0.0997), (s_4, -0.0756)) | ((s_7, -0.1965), (s_3, 0.2730)) |
{{R}}_{2} | ((s_6, 0.0081), (s_4, 0.1537)) | ((s_7, 0.3642), (s_2, 0.1177)) | ((s_0, 0), (s_8, 0)) |
{{R}}_{3} | ((s_7, -0.0292), (s_3, -0.1356)) | ((s_6, 0.4762), (s_4, 0.1480)) | ((s_8, 0), (s_0, 0)) |
{{R}}_{4} | ((s_0, 0), (s_8, 0)) | ((s_7, 0.0485), (s_2, 0.2979)) | ((s_5, 0.4993), (s_5, -0.3450)) |
{{R}}_{5} | ((s_7, 0.0101), (s_3, 0.0473)) | ((s_5, -0.3156), (s_7, -0.1305)) | ((s_7, -0.3557), (s_4, -0.3383)) |
{{R}}_{6} | ((s_7, -0.0726), (s_3, -0.1504)) | ((s_7, 0.0101), (s_3, 0.2390)) | ((s_0, 0), (s_8, 0)) |
{{R}}_{7} | ((s_5, 0.3996), (s_6, 0.0833)) | ((s_4, -0.1232), (s_7, 0.0370)) | ((s_7, 0.3708), (s_2, -0.1898)) |
{{R}}_{8} | ((s_7, -0.4816), (s_4, -0.3182)) | ((s_7, 0.2618), (s_2, 0.3874)) | ((s_7, 0.3997), (s_2, -0.1125)) |
{{R}}_{9} | ((s_5, -0.0390), (s_7, -0.2683)) | ((s_0, 0), (s_8, 0)) | ((s_7, 0.1479), (s_2, 0.1867)) |
{{G}}_{4} | {{G}}_{5} | {{G}}_{6} | |
{{R}}_{1} | ((s_6, 0.2466), (s_4, -0.1424)) | ((s_5, -0.1877), (s_7, -0.4491)) | ((s_7, 0.2954), (s_3, -0.1998)) |
{{R}}_{2} | ((s_7, 0.2201), (s_3, -0.4669)) | ((s_6, -0.0280), (s_6, -0.0258)) | ((s_7, -0.1752), (s_3, -0.0620)) |
{{R}}_{3} | ((s_6, -0.2876), (s_6, -0.3069)) | ((s_6, -0.0466), (s_4, -0.2966)) | ((s_6, -0.0874), (s_6, -0.0255)) |
{{R}}_{4} | ((s_5, 0.2866), (s_7, -0.3760)) | ((s_6, 0.0168), (s_4, 0.4795)) | ((s_6, 0.4588), (s_4, -0.4168)) |
{{R}}_{5} | ((s_5, -0.3423), (s_7, -0.3634)) | ((s_5, -0.1427), (s_7, -0.4539)) | ((s_7, -0.2352), (s_3, -0.0154)) |
{{R}}_{6} | ((s_7, -0.1643), (s_3, 0.4871)) | ((s_5, -0.3171), (s_7, -0.2974)) | ((s_7, -0.4421), (s_3, 0.3799)) |
{{R}}_{7} | ((s_7, 0.3384), (s_2, 0.3524)) | ((s_7, 0.2766), (s_2, -0.3623)) | ((s_5, 0.2401), (s_7, -0.3311)) |
{{R}}_{8} | ((s_5, -0.4488), (s_7, -0.3605)) | ((s_7, 0.4941, (s_2, -0.0552)) | ((s_5, 0.4849), (s_5, -0.1040)) |
{{R}}_{9} | ((s_6, 0.0488), (s_4, 0.3846)) | ((s_7, 0.0848), (s_3, -0.0375)) | ((s_0, 0), (s_8, 0)) |
{{G}}_{1} | {{G}}_{2} | {{G}}_{3} | |
{{R}}_{1} | ((s_6, 0.0539), (s_5, -0.0285)) | ((s_6, -0.2441), (s_5, -0.3317)) | ((s_7, -0.4061), (s_4, -0.2107)) |
{{R}}_{2} | ((s_5, 0.4486), (s_5, 0.2840)) | ((s_7, 0.0878), (s_3, -0.0722)) | ((s_0, 0), (s_8, 0)) |
{{R}}_{3} | ((s_6, 0.4378), (s_4, 0.1766)) | ((s_6, 0.1349), (s_5, -0.1320)) | ((s_8, 0), (s_0, 0)) |
{{R}}_{4} | ((s_0, 0), (s_8, 0)) | ((s_7, -0.2648), (s_3, 0.1142)) | ((s_5, 0.2848), (s_5, 0.0870)) |
{{R}}_{5} | ((s_6, 0.4812), (s_4, 0.3434)) | ((s_4, 0.3848), (s_7, 0.1293)) | ((s_6, 0.4298), (s_4, 0.1621)) |
{{R}}_{6} | ((s_6, 0.3903), (s_4, 0.1630)) | ((s_7, -0.3064), (s_4, 0.0374)) | ((s_0, 0), (s_8, 0)) |
{{R}}_{7} | ((s_5, -0.1340), (s_7, 0.2730)) | ((s_4, -0.3785), (s_7, 0.2604)) | ((s_7, 0.1988), (s_2, 0.3094)) |
{{R}}_{8} | ((s_6, -0.0415), (s_5, -0.1043)) | ((s_7, -0.0291), (s_3, 0.2056)) | ((s_7, 0.2309), (s_2, 0.3916)) |
{{R}}_{9} | ((s_4, 0.4567), (s_7, 0.1722)) | ((s_0, 0), (s_8, 0)) | ((s_7, -0.0436), (s_3, -0.2953)) |
{{G}}_{4} | {{G}}_{5} | {{G}}_{6} | |
{{R}}_{1} | ((s_6, -0.4600), (s_5, 0.3085)) | ((s_4, 0.2348), (s_7, 0.1206)) | ((s_7, -0.1581), (s_4, 0.0392)) |
{{R}}_{2} | ((s_7, -0.4190), (s_4, 0.1904)) | ((s_5, 0.3148), (s_7, -0.2517)) | ((s_6, 0.3139), (s_4, 0.1675)) |
{{R}}_{3} | ((s_5, 0.0255), (s_7, -0.3929)) | ((s_5, 0.2969), (s_6, -0.4309)) | ((s_5, 0.3897), (s_7, -0.3847)) |
{{R}}_{4} | ((s_5, -0.3703), (s_7, 0.1945)) | ((s_5, 0.3582), (s_6, -0.2940)) | ((s_6, -0.0676), (s_, -0.2575)) |
{{R}}_{5} | ((s_4, 0.0599), (s_7, 0.2022)) | ((s_4, 0.2756), (s_7, 0.1176)) | ((s_6, 0.2501), (s_4, 0.2387)) |
{{R}}_{6} | ((s_6, 0.1459), (s_5, 0.0155)) | ((s_4, 0.1177), (s_7, 0.2162)) | ((s_6, 0.0339), (s_5, -0.4345)) |
{{R}}_{7} | ((s_7, -0.2744), (s_4, 0.0196)) | ((s_7, -0.3088), (s_4, -0.0700)) | ((s_5, -0.2523), (s_7, 0.1062)) |
{{R}}_{8} | ((s_4, -0.0354), (s_7, 0.2039)) | ((s_7, -0.0325), (s_4, -0.4910)) | ((s_5, -0.0211), (s_6, -0.1888)) |
{{R}}_{9} | ((s_5, 0.3467), (s_6, -0.2952)) | ((s_6, 0.2762), (s_4, 0.4843)) | ((s_0, 0), (s_8, 0)) |
{{R}}_{1} | {{R}}_{2} | {{R}}_{3} | {{R}}_{4} | {{R}}_{5} | {{R}}_{6} | {{R}}_{7} | {{R}}_{8} | {{R}}_{9} | |
{{R}}_{1} | 0 | 0.8665 | 0.0101 | 1.6448 | 1.5077 | 1.2851 | 0.8915 | -0.3694 | 2.7643 |
{{R}}_{2} | -0.8665 | 0 | -0.9769 | 0.7783 | 0.6413 | 0.4187 | 0.0251 | -1.2359 | 1.8979 |
{{R}}_{3} | -0.0101 | 0.9769 | 0 | 1.7553 | 1.6182 | 1.3956 | 1.0020 | -0.2589 | 2.8748 |
{{R}}_{4} | -1.6448 | -0.7783 | -1.7553 | 0 | -0.1371 | -0.3597 | -0.7533 | -2.0142 | 1.1195 |
{{R}}_{5} | -1.5077 | -0.6413 | -1.6182 | 0.1371 | 0 | -0.2226 | -0.6162 | -1.8771 | 1.2566 |
{{R}}_{6} | -1.2851 | -0.4187 | -1.3956 | 0.3597 | 0.2226 | 0 | -0.3936 | -1.6545 | 1.4792 |
{{R}}_{7} | -0.8915 | -0.0251 | -1.0020 | 0.7533 | 0.6162 | 0.3936 | 0 | -1.2609 | 1.8728 |
{{R}}_{8} | 0.3694 | 1.2359 | 0.2589 | 2.0142 | 1.8771 | 1.6545 | 1.2609 | 0 | 3.1337 |
{{R}}_{9} | -2.7643 | -1.8979 | -2.8748 | -1.1195 | -1.2566 | -1.4792 | -1.8728 | -3.1337 | 0 |
{{G}}_{1} | {{G}}_{2} | {{G}}_{3} | |
{{R}}_{1} | ((s_4, 0.3805), (s_7, 0.1469)) | ((s_4, 0.0216), (s_7, 0.1384)) | ((s_3, 0.4930), (s_8, -0.3496)) |
{{R}}_{2} | ((s_4, -0.1115), (s_7, 0.2509)) | ((s_5, 0.2350), (s_6, 0.4675)) | ((s_0, 0), (s_8, 0)) |
{{R}}_{3} | ((s_5, -0.2826), (s_7, -0.1423)) | ((s_4, 0.3307), (s_7, 0.2019)) | ((s_8, 0), (s_0, 0)) |
{{R}}_{4} | ((s_0, 0), (s_8, 0)) | ((s_5, -0.1305), (s_7, -0.4475)) | ((s_3, -0.3221), (s_8, -0.2137)) |
{{R}}_{5} | ((s_5, -0.2428), (s_7, -0.0783)) | ((s_3, 0.0013), (s_8, -0.1927)) | ((s_3, 0.3777), (s_8, -0.3066)) |
{{R}}_{6} | ((s_5, -0.3257), (s_7, -0.1476)) | ((s_5, -0.1706), (s_7, -0.0775)) | ((s_0, 0), (s_8, 0)) |
{{R}}_{7} | ((s_3, 0.4426), (s_8, -0.3220)) | ((s_2, 0.4665), (s_8, -0.1625)) | ((s_4, 0.0118), (s_7, 0.4271)) |
{{R}}_{8} | ((s_4, 0.3003), (s_7, 0.1209)) | ((s_5, 0.1080), (s_7, -0.4073)) | ((s_4, 0.0193), (s_7, 0.4427)) |
{{R}}_{9} | ((s_3, 0.1395), (s_8, 0.2045)) | ((s_0, 0), (s_8, 0)) | ((s_4, -0.2276), (s_7, 0.4976)) |
{{G}}_{4} | {{G}}_{5} | {{G}}_{6} | |
{{R}}_{1} | ((s_5, -0.3960), (s_7, -0.3335)) | ((s_4, -0.3952), (s_8, -0.4655)) | ((s_5, -0.4962), (s_7, 0.2788)) |
{{R}}_{2} | ((s_6, -0.4103), (s_6, 0.0011)) | ((s_5, -0.4401), (s_7, 0.3290)) | ((s_4, 0.0492), (s_7, 0.3103)) |
{{R}}_{3} | ((s_4, 0.1512), (s_7, 0.3477)) | ((s_5, -0.4562), (s_7, -0.3610)) | ((s_3, 0.3673), (s_8, -0.2075)) |
{{R}}_{4} | ((s_4, -0.1886), (s_8, -0.3687)) | ((s_5, -0.4008), (s_7, -0.2775)) | ((s_4, -0.2445), (s_7, 0.4421)) |
{{R}}_{5} | ((s_3, 0.3309), (s_8, -0.3651)) | ((s_4, -0.3597), (s_8, -0.4672)) | ((s_4, -0.0015), (s_7, 0.3274)) |
{{R}}_{6} | ((s_5, 0.1621), (s_7, -0.4997)) | ((s_4, -0.4967), (s_8, -0.4136)) | ((s_4, -0.1684), (s_7, 0.4031)) |
{{R}}_{7} | ((s_6, -0.2606), (s_6, 0.1089)) | ((s_6, -0.1166), (s_6, -0.4517)) | ((s_3, -0.0639), (s_8, -0.1300)) |
{{R}}_{8} | ((s_3, 0.2513), (s_8, -0.3643)) | ((s_6, 0.1821), (s_5, 0.2339)) | ((s_3, 0.0888), (s_8, -0.3458)) |
{{R}}_{9} | ((s_4, 0.4321), (s_7, -0.1166)) | ((s_5, 0.4627), (s_6, 0.0617)) | ((s_0, 0), (s_8, 0)) |
