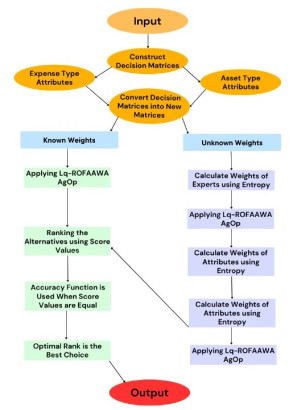
In this article, we presented two novel approaches for group decision-making (GDM) that were derived from the initiated linguistic q-rung orthopair fuzzy Aczel-Alsina weighted arithmetic (Lq-ROFAAWA) aggregation operator (AgOp) using linguistic q-rung orthopair fuzzy numbers (Lq-ROFNs). To introduce these GDM techniques, we first defined new operational laws for Lq-ROFNs based on Aczel-Alsina t-norm and t-conorm. The developed scalar multiplication and addition operations of Lq-ROFNs addressed the limitations of operations when q=1. The first proposed GDM methodology assumed that both experts' weights and attribute weights were fully known, while the second technique assumed that both sets of weights were entirely unknown. We also discussed properties of Lq-ROFNs under the Lq-ROFAAWA operators, such as idempotency, boundedness, and monotonicity. Furthermore, we solved problems related to environmental and economic issues, such as ranking countries by air pollution, selecting the best company for bank investments, and choosing the best electric vehicle design. Finally, we validated the proposed GDM approaches using three validity tests and performed a sensitivity analysis to compare them with preexisting models.
Citation: Ghous Ali, Kholood Alsager, Asad Ali. Novel linguistic q-rung orthopair fuzzy Aczel-Alsina aggregation operators for group decision-making with applications[J]. AIMS Mathematics, 2024, 9(11): 32328-32365. doi: 10.3934/math.20241551
[1] | Tahir Mahmood, Azam, Ubaid ur Rehman, Jabbar Ahmmad . Prioritization and selection of operating system by employing geometric aggregation operators based on Aczel-Alsina t-norm and t-conorm in the environment of bipolar complex fuzzy set. AIMS Mathematics, 2023, 8(10): 25220-25248. doi: 10.3934/math.20231286 |
[2] | Attaullah, Shahzaib Ashraf, Noor Rehman, Asghar Khan, Muhammad Naeem, Choonkil Park . Improved VIKOR methodology based on $ q $-rung orthopair hesitant fuzzy rough aggregation information: application in multi expert decision making. AIMS Mathematics, 2022, 7(5): 9524-9548. doi: 10.3934/math.2022530 |
[3] | Wajid Ali, Tanzeela Shaheen, Iftikhar Ul Haq, Hamza Toor, Faraz Akram, Harish Garg, Md. Zia Uddin, Mohammad Mehedi Hassan . Aczel-Alsina-based aggregation operators for intuitionistic hesitant fuzzy set environment and their application to multiple attribute decision-making process. AIMS Mathematics, 2023, 8(8): 18021-18039. doi: 10.3934/math.2023916 |
[4] | Chunxiao Lu, Zeeshan Ali, Peide Liu . Selection of artificial neutral networks based on cubic intuitionistic fuzzy Aczel-Alsina aggregation operators. AIMS Mathematics, 2024, 9(10): 27797-27833. doi: 10.3934/math.20241350 |
[5] | Aziz Khan, Shahzaib Ashraf, Saleem Abdullah, Muhammad Ayaz, Thongchai Botmart . A novel decision aid approach based on spherical hesitant fuzzy Aczel-Alsina geometric aggregation information. AIMS Mathematics, 2023, 8(3): 5148-5174. doi: 10.3934/math.2023258 |
[6] | Saba Ijaz, Kifayat Ullah, Maria Akram, Dragan Pamucar . Approaches to multi-attribute group decision-making based on picture fuzzy prioritized Aczel–Alsina aggregation information. AIMS Mathematics, 2023, 8(7): 16556-16583. doi: 10.3934/math.2023847 |
[7] | Attaullah, Shahzaib Ashraf, Noor Rehman, Asghar Khan, Choonkil Park . A decision making algorithm for wind power plant based on q-rung orthopair hesitant fuzzy rough aggregation information and TOPSIS. AIMS Mathematics, 2022, 7(4): 5241-5274. doi: 10.3934/math.2022292 |
[8] | Sumbal Ali, Asad Ali, Ahmad Bin Azim, Ahmad ALoqaily, Nabil Mlaiki . Averaging aggregation operators under the environment of q-rung orthopair picture fuzzy soft sets and their applications in MADM problems. AIMS Mathematics, 2023, 8(4): 9027-9053. doi: 10.3934/math.2023452 |
[9] | Adel Fahad Alrasheedi, Jungeun Kim, Rukhsana Kausar . q-Rung orthopair fuzzy information aggregation and their application towards material selection. AIMS Mathematics, 2023, 8(8): 18780-18808. doi: 10.3934/math.2023956 |
[10] | Dilshad Alghazzwi, Arshad Ali, Ahmad Almutlg, E. A. Abo-Tabl, A. A. Azzam . A novel structure of $ q $-rung orthopair fuzzy sets in ring theory. AIMS Mathematics, 2023, 8(4): 8365-8385. doi: 10.3934/math.2023422 |
In this article, we presented two novel approaches for group decision-making (GDM) that were derived from the initiated linguistic q-rung orthopair fuzzy Aczel-Alsina weighted arithmetic (Lq-ROFAAWA) aggregation operator (AgOp) using linguistic q-rung orthopair fuzzy numbers (Lq-ROFNs). To introduce these GDM techniques, we first defined new operational laws for Lq-ROFNs based on Aczel-Alsina t-norm and t-conorm. The developed scalar multiplication and addition operations of Lq-ROFNs addressed the limitations of operations when q=1. The first proposed GDM methodology assumed that both experts' weights and attribute weights were fully known, while the second technique assumed that both sets of weights were entirely unknown. We also discussed properties of Lq-ROFNs under the Lq-ROFAAWA operators, such as idempotency, boundedness, and monotonicity. Furthermore, we solved problems related to environmental and economic issues, such as ranking countries by air pollution, selecting the best company for bank investments, and choosing the best electric vehicle design. Finally, we validated the proposed GDM approaches using three validity tests and performed a sensitivity analysis to compare them with preexisting models.
Nowadays, aggregation operators (AgOps) are playing a significant role as powerful mathematical tools for combining various inputs into an output, considering the uncertainty associated with these input values. When investigating environmental and economic issues, AgOps and their fuzzy extensions can help in finding solutions by integrating multiple vital factors and stakeholders' preferences. The revolutionized concept of fuzzy set theory was launched by Zadeh [1] in 1965 as a modification of crisp set theory. The fuzzy set theory has emerged as an effective mathematical tool in decision science, catalyzing improvements in various fields by enhancing our capacity to model, scrutinize, and control complicated systems involving uncertainty [2]. To date, numerous important studies have been presented about collective decision-making using the combination of fuzzy set and rough set [3]. For instance, Wang et al. [4] presented a hybrid decision-making model that addresses uncertainty and relationships across criteria by combining rough fuzzy judgments with Choquet-like integrals. Additionally, Wang and Zhang [5] proposed new methods of analysis for intuitionistic fuzzy covering-based rough sets utilizing the intuitionistic fuzzy granular matrix. Due to the lack of a non-membership part in fuzzy set theory, Atanassov [6] extended the study of fuzzy sets by adding a non-membership function called intuitionistic fuzzy set (IFS), which can tackle non-membership degrees. Later, Yager [7] extended the idea of IFSs and presented a new concept of Pythagorean fuzzy sets that enlarge the space of input membership and non-membership values, but the sum of their square is restricted by unity. Additionally, Yager [8] lifted this study to its peak by presenting the idea of generalized or q-rung orthopair fuzzy sets (q-ROFSs) in which the sum of qth power of the membership and non-membership values is bounded by 1.
The fuzzy sets, IFSs, Pythagorean fuzzy sets, and q-ROFSs are quantitative mathematical tools. Besides, for the evaluation of alternatives in qualitative format, Zadeh [9] introduced the idea of linguistic variables (LVs). For instance, to precisely estimate the structure of any house, the terms extremely good, very good, good, fair, poor, etc. are used rather than numerical values. Following the concept of LVs by Zadeh, Chen et al. [10] introduced the idea of linguistic IFSs for dealing with linguistic IF numbers. To date, several significant studies have been reported for group decision-making based on linguistic IF numbers and other uncertainty theories. The process of collective decision-making in which a group of experts estimates the rank of given alternatives to choose the most suitable alternative from a group of options is called group decision-making (GDM), which is very significant in several fields [11]. For example, Akram et al. [12] introduced a GDM method by the fusion of 2-tuple linguistic Fermatean fuzzy sets with traditional MULTIMOORA (Multiple Objective Optimization on the basis of Ratio Analysis plus Full Multiplicative Form) technique, and explored its application in urban quality management. Seikh and Mandal [13] presented an interval-valued Fermatean fuzzy Dombi AgOps-based GDM method using the concepts of the PROMETHEE II technique under SWARA (Step-wise Weight Assessment Ratio Analysis) method. Liu et al. [14] integrated the evidence theory of Dempster-Shafer with linguistic IF numbers for GDM (see also, Yuan et al. [15]). Gou et al. [16] improved the classical ORESTE (Organization, Rangement Et Synthese De Donnes Relationnelles) method with linguistic preference orderings for GDM in medical domain. Cheng et al. [17] presented a model for large-scale GDM. Seikh and Mandal [18,19] introduced q-ROF Archimedean and Frank AgOps and explored their applications in selecting the best site for a software house and the best way for industrialization, respectively. Garg and Kumar [20] proposed an AgOps-based GDM method using a possibility degree measure of linguistic IF numbers. Besides, Garg and Kumar [21] introduced another AgOps-based GDM approach under the set-pair investigation of linguistic IF numbers (see also, Garg et al. [22]). Kumar and Chen [23] proposed an improvement in the linguistic interval-valued IF averaging AgOps and explored its application to GDM. Moreover, Kumar and Chen [24] suggested an advanced multiple-criteria GDM method using linguistic IF averaging AgOps for handling linguistic IF information. Liu et al. [25] introduced an emergency GDM method based on linguistic IF sets. Gou et al. [26] developed an improved VIKOR method based on the probabilistic double hierarchy linguistic term set, and explored its applications in smart healthcare domain. Tang and Meng [27] proposed linguistic IF Hamacher AgOps and studied their application in GDM. Kumar and Chen [28] introduced a GDM approach based on Yager's operation for the aggregation of linguistic intuitionistic fuzzy information. Verma and Agarwal [29] developed an AgOps-based generalized GDM approach under a linguistic interval-valued Pythagorean fuzzy environment. Fahmi et al. [30] introduced a novel GDM method based on cubic linguistic hesitant fuzzy AgOps. Kumar and Chen [31] suggested novel q-ROF Yager prioritized weighted arithmetic AgOPs with their application in GDM. Cheng et al. [32] proposed a large-scale GDM model that integrates risk attitudes by addressing the uncertainties in group decision environments.
After the successful production and implementation of various linguistic IF set-based AgOps, a number of scholars were attracted to the study of linguistic q-ROFS-based AgOps [33]. For instance, Lin et al. [34] introduced a powerful generalization of linguistic IF sets called linguistic q-ROFSs (Lq-ROFSs) and their related properties. Liu et al. [35] proposed a novel GDM method using power Bonferroni mean-based AgOps for the aggregation of Lq-ROFNs. Additionally, they [36] introduced another GDM approach based on linguistic q‐ROF power Muirhead mean AgOps using the entropy weight strategy. Ranjan et al. [37] proposed novel Archimedean operations-based AgOps using probabilistic linguistic q-ROFSs for GDM (see also Wang et al. [38]; Ali et al. [39]). Deb et al. [40] developed linguistic q-ROF prioritized AgOps under Hamacher operations and explored their applications to multiple attribute GDM. Liu et al. [41] established a new GDM model based on linguistic q-ROF generalized point-weighted AgOps. Akram et al. [42] developed a novel framework under Einstein's operations for linguistic q-ROF numbers and solved certain daily life applications in the mobile industry. Jana et al. [43] launched a new linguistic q-ROF Choquet integral method and discussed the evaluation criteria of sustainable strategies for urban parcel delivery. Naz et al. [44] presented a GDM model by incorporating power Muirhead mean operations for the aggregation of 2-tuple linguistic q-ROF information.
In the beginning of the 1980s, Aczel-Alsina t-norm (TN) and t-conorm (TCN) were first proposed by Aczel and Alsina [45], which are updated versions of the algebraic TN and TCN, respectively. Inspection of the literature reveals that more accurate decision-making is performed by the Aczel-Alsina's TN and TCN, in comparison to other TNs and TCNs. To justify this, Farahbod and Efekhari [46] compared various TN operators for classification issues. Aczel-Alsina operators are superior to the other operators due to the least error. Consequently, researchers have concentrated on Aczel-Alsina TN and TCN-based AgOps for each of the theories mentioned above. For example, Mahmood et al. [47] discussed the use of Aczel-Alsina TN and TCN-based AgOps in the bipolar complex fuzzy environment and studied their application in choosing the optimal operating system (see also, Mahmood and Ali [48]). Garg et al. [49] presented Choquet integral-based Aczel-Alsina AgOps using interval-valued IFSs, and studied their application in decision-making. Liu et al. [50] introduced prioritized AgOps using Aczel-Alsina TN and TCN operations for aggregating complex IF information. Recently, Rehman et al. [51] initiated m-polar fuzzy Aczel-Alsina AgOps and investigated their applications to identify suitable sites for wind power and desalination plants. Ali et al. [52] established a novel decision-making method under Yager's TN and TCN for the aggregation of m-polar fuzzy information. Additionally, Ali et al. [53] presented a GDM strategy using Aczel-Alsina's operations and IF soft information. For more contributions related Aczel-Alsina's TN and TCN, the readers are referred to [54,55]. It can be easily observed that Lq-ROF information is not aggregated using Aczel-Alsina's operations to date. Motivated by all these concerns, in this study, we propose two novel GDM approaches based on linguistic q-ROF sets with Aczel-Alsina TN and TCN that are omitted in the literature.
The Following are our major motivations for this study:
1) In group decision-making (or GDM), Lq-ROFS-based models play a crucial role, as they allow for linguistic assessments, enabling better management of the ambiguity and uncertainty inherent in the information.
2) Compared to linguistic IFS theory, Lq-ROFSs provide greater flexibility in modeling uncertainty and reluctance in decision-making. They improve the correctness of decisions made in complicated situations by enabling wider representation of both membership and non-membership degrees.
3) To achieve a more flexible and nuanced consensus, the Aczel-Alsina TN and TCN-based AgOps are widely used in GDM [46,48], which effectively balances a range of inputs by adjusting the influence of extreme values. The accuracy of collective decisions is increased by its parametric structure, which enables effortless changes between challenging and comfortable aggregation.
4) The aggregation of Lq-ROFNs is unattended using the exceptional aggregation features of Aczel-Alsina TN and TCN. Motivated by these settings, two novel GDM methods are initiated by combining the aggregation features of Aczel-Alsina TN and TCN with Lq-ROFSs.
Moreover, we investigate some limitations in Kumar and Chen's [24] scalar multiplication and addition operations of linguistic IF sets in some specific situations. Then, we suggest new addition and scalar multiplication operations of Lq-ROFNs based on Aczel Alsina's TN and TCN [45]. When q=1, the suggested addition and multiplication operations of Lq-ROFNs can overcome the deficiencies of Kumar and Chen's [24] multiplication and addition operations. For a more detailed review of AgOps and GDM approaches, the readers are referred to [56].
The significant contributions of this study are given below:
1) A new kind of averaging weighted AgOps based on Aczel Alsina's TN and TCN operations is initiated for the aggregation of Lq-ROFNs.
2) Some basic properties of suggested AgOps are investigated.
3) Next, two novel GDM methodologies based on the suggested Aczel Alsina's operations-based AgOps are developed to solve decision-making problems. The first GDM approach considers known weights in the aggregation process, while the second GDM method deals with unknown weights of experts and attributes.
4) To validate the applicability scope of our suggested GDM method, five numerical examples are solved using our suggested algorithms for each methodology.
5) Finally, to verify the authenticity and reliability of our proposed approach, a brief comparison is depicted between the suggested and existing approaches, and between the different values of q.
The remaining sections are structured as follows: In Section 2, we provide fundamental notions, including the linguistic term set, linguistic q-ROFS, Aczel-Alsina TN, and TCN. In Section 3, we develop some basic operations for linguistic q-ROFSs based on Aczel-Alsina TN and TCN. Subsequently, based on these operations, the linguistic q-ROF Aczel-Alsina weighted averaging (Lq-ROFAAWA) operators, along with their basic operations and properties, are presented in this section. In Section 4, we propose two GDM approaches considering both known and unknown weights for the aggregation of linguistic q-ROF numbers based on the initiated (Lq-ROFAAWA) operators and successfully implementing them in different practical situations. Section 5 validates our introduced approaches using existing tests and also compares them with certain preexisting methods. Finally, in Section 6, we provide concluding arguments and future directions.
In this section, we review some fundamental notions related to a linguistic term set, q-ROFSs, and Aczel-Alsina TN and TCN. Further, we describe shortcomings in the multiplication and addition operations presented by Kumar and Chen [28].
Definition 2.1. [10,21] Suppose L={a0,a1,…,ah} is a linguistic term set (LTS) having an odd cardinal number, here h is an even positive integer, and at is a reference value for the corresponding linguistic variable.
The following characteristics hold for a LTS L={a0,a1,...,ah} where h is an even positive integer [10,21,58]:
1) ¬(ak)=ah−k,
2) ak≤at⇔k≤t,
3) min(ak,at)=at⇔ak≥at,
4) max(ak,at)=ak⇔ak≥at.
Now, we define a continuous LTS (CLTS) L[0,h] as below [59]:
L[0,h]={az|a0≤az≤ah}, | (2.1) |
where h is an even positive integer.
Definition 2.2. [10] Suppose L[0,h]={az|a0≤az≤ah} is a CLTS, where h is an even positive integer and suppose U={u1,u2,...,un} is a finite universal set. Then, the mathematical expression of linguistic intuitionistic fuzzy set (LIFS) M in U is provided by
M={⟨u,aξ(u),aη(u)⟩|u∈U}, | (2.2) |
where aξ(u) and aη(u) represent the MD and NMD of object u∈M, respectively. Note that aξ(u), aη(u)∈L[0,h] and clearly the MD aξ(u) and NMD aη(u) satisfy the condition 0≤ξ(u)+η(u)≤h.
The pair ⟨aξ(u),aη(u)⟩ in LIFS M={⟨u,aξ(u),aη(u)|u∈U} denotes a LIFN. Let Γ[0,h] represent a collection of LIFNs provided in the CLTS L[0,h]={az|a0≤az≤ah}, where h is an even positive integer.
Definition 2.3. [35] Suppose L[0,h]={az|a0≤az≤ah} is a CLTS, where h is an even positive integer and suppose U={u1,u2,...,un} is a finite universal set. Then, the mathematical expression of linguistic q-rung orthopair fuzzy set (Lq-ROFS) Q on U is given as:
Q={⟨u,aξ(u),aη(u)⟩|u∈U}, | (2.3) |
where aξ(u) and aη(u) are respectively denoted the MD and NMD of element u belong to the Lq-ROFS Q, respectively. Here aξ(u),aη(u)∈L[0,h] that satisfy the conditions 0≤ξ(u)≤h, 0≤η(u)≤h and 0≤(ξ(u))q+(η(u))q≤hq where q≥1.
The pair ⟨aξ(u),aη(u)⟩ in Lq-ROFS Q={⟨u,aξ(u),aη(u)⟩|u∈U} denotes a linguistic q-ROF number (Lq-ROFN). Let Γ[0,h] represent a set of Lq-ROFNs provided on the CLTS L[0,h]={az|a0≤az≤ah} where h is an even positive integer. Let aπ(u)=a(hq−ξq−ηq)1/q, then the term aπ(u) is the hesitancy degree (HD) of the element u being a member of the Lq-ROFS Q.
In the following, we recall the notions of score and accuracy functions for Lq-ROFNs.
Definition 2.4. [35] Given a CLTS L[0,h]={az|a0≤az≤ah} and an Lq-ROFN σ=(aξ,aη) and (aξ,aη)∈L, then the value of score function of Lq-ROFN ⟨aξu,aηu⟩ is defined as:
D=a((hq+ηq−ξq)/2)1/q, |
and the value of accuracy function is defined as:
J=a(ξq+ηq)1/q. |
Then, the comparison of any two Lq-ROFNs σ1 and σ2 is defined as follows [35]:
1) If D(σ1)≥D(σ2), then σ1≥σ2, in other words the Lq-ROFN σ1 is considered to be superior to the Lq-ROFN σ2.
2) If D(σ1)=D(σ2), then their exact ranking is computed using the accuracy function as below:
(a) If J(σ1)>J(σ2), then σ1≥σ2,
(b) If J(σ1)=J(σ2), then σ1=σ2.
Definition 2.5. [45] For λ∈[0,∞] and σ1,σ2∈[0,1], the Aczel-Alsina TN (AA−TN) TλA(σ1,σ2) and Aczel-Alsina TCN (AA−TCN) SλA(σ1,σ2) are respectively given as:
TλA(σ1,σ2)={Tdra(σ1,σ2),ifλ=0,min(σ1,σ2),ifλ=∞,1−exp−((−logσ1)λ+(−logσ2)λ)1λ,otherwise. |
SλA(σ1,σ2)={Sdra(σ1,σ2),ifλ=0,max(σ1,σ2),ifλ=∞,1−exp−((−log(1−σ1))λ+(−log(1−σ2))λ)1λ,otherwise. |
Definition 2.6. [24] Suppose σ1=⟨aξ1,aη1⟩,σ2=⟨aξ2,aη2⟩ and σ=⟨aξ,aη⟩ be Lq-ROFNs, where σ1,σ2,σ∈Γ[0,h] and h is an even positive integer. Kumar and Chen's [24] scalar product and addition operations of the Lq-ROFNs σ1=⟨aξ1,aη1⟩,σ2=⟨aξ2,aη2⟩ and σ=⟨aξ,aη⟩ are defined as follows:
σ1⨁σ2=⟨ah(q√1ε(1−∏2i=1(1−ε(ξih)q))),ah(q√(1−1ε(1−∏2i=1(1−ε(1−(ηih)q)))))⟩,ψσ=⟨ahq√1ε(1−(1−ε(ξh)q)ψ),ahq√1−1ε(1−(1−ε(1−(ηh)q))ψ)⟩, |
where ψ>0 and 0<ε<1.
Some shortcomings of Kumar and Chen's [28] addition and scalar multiplication operations are shown in the following examples, respectively.
Example 2.1. Suppose σ1=⟨a7.5,a0.35⟩, and σ2=⟨a7.4,a0.5⟩ be Lq-ROFNs, where σ1,σ2∈Γ[0,8] and h=8. By Kumar and Chen's addition operation defined in Definition 2.6 of Lq-ROFNs σ1=⟨aξ1,aη1⟩, and σ2=⟨aξ2,aη2⟩ with q = 1 and ε=0.99, we get
σ1⨁σ2=⟨ah(q√1ε(1−∏2i=1(1−ε(ξih)q))),ah(q√(1−1ε(1−∏2i=1(1−ε(1−(ηih)q)))))⟩.σ1⨁σ2=⟨a8(10.99(1−(1−0.99(7.58))(1−0.99(7.48))))),a8((1−10.99(1−(1−0.99(1−(0.358)(1−0.99(1−0.58))))⟩=⟨a8.03a−0.04⟩. |
The MD of accumulated Lq-ROFN ⟨a8.03,a−0.04⟩ with q=1 is a8.03>a8, which is not suitable because the MD of this Lq-ROFN with q=1 must be lies in the Γ[0,8] and the NMD of this Lq-ROFN with q=1 must be lies in the Γ[0,8] but a−0.04<a0. Hence, the Kumar and Chen's addition operation [24] has the drawback that is observed in the above example.
Example 2.2. Suppose σ=⟨a5.20,a1.60⟩ is a Lq-ROFN with q=1, where σ∈Γ[0,8] and h=8. Now, the Kumar and Chen's [24] scalar product operation defined in Definition 2.6 of Lq-ROFN with q=1,ψ=5 and ε=0.99 is defined as follows:
ψσ=⟨ahq√1ε(1−(1−ε(ξh)q)ψ),ahq√1−1ε(1−(1−ε(1−(ηh)q))ψ)⟩,ψσ=⟨a8(10.99(1−(1−0.99(5.208))5)),a8(1−10.99(1−(1−0.99(1−(1.608)))5))⟩=⟨a8.03,a−0.07⟩. |
The MD of accumulated Lq-ROFN ⟨a8.03,a−0.07⟩ with q=1 is a8.03>a8, which is not suitable because the MD of this Lq-ROFN with q=1 should be lies in the Γ[0,8] and the NMD of this Lq-ROFN with q=1 should be lies in the Γ[0,8] but a−0.07<a0. Hence, Kumar and Chen's [24] operation has some drawbacks which are mentioned in the above example.
In this section, we first develop basic operations based on Aczel Alsina's TN and TCN for Lq-ROFNs, then, by applying them on Examples 2.1 and 2.2 as provided in previous section (see following Examples 3.1 and 3.2), we verify that the proposed operations overcome the difficulties in Kumar and Chen's [24] scalar product and addition operations. Moreover, we present linguistic q-ROF Aczel-Alsina weighted arithmetic AgOps with their basic properties.
Definition 3.1. Suppose σ1=⟨aξ1,aη1⟩,σ2=⟨aξ2,aη2⟩ and σ=⟨aξ,aη⟩ are Lq-ROFNs, where σ1,σ2,σ∈Γ[0,h] and h is an even positive integer. The suggested addition and scalar multiplication operation of the Lq-ROFNs σ1=⟨aξ1,aη1⟩,σ2=⟨aξ2,aη2⟩ and σ=⟨aξ,aη⟩ are defined as follows:
σ1⨁σ2=⟨ah(q√(1−exp−((−log(1−(ξσ1h)q))λ+(−log(1−(ξσ2h)q))λ)1/λ))),ah(exp−((−log(ησ1h))λ+(−log(ησ2h))λ)1/λ)⟩, | (3.1) |
σ1⨂σ2=⟨ah(exp−((−log(ξσ1h))λ+(−log(ξσ2h))λ)1/λ),ah(q√(1−exp−((−log(1−(ησ1h)q))λ+(−log(1−(ησ2h)q))λ)1/λ)))⟩, | (3.2) |
ψσ=⟨ahq√1−exp−(ψ(−log(1−(ξσh)q))λ)1/λ,ah(exp−(ψ(−log(ησh))λ)1/λ)⟩, | (3.3) |
σψ=⟨ah(exp−(ψ(−log(ξσh))λ)1/λ),ahq√1−exp−(ψ(−log(1−(ησh)q))λ)1/λ⟩, | (3.4) |
where λ>0 and ψ>0.