{{R}}_{1} | {{R}}_{2} | {{R}}_{3} | {{R}}_{4} | {{R}}_{5} | {{R}}_{6} | {{R}}_{7} | {{R}}_{8} | {{R}}_{9} | |
{{R}}_{1} | 0 | 0.8665 | 0.0101 | 1.6448 | 1.5077 | 1.2851 | 0.8915 | -0.3694 | 2.7643 |
{{R}}_{2} | -0.8665 | 0 | -0.9769 | 0.7783 | 0.6413 | 0.4187 | 0.0251 | -1.2359 | 1.8979 |
{{R}}_{3} | -0.0101 | 0.9769 | 0 | 1.7553 | 1.6182 | 1.3956 | 1.0020 | -0.2589 | 2.8748 |
{{R}}_{4} | -1.6448 | -0.7783 | -1.7553 | 0 | -0.1371 | -0.3597 | -0.7533 | -2.0142 | 1.1195 |
{{R}}_{5} | -1.5077 | -0.6413 | -1.6182 | 0.1371 | 0 | -0.2226 | -0.6162 | -1.8771 | 1.2566 |
{{R}}_{6} | -1.2851 | -0.4187 | -1.3956 | 0.3597 | 0.2226 | 0 | -0.3936 | -1.6545 | 1.4792 |
{{R}}_{7} | -0.8915 | -0.0251 | -1.0020 | 0.7533 | 0.6162 | 0.3936 | 0 | -1.2609 | 1.8728 |
{{R}}_{8} | 0.3694 | 1.2359 | 0.2589 | 2.0142 | 1.8771 | 1.6545 | 1.2609 | 0 | 3.1337 |
{{R}}_{9} | -2.7643 | -1.8979 | -2.8748 | -1.1195 | -1.2566 | -1.4792 | -1.8728 | -3.1337 | 0 |
{{G}}_{1} | {{G}}_{2} | {{G}}_{3} | |
{{R}}_{1} | ((s_6, -0.0961), (s_6, -0.3603)) | ((s_2, 0.1718), (s_7, 0.1783)) | ((s_4, 0.0624), (s_6, 0.2396)) |
{{R}}_{2} | ((s_3, -0.3998), (s_7, 0.0990)) | ((s_5, -0.2385), (s_5, 0.4972)) | ((s_1, 0.4422), (s_8, -0.2737)) |
{{R}}_{3} | ((s_4, 0.2422), (s_6, 0.0676)) | ((s_4, 0.3482), (s_6, 0.1491)) | ((s_8, 0), (s_0, 0)) |
{{R}}_{4} | ((s_6, -0.1292), (s_6, -0.1601)) | ((s_3, 0.4843), (s_6, 0.3538)) | ((s_2, -0.4878), (s_7, 0.4843)) |
{{R}}_{5} | ((s_7, -0.0444), (s_4, 0.2884)) | ((s_6, 0.3337), (s_6, -0.4017)) | ((s_6, -0.0684), (s_5, -0.4954)) |
{{R}}_{6} | ((s_4, -0.2807), (s_6, 0.3164)) | ((s_7, -0.3151), (s_5, -0.0977)) | ((s_1, -0.0599), (s_8, -0.2005)) |
{{R}}_{7} | ((s_4, 0.0689), (s_6, 0.2841)) | ((s_2, 0.0635, (s_7, 0.4490)) | ((s_4, 0.4322), (s_6, -0.2882)) |
{{R}}_{8} | ((s_3, 0.4526), (s_7, -0.3928)) | ((s_6, 0.4542), (s_5, -0.110)) | ((s_5, -0.2896), (s_6, -0.4666)) |
{{R}}_{9} | ((s_5, 0.2397), (s_6, 0.3559)) | ((s_5, 0.3566), (s_6, 0.1203)) | ((s_4, -0.2063), (s_6, 0.1743)) |
{{G}}_{4} | {{G}}_{5} | {{G}}_{6} | |
{{R}}_{1} | ((s_3, -0.2832), (s_7, -0.0381)) | ((s_3, 0.2726), (s_7, -0.1462)) | ((s_8, 0), (s_0, 0)) |
{{R}}_{2} | ((s_6, 0.4719), (s_5, -0.0714)) | ((s_7, -0.3232), (s_5, -0.1548)) | ((s_3, 0.3950), (s_6, 0.4988)) |
{{R}}_{3} | ((s_4, -0.2593), (s_7, -0.4324)) | ((s_3, -0.4913), (s_7, 0.1456)) | ((s_7, -0.1266), (s_5, -0.3632)) |
{{R}}_{4} | ((s_4, 0.4649), (s_6, 0.3020)) | ((s_3, 0.3650), (s_7, -0.1510)) | ((s_3, 0.1467), (s_7, -0.2130)) |
{{R}}_{5} | ((s_3, -0.0851), (s_7, 0.0082)) | ((s_4, -0.4871), (s_7, -0.3113)) | ((s_4, -0.4392), (s_6, 0.4802)) |
{{R}}_{6} | ((s_8, 0), (s_0, 0) | ((s_2, 0.3524), (s_7, 0.3384)) | ((s_3, 0.1466), (s_7, -0.2670)) |
{{R}}_{7} | ((s_7, -0.2974), (s_5, -0.3171)) | ((s_6, 0.4789), (s_5, -0.1396)) | ((s_7, -0.1059), (s_5, -0.3389)) |
{{R}}_{8} | ((s_3, -0.4455), (s_7, 0.1303)) | ((s_8, 0, (s_0, 0) | ((s_3, -0.2232), (s_7, 0.1423)) |
{{R}}_{9} | ((s_3, 0.1835), (s_7, 0.1663)) | ((s_4, 0.1105), (s_6, 0.1671)) | ((s_2, 0.1042), (s_7, 0.4790)) |
{{G}}_{1} | {{G}}_{2} | {{G}}_{3} | |
{{R}}_{1} | ((s_7, -0.3993), (s_5, 0.0934)) | ((s_3, -0.0159), (s_7, -0.1225)) | ((s_5, -0.4604), (s_6, 0.0193)) |
{{R}}_{2} | ((s_4, -0.0714), (s_7, -0.4190)) | ((s_5, 0.4034), (s_5, 0.1645)) | ((s_2, -0.0902), (s_8, -0.3910)) |
{{R}}_{3} | ((s_5, 0.3549), (s_6, -0.4932)) | ((s_5, 0.0447), (s_6, -0.1950)) | ((s_8, 0), (s_0, 0)) |
{{R}}_{4} | ((s_7, -0.4227), (s_5, 0.2854)) | ((s_4, 0.2667), (s_6, 0.0106)) | ((s_2, -0.0130), (s_7, 0.3257)) |
{{R}}_{5} | ((s_7, 0.3222), (s_4, -0.1602)) | ((s_7, -0.2953), (s_5, 0.2628)) | ((s_6, 0.2297), (s_5, 0.2811)) |
{{R}}_{6} | ((s_5, -0.0728), (s_6, -0.2464)) | ((s_7, -0.0160), (s_5, -0.4076)) | ((s_1, 0.3354), (s_8, -0.2997)) |
{{R}}_{7} | ((s_5, 0.2154), (s_6, -0.2788)) | ((s_3, -0.1290), (s_7, 0.1869)) | ((s_5, -0.1174), (s_5, 0.4940)) |
{{R}}_{8} | ((s_5, -0.2994), (s_6, 0.0500)) | ((s_7, -0.1991), (s_5, -0.4203)) | ((s_5, 0.1376), (s_5, 0.3183)) |
{{R}}_{9} | ((s_6, 0.1205), (s_6, -0.2067)) | ((s_6, -0.0101), (s_6, -0.2236)) | ((s_4, 0.2872), (s_6, -0.0462)) |
{{G}}_{4} | {{G}}_{5} | {{G}}_{6} | |
{{R}}_{1} | ((s_4, 0.3587), (s_6, 0.2841)) | ((s_5, -0.2479), (s_6, 0.2093)) | ((s_8, 0), (s_0, 0)) |
{{R}}_{2} | ((s_7, 0.1011), (s_4, 0.3037)) | ((s_7, 0.2000), (s_5, -0.4528)) | ((s_5, -0.4211), (s_6, -0.0267)) |
{{R}}_{3} | ((s_5, 0.2174), (s_6, -0.1365)) | ((s_4, 0.0702), (s_7, -0.4642)) | ((s_7, 0.2473), (s_4, 0.0.1865)) |
{{R}}_{4} | ((s_6, -0.2367), (s_6, 0.4050)) | ((s_5, -0.1702), (s_6, 0.2042)) | ((s_4, 0.3580), (s_6, 0.2737)) |
{{R}}_{5} | ((s_5, -0.4653), (s_6, 0.3357)) | ((s_5, -0.0475), (s_6, 0.0333)) | ((s_5, -0.2768), (s_6, -0.0457)) |
{{R}}_{6} | ((s_8, 0), (s_0, 0) | ((s_4, -0.0795), (s_7, -0.2330)) | ((s_4, 0.3579), (s_6, 0.2165)) |
{{R}}_{7} | ((s_7, 0.2423), (s_4, 0.0825)) | ((s_7, 0.0749), (s_4, 0.2785)) | ((s_7, 0.2615), (s_4, 0.2088)) |
{{R}}_{8} | ((s_4, 0.2103), (s_6, 0.4752)) | ((s_8, 0), (s_0, 0)) | ((s_4, 0.0172), (s_7, -0.3362)) |
{{R}}_{9} | ((s_5, -0.2350), (s_6, 0.1438)) | ((s_5, 0.4272), (s_6, -0.4952)) | ((s_3, 0.3536), (s_7, 0.0678)) |
{{R}}_{1} | {{R}}_{2} | {{R}}_{3} | {{R}}_{4} | {{R}}_{5} | {{R}}_{6} | {{R}}_{7} | {{R}}_{8} | {{R}}_{9} | |
{{R}}_{1} | 0 | 0.3827 | -0.7907 | 1.2319 | -0.3662 | 0.3409 | -0.8652 | -0.3901 | 1.0152 |
{{R}}_{2} | -0.3827 | 0 | -1.1735 | 0.8492 | -0.7489 | -0.0142 | -1.2480 | -0.7729 | 0.6325 |
{{R}}_{3} | 0.7907 | 1.1735 | 0 | 2.0226 | 0.4245 | 1.1316 | -0.0745 | 0.4006 | 1.8059 |
{{R}}_{4} | -1.2319 | -0.8492 | -2.0226 | 0 | -1.5981 | -0.8910 | -2.0972 | -1.6220 | -0.2167 |
{{R}}_{5} | 0.3662 | 0.7489 | -0.4245 | 1.5981 | 0 | 0.7071 | -0.4990 | -0.0239 | 1.3814 |
{{R}}_{6} | -0.3409 | 0.0142 | -1.1316 | 0.8910 | -0.7071 | 0 | -1.2061 | -0.7310 | 0.6743 |
{{R}}_{7} | 0.8652 | 1.2480 | 0.0745 | 2.0972 | 0.4990 | 1.2061 | 0 | 0.4751 | 1.8804 |
{{R}}_{8} | 0.3901 | 0.7729 | -0.4006 | 1.6220 | 0.0239 | 0.7310 | -0.4751 | 0 | 1.4053 |
{{R}}_{9} | -1.0152 | -0.6325 | -1.8059 | 0.2167 | -1.3814 | -0.6743 | -1.8804 | -1.4053 | 0 |
{{G}}_{1} | {{G}}_{2} | {{G}}_{3} | |
{{R}}_{1} | ((s_8, -0.3564), (s_4, -0.3858)) | ((s_6, 0.4936), (s_5, 0.0110)) | ((s_8, -0.2665), (s_3, 0.1101)) |
{{R}}_{2} | ((s_7, -0.2411), (s_5, -0.1498)) | ((s_7, 0.3627), (s_4, -0.4322)) | ((s_7, 0.3432), (s_4, 0.4644)) |
{{R}}_{3} | ((s_7, 0.2739), (s_4, 0.0657)) | ((s_7, 0.2565), (s_4, 0.0607)) | ((s_8, 0), (s_0, 0)) |
{{R}}_{4} | ((s_8, -0.3629), (s_4, -0.2386)) | ((s_7, 0.0038), (s_4, 0.2273)) | ((s_7, 0.3607), (s_4, 0.1157)) |
{{R}}_{5} | ((s_8, -0.1661), (s_3, -0.3069)) | ((s_8, -0.2934), (s_4, -0.3587)) | ((s_8, -0.1188), (s_3, -0.3242)) |
{{R}}_{6} | ((s_7, 0.1317), (s_4, 0.1317)) | ((s_8, -0.2266), (s_3, 0.1496)) | ((s_7, 0.1878), (s_5, -0.3895)) |
{{R}}_{7} | ((s_7, -0.2285), (s_4, 0.1055)) | ((s_6, 0.4407), (s_5, 0.3486)) | ((s_8, -0.2328), (s_3, -0.2034)) |
{{R}}_{8} | ((s_7, 0.0526), (s_4, 0.3772)) | ((s_8, -0.2702), (s_3, 0.1405)) | ((s_8, -0.2091), (s_3, -0.3033)) |
{{R}}_{9} | ((s_8, -0.4920), (s_4, 0.1640)) | ((s_8, -0.4750), (s_4, 0.0379)) | ((s_8, -0.2929), (s_3, 0.0695)) |
{{G}}_{4} | {{G}}_{5} | {{G}}_{6} | |
{{R}}_{1} | ((s_6, 0.1071), (s_5, 0.2954)) | ((s_6, 0.1183), (s_5, 0.3971)) | ((s_8, 0), (s_0, 0)) |
{{R}}_{2} | ((s_8, -0.4129), (s_4, 0.4646)) | ((s_8, 0.4224), (s_4, -0.1227)) | ((s_7, 0.4061), (s_4, -0.2140)) |
{{R}}_{3} | ((s_7, 0.3846), (s_5, -0.1023)) | ((s_6, -0.3507), (s_6, -0.2773)) | ((s_8, -0.1085), (s_3, -0.4259)) |
{{R}}_{4} | ((s_7, -0.0854), (s_5, 0.3467)) | ((s_6, 0.1696), (s_5, 0.3921)) | ((s_7, 0.3556), (s_4, 0.0171)) |
{{R}}_{5} | ((s_6, 0.2155), (s_5, 0.3451)) | ((s_6, 0.2498), (s_5, 0.2271)) | ((s_7, 0.4379), (s_4, -0.2282)) |
{{R}}_{6} | ((s_8, 0), (s_0, 0)) | ((s_6, -0.4586), (s_6, -0.0364)) | ((s_7, 0.3556), (s_4, -0.0279)) |
{{R}}_{7} | ((s_8, -0.3462), (s_3, 0.3498)) | ((s_8, -0.4904), (s_4, -0.3572)) | ((s_8, -0.1064), (s_3, -0.4116)) |
{{R}}_{8} | ((s_6, 0.0137), (s_5, 0.4829)) | ((s_8, 0), (s_0, 0)) | ((s_7, 0.2733), (s_4, 0.3421)) |
{{R}}_{9} | ((s_6, 0.3539), (s_5, 0.1601)) | ((s_7, -0.4486), (s_5, 0.2670)) | ((s_7, 0.0940), (s_5, -0.2737)) |
{{R}}_{1} | {{R}}_{2} | {{R}}_{3} | {{R}}_{4} | {{R}}_{5} | {{R}}_{6} | {{R}}_{7} | {{R}}_{8} | {{R}}_{9} | |
{{R}}_{1} | 0 | -0.6860 | -0.2921 | 0.0886 | -0.5449 | -0.3772 | -1.0436 | -0.7952 | -0.0798 |
{{R}}_{2} | 0.6860 | 0 | 0.3939 | 0.7745 | 0.1410 | 0.3088 | -0.3576 | -0.1093 | 0.6062 |
{{R}}_{3} | 0.2921 | -0.3939 | 0 | 0.3807 | -0.2529 | -0.0851 | -0.7515 | -0.5032 | 0.2123 |
{{R}}_{4} | -0.0886 | -0.7745 | -0.3807 | 0 | -0.6335 | -0.4658 | -1.1322 | -0.8838 | -0.1684 |
{{R}}_{5} | 0.5449 | -0.1410 | 0.2529 | 0.6335 | 0 | 0.1677 | -0.4987 | -0.2503 | 0.4652 |
{{R}}_{6} | 0.3772 | -0.3088 | 0.0851 | 0.4658 | -0.1677 | 0 | -0.6664 | -0.4180 | 0.2974 |
{{R}}_{7} | 1.0436 | 0.3576 | 0.7515 | 1.1322 | 0.4987 | 0.6664 | 0 | 0.2484 | 0.9638 |
{{R}}_{8} | 0.7952 | 0.1093 | 0.5032 | 0.8838 | 0.2503 | 0.4180 | -0.2484 | 0 | 0.7155 |
{{R}}_{9} | 0.0798 | -0.6062 | -0.2123 | 0.1684 | -0.4652 | -0.2974 | -0.9638 | -0.7155 | 0 |
Parameter | £_{1} | £_{2} | £_{3} | £_{4} | £_{5} | £_{6} | £_{7} | £_{8} | £_{9} |
q=1 | 9.2419 | 0.4522 | 8.0325 | -5.5686 | -5.6983 | -2.6495 | -0.7889 | 12.5685 | -15.5897 |
q=2 | 8.3757 | 0.8741 | 8.8384 | -6.4119 | -5.5053 | -2.9184 | 0.4589 | 12.1618 | -15.8735 |
q=3 | 8.6811 | 0.4570 | 9.9984 | -6.2013 | -4.6643 | -3.2330 | 0.3786 | 11.4614 | -16.8780 |
q=4 | 9.2018 | 0.1342 | 10.9091 | -6.9945 | -3.6352 | -3.6145 | 0.4292 | 11.1241 | -17.5541 |
q=5 | 9.0140 | -0.0512 | 11.6425 | -7.1200 | -3.0797 | -4.0100 | 0.5400 | 11.0921 | -18.0277 |
q=6 | 9.4112 | -0.4079 | 12.0041 | -7.2024 | -2.5031 | -4.4406 | 0.7780 | 10.7475 | -18.3869 |
q=7 | 9.0445 | -0.8170 | 12.9281 | -7.2637 | -1.8192 | -4.8432 | 0.9374 | 10.5033 | -18.6703 |
q=8 | 9.0544 | -0.9737 | 13.3726 | -7.3163 | -1.3976 | -5.2068 | 1.1006 | 10.2916 | -18.9248 |
Parameter | £_{1} | £_{2} | £_{3} | £_{4} | £_{5} | £_{6} | £_{7} | £_{8} | £_{9} |
{\kappa}=1 | -1.2161 | -0.3893 | 1.6164 | -6.7267 | 5.3976 | -7.5968 | 8.8638 | 0.7522 | -0.7012 |
{\kappa}=2 | 6.3461 | -1.8035 | 8.3705 | -7.0144 | -3.2918 | 0.3788 | 2.6722 | 9.5008 | -15.1586 |
{\kappa}=3 | 8.6007 | 0.6820 | 9.3537 | -6.3231 | -5.0894 | -3.0861 | 0.4563 | 11.8046 | -16.3987 |
{\kappa}=4 | 11.5855 | 2.7295 | 1.3611 | -2.0245 | -11.4463 | 0.5527 | -6.1084 | 15.4832 | -12.1329 |
Parameter | Ranking |
q=1 | {{R}}_{8} > {{R}}_{1} > {{R}}_{3} > {{R}}_{2} > {{R}}_{7} > {{R}}_{6} > {{R}}_{5} > {{R}}_{4} > {{R}}_{9} |
q=2 | {{R}}_{8} > {{R}}_{3} > {{R}}_{1} > {{R}}_{2} > {{R}}_{7} > {{R}}_{6} > {{R}}_{5} > {{R}}_{4} > {{R}}_{9} |
q=3 | {{R}}_{8} > {{R}}_{3} > {{R}}_{1} > {{R}}_{2} > {{R}}_{7} > {{R}}_{6} > {{R}}_{5} > {{R}}_{4} > {{R}}_{9} |
q=4 | {{R}}_{8} > {{R}}_{3} > {{R}}_{1} > {{R}}_{7} > {{R}}_{2} > {{R}}_{6} > {{R}}_{5} > {{R}}_{4} > {{R}}_{9} |
q=5 | {{R}}_{8} > {{R}}_{3} > {{R}}_{1} > {{R}}_{7} > {{R}}_{2} > {{R}}_{5} > {{R}}_{6} > {{R}}_{4} > {{R}}_{9} |
q=6 | {{R}}_{8} > {{R}}_{3} > {{R}}_{1} > {{R}}_{7} > {{R}}_{2} > {{R}}_{5} > {{R}}_{6} > {{R}}_{4} > {{R}}_{9} |
q=7 | {{R}}_{8} > {{R}}_{3} > {{R}}_{1} > {{R}}_{7} > {{R}}_{2} > {{R}}_{5} > {{R}}_{6} > {{R}}_{4} > {{R}}_{9} |
q=8 | {{R}}_{8} > {{R}}_{3} > {{R}}_{1} > {{R}}_{7} > {{R}}_{2} > {{R}}_{5} > {{R}}_{6} > {{R}}_{4} > {{R}}_{9} |
Parameter | Ranking |
{\kappa} =1 | {{R}}_{7} > {{R}}_{5} > {{R}}_{3} > {{R}}_{8} > {{R}}_{2} > {{R}}_{9} > {{R}}_{1} > {{R}}_{4} > {{R}}_{6} |
{\kappa} =2 | {{R}}_{8} > {{R}}_{3} > {{R}}_{1} > {{R}}_{7} > {{R}}_{6} > {{R}}_{2} > {{R}}_{5} > {{R}}_{4} > {{R}}_{9} |
{\kappa} =3 | {{R}}_{8} > {{R}}_{3} > {{R}}_{1} > {{R}}_{2} > {{R}}_{7} > {{R}}_{6} > {{R}}_{5} > {{R}}_{4} > {{R}}_{9} |
{\kappa} =4 | {{R}}_{8} > {{R}}_{1} > {{R}}_{2} > {{R}}_{3} > {{R}}_{6} > {{R}}_{4} > {{R}}_{7} > {{R}}_{5} > {{R}}_{9} |
Parameter | £_{1} | £_{2} | £_{3} | £_{4} | £_{5} | £_{6} | £_{7} | £_{8} | £_{9} |
q=1 | 0.1530 | -3.1792 | 7.1507 | -12.0965 | 5.5735 | -1.8540 | 8.0698 | 3.1966 | -7.0140 |
q=2 | 0.1057 | -2.9132 | 7.8509 | -11.0315 | 4.3727 | -2.5697 | 8.5268 | 3.7767 | -8.1183 |
q=3 | 1.0370 | -2.8208 | 7.4580 | -10.1699 | 3.4083 | -2.4021 | 8.1027 | 4.3676 | -8.9809 |
q=4 | 2.0292 | -2.8761 | 6.8321 | -9.6699 | 2.5850 | -1.9598 | 7.6085 | 5.0272 | -9.5762 |
q=5 | 2.6374 | -2.9743 | 6.9033 | -9.3430 | 2.0591 | -1.4359 | 7.1377 | 4.9781 | -9.9625 |
q=6 | 3.1882 | -3.1053 | 6.7752 | -9.1052 | 1.5023 | -0.8554 | 6.6762 | 5.1364 | -10.2125 |
q=7 | 3.9542 | -3.2434 | 6.3435 | -8.9285 | 1.0057 | -0.2839 | 6.2217 | 5.2570 | -10.3264 |
q=8 | 4.2588 | -3.3906 | 6.2811 | -8.8002 | 0.5206 | 0.2603 | 5.7110 | 5.4004 | -10.2414 |
Parameter | £_{1} | £_{2} | £_{3} | £_{4} | £_{5} | £_{6} | £_{7} | £_{8} | £_{9} |
{\kappa}=1 | 3.4005 | 2.5863 | 1.4628 | -5.4874 | -8.1530 | 2.2088 | -0.6670 | 12.0452 | -7.3961 |
{\kappa}=2 | 3.7906 | -0.9149 | 11.8515 | -14.4767 | 1.8085 | -0.3653 | 6.5394 | 9.4213 | -17.6544 |
{\kappa}=3 | 0.5583 | -2.8586 | 7.6750 | -10.5288 | 3.8543 | -2.5370 | 8.3456 | 4.0695 | -8.5783 |
{\kappa}=4 | -2.6107 | -3.5564 | 1.8935 | -13.2726 | 12.1627 | -7.5384 | 11.5950 | 0.5158 | 0.8112 |
Parameter | Ranking |
q=1 | {{R}}_{7} > {{R}}_{3} > {{R}}_{5} > {{R}}_{8} > {{R}}_{1} > {{R}}_{6} > {{R}}_{2} > {{R}}_{9} > {{R}}_{4} |
q=2 | {{R}}_{7} > {{R}}_{3} > {{R}}_{5} > {{R}}_{8} > {{R}}_{1} > {{R}}_{6} > {{R}}_{2} > {{R}}_{9} > {{R}}_{4} |
q=3 | {{R}}_{7} > {{R}}_{3} > {{R}}_{8} > {{R}}_{5} > {{R}}_{1} > {{R}}_{6} > {{R}}_{2} > {{R}}_{9} > {{R}}_{4} |
q=4 | {{R}}_{7} > {{R}}_{3} > {{R}}_{8} > {{R}}_{5} > {{R}}_{1} > {{R}}_{6} > {{R}}_{2} > {{R}}_{9} > {{R}}_{4} |
q=5 | {{R}}_{7} > {{R}}_{3} > {{R}}_{8} > {{R}}_{1} > {{R}}_{5} > {{R}}_{6} > {{R}}_{2} > {{R}}_{4} > {{R}}_{9} |
q=6 | {{R}}_{3} > {{R}}_{7} > {{R}}_{8} > {{R}}_{1} > {{R}}_{5} > {{R}}_{6} > {{R}}_{2} > {{R}}_{4} > {{R}}_{9} |
q=7 | {{R}}_{3} > {{R}}_{7} > {{R}}_{8} > {{R}}_{1} > {{R}}_{5} > {{R}}_{6} > {{R}}_{2} > {{R}}_{4} > {{R}}_{9} |
q=8 | {{R}}_{3} > {{R}}_{7} > {{R}}_{8} > {{R}}_{1} > {{R}}_{5} > {{R}}_{6} > {{R}}_{2} > {{R}}_{4} > {{R}}_{9} |