Example 3.1. Let σ1=⟨7.50,0.35⟩ and σ2=⟨7.40,0.50⟩ be two Lq-ROFNs, where σ1,σ2∈Γ[0,8]. Let λ=2, and q=1. Based on the suggested operations provided in Definition 3.1, we get
σ1⨁σ2=⟨ah(q√(1−exp−((−log(1−(ξσ1h)q))λ+(−log(1−(ξσ2h)q))λ)1/λ))),ah(exp−((−log(ησ1h))λ+(−log(ησ2h))λ)1/λ)⟩,σ1⨁σ2=⟨a8((1−exp−((−log(1−7.58))2+(−log(1−7.48)))2)1/2)),a8(exp−√(−log(0.358))2+(−log(0.58))2)⟩,=⟨a7.82a0.12⟩. |
The above Example 3.1 shows that the suggested addition operation of Lq-ROFNs based on the Aczel Alsina's TN and TCN overcomes the deficiencies of addition operation proposed by Kumar and Chen [24] for Lq-ROFNs in Example 2.1 with q=1.
Example 3.2. Let σ=⟨5.20,1.60⟩ be a Lq-ROFN where σ∈Γ[0,8]. Let λ=2, ψ=5 and q=1. Using the suggested scalar multiplication operation given in Definition 3.1, we get
ψσ=⟨ahq√1−exp−(ψ(−log(1−(ξσh)q))λ)1/λ,ah(exp−(ψ(−log(ησh))λ)1/λ)⟩,5σ=⟨a8(1−exp−(5(−log(1−5.208))2)1/2),ah(exp−(5(−log(1.608))2)1/2)⟩,=⟨a7.24,a0.21⟩. |
The above Example 3.2 shows that the suggested concept of scalar product with Lq-ROFNs based on the Aczel Alsina's TN and TCN overcomes the difficulties of Kumar and Chen's [24] scalar product of Lq-ROFNs as investigated in Example 2.2 with q=1.
Theorem 3.1. Suppose σ1=⟨aξ1,aη1⟩, σ2=⟨aξ2,aη2⟩ and σ=⟨aξ,aη⟩ are Lq-ROFNs with σ1,σ2,σ∈Γ[0,h] and h is an even positive integer. The suggested addition and scalar product of Lq-ROFkNs defined in Definition 3.1 verify the following conditions:
1) σ1⨁σ2=σ2⨁σ1,
2) ψ(σ1⨁σ2)=ψσ1⨁ψσ2,
3) (ψ1⨁ψ2)σ=ψ1σ⨁ψ2σ.
Now, we introduce the Lq-ROFAAWA operator based on suggested addition and scalar product of Lq-ROFNs.
Definition 3.2. Suppose σ1=⟨aξ1,aη1⟩, σ2=⟨aξ2,aη2⟩…, and σt=⟨aξt,aηt⟩ are Lq-ROFNs, where σ1,σ2,…,σt∈Γ[0,h] and h is an even positive integer. The suggested Lq-ROFAAWA AgOp is defined as:
Lq−ROFAAWA(σ1,σ2,…,σt)=w1σ1⨁w2σ2⨁…⨁wtσt=⟨ahq√1−exp−(∑tr=1wr(−log(1−(ξσrh)q))λ)1/λ,ahexp−(∑tr=1wr(−log(ησrh))λ)1/λ⟩, | (3.5) |
where wr represents the weight for each Lq-ROFN σt, wr∈[0,h],r=1,2,…,t, with ∑tr=1wr=1 and λ>0.
Theorem 3.2. The aggregated value of the Lq-ROFNs σ1=⟨aξ1,aη1⟩, σ2=⟨aξ2,aη2⟩…, σt=⟨aξt,aηt⟩ using the suggested Lq-ROFAAWA AgOp defined in Eq (3.5) is a Lq-ROFN, where σ1,σ2,…,σt∈Γ[0,h].
Now, we discuss some basic notions based on suggested Lq-ROFAAWA AgOp as follows:
Theorem 3.3. (Idempotency). Suppose σ1=⟨aξ1,aη1⟩,σ2=⟨aξ2,aη2⟩…, σn=⟨aξn,aηn⟩ are Lq-ROFNs and w1,w2,…,wn are the weights of Lq-ROFNs, respectively, and wr∈[0,h] where r=1,2,…,n with ∑nr=1wr=1. If σ1=σ2=…=σn=σ, then, Lq-ROFAAWA(σ1,σ2,…,σn)=σ.
Theorem 3.4. (Boundedness). Suppose σ1=⟨aξ1,aη1⟩,σ2=⟨aξ2,aη2⟩…, σn=⟨aξn,aηn⟩ are Lq-ROFNs, and let σ−=min{σ1,σ2,…,σn} and let σ+=max{σ1,σ2,…,σn}. Then, σ−≤Lq−ROFAAWA(σ1,σ2,…,σn)≤σ+.
Theorem 3.5. (Monotonicity). Suppose σ1,σ2,…,σn and σ′1,σ′2,…,σ′n are Lq-ROFNs. If σr≤σ′r where r=1,2,…,n. Then,
Lq−ROFAAWA(σ1,σ2,…,σn)≤Lq−ROFAAWA(σ′1,σ′2,…,σ′n). |
This section provides two GDM approaches based on the suggested Lq-ROFAAWA AgOp. The first recommended GDM approach assumes the condition that weights of experts and weights of attributes are provided, and the other GDM model assumes the condition that weights of experts and weights of attributes are not provided.
The suggested first GDM method is based on the proposed Lq-ROFAAWA AgOp in which weights of experts and weights of attributes are provided. Let R1,R2,…,Rm be alternatives and let S1,S2,…,Sn be attributes where w1,w2,…,wn are the weights of the attributes S1,S2,…,Sn, respectively with ∑nl=1wl=1 where l=1,2,…,n and wl≥0. Suppose Ex1,Ex2,…,Ext are experts and let β1,β2,…,βt be the weights of the experts Ex1,Ex2,…,Ext, respectively with ∑ti=1βi=1, where βi≥0,i=1,2,…,t. Every expert Exi estimates alternative Rk related to attribute Sl to construct the decision matrix D′p=(ϖ′pkl)m×n by the Lq-ROFN ϖ′pkl=⟨aξ′pkl,aη′pkl⟩ as below:
S1S2…SnD′p=R1R2⋮Rm(ϖ′p11ϖp12⋯ϖ′p1nϖ′p21ϖ′p22⋯ϖ′p2n⋮⋮⋱⋮ϖ′pm1ϖ′pm2⋯ϖ′pmn), |
where p=1,2,…,t.
Step 1: First of all convert decision matrix D′p=(ϖ′pkl)m×n=(⟨aξ′pkl,aη′pkl⟩)m×n into the new-decision matrix Dp=(ϖpkl)m×n=(⟨aξpkl,aηpkl⟩)m×n as given below:
ϖpkl={⟨aξ′pkl,aη′pkl⟩,ifSlisaassettype,⟨aξ′pkl,aη′pkl⟩,ifSlisaexpensetype, | (4.1) |
where k=1,2,…,m,l=1,2,…,n and p=1,2,…,t.
Step 2: Now using Eq (3.5), we accumulate the Lq-ROFNs ϖ1kl,ϖ2kl,…,ϖtkl defined in decision matrices D1=(ϖ1kl)m×n,D2=(ϖ2kl)m×n,…,Dt=(ϖtkl)m×n, respectively, where the accumulated Lq-ROFN ϖkl for constructing the cumulative decision matrix D=(ϖkl)m×n=(⟨aξkl,aηkl⟩)m×n by applying the suggested Lq-ROFAAWA AgOp are given as:
ϖkl=Lq−ROFAAWA(ϖ1kl,ϖ2kl,…,ϖtkl),=⟨ahq√1−exp−(∑ti=1βi(−log(1−(ξ′pklh)q))λ)1/λ,ahexp−(∑ti=1βi(−log(η′pklh))λ)1/λ⟩, | (4.2) |
where k=1,2,…,m,l=1,2,…,n and p=1,2,…,t with λ>0.
Step 3: By the Lq-ROFAAWA AgOp, accumulate the Lq-ROFNs ϖk1,ϖk2,…,ϖkn defined in kth row of cumulative decision matrix D=(ϖkl)m×n=(⟨aξkl,aηkl⟩)m×n to get the overall accumulated Lq-ROFN ϖk=⟨aξk,aηk⟩ of alternative Rk, which is calculated by the following formula:
ϖk=⟨ahq√1−exp−(∑nl=1wl(−log(1−(ξklh)q))λ)1/λ,ahexp−(∑nl=1wl(−log(ηklh))λ)1/λ⟩, | (4.3) |
where k=1,2,…,m and λ>0.
Step 4: Next, we compute the ranking value Y(ϖk) of the overall accumulated Lq-ROFNs of corresponding alternative Rk using the formula given below:
Y(ϖk)=1h(h−πk2)(ξk+πk2), | (4.4) |
where πk=(hq−ξq−ηq)1/q and k=1,2,…,m.
Step 5: By comparing the ranking values Y(ϖ1),Y(ϖ2),…,Y(ϖk) of the alternatives R1,R2,…,Rk, the larger ranking value Y(ϖk) gives the better ranking order (RO) of the corresponding alternative Rk. If the ranking values of alternatives Rx and Ry are the same, i.e., Y(ϖx)=Y(ϖy), then calculate the values of accuracy function J(ϖx) and J(ϖy) using Definition 2.4. The larger accuracy value represents the better RO of alternative Rk. If Y(ϖx)=Y(ϖy) and J(ϖx)=J(ϖy), then the alternatives Rx and Ry have the same ROs.
In the following three examples, we verify the applicability of our first suggested GDM method based on Lq-ROFNs.
Example 4.1 Air pollution is a crucial environmental issue that poses serious risks to human health, climate, and the ecosystems. Air pollution contains harmful substances in the atmosphere from different sources, including agriculture, vehicle exhaust, and industrial emissions. It substantially impacts health, leading to cardiovascular issues, premature death, and respiratory diseases, specifically among vulnerable populations like the elderly and children. Combatting air pollution needs stricter emission regulations, increased public transportation use, and cleaner technologies. A coordinated effort among individuals, industries, and governments is compulsory to create a healthier environment. Suppose that the government of Pakistan wants to rank the most air-polluted areas/cities of the country to make some rules or to announce public holidays in the cities where the situation is very severe. For this crucial task, the Government of Pakistan hired a group of experts Ex1,Ex2,Ex3, and Ex4 to evaluate all the alternatives concerning significant parameters. Consider the alternatives representing different cities of Pakistan, and the attributes that serve as the factors that increase the concentration of air pollution. The alternatives R1 (Karachi), R2 (Lahore), R3 (Faisalabad), and R4 (Sarghoda) are estimated by experts Ex1,Ex2,Ex3, and Ex4 over the attributes S1,S2,S3,S4, and S5. The attributes S1,S2,S3,S4, and S5 represent the burning of fossil fuels, increase in factories, transportation, agricultural practices, and power plants, respectively, where w={w1(0.25),w2(0.20),w3(0.15),w4(0.18),w5(0.22)} are weights allocated to attributes, and β1=0.25,β2=0.30,β3=0.20, and β4=0.25 are the weights of experts. The experts estimate every alternative Rk using the Lq-ROFNs ϖ′pkl on the basis of LTS={a0 = very hazardous, a1 = hazardous, a2 = very unhealthy, a3 = unhealthy, a4 = unhealthy for easily affected groups, a5 = moderate, a6 = good, a7 = very good, a8 = extremely good} in relation to attribute Sl to create decision-matrices D′1=(ϖ′1kl)4×5,D′2=(ϖ′2kl)4×5,D′3=(ϖ′3kl)4×5, and D′4=(ϖ′4kl)4×5, which are given as follows:
S1S2S3S4S5D′1=R1R2R3R4(⟨a7,a1⟩⟨a6,a2⟩⟨a4,a3⟩⟨a7,a1⟩⟨a5,a2⟩⟨a6,a2⟩⟨a5,a2⟩⟨a6,a1⟩⟨a6,a2⟩⟨a7,a1⟩⟨a6,a1⟩⟨a5,a3⟩⟨a7,a1⟩⟨a5,a1⟩⟨a3,a4⟩⟨a5,a2⟩⟨a7,a1⟩⟨a4,a3⟩⟨a6,a1⟩⟨a4,a4⟩), |
S1S2S3S4S5D′2=R1R2R3R4(⟨a7,a1⟩⟨a4,a4⟩⟨a6,a2⟩⟨a5,a2⟩⟨a3,a5⟩⟨a7,a1⟩⟨a5,a1⟩⟨a6,a1⟩⟨a5,a2⟩⟨a4,a3⟩⟨a5,a2⟩⟨a6,a1⟩⟨a7,a1⟩⟨a5,a3⟩⟨a4,a4⟩⟨a6,a2⟩⟨a4,a3⟩⟨a5,a2⟩⟨a7,a1⟩⟨a5,a3⟩), |
S1S2S3S4S5D′3=R1R2R3R4(⟨a6,a1⟩⟨a5,a2⟩⟨a3,a4⟩⟨a7,a1⟩⟨a5,a2⟩⟨a7,a2⟩⟨a6,a2⟩⟨a7,a1⟩⟨a6,a2⟩⟨a5,a1⟩⟨a5,a2⟩⟨a5,a2⟩⟨a6,a1⟩⟨a4,a3⟩⟨a3,a1⟩⟨a6,a2⟩⟨a7,a1⟩⟨a5,a1⟩⟨a5,a2⟩⟨a4,a4⟩), |
S1S2S3S4S5D′4=R1R2R3R4(⟨a5,a3⟩⟨a4,a4⟩⟨a7,a1⟩⟨a5,a1⟩⟨a4,a2⟩⟨a6,a1⟩⟨a7,a1⟩⟨a6,a1⟩⟨a5,a2⟩⟨a6,a1⟩⟨a5,a2⟩⟨a3,a4⟩⟨a6,a2⟩⟨a3,a3⟩⟨a5,a2⟩⟨a4,a3⟩⟨a5,a1⟩⟨a4,a2⟩⟨a6,a2⟩⟨a5,a2⟩). |
Now, we utilize the suggested first GDM method as below:
Step 1: Since all the attributes are asset type, we get the new-decision matrices D1=(ϖ1kl)4×5=(⟨aξ1kl,aη′1kl⟩)4×5, D2=(ϖ2kl)4×5=(⟨aξ2kl,aη′2kl⟩)4×5, D3=(ϖ3kl)4×5=(⟨aξ3kl,aη′3kl⟩)4×5, and D4=(ϖ4kl)4×5=(⟨aξ4kl,aη′4kl⟩)4×5.
Step 2: By the Eq (4.2), we accumulate the Lq-ROFNs ϖkl using the Lq-ROFNsl ϖ1kl,ϖ2kl,ϖ3kl, and ϖ4kl that belongs to the decision matrices D1=(ϖ1kl)4×5=(⟨aξ1kl,aη′1kl⟩)4×5, D2=(ϖ2kl)4×5=(⟨aξ2kl,aη′2kl⟩)4×5, D3=(ϖ3kl)4×5=(⟨aξ3kl,aη′3kl⟩)4×5, and D4=(ϖ4kl)4×5=(⟨aξ4kl,aη′4kl⟩)4×5 to construct the cumulative decision matrix D=(ϖkl)4×5=(⟨aξkl,aηkl⟩)4×5 with k=1,2,…,4 and l=1,2,…,5 as given below for q=3:
S1S2S3S4S5D=R1R2R3R4(⟨a6.67,a1.23⟩⟨a5.18,a2.76⟩⟨a6.21,a1.94⟩⟨a6.50,a1.19⟩⟨a4.54,a2.44⟩⟨a6.68,a1.16⟩⟨a6.23,a1.32⟩⟨a6.35,a1.00⟩⟨a5.60,a2.00⟩⟨a6.22,a1.29⟩⟨a5.39,a1.63⟩⟨a5.34,a1.91⟩⟨a6.72,a1.16⟩⟨a4.64,a2.08⟩⟨a4.24,a2.24⟩⟨a5.59,a2.18⟩⟨a6.48,a1.29⟩⟨a4.65,a1.83⟩⟨a6.42,a1.32⟩⟨a4.69,a1.96⟩). |
Step 3: Using Eq (4.3), we combine every accumulative Lq-ROFN ϖk=⟨aξk,aηk⟩ of corresponding alternative Rk, with k=1,2,3,4 that are: ϖ1=⟨a6.18,a1.74⟩, ϖ2=⟨a6.32,a1.42⟩, ϖ3=⟨a5.66,a1.80⟩, and ϖ4=⟨a5.92,a1.75⟩.
Step 4: Next, by applying Eq (4.4), we calculate the ranking values Y(ϖ1), Y(ϖ2), Y(ϖ3), and Y(ϖ4), which are Y(ϖ1)=5.64, Y(ϖ2)=5.76, Y(ϖ3)=5.22, and Y(ϖ4)=5.42.
Step 5: From Step 4, we see that Y(ϖ2)>Y(ϖ1)>Y(ϖ4)>Y(ϖ3), Hence, the more polluted city out of Rk cities with k=1,2,3,4 is R2.
Example 4.2. In today's interconnected world, marked by global unity and progress, students are increasingly prioritizing obtaining education in foreign countries. However, they often encounter various challenges in pursuing this path. Thus, finding a suitable foreign university for a student involves various challenges. Some common problems include: Financial constraints, academic requirements, language proficiency, and so on. To find a good foreign university, students seek assistance from various experts, including educational consultants, career counselors, admissions advisors, alumni networks, international student offices, standardized test prep centers, etc. Suppose a student wants to get admission for higher studies in a suitable foreign university and to find best option from the alternatives R1, R2,…,R6, the student takes services of different experts such as Ex1,Ex2,…,Ex6. Consider the favorable attributes are S1 (Academic Reputation), S2 (Faculty Expertise), S3 (Research Opportunities), S4 (Financial Aid), S5 (Innovation), S6 (Student Services), and S7 (Cost), with the help of experts Ex1, Ex2,…, and Ex6. Suppose the attributes S1, S2, …, S7 having weights w={w1(0.20),w2(0.15),w3(0.05),w4(0.03),w5(0.12),w6(0.32),w7(0.13)} and the experts' weights are β1=0.13, β2=0.20, β3=0.25, β4=0.10, β5=0.17, β6=0.15. The experts estimate each alternative Rk through the Lq-ROFNs ϖ′pkl on the basis of LTS={a0=extremelypoor,a1=verypoor,a2=poor, a3=slightlypoor,a4=fair,a5=slightlygood, a6=good,a7=verygood,a8=extremelygood} in relation to each attribute Sl to create the decision-matrices D′1=(ϖ′1kl)6×7,D′2=(ϖ′2kl)6×7,D′3=(ϖ′3kl)6×7,D′4=(ϖ′4kl)6×7,D′5=(ϖ′5kl)6×7, and D′6=(ϖ′6kl)6×7 that is given as follows:
S1S2S3S4S5S6S7D′1=R1R2R3R4R5R6(⟨a5,a2⟩⟨a7,a1⟩⟨a4,a3⟩⟨a6,a1⟩⟨a4,a4⟩⟨a5,a2⟩⟨a4,a3⟩⟨a6,a1⟩⟨a5,a3⟩⟨a7,a1⟩⟨a5,a1⟩⟨a5,a1⟩⟨a4,a4⟩⟨a7,a1⟩⟨a6,a2⟩⟨a5,a2⟩⟨a6,a1⟩⟨a6,a2⟩⟨a7,a1⟩⟨a5,a1⟩⟨a3,a2⟩⟨a7,a1⟩⟨a6,a2⟩⟨a4,a3⟩⟨a7,a1⟩⟨a5,a2⟩⟨a6,a1⟩⟨a5,a3⟩⟨a6,a1⟩⟨a5,a2⟩⟨a4,a4⟩⟨a5,a1⟩⟨a5,a3⟩⟨a5,a1⟩⟨a5,a3⟩⟨a5,a1⟩⟨a6,a1⟩⟨a5,a3⟩⟨a6,a1⟩⟨a3,a2⟩⟨a5,a2⟩⟨a4,a3⟩), |
S1S2S3S4S5S6S7D′2=R1R2R3R4R5R6(⟨a4,a3⟩⟨a5,a2⟩⟨a4,a4⟩⟨a6,a1⟩⟨a4,a3⟩⟨a7,a1⟩⟨a5,a2⟩⟨a4,a1⟩⟨a6,a3⟩⟨a5,a1⟩⟨a4,a2⟩⟨a4,a4⟩⟨a6,a1⟩⟨a4,a1⟩⟨a6,a1⟩⟨a5,a3⟩⟨a6,a2⟩⟨a6,a1⟩⟨a4,a3⟩⟨a5,a2⟩⟨a5,a1⟩⟨a6,a1⟩⟨a5,a2⟩⟨a6,a2⟩⟨a5,a1⟩⟨a5,a2⟩⟨a4,a2⟩⟨a5,a3⟩⟨a5,a1⟩⟨a4,a2⟩⟨a6,a2⟩⟨a5,a3⟩⟨a4,a3⟩⟨a5,a1⟩⟨a4,a3⟩⟨a5,a2⟩⟨a4,a2⟩⟨a5,a3⟩⟨a6,a2⟩⟨a4,a1⟩⟨a5,a2⟩⟨a6,a1⟩), |
S1S2S3S4S5S6S7D′3=R1R2R3R4R5R6(⟨a7,a1⟩⟨a5,a1⟩⟨a4,a3⟩⟨a4,a1⟩⟨a6,a1⟩⟨a5,a2⟩⟨a4,a2⟩⟨a5,a2⟩⟨a4,a3⟩⟨a6,a1⟩⟨a4,a1⟩⟨a6,a2⟩⟨a4,a1⟩⟨a5,a2⟩⟨a6,a2⟩⟨a4,a2⟩⟨a5,a1⟩⟨a3,a2⟩⟨a6,a1⟩⟨a4,a1⟩⟨a6,a2⟩⟨a4,a2⟩⟨a5,a2⟩⟨a4,a1⟩⟨a5,a1⟩⟨a4,a2⟩⟨a5,a1⟩⟨a5,a3⟩⟨a4,a2⟩⟨a6,a2⟩⟨a4,a4⟩⟨a3,a1⟩⟨a5,a2⟩⟨a6,a1⟩⟨a4,a3⟩⟨a4,a2⟩⟨a5,a2⟩⟨a6,a1⟩⟨a4,a1⟩⟨a3,a2⟩⟨a6,a2⟩⟨a5,a3⟩), |
S1S2S3S4S5S6S7D′4=R1R2R3R4R5R6(⟨a4,a1⟩⟨a6,a1⟩⟨a5,a3⟩⟨a5,a5⟩⟨a4,a1⟩⟨a6,a2⟩⟨a4,a2⟩⟨a4,a1⟩⟨a5,a2⟩⟨a6,a1⟩⟨a5,a2⟩⟨a6,a1⟩⟨a4,a1⟩⟨a5,a1⟩⟨a6,a2⟩⟨a5,a2⟩⟨a6,a1⟩⟨a6,a2⟩⟨a7,a1⟩⟨a5,a1⟩⟨a3,a2⟩⟨a6,a1⟩⟨a6,a2⟩⟨a5,a3⟩⟨a4,a1⟩⟨a6,a2⟩⟨a5,a2⟩⟨a7,a1⟩⟨a5,a1⟩⟨a6,a2⟩⟨a4,a4⟩⟨a7,a1⟩⟨a6,a2⟩⟨a5,a2⟩⟨a5,a3⟩⟨a6,a2⟩⟨a5,a1⟩⟨a6,a2⟩⟨a7,a1⟩⟨a4,a1⟩⟨a5,a2⟩⟨a6,a1⟩), |
S1S2S3S4S5S6S7D′5=R1R2R3R4R5R6(⟨a4,a1⟩⟨a5,a2⟩⟨a6,a1⟩⟨a5,a2⟩⟨a6,a1⟩⟨a4,a1⟩⟨a5,a1⟩⟨a4,a1⟩⟨a6,a1⟩⟨a5,a3⟩⟨a5,a5⟩⟨a4,a1⟩⟨a6,a2⟩⟨a4,a2⟩⟨a6,a2⟩⟨a4,a2⟩⟨a5,a1⟩⟨a3,a2⟩⟨a6,a1⟩⟨a4,a1⟩⟨a6,a2⟩⟨a6,a1⟩⟨a5,a3⟩⟨a6,a2⟩⟨a6,a1⟩⟨a4,a3⟩⟨a5,a2⟩⟨a5,a1⟩⟨a5,a2⟩⟨a7,a1⟩⟨a4,a3⟩⟨a6,a1⟩⟨a4,a4⟩⟨a5,a2⟩⟨a4,a3⟩⟨a5,a1⟩⟨a6,a2⟩⟨a4,a4⟩⟨a7,a1⟩⟨a6,a2⟩⟨a5,a2⟩⟨a5,a3⟩), |
S1S2S3S4S5S6S7D′6=R1R2R3R4R5R6(⟨a5,a2⟩⟨a6,a1⟩⟨a5,a2⟩⟨a7,a1⟩⟨a5,a2⟩⟨a6,a1⟩⟨a5,a3⟩⟨a5,a3⟩⟨a6,a2⟩⟨a4,a2⟩⟨a5,a1⟩⟨a6,a2⟩⟨a5,a3⟩⟨a4,a1⟩⟨a5,a1⟩⟨a6,a1⟩⟨a4,a2⟩⟨a5,a3⟩⟨a6,a2⟩⟨a5,a2⟩⟨a4,a1⟩⟨a5,a1⟩⟨a6,a2⟩⟨a4,a4⟩⟨a7,a1⟩⟨a6,a2⟩⟨a5,a2⟩⟨a5,a3⟩⟨a5,a2⟩⟨a7,a1⟩⟨a4,a3⟩⟨a6,a1⟩⟨a4,a4⟩⟨a5,a2⟩⟨a4,a3⟩⟨a6,a1⟩⟨a5,a3⟩⟨a6,a2⟩⟨a6,a1⟩⟨a4,a3⟩⟨a5,a2⟩⟨a5,a1⟩). |
Now, we again utilize the suggested first GDM method to solve this example as below:
Step 1: Since all the attributes are asset type, so, we get the new-decision matrices as: D1=(ϖ1kl)6×7=(⟨aξ1kl,aη′1kl⟩)6×7, D2=(ϖ2kl)6×7=(⟨aξ2kl,aη′2kl⟩)6×7, D3=(ϖ3kl)6×7=(⟨aξ3kl,aη′3kl⟩)6×7, D4=(ϖ4kl)6×7=(⟨aξ4kl,aη′4kl⟩)6×7, D5=(ϖ5kl)6×7=(⟨aξ5kl,aη′5kl⟩)6×7 and D6=(ϖ6kl)6×7=(⟨aξ6kl,aη′6kl⟩)6×7.