Parameter | Ranking |
{\kappa} =1 | {{R}}_{8} > {{R}}_{1} > {{R}}_{2} > {{R}}_{6} > {{R}}_{3} > {{R}}_{7} > {{R}}_{4} > {{R}}_{9} > {{R}}_{5} |
{\kappa} =2 | {{R}}_{3} > {{R}}_{8} > {{R}}_{7} > {{R}}_{1} > {{R}}_{5} > {{R}}_{6} > {{R}}_{2} > {{R}}_{4} > {{R}}_{9} |
{\kappa} =3 | {{R}}_{7} > {{R}}_{3} > {{R}}_{8} > {{R}}_{5} > {{R}}_{1} > {{R}}_{6} > {{R}}_{2} > {{R}}_{9} > {{R}}_{4} |
{\kappa} =4 | {{R}}_{5} > {{R}}_{7} > {{R}}_{3} > {{R}}_{9} > {{R}}_{8} > {{R}}_{1} > {{R}}_{2} > {{R}}_{6} > {{R}}_{4} |
Alternatives | EDAS | Ranking | CODAS | Ranking | TOPSIS | Ranking |
{{R}}_{1} | 0.6255 | III | 8.6007 | III | 0.6850 | III |
{{R}}_{2} | 0.7340 | II | 0.6820 | IV | 0.6278 | IV |
{{R}}_{3} | 0.5452 | VI | 9.3537 | II | 0.7280 | II |
{{R}}_{4} | 0.3315 | VIII | -6.3231 | VIII | 0.5059 | VIII |
{{R}}_{5} | 0.4210 | VII | -5.0894 | VII | 0.5399 | VII |
{{R}}_{6} | 0.5998 | IV | -3.0861 | VI | 0.5812 | VI |
{{R}}_{7} | 0.5728 | V | 0.4563 | V | 0.6136 | V |
{{R}}_{8} | 0.8143 | I | 11.8046 | I | 0.7400 | I |
{{R}}_{9} | 0.1015 | IX | -16.3987 | IX | 0.4010 | IX |
Alternatives | EDAS | Ranking | CODAS | Ranking | TOPSIS | Ranking |
{{R}}_{1} | 0.4897 | VI | 0.5583 | V | 0.3909 | V |
{{R}}_{2} | 0.2840 | VII | -2.8586 | VII | 0.3490 | VII |
{{R}}_{3} | 0.8716 | I | 7.6750 | II | 0.4678 | II |
{{R}}_{4} | 0.2296 | IX | -10.5288 | IX | 0.2593 | IX |
{{R}}_{5} | 0.6853 | II | 3.8543 | IV | 0.4197 | IV |
{{R}}_{6} | 0.2521 | VIII | -2.5370 | VI | 0.3634 | VI |
{{R}}_{7} | 0.5000 | IV | 8.3456 | I | 0.4727 | I |
{{R}}_{8} | 0.5000 | III | 4.0695 | III | 0.4274 | III |
{{R}}_{9} | 0.5000 | V | -8.5783 | VIII | 0.2784 | VIII |
Criterion | Explanation |
Weight density | This criterion takes into account the physical weight of the robot. In general, consumer chooses a lighter robot. The weight density is usually expressed in kg ({{G}}_{1}) . |
Replicability | This refers to a robot's ability to repeat a task over and over again. More replicability is often preferred. Replicability is usually measured in millimeters ({{G}}_{2}) . |
Freight capacity | The highest total weight a robot can lift in one turn is referred to as freight capacity. Being more is often preferred. The weight density is usually expressed in kg ({{G}}_{3}) . |
Maximum reach | This is the average of the maximum vertical and horizontal distances a robot's arm can extend to complete a task. It is common to want to be more. The robot's maximum reach is typically measured in millimeters ({{G}}_{4}) . |
Average power consumption | It refers to the robot's average power consumption in units of electricity. It is often desirable that a robot consumes less energy. The robot's power consumption is usually measured in kilowatts ({{G}}_{5}) . |
Motion of a robot | The motion of a robot at a reference point near the end effector's tip is referred to as robot motion. Trajectory, speed, acceleration, and acceleration derivative are commonly used to describe robot motion. The robot's motion is usually expressed in ms^{-1} or ms^{-2} \; ({{G}}_{6}) |
Sr. No. | Criteria | Units | Symbol |
1 | Weight density | Kg | {{G}}_{1} |
2 | Replicability | (+/-) mm | {{G}}_{2} |
3 | Freight capacity | Kg | {{G}}_{3} |
4 | Maximum Reach | mm | {{G}}_{4} |
5 | Average Power Consumption | KW | {{G}}_{5} |
6 | Motion of a robot | ms^{-2} | {{G}}_{6} |
Linguistic variables | 2TL q -ROFNs |
Certainly high value (CHV) | ((s_{8}, 0), (s_{0}, 0)) |
Very high value (VHV) | ((s_{7}, 0), (s_{1}, 0)) |
High value(HV) | ((s_{6}, 0), (s_{2}, 0)) |
Above average value (AAV) | ((s_{5}, 0), (s_{3}, 0)) |
Average vlaue (AV) | ((s_{4}, 0), (s_{4}, 0)) |
Under average value (UAV) | ((s_{3}, 0), (s_{5}, 0)) |
Low value (LV) | ((s_{2}, 0), (s_{6}, 0)) |
Very low value (VLV) | ((s_{1}, 0), (s_{7}, 0)) |
Certainly low value (CLV) | ((s_{0}, 0), (s_{8}, 0)) |
Experts | Alternatives | Attributes | |||||
{{G}}_{1} | {{G}}_{2} | {{G}}_{3} | {{G}}_{4} | {{G}}_{5} | {{G}}_{6} | ||
\Theta_{1} | |||||||
{{R}}_{1} | AV | LV | VHV | VLV | CLV | CHV | |
{{R}}_{2} | HV | UAV | CLV | AAV | VHV | LV | |
{{R}}_{3} | VHV | VLV | CHV | HV | UAV | CLV | |
{{R}}_{4} | CLV | AAV | LV | VHV | VLV | AAV | |
{{R}}_{5} | CHV | HV | UAV | CLV | LV | AV | |
{{R}}_{6} | LV | VHV | VLV | CHV | AV | HV | |
{{R}}_{7} | UAV | CLV | AAV | AV | HV | VLV | |
{{R}}_{8} | AAV | AV | HV | UAV | CHV | VHV | |
{{R}}_{9} | VLV | CHV | AV | LV | AAV | UAV | |
\Theta_{2} | |||||||
{{R}}_{1} | VLV | AV | HV | UAV | LV | CHV | |
{{R}}_{2} | LV | VHV | VLV | CHV | CLV | AAV | |
{{R}}_{3} | AV | HV | UAV | CLV | AAV | VHV | |
{{R}}_{4} | CLV | AAV | LV | VHV | VLV | UAV | |
{{R}}_{5} | VHV | VLV | CHV | HV | UAV | AV | |
{{R}}_{6} | HV | UAV | CLV | AAV | AV | LV | |
{{R}}_{7} | AAV | LV | VHV | AV | CHV | CLV | |
{{R}}_{8} | UAV | CHV | AV | LV | VHV | VLV | |
{{R}}_{9} | CHV | CLV | AAV | VLV | HV | AV | |
\Theta_{3} | |||||||
{{R}}_{1} | AV | UAV | LV | AAV | HV | VLV | |
{{R}}_{2} | LV | VHV | AV | HV | UAV | AAV | |
{{R}}_{3} | VHV | VLV | CHV | AV | LV | UAV | |
{{R}}_{4} | CHV | AV | UAV | VLV | AAV | HV | |
{{R}}_{5} | HV | CHV | VLV | UAV | CLV | VHV | |
{{R}}_{6} | AAV | LV | CLV | CHV | VLV | AV | |
{{R}}_{7} | CLV | AAV | HV | VHV | AV | CHV | |
{{R}}_{8} | VLV | HV | VHV | CLV | CHV | LV | |
{{R}}_{9} | UAV | CLV | AAV | LV | VHV | CLV | |
\Theta_{4} | |||||||
{{R}}_{1} | CHV | LV | UAV | AV | AAV | HV | |
{{R}}_{2} | LV | HV | CLV | UAV | CHV | AAV | |
{{R}}_{3} | UAV | VHV | CHV | HV | VLV | CHV | |
{{R}}_{4} | AV | AAV | VLV | CLV | HV | LV | |
{{R}}_{5} | VLV | CLV | AV | LV | VHV | UAV | |
{{R}}_{6} | AAV | CHV | LV | VLV | CLV | AV | |
{{R}}_{7} | VHV | VLV | AAV | CHV | AV | VHV | |
{{R}}_{8} | HV | AV | VHV | AAV | UAV | VLV | |
{{R}}_{9} | CLV | UAV | HV | VHV | LV | CLV |
Experts | Alternatives | Attributes | |||||
{{G}}_{1} | {{G}}_{2} | {{G}}_{3} | {{G}}_{4} | {{G}}_{5} | {{G}}_{6} | ||
\Theta_{1} | |||||||
{{R}}_{1} | ((s_{4}, 0), (s_{4}, 0)) | ((s_{2}, 0), (s_{6}, 0)) | ((s_{7}, 0), (s_{1}, 0)) | ((s_{1}, 0), (s_{7}, 0)) | ((s_{0}, 0), (s_{8}, 0)) | ((s_{8}, 0), (s_{0}, 0)) | |
{{R}}_{2} | ((s_{6}, 0), (s_{2}, 0)) | ((s_{3}, 0), (s_{5}, 0)) | ((s_{0}, 0), (s_{8}, 0)) | ((s_{5}, 0), (s_{3}, 0)) | ((s_{7}, 0), (s_{1}, 0)) | ((s_{2}, 0), (s_{6}, 0)) | |
{{R}}_{3} | ((s_{7}, 0), (s_{1}, 0)) | ((s_{1}, 0), (s_{7}, 0)) | ((s_{8}, 0), (s_{0}, 0)) | ((s_{6}, 0), (s_{2}, 0)) | ((s_{3}, 0), (s_{5}, 0)) | ((s_{0}, 0), (s_{8}, 0)) | |
{{R}}_{4} | ((s_{0}, 0), (s_{8}, 0)) | ((s_{5}, 0), (s_{3}, 0)) | ((s_{2}, 0), (s_{6}, 0)) | ((s_{7}, 0), (s_{1}, 0)) | ((s_{1}, 0), (s_{7}, 0)) | ((s_{5}, 0), (s_{3}, 0)) | |
{{R}}_{5} | ((s_{8}, 0), (s_{0}, 0)) | ((s_{6}, 0), (s_{2}, 0)) | ((s_{3}, 0), (s_{5}, 0)) | ((s_{0}, 0), (s_{8}, 0)) | ((s_{2}, 0), (s_{6}, 0)) | ((s_{4}, 0), (s_{4}, 0)) | |
{{R}}_{6} | ((s_{2}, 0), (s_{6}, 0)) | ((s_{7}, 0), (s_{1}, 0)) | ((s_{1}, 0), (s_{7}, 0)) | ((s_{8}, 0), (s_{0}, 0)) | ((s_{4}, 0), (s_{4}, 0)) | ((s_{6}, 0), (s_{2}, 0)) | |
{{R}}_{7} | ((s_{3}, 0), (s_{5}, 0)) | ((s_{0}, 0), (s_{8}, 0)) | ((s_{5}, 0), (s_{3}, 0)) | ((s_{4}, 0), (s_{4}, 0)) | ((s_{6}, 0), (s_{2}, 0)) | ((s_{1}, 0), (s_{7}, 0)) | |
{{R}}_{8} | ((s_{5}, 0), (s_{3}, 0)) | ((s_{4}, 0), (s_{4}, 0)) | ((s_{6}, 0), (s_{2}, 0)) | ((s_{3}, 0), (s_{5}, 0)) | ((s_{8}, 0), (s_{0}, 0)) | ((s_{7}, 0), (s_{1}, 0)) | |
{{R}}_{9} | ((s_{1}, 0), (s_{7}, 0)) | ((s_{8}, 0), (s_{0}, 0)) | ((s_{4}, 0), (s_{4}, 0)) | ((s_{2}, 0), (s_{6}, 0)) | ((s_{5}, 0), (s_{3}, 0)) | ((s_{3}, 0), (s_{5}, 0)) | |
\Theta_{2} | |||||||
{{R}}_{1} | ((s_{1}, 0), (s_{7}, 0)) | ((s_{4}, 0), (s_{4}, 0)) | ((s_{6}, 0), (s_{2}, 0)) | ((s_{3}, 0), (s_{5}, 0)) | ((s_{2}, 0), (s_{6}, 0)) | ((s_{8}, 0), (s_{0}, 0)) | |
{{R}}_{2} | ((s_{2}, 0), (s_{6}, 0)) | ((s_{7}, 0), (s_{1}, 0)) | ((s_{1}, 0), (s_{7}, 0)) | ((s_{8}, 0), (s_{0}, 0)) | ((s_{0}, 0), (s_{8}, 0)) | ((s_{5}, 0), (s_{3}, 0)) | |
{{R}}_{3} | ((s_{4}, 0), (s_{4}, 0)) | ((s_{6}, 0), (s_{2}, 0)) | ((s_{3}, 0), (s_{5}, 0)) | ((s_{0}, 0), (s_{8}, 0)) | ((s_{5}, 0), (s_{3}, 0)) | ((s_{7}, 0), (s_{1}, 0)) | |
{{R}}_{4} | ((s_{0}, 0), (s_{8}, 0)) | ((s_{5}, 0), (s_{3}, 0)) | ((s_{2}, 0), (s_{6}, 0)) | ((s_{7}, 0), (s_{1}, 0)) | ((s_{1}, 0), (s_{7}, 0)) | ((s_{3}, 0), (s_{5}, 0)) | |
{{R}}_{5} | ((s_{7}, 0), (s_{1}, 0)) | ((s_{1}, 0), (s_{7}, 0)) | ((s_{8}, 0), (s_{0}, 0)) | ((s_{6}, 0), (s_{2}, 0)) | ((s_{3}, 0), (s_{5}, 0)) | ((s_{4}, 0), (s_{4}, 0)) | |
{{R}}_{6} | ((s_{6}, 0), (s_{2}, 0)) | ((s_{3}, 0), (s_{5}, 0)) | ((s_{0}, 0), (s_{8}, 0)) | ((s_{5}, 0), (s_{3}, 0)) | ((s_{4}, 0), (s_{4}, 0)) | ((s_{2}, 0), (s_{6}, 0)) | |
{{R}}_{7} | ((s_{5}, 0), (s_{3}, 0)) | ((s_{2}, 0), (s_{6}, 0)) | ((s_{7}, 0), (s_{1}, 0)) | ((s_{4}, 0), (s_{4}, 0)) | ((s_{8}, 0), (s_{0}, 0)) | ((s_{0}, 0), (s_{8}, 0)) | |
{{R}}_{8} | ((s_{3}, 0), (s_{5}, 0)) | ((s_{8}, 0), (s_{0}, 0)) | ((s_{4}, 0), (s_{4}, 0)) | ((s_{2}, 0), (s_{6}, 0)) | ((s_{7}, 0), (s_{1}, 0)) | ((s_{1}, 0), (s_{7}, 0)) | |
{{R}}_{9} | ((s_{8}, 0), (s_{0}, 0)) | ((s_{0}, 0), (s_{8}, 0)) | ((s_{5}, 0), (s_{3}, 0)) | ((s_{1}, 0), (s_{7}, 0)) | ((s_{6}, 0), (s_{2}, 0)) | ((s_{4}, 0), (s_{4}, 0)) | |
\Theta_{3} | |||||||
{{R}}_{1} | ((s_{4}, 0), (s_{4}, 0)) | ((s_{3}, 0), (s_{5}, 0)) | ((s_{2}, 0), (s_{6}, 0)) | ((s_{5}, 0), (s_{3}, 0)) | ((s_{6}, 0), (s_{2}, 0)) | ((s_{1}, 0), (s_{7}, 0)) | |
{{R}}_{2} | ((s_{2}, 0), (s_{6}, 0)) | ((s_{7}, 0), (s_{1}, 0)) | ((s_{4}, 0), (s_{4}, 0)) | ((s_{6}, 0), (s_{2}, 0)) | ((s_{3}, 0), (s_{5}, 0)) | ((s_{5}, 0), (s_{3}, 0)) | |
{{R}}_{3} | ((s_{7}, 0), (s_{1}, 0)) | ((s_{1}, 0), (s_{7}, 0)) | ((s_{8}, 0), (s_{0}, 0)) | ((s_{4}, 0), (s_{4}, 0)) | ((s_{2}, 0), (s_{6}, 0)) | ((s_{3}, 0), (s_{5}, 0)) | |
{{R}}_{4} | ((s_{8}, 0), (s_{0}, 0)) | ((s_{4}, 0), (s_{4}, 0)) | ((s_{3}, 0), (s_{5}, 0)) | ((s_{1}, 0), (s_{7}, 0)) | ((s_{5}, 0), (s_{3}, 0)) | ((s_{6}, 0), (s_{2}, 0)) | |
{{R}}_{5} | ((s_{6}, 0), (s_{2}, 0)) | ((s_{8}, 0), (s_{0}, 0)) | ((s_{1}, 0), (s_{7}, 0)) | ((s_{3}, 0), (s_{5}, 0)) | ((s_{0}, 0), (s_{8}, 0)) | ((s_{7}, 0), (s_{1}, 0)) | |
{{R}}_{6} | ((s_{5}, 0), (s_{3}, 0)) | ((s_{2}, 0), (s_{6}, 0)) | ((s_{0}, 0), (s_{8}, 0)) | ((s_{8}, 0), (s_{0}, 0)) | ((s_{1}, 0), (s_{7}, 0)) | ((s_{4}, 0), (s_{4}, 0)) | |
{{R}}_{7} | ((s_{0}, 0), (s_{8}, 0)) | ((s_{5}, 0), (s_{3}, 0)) | ((s_{6}, 0), (s_{2}, 0)) | ((s_{7}, 0), (s_{1}, 0)) | ((s_{4}, 0), (s_{4}, 0)) | ((s_{8}, 0), (s_{0}, 0)) | |
{{R}}_{8} | ((s_{1}, 0), (s_{7}, 0)) | ((s_{6}, 0), (s_{2}, 0)) | ((s_{7}, 0), (s_{1}, 0)) | ((s_{0}, 0), (s_{8}, 0)) | ((s_{8}, 0), (s_{0}, 0)) | ((s_{2}, 0), (s_{6}, 0)) | |
{{R}}_{9} | ((s_{3}, 0), (s_{5}, 0)) | ((s_{0}, 0), (s_{8}, 0)) | ((s_{5}, 0), (s_{3}, 0)) | ((s_{2}, 0), (s_{6}, 0)) | ((s_{7}, 0), (s_{1}, 0)) | ((s_{0}, 0), (s_{8}, 0)) | |
\Theta_{4} | |||||||
{{R}}_{1} | ((s_{8}, 0), (s_{0}, 0)) | ((s_{2}, 0), (s_{6}, 0)) | ((s_{3}, 0), (s_{5}, 0)) | ((s_{4}, 0), (s_{4}, 0)) | ((s_{5}, 0), (s_{3}, 0)) | ((s_{6}, 0), (s_{2}, 0)) | |
{{R}}_{2} | ((s_{2}, 0), (s_{6}, 0)) | ((s_{6}, 0), (s_{2}, 0)) | ((s_{0}, 0), (s_{8}, 0)) | ((s_{3}, 0), (s_{5}, 0)) | ((s_{8}, 0), (s_{0}, 0)) | ((s_{5}, 0), (s_{3}, 0)) | |
{{R}}_{3} | ((s_{3}, 0), (s_{5}, 0)) | ((s_{7}, 0), (s_{1}, 0)) | ((s_{8}, 0), (s_{0}, 0)) | ((s_{6}, 0), (s_{2}, 0)) | ((s_{1}, 0), (s_{7}, 0)) | ((s_{8}, 0), (s_{0}, 0)) | |
{{R}}_{4} | ((s_{4}, 0), (s_{4}, 0)) | ((s_{5}, 0), (s_{3}, 0)) | ((s_{1}, 0), (s_{7}, 0)) | ((s_{0}, 0), (s_{8}, 0)) | ((s_{6}, 0), (s_{2}, 0)) | ((s_{2}, 0), (s_{6}, 0)) | |
{{R}}_{5} | ((s_{1}, 0), (s_{7}, 0)) | ((s_{0}, 0), (s_{8}, 0)) | ((s_{4}, 0), (s_{4}, 0)) | ((s_{2}, 0), (s_{6}, 0)) | ((s_{7}, 0), (s_{1}, 0)) | ((s_{3}, 0), (s_{5}, 0)) | |
{{R}}_{6} | ((s_{5}, 0), (s_{3}, 0)) | ((s_{8}, 0), (s_{0}, 0)) | ((s_{2}, 0), (s_{6}, 0)) | ((s_{1}, 0), (s_{7}, 0)) | ((s_{0}, 0), (s_{8}, 0)) | ((s_{4}, 0), (s_{4}, 0)) | |
{{R}}_{7} | ((s_{7}, 0), (s_{1}, 0)) | ((s_{1}, 0), (s_{7}, 0)) | ((s_{5}, 0), (s_{3}, 0)) | ((s_{8}, 0), (s_{0}, 0)) | ((s_{4}, 0), (s_{4}, 0)) | ((s_{7}, 0), (s_{1}, 0)) | |
{{R}}_{8} | ((s_{6}, 0), (s_{2}, 0)) | ((s_{4}, 0), (s_{4}, 0)) | ((s_{7}, 0), (s_{1}, 0)) | ((s_{5}, 0), (s_{3}, 0)) | ((s_{3}, 0), (s_{5}, 0)) | ((s_{1}, 0), (s_{7}, 0)) | |
{{R}}_{9} | ((s_{0}, 0), (s_{8}, 0)) | ((s_{3}, 0), (s_{5}, 0)) | ((s_{6}, 0), (s_{2}, 0)) | ((s_{7}, 0), (s_{1}, 0)) | ((s_{2}, 0), (s_{6}, 0)) | ((s_{0}, 0), (s_{8}, 0)) |
{{G}}_{1} | {{G}}_{2} | {{G}}_{3} | |
{{R}}_{1} | ((s_7, -0.3891), (s_4, -0.2277)) | ((s_6, 0.0997), (s_4, -0.0756)) | ((s_7, -0.1965), (s_3, 0.2730)) |
{{R}}_{2} | ((s_6, 0.0081), (s_4, 0.1537)) | ((s_7, 0.3642), (s_2, 0.1177)) | ((s_0, 0), (s_8, 0)) |
{{R}}_{3} | ((s_7, -0.0292), (s_3, -0.1356)) | ((s_6, 0.4762), (s_4, 0.1480)) | ((s_8, 0), (s_0, 0)) |
{{R}}_{4} | ((s_0, 0), (s_8, 0)) | ((s_7, 0.0485), (s_2, 0.2979)) | ((s_5, 0.4993), (s_5, -0.3450)) |
{{R}}_{5} | ((s_7, 0.0101), (s_3, 0.0473)) | ((s_5, -0.3156), (s_7, -0.1305)) | ((s_7, -0.3557), (s_4, -0.3383)) |
{{R}}_{6} | ((s_7, -0.0726), (s_3, -0.1504)) | ((s_7, 0.0101), (s_3, 0.2390)) | ((s_0, 0), (s_8, 0)) |
{{R}}_{7} | ((s_5, 0.3996), (s_6, 0.0833)) | ((s_4, -0.1232), (s_7, 0.0370)) | ((s_7, 0.3708), (s_2, -0.1898)) |
{{R}}_{8} | ((s_7, -0.4816), (s_4, -0.3182)) | ((s_7, 0.2618), (s_2, 0.3874)) | ((s_7, 0.3997), (s_2, -0.1125)) |
{{R}}_{9} | ((s_5, -0.0390), (s_7, -0.2683)) | ((s_0, 0), (s_8, 0)) | ((s_7, 0.1479), (s_2, 0.1867)) |
{{G}}_{4} | {{G}}_{5} | {{G}}_{6} | |
{{R}}_{1} | ((s_6, 0.2466), (s_4, -0.1424)) | ((s_5, -0.1877), (s_7, -0.4491)) | ((s_7, 0.2954), (s_3, -0.1998)) |
{{R}}_{2} | ((s_7, 0.2201), (s_3, -0.4669)) | ((s_6, -0.0280), (s_6, -0.0258)) | ((s_7, -0.1752), (s_3, -0.0620)) |
{{R}}_{3} | ((s_6, -0.2876), (s_6, -0.3069)) | ((s_6, -0.0466), (s_4, -0.2966)) | ((s_6, -0.0874), (s_6, -0.0255)) |
{{R}}_{4} | ((s_5, 0.2866), (s_7, -0.3760)) | ((s_6, 0.0168), (s_4, 0.4795)) | ((s_6, 0.4588), (s_4, -0.4168)) |
{{R}}_{5} | ((s_5, -0.3423), (s_7, -0.3634)) | ((s_5, -0.1427), (s_7, -0.4539)) | ((s_7, -0.2352), (s_3, -0.0154)) |
{{R}}_{6} | ((s_7, -0.1643), (s_3, 0.4871)) | ((s_5, -0.3171), (s_7, -0.2974)) | ((s_7, -0.4421), (s_3, 0.3799)) |