Step 2: Using the Eq (4.2) we accumulate the Lq-ROFNs ϖkl by the Lq-ROFNs ϖ1kl,ϖ2kl,ϖ3kl,ϖ4kl,ϖ5kl and ϖ6kl that belongs to the decision matrices D1=(ϖ1kl)6×7=(⟨aξ1kl,aη′1kl⟩)6×7, D2=(ϖ2kl)6×7=(⟨aξ2kl,aη′2kl⟩)6×7, D3=(ϖ3kl)6×7=(⟨aξ3kl,aη′3kl⟩)6×7, D4=(ϖ4kl)6×7=(⟨aξ4kl,aη′4kl⟩)6×7, D5=(ϖ5kl)6×7=(⟨aξ5kl,aη′5kl⟩)6×7, and D6=(ϖ6kl)6×7=(⟨aξ6kl,aη′6kl⟩)6×7 to construct the cumulative decision matrix D=(ϖkl)6×7=(⟨aξkl,aηkl⟩)6×7 with k=1,2,…6, and l=1,2,…7 as given below for q=3:
S1S2S3S4S5S6S7D=R1R2R3R4R5R6(⟨a6.08,a1.42⟩⟨a5.95,a1.25⟩⟨a5.02,a2.27⟩⟨a6.02,a1.23⟩⟨a5.45,a1.50⟩⟨a6.12,a1.34⟩⟨a4.67,a1.90⟩⟨a5.00,a1.33⟩⟨a5.60,a2.11⟩⟨a5.99,a1.26⟩⟨a4.69,a1.45⟩⟨a5.55,a1.59⟩⟨a5.37,a1.45⟩⟨a5.68,a1.29⟩⟨a5.91,a1.51⟩⟨a5.06,a1.88⟩⟨a5.54,a1.23⟩⟨a5.43,a1.78⟩⟨a6.31,a1.30⟩⟨a4.72,a1.23⟩⟨a5.45,a1.51⟩⟨a6.04,a1.16⟩⟨a5.53,a2.12⟩⟨a5.36,a1.88⟩⟨a6.27,a1.00⟩⟨a5.23,a2.12⟩⟨a5.14,a1.48⟩⟨a5.66,a2.03⟩⟨a5.11,a1.43⟩⟨a6.39,a1.55⟩⟨a4.96,a3.06⟩⟨a5.84,a1.18⟩⟨a4.90,a2.74⟩⟨a5.39,a1.29⟩⟨a4.37,a3.00⟩⟨a5.30,a1.41⟩⟨a5.38,a1.70⟩⟨a5.61,a1.97⟩⟨a6.34,a1.12⟩⟨a4.81,a1.64⟩⟨a5.39,a2.00⟩⟨a5.41,a1.65⟩). |
Step 3: By applying Eq (4.3), we combine each accumulative Lq-ROFN ϖk=⟨aξk,aηk⟩ of respective alternative Rk, with k=1,2,…,6 that are: ϖ1=⟨a5.87,a1.45⟩, ϖ2=⟨a5.44,a1.49⟩, ϖ3=⟨a5.56,a1.43⟩, ϖ4=⟨a5.58,a1.60⟩, ϖ5=⟨a5.52,a1.67⟩, and ϖ6=⟨a5.38,a1.70⟩.
Step 4: Further, using Eq (4.4), we calculate the ranking values Y(ϖ1), Y(ϖ2), Y(ϖ3), Y(ϖ4), Y(ϖ5) and Y(ϖ6) that are provided as: Y(ϖ1)=5.38, Y(ϖ2)=5.06, Y(ϖ3)=5.14, Y(ϖ4)=5.16, Y(ϖ5)=5.12, and Y(ϖ6)=5.02.
Step 5: From previous Step, we observe that Y(ϖ1)>Y(ϖ4)>Y(ϖ3)>Y(ϖ5)>Y(ϖ2)>Y(ϖ6). Thus, the best university out of all universities Rk with k=1,2,…,6 is R1.
Example 4.3 These days, banks strategically invest in diverse businesses as a means to mitigate losses. However, selecting the right businesses is no easy task. To address this challenge and safeguard their interests, banks rely on the opinions and evaluation reports of various experts. These experts specialize in the specific fields in which the bank intends to invest. Their insights provide crucial guidance, allowing the bank to make well-informed decisions, minimize risks, and enhance the overall effectiveness of their investment strategies. Consider a bank ABC wants to put money into a most profitable factory from different factories like a car factory (R1), a food factory (R2), a computer factory (R3) and an arms factory (R4). For this crucial task, the experts Ex1,Ex3, and Ex3 evaluate the alternatives R1,R2,R3, and R4 under the attributes of benefit related such as S1 (analysis of risk), S2(development analysis), S3(sociopolitical influence analysis), and S4(analysis of environmental effect). The weights of attributes are w={w1(0.30),w2(0.10),w3(0.20),w4(0.40)} and weights of experts are β1=0.243,β2=0.514, and β3=0.243. The experts estimate every alternative Rk using Lq-ROFNs ϖ′pkl on the grounds of LTS={a0=extremelypoor,a1=verypoor, a2=poor,a3=slightlypoor,a4=fair, a5=slightlygood,a6=good, a7=verygood,a8=extremelygood} in relation to each attribute Sl to create decision-matrices D′1=(ϖ′1kl)4×5,D′2=(ϖ′2kl)4×5, and D′3=(ϖ′3kl)4×5, which are given as follows:
S1S2S3S4D′1=R1R2R3R4(⟨a6,a1⟩⟨a3,a1⟩⟨a3,a3⟩⟨a1,a6⟩⟨a3,a4⟩⟨a3,a4⟩⟨a2,a5⟩⟨a2,a4⟩⟨a1,a3⟩⟨a2,a3⟩⟨a3,a2⟩⟨a6,a1⟩⟨a6,a2⟩⟨a4,a3⟩⟨a5,a1⟩⟨a7,a1⟩), |
S1S2S3S4D′2=R1R2R3R4(⟨a3,a2⟩⟨a4,a1⟩⟨a3,a4⟩⟨a2,a3⟩⟨a5,a2⟩⟨a2,a1⟩⟨a3,a4⟩⟨a2,a5⟩⟨a2,a3⟩⟨a3,a3⟩⟨a1,a2⟩⟨a3,a3⟩⟨a5,a2⟩⟨a3,a3⟩⟨a5,a2⟩⟨a4,a1⟩), |
S1S2S3S4D′3=R1R2R3R4(⟨a3,a3⟩⟨a3,a5⟩⟨a6,a1⟩⟨a2,a6⟩⟨a3,a2⟩⟨a2,a4⟩⟨a2,a1⟩⟨a3,a4⟩⟨a6,a1⟩⟨a2,a5⟩⟨a3,a4⟩⟨a1,a3⟩⟨a5,a1⟩⟨a4,a4⟩⟨a6,a2⟩⟨a5,a2⟩). |
We now repeat the suggested first GDM method on this example as below:
Step 1: Since all the attributes are asset type, we get the new-decision matrices D1=(ϖ1kl)4×4=(⟨aξ1kl,aη′1kl⟩)4×4, D2=(ϖ2kl)4×4=(⟨aξ2kl,aη′2kl⟩)4×4, D3=(ϖ3kl)4×4=(⟨aξ3kl,aη′3kl⟩)4×4, and D4=(ϖ4kl)4×4=(⟨aξ4kl,aη′4kl⟩)4×4.
Step 2: From Eq (4.2), we accumulate Lq-ROFNs ϖkl using the Lq-ROFNs ϖ1kl,ϖ2kl,ϖ3kl, and ϖ4kl that belongs to the decision matrices D1=(ϖ1kl)4×4=(⟨aξ1kl,aη′1kl⟩)4×4, \mathbb{D}^{2} = \bigg(\varpi_{kl}^{2}\bigg)_{4\times 4} = \bigg(\bigg\langle \mathfrak{a}_{\xi_{kl}^{2}}, \; \mathfrak{a}_{\eta_{kl}^{'2}} \bigg\rangle\bigg)_{4\times 4} , \mathbb{D}^{3} = \bigg(\varpi_{kl}^{3}\bigg)_{4\times 4} = \bigg(\bigg\langle \mathfrak{a}_{\xi_{kl}^{3}}, \; \mathfrak{a}_{\eta_{kl}^{'3}} \bigg\rangle\bigg)_{4\times 4} , and \mathbb{D}^{4} = \bigg(\varpi_k{kl}^{4}\bigg)_{4\times 4} = \bigg(\bigg\langle \mathfrak{a}_{\xi_{kl}^{4}}, \; \mathfrak{a}_{\eta_{kl}^{'4}} \bigg\rangle\bigg)_{4\times 4} . to construct the cumulative decision matrix \mathbb{D} = \bigg(\varpi_{kl}\bigg)_{4\times 4} = \bigg(\bigg\langle \mathfrak{a}_{\xi_{kl}}, \; \mathfrak{a}_{\eta_{kl}} k\bigg\rangle\bigg)_{4\times 4} with k = 1, 2, \ldots4 and l = 1, 2, \ldots4 that is given below for q = 3 :
\begin{aligned} & \begin{array}{llll} \quad\quad\quad\quad\quad \quad\quad\mathcal{S}_{1} &\quad\quad\quad\quad\mathcal{S}_{2} &\quad\quad\quad\quad \mathcal{S}_{3} & \quad\quad\quad\quad\mathcal{S}_{4} \end{array} \\ & \mathbb{D} = \begin{array}{c} \mathcal{R}_{1} \\ \mathcal{R}_{2}\\ \mathcal{R}_{3}\\ \mathcal{R}_{4} \end{array}\left(\begin{array}{ccccc} \langle \mathfrak{a}_{4.97},\; \mathfrak{a}_{1.78}\rangle & \langle \mathfrak{a}_{3.68},\; \mathfrak{a}_{1.37}\rangle & \langle \mathfrak{a}_{4.97},\; \mathfrak{a}_{2.32}\rangle & \langle \mathfrak{a}_{1.90},\; \mathfrak{a}_{3.86}\rangle \\ \langle \mathfrak{a}_{4.55},\; \mathfrak{a}_{2.29}\rangle & \langle \mathfrak{a}_{2.47},\; \mathfrak{a}_{1.67}\rangle & \langle \mathfrak{a}_{2.72},\; \mathfrak{a}_{2.50}\rangle & \langle \mathfrak{a}_{2.47},\; \mathfrak{a}_{4.44}\rangle \\ \langle \mathfrak{a}_{4.95},\; \mathfrak{a}_{2.11}\rangle & \langle \mathfrak{a}_{2.72},\; \mathfrak{a}_{3.31}\rangle & \langle \mathfrak{a}_{2.67},\; \mathfrak{a}_{2.29}\rangle & \langle \mathfrak{a}_{4.96},\; \mathfrak{a}_{2.11}\rangle \\ \langle \mathfrak{a}_{5.38},\; \mathfrak{a}_{1.64}\rangle & \langle \mathfrak{a}_{3.66},\; \mathfrak{a}_{3.19}\rangle & \langle \mathfrak{a}_{5.38},\; \mathfrak{a}_{1.64}\rangle & \langle \mathfrak{a}_{6.06},\; \mathfrak{a}_{1.23}\rangle \end{array}\right). & \end{aligned} |
Step 3: Using Eq (4.3), we combine every accumulative L q -ROFN \varpi_{k} = \langle \mathfrak{a}_{\xi_{k}}, \; \mathfrak{a}_{\eta_{k}}\rangle of corresponding alternative \mathcal{R}_{k} with k = 1, 2, \ldots, 4 are \varpi_{1} = \langle \mathfrak{a}_{4.50}, \; \mathfrak{a}_{2.34}\rangle , \varpi_{2} = \langle \mathfrak{a}_{3.81}, \; \mathfrak{a}_{2.77}\rangle , \varpi_{3} = \langle \mathfrak{a}_{4.71}, \; \mathfrak{a}_{2.22}\rangle , and \varpi_{4} = \langle \mathfrak{a}_{5.68}, \; \mathfrak{a}_{1.52}\rangle .
Step 4: Next, by Eq (4.4), we calculate the ranking values \mathcal{Y}(\varpi_{1}) , \mathcal{Y}(\varpi_{2}) , \mathcal{Y}(\varpi_{3}) , and \mathcal{Y}(\varpi_{4}) that are: \mathcal{Y}(\varpi_{1}) = 4.44 , \mathcal{Y}(\varpi_{2}) = 4.03 , \mathcal{Y}(\varpi_{3}) = 4.57 , and \mathcal{Y}(\varpi_{4}) = 5.23 .
Step 5: From preceding Step, we see that \mathcal{Y}(\varpi_{4}) > \mathcal{Y}(\varpi_{3}) > \mathcal{Y}(\varpi_{1}) > \mathcal{Y}(\varpi_{2}). Hence, the best business out of all businesses \mathcal{R}_{k} with k = 1, 2, \ldots, 4 is \mathcal{R}_{4}.
This subsection is devoted to designing the second G^DM approach based on the suggested L q -ROFAAWA AgOp with the completely unknown weights of the experts and attributes.
Let \mathcal{R}_{1}, \mathcal{R}_{2}, \ldots, \mathcal{R}_{m} be alternatives and let \mathcal{S}_{1}, \mathcal{S}_{2}, \ldots, \mathcal{S}_{n} be attributes. Suppose \mathcal{E}\mathfrak{x}_{1}, \mathcal{E}\mathfrak{x}_{2}, \ldots, \mathcal{E}\mathfrak{x}_{t} are experts and every expert \mathcal{E}\mathfrak{x}_{i} estimate each alternative \mathcal{R}_{k} regarding each attribute \mathcal{S}_{l} to construct the decision matrix \mathbb{D}^{'p} = \bigg(\varpi_{kl}^{'p}\bigg)_{m\times n} using L q -ROFN \varpi_{kl}^{'p} = \bigg\langle \mathfrak{a}_{\xi_{kl}^{'p}}, \; \mathfrak{a}_{\eta_{kl}^{'p}} \bigg\rangle as below:
\begin{aligned} & \begin{array}{llll} \quad\quad\quad\quad\quad\quad\quad \mathcal{S}_{1} & \mathcal{S}_{2} & \ldots & \mathcal{S}_{n} \end{array} \\ & \mathbb{D}^{'p} = \begin{array}{c} \mathcal{R}_{1} \\ \mathcal{R}_{2}\\ \vdots \\ \mathcal{R}_{m} \end{array}\left(\begin{array}{cccc} \varpi_{11}^{'p} & \varpi_{12}^{p} & \cdots & \varpi_{1 n}^{'p} \\ \varpi_{21}^{'p} & \varpi_{22}^{'p} & \cdots & \varpi_{2 n}^{'p} \\ \vdots & \vdots & \ddots & \vdots \\ \varpi_{m 1}^{'p} & \varpi_{m 2}^{'p} & \cdots & \varpi_{m n}^{'p} \end{array}\right), \\ & \end{aligned} |
where p = 1, 2, \ldots, t .
Step 1: First of all covert each decision matrix \mathbb{D}^{'p} = \bigg(\varpi_{kl}^{'p}\bigg)_{m\times n} = \bigg(\bigg\langle \mathfrak{a}_{\xi_{kl}^{'p}}, \; \mathfrak{a}_{\eta_{kl}^{'p}} \bigg\rangle\bigg)_{m\times n} into the new-decision matrix \mathbb{D}^{p} = \bigg(\varpi_{kl}^{p}\bigg)_{m\times n} = \bigg(\bigg\langle \mathfrak{a}_{\xi_{kl}^{p}}, \; \mathfrak{a}_{\eta_{kl}^{'p}} \bigg\rangle\bigg)_{m\times n} as given below:
\begin{equation} \varpi_{kl}^{p} = \begin{cases} \big\langle \mathfrak{a}_{\xi_{kl}^{'p}},\; \mathfrak{a}_{\eta_{kl}^{'p}} \big\rangle,& \text{if}\; \mathcal{S}_{l}\; {\rm is\; an\; asset\; type},\\ \big\langle \mathfrak{a}_{\xi_{kl}^{'p}},\; \mathfrak{a}_{\eta_{kl}^{'p}} \big\rangle,& \text{if}\; \mathcal{S}_{l}\; {\rm is\; an\; expense\; type}, \end{cases} \end{equation} | (4.5) |
here k = 1, 2, \ldots, m, l = 1, 2, \ldots, n and p = 1, 2, \ldots, t.
Step 2: The entropy \varsigma^{p} of the decision-maker \mathcal{E}\mathfrak{x}_{i} is calculated using the L q -ROFNs provided in the new-decision matrix \mathbb{D}^{p} = \bigg(\varpi_{kl}^{p}\bigg)_{m\times n} = \bigg(\bigg\langle \mathfrak{a}_{\xi_{kl}^{p}}, \; \mathfrak{a}_{\eta_{kl}^{p}} \bigg\rangle\bigg)_{m\times n} as below:
\begin{align} \varsigma^{p}\; = \; &\frac{1}{mn}\sum\limits_{k = 1}^{m}\sum\limits_{l = 1}^{n}\bigg\{1-\frac{1}{\mathfrak{h}^{2}}(\xi_{kl}^{p}-\eta_{kl}^{p})(\xi_{kl}^{p}+\eta_{kl}^{p}) \bigg\} ,\\ \end{align} | (4.6) |
where k = 1, 2, \ldots, m, l = 1, 2, \ldots, n and p = 1, 2, \ldots, t.
Next, the weights \beta_{p} of experts \mathcal{E}\mathfrak{x}_{i} are calculated as:
\begin{align} \beta_{p}\; = \; &\frac{\varsigma^{p}}{\sum\nolimits_{p = 1}^{t}\varsigma^{p}}, \end{align} | (4.7) |
where \beta_{p}\in[0, 1] and \sum_{p = 1}^{t}\beta_{p} = 1.
Step 3: Further, we accumulate the L q -ROFNs \varpi_{kl}^{1}, \varpi_{kl}^{2}, \ldots, \varpi_{kl}^{t} given in decision matrices \mathbb{D}^{1} = \bigg(\varpi_{kl}^{1}\bigg)_{m\times n}, \mathbb{D}^{2} = \bigg(\varpi_{kl}^{2}\bigg)_{m\times n}, \ldots, \mathbb{D}^{t} = \bigg(\varpi_{kl}^{t}\bigg)_{m\times n} , respectively, by applying the suggested L q -ROFAAWA AgOp in the form of an accumulated L q -ROFN \varpi_{kl} that is helpful in the construction of cumulative decision matrix \mathbb{D} = \bigg(\varpi_{kl}\bigg)_{m\times n} = \bigg(\bigg\langle \mathfrak{a}_{\xi_{kl}}, \; \mathfrak{a}_{\eta_{kl}} \bigg\rangle\bigg)_{m\times n} , where
\begin{align} \varpi_{kl} = \; &Lq-ROFAAWA(\varpi_{kl}^{1},\varpi_{kl}^{2},\ldots,\varpi_{kl}^{t}),\\ = \; &\left\langle \mathfrak{a}_{\mathfrak{h}\sqrt[q]{1- \exp^{- \bigg(\sum\limits_{p = 1}^{t}\beta_{p}\big(-\log(1-(\frac{\xi_{kl}^{'p}}{\mathfrak{h}})^{q})\big)^{\lambda}\bigg)^{1/\lambda}}}},\; \mathfrak{a}_{\mathfrak{h}\exp^{-\bigg(\sum\limits_{p = 1}^{t}\beta_{p}\big(-\log(\frac{\eta_{kl}^{'p}}{\mathfrak{h}})\big)^{\lambda}\bigg)^{1/\lambda}}}\right \rangle, \end{align} | (4.8) |
where k = 1, 2, \ldots, m, l = 1, 2, \ldots, n , and p = 1, 2, \ldots, t with \lambda > 0.
Step 4: The entropy \varsigma_{l} of the attribute \mathcal{S}_{l} is calculated using the L q -ROFNs used in the non-decision matrix \mathbb{D}^{p} = \bigg(\varpi_{kl}^{p}\bigg)_{m\times n} = \bigg(\bigg\langle \mathfrak{a}_{\xi_{kl}^{p}}, \; \mathfrak{a}_{\eta_{kl}^{'p}} \bigg\rangle\bigg)_{m\times n} as below:
\begin{align} \varsigma_{l}\; = \; &\frac{1}{m}\sum\limits_{k = 1}^{m}\bigg\{1-\frac{1}{\mathfrak{h}^{2}}(\xi_{kl}-\eta_{kl})(\xi_{kl}+\eta_{kl}) \bigg\},\\ \end{align} | (4.9) |
where k = 1, 2, \ldots, m and l = 1, 2, \ldots, n.
The weights \mathfrak{w}_{l} of attribute \mathcal{S}_{l} are calculated by
\begin{align} \mathfrak{w}_{l}\; = \; &\frac{\varsigma_{l}}{\sum\nolimits_{l = 1}^{n}\varsigma_{l}} ,\\ \end{align} | (4.10) |
where \mathfrak{w}_{l}\in[0, 1] and \sum_{l = 1}^{n}\mathfrak{w}_{l} = 1.
Step 5: Using the following formula of L q -ROFAAWA AgOp, we accumulate the L q -ROFNs \varpi_{k1}, \varpi_{k2}, \ldots, \varpi_{kn} given in kth row of cumulative decision matrix \mathbb{D} = \bigg(\varpi_{kl}\bigg)_{m\times n} = \bigg(\bigg\langle \mathfrak{a}_{\xi_{kl}}, \; \mathfrak{a}_{\eta_{kl}} \bigg\rangle\bigg)_{m\times n} to obtain the overall accumulated L q -ROFN \varpi_{k} = \langle \mathfrak{a}_{\xi_{k}}, \; \mathfrak{a}_{\eta_{k}}\rangle of alternative \mathcal{R}_{k} .
\begin{align} \varpi_{k} = \; &\left\langle \mathfrak{a}_{\mathfrak{h}\sqrt[q]{1- \exp^{- \bigg(\sum\nolimits_{l = 1}^{n}\mathfrak{w}_{l}\big(-\log(1-(\frac{\xi_{kl}}{\mathfrak{h}})^{q})\big)^{\lambda}\bigg)^{1/\lambda}}}},\; \mathfrak{a}_{\mathfrak{h}\exp^{-\bigg(\sum\nolimits_{l = 1}^{n}\mathfrak{w}_{l}\big(-\log(\frac{\eta_{kl}}{\mathfrak{h}})\big)^{\lambda}\bigg)^{1/\lambda}}}\right \rangle, \end{align} | (4.11) |
where k = 1, 2, \ldots, m and \lambda > 0.
Step 6: We now find the ranking value \mathcal{Y}(\varpi_{k}) of corresponding alternative \mathcal{R}_{k} using the overall accumulated L q -ROFNs as follows:
\begin{align} \mathcal{Y}(\varpi_{k}) = \; &\frac{1}{\mathfrak{h}}(\mathfrak{h}-\frac{\pi_{k}}{2})(\xi_{k}+\frac{\pi_{k}}{2}), \end{align} | (4.12) |
where \pi_{k} = (\mathfrak{h}^{q}-\xi^{q}-\eta^{q})^{1/q} and k = 1, 2, \ldots, m.
Step 7: At last, by comparing the ranking values \mathcal{Y}(\varpi_{1}), \mathcal{Y}(\varpi_{2}), \ldots, \mathcal{Y}(\varpi_{k}) of the alternatives \mathcal{R}_{1}, \mathcal{R}_{2}, \ldots, \mathcal{R}_{k}, respectively, find the larger ranking value \mathcal{Y}(\varpi_{k}) that gives the better \mathcal{RO} of the corresponding alternative \mathcal{R}_{k}. If the ranking values of alternatives \mathcal{R}_{x} and \mathcal{R}_{y} are same, i.e., \mathcal{Y}(\varpi_{x}) = \mathcal{Y}(\varpi_{y}) , then calculate the values of accuracy function \mathcal{J}(\varpi_{x}) and \mathcal{J}(\varpi_{y}) . The larger accuracy value represents the better \mathcal{RO} . For two or more alternatives, if \mathcal{Y}(\varpi_{x}) = \mathcal{Y}(\varpi_{y}) and \mathcal{J}(\varpi_{x}) = \mathcal{J}(\varpi_{y}) , then the alternatives \mathcal{R}_{x} and \mathcal{R}_{y} have the same \mathcal{RO}s .
In the following, we apply the developed second GDM method on three examples.