{{R}}_{7} | ((s_7, 0.3384), (s_2, 0.3524)) | ((s_7, 0.2766), (s_2, -0.3623)) | ((s_5, 0.2401), (s_7, -0.3311)) |
{{R}}_{8} | ((s_5, -0.4488), (s_7, -0.3605)) | ((s_7, 0.4941, (s_2, -0.0552)) | ((s_5, 0.4849), (s_5, -0.1040)) |
{{R}}_{9} | ((s_6, 0.0488), (s_4, 0.3846)) | ((s_7, 0.0848), (s_3, -0.0375)) | ((s_0, 0), (s_8, 0)) |
{{G}}_{1} | {{G}}_{2} | {{G}}_{3} | |
{{R}}_{1} | ((s_6, 0.0539), (s_5, -0.0285)) | ((s_6, -0.2441), (s_5, -0.3317)) | ((s_7, -0.4061), (s_4, -0.2107)) |
{{R}}_{2} | ((s_5, 0.4486), (s_5, 0.2840)) | ((s_7, 0.0878), (s_3, -0.0722)) | ((s_0, 0), (s_8, 0)) |
{{R}}_{3} | ((s_6, 0.4378), (s_4, 0.1766)) | ((s_6, 0.1349), (s_5, -0.1320)) | ((s_8, 0), (s_0, 0)) |
{{R}}_{4} | ((s_0, 0), (s_8, 0)) | ((s_7, -0.2648), (s_3, 0.1142)) | ((s_5, 0.2848), (s_5, 0.0870)) |
{{R}}_{5} | ((s_6, 0.4812), (s_4, 0.3434)) | ((s_4, 0.3848), (s_7, 0.1293)) | ((s_6, 0.4298), (s_4, 0.1621)) |
{{R}}_{6} | ((s_6, 0.3903), (s_4, 0.1630)) | ((s_7, -0.3064), (s_4, 0.0374)) | ((s_0, 0), (s_8, 0)) |
{{R}}_{7} | ((s_5, -0.1340), (s_7, 0.2730)) | ((s_4, -0.3785), (s_7, 0.2604)) | ((s_7, 0.1988), (s_2, 0.3094)) |
{{R}}_{8} | ((s_6, -0.0415), (s_5, -0.1043)) | ((s_7, -0.0291), (s_3, 0.2056)) | ((s_7, 0.2309), (s_2, 0.3916)) |
{{R}}_{9} | ((s_4, 0.4567), (s_7, 0.1722)) | ((s_0, 0), (s_8, 0)) | ((s_7, -0.0436), (s_3, -0.2953)) |
{{G}}_{4} | {{G}}_{5} | {{G}}_{6} | |
{{R}}_{1} | ((s_6, -0.4600), (s_5, 0.3085)) | ((s_4, 0.2348), (s_7, 0.1206)) | ((s_7, -0.1581), (s_4, 0.0392)) |
{{R}}_{2} | ((s_7, -0.4190), (s_4, 0.1904)) | ((s_5, 0.3148), (s_7, -0.2517)) | ((s_6, 0.3139), (s_4, 0.1675)) |
{{R}}_{3} | ((s_5, 0.0255), (s_7, -0.3929)) | ((s_5, 0.2969), (s_6, -0.4309)) | ((s_5, 0.3897), (s_7, -0.3847)) |
{{R}}_{4} | ((s_5, -0.3703), (s_7, 0.1945)) | ((s_5, 0.3582), (s_6, -0.2940)) | ((s_6, -0.0676), (s_, -0.2575)) |
{{R}}_{5} | ((s_4, 0.0599), (s_7, 0.2022)) | ((s_4, 0.2756), (s_7, 0.1176)) | ((s_6, 0.2501), (s_4, 0.2387)) |
{{R}}_{6} | ((s_6, 0.1459), (s_5, 0.0155)) | ((s_4, 0.1177), (s_7, 0.2162)) | ((s_6, 0.0339), (s_5, -0.4345)) |
{{R}}_{7} | ((s_7, -0.2744), (s_4, 0.0196)) | ((s_7, -0.3088), (s_4, -0.0700)) | ((s_5, -0.2523), (s_7, 0.1062)) |
{{R}}_{8} | ((s_4, -0.0354), (s_7, 0.2039)) | ((s_7, -0.0325), (s_4, -0.4910)) | ((s_5, -0.0211), (s_6, -0.1888)) |
{{R}}_{9} | ((s_5, 0.3467), (s_6, -0.2952)) | ((s_6, 0.2762), (s_4, 0.4843)) | ((s_0, 0), (s_8, 0)) |
{{R}}_{1} | {{R}}_{2} | {{R}}_{3} | {{R}}_{4} | {{R}}_{5} | {{R}}_{6} | {{R}}_{7} | {{R}}_{8} | {{R}}_{9} | |
{{R}}_{1} | 0 | 0.8665 | 0.0101 | 1.6448 | 1.5077 | 1.2851 | 0.8915 | -0.3694 | 2.7643 |
{{R}}_{2} | -0.8665 | 0 | -0.9769 | 0.7783 | 0.6413 | 0.4187 | 0.0251 | -1.2359 | 1.8979 |
{{R}}_{3} | -0.0101 | 0.9769 | 0 | 1.7553 | 1.6182 | 1.3956 | 1.0020 | -0.2589 | 2.8748 |
{{R}}_{4} | -1.6448 | -0.7783 | -1.7553 | 0 | -0.1371 | -0.3597 | -0.7533 | -2.0142 | 1.1195 |
{{R}}_{5} | -1.5077 | -0.6413 | -1.6182 | 0.1371 | 0 | -0.2226 | -0.6162 | -1.8771 | 1.2566 |
{{R}}_{6} | -1.2851 | -0.4187 | -1.3956 | 0.3597 | 0.2226 | 0 | -0.3936 | -1.6545 | 1.4792 |
{{R}}_{7} | -0.8915 | -0.0251 | -1.0020 | 0.7533 | 0.6162 | 0.3936 | 0 | -1.2609 | 1.8728 |
{{R}}_{8} | 0.3694 | 1.2359 | 0.2589 | 2.0142 | 1.8771 | 1.6545 | 1.2609 | 0 | 3.1337 |
{{R}}_{9} | -2.7643 | -1.8979 | -2.8748 | -1.1195 | -1.2566 | -1.4792 | -1.8728 | -3.1337 | 0 |
{{G}}_{1} | {{G}}_{2} | {{G}}_{3} | |
{{R}}_{1} | ((s_4, 0.3805), (s_7, 0.1469)) | ((s_4, 0.0216), (s_7, 0.1384)) | ((s_3, 0.4930), (s_8, -0.3496)) |
{{R}}_{2} | ((s_4, -0.1115), (s_7, 0.2509)) | ((s_5, 0.2350), (s_6, 0.4675)) | ((s_0, 0), (s_8, 0)) |
{{R}}_{3} | ((s_5, -0.2826), (s_7, -0.1423)) | ((s_4, 0.3307), (s_7, 0.2019)) | ((s_8, 0), (s_0, 0)) |
{{R}}_{4} | ((s_0, 0), (s_8, 0)) | ((s_5, -0.1305), (s_7, -0.4475)) | ((s_3, -0.3221), (s_8, -0.2137)) |
{{R}}_{5} | ((s_5, -0.2428), (s_7, -0.0783)) | ((s_3, 0.0013), (s_8, -0.1927)) | ((s_3, 0.3777), (s_8, -0.3066)) |
{{R}}_{6} | ((s_5, -0.3257), (s_7, -0.1476)) | ((s_5, -0.1706), (s_7, -0.0775)) | ((s_0, 0), (s_8, 0)) |
{{R}}_{7} | ((s_3, 0.4426), (s_8, -0.3220)) | ((s_2, 0.4665), (s_8, -0.1625)) | ((s_4, 0.0118), (s_7, 0.4271)) |
{{R}}_{8} | ((s_4, 0.3003), (s_7, 0.1209)) | ((s_5, 0.1080), (s_7, -0.4073)) | ((s_4, 0.0193), (s_7, 0.4427)) |
{{R}}_{9} | ((s_3, 0.1395), (s_8, 0.2045)) | ((s_0, 0), (s_8, 0)) | ((s_4, -0.2276), (s_7, 0.4976)) |
{{G}}_{4} | {{G}}_{5} | {{G}}_{6} | |
{{R}}_{1} | ((s_5, -0.3960), (s_7, -0.3335)) | ((s_4, -0.3952), (s_8, -0.4655)) | ((s_5, -0.4962), (s_7, 0.2788)) |
{{R}}_{2} | ((s_6, -0.4103), (s_6, 0.0011)) | ((s_5, -0.4401), (s_7, 0.3290)) | ((s_4, 0.0492), (s_7, 0.3103)) |
{{R}}_{3} | ((s_4, 0.1512), (s_7, 0.3477)) | ((s_5, -0.4562), (s_7, -0.3610)) | ((s_3, 0.3673), (s_8, -0.2075)) |
{{R}}_{4} | ((s_4, -0.1886), (s_8, -0.3687)) | ((s_5, -0.4008), (s_7, -0.2775)) | ((s_4, -0.2445), (s_7, 0.4421)) |
{{R}}_{5} | ((s_3, 0.3309), (s_8, -0.3651)) | ((s_4, -0.3597), (s_8, -0.4672)) | ((s_4, -0.0015), (s_7, 0.3274)) |
{{R}}_{6} | ((s_5, 0.1621), (s_7, -0.4997)) | ((s_4, -0.4967), (s_8, -0.4136)) | ((s_4, -0.1684), (s_7, 0.4031)) |
{{R}}_{7} | ((s_6, -0.2606), (s_6, 0.1089)) | ((s_6, -0.1166), (s_6, -0.4517)) | ((s_3, -0.0639), (s_8, -0.1300)) |
{{R}}_{8} | ((s_3, 0.2513), (s_8, -0.3643)) | ((s_6, 0.1821), (s_5, 0.2339)) | ((s_3, 0.0888), (s_8, -0.3458)) |
{{R}}_{9} | ((s_4, 0.4321), (s_7, -0.1166)) | ((s_5, 0.4627), (s_6, 0.0617)) | ((s_0, 0), (s_8, 0)) |
{{R}}_{1} | {{R}}_{2} | {{R}}_{3} | {{R}}_{4} | {{R}}_{5} | {{R}}_{6} | {{R}}_{7} | {{R}}_{8} | {{R}}_{9} | |
{{R}}_{1} | 0 | 0.8665 | 0.0101 | 1.6448 | 1.5077 | 1.2851 | 0.8915 | -0.3694 | 2.7643 |
{{R}}_{2} | -0.8665 | 0 | -0.9769 | 0.7783 | 0.6413 | 0.4187 | 0.0251 | -1.2359 | 1.8979 |
{{R}}_{3} | -0.0101 | 0.9769 | 0 | 1.7553 | 1.6182 | 1.3956 | 1.0020 | -0.2589 | 2.8748 |
{{R}}_{4} | -1.6448 | -0.7783 | -1.7553 | 0 | -0.1371 | -0.3597 | -0.7533 | -2.0142 | 1.1195 |
{{R}}_{5} | -1.5077 | -0.6413 | -1.6182 | 0.1371 | 0 | -0.2226 | -0.6162 | -1.8771 | 1.2566 |
{{R}}_{6} | -1.2851 | -0.4187 | -1.3956 | 0.3597 | 0.2226 | 0 | -0.3936 | -1.6545 | 1.4792 |
{{R}}_{7} | -0.8915 | -0.0251 | -1.0020 | 0.7533 | 0.6162 | 0.3936 | 0 | -1.2609 | 1.8728 |
{{R}}_{8} | 0.3694 | 1.2359 | 0.2589 | 2.0142 | 1.8771 | 1.6545 | 1.2609 | 0 | 3.1337 |
{{R}}_{9} | -2.7643 | -1.8979 | -2.8748 | -1.1195 | -1.2566 | -1.4792 | -1.8728 | -3.1337 | 0 |
{{G}}_{1} | {{G}}_{2} | {{G}}_{3} | |
{{R}}_{1} | ((s_6, -0.0961), (s_6, -0.3603)) | ((s_2, 0.1718), (s_7, 0.1783)) | ((s_4, 0.0624), (s_6, 0.2396)) |
{{R}}_{2} | ((s_3, -0.3998), (s_7, 0.0990)) | ((s_5, -0.2385), (s_5, 0.4972)) | ((s_1, 0.4422), (s_8, -0.2737)) |
{{R}}_{3} | ((s_4, 0.2422), (s_6, 0.0676)) | ((s_4, 0.3482), (s_6, 0.1491)) | ((s_8, 0), (s_0, 0)) |
{{R}}_{4} | ((s_6, -0.1292), (s_6, -0.1601)) | ((s_3, 0.4843), (s_6, 0.3538)) | ((s_2, -0.4878), (s_7, 0.4843)) |
{{R}}_{5} | ((s_7, -0.0444), (s_4, 0.2884)) | ((s_6, 0.3337), (s_6, -0.4017)) | ((s_6, -0.0684), (s_5, -0.4954)) |
{{R}}_{6} | ((s_4, -0.2807), (s_6, 0.3164)) | ((s_7, -0.3151), (s_5, -0.0977)) | ((s_1, -0.0599), (s_8, -0.2005)) |
{{R}}_{7} | ((s_4, 0.0689), (s_6, 0.2841)) | ((s_2, 0.0635, (s_7, 0.4490)) | ((s_4, 0.4322), (s_6, -0.2882)) |
{{R}}_{8} | ((s_3, 0.4526), (s_7, -0.3928)) | ((s_6, 0.4542), (s_5, -0.110)) | ((s_5, -0.2896), (s_6, -0.4666)) |
{{R}}_{9} | ((s_5, 0.2397), (s_6, 0.3559)) | ((s_5, 0.3566), (s_6, 0.1203)) | ((s_4, -0.2063), (s_6, 0.1743)) |
{{G}}_{4} | {{G}}_{5} | {{G}}_{6} | |
{{R}}_{1} | ((s_3, -0.2832), (s_7, -0.0381)) | ((s_3, 0.2726), (s_7, -0.1462)) | ((s_8, 0), (s_0, 0)) |
{{R}}_{2} | ((s_6, 0.4719), (s_5, -0.0714)) | ((s_7, -0.3232), (s_5, -0.1548)) | ((s_3, 0.3950), (s_6, 0.4988)) |
{{R}}_{3} | ((s_4, -0.2593), (s_7, -0.4324)) | ((s_3, -0.4913), (s_7, 0.1456)) | ((s_7, -0.1266), (s_5, -0.3632)) |
{{R}}_{4} | ((s_4, 0.4649), (s_6, 0.3020)) | ((s_3, 0.3650), (s_7, -0.1510)) | ((s_3, 0.1467), (s_7, -0.2130)) |
{{R}}_{5} | ((s_3, -0.0851), (s_7, 0.0082)) | ((s_4, -0.4871), (s_7, -0.3113)) | ((s_4, -0.4392), (s_6, 0.4802)) |
{{R}}_{6} | ((s_8, 0), (s_0, 0) | ((s_2, 0.3524), (s_7, 0.3384)) | ((s_3, 0.1466), (s_7, -0.2670)) |
{{R}}_{7} | ((s_7, -0.2974), (s_5, -0.3171)) | ((s_6, 0.4789), (s_5, -0.1396)) | ((s_7, -0.1059), (s_5, -0.3389)) |
{{R}}_{8} | ((s_3, -0.4455), (s_7, 0.1303)) | ((s_8, 0, (s_0, 0) | ((s_3, -0.2232), (s_7, 0.1423)) |
{{R}}_{9} | ((s_3, 0.1835), (s_7, 0.1663)) | ((s_4, 0.1105), (s_6, 0.1671)) | ((s_2, 0.1042), (s_7, 0.4790)) |
{{G}}_{1} | {{G}}_{2} | {{G}}_{3} | |
{{R}}_{1} | ((s_7, -0.3993), (s_5, 0.0934)) | ((s_3, -0.0159), (s_7, -0.1225)) | ((s_5, -0.4604), (s_6, 0.0193)) |
{{R}}_{2} | ((s_4, -0.0714), (s_7, -0.4190)) | ((s_5, 0.4034), (s_5, 0.1645)) | ((s_2, -0.0902), (s_8, -0.3910)) |
{{R}}_{3} | ((s_5, 0.3549), (s_6, -0.4932)) | ((s_5, 0.0447), (s_6, -0.1950)) | ((s_8, 0), (s_0, 0)) |
{{R}}_{4} | ((s_7, -0.4227), (s_5, 0.2854)) | ((s_4, 0.2667), (s_6, 0.0106)) | ((s_2, -0.0130), (s_7, 0.3257)) |
{{R}}_{5} | ((s_7, 0.3222), (s_4, -0.1602)) | ((s_7, -0.2953), (s_5, 0.2628)) | ((s_6, 0.2297), (s_5, 0.2811)) |
{{R}}_{6} | ((s_5, -0.0728), (s_6, -0.2464)) | ((s_7, -0.0160), (s_5, -0.4076)) | ((s_1, 0.3354), (s_8, -0.2997)) |
{{R}}_{7} | ((s_5, 0.2154), (s_6, -0.2788)) | ((s_3, -0.1290), (s_7, 0.1869)) | ((s_5, -0.1174), (s_5, 0.4940)) |
{{R}}_{8} | ((s_5, -0.2994), (s_6, 0.0500)) | ((s_7, -0.1991), (s_5, -0.4203)) | ((s_5, 0.1376), (s_5, 0.3183)) |
{{R}}_{9} | ((s_6, 0.1205), (s_6, -0.2067)) | ((s_6, -0.0101), (s_6, -0.2236)) | ((s_4, 0.2872), (s_6, -0.0462)) |
{{G}}_{4} | {{G}}_{5} | {{G}}_{6} | |
{{R}}_{1} | ((s_4, 0.3587), (s_6, 0.2841)) | ((s_5, -0.2479), (s_6, 0.2093)) | ((s_8, 0), (s_0, 0)) |
{{R}}_{2} | ((s_7, 0.1011), (s_4, 0.3037)) | ((s_7, 0.2000), (s_5, -0.4528)) | ((s_5, -0.4211), (s_6, -0.0267)) |
{{R}}_{3} | ((s_5, 0.2174), (s_6, -0.1365)) | ((s_4, 0.0702), (s_7, -0.4642)) | ((s_7, 0.2473), (s_4, 0.0.1865)) |
{{R}}_{4} | ((s_6, -0.2367), (s_6, 0.4050)) | ((s_5, -0.1702), (s_6, 0.2042)) | ((s_4, 0.3580), (s_6, 0.2737)) |
{{R}}_{5} | ((s_5, -0.4653), (s_6, 0.3357)) | ((s_5, -0.0475), (s_6, 0.0333)) | ((s_5, -0.2768), (s_6, -0.0457)) |
{{R}}_{6} | ((s_8, 0), (s_0, 0) | ((s_4, -0.0795), (s_7, -0.2330)) | ((s_4, 0.3579), (s_6, 0.2165)) |
{{R}}_{7} | ((s_7, 0.2423), (s_4, 0.0825)) | ((s_7, 0.0749), (s_4, 0.2785)) | ((s_7, 0.2615), (s_4, 0.2088)) |
{{R}}_{8} | ((s_4, 0.2103), (s_6, 0.4752)) | ((s_8, 0), (s_0, 0)) | ((s_4, 0.0172), (s_7, -0.3362)) |
{{R}}_{9} | ((s_5, -0.2350), (s_6, 0.1438)) | ((s_5, 0.4272), (s_6, -0.4952)) | ((s_3, 0.3536), (s_7, 0.0678)) |
{{R}}_{1} | {{R}}_{2} | {{R}}_{3} | {{R}}_{4} | {{R}}_{5} | {{R}}_{6} | {{R}}_{7} | {{R}}_{8} | {{R}}_{9} | |
{{R}}_{1} | 0 | 0.3827 | -0.7907 | 1.2319 | -0.3662 | 0.3409 | -0.8652 | -0.3901 | 1.0152 |
{{R}}_{2} | -0.3827 | 0 | -1.1735 | 0.8492 | -0.7489 | -0.0142 | -1.2480 | -0.7729 | 0.6325 |
{{R}}_{3} | 0.7907 | 1.1735 | 0 | 2.0226 | 0.4245 | 1.1316 | -0.0745 | 0.4006 | 1.8059 |
{{R}}_{4} | -1.2319 | -0.8492 | -2.0226 | 0 | -1.5981 | -0.8910 | -2.0972 | -1.6220 | -0.2167 |
{{R}}_{5} | 0.3662 | 0.7489 | -0.4245 | 1.5981 | 0 | 0.7071 | -0.4990 | -0.0239 | 1.3814 |
{{R}}_{6} | -0.3409 | 0.0142 | -1.1316 | 0.8910 | -0.7071 | 0 | -1.2061 | -0.7310 | 0.6743 |
{{R}}_{7} | 0.8652 | 1.2480 | 0.0745 | 2.0972 | 0.4990 | 1.2061 | 0 | 0.4751 | 1.8804 |
{{R}}_{8} | 0.3901 | 0.7729 | -0.4006 | 1.6220 | 0.0239 | 0.7310 | -0.4751 | 0 | 1.4053 |
{{R}}_{9} | -1.0152 | -0.6325 | -1.8059 | 0.2167 | -1.3814 | -0.6743 | -1.8804 | -1.4053 | 0 |
{{G}}_{1} | {{G}}_{2} | {{G}}_{3} | |
{{R}}_{1} | ((s_8, -0.3564), (s_4, -0.3858)) | ((s_6, 0.4936), (s_5, 0.0110)) | ((s_8, -0.2665), (s_3, 0.1101)) |
{{R}}_{2} | ((s_7, -0.2411), (s_5, -0.1498)) | ((s_7, 0.3627), (s_4, -0.4322)) | ((s_7, 0.3432), (s_4, 0.4644)) |
{{R}}_{3} | ((s_7, 0.2739), (s_4, 0.0657)) | ((s_7, 0.2565), (s_4, 0.0607)) | ((s_8, 0), (s_0, 0)) |
{{R}}_{4} | ((s_8, -0.3629), (s_4, -0.2386)) | ((s_7, 0.0038), (s_4, 0.2273)) | ((s_7, 0.3607), (s_4, 0.1157)) |
{{R}}_{5} | ((s_8, -0.1661), (s_3, -0.3069)) | ((s_8, -0.2934), (s_4, -0.3587)) | ((s_8, -0.1188), (s_3, -0.3242)) |
{{R}}_{6} | ((s_7, 0.1317), (s_4, 0.1317)) | ((s_8, -0.2266), (s_3, 0.1496)) | ((s_7, 0.1878), (s_5, -0.3895)) |
{{R}}_{7} | ((s_7, -0.2285), (s_4, 0.1055)) | ((s_6, 0.4407), (s_5, 0.3486)) | ((s_8, -0.2328), (s_3, -0.2034)) |
{{R}}_{8} | ((s_7, 0.0526), (s_4, 0.3772)) | ((s_8, -0.2702), (s_3, 0.1405)) | ((s_8, -0.2091), (s_3, -0.3033)) |
{{R}}_{9} | ((s_8, -0.4920), (s_4, 0.1640)) | ((s_8, -0.4750), (s_4, 0.0379)) | ((s_8, -0.2929), (s_3, 0.0695)) |
{{G}}_{4} | {{G}}_{5} | {{G}}_{6} | |
{{R}}_{1} | ((s_6, 0.1071), (s_5, 0.2954)) | ((s_6, 0.1183), (s_5, 0.3971)) | ((s_8, 0), (s_0, 0)) |
{{R}}_{2} | ((s_8, -0.4129), (s_4, 0.4646)) | ((s_8, 0.4224), (s_4, -0.1227)) | ((s_7, 0.4061), (s_4, -0.2140)) |
{{R}}_{3} | ((s_7, 0.3846), (s_5, -0.1023)) | ((s_6, -0.3507), (s_6, -0.2773)) | ((s_8, -0.1085), (s_3, -0.4259)) |
{{R}}_{4} | ((s_7, -0.0854), (s_5, 0.3467)) | ((s_6, 0.1696), (s_5, 0.3921)) | ((s_7, 0.3556), (s_4, 0.0171)) |
{{R}}_{5} | ((s_6, 0.2155), (s_5, 0.3451)) | ((s_6, 0.2498), (s_5, 0.2271)) | ((s_7, 0.4379), (s_4, -0.2282)) |
{{R}}_{6} | ((s_8, 0), (s_0, 0)) | ((s_6, -0.4586), (s_6, -0.0364)) | ((s_7, 0.3556), (s_4, -0.0279)) |
{{R}}_{7} | ((s_8, -0.3462), (s_3, 0.3498)) | ((s_8, -0.4904), (s_4, -0.3572)) | ((s_8, -0.1064), (s_3, -0.4116)) |
{{R}}_{8} | ((s_6, 0.0137), (s_5, 0.4829)) | ((s_8, 0), (s_0, 0)) | ((s_7, 0.2733), (s_4, 0.3421)) |
{{R}}_{9} | ((s_6, 0.3539), (s_5, 0.1601)) | ((s_7, -0.4486), (s_5, 0.2670)) | ((s_7, 0.0940), (s_5, -0.2737)) |
{{R}}_{1} | {{R}}_{2} | {{R}}_{3} | {{R}}_{4} | {{R}}_{5} | {{R}}_{6} | {{R}}_{7} | {{R}}_{8} | {{R}}_{9} | |
{{R}}_{1} | 0 | -0.6860 | -0.2921 | 0.0886 | -0.5449 | -0.3772 | -1.0436 | -0.7952 | -0.0798 |
{{R}}_{2} | 0.6860 | 0 | 0.3939 | 0.7745 | 0.1410 | 0.3088 | -0.3576 | -0.1093 | 0.6062 |
{{R}}_{3} | 0.2921 | -0.3939 | 0 | 0.3807 | -0.2529 | -0.0851 | -0.7515 | -0.5032 | 0.2123 |
{{R}}_{4} | -0.0886 | -0.7745 | -0.3807 | 0 | -0.6335 | -0.4658 | -1.1322 | -0.8838 | -0.1684 |
{{R}}_{5} | 0.5449 | -0.1410 | 0.2529 | 0.6335 | 0 | 0.1677 | -0.4987 | -0.2503 | 0.4652 |