Example 4.4. The selection of a mobile phone requires a complex decision-making exercise. The users usually consider different features, including processing power, battery life, and camera quality. Moreover, the operating system, and software updates are also crucial characteristics in the selection procedure. There is another critical factor, i.e., budget constraints. The user's reviews, recommendations of experts, and brand reputation also assist the decision-making process. Consider a user who wants to buy the best mobile phone from the alternatives \mathcal{R}_{1} , \mathcal{R}_{2} , \mathcal{R}_{3} , and \mathcal{R}_{4} , and the attributes are \mathcal{S}_{1} (camera quality), \mathcal{S}_{2} (security alerts), \mathcal{S}_{3} (innovation), \mathcal{S}_{4} (reliability), and \mathcal{S}_{5} (brand reputation), which are estimated by the experts \mathcal{E}\mathfrak{x}_{1} , \mathcal{E}\mathfrak{x}_{2} , and \mathcal{E}\mathfrak{x}_{3} . The experts estimate each alternative \mathcal{R}_{k} using L q -ROFNs \varpi_{kl}^{'p} on the basis of L^{T}S = \{\mathfrak{a}_{0} = {\rm extremely\; poor}, \mathfrak{a}_{1} = {\rm too\; poor}\; \mathfrak{a}_{2} = {\rm very\; poor}, \mathfrak{a}_{3} = {\rm poor}, \mathfrak{a}_{4} = {\rm fair}, \mathfrak{a}_{5} = {\rm little\; poor}, \mathfrak{a}_{6} = {\rm slightly\; good}, \mathfrak{a}_{7} = {\rm good}, \mathfrak{a}_{8} = {\rm very\; good}, \mathfrak{a}_{9} = {\rm too\; good}, \mathfrak{a}_{10} = {\rm {extremely}\; good}\} in relation to attribute \mathcal{S}_{l} to create decision-matrices \mathbb{D}^{'1} = \bigg(\varpi_{kl}^{'1}\bigg)_{6\times 7}, \mathbb{D}^{'2} = \bigg(\varpi_{kl}^{'2}\bigg)_{6\times 7}, and \mathbb{D}^{'3} = \bigg(\varpi_{kl}^{'3}\bigg)_{6\times 7} that are given as below:
\begin{aligned} & \begin{array}{lllll} \quad\quad\quad\quad\quad\quad\quad \mathcal{S}_{1} &\quad\quad\mathcal{S}_{2} & \quad\quad\mathcal{S}_{3} & \quad\quad\mathcal{S}_{4} &\quad\quad\quad\mathcal{S}_{5} \end{array} \\ & \mathbb{D}^{'1} = \begin{array}{c} \mathcal{R}_{1} \\ \mathcal{R}_{2}\\ \mathcal{R}_{3}\\ \mathcal{R}_{4} \end{array}\left(\begin{array}{ccccc} \langle \mathfrak{a}_{5},\; \mathfrak{a}_{4}\rangle & \langle \mathfrak{a}_{5},\; \mathfrak{a}_{3}\rangle & \langle \mathfrak{a}_{6},\; \mathfrak{a}_{2}\rangle & \langle \mathfrak{a}_{4},\; \mathfrak{a}_{5}\rangle & \langle \mathfrak{a}_{6},\; \mathfrak{a}_{3}\rangle \\ \langle \mathfrak{a}_{7},\; \mathfrak{a}_{2}\rangle & \langle \mathfrak{a}_{3},\; \mathfrak{a}_{6}\rangle & \langle \mathfrak{a}_{5},\; \mathfrak{a}_{3}\rangle & \langle \mathfrak{a}_{3},\; \mathfrak{a}_{6}\rangle & \langle \mathfrak{a}_{5},\; \mathfrak{a}_{4}\rangle\\ \langle \mathfrak{a}_{6},\; \mathfrak{a}_{3}\rangle & \langle \mathfrak{a}_{4},\; \mathfrak{a}_{4}\rangle & \langle \mathfrak{a}_{4},\; \mathfrak{a}_{5}\rangle & \langle \mathfrak{a}_{5},\; \mathfrak{a}_{4}\rangle & \langle \mathfrak{a}_{7},\; \mathfrak{a}_{2}\rangle\\ \langle \mathfrak{a}_{4},\; \mathfrak{a}_{4}\rangle & \langle \mathfrak{a}_{6},\; \mathfrak{a}_{3}\rangle & \langle \mathfrak{a}_{3},\; \mathfrak{a}_{6}\rangle & \langle \mathfrak{a}_{7},\; \mathfrak{a}_{2}\rangle & \langle \mathfrak{a}_{5},\; \mathfrak{a}_{4}\rangle \end{array}\right), & \end{aligned} |
\begin{aligned} & \begin{array}{lllll} \quad\quad\quad\quad\quad\quad\quad \mathcal{S}_{1} &\quad\quad\mathcal{S}_{2} & \quad\quad\mathcal{S}_{3} & \quad\quad\mathcal{S}_{4} &\quad\quad\quad\mathcal{S}_{5} \end{array} \\ & \mathbb{D}^{'2} = \begin{array}{c} \mathcal{R}_{1} \\ \mathcal{R}_{2}\\ \mathcal{R}_{3}\\ \mathcal{R}_{4} \end{array}\left(\begin{array}{ccccc} \langle \mathfrak{a}_{6},\; \mathfrak{a}_{2}\rangle & \langle \mathfrak{a}_{4},\; \mathfrak{a}_{5}\rangle & \langle \mathfrak{a}_{6},\; \mathfrak{a}_{3}\rangle & \langle \mathfrak{a}_{5},\; \mathfrak{a}_{3}\rangle & \langle \mathfrak{a}_{6},\; \mathfrak{a}_{2}\rangle \\ \langle \mathfrak{a}_{5},\; \mathfrak{a}_{3}\rangle & \langle \mathfrak{a}_{3},\; \mathfrak{a}_{6}\rangle & \langle \mathfrak{a}_{5},\; \mathfrak{a}_{4}\rangle & \langle \mathfrak{a}_{3},\; \mathfrak{a}_{6}\rangle & \langle \mathfrak{a}_{5},\; \mathfrak{a}_{3}\rangle\\ \langle \mathfrak{a}_{4},\; \mathfrak{a}_{5}\rangle & \langle \mathfrak{a}_{5},\; \mathfrak{a}_{4}\rangle & \langle \mathfrak{a}_{7},\; \mathfrak{a}_{2}\rangle & \langle \mathfrak{a}_{4},\; \mathfrak{a}_{4}\rangle & \langle \mathfrak{a}_{4},\; \mathfrak{a}_{5}\rangle\\ \langle \mathfrak{a}_{3},\; \mathfrak{a}_{6}\rangle & \langle \mathfrak{a}_{7},\; \mathfrak{a}_{2}\rangle & \langle \mathfrak{a}_{5},\; \mathfrak{a}_{4}\rangle & \langle \mathfrak{a}_{6},\; \mathfrak{a}_{3}\rangle & \langle \mathfrak{a}_{3},\; \mathfrak{a}_{6}\rangle \end{array}\right), & \end{aligned} |
\begin{aligned} & \begin{array}{lllll} \quad\quad\quad\quad\quad\quad\quad \mathcal{S}_{1} &\quad\quad\mathcal{S}_{2} & \quad\quad\mathcal{S}_{3} & \quad\quad\mathcal{S}_{4} &\quad\quad\quad\mathcal{S}_{5} \end{array} \\ & \mathbb{D}^{'3} = \begin{array}{c} \mathcal{R}_{1} \\ \mathcal{R}_{2}\\ \mathcal{R}_{3}\\ \mathcal{R}_{4} \end{array}\left(\begin{array}{ccccc} \langle \mathfrak{a}_{3},\; \mathfrak{a}_{6}\rangle & \langle \mathfrak{a}_{7},\; \mathfrak{a}_{2}\rangle & \langle \mathfrak{a}_{5},\; \mathfrak{a}_{4}\rangle & \langle \mathfrak{a}_{4},\; \mathfrak{a}_{4}\rangle & \langle \mathfrak{a}_{4},\; \mathfrak{a}_{5}\rangle \\ \langle \mathfrak{a}_{4},\; \mathfrak{a}_{5}\rangle & \langle \mathfrak{a}_{5},\; \mathfrak{a}_{4}\rangle & \langle \mathfrak{a}_{7},\; \mathfrak{a}_{2}\rangle & \langle \mathfrak{a}_{5},\; \mathfrak{a}_{4}\rangle & \langle \mathfrak{a}_{6},\; \mathfrak{a}_{3}\rangle\\ \langle \mathfrak{a}_{7},\; \mathfrak{a}_{2}\rangle & \langle \mathfrak{a}_{4},\; \mathfrak{a}_{4}\rangle & \langle \mathfrak{a}_{4},\; \mathfrak{a}_{5}\rangle & \langle \mathfrak{a}_{5},\; \mathfrak{a}_{4}\rangle & \langle \mathfrak{a}_{7},\; \mathfrak{a}_{2}\rangle\\ \langle \mathfrak{a}_{5},\; \mathfrak{a}_{4}\rangle & \langle \mathfrak{a}_{6},\; \mathfrak{a}_{3}\rangle & \langle \mathfrak{a}_{3},\; \mathfrak{a}_{6}\rangle & \langle \mathfrak{a}_{3},\; \mathfrak{a}_{6}\rangle & \langle \mathfrak{a}_{7},\; \mathfrak{a}_{2}\rangle \end{array}\right). & \end{aligned} |
We now utilize the suggested second GDM method to solve this example as below:
Step 1: As we know all the attributes are asset type, we get the new-decision matrices \mathbb{D}^{1} = \bigg(\varpi_{kl}^{1}\bigg)_{4\times 5} = \bigg(\bigg\langle \mathfrak{a}_{\xi_{kl}^{1}}, \; \mathfrak{a}_{\eta_{kl}^{'1}} \bigg\rangle\bigg)_{4\times 5} , \mathbb{D}^{2} = \bigg(\varpi_{kl}^{2}\bigg)_{4\times 5} = \bigg(\bigg\langle \mathfrak{a}_{\xi_{kl}^{2}}, \; \mathfrak{a}_{\eta_{kl}^{'2}} \bigg\rangle\bigg)_{4\times 5} , and \mathbb{D}^{3} = \bigg(\varpi_{kl}^{3}\bigg)_{4\times 5} = \bigg(\bigg\langle \mathfrak{a}_{\xi_{kl}^{3}}, \; \mathfrak{a}_{\eta_{kl}^{'3}} \bigg\rangle\bigg)_{4\times 5}.
Step 2: Using Eq (4.6), we get the entropies \varsigma^{1}, \varsigma^{2}, and \varsigma^{3} of the experts \mathcal{E}\mathfrak{x}_{1}, \mathcal{E}\mathfrak{x}_{2}, and \mathcal{E}\mathfrak{x}_{3} , respectively, which are \varsigma^{1} = 0.7925 , \varsigma^{2} = 0.7910 , and \varsigma^{3} = 0.7840. The weights of experts are calculated using Eq (4.7) such that \beta_{1} = 0.3347, \beta_{2} = 0.3341 and \beta_{3} = 0.3312.
Step 3: By applying Eq (4.8), we find the accumulated L q -ROFNs \varpi_{kl} from the L q -ROFNs \varpi_{kl}^{1}, \varpi_{kl}^{2}, and \varpi_{kl}^{3} belongs to the new-decision matrices \mathbb{D}^{1} = \bigg(\varpi_{kl}^{1}\bigg)_{4\times 5} = \bigg(\bigg\langle \mathfrak{a}_{\xi_{kl}^{1}}, \; \mathfrak{a}_{\eta_{kl}^{'1}} \bigg\rangle\bigg)_{4\times 5} , \mathbb{D}^{2} = \bigg(\varpi_{kl}^{2}\bigg)_{4\times 5} = \bigg(\bigg\langle \mathfrak{a}_{\xi_{kl}^{2}}, \; \mathfrak{a}_{\eta_{kl}^{'2}} \bigg\rangle\bigg)_{4\times 5} , and \mathbb{D}^{3} = \bigg(\varpi_{kl}^{3}\bigg)_{4\times 5} = \bigg(\bigg\langle \mathfrak{a}_{\xi_{kl}^{3}}, \; \mathfrak{a}_{\eta_{kl}^{'3}} \bigg\rangle\bigg)_{4\times 5} to construct the cumulative decision matrix \mathbb{D} = \bigg(\varpi_{kl}\bigg)_{4\times 5} = \bigg(\bigg\langle \mathfrak{a}_{\xi_{kl}}, \; \mathfrak{a}_{\eta_{kl}} \bigg\rangle\bigg)_{4\times 5} with k = 1, 2, \ldots, 4 , and l = 1, 2, \ldots, 5 as below:
\begin{aligned} & \begin{array}{lllll} \quad\quad\quad\quad\quad\quad\quad \mathcal{S}_{1} &\quad\quad\quad\quad\mathcal{S}_{2} & \quad\quad\quad\quad\mathcal{S}_{3} & \quad\quad\quad\quad\mathcal{S}_{4} &\quad\quad\quad\quad\mathcal{S}_{5} \end{array} \\ & \mathbb{D} = \begin{array}{c} \mathcal{R}_{1} \\ \mathcal{R}_{2}\\ \mathcal{R}_{3}\\ \mathcal{R}_{4} \end{array}\left(\begin{array}{ccccc} \langle \mathfrak{a}_{5.29},\; \mathfrak{a}_{3.29}\rangle & \langle \mathfrak{a}_{6.09},\; \mathfrak{a}_{2.93}\rangle & \langle \mathfrak{a}_{5.76},\; \mathfrak{a}_{2.79}\rangle & \langle \mathfrak{a}_{4.47},\; \mathfrak{a}_{3.82}\rangle & \langle \mathfrak{a}_{5.68},\; \mathfrak{a}_{3.37}\rangle \\ \langle \mathfrak{a}_{6.10},\; \mathfrak{a}_{2.93}\rangle & \langle \mathfrak{a}_{4.25},\; \mathfrak{a}_{5.10}\rangle & \langle \mathfrak{a}_{6.15},\; \mathfrak{a}_{2.79}\rangle & \langle \mathfrak{a}_{4.25},\; \mathfrak{a}_{5.10}\rangle & \langle \mathfrak{a}_{5.46},\; \mathfrak{a}_{3.56}\rangle\\ \langle \mathfrak{a}_{6.26},\; \mathfrak{a}_{2.93}\rangle & \langle \mathfrak{a}_{4.47},\; \mathfrak{a}_{4.00}\rangle & \langle \mathfrak{a}_{6.03},\; \mathfrak{a}_{3.36}\rangle & \langle \mathfrak{a}_{4.77},\; \mathfrak{a}_{4.00}\rangle & \langle \mathfrak{a}_{6.63},\; \mathfrak{a}_{2.65}\rangle\\ \langle \mathfrak{a}_{4.37},\; \mathfrak{a}_{4.47}\rangle & \langle \mathfrak{a}_{6.46},\; \mathfrak{a}_{2.58}\rangle & \langle \mathfrak{a}_{4.27},\; \mathfrak{a}_{5.16}\rangle & \langle \mathfrak{a}_{6.26},\; \mathfrak{a}_{3.01}\rangle & \langle \mathfrak{a}_{6.16},\; \mathfrak{a}_{3.30}\rangle \end{array}\right) .\\ & \end{aligned} |
Step 4: Using Eq (4.9), the entropies \varsigma_{1}, \varsigma_{2}, \varsigma_{3}, \varsigma_{4} , and \varsigma_{5} of the attributes \mathcal{S}_{1}, \mathcal{S}_{2}, \mathcal{S}_{3}, \mathcal{S}_{4} , and \mathcal{S}_{5} are calculated as: \varsigma_{1} = 0.81, \varsigma_{2} = 0.85, \varsigma_{3} = 0.82, \varsigma_{4} = 0.91 , and \varsigma_{5} = 0.75 . Moreover, the weights of attributes \mathfrak{w} = \{\mathfrak{w}_{1}(0.20), \mathfrak{w}_{2}(0.21), \mathfrak{w}_{3}(0.19), \mathfrak{w}_{4}(0.22), \mathfrak{w}_{5}(0.18) \} are computed by Eq (4.10).
Step 5: Next, from the Eq (4.11), we combine accumulated L q -ROFNs \varpi_{k} = \langle \mathfrak{a}_{\xi_{k}}, \; \mathfrak{a}_{\eta_{k}}\rangle of corresponding alternative \mathcal{R}_{k} , with k = 1, 2, 3, 4 , which are given as \varpi_{1} = \langle \mathfrak{a}_{5.59}, \; \mathfrak{a}_{3.24}\rangle , \varpi_{2} = \langle \mathfrak{a}_{5.55}, \; \mathfrak{a}_{3, 69}\rangle , \varpi_{3} = \langle \mathfrak{a}_{5.91}, \; \mathfrak{a}_{3.46}\rangle , and \varpi_{4} = \langle \mathfrak{a}_{5.88}, \; \mathfrak{a}_{3.47}\rangle .
Step 6: Using Eq (4.12), we calculate the ranking values \mathcal{Y}(\varpi_{1}) , \mathcal{Y}(\varpi_{2}) , \mathcal{Y}(\varpi_{3}) , and \mathcal{Y}(\varpi_{4}) as follows: \mathcal{Y}(\varpi_{1}) = 5.50 , \mathcal{Y}(\varpi_{2}) = 5.49 , \mathcal{Y}(\varpi_{3}) = 5.71 , and \mathcal{Y}(\varpi_{4}) = 5.69.
Step 7: From Step 6, we see that \mathcal{Y}(\varpi_{3}) > \mathcal{Y}(\varpi_{4}) > \mathcal{Y}(\varpi_{1}) > \mathcal{Y}(\varpi_{2}), Hence, the best mobile phone from the collection \mathcal{R}_{k} with k = 1, 2, 3, 4 is \mathcal{R}_{3}.
Example 4.5. Everyone can observe the rapid progress occurring globally in various fields. Similarly, the car manufacturing industry is undergoing a transformative shift from conventional fuel to electronic technology. The escalating concerns related to global warming, primarily fueled by high fuel consumption, have led experts to believe that oil reserves may deplete in the coming years. This realization has prompted car manufacturing companies to swiftly transition towards the production of electric vehicles (EVs). The performance of electric vehicles depends on various factors, including battery efficiency, charging infrastructure, and advancements in electric propulsion systems. This paradigm shift reflects a collective effort to address environmental challenges and align with a sustainable future. Assume that the Engineering Council of Pakistan is organizing a car exhibition featuring a competition for electronic cars, where prizes will be awarded to the top three position holders. To select the best designs, the services of Pakistan's leading automobile experts will be enlisted. Suppose \mathcal{R}_{1} , \mathcal{R}_{2} , \mathcal{R}_{3} , and \mathcal{R}_{4} are the alternatives (samples) and the attributes are \mathcal{S}_{1} (battery performance), \mathcal{S}_{2} (power and acceleration), \mathcal{S}_{3} (design and aesthetics), \mathcal{S}_{4} (charging infrastructure compatibility), and \mathcal{S}_{5} (energy efficiency), which are estimated by the experts \mathcal{E}\mathfrak{x}_{1} , \mathcal{E}\mathfrak{x}_{2} , and \mathcal{E}\mathfrak{x}_{3} . The experts estimate each alternative \mathcal{R}_{k} in the form of L q -ROFNs \varpi_{kl}^{'p} on the basis of L^{T}S = \{\mathfrak{a}_{0} = {\rm extremely\; poor}, \; \mathfrak{a}_{1} = {\rm very\; poor}, \; \mathfrak{a}_{2} = {\rm poor}, \mathfrak{a}_{3} = {\rm slightly\; poor}, \; \mathfrak{a}_{4} = {\rm fair}, \; \mathfrak{a}_{5} = {\rm good}, \mathfrak{a}_{6} = {\rm very\; good}, \; \mathfrak{a}_{7} = {\rm too\; good}, \; \mathfrak{a}_{8} = {\rm {extremely}\; good}\} regarding every attribute \mathcal{S}_{l} to create decision-matrices \mathbb{D}^{'1} = \bigg(\varpi_{kl}^{'1}\bigg)_{6\times 7}, \mathbb{D}^{'2} = \bigg(\varpi_{kl}^{'2}\bigg)_{6\times 7}, and \mathbb{D}^{'3} = \bigg(\varpi_{kl}^{'3}\bigg)_{6\times 7} , which are given as:
\begin{aligned} & \begin{array}{lllll} \quad\quad\quad\quad\quad\quad\quad \mathcal{S}_{1} &\quad\quad\mathcal{S}_{2} & \quad\quad\mathcal{S}_{3} & \quad\quad\mathcal{S}_{4} &\quad\quad\quad\mathcal{S}_{5} \end{array} \\ & \mathbb{D}^{'1} = \begin{array}{c} \mathcal{R}_{1} \\ \mathcal{R}_{2}\\ \mathcal{R}_{3}\\ \mathcal{R}_{4} \end{array}\left(\begin{array}{ccccc} \langle \mathfrak{a}_{6},\; \mathfrak{a}_{2}\rangle & \langle \mathfrak{a}_{5},\; \mathfrak{a}_{3}\rangle & \langle \mathfrak{a}_{4},\; \mathfrak{a}_{1}\rangle & \langle \mathfrak{a}_{3},\; \mathfrak{a}_{4}\rangle & \langle \mathfrak{a}_{5},\; \mathfrak{a}_{2}\rangle \\ \langle \mathfrak{a}_{5},\; \mathfrak{a}_{1}\rangle & \langle \mathfrak{a}_{4},\; \mathfrak{a}_{3}\rangle & \langle \mathfrak{a}_{6},\; \mathfrak{a}_{1}\rangle & \langle \mathfrak{a}_{5},\; \mathfrak{a}_{2}\rangle & \langle \mathfrak{a}_{4},\; \mathfrak{a}_{1}\rangle\\ \langle \mathfrak{a}_{8},\; \mathfrak{a}_{0}\rangle & \langle \mathfrak{a}_{5},\; \mathfrak{a}_{1}\rangle & \langle \mathfrak{a}_{4},\; \mathfrak{a}_{3}\rangle & \langle \mathfrak{a}_{6},\; \mathfrak{a}_{2}\rangle & \langle \mathfrak{a}_{6},\; \mathfrak{a}_{1}\rangle\\ \langle \mathfrak{a}_{7},\; \mathfrak{a}_{1}\rangle & \langle \mathfrak{a}_{5},\; \mathfrak{a}_{2}\rangle & \langle \mathfrak{a}_{4},\; \mathfrak{a}_{1}\rangle & \langle \mathfrak{a}_{6},\; \mathfrak{a}_{2}\rangle & \langle \mathfrak{a}_{6},\; \mathfrak{a}_{1}\rangle \end{array}\right), & \end{aligned} |
\begin{aligned} & \begin{array}{lllll} \quad\quad\quad\quad\quad\quad\quad \mathcal{S}_{1} &\quad\quad\mathcal{S}_{2} & \quad\quad\mathcal{S}_{3} & \quad\quad\mathcal{S}_{4} &\quad\quad\quad\mathcal{S}_{5} \end{array} \\ & \mathbb{D}^{'2} = \begin{array}{c} \mathcal{R}_{1} \\ \mathcal{R}_{2}\\ \mathcal{R}_{3}\\ \mathcal{R}_{4} \end{array}\left(\begin{array}{ccccc} \langle \mathfrak{a}_{6},\; \mathfrak{a}_{2}\rangle & \langle \mathfrak{a}_{4},\; \mathfrak{a}_{2}\rangle & \langle \mathfrak{a}_{6},\; \mathfrak{a}_{1}\rangle & \langle \mathfrak{a}_{7},\; \mathfrak{a}_{1}\rangle & \langle \mathfrak{a}_{5},\; \mathfrak{a}_{2}\rangle \\ \langle \mathfrak{a}_{5},\; \mathfrak{a}_{1}\rangle & \langle \mathfrak{a}_{2},\; \mathfrak{a}_{6}\rangle & \langle \mathfrak{a}_{5},\; \mathfrak{a}_{2}\rangle & \langle \mathfrak{a}_{4},\; \mathfrak{a}_{3}\rangle & \langle \mathfrak{a}_{5},\; \mathfrak{a}_{1}\rangle\\ \langle \mathfrak{a}_{7},\; \mathfrak{a}_{1}\rangle & \langle \mathfrak{a}_{5},\; \mathfrak{a}_{1}\rangle & \langle \mathfrak{a}_{8},\; \mathfrak{a}_{0}\rangle & \langle \mathfrak{a}_{4},\; \mathfrak{a}_{4}\rangle & \langle \mathfrak{a}_{5},\; \mathfrak{a}_{2}\rangle\\ \langle \mathfrak{a}_{5},\; \mathfrak{a}_{2}\rangle & \langle \mathfrak{a}_{6},\; \mathfrak{a}_{2}\rangle & \langle \mathfrak{a}_{5},\; \mathfrak{a}_{1}\rangle & \langle \mathfrak{a}_{4},\; \mathfrak{a}_{3}\rangle & \langle \mathfrak{a}_{5},\; \mathfrak{a}_{3}\rangle \end{array}\right), & \end{aligned} |
\begin{aligned} & \begin{array}{lllll} \quad\quad\quad\quad\quad\quad\quad \mathcal{S}_{1} &\quad\quad\mathcal{S}_{2} & \quad\quad\mathcal{S}_{3} & \quad\quad\mathcal{S}_{4} &\quad\quad\quad\mathcal{S}_{5} \end{array} \\ & \mathbb{D}^{'3} = \begin{array}{c} \mathcal{R}_{1} \\ \mathcal{R}_{2}\\ \mathcal{R}_{3}\\ \mathcal{R}_{4} \end{array}\left(\begin{array}{ccccc} \langle \mathfrak{a}_{4},\; \mathfrak{a}_{4}\rangle & \langle \mathfrak{a}_{7},\; \mathfrak{a}_{1}\rangle & \langle \mathfrak{a}_{6},\; \mathfrak{a}_{1}\rangle & \langle \mathfrak{a}_{5},\; \mathfrak{a}_{3}\rangle & \langle \mathfrak{a}_{5},\; \mathfrak{a}_{2}\rangle \\ \langle \mathfrak{a}_{5},\; \mathfrak{a}_{1}\rangle & \langle \mathfrak{a}_{4},\; \mathfrak{a}_{2}\rangle & \langle \mathfrak{a}_{7},\; \mathfrak{a}_{1}\rangle & \langle \mathfrak{a}_{5},\; \mathfrak{a}_{2}\rangle & \langle \mathfrak{a}_{4},\; \mathfrak{a}_{3}\rangle\\ \langle \mathfrak{a}_{7},\; \mathfrak{a}_{1}\rangle & \langle \mathfrak{a}_{5},\; \mathfrak{a}_{3}\rangle & \langle \mathfrak{a}_{6},\; \mathfrak{a}_{1}\rangle & \langle \mathfrak{a}_{7},\; \mathfrak{a}_{1}\rangle & \langle \mathfrak{a}_{4},\; \mathfrak{a}_{2}\rangle\\ \langle \mathfrak{a}_{6},\; \mathfrak{a}_{1}\rangle & \langle \mathfrak{a}_{6},\; \mathfrak{a}_{1}\rangle & \langle \mathfrak{a}_{5},\; \mathfrak{a}_{3}\rangle & \langle \mathfrak{a}_{4},\; \mathfrak{a}_{2}\rangle & \langle \mathfrak{a}_{7},\; \mathfrak{a}_{1}\rangle \end{array}\right). & \end{aligned} |
We again utilize the proposed second GDM method on this example as below:
Step 1: Since all the attributes are asset type, we get the new-decision matrices \mathbb{D}^{1} = \bigg(\varpi_{kl}^{1}\bigg)_{4\times 5} = \bigg(\bigg\langle \mathfrak{a}_{\xi_{kl}^{1}}, \; \mathfrak{a}_{\eta_{kl}^{'1}} \bigg\rangle\bigg)_{4\times 5} , \mathbb{D}^{2} = \bigg(\varpi_{kl}^{2}\bigg)_{4\times 5} = \bigg(\bigg\langle \mathfrak{a}_{\xi_{kl}^{2}}, \; \mathfrak{a}_{\eta_{kl}^{'2}} \bigg\rangle\bigg)_{4\times 5} , and \mathbb{D}^{3} = \bigg(\varpi_{kl}^{3}\bigg)_{4\times 5} = \bigg(\bigg\langle \mathfrak{a}_{\xi_{kl}^{3}}, \; \mathfrak{a}_{\eta_{kl}^{'3}} \bigg\rangle\bigg)_{4\times 5}.
Step 2: Using Eq (4.6), we get the entropies \varsigma^{1}, \varsigma^{2}, and \varsigma^{3} of the experts \mathcal{E}\mathfrak{x}_{1}, \mathcal{E}\mathfrak{x}_{2}, and \mathcal{E}\mathfrak{x}_{3} , respectively, which are \varsigma^{1} = 0.6161 , \varsigma^{2} = 0.6490 , and \varsigma^{3} = 0.5802. The weights of experts are find out by applying the Eq (4.7), which are provided by \beta_{1} = 0.34, \beta_{2} = 0.35 , and \beta_{3} = 0.31.
Step 3: Based on Eq (4.8), we calculate the accumulated L q -ROFNs \varpi_{kl} using the L q -ROFNs \varpi_{kl}^{1}, \varpi_{kl}^{2}, and \varpi_{kl}^{3} , which belongs to the decision matrices \mathbb{D}^{1} = \bigg(\varpi_{kl}^{1}\bigg)_{4\times 5} = \bigg(\bigg\langle \mathfrak{a}_{\xi_{kl}^{1}}, \; \mathfrak{a}_{\eta_{kl}^{'1}} \bigg\rangle\bigg)_{4\times 5} , \mathbb{D}^{2} = \bigg(\varpi_{kl}^{2}\bigg)_{4\times 5} = \bigg(\bigg\langle \mathfrak{a}_{\xi_{kl}^{2}}, \; \mathfrak{a}_{\eta_{kl}^{'2}} \bigg\rangle\bigg)_{4\times 5} , and \mathbb{D}^{3} = \bigg(\varpi_{kl}^{3}\bigg)_{4\times 5} = \bigg(\bigg\langle \mathfrak{a}_{\xi_{kl}^{3}}, \; \mathfrak{a}_{\eta_{kl}^{'3}} \bigg\rangle\bigg)_{4\times 5} to construct the cumulative decision matrix \mathbb{D} = \bigg(\varpi_{kl}\bigg)_{4\times 5} = \bigg(\bigg\langle \mathfrak{a}_{\xi_{kl}}, \; \mathfrak{a}_{\eta_{kl}} \bigg\rangle\bigg)_{4\times 5} with k = 1, 2, \ldots, 4 , and l = 1, 2, \ldots, 5 as given below:
\begin{aligned} & \begin{array}{lllll} \quad\quad\quad\quad\quad\quad\quad \mathcal{S}_{1} &\quad\quad\quad\quad\mathcal{S}_{2} & \quad\quad\quad\quad\mathcal{S}_{3} & \quad\quad\quad\quad\mathcal{S}_{4} &\quad\quad\quad\quad\mathcal{S}_{5} \end{array} \\ & \mathbb{D} = \begin{array}{c} \mathcal{R}_{1} \\ \mathcal{R}_{2}\\ \mathcal{R}_{3}\\ \mathcal{R}_{4} \end{array}\left(\begin{array}{ccccc} \langle \mathfrak{a}_{5.74},\; \mathfrak{a}_{2.37}\rangle & \langle \mathfrak{a}_{6.24},\; \mathfrak{a}_{1.73}\rangle & \langle \mathfrak{a}_{5.71},\; \mathfrak{a}_{1.00}\rangle & \langle \mathfrak{a}_{6.31},\; \mathfrak{a}_{1.96}\rangle & \langle \mathfrak{a}_{5.00},\; \mathfrak{a}_{2.00}\rangle \\ \langle \mathfrak{a}_{5.00},\; \mathfrak{a}_{1.00}\rangle & \langle \mathfrak{a}_{3.74},\; \mathfrak{a}_{3.02}\rangle & \langle \mathfrak{a}_{6.39},\; \mathfrak{a}_{1.23}\rangle & \langle \mathfrak{a}_{4.77},\; \mathfrak{a}_{2.27}\rangle & \langle \mathfrak{a}_{4.51},\; \mathfrak{a}_{1.31}\rangle\\ \langle \mathfrak{a}_{8.00},\; \mathfrak{a}_{0.00}\rangle & \langle \mathfrak{a}_{5.00},\; \mathfrak{a}_{1.31}\rangle & \langle \mathfrak{a}_{8.00},\; \mathfrak{a}_{0.00}\rangle & \langle \mathfrak{a}_{6.36},\; \mathfrak{a}_{1.83}\rangle & \langle \mathfrak{a}_{5.39},\; \mathfrak{a}_{1.52}\rangle\\ \langle \mathfrak{a}_{6.43},\; \mathfrak{a}_{1.23}\rangle & \langle \mathfrak{a}_{5.78},\; \mathfrak{a}_{1.56}\rangle & \langle \mathfrak{a}_{4.78},\; \mathfrak{a}_{1.30}\rangle & \langle \mathfrak{a}_{5.27},\; \mathfrak{a}_{2.27}\rangle & \langle \mathfrak{a}_{6.39},\; \mathfrak{a}_{1.35}\rangle \end{array}\right). & \end{aligned} |
Step 4: The entropies \varsigma_{1}, \varsigma_{2}, \varsigma_{3}, \varsigma_{4} , and \varsigma_{5} of the attributes \mathcal{S}_{1}, \mathcal{S}_{2}, \mathcal{S}_{3}, \mathcal{S}_{4} , and \mathcal{S}_{5} are respectively calculated as \varsigma_{1} = 0.39, \varsigma_{2} = 0.63, \varsigma_{3} = 0.39, \varsigma_{4} = 0.56 , and \varsigma_{5} = 0.58 using the Eq (4.9). The weights of attributes \mathfrak{w} = \{\mathfrak{w}_{1}(0.15), \mathfrak{w}_{2}(0.25), \mathfrak{w}_{3}(0.15), \mathfrak{w}_{4}(0.22), \mathfrak{w}_{5}(0.23) \} are find out by applying the Eq (4.10).
Step 5: Based on Eq (4.11), we combine the accumulated L q -ROFNs \varpi_{k} = \langle \mathfrak{a}_{\xi_{k}}, \; \mathfrak{a}_{\eta_{k}}\rangle of each alternative \mathcal{R}_{k} with k = 1, 2, 3, 4 , which are provided as \varpi_{1} = \langle \mathfrak{a}_{5.96}, \; \mathfrak{a}_{1.81}\rangle , \varpi_{2} = \langle \mathfrak{a}_{5.26}, \; \mathfrak{a}_{1.75}\rangle , \varpi_{3} = \langle \mathfrak{a}_{8.00}, \; \mathfrak{a}_{0.00}\rangle , and \varpi_{4} = \langle \mathfrak{a}_{5.97}, \; \mathfrak{a}_{1.65}\rangle
Step 6: Next, using Eq (4.12), we calculate the ranking values \mathcal{Y}(\varpi_{1}) , \mathcal{Y}(\varpi_{2}) , \mathcal{Y}(\varpi_{3}) , and \mathcal{Y}(\varpi_{4}) of each object as follows: \mathcal{Y}(\varpi_{1}) = 5.43 , \mathcal{Y}(\varpi_{2}) = 4.89 , \mathcal{Y}(\varpi_{3}) = 8.00 , and \mathcal{Y}(\varpi_{4}) = 5.42.
Step 7: From Step 6, we see that \mathcal{Y}(\varpi_{3}) > \mathcal{Y}(\varpi_{1}) > \mathcal{Y}(\varpi_{4}) > \mathcal{Y}(\varpi_{2}), Hence, the best car out of collection \mathcal{R}_{k} with k = 1, 2, 3, 4 is \mathcal{R}_{3}.
The flowchart diagram shown in Figure 1 is suggested to the reader for a better understanding of the process discussed in the above GDM methodologies.
To estimate the authenticity of decision-making approaches, Wang and Triantaphyllou [38] discussed three test criteria as below:
Test 1: In the case of an efficient GDM process, the rank of the optimal object is not affected by changing the sub-optimal object by any bad object without altering the significance of corresponding decision attribute.
Test 2: The transitive property must be held to get an effective decision making approach.
Test 3: If a GDM situation is divided into a sub-GDM parts, and applied the suggested GDM approach to both scenarios, then the ranking of alternatives of sub-GDM problems should be same as the ranking of alternatives as original GDM problem.
First, we verify the reliability and authenticity of our suggested first GDM method by implementing these test criteria to Example 4.2 as given in the previous section.
Test 1: For estimating the authenticity of our suggested first GDM technique using Test 1, we alternate the sub-optimal object \mathcal{R}_{3} in the provided decision matrices \mathbb{D}^{'1}, \mathbb{D}^{'2}, \mathbb{D}^{'3}, \mathbb{D}^{'4}, \mathbb{D}^{'5}, and \mathbb{D}^{'6} of Example 4.2, which are estimated from the experts \mathcal{E}\mathfrak{x}_{s}, where s = 1, 2, 3, \ldots, 6 as given below:
\begin{aligned} & \begin{array}{lllllll} \quad\quad\quad\quad\quad\quad\quad \mathcal{S}_{1} &\quad\quad\mathcal{S}_{2} & \quad\quad\mathcal{S}_{3} & \quad\quad\quad\mathcal{S}_{4} &\quad\quad\quad\mathcal{S}_{5} &\quad\quad\mathcal{S}_{6} & \quad\quad\mathcal{S}_{7} \end{array} \\ & \mathbb{D}^{'1} = \begin{array}{c} \mathcal{R}_{1} \\ \mathcal{R}_{2}\\ \mathcal{R}_{3}\\ \mathcal{R}_{4}\\ \mathcal{R}_{5}\\ \mathcal{R}_{6} \end{array}\left(\begin{array}{ccccccc} \langle \mathfrak{a}_{5},\; \mathfrak{a}_{2}\rangle & \langle \mathfrak{a}_{7},\; \mathfrak{a}_{1}\rangle & \langle \mathfrak{a}_{4},\; \mathfrak{a}_{3}\rangle & \langle \mathfrak{a}_{6},\; \mathfrak{a}_{1}\rangle & \langle \mathfrak{a}_{4},\; \mathfrak{a}_{4}\rangle & \langle \mathfrak{a}_{5},\; \mathfrak{a}_{2}\rangle & \langle \mathfrak{a}_{4},\; \mathfrak{a}_{3}\rangle\\ \langle \mathfrak{a}_{6},\; \mathfrak{a}_{1}\rangle & \langle \mathfrak{a}_{5},\; \mathfrak{a}_{3}\rangle & \langle \mathfrak{a}_{7},\; \mathfrak{a}_{1}\rangle & \langle \mathfrak{a}_{5},\; \mathfrak{a}_{1}\rangle & \langle \mathfrak{a}_{5},\; \mathfrak{a}_{1}\rangle & \langle \mathfrak{a}_{4},\; \mathfrak{a}_{4}\rangle & \langle \mathfrak{a}_{7},\; \mathfrak{a}_{1}\rangle\\ \langle \mathfrak{a}_{6},\; \mathfrak{a}_{2}\rangle & \langle \mathfrak{a}_{5},\; \mathfrak{a}_{2}\rangle & \langle \mathfrak{a}_{6},\; \mathfrak{a}_{1}\rangle & \langle \mathfrak{a}_{6},\; \mathfrak{a}_{2}\rangle & \langle \mathfrak{a}_{7},\; \mathfrak{a}_{1}\rangle & \langle \mathfrak{a}_{5},\; \mathfrak{a}_{1}\rangle & \langle \mathfrak{a}_{3},\; \mathfrak{a}_{2}\rangle\\ \langle \mathfrak{a}_{7},\; \mathfrak{a}_{1}\rangle & \langle \mathfrak{a}_{6},\; \mathfrak{a}_{2}\rangle & \langle \mathfrak{a}_{4},\; \mathfrak{a}_{3}\rangle & \langle \mathfrak{a}_{7},\; \mathfrak{a}_{1}\rangle & \langle \mathfrak{a}_{5},\; \mathfrak{a}_{2}\rangle & \langle \mathfrak{a}_{6},\; \mathfrak{a}_{1}\rangle & \langle \mathfrak{a}_{5},\; \mathfrak{a}_{3}\rangle\\ \langle \mathfrak{a}_{6},\; \mathfrak{a}_{1}\rangle & \langle \mathfrak{a}_{5},\; \mathfrak{a}_{2}\rangle & \langle \mathfrak{a}_{4},\; \mathfrak{a}_{4}\rangle & \langle \mathfrak{a}_{5},\; \mathfrak{a}_{1}\rangle & \langle \mathfrak{a}_{5},\; \mathfrak{a}_{3}\rangle & \langle \mathfrak{a}_{5},\; \mathfrak{a}_{1}\rangle & \langle \mathfrak{a}_{5},\; \mathfrak{a}_{3}\rangle\\ \langle \mathfrak{a}_{5},\; \mathfrak{a}_{1}\rangle & \langle \mathfrak{a}_{6},\; \mathfrak{a}_{1}\rangle & \langle \mathfrak{a}_{5},\; \mathfrak{a}_{3}\rangle & \langle \mathfrak{a}_{6},\; \mathfrak{a}_{1}\rangle & \langle \mathfrak{a}_{3},\; \mathfrak{a}_{2}\rangle & \langle \mathfrak{a}_{5},\; \mathfrak{a}_{2}\rangle & \langle \mathfrak{a}_{4},\; \mathfrak{a}_{3}\rangle\\ \end{array}\right), & \end{aligned} |
\begin{aligned} & \begin{array}{lllllll} \quad\quad\quad\quad\quad\quad\quad \mathcal{S}_{1} &\quad\quad\mathcal{S}_{2} & \quad\quad\mathcal{S}_{3} & \quad\quad\quad\mathcal{S}_{4} &\quad\quad\quad\mathcal{S}_{5} &\quad\quad\mathcal{S}_{6} & \quad\quad\mathcal{S}_{7} \end{array} \\ & \mathbb{D}^{'2} = \begin{array}{c} \mathcal{R}_{1} \\ \mathcal{R}_{2}\\ \mathcal{R}_{3}\\ \mathcal{R}_{4}\\ \mathcal{R}_{5}\\ \mathcal{R}_{6} \end{array}\left(\begin{array}{ccccccc} \langle \mathfrak{a}_{4},\; \mathfrak{a}_{3}\rangle & \langle \mathfrak{a}_{5},\; \mathfrak{a}_{2}\rangle & \langle \mathfrak{a}_{4},\; \mathfrak{a}_{4}\rangle & \langle \mathfrak{a}_{6},\; \mathfrak{a}_{1}\rangle & \langle \mathfrak{a}_{4},\; \mathfrak{a}_{3}\rangle & \langle \mathfrak{a}_{7},\; \mathfrak{a}_{1}\rangle & \langle \mathfrak{a}_{5},\; \mathfrak{a}_{2}\rangle\\ \langle \mathfrak{a}_{4},\; \mathfrak{a}_{1}\rangle & \langle \mathfrak{a}_{6},\; \mathfrak{a}_{3}\rangle & \langle \mathfrak{a}_{5},\; \mathfrak{a}_{1}\rangle & \langle \mathfrak{a}_{4},\; \mathfrak{a}_{2}\rangle & \langle \mathfrak{a}_{4},\; \mathfrak{a}_{4}\rangle & \langle \mathfrak{a}_{6},\; \mathfrak{a}_{1}\rangle & \langle \mathfrak{a}_{4},\; \mathfrak{a}_{1}\rangle\\ \langle \mathfrak{a}_{6},\; \mathfrak{a}_{1}\rangle & \langle \mathfrak{a}_{5},\; \mathfrak{a}_{3}\rangle & \langle \mathfrak{a}_{6},\; \mathfrak{a}_{2}\rangle & \langle \mathfrak{a}_{6},\; \mathfrak{a}_{1}\rangle & \langle \mathfrak{a}_{4},\; \mathfrak{a}_{3}\rangle & \langle \mathfrak{a}_{5},\; \mathfrak{a}_{2}\rangle & \langle \mathfrak{a}_{5},\; \mathfrak{a}_{1}\rangle\\ \langle \mathfrak{a}_{6},\; \mathfrak{a}_{1}\rangle & \langle \mathfrak{a}_{5},\; \mathfrak{a}_{2}\rangle & \langle \mathfrak{a}_{6},\; \mathfrak{a}_{2}\rangle & \langle \mathfrak{a}_{5},\; \mathfrak{a}_{1}\rangle & \langle \mathfrak{a}_{5},\; \mathfrak{a}_{2}\rangle & \langle \mathfrak{a}_{4},\; \mathfrak{a}_{2}\rangle & \langle \mathfrak{a}_{5},\; \mathfrak{a}_{3}\rangle\\ \langle \mathfrak{a}_{5},\; \mathfrak{a}_{1}\rangle & \langle \mathfrak{a}_{4},\; \mathfrak{a}_{2}\rangle & \langle \mathfrak{a}_{6},\; \mathfrak{a}_{2}\rangle & \langle \mathfrak{a}_{5},\; \mathfrak{a}_{3}\rangle & \langle \mathfrak{a}_{4},\; \mathfrak{a}_{3}\rangle & \langle \mathfrak{a}_{5},\; \mathfrak{a}_{1}\rangle & \langle \mathfrak{a}_{4},\; \mathfrak{a}_{3}\rangle\\ \langle \mathfrak{a}_{5},\; \mathfrak{a}_{2}\rangle & \langle \mathfrak{a}_{4},\; \mathfrak{a}_{2}\rangle & \langle \mathfrak{a}_{5},\; \mathfrak{a}_{3}\rangle & \langle \mathfrak{a}_{6},\; \mathfrak{a}_{2}\rangle & \langle \mathfrak{a}_{4},\; \mathfrak{a}_{1}\rangle & \langle \mathfrak{a}_{5},\; \mathfrak{a}_{2}\rangle & \langle \mathfrak{a}_{6},\; \mathfrak{a}_{1}\rangle\\ \end{array}\right), & \end{aligned} |
\begin{aligned} & \begin{array}{lllllll} \quad\quad\quad\quad\quad\quad\quad \mathcal{S}_{1} &\quad\quad\mathcal{S}_{2} & \quad\quad\mathcal{S}_{3} & \quad\quad\quad\mathcal{S}_{4} &\quad\quad\quad\mathcal{S}_{5} &\quad\quad\mathcal{S}_{6} & \quad\quad\mathcal{S}_{7} \end{array} \\ & \mathbb{D}^{'3} = \begin{array}{c} \mathcal{R}_{1} \\ \mathcal{R}_{2}\\ \mathcal{R}_{3}\\ \mathcal{R}_{4}\\ \mathcal{R}_{5}\\ \mathcal{R}_{6} \end{array}\left(\begin{array}{ccccccc} \langle \mathfrak{a}_{7},\; \mathfrak{a}_{1}\rangle & \langle \mathfrak{a}_{5},\; \mathfrak{a}_{1}\rangle & \langle \mathfrak{a}_{4},\; \mathfrak{a}_{3}\rangle & \langle \mathfrak{a}_{4},\; \mathfrak{a}_{1}\rangle & \langle \mathfrak{a}_{6},\; \mathfrak{a}_{1}\rangle & \langle \mathfrak{a}_{5},\; \mathfrak{a}_{2}\rangle & \langle \mathfrak{a}_{4},\; \mathfrak{a}_{2}\rangle\\ \langle \mathfrak{a}_{5},\; \mathfrak{a}_{2}\rangle & \langle \mathfrak{a}_{4},\; \mathfrak{a}_{3}\rangle & \langle \mathfrak{a}_{6},\; \mathfrak{a}_{1}\rangle & \langle \mathfrak{a}_{4},\; \mathfrak{a}_{1}\rangle & \langle \mathfrak{a}_{6},\; \mathfrak{a}_{2}\rangle & \langle \mathfrak{a}_{4},\; \mathfrak{a}_{1}\rangle & \langle \mathfrak{a}_{5},\; \mathfrak{a}_{2}\rangle\\ \langle \mathfrak{a}_{6},\; \mathfrak{a}_{2}\rangle & \langle \mathfrak{a}_{4},\; \mathfrak{a}_{2}\rangle & \langle \mathfrak{a}_{5},\; \mathfrak{a}_{1}\rangle & \langle \mathfrak{a}_{3},\; \mathfrak{a}_{2}\rangle & \langle \mathfrak{a}_{6},\; \mathfrak{a}_{1}\rangle & \langle \mathfrak{a}_{4},\; \mathfrak{a}_{1}\rangle & \langle \mathfrak{a}_{6},\; \mathfrak{a}_{2}\rangle\\ \langle \mathfrak{a}_{4},\; \mathfrak{a}_{2}\rangle & \langle \mathfrak{a}_{5},\; \mathfrak{a}_{2}\rangle & \langle \mathfrak{a}_{4},\; \mathfrak{a}_{1}\rangle & \langle \mathfrak{a}_{5},\; \mathfrak{a}_{1}\rangle & \langle \mathfrak{a}_{4},\; \mathfrak{a}_{2}\rangle & \langle \mathfrak{a}_{5},\; \mathfrak{a}_{1}\rangle & \langle \mathfrak{a}_{5},\; \mathfrak{a}_{3}\rangle\\ \langle \mathfrak{a}_{4},\; \mathfrak{a}_{2}\rangle & \langle \mathfrak{a}_{6},\; \mathfrak{a}_{2}\rangle & \langle \mathfrak{a}_{4},\; \mathfrak{a}_{4}\rangle & \langle \mathfrak{a}_{3},\; \mathfrak{a}_{1}\rangle & \langle \mathfrak{a}_{5},\; \mathfrak{a}_{2}\rangle & \langle \mathfrak{a}_{6},\; \mathfrak{a}_{1}\rangle & \langle \mathfrak{a}_{4},\; \mathfrak{a}_{3}\rangle\\ \langle \mathfrak{a}_{4},\; \mathfrak{a}_{2}\rangle & \langle \mathfrak{a}_{5},\; \mathfrak{a}_{2}\rangle & \langle \mathfrak{a}_{6},\; \mathfrak{a}_{1}\rangle & \langle \mathfrak{a}_{4},\; \mathfrak{a}_{1}\rangle & \langle \mathfrak{a}_{3},\; \mathfrak{a}_{2}\rangle & \langle \mathfrak{a}_{6},\; \mathfrak{a}_{2}\rangle & \langle \mathfrak{a}_{5},\; \mathfrak{a}_{3}\rangle\\ \end{array}\right), & \end{aligned} |
\begin{aligned} & \begin{array}{lllllll} \quad\quad\quad\quad\quad\quad\quad \mathcal{S}_{1} &\quad\quad\mathcal{S}_{2} & \quad\quad\mathcal{S}_{3} & \quad\quad\quad\mathcal{S}_{4} &\quad\quad\quad\mathcal{S}_{5} &\quad\quad\mathcal{S}_{6} & \quad\quad\mathcal{S}_{7} \end{array} \\ & \mathbb{D}^{'4} = \begin{array}{c} \mathcal{R}_{1} \\ \mathcal{R}_{2}\\ \mathcal{R}_{3}\\ \mathcal{R}_{4}\\ \mathcal{R}_{5}\\ \mathcal{R}_{6} \end{array}\left(\begin{array}{ccccccc} \langle \mathfrak{a}_{4},\; \mathfrak{a}_{1}\rangle & \langle \mathfrak{a}_{6},\; \mathfrak{a}_{1}\rangle & \langle \mathfrak{a}_{5},\; \mathfrak{a}_{3}\rangle & \langle \mathfrak{a}_{5},\; \mathfrak{a}_{5}\rangle & \langle \mathfrak{a}_{4},\; \mathfrak{a}_{1}\rangle & \langle \mathfrak{a}_{6},\; \mathfrak{a}_{2}\rangle & \langle \mathfrak{a}_{4},\; \mathfrak{a}_{2}\rangle\\ \langle \mathfrak{a}_{4},\; \mathfrak{a}_{1}\rangle & \langle \mathfrak{a}_{5},\; \mathfrak{a}_{2}\rangle & \langle \mathfrak{a}_{6},\; \mathfrak{a}_{1}\rangle & \langle \mathfrak{a}_{5},\; \mathfrak{a}_{2}\rangle & \langle \mathfrak{a}_{6},\; \mathfrak{a}_{1}\rangle & \langle \mathfrak{a}_{4},\; \mathfrak{a}_{1}\rangle & \langle \mathfrak{a}_{5},\; \mathfrak{a}_{1}\rangle\\ \langle \mathfrak{a}_{6},\; \mathfrak{a}_{2}\rangle & \langle \mathfrak{a}_{5},\; \mathfrak{a}_{2}\rangle & \langle \mathfrak{a}_{6},\; \mathfrak{a}_{1}\rangle & \langle \mathfrak{a}_{6},\; \mathfrak{a}_{2}\rangle & \langle \mathfrak{a}_{7},\; \mathfrak{a}_{1}\rangle & \langle \mathfrak{a}_{5},\; \mathfrak{a}_{1}\rangle & \langle \mathfrak{a}_{3},\; \mathfrak{a}_{2}\rangle\\ \langle \mathfrak{a}_{6},\; \mathfrak{a}_{1}\rangle & \langle \mathfrak{a}_{6},\; \mathfrak{a}_{2}\rangle & \langle \mathfrak{a}_{5},\; \mathfrak{a}_{3}\rangle & \langle \mathfrak{a}_{4},\; \mathfrak{a}_{1}\rangle & \langle \mathfrak{a}_{6},\; \mathfrak{a}_{2}\rangle & \langle \mathfrak{a}_{5},\; \mathfrak{a}_{2}\rangle & \langle \mathfrak{a}_{7},\; \mathfrak{a}_{1}\rangle\\ \langle \mathfrak{a}_{5},\; \mathfrak{a}_{1}\rangle & \langle \mathfrak{a}_{6},\; \mathfrak{a}_{2}\rangle & \langle \mathfrak{a}_{4},\; \mathfrak{a}_{4}\rangle & \langle \mathfrak{a}_{7},\; \mathfrak{a}_{1}\rangle & \langle \mathfrak{a}_{6},\; \mathfrak{a}_{2}\rangle & \langle \mathfrak{a}_{5},\; \mathfrak{a}_{2}\rangle & \langle \mathfrak{a}_{5},\; \mathfrak{a}_{3}\rangle\\ \langle \mathfrak{a}_{6},\; \mathfrak{a}_{2}\rangle & \langle \mathfrak{a}_{5},\; \mathfrak{a}_{1}\rangle & \langle \mathfrak{a}_{6},\; \mathfrak{a}_{2}\rangle & \langle \mathfrak{a}_{7},\; \mathfrak{a}_{1}\rangle & \langle \mathfrak{a}_{4},\; \mathfrak{a}_{1}\rangle & \langle \mathfrak{a}_{5},\; \mathfrak{a}_{2}\rangle & \langle \mathfrak{a}_{6},\; \mathfrak{a}_{1}\rangle\\ \end{array}\right), & \end{aligned} |
\begin{aligned} & \begin{array}{lllllll} \quad\quad\quad\quad\quad\quad\quad \mathcal{S}_{1} &\quad\quad\mathcal{S}_{2} & \quad\quad\mathcal{S}_{3} & \quad\quad\quad\mathcal{S}_{4} &\quad\quad\quad\mathcal{S}_{5} &\quad\quad\mathcal{S}_{6} & \quad\quad\mathcal{S}_{7} \end{array} \\ & \mathbb{D}^{'5} = \begin{array}{c} \mathcal{R}_{1} \\ \mathcal{R}_{2}\\ \mathcal{R}_{3}\\ \mathcal{R}_{4}\\ \mathcal{R}_{5}\\ \mathcal{R}_{6} \end{array}\left(\begin{array}{ccccccc} \langle \mathfrak{a}_{4},\; \mathfrak{a}_{1}\rangle & \langle \mathfrak{a}_{5},\; \mathfrak{a}_{2}\rangle & \langle \mathfrak{a}_{6},\; \mathfrak{a}_{1}\rangle & \langle \mathfrak{a}_{5},\; \mathfrak{a}_{2}\rangle & \langle \mathfrak{a}_{6},\; \mathfrak{a}_{1}\rangle & \langle \mathfrak{a}_{4},\; \mathfrak{a}_{1}\rangle & \langle \mathfrak{a}_{5},\; \mathfrak{a}_{1}\rangle\\ \langle \mathfrak{a}_{4},\; \mathfrak{a}_{1}\rangle & \langle \mathfrak{a}_{6},\; \mathfrak{a}_{1}\rangle & \langle \mathfrak{a}_{5},\; \mathfrak{a}_{3}\rangle & \langle \mathfrak{a}_{5},\; \mathfrak{a}_{5}\rangle & \langle \mathfrak{a}_{4},\; \mathfrak{a}_{1}\rangle & \langle \mathfrak{a}_{6},\; \mathfrak{a}_{2}\rangle & \langle \mathfrak{a}_{4},\; \mathfrak{a}_{2}\rangle\\ \langle \mathfrak{a}_{6},\; \mathfrak{a}_{2}\rangle & \langle \mathfrak{a}_{4},\; \mathfrak{a}_{2}\rangle & \langle \mathfrak{a}_{5},\; \mathfrak{a}_{1}\rangle & \langle \mathfrak{a}_{3},\; \mathfrak{a}_{2}\rangle & \langle \mathfrak{a}_{6},\; \mathfrak{a}_{1}\rangle & \langle \mathfrak{a}_{4},\; \mathfrak{a}_{1}\rangle & \langle \mathfrak{a}_{6},\; \mathfrak{a}_{2}\rangle\\ \langle \mathfrak{a}_{6},\; \mathfrak{a}_{1}\rangle & \langle \mathfrak{a}_{5},\; \mathfrak{a}_{3}\rangle & \langle \mathfrak{a}_{6},\; \mathfrak{a}_{2}\rangle & \langle \mathfrak{a}_{6},\; \mathfrak{a}_{1}\rangle & \langle \mathfrak{a}_{4},\; \mathfrak{a}_{3}\rangle & \langle \mathfrak{a}_{5},\; \mathfrak{a}_{2}\rangle & \langle \mathfrak{a}_{5},\; \mathfrak{a}_{1}\rangle\\ \langle \mathfrak{a}_{5},\; \mathfrak{a}_{2}\rangle & \langle \mathfrak{a}_{7},\; \mathfrak{a}_{1}\rangle & \langle \mathfrak{a}_{4},\; \mathfrak{a}_{3}\rangle & \langle \mathfrak{a}_{6},\; \mathfrak{a}_{1}\rangle & \langle \mathfrak{a}_{4},\; \mathfrak{a}_{4}\rangle & \langle \mathfrak{a}_{5},\; \mathfrak{a}_{2}\rangle & \langle \mathfrak{a}_{4},\; \mathfrak{a}_{3}\rangle\\ \langle \mathfrak{a}_{5},\; \mathfrak{a}_{1}\rangle & \langle \mathfrak{a}_{6},\; \mathfrak{a}_{2}\rangle & \langle \mathfrak{a}_{4},\; \mathfrak{a}_{4}\rangle & \langle \mathfrak{a}_{7},\; \mathfrak{a}_{1}\rangle & \langle \mathfrak{a}_{6},\; \mathfrak{a}_{2}\rangle & \langle \mathfrak{a}_{5},\; \mathfrak{a}_{2}\rangle & \langle \mathfrak{a}_{5},\; \mathfrak{a}_{3}\rangle\\ \end{array}\right), & \end{aligned} |
\begin{aligned} & \begin{array}{lllllll} \quad\quad\quad\quad\quad\quad\quad \mathcal{S}_{1} &\quad\quad\mathcal{S}_{2} & \quad\quad\mathcal{S}_{3} & \quad\quad\quad\mathcal{S}_{4} &\quad\quad\quad\mathcal{S}_{5} &\quad\quad\mathcal{S}_{6} & \quad\quad\mathcal{S}_{7} \end{array} \\ & \mathbb{D}^{'6} = \begin{array}{c} \mathcal{R}_{1} \\ \mathcal{R}_{2}\\ \mathcal{R}_{3}\\ \mathcal{R}_{4}\\ \mathcal{R}_{5}\\ \mathcal{R}_{6} \end{array}\left(\begin{array}{ccccccc} \langle \mathfrak{a}_{5},\; \mathfrak{a}_{2}\rangle & \langle \mathfrak{a}_{6},\; \mathfrak{a}_{1}\rangle & \langle \mathfrak{a}_{5},\; \mathfrak{a}_{2}\rangle & \langle \mathfrak{a}_{7},\; \mathfrak{a}_{1}\rangle & \langle \mathfrak{a}_{5},\; \mathfrak{a}_{2}\rangle & \langle \mathfrak{a}_{6},\; \mathfrak{a}_{1}\rangle & \langle \mathfrak{a}_{5},\; \mathfrak{a}_{3}\rangle\\ \langle \mathfrak{a}_{5},\; \mathfrak{a}_{3}\rangle & \langle \mathfrak{a}_{6},\; \mathfrak{a}_{2}\rangle & \langle \mathfrak{a}_{4},\; \mathfrak{a}_{2}\rangle & \langle \mathfrak{a}_{5},\; \mathfrak{a}_{1}\rangle & \langle \mathfrak{a}_{6},\; \mathfrak{a}_{2}\rangle & \langle \mathfrak{a}_{5},\; \mathfrak{a}_{3}\rangle & \langle \mathfrak{a}_{4},\; \mathfrak{a}_{1}\rangle\\ \langle \mathfrak{a}_{5},\; \mathfrak{a}_{1}\rangle & \langle \mathfrak{a}_{6},\; \mathfrak{a}_{1}\rangle & \langle \mathfrak{a}_{4},\; \mathfrak{a}_{2}\rangle & \langle \mathfrak{a}_{5},\; \mathfrak{a}_{3}\rangle & \langle \mathfrak{a}_{6},\; \mathfrak{a}_{2}\rangle & \langle \mathfrak{a}_{5},\; \mathfrak{a}_{2}\rangle & \langle \mathfrak{a}_{4},\; \mathfrak{a}_{1}\rangle\\ \langle \mathfrak{a}_{5},\; \mathfrak{a}_{1}\rangle & \langle \mathfrak{a}_{6},\; \mathfrak{a}_{2}\rangle & \langle \mathfrak{a}_{4},\; \mathfrak{a}_{4}\rangle & \langle \mathfrak{a}_{7},\; \mathfrak{a}_{1}\rangle & \langle \mathfrak{a}_{6},\; \mathfrak{a}_{2}\rangle & \langle \mathfrak{a}_{5},\; \mathfrak{a}_{2}\rangle & \langle \mathfrak{a}_{5},\; \mathfrak{a}_{3}\rangle\\ \langle \mathfrak{a}_{5},\; \mathfrak{a}_{2}\rangle & \langle \mathfrak{a}_{7},\; \mathfrak{a}_{1}\rangle & \langle \mathfrak{a}_{4},\; \mathfrak{a}_{3}\rangle & \langle \mathfrak{a}_{6},\; \mathfrak{a}_{1}\rangle & \langle \mathfrak{a}_{4},\; \mathfrak{a}_{4}\rangle & \langle \mathfrak{a}_{5},\; \mathfrak{a}_{2}\rangle & \langle \mathfrak{a}_{4},\; \mathfrak{a}_{3}\rangle\\ \langle \mathfrak{a}_{6},\; \mathfrak{a}_{1}\rangle & \langle \mathfrak{a}_{5},\; \mathfrak{a}_{3}\rangle & \langle \mathfrak{a}_{6},\; \mathfrak{a}_{2}\rangle & \langle \mathfrak{a}_{6},\; \mathfrak{a}_{1}\rangle & \langle \mathfrak{a}_{4},\; \mathfrak{a}_{3}\rangle & \langle \mathfrak{a}_{5},\; \mathfrak{a}_{2}\rangle & \langle \mathfrak{a}_{5},\; \mathfrak{a}_{1}\rangle\\ \end{array}\right). & \end{aligned} |
The accumulated L q -ROFNs of the bad alternative \mathcal{R}_{3}^{'} in the given decision making matrices \mathbb{D}^{'1}, \mathbb{D}^{'2}, \mathbb{D}^{'3}, \mathbb{D}^{'4}, \mathbb{D}^{'5}, and \mathbb{D}^{'6} are chosen arbitrary. Thus, the developed decision matrices are \mathbb{D}^{'*1}, \mathbb{D}^{'*2}, \mathbb{D}^{'*3}, \mathbb{D}^{'*4}, \mathbb{D}^{'*5}, and \mathbb{D}^{'*6} as below:
\begin{aligned} & \begin{array}{lllllll} \quad\quad\quad\quad\quad\quad\quad \mathcal{S}_{1} &\quad\quad\mathcal{S}_{2} & \quad\quad\mathcal{S}_{3} & \quad\quad\quad\mathcal{S}_{4} &\quad\quad\quad\mathcal{S}_{5} &\quad\quad\mathcal{S}_{6} & \quad\quad\mathcal{S}_{7} \end{array} \\ & \mathbb{D}^{'*1} = \begin{array}{c} \mathcal{R}_{1} \\ \mathcal{R}_{2}\\ \mathcal{R}_{3}^{'}\\ \mathcal{R}_{4}\\ \mathcal{R}_{5}\\ \mathcal{R}_{6} \end{array}\left(\begin{array}{ccccccc} \langle \mathfrak{a}_{5},\; \mathfrak{a}_{2}\rangle & \langle \mathfrak{a}_{7},\; \mathfrak{a}_{1}\rangle & \langle \mathfrak{a}_{4},\; \mathfrak{a}_{3}\rangle & \langle \mathfrak{a}_{6},\; \mathfrak{a}_{1}\rangle & \langle \mathfrak{a}_{4},\; \mathfrak{a}_{4}\rangle & \langle \mathfrak{a}_{5},\; \mathfrak{a}_{2}\rangle & \langle \mathfrak{a}_{4},\; \mathfrak{a}_{3}\rangle\\ \langle \mathfrak{a}_{6},\; \mathfrak{a}_{1}\rangle & \langle \mathfrak{a}_{5},\; \mathfrak{a}_{3}\rangle & \langle \mathfrak{a}_{7},\; \mathfrak{a}_{1}\rangle & \langle \mathfrak{a}_{5},\; \mathfrak{a}_{1}\rangle & \langle \mathfrak{a}_{5},\; \mathfrak{a}_{1}\rangle & \langle \mathfrak{a}_{4},\; \mathfrak{a}_{4}\rangle & \langle \mathfrak{a}_{7},\; \mathfrak{a}_{1}\rangle\\ \langle \mathfrak{a}_{4},\; \mathfrak{a}_{2}\rangle & \langle \mathfrak{a}_{5},\; \mathfrak{a}_{2}\rangle & \langle \mathfrak{a}_{5},\; \mathfrak{a}_{1}\rangle & \langle \mathfrak{a}_{7},\; \mathfrak{a}_{1}\rangle & \langle \mathfrak{a}_{4},\; \mathfrak{a}_{3}\rangle & \langle \mathfrak{a}_{6},\; \mathfrak{a}_{2}\rangle & \langle \mathfrak{a}_{4},\; \mathfrak{a}_{1}\rangle\\ \langle \mathfrak{a}_{7},\; \mathfrak{a}_{1}\rangle & \langle \mathfrak{a}_{6},\; \mathfrak{a}_{2}\rangle & \langle \mathfrak{a}_{4},\; \mathfrak{a}_{3}\rangle & \langle \mathfrak{a}_{7},\; \mathfrak{a}_{1}\rangle & \langle \mathfrak{a}_{5},\; \mathfrak{a}_{2}\rangle & \langle \mathfrak{a}_{6},\; \mathfrak{a}_{1}\rangle & \langle \mathfrak{a}_{5},\; \mathfrak{a}_{3}\rangle\\ \langle \mathfrak{a}_{6},\; \mathfrak{a}_{1}\rangle & \langle \mathfrak{a}_{5},\; \mathfrak{a}_{2}\rangle & \langle \mathfrak{a}_{4},\; \mathfrak{a}_{4}\rangle & \langle \mathfrak{a}_{5},\; \mathfrak{a}_{1}\rangle & \langle \mathfrak{a}_{5},\; \mathfrak{a}_{3}\rangle & \langle \mathfrak{a}_{5},\; \mathfrak{a}_{1}\rangle & \langle \mathfrak{a}_{5},\; \mathfrak{a}_{3}\rangle\\ \langle \mathfrak{a}_{5},\; \mathfrak{a}_{1}\rangle & \langle \mathfrak{a}_{6},\; \mathfrak{a}_{1}\rangle & \langle \mathfrak{a}_{5},\; \mathfrak{a}_{3}\rangle & \langle \mathfrak{a}_{6},\; \mathfrak{a}_{1}\rangle & \langle \mathfrak{a}_{3},\; \mathfrak{a}_{2}\rangle & \langle \mathfrak{a}_{5},\; \mathfrak{a}_{2}\rangle & \langle \mathfrak{a}_{4},\; \mathfrak{a}_{3}\rangle\\ \end{array}\right), & \end{aligned} |
\begin{aligned} & \begin{array}{lllllll} \quad\quad\quad\quad\quad\quad\quad \mathcal{S}_{1} &\quad\quad\mathcal{S}_{2} & \quad\quad\mathcal{S}_{3} & \quad\quad\quad\mathcal{S}_{4} &\quad\quad\quad\mathcal{S}_{5} &\quad\quad\mathcal{S}_{6} & \quad\quad\mathcal{S}_{7} \end{array} \\ & \mathbb{D}^{'*2} = \begin{array}{c} \mathcal{R}_{1} \\ \mathcal{R}_{2}\\ \mathcal{R}_{3}^{'}\\ \mathcal{R}_{4}\\ \mathcal{R}_{5}\\ \mathcal{R}_{6} \end{array}\left(\begin{array}{ccccccc} \langle \mathfrak{a}_{4},\; \mathfrak{a}_{3}\rangle & \langle \mathfrak{a}_{5},\; \mathfrak{a}_{2}\rangle & \langle \mathfrak{a}_{4},\; \mathfrak{a}_{4}\rangle & \langle \mathfrak{a}_{6},\; \mathfrak{a}_{1}\rangle & \langle \mathfrak{a}_{4},\; \mathfrak{a}_{3}\rangle & \langle \mathfrak{a}_{7},\; \mathfrak{a}_{1}\rangle & \langle \mathfrak{a}_{5},\; \mathfrak{a}_{2}\rangle\\ \langle \mathfrak{a}_{4},\; \mathfrak{a}_{1}\rangle & \langle \mathfrak{a}_{6},\; \mathfrak{a}_{3}\rangle & \langle \mathfrak{a}_{5},\; \mathfrak{a}_{1}\rangle & \langle \mathfrak{a}_{4},\; \mathfrak{a}_{2}\rangle & \langle \mathfrak{a}_{4},\; \mathfrak{a}_{4}\rangle & \langle \mathfrak{a}_{6},\; \mathfrak{a}_{1}\rangle & \langle \mathfrak{a}_{4},\; \mathfrak{a}_{1}\rangle\\ \langle \mathfrak{a}_{5},\; \mathfrak{a}_{3}\rangle & \langle \mathfrak{a}_{4},\; \mathfrak{a}_{3}\rangle & \langle \mathfrak{a}_{6},\; \mathfrak{a}_{2}\rangle & \langle \mathfrak{a}_{7},\; \mathfrak{a}_{1}\rangle & \langle \mathfrak{a}_{5},\; \mathfrak{a}_{2}\rangle & \langle \mathfrak{a}_{4},\; \mathfrak{a}_{3}\rangle & \langle \mathfrak{a}_{6},\; \mathfrak{a}_{1}\rangle\\ \langle \mathfrak{a}_{6},\; \mathfrak{a}_{1}\rangle & \langle \mathfrak{a}_{5},\; \mathfrak{a}_{2}\rangle & \langle \mathfrak{a}_{6},\; \mathfrak{a}_{2}\rangle & \langle \mathfrak{a}_{5},\; \mathfrak{a}_{1}\rangle & \langle \mathfrak{a}_{5},\; \mathfrak{a}_{2}\rangle & \langle \mathfrak{a}_{4},\; \mathfrak{a}_{2}\rangle & \langle \mathfrak{a}_{5},\; \mathfrak{a}_{3}\rangle\\ \langle \mathfrak{a}_{5},\; \mathfrak{a}_{1}\rangle & \langle \mathfrak{a}_{4},\; \mathfrak{a}_{2}\rangle & \langle \mathfrak{a}_{6},\; \mathfrak{a}_{2}\rangle & \langle \mathfrak{a}_{5},\; \mathfrak{a}_{3}\rangle & \langle \mathfrak{a}_{4},\; \mathfrak{a}_{3}\rangle & \langle \mathfrak{a}_{5},\; \mathfrak{a}_{1}\rangle & \langle \mathfrak{a}_{4},\; \mathfrak{a}_{3}\rangle\\ \langle \mathfrak{a}_{5},\; \mathfrak{a}_{2}\rangle & \langle \mathfrak{a}_{4},\; \mathfrak{a}_{2}\rangle & \langle \mathfrak{a}_{5},\; \mathfrak{a}_{3}\rangle & \langle \mathfrak{a}_{6},\; \mathfrak{a}_{2}\rangle & \langle \mathfrak{a}_{4},\; \mathfrak{a}_{1}\rangle & \langle \mathfrak{a}_{5},\; \mathfrak{a}_{2}\rangle & \langle \mathfrak{a}_{6},\; \mathfrak{a}_{1}\rangle\\ \end{array}\right), & \end{aligned} |
\begin{aligned} & \begin{array}{lllllll} \quad\quad\quad\quad\quad\quad\quad \mathcal{S}_{1} &\quad\quad\mathcal{S}_{2} & \quad\quad\mathcal{S}_{3} & \quad\quad\quad\mathcal{S}_{4} &\quad\quad\quad\mathcal{S}_{5} &\quad\quad\mathcal{S}_{6} & \quad\quad\mathcal{S}_{7} \end{array} \\ & \mathbb{D}^{'*3} = \begin{array}{c} \mathcal{R}_{1} \\ \mathcal{R}_{2}\\ \mathcal{R}_{3}^{'}\\ \mathcal{R}_{4}\\ \mathcal{R}_{5}\\ \mathcal{R}_{6} \end{array}\left(\begin{array}{ccccccc} \langle \mathfrak{a}_{7},\; \mathfrak{a}_{1}\rangle & \langle \mathfrak{a}_{5},\; \mathfrak{a}_{1}\rangle & \langle \mathfrak{a}_{4},\; \mathfrak{a}_{3}\rangle & \langle \mathfrak{a}_{4},\; \mathfrak{a}_{1}\rangle & \langle \mathfrak{a}_{6},\; \mathfrak{a}_{1}\rangle & \langle \mathfrak{a}_{5},\; \mathfrak{a}_{2}\rangle & \langle \mathfrak{a}_{4},\; \mathfrak{a}_{2}\rangle\\ \langle \mathfrak{a}_{5},\; \mathfrak{a}_{2}\rangle & \langle \mathfrak{a}_{4},\; \mathfrak{a}_{3}\rangle & \langle \mathfrak{a}_{6},\; \mathfrak{a}_{1}\rangle & \langle \mathfrak{a}_{4},\; \mathfrak{a}_{1}\rangle & \langle \mathfrak{a}_{6},\; \mathfrak{a}_{2}\rangle & \langle \mathfrak{a}_{4},\; \mathfrak{a}_{1}\rangle & \langle \mathfrak{a}_{5},\; \mathfrak{a}_{2}\rangle\\ \langle \mathfrak{a}_{3},\; \mathfrak{a}_{1}\rangle & \langle \mathfrak{a}_{5},\; \mathfrak{a}_{2}\rangle & \langle \mathfrak{a}_{6},\; \mathfrak{a}_{2}\rangle & \langle \mathfrak{a}_{7},\; \mathfrak{a}_{1}\rangle & \langle \mathfrak{a}_{4},\; \mathfrak{a}_{2}\rangle & \langle \mathfrak{a}_{5},\; \mathfrak{a}_{2}\rangle & \langle \mathfrak{a}_{6},\; \mathfrak{a}_{1}\rangle\\ \langle \mathfrak{a}_{4},\; \mathfrak{a}_{2}\rangle & \langle \mathfrak{a}_{5},\; \mathfrak{a}_{2}\rangle & \langle \mathfrak{a}_{4},\; \mathfrak{a}_{1}\rangle & \langle \mathfrak{a}_{5},\; \mathfrak{a}_{1}\rangle & \langle \mathfrak{a}_{4},\; \mathfrak{a}_{2}\rangle & \langle \mathfrak{a}_{5},\; \mathfrak{a}_{1}\rangle & \langle \mathfrak{a}_{5},\; \mathfrak{a}_{3}\rangle\\ \langle \mathfrak{a}_{4},\; \mathfrak{a}_{2}\rangle & \langle \mathfrak{a}_{6},\; \mathfrak{a}_{2}\rangle & \langle \mathfrak{a}_{4},\; \mathfrak{a}_{4}\rangle & \langle \mathfrak{a}_{3},\; \mathfrak{a}_{1}\rangle & \langle \mathfrak{a}_{5},\; \mathfrak{a}_{2}\rangle & \langle \mathfrak{a}_{6},\; \mathfrak{a}_{1}\rangle & \langle \mathfrak{a}_{4},\; \mathfrak{a}_{3}\rangle\\ \langle \mathfrak{a}_{4},\; \mathfrak{a}_{2}\rangle & \langle \mathfrak{a}_{5},\; \mathfrak{a}_{2}\rangle & \langle \mathfrak{a}_{6},\; \mathfrak{a}_{1}\rangle & \langle \mathfrak{a}_{4},\; \mathfrak{a}_{1}\rangle & \langle \mathfrak{a}_{3},\; \mathfrak{a}_{2}\rangle & \langle \mathfrak{a}_{6},\; \mathfrak{a}_{2}\rangle & \langle \mathfrak{a}_{5},\; \mathfrak{a}_{3}\rangle\\ \end{array}\right), & \end{aligned} |
\begin{aligned} & \begin{array}{lllllll} \quad\quad\quad\quad\quad\quad\quad \mathcal{S}_{1} &\quad\quad\mathcal{S}_{2} & \quad\quad\mathcal{S}_{3} & \quad\quad\quad\mathcal{S}_{4} &\quad\quad\quad\mathcal{S}_{5} &\quad\quad\mathcal{S}_{6} & \quad\quad\mathcal{S}_{7} \end{array} \\ & \mathbb{D}^{'*4} = \begin{array}{c} \mathcal{R}_{1} \\ \mathcal{R}_{2}\\ \mathcal{R}_{3}^{'}\\ \mathcal{R}_{4}\\ \mathcal{R}_{5}\\ \mathcal{R}_{6} \end{array}\left(\begin{array}{ccccccc} \langle \mathfrak{a}_{4},\; \mathfrak{a}_{1}\rangle & \langle \mathfrak{a}_{6},\; \mathfrak{a}_{1}\rangle & \langle \mathfrak{a}_{5},\; \mathfrak{a}_{3}\rangle & \langle \mathfrak{a}_{5},\; \mathfrak{a}_{5}\rangle & \langle \mathfrak{a}_{4},\; \mathfrak{a}_{1}\rangle & \langle \mathfrak{a}_{6},\; \mathfrak{a}_{2}\rangle & \langle \mathfrak{a}_{4},\; \mathfrak{a}_{2}\rangle\\ \langle \mathfrak{a}_{4},\; \mathfrak{a}_{1}\rangle & \langle \mathfrak{a}_{5},\; \mathfrak{a}_{2}\rangle & \langle \mathfrak{a}_{6},\; \mathfrak{a}_{1}\rangle & \langle \mathfrak{a}_{5},\; \mathfrak{a}_{2}\rangle & \langle \mathfrak{a}_{6},\; \mathfrak{a}_{1}\rangle & \langle \mathfrak{a}_{4},\; \mathfrak{a}_{1}\rangle & \langle \mathfrak{a}_{5},\; \mathfrak{a}_{1}\rangle\\ \langle \mathfrak{a}_{4},\; \mathfrak{a}_{2}\rangle & \langle \mathfrak{a}_{7},\; \mathfrak{a}_{1}\rangle & \langle \mathfrak{a}_{5},\; \mathfrak{a}_{1}\rangle & \langle \mathfrak{a}_{4},\; \mathfrak{a}_{3}\rangle & \langle \mathfrak{a}_{6},\; \mathfrak{a}_{1}\rangle & \langle \mathfrak{a}_{6},\; \mathfrak{a}_{2}\rangle & \langle \mathfrak{a}_{5},\; \mathfrak{a}_{2}\rangle\\ \langle \mathfrak{a}_{6},\; \mathfrak{a}_{1}\rangle & \langle \mathfrak{a}_{6},\; \mathfrak{a}_{2}\rangle & \langle \mathfrak{a}_{5},\; \mathfrak{a}_{3}\rangle & \langle \mathfrak{a}_{4},\; \mathfrak{a}_{1}\rangle & \langle \mathfrak{a}_{6},\; \mathfrak{a}_{2}\rangle & \langle \mathfrak{a}_{5},\; \mathfrak{a}_{2}\rangle & \langle \mathfrak{a}_{7},\; \mathfrak{a}_{1}\rangle\\ \langle \mathfrak{a}_{5},\; \mathfrak{a}_{1}\rangle & \langle \mathfrak{a}_{6},\; \mathfrak{a}_{2}\rangle & \langle \mathfrak{a}_{4},\; \mathfrak{a}_{4}\rangle & \langle \mathfrak{a}_{7},\; \mathfrak{a}_{1}\rangle & \langle \mathfrak{a}_{6},\; \mathfrak{a}_{2}\rangle & \langle \mathfrak{a}_{5},\; \mathfrak{a}_{2}\rangle & \langle \mathfrak{a}_{5},\; \mathfrak{a}_{3}\rangle\\ \langle \mathfrak{a}_{6},\; \mathfrak{a}_{2}\rangle & \langle \mathfrak{a}_{5},\; \mathfrak{a}_{1}\rangle & \langle \mathfrak{a}_{6},\; \mathfrak{a}_{2}\rangle & \langle \mathfrak{a}_{7},\; \mathfrak{a}_{1}\rangle & \langle \mathfrak{a}_{4},\; \mathfrak{a}_{1}\rangle & \langle \mathfrak{a}_{5},\; \mathfrak{a}_{2}\rangle & \langle \mathfrak{a}_{6},\; \mathfrak{a}_{1}\rangle\\ \end{array}\right), & \end{aligned} |
\begin{aligned} & \begin{array}{lllllll} \quad\quad\quad\quad\quad\quad\quad \mathcal{S}_{1} &\quad\quad\mathcal{S}_{2} & \quad\quad\mathcal{S}_{3} & \quad\quad\quad\mathcal{S}_{4} &\quad\quad\quad\mathcal{S}_{5} &\quad\quad\mathcal{S}_{6} & \quad\quad\mathcal{S}_{7} \end{array} \\ & \mathbb{D}^{'*5} = \begin{array}{c} \mathcal{R}_{1} \\ \mathcal{R}_{2}\\ \mathcal{R}_{3}^{'}\\ \mathcal{R}_{4}\\ \mathcal{R}_{5}\\ \mathcal{R}_{6} \end{array}\left(\begin{array}{ccccccc} \langle \mathfrak{a}_{4},\; \mathfrak{a}_{1}\rangle & \langle \mathfrak{a}_{5},\; \mathfrak{a}_{2}\rangle & \langle \mathfrak{a}_{6},\; \mathfrak{a}_{1}\rangle & \langle \mathfrak{a}_{5},\; \mathfrak{a}_{2}\rangle & \langle \mathfrak{a}_{6},\; \mathfrak{a}_{1}\rangle & \langle \mathfrak{a}_{4},\; \mathfrak{a}_{1}\rangle & \langle \mathfrak{a}_{5},\; \mathfrak{a}_{1}\rangle\\ \langle \mathfrak{a}_{4},\; \mathfrak{a}_{1}\rangle & \langle \mathfrak{a}_{6},\; \mathfrak{a}_{1}\rangle & \langle \mathfrak{a}_{5},\; \mathfrak{a}_{3}\rangle & \langle \mathfrak{a}_{5},\; \mathfrak{a}_{5}\rangle & \langle \mathfrak{a}_{4},\; \mathfrak{a}_{1}\rangle & \langle \mathfrak{a}_{6},\; \mathfrak{a}_{2}\rangle & \langle \mathfrak{a}_{4},\; \mathfrak{a}_{2}\rangle\\ \langle \mathfrak{a}_{5},\; \mathfrak{a}_{3}\rangle & \langle \mathfrak{a}_{4},\; \mathfrak{a}_{3}\rangle & \langle \mathfrak{a}_{6},\; \mathfrak{a}_{2}\rangle & \langle \mathfrak{a}_{3},\; \mathfrak{a}_{2}\rangle & \langle \mathfrak{a}_{5},\; \mathfrak{a}_{2}\rangle & \langle \mathfrak{a}_{5},\; \mathfrak{a}_{1}\rangle & \langle \mathfrak{a}_{7},\; \mathfrak{a}_{1}\rangle\\ \langle \mathfrak{a}_{6},\; \mathfrak{a}_{1}\rangle & \langle \mathfrak{a}_{5},\; \mathfrak{a}_{3}\rangle & \langle \mathfrak{a}_{6},\; \mathfrak{a}_{2}\rangle & \langle \mathfrak{a}_{6},\; \mathfrak{a}_{1}\rangle & \langle \mathfrak{a}_{4},\; \mathfrak{a}_{3}\rangle & \langle \mathfrak{a}_{5},\; \mathfrak{a}_{2}\rangle & \langle \mathfrak{a}_{5},\; \mathfrak{a}_{1}\rangle\\ \langle \mathfrak{a}_{5},\; \mathfrak{a}_{2}\rangle & \langle \mathfrak{a}_{7},\; \mathfrak{a}_{1}\rangle & \langle \mathfrak{a}_{4},\; \mathfrak{a}_{3}\rangle & \langle \mathfrak{a}_{6},\; \mathfrak{a}_{1}\rangle & \langle \mathfrak{a}_{4},\; \mathfrak{a}_{4}\rangle & \langle \mathfrak{a}_{5},\; \mathfrak{a}_{2}\rangle & \langle \mathfrak{a}_{4},\; \mathfrak{a}_{3}\rangle\\ \langle \mathfrak{a}_{5},\; \mathfrak{a}_{1}\rangle & \langle \mathfrak{a}_{6},\; \mathfrak{a}_{2}\rangle & \langle \mathfrak{a}_{4},\; \mathfrak{a}_{4}\rangle & \langle \mathfrak{a}_{7},\; \mathfrak{a}_{1}\rangle & \langle \mathfrak{a}_{6},\; \mathfrak{a}_{2}\rangle & \langle \mathfrak{a}_{5},\; \mathfrak{a}_{2}\rangle & \langle \mathfrak{a}_{5},\; \mathfrak{a}_{3}\rangle\\ \end{array}\right), & \end{aligned} |
\begin{aligned} & \begin{array}{lllllll} \quad\quad\quad\quad\quad\quad\quad \mathcal{S}_{1} &\quad\quad\mathcal{S}_{2} & \quad\quad\mathcal{S}_{3} & \quad\quad\quad\mathcal{S}_{4} &\quad\quad\quad\mathcal{S}_{5} &\quad\quad\mathcal{S}_{6} & \quad\quad\mathcal{S}_{7} \end{array} \\ & \mathbb{D}^{'*6} = \begin{array}{c} \mathcal{R}_{1} \\ \mathcal{R}_{2}\\ \mathcal{R}_{3}^{'}\\ \mathcal{R}_{4}\\ \mathcal{R}_{5}\\ \mathcal{R}_{6} \end{array}\left(\begin{array}{ccccccc} \langle \mathfrak{a}_{5},\; \mathfrak{a}_{2}\rangle & \langle \mathfrak{a}_{6},\; \mathfrak{a}_{1}\rangle & \langle \mathfrak{a}_{5},\; \mathfrak{a}_{2}\rangle & \langle \mathfrak{a}_{7},\; \mathfrak{a}_{1}\rangle & \langle \mathfrak{a}_{5},\; \mathfrak{a}_{2}\rangle & \langle \mathfrak{a}_{6},\; \mathfrak{a}_{1}\rangle & \langle \mathfrak{a}_{5},\; \mathfrak{a}_{3}\rangle\\ \langle \mathfrak{a}_{5},\; \mathfrak{a}_{3}\rangle & \langle \mathfrak{a}_{6},\; \mathfrak{a}_{2}\rangle & \langle \mathfrak{a}_{4},\; \mathfrak{a}_{2}\rangle & \langle \mathfrak{a}_{5},\; \mathfrak{a}_{1}\rangle & \langle \mathfrak{a}_{6},\; \mathfrak{a}_{2}\rangle & \langle \mathfrak{a}_{5},\; \mathfrak{a}_{3}\rangle & \langle \mathfrak{a}_{4},\; \mathfrak{a}_{1}\rangle\\ \langle \mathfrak{a}_{6},\; \mathfrak{a}_{2}\rangle & \langle \mathfrak{a}_{5},\; \mathfrak{a}_{2}\rangle & \langle \mathfrak{a}_{4},\; \mathfrak{a}_{3}\rangle & \langle \mathfrak{a}_{5},\; \mathfrak{a}_{3}\rangle & \langle \mathfrak{a}_{7},\; \mathfrak{a}_{1}\rangle & \langle \mathfrak{a}_{6},\; \mathfrak{a}_{2}\rangle & \langle \mathfrak{a}_{3},\; \mathfrak{a}_{2}\rangle\\ \langle \mathfrak{a}_{5},\; \mathfrak{a}_{1}\rangle & \langle \mathfrak{a}_{6},\; \mathfrak{a}_{2}\rangle & \langle \mathfrak{a}_{4},\; \mathfrak{a}_{4}\rangle & \langle \mathfrak{a}_{7},\; \mathfrak{a}_{1}\rangle & \langle \mathfrak{a}_{6},\; \mathfrak{a}_{2}\rangle & \langle \mathfrak{a}_{5},\; \mathfrak{a}_{2}\rangle & \langle \mathfrak{a}_{5},\; \mathfrak{a}_{3}\rangle\\ \langle \mathfrak{a}_{5},\; \mathfrak{a}_{2}\rangle & \langle \mathfrak{a}_{7},\; \mathfrak{a}_{1}\rangle & \langle \mathfrak{a}_{4},\; \mathfrak{a}_{3}\rangle & \langle \mathfrak{a}_{6},\; \mathfrak{a}_{1}\rangle & \langle \mathfrak{a}_{4},\; \mathfrak{a}_{4}\rangle & \langle \mathfrak{a}_{5},\; \mathfrak{a}_{2}\rangle & \langle \mathfrak{a}_{4},\; \mathfrak{a}_{3}\rangle\\ \langle \mathfrak{a}_{6},\; \mathfrak{a}_{1}\rangle & \langle \mathfrak{a}_{5},\; \mathfrak{a}_{3}\rangle & \langle \mathfrak{a}_{6},\; \mathfrak{a}_{2}\rangle & \langle \mathfrak{a}_{6},\; \mathfrak{a}_{1}\rangle & \langle \mathfrak{a}_{4},\; \mathfrak{a}_{3}\rangle & \langle \mathfrak{a}_{5},\; \mathfrak{a}_{2}\rangle & \langle \mathfrak{a}_{5},\; \mathfrak{a}_{1}\rangle\\ \end{array}\right). & \end{aligned} |
The ranking values of \mathcal{Y}(\varpi_{1}) , \mathcal{Y}(\varpi_{2}) , \mathcal{Y}(\varpi_{3}) , \mathcal{Y}(\varpi_{4}) , \mathcal{Y}(\varpi_{5}) , and \mathcal{Y}(\varpi_{6}) are calculated as \mathcal{Y}(\varpi_{1}) = 5.38 , \mathcal{Y}(\varpi_{2}) = 5.06 , \mathcal{Y}(\varpi_{3}) = 5.23 , \mathcal{Y}(\varpi_{4}) = 5.16 , \mathcal{Y}(\varpi_{5}) = 5.12 , and \mathcal{Y}(\varpi_{6}) = 5.02. Next, by applying the suggested first GDM approach on the above determined decision matrices \mathbb{D}^{'*1}, \mathbb{D}^{'*2}, \mathbb{D}^{'*3}, \mathbb{D}^{'*4}, \mathbb{D}^{'*5}, and \mathbb{D}^{'*6} . Clearly, \mathcal{Y}(\varpi_{1}) > \mathcal{Y}(\varpi_{3}) > \mathcal{Y}(\varpi_{4}) > \mathcal{Y}(\varpi_{5}) > \mathcal{Y}(\varpi_{2}) > \mathcal{Y}(\varpi_{6}). So, the most suitable choice from all the other choices \mathcal{R}_{k} with k = 1, 2, \ldots, 6 is \mathcal{R}_{1}. Note that the object \mathcal{R}_{1} is the best alternative by applying the suggested first GDM approach on both the original decision matrices and the substituted decision matrices. Hence, the suggested first GDM method verifies Test Criterion 1.
Tests Criteria 2 ^{\rm nd} and 3 ^{\rm rd} : For investigating the authenticity of the first GDM method using 2nd and 3rd test criteria, we divide the original GDM situation of Example 4.2 into three sub-GDM parts where the objects are \{\mathcal{R}_{1}, \mathcal{R}_{3}, \mathcal{R}_{4}, \mathcal{R}_{5}\} , \{\mathcal{R}_{2}, \mathcal{R}_{3}, \mathcal{R}_{4}, \mathcal{R}_{5}\} , and \{\mathcal{R}_{3}, \mathcal{R}_{5}, \mathcal{R}_{2}, \mathcal{R}_{6}\} . Now, by employing the suggested first GDM method on these sub-problems, we obtain the rankings as ''\mathcal{R}_{1} > \mathcal{R}_{4} > \mathcal{R}_{3} > \mathcal{R}_{5}", ''\mathcal{R}_{4} > \mathcal{R}_{3} > \mathcal{R}_{5} > \mathcal{R}_{2}" , and ''\mathcal{R}_{3} > \mathcal{R}_{5} > \mathcal{R}_{2} > \mathcal{R}_{6}" . To verify the transitivity, these three rankings of sub-GDM problems are merged, and the final ranking is provided by ''\mathcal{R}_{1} > \mathcal{R}_{4} > \mathcal{R}_{3} > \mathcal{R}_{5}, \mathcal{R}_{2} > \mathcal{R}_{6}", which is similar to the ranking orders of alternatives \mathcal{R}_{1}, \mathcal{R}_{2}, \mathcal{R}_{3}, \mathcal{R}_{4}, \mathcal{R}_{5} and \mathcal{R}_{6} as determined in the Example 4.2. Thus, the suggested 1 ^{\rm st} GDM method verifies the Tests Criteria 2 ^{\rm nd} and 3 ^{\rm rd} .
We are now ready to check the reliability of the suggested second GDM method.
Test 1: For estimating the authenticity of our suggested 1 {\rm st} GDM method with Test 1, we put a substitute of the sub-optimal object \mathcal{R}_{3} in the decision matrices \mathbb{D}^{'1}, \mathbb{D}^{'2}, and \mathbb{D}^{'3} of Example 4.5, so, we get
\begin{aligned} & \begin{array}{lllll} \quad\quad\quad\quad\quad\quad\quad \mathcal{S}_{1} &\quad\quad\mathcal{S}_{2} & \quad\quad\mathcal{S}_{3} & \quad\quad\mathcal{S}_{4} &\quad\quad\quad\mathcal{S}_{5} \end{array} \\ & \mathbb{D}^{'1} = \begin{array}{c} \mathcal{R}_{1} \\ \mathcal{R}_{2}\\ \mathcal{R}_{3}\\ \mathcal{R}_{4} \end{array}\left(\begin{array}{ccccc} \langle \mathfrak{a}_{6},\; \mathfrak{a}_{2}\rangle & \langle \mathfrak{a}_{5},\; \mathfrak{a}_{3}\rangle & \langle \mathfrak{a}_{4},\; \mathfrak{a}_{1}\rangle & \langle \mathfrak{a}_{3},\; \mathfrak{a}_{4}\rangle & \langle \mathfrak{a}_{5},\; \mathfrak{a}_{2}\rangle \\ \langle \mathfrak{a}_{5},\; \mathfrak{a}_{1}\rangle & \langle \mathfrak{a}_{4},\; \mathfrak{a}_{3}\rangle & \langle \mathfrak{a}_{6},\; \mathfrak{a}_{1}\rangle & \langle \mathfrak{a}_{5},\; \mathfrak{a}_{2}\rangle & \langle \mathfrak{a}_{4},\; \mathfrak{a}_{1}\rangle\\ \langle \mathfrak{a}_{8},\; \mathfrak{a}_{0}\rangle & \langle \mathfrak{a}_{5},\; \mathfrak{a}_{1}\rangle & \langle \mathfrak{a}_{4},\; \mathfrak{a}_{3}\rangle & \langle \mathfrak{a}_{6},\; \mathfrak{a}_{2}\rangle & \langle \mathfrak{a}_{6},\; \mathfrak{a}_{1}\rangle\\ \langle \mathfrak{a}_{7},\; \mathfrak{a}_{1}\rangle & \langle \mathfrak{a}_{5},\; \mathfrak{a}_{2}\rangle & \langle \mathfrak{a}_{4},\; \mathfrak{a}_{1}\rangle & \langle \mathfrak{a}_{6},\; \mathfrak{a}_{2}\rangle & \langle \mathfrak{a}_{6},\; \mathfrak{a}_{1}\rangle \end{array}\right), & \end{aligned} |
\begin{aligned} & \begin{array}{lllll} \quad\quad\quad\quad\quad\quad\quad \mathcal{S}_{1} &\quad\quad\mathcal{S}_{2} & \quad\quad\mathcal{S}_{3} & \quad\quad\mathcal{S}_{4} &\quad\quad\quad\mathcal{S}_{5} \end{array} \\ & \mathbb{D}^{'2} = \begin{array}{c} \mathcal{R}_{1} \\ \mathcal{R}_{2}\\ \mathcal{R}_{3}\\ \mathcal{R}_{4} \end{array}\left(\begin{array}{ccccc} \langle \mathfrak{a}_{6},\; \mathfrak{a}_{2}\rangle & \langle \mathfrak{a}_{4},\; \mathfrak{a}_{2}\rangle & \langle \mathfrak{a}_{6},\; \mathfrak{a}_{1}\rangle & \langle \mathfrak{a}_{7},\; \mathfrak{a}_{1}\rangle & \langle \mathfrak{a}_{5},\; \mathfrak{a}_{2}\rangle \\ \langle \mathfrak{a}_{5},\; \mathfrak{a}_{1}\rangle & \langle \mathfrak{a}_{2},\; \mathfrak{a}_{6}\rangle & \langle \mathfrak{a}_{5},\; \mathfrak{a}_{2}\rangle & \langle \mathfrak{a}_{4},\; \mathfrak{a}_{3}\rangle & \langle \mathfrak{a}_{5},\; \mathfrak{a}_{1}\rangle\\ \langle \mathfrak{a}_{7},\; \mathfrak{a}_{1}\rangle & \langle \mathfrak{a}_{5},\; \mathfrak{a}_{1}\rangle & \langle \mathfrak{a}_{8},\; \mathfrak{a}_{0}\rangle & \langle \mathfrak{a}_{4},\; \mathfrak{a}_{4}\rangle & \langle \mathfrak{a}_{5},\; \mathfrak{a}_{2}\rangle\\ \langle \mathfrak{a}_{5},\; \mathfrak{a}_{2}\rangle & \langle \mathfrak{a}_{6},\; \mathfrak{a}_{2}\rangle & \langle \mathfrak{a}_{5},\; \mathfrak{a}_{1}\rangle & \langle \mathfrak{a}_{4},\; \mathfrak{a}_{3}\rangle & \langle \mathfrak{a}_{5},\; \mathfrak{a}_{3}\rangle \end{array}\right), & \end{aligned} |
\begin{aligned} & \begin{array}{lllll} \quad\quad\quad\quad\quad\quad\quad \mathcal{S}_{1} &\quad\quad\mathcal{S}_{2} & \quad\quad\mathcal{S}_{3} & \quad\quad\mathcal{S}_{4} &\quad\quad\quad\mathcal{S}_{5} \end{array} \\ & \mathbb{D}^{'3} = \begin{array}{c} \mathcal{R}_{1} \\ \mathcal{R}_{2}\\ \mathcal{R}_{3}\\ \mathcal{R}_{4} \end{array}\left(\begin{array}{ccccc} \langle \mathfrak{a}_{4},\; \mathfrak{a}_{4}\rangle & \langle \mathfrak{a}_{7},\; \mathfrak{a}_{1}\rangle & \langle \mathfrak{a}_{6},\; \mathfrak{a}_{1}\rangle & \langle \mathfrak{a}_{5},\; \mathfrak{a}_{3}\rangle & \langle \mathfrak{a}_{5},\; \mathfrak{a}_{2}\rangle \\ \langle \mathfrak{a}_{5},\; \mathfrak{a}_{1}\rangle & \langle \mathfrak{a}_{4},\; \mathfrak{a}_{2}\rangle & \langle \mathfrak{a}_{7},\; \mathfrak{a}_{1}\rangle & \langle \mathfrak{a}_{5},\; \mathfrak{a}_{2}\rangle & \langle \mathfrak{a}_{4},\; \mathfrak{a}_{3}\rangle\\ \langle \mathfrak{a}_{7},\; \mathfrak{a}_{1}\rangle & \langle \mathfrak{a}_{5},\; \mathfrak{a}_{3}\rangle & \langle \mathfrak{a}_{6},\; \mathfrak{a}_{1}\rangle & \langle \mathfrak{a}_{7},\; \mathfrak{a}_{1}\rangle & \langle \mathfrak{a}_{4},\; \mathfrak{a}_{2}\rangle\\ \langle \mathfrak{a}_{6},\; \mathfrak{a}_{1}\rangle & \langle \mathfrak{a}_{6},\; \mathfrak{a}_{1}\rangle & \langle \mathfrak{a}_{5},\; \mathfrak{a}_{3}\rangle & \langle \mathfrak{a}_{4},\; \mathfrak{a}_{2}\rangle & \langle \mathfrak{a}_{7},\; \mathfrak{a}_{1}\rangle \end{array}\right). & \end{aligned} |
The accumulated L q -ROFNs of the bad alternative \mathcal{R}_{3}^{'} are chosen arbitrarily, and are respectively inserted in the given matrices \mathbb{D}^{'1}, \mathbb{D}^{'2}, and \mathbb{D}^{'3} . Thus, the computed decision matrices \mathbb{D}^{'*1}, \mathbb{D}^{'*2}, and \mathbb{D}^{'*3} are given as:
\begin{aligned} & \begin{array}{lllll} \quad\quad\quad\quad\quad\quad\quad \mathcal{S}_{1} &\quad\quad\mathcal{S}_{2} & \quad\quad\mathcal{S}_{3} & \quad\quad\mathcal{S}_{4} &\quad\quad\quad\mathcal{S}_{5} \end{array} \\ & \mathbb{D}^{'*1} = \begin{array}{c} \mathcal{R}_{1} \\ \mathcal{R}_{2}\\ \mathcal{R}_{3'}\\ \mathcal{R}_{4} \end{array}\left(\begin{array}{ccccc} \langle \mathfrak{a}_{6},\; \mathfrak{a}_{2}\rangle & \langle \mathfrak{a}_{5},\; \mathfrak{a}_{3}\rangle & \langle \mathfrak{a}_{4},\; \mathfrak{a}_{1}\rangle & \langle \mathfrak{a}_{3},\; \mathfrak{a}_{4}\rangle & \langle \mathfrak{a}_{5},\; \mathfrak{a}_{2}\rangle \\ \langle \mathfrak{a}_{5},\; \mathfrak{a}_{1}\rangle & \langle \mathfrak{a}_{4},\; \mathfrak{a}_{3}\rangle & \langle \mathfrak{a}_{6},\; \mathfrak{a}_{1}\rangle & \langle \mathfrak{a}_{5},\; \mathfrak{a}_{2}\rangle & \langle \mathfrak{a}_{4},\; \mathfrak{a}_{1}\rangle\\ \langle \mathfrak{a}_{5},\; \mathfrak{a}_{2}\rangle & \langle \mathfrak{a}_{5},\; \mathfrak{a}_{1}\rangle & \langle \mathfrak{a}_{8},\; \mathfrak{a}_{0}\rangle & \langle \mathfrak{a}_{4},\; \mathfrak{a}_{4}\rangle & \langle \mathfrak{a}_{7},\; \mathfrak{a}_{1}\rangle\\ \langle \mathfrak{a}_{7},\; \mathfrak{a}_{1}\rangle & \langle \mathfrak{a}_{5},\; \mathfrak{a}_{2}\rangle & \langle \mathfrak{a}_{4},\; \mathfrak{a}_{1}\rangle & \langle \mathfrak{a}_{6},\; \mathfrak{a}_{2}\rangle & \langle \mathfrak{a}_{6},\; \mathfrak{a}_{1}\rangle \end{array}\right), & \end{aligned} |
\begin{aligned} & \begin{array}{lllll} \quad\quad\quad\quad\quad\quad\quad \mathcal{S}_{1} &\quad\quad\mathcal{S}_{2} & \quad\quad\mathcal{S}_{3} & \quad\quad\mathcal{S}_{4} &\quad\quad\quad\mathcal{S}_{5} \end{array} \\ & \mathbb{D}^{'*2} = \begin{array}{c} \mathcal{R}_{1} \\ \mathcal{R}_{2}\\ \mathcal{R}_{3'}\\ \mathcal{R}_{4} \end{array}\left(\begin{array}{ccccc} \langle \mathfrak{a}_{6},\; \mathfrak{a}_{2}\rangle & \langle \mathfrak{a}_{4},\; \mathfrak{a}_{2}\rangle & \langle \mathfrak{a}_{6},\; \mathfrak{a}_{1}\rangle & \langle \mathfrak{a}_{7},\; \mathfrak{a}_{1}\rangle & \langle \mathfrak{a}_{5},\; \mathfrak{a}_{2}\rangle \\ \langle \mathfrak{a}_{5},\; \mathfrak{a}_{1}\rangle & \langle \mathfrak{a}_{2},\; \mathfrak{a}_{6}\rangle & \langle \mathfrak{a}_{5},\; \mathfrak{a}_{2}\rangle & \langle \mathfrak{a}_{4},\; \mathfrak{a}_{3}\rangle & \langle \mathfrak{a}_{5},\; \mathfrak{a}_{1}\rangle\\ \langle \mathfrak{a}_{4},\; \mathfrak{a}_{3}\rangle & \langle \mathfrak{a}_{5},\; \mathfrak{a}_{3}\rangle & \langle \mathfrak{a}_{6},\; \mathfrak{a}_{1}\rangle & \langle \mathfrak{a}_{8},\; \mathfrak{a}_{0}\rangle & \langle \mathfrak{a}_{5},\; \mathfrak{a}_{2}\rangle\\ \langle \mathfrak{a}_{5},\; \mathfrak{a}_{2}\rangle & \langle \mathfrak{a}_{6},\; \mathfrak{a}_{2}\rangle & \langle \mathfrak{a}_{5},\; \mathfrak{a}_{1}\rangle & \langle \mathfrak{a}_{4},\; \mathfrak{a}_{3}\rangle & \langle \mathfrak{a}_{5},\; \mathfrak{a}_{3}\rangle \end{array}\right), & \end{aligned} |
\begin{aligned} & \begin{array}{lllll} \quad\quad\quad\quad\quad\quad\quad \mathcal{S}_{1} &\quad\quad\mathcal{S}_{2} & \quad\quad\mathcal{S}_{3} & \quad\quad\mathcal{S}_{4} &\quad\quad\quad\mathcal{S}_{5} \end{array} \\ & \mathbb{D}^{'*3} = \begin{array}{c} \mathcal{R}_{1} \\ \mathcal{R}_{2}\\ \mathcal{R}_{3'}\\ \mathcal{R}_{4} \end{array}\left(\begin{array}{ccccc} \langle \mathfrak{a}_{4},\; \mathfrak{a}_{4}\rangle & \langle \mathfrak{a}_{7},\; \mathfrak{a}_{1}\rangle & \langle \mathfrak{a}_{6},\; \mathfrak{a}_{1}\rangle & \langle \mathfrak{a}_{5},\; \mathfrak{a}_{3}\rangle & \langle \mathfrak{a}_{5},\; \mathfrak{a}_{2}\rangle \\ \langle \mathfrak{a}_{5},\; \mathfrak{a}_{1}\rangle & \langle \mathfrak{a}_{4},\; \mathfrak{a}_{2}\rangle & \langle \mathfrak{a}_{7},\; \mathfrak{a}_{1}\rangle & \langle \mathfrak{a}_{5},\; \mathfrak{a}_{2}\rangle & \langle \mathfrak{a}_{4},\; \mathfrak{a}_{3}\rangle\\ \langle \mathfrak{a}_{5},\; \mathfrak{a}_{3}\rangle & \langle \mathfrak{a}_{5},\; \mathfrak{a}_{2}\rangle & \langle \mathfrak{a}_{4},\; \mathfrak{a}_{3}\rangle & \langle \mathfrak{a}_{6},\; \mathfrak{a}_{1}\rangle & \langle \mathfrak{a}_{6},\; \mathfrak{a}_{2}\rangle\\ \langle \mathfrak{a}_{6},\; \mathfrak{a}_{1}\rangle & \langle \mathfrak{a}_{6},\; \mathfrak{a}_{1}\rangle & \langle \mathfrak{a}_{5},\; \mathfrak{a}_{3}\rangle & \langle \mathfrak{a}_{4},\; \mathfrak{a}_{2}\rangle & \langle \mathfrak{a}_{7},\; \mathfrak{a}_{1}\rangle \end{array}\right). & \end{aligned} |
Consequently, by applying the suggested second GDM method on the above developed decision matrices \mathbb{D}^{'*1}, \mathbb{D}^{'*2}, and \mathbb{D}^{'*3} , the ranking values \mathcal{Y}(\varpi_{1}) , \mathcal{Y}(\varpi_{2}) , \mathcal{Y}(\varpi_{3}) , and \mathcal{Y}(\varpi_{4}) are calculated as: \mathcal{Y}(\varpi_{1}) = 5.41 , \mathcal{Y}(\varpi_{2}) = 4.94 , \mathcal{Y}(\varpi_{3}) = 8.00 , and \mathcal{Y}(\varpi_{4}) = 5.48 . As \mathcal{Y}(\varpi_{3}) > \mathcal{Y}(\varpi_{4}) > \mathcal{Y}(\varpi_{1}) > \mathcal{Y}(\varpi_{2}), so, the most suitable alternative from the alternatives \mathcal{R}_{k} with k = 1, 2, \ldots, 4 is \mathcal{R}_{3}. It can be observed that the optimal object is \mathcal{R}_{3} in both the original and transformed decision problems. Hence, it is verified that the suggested second GDM method holds the requirements of Test Pattern 1.
Test Criteria 2 ^{\rm nd} and 3 ^{\rm rd} : To investigate the reliability of the second GDM method, we divide the genuine GDM dilemma of Example 4.5 into three dependent GDM questions that include the objects as: \{\mathcal{R}_{3}, \mathcal{R}_{2}, \mathcal{R}_{4} \} , \{\mathcal{R}_{3}, \mathcal{R}_{1}, \mathcal{R}_{4}\} , and \{\mathcal{R}_{2}, \mathcal{R}_{1}, \mathcal{R}_{4}\} . Next, by employing the suggested 2 ^{\rm nd} GDM method on these sub-problems, we obtain the ranks as follows: '\mathcal{R}_{3} > \mathcal{R}_{4} > \mathcal{R}_{2} ', '\mathcal{R}_{3} > \mathcal{R}_{1} > \mathcal{R}_{4} ' and '\mathcal{R}_{1} > \mathcal{R}_{4} > \mathcal{R}_{2} '. Further, we combine these determined ranks and find the final rankings as '\mathcal{R}_{3} > \mathcal{R}_{1} > \mathcal{R}_{4} > \mathcal{R}_{2} ', which is the same as computed in the Example 4.5, and thus, it showed the transitive property. Thus, the suggested second GDM method verifies the Test Criteria 2 ^{\rm nd} and 3 ^{\rm rd} .
In this section, we compare our suggested GDM methods with some approaches, including Kumar and Chen's GDM technique [24]. In addition, we investigate the effect of q in the L q -ROFNs for Example 4.3 as presented in the previous section.
One may verify from Table 1 that the optimal decision object is invariant in the case of the first GDM method and preexisting approaches [10,20,24,28,40,57], that is, \mathcal{R}_4 . Moreover, there is a minor change in the rankings of sub-optimal objects. In [60], a GDM method is presented based on q -ROF numbers using Aczel-Alsina TN and TCN operations. The current study extended the method introduced in [60] using linguistic terms, and proposed L q -ROFAAWA operators. In certain situations, L q -ROFNs perform better than q -ROFNs for the following reason: L q -ROFNs simplify the process for decision-makers by representing uncertainty with linguistic terms (such as high, medium, and low), making it easier to express preferences. In contrast, q -ROFNs rely on numerical information, which can be more challenging for non-experts to interpret. Additionally, note that the parameter q in the L q -ROFNs plays an important role in group decision making problems. In the preceding section, a GDM problem is solved in Example 4.3 by considering q = 3 . Now, to examine the effect of q -rung parameter on this problem again, we solve for every q\in\{1, 2, 4, 5, \ldots, 10\} , where Aczel-Alsina parameter is \lambda = 2 in the algorithmic process. The calculated ranking value for each alternative is given in Table 2 for every q\in\{1, 2, 3, \ldots, 10\} . From Table 2, it is clear that the ranking values are changed for all q\in\{1, 2, 3, \ldots, 10\} , but the overall ranking orders are invariant, which yields a very interesting fact that the applicability scope of suggested approach for GDM using Lq-ROFAAWA aggregation operator is higher than existing models, e.g., [24].
1 ^{\rm st} -GDM method | Ranking orders |
Liu and Wang's GDM technique [57] | \mathcal{R}_{4} > \mathcal{R}_{3} > \mathcal{R}_{2} > \mathcal{R}_{1} |
Chen et al.'s GDM method [10] | \mathcal{R}_{4} > \mathcal{R}_{3} > \mathcal{R}_{1} > \mathcal{R}_{2} |
Kumar and Chen's GDM technique [24] | \mathcal{R}_{4} > \mathcal{R}_{3} > \mathcal{R}_{1} > \mathcal{R}_{2} |
Garg and Kumar's GDM method [20] | \mathcal{R}_{4} > \mathcal{R}_{1} > \mathcal{R}_{3} > \mathcal{R}_{2} |
Nayana et al. GDM technique [40] | \mathcal{R}_{4} > \mathcal{R}_{1} > \mathcal{R}_{3} > \mathcal{R}_{2} |
Kumar and Chen's GDM method [28] | \mathcal{R}_{4} > \mathcal{R}_{3} > \mathcal{R}_{1} > \mathcal{R}_{2} |
The suggested first GDM approach | \mathcal{R}_{4} > \mathcal{R}_{3} > \mathcal{R}_{1} > \mathcal{R}_{2} |
Parameter ( q ) | \mathcal{Y}(\varpi_{1}) | \mathcal{Y}(\varpi_{2}) | \mathcal{Y}(\varpi_{3}) | \mathcal{Y}(\varpi_{4}) | Ranking orders (or \mathcal{RO}s ) |
q=1 | 4.08 | 3.60 | 4.21 | 5.50 | \mathcal{R}_{4} > \mathcal{R}_{3} > \mathcal{R}_{1} > \mathcal{R}_{2} |
q=2 | 4.39 | 4.01 | 4.54 | 4.76 | \mathcal{R}_{4} > \mathcal{R}_{3} > \mathcal{R}_{1} > \mathcal{R}_{2} |
q=3 | 4.44 | 4.03 | 4.57 | 5.23 | \mathcal{R}_{4} > \mathcal{R}_{3} > \mathcal{R}_{1} > \mathcal{R}_{2} |
q=4 | 4.45 | 4.04 | 4.56 | 5.12 | \mathcal{R}_{4} > \mathcal{R}_{3} > \mathcal{R}_{1} > \mathcal{R}_{2} |
q=5 | 4.51 | 4.11 | 4.60 | 5.10 | \mathcal{R}_{4} > \mathcal{R}_{3} > \mathcal{R}_{1} > \mathcal{R}_{2} |
q=6 | 4.75 | 4.30 | 4.82 | 5.31 | \mathcal{R}_{4} > \mathcal{R}_{3} > \mathcal{R}_{1} > \mathcal{R}_{2} |
q=7 | 4.71 | 4.27 | 4.77 | 5.23 | \mathcal{R}_{4} > \mathcal{R}_{3} > \mathcal{R}_{1} > \mathcal{R}_{2} |
q=8 | 4.65 | 4.22 | 4.71 | 5.14 | \mathcal{R}_{4} > \mathcal{R}_{3} > \mathcal{R}_{1} > \mathcal{R}_{2} |
q=9 | 4.73 | 4.29 | 4.78 | 5.22 | \mathcal{R}_{4} > \mathcal{R}_{3} > \mathcal{R}_{1} > \mathcal{R}_{2} |
q=10 | 4.69 | 4.27 | 4.75 | 5.18 | \mathcal{R}_{4} > \mathcal{R}_{3} > \mathcal{R}_{1} > \mathcal{R}_{2} |
From Table 2, it can be observed that by varying the range of parameter q from 1 to 10, the ranking order \mathcal{R}_{4} > \mathcal{R}_{3} > \mathcal{R}_{1} > \mathcal{R}_{2} remains the same (see also Figure 2). This does not imply that the approach is generally insensitive. Sensitivity refers to the extent to which the output changes in response to variations in the input. The ranking order is determined by the input information, while q serves as a parameter. Additionally, it may indicate certain aspects of the proposed technique's sensitivity, as outlined below:
Robustness: If the approach is robust, it can yield consistent results even when the parameters are changed. This may be a good thing as it suggests reliability.
System stability: Stability may be inherent in the system or data-set being evaluated. If the characteristics or criteria that influence the ranking remain dominant despite changes in q , the key components of the ranking may remain robust and consistent across a range of q values. This would suggest a stable and potentially well-functioning system.
Like every preexisting GDM model, our proposed methodologies have their pros and cons. The advantages are discussed in the previous sections, however, in this section, we will discuss certain limitations of the developed methods that we identified during this study. The first limitation of the presented work is the complexity in computations when dealing with large datasets. This limitation, however, may be resolved with the utilization of mathematical software like MATLAB, MAPLE, etc. The second limitation of the proposed study is its inefficiency in handling the neutral part of the data.
In this article, we developed two novel operations using L q -ROFNs based on the Aczel-Alsina's t -conorm (TCN) and t -norm (TN), i.e., addition and scalar multiplication. These operations overcome the flaws of Kumar and Chen's addition and scalar product operations [24] of LIFNs. Moreover, we have introduced the linguistic q -rung orthopair fuzzy Aczel-Alsina weighted arithmetic (L q -ROFAAWA) aggregation operator of L q -ROFNs using presented addition and scalar multiplication operations. Further, we have suggested two types of new group decision-making (GDM) techniques based on the suggested L q -ROFAAWA aggregation operator. The proposed first GDM method assumes the condition that the weights of experts and weights of attributes are known completely. However, the suggested second GDM method considers the condition that the weights of experts and the weights of attributes are unknown. Moreover, we applied the suggested methodologies to different environmental and economic real-world issues and successfully solved them, i.e., ranking of countries regarding air pollution, selection of best company for bank to invest, and selection of best electric vehicle design. In the end, we have validated our proposed GDM methods with three tests and compared them with preexisting GDM methods.
Consequently, the suggested GDM techniques overcome the deficiencies in different recent works, including Chen et al.'s GDM method [10], Kumar and Chen's GDM technique [24], Garg and Kumar's GDM technique [20], Liu and Wang's GDM technique [57], Tang and Meng's GDM technique [27], and Kumar and Chen's GDM method [28] because they cannot differentiate the ranking positions of objects. In future research, this study can be extended to different domains, such as Aczel-Alsina operators based on linguistic fuzzy measurements and rough attributes as studied in [4]; approximation of linguistic q -rung orthopair fuzzy information with covering-based rough sets using the concepts presented in [5]; and Aczel-Alsina operators based on linguistic quasi-rung fuzzy sets as proposed in [61,62].
Ghous Ali: conceptualization, data curation, formal analysis, supervision, validation, methodology, visualization, writing-review and editing; Kholood Alsager: funding acquisition, investigation, writing-review and editing; Asad Ali: Methodology, writing-original draft. All authors have read and approved the final version of the manuscript for publication.
The researchers would like to thank the Deanship of Graduate Studies and Scientific Research at Qassim University for financial support (QU-APC-2024-9/1).
The authors declare no conflict of interest.
Proof. 1) Based on Definition 3.1, we get
\begin{align*} \sigma_{1}\bigoplus\sigma_{2}\; = \; &\Bigg{\langle} \mathfrak{a}_{\mathfrak{h}\bigg( \sqrt[q]{(1-\exp^{-((-\log(1-(\frac{\xi_{\sigma_{1}}}{\mathfrak{h}})^{q}))^{\lambda}+(-\log(1-(\frac{\xi_{\sigma_{2}}}{\mathfrak{h}})^{q}))^{\lambda})^{1/\lambda})})}\bigg)}, \\& \mathfrak{a}_{\mathfrak{h}\bigg( \exp^{-({(-\log(\frac{\eta_{\sigma_{1}}}{\mathfrak{h}}))^{\lambda}+(-\log(\frac{\eta_{\sigma_{2}}}{\mathfrak{h}}))^{\lambda}})^{1/\lambda}}\bigg)}\Bigg\rangle ,\\ \; = \; &\Bigg{\langle} \mathfrak{a}_{\mathfrak{h}\bigg( \sqrt[q]{(1-\exp^{-((-\log(1-(\frac{\xi_{\sigma_{2}}}{\mathfrak{h}})^{q}))^{\lambda}+(-\log(1-(\frac{\xi_{\sigma_{1}}}{\mathfrak{h}})^{q}))^{\lambda})^{1/\lambda})})}\bigg)}, \\& \mathfrak{a}_{\mathfrak{h}\bigg( \exp^{-({(-\log(\frac{\eta_{\sigma_{2}}}{\mathfrak{h}}))^{\lambda}+(-\log(\frac{\eta_{\sigma_{1}}}{\mathfrak{h}}))^{\lambda}})^{1/\lambda}}\bigg)}\Bigg\rangle, \\ \; = \; &\sigma_{2}\bigoplus\sigma_{1}, \end{align*} |
where \lambda > 0 .
2) Again using Definition 3.1, we have
\begin{align*} \psi(\sigma_{1}\bigoplus\sigma_{2}) = \; &\Bigg{\langle} \mathfrak{a}_{\mathfrak{h}\bigg( \sqrt[q]{(1-\exp^{-\Big(\psi\big((-\log(1-(\frac{\xi_{\sigma_{1}}}{\mathfrak{h}})^{q}))^{\lambda}+(-\log(1-(\frac{\xi_{\sigma_{2}}}{\mathfrak{h}})^{q}))^{\lambda}\big)\Big)^{1/\lambda}})}\bigg)}, \\& \mathfrak{a}_{\mathfrak{h}\bigg( \exp^{-\Big(\psi\big((-\log(\frac{\eta_{\sigma_{1}}}{\mathfrak{h}}))^{\lambda}+(-\log(\frac{\eta_{\sigma_{2}}}{\mathfrak{h}}))^{\lambda}\big)\Big)^{1/\lambda}}\bigg)}\Bigg\rangle, \end{align*} |
\begin{align*} = \; &\Bigg{\langle} \mathfrak{a}_{\mathfrak{h}\bigg( \sqrt[q]{(1-\exp^{-\Big(\psi\big(-\log(1-(\frac{\xi_{\sigma_{1}}}{\mathfrak{h}})^{q})\big)^{\lambda}+\psi\big(-\log(1-(\frac{\xi_{\sigma_{2}}}{\mathfrak{h}})^{q})\big)^{\lambda}\Big)^{1/\lambda}})}\bigg)}, \\& \mathfrak{a}_{\mathfrak{h}\bigg( \exp^{-\Big(\psi\big(-\log(\frac{\eta_{\sigma_{1}}}{\mathfrak{h}})\big)^{\lambda}+\psi\big(-\log(\frac{\eta_{\sigma_{2}}}{\mathfrak{h}})\big)^{\lambda}\Big)^{1/\lambda}}\bigg)}\Bigg\rangle,\\ = \; &\psi\sigma_{1}\bigoplus\psi\sigma_{2}, \end{align*} |
where \psi > 0 and \lambda > 0 .
3) Similarly, by Definition 3.1. We obtain:
\begin{align*} (\psi_{1}\bigoplus\psi_{2})\sigma\; = \; &\bigg\langle \mathfrak{a}_{\mathfrak{h}\sqrt[q]{1- \exp^{- \Big((\psi_{1}+\psi_{2})\big(-\log(1-(\frac{\xi_{\sigma}}{\mathfrak{h}})^{q})\big)^{\lambda}\Big)^{1/\lambda}}}},\\& \mathfrak{a}_{\exp^{-\Big((\psi_{1}+\psi_{2})\big(-\log(\frac{\eta_{\sigma}}{\mathfrak{h}})\big)^{\lambda}\Big)^{1/\lambda}}}\bigg\rangle \\ = \; &\bigg\langle \mathfrak{a}_{\mathfrak{h}\sqrt[q]{1- \exp^{- \Big(\psi_{1}\big(-\log(1-(\frac{\xi_{\sigma}}{\mathfrak{h}})^{q})\big)^{\lambda}+\psi_{2}\big(-\log(1-(\frac{\xi_{\sigma}}{\mathfrak{h}})^{q})\big)^{\lambda}\Big)^{1/\lambda}}}},\\&\mathfrak{a}_{\exp^{-\Big((\psi_{1}\big(-\log(\frac{\eta_{\sigma}}{\mathfrak{h}})\big)^{\lambda}+\psi_{2}\big(-\log(\frac{\eta_{\sigma}}{\mathfrak{h}})\big)^{\lambda}\Big)^{1/\lambda}}}\bigg\rangle \\ = \; &\psi_{1}\sigma\bigoplus\psi_{2}\sigma, \end{align*} |
where \psi_{1} > 0, \psi_{2} > 0 and \lambda > 0 .
Proof. Suppose \sigma_{1} = \langle \mathfrak{a}_{\xi_{1}}, \mathfrak{a}_{\eta_{1}}\rangle , \sigma_{2} = \langle \mathfrak{a}_{\xi_{2}}, \; \mathfrak{a}_{\eta_{2}}\rangle \ldots , and \sigma_{t} = \langle \mathfrak{a}_{\xi_{t}}, \; \mathfrak{a}_{\eta_{t}}\rangle are L q -ROFNs where \sigma_{1}, \sigma_{2}, \ldots, \sigma_{t}\in\Gamma_{[0, \mathfrak{h}]} and \mathfrak{h} is an even positive integer. We show this result using the mathematical induction principle as below:
1) When t = 2 , we get,
\begin{align*} \mathfrak{w}_{1}\sigma_{1}\; = \; &\left\langle \mathfrak{a}_{\mathfrak{h}\sqrt[q]{1- \exp^{- (\mathfrak{w}_{1}(-\log(1-(\frac{\xi_{\sigma_{1}}}{\mathfrak{h}})^{q}))^{\lambda})^{1/\lambda}}}},\; \mathfrak{a}_{\exp^{\mathfrak{w}_{1}(-\log(\frac{\eta_{\sigma_{1}}}{\mathfrak{h}}))^{\lambda})^{1/\lambda}}}\right \rangle.\\ \mathfrak{w}_{2}\sigma_{2}\; = \; &\left\langle \mathfrak{a}_{\mathfrak{h}\sqrt[q]{1- \exp^{- (\mathfrak{w}_{2}(-\log(1-(\frac{\xi_{\sigma_{2}}}{\mathfrak{h}})^{q}))^{\lambda})^{1/\lambda}}}},\; \mathfrak{a}_{\exp^{\mathfrak{w}_{2}(-\log(\frac{\eta_{\sigma_{2}}}{\mathfrak{h}}))^{\lambda})^{1/\lambda}}}\right \rangle.\\ Lq-ROFAAWA(\sigma_{1}, \sigma_{2})\; = \; &\left\langle \mathfrak{a}_{\mathfrak{h}\sqrt[q]{1- \exp^{- (\mathfrak{w}_{1}(-\log(1-(\frac{\xi_{\sigma_{1}}}{\mathfrak{h}})^{q}))^{\lambda})^{1/\lambda}}}},\; \mathfrak{a}_{\exp^{\mathfrak{w}_{1}(-\log(\frac{\eta_{\sigma_{1}}}{\mathfrak{h}}))^{\lambda})^{1/\lambda}}}\right \rangle \\&\bigoplus\left\langle \mathfrak{a}_{\mathfrak{h}\sqrt[q]{1- \exp^{- (\mathfrak{w}_{2}(-\log(1-(\frac{\xi_{\sigma_{2}}}{\mathfrak{h}})^{q}))^{\lambda})^{1/\lambda}}}},\; \mathfrak{a}_{\exp^{\mathfrak{w}_{2}(-\log(\frac{\eta_{\sigma_{2}}}{\mathfrak{h}}))^{\lambda})^{1/\lambda}}}\right \rangle,\\ = \; &\left\langle \mathfrak{a}_{h\sqrt[q]{1- \exp^{- (\sum\nolimits_{r = 1}^{2}\mathfrak{w}_{r}(-\log(1-(\frac{\xi_{\sigma_{r}}}{\mathfrak{h}})^{q}))^{\lambda})^{1/\lambda}}}},\; \mathfrak{a}_{\exp^{-(\sum\nolimits_{r = 1}^{2}\mathfrak{w}_{r}(-\log(\frac{\eta_{\sigma_{r}}}{\mathfrak{h}}))^{\lambda})^{1/\lambda}}}\right \rangle. \end{align*} |
Thus, the aggregated value L q -ROFAAWA( \sigma_{1} , \sigma_{2} ) of L q -ROFNs \sigma_{1} = \langle \mathfrak{a}_{\xi_{1}}, \mathfrak{a}_{\eta_{1}}\rangle and \sigma_{2} = \langle \mathfrak{a}_{\xi_{2}}, \; \mathfrak{a}_{\eta_{2}}\rangle using the suggested L q -ROFAAWA AgOp defined in Eq (3.5) is a L q -ROFN.
2) Suppose the aggregated value L q -ROFAAWA (\sigma_{1}, \sigma_{2}, \ldots, \sigma_{k}) of the L q -ROFNs \sigma_{1} = \langle \mathfrak{a}_{\xi_{1}}, \mathfrak{a}_{\eta_{1}}\rangle , \sigma_{2} = \langle \mathfrak{a}_{\xi_{2}}, \; \mathfrak{a}_{\eta_{2}}\rangle\ldots , and \sigma_{k} = \langle \mathfrak{a}_{\xi_{k}}, \; \mathfrak{a}_{\eta_{k}}\rangle by applying the suggested L q -ROFAAWA AgOp given in Equation (3.5) is a L q -ROFN for t = k , then
\begin{align*} Lq-ROFAAWA(\sigma_{1}, \sigma_{2},\ldots, \sigma_{k})\; = \; &\mathfrak{w}_{1}\sigma_{1}\bigoplus \mathfrak{w}_{2}\sigma_{2}\bigoplus\ldots\bigoplus \mathfrak{w}_{k}\sigma_{k} \\ = \; &\bigg\langle \mathfrak{a}_{\mathfrak{h}\sqrt[q]{1- \exp^{- (\sum\nolimits_{r = 1}^{k}\mathfrak{w}_{r}(-\log(1-(\frac{\xi_{\sigma_{\mathfrak{r}}}}{\mathfrak{h}})^{q}))^{\lambda})^{1/\lambda}}}},\\&\mathfrak{a}_{\exp^{-(\sum\nolimits_{\mathfrak{r} = 1}^{k}\mathfrak{w}_{\mathfrak{r}}(-\log(\frac{\eta_{\sigma_{\mathfrak{r}}}}{\mathfrak{h}}))^{\lambda})^{1/\lambda}}}\bigg\rangle. \end{align*} |
Thus, the aggregated value of L q -ROFAAWA (\sigma_{1}, \sigma_{2}, \ldots, \sigma_{k}) of the \sigma_{1} = \langle \mathfrak{a}_{\xi_{1}}, \mathfrak{a}_{\eta_{1}}\rangle , \sigma_{2} = \langle \mathfrak{a}_{\xi_{2}}, \; \mathfrak{a}_{\eta_{2}}\rangle\ldots , and \sigma_{k} = \langle \mathfrak{a}_{\xi_{k}}, \; \mathfrak{a}_{\eta_{k}}\rangle is a L q -ROFN.
3) For t = k+1, we get
\begin{align*} Lq-ROFAAWA(\sigma_{1}, \sigma_{2},\ldots, \sigma_{k+1})\; = \; &\mathfrak{w}_{1}\sigma_{1}\bigoplus \mathfrak{w}_{2}\sigma_{2}\bigoplus\ldots\bigoplus \mathfrak{w}_{k+1}\sigma_{k+1} \\ = \; &\bigg\langle \mathfrak{a}_{\mathfrak{h}\sqrt[q]{1- \exp^{- (\sum\nolimits_{r = 1}^{k}\mathfrak{w}_{r}(-\log(1-(\frac{\xi_{\sigma_{r}}}{\mathfrak{h}})^{q}))^{\lambda})^{1/\lambda}}}},\\&\mathfrak{a}_{\exp^{-(\sum\nolimits_{r = 1}^{k}\mathfrak{w}_{r}(-\log(\frac{\eta_{\sigma_{r}}}{\mathfrak{h}}))^{\lambda})^{1/\lambda}}}\bigg\rangle\\&\bigoplus\bigg\langle \mathfrak{a}_{\mathfrak{h}\sqrt[q]{1- \exp^{- (\mathfrak{w}_{1}(-\log(1-(\frac{\xi_{\sigma_{1}}}{\mathfrak{h}})^{q}))^{\lambda})^{1/\lambda}}}},\\&\; \mathfrak{a}_{\exp^{-(\mathfrak{w}_{1}(-\log(\frac{\eta_{\sigma_{1}}}{\mathfrak{h}}))^{\lambda})^{1/\lambda}}}\bigg\rangle\\ = \; &\bigg\langle \mathfrak{a}_{\mathfrak{h}\sqrt[q]{1- \exp^{- (\sum\nolimits_{r = 1}^{k+1}\mathfrak{w}_{r}(-\log(1-(\frac{\xi_{\sigma_{\mathfrak{r}}}}{\mathfrak{h}})^{q}))^{\lambda})^{1/\lambda}}}},\\&\mathfrak{a}_{\exp^{-(\sum\nolimits_{\mathfrak{r} = 1}^{k+1}\mathfrak{w}_{\mathfrak{r}}(-\log(\frac{\eta_{\sigma_{\mathfrak{r}}}}{\mathfrak{h}}))^{\lambda})^{1/\lambda}}}\bigg\rangle. \end{align*} |
Hence, the computed value of the L q -ROFNs \sigma_{1} = \langle \mathfrak{a}_{\xi_{1}}, \mathfrak{a}_{\eta_{1}}\rangle, \sigma_{2} = \langle \mathfrak{a}_{\xi_{2}}, \; \mathfrak