{{R}}_{6} | 0.3772 | -0.3088 | 0.0851 | 0.4658 | -0.1677 | 0 | -0.6664 | -0.4180 | 0.2974 |
{{R}}_{7} | 1.0436 | 0.3576 | 0.7515 | 1.1322 | 0.4987 | 0.6664 | 0 | 0.2484 | 0.9638 |
{{R}}_{8} | 0.7952 | 0.1093 | 0.5032 | 0.8838 | 0.2503 | 0.4180 | -0.2484 | 0 | 0.7155 |
{{R}}_{9} | 0.0798 | -0.6062 | -0.2123 | 0.1684 | -0.4652 | -0.2974 | -0.9638 | -0.7155 | 0 |
Parameter | £_{1} | £_{2} | £_{3} | £_{4} | £_{5} | £_{6} | £_{7} | £_{8} | £_{9} |
q=1 | 9.2419 | 0.4522 | 8.0325 | -5.5686 | -5.6983 | -2.6495 | -0.7889 | 12.5685 | -15.5897 |
q=2 | 8.3757 | 0.8741 | 8.8384 | -6.4119 | -5.5053 | -2.9184 | 0.4589 | 12.1618 | -15.8735 |
q=3 | 8.6811 | 0.4570 | 9.9984 | -6.2013 | -4.6643 | -3.2330 | 0.3786 | 11.4614 | -16.8780 |
q=4 | 9.2018 | 0.1342 | 10.9091 | -6.9945 | -3.6352 | -3.6145 | 0.4292 | 11.1241 | -17.5541 |
q=5 | 9.0140 | -0.0512 | 11.6425 | -7.1200 | -3.0797 | -4.0100 | 0.5400 | 11.0921 | -18.0277 |
q=6 | 9.4112 | -0.4079 | 12.0041 | -7.2024 | -2.5031 | -4.4406 | 0.7780 | 10.7475 | -18.3869 |
q=7 | 9.0445 | -0.8170 | 12.9281 | -7.2637 | -1.8192 | -4.8432 | 0.9374 | 10.5033 | -18.6703 |
q=8 | 9.0544 | -0.9737 | 13.3726 | -7.3163 | -1.3976 | -5.2068 | 1.1006 | 10.2916 | -18.9248 |
Parameter | £_{1} | £_{2} | £_{3} | £_{4} | £_{5} | £_{6} | £_{7} | £_{8} | £_{9} |
{\kappa}=1 | -1.2161 | -0.3893 | 1.6164 | -6.7267 | 5.3976 | -7.5968 | 8.8638 | 0.7522 | -0.7012 |
{\kappa}=2 | 6.3461 | -1.8035 | 8.3705 | -7.0144 | -3.2918 | 0.3788 | 2.6722 | 9.5008 | -15.1586 |
{\kappa}=3 | 8.6007 | 0.6820 | 9.3537 | -6.3231 | -5.0894 | -3.0861 | 0.4563 | 11.8046 | -16.3987 |
{\kappa}=4 | 11.5855 | 2.7295 | 1.3611 | -2.0245 | -11.4463 | 0.5527 | -6.1084 | 15.4832 | -12.1329 |
Parameter | Ranking |
q=1 | {{R}}_{8} > {{R}}_{1} > {{R}}_{3} > {{R}}_{2} > {{R}}_{7} > {{R}}_{6} > {{R}}_{5} > {{R}}_{4} > {{R}}_{9} |
q=2 | {{R}}_{8} > {{R}}_{3} > {{R}}_{1} > {{R}}_{2} > {{R}}_{7} > {{R}}_{6} > {{R}}_{5} > {{R}}_{4} > {{R}}_{9} |
q=3 | {{R}}_{8} > {{R}}_{3} > {{R}}_{1} > {{R}}_{2} > {{R}}_{7} > {{R}}_{6} > {{R}}_{5} > {{R}}_{4} > {{R}}_{9} |
q=4 | {{R}}_{8} > {{R}}_{3} > {{R}}_{1} > {{R}}_{7} > {{R}}_{2} > {{R}}_{6} > {{R}}_{5} > {{R}}_{4} > {{R}}_{9} |
q=5 | {{R}}_{8} > {{R}}_{3} > {{R}}_{1} > {{R}}_{7} > {{R}}_{2} > {{R}}_{5} > {{R}}_{6} > {{R}}_{4} > {{R}}_{9} |
q=6 | {{R}}_{8} > {{R}}_{3} > {{R}}_{1} > {{R}}_{7} > {{R}}_{2} > {{R}}_{5} > {{R}}_{6} > {{R}}_{4} > {{R}}_{9} |
q=7 | {{R}}_{8} > {{R}}_{3} > {{R}}_{1} > {{R}}_{7} > {{R}}_{2} > {{R}}_{5} > {{R}}_{6} > {{R}}_{4} > {{R}}_{9} |
q=8 | {{R}}_{8} > {{R}}_{3} > {{R}}_{1} > {{R}}_{7} > {{R}}_{2} > {{R}}_{5} > {{R}}_{6} > {{R}}_{4} > {{R}}_{9} |
Parameter | Ranking |
{\kappa} =1 | {{R}}_{7} > {{R}}_{5} > {{R}}_{3} > {{R}}_{8} > {{R}}_{2} > {{R}}_{9} > {{R}}_{1} > {{R}}_{4} > {{R}}_{6} |
{\kappa} =2 | {{R}}_{8} > {{R}}_{3} > {{R}}_{1} > {{R}}_{7} > {{R}}_{6} > {{R}}_{2} > {{R}}_{5} > {{R}}_{4} > {{R}}_{9} |
{\kappa} =3 | {{R}}_{8} > {{R}}_{3} > {{R}}_{1} > {{R}}_{2} > {{R}}_{7} > {{R}}_{6} > {{R}}_{5} > {{R}}_{4} > {{R}}_{9} |
{\kappa} =4 | {{R}}_{8} > {{R}}_{1} > {{R}}_{2} > {{R}}_{3} > {{R}}_{6} > {{R}}_{4} > {{R}}_{7} > {{R}}_{5} > {{R}}_{9} |
Parameter | £_{1} | £_{2} | £_{3} | £_{4} | £_{5} | £_{6} | £_{7} | £_{8} | £_{9} |
q=1 | 0.1530 | -3.1792 | 7.1507 | -12.0965 | 5.5735 | -1.8540 | 8.0698 | 3.1966 | -7.0140 |
q=2 | 0.1057 | -2.9132 | 7.8509 | -11.0315 | 4.3727 | -2.5697 | 8.5268 | 3.7767 | -8.1183 |
q=3 | 1.0370 | -2.8208 | 7.4580 | -10.1699 | 3.4083 | -2.4021 | 8.1027 | 4.3676 | -8.9809 |
q=4 | 2.0292 | -2.8761 | 6.8321 | -9.6699 | 2.5850 | -1.9598 | 7.6085 | 5.0272 | -9.5762 |
q=5 | 2.6374 | -2.9743 | 6.9033 | -9.3430 | 2.0591 | -1.4359 | 7.1377 | 4.9781 | -9.9625 |
q=6 | 3.1882 | -3.1053 | 6.7752 | -9.1052 | 1.5023 | -0.8554 | 6.6762 | 5.1364 | -10.2125 |
q=7 | 3.9542 | -3.2434 | 6.3435 | -8.9285 | 1.0057 | -0.2839 | 6.2217 | 5.2570 | -10.3264 |
q=8 | 4.2588 | -3.3906 | 6.2811 | -8.8002 | 0.5206 | 0.2603 | 5.7110 | 5.4004 | -10.2414 |
Parameter | £_{1} | £_{2} | £_{3} | £_{4} | £_{5} | £_{6} | £_{7} | £_{8} | £_{9} |
{\kappa}=1 | 3.4005 | 2.5863 | 1.4628 | -5.4874 | -8.1530 | 2.2088 | -0.6670 | 12.0452 | -7.3961 |
{\kappa}=2 | 3.7906 | -0.9149 | 11.8515 | -14.4767 | 1.8085 | -0.3653 | 6.5394 | 9.4213 | -17.6544 |
{\kappa}=3 | 0.5583 | -2.8586 | 7.6750 | -10.5288 | 3.8543 | -2.5370 | 8.3456 | 4.0695 | -8.5783 |
{\kappa}=4 | -2.6107 | -3.5564 | 1.8935 | -13.2726 | 12.1627 | -7.5384 | 11.5950 | 0.5158 | 0.8112 |
Parameter | Ranking |
q=1 | {{R}}_{7} > {{R}}_{3} > {{R}}_{5} > {{R}}_{8} > {{R}}_{1} > {{R}}_{6} > {{R}}_{2} > {{R}}_{9} > {{R}}_{4} |
q=2 | {{R}}_{7} > {{R}}_{3} > {{R}}_{5} > {{R}}_{8} > {{R}}_{1} > {{R}}_{6} > {{R}}_{2} > {{R}}_{9} > {{R}}_{4} |
q=3 | {{R}}_{7} > {{R}}_{3} > {{R}}_{8} > {{R}}_{5} > {{R}}_{1} > {{R}}_{6} > {{R}}_{2} > {{R}}_{9} > {{R}}_{4} |
q=4 | {{R}}_{7} > {{R}}_{3} > {{R}}_{8} > {{R}}_{5} > {{R}}_{1} > {{R}}_{6} > {{R}}_{2} > {{R}}_{9} > {{R}}_{4} |
q=5 | {{R}}_{7} > {{R}}_{3} > {{R}}_{8} > {{R}}_{1} > {{R}}_{5} > {{R}}_{6} > {{R}}_{2} > {{R}}_{4} > {{R}}_{9} |
q=6 | {{R}}_{3} > {{R}}_{7} > {{R}}_{8} > {{R}}_{1} > {{R}}_{5} > {{R}}_{6} > {{R}}_{2} > {{R}}_{4} > {{R}}_{9} |
q=7 | {{R}}_{3} > {{R}}_{7} > {{R}}_{8} > {{R}}_{1} > {{R}}_{5} > {{R}}_{6} > {{R}}_{2} > {{R}}_{4} > {{R}}_{9} |
q=8 | {{R}}_{3} > {{R}}_{7} > {{R}}_{8} > {{R}}_{1} > {{R}}_{5} > {{R}}_{6} > {{R}}_{2} > {{R}}_{4} > {{R}}_{9} |
Parameter | Ranking |
{\kappa} =1 | {{R}}_{8} > {{R}}_{1} > {{R}}_{2} > {{R}}_{6} > {{R}}_{3} > {{R}}_{7} > {{R}}_{4} > {{R}}_{9} > {{R}}_{5} |
{\kappa} =2 | {{R}}_{3} > {{R}}_{8} > {{R}}_{7} > {{R}}_{1} > {{R}}_{5} > {{R}}_{6} > {{R}}_{2} > {{R}}_{4} > {{R}}_{9} |
{\kappa} =3 | {{R}}_{7} > {{R}}_{3} > {{R}}_{8} > {{R}}_{5} > {{R}}_{1} > {{R}}_{6} > {{R}}_{2} > {{R}}_{9} > {{R}}_{4} |
{\kappa} =4 | {{R}}_{5} > {{R}}_{7} > {{R}}_{3} > {{R}}_{9} > {{R}}_{8} > {{R}}_{1} > {{R}}_{2} > {{R}}_{6} > {{R}}_{4} |
Alternatives | EDAS | Ranking | CODAS | Ranking | TOPSIS | Ranking |
{{R}}_{1} | 0.6255 | III | 8.6007 | III | 0.6850 | III |
{{R}}_{2} | 0.7340 | II | 0.6820 | IV | 0.6278 | IV |
{{R}}_{3} | 0.5452 | VI | 9.3537 | II | 0.7280 | II |
{{R}}_{4} | 0.3315 | VIII | -6.3231 | VIII | 0.5059 | VIII |
{{R}}_{5} | 0.4210 | VII | -5.0894 | VII | 0.5399 | VII |
{{R}}_{6} | 0.5998 | IV | -3.0861 | VI | 0.5812 | VI |
{{R}}_{7} | 0.5728 | V | 0.4563 | V | 0.6136 | V |
{{R}}_{8} | 0.8143 | I | 11.8046 | I | 0.7400 | I |
{{R}}_{9} | 0.1015 | IX | -16.3987 | IX | 0.4010 | IX |
Alternatives | EDAS | Ranking | CODAS | Ranking | TOPSIS | Ranking |
{{R}}_{1} | 0.4897 | VI | 0.5583 | V | 0.3909 | V |
{{R}}_{2} | 0.2840 | VII | -2.8586 | VII | 0.3490 | VII |
{{R}}_{3} | 0.8716 | I | 7.6750 | II | 0.4678 | II |
{{R}}_{4} | 0.2296 | IX | -10.5288 | IX | 0.2593 | IX |
{{R}}_{5} | 0.6853 | II | 3.8543 | IV | 0.4197 | IV |
{{R}}_{6} | 0.2521 | VIII | -2.5370 | VI | 0.3634 | VI |
{{R}}_{7} | 0.5000 | IV | 8.3456 | I | 0.4727 | I |
{{R}}_{8} | 0.5000 | III | 4.0695 | III | 0.4274 | III |
{{R}}_{9} | 0.5000 | V | -8.5783 | VIII | 0.2784 | VIII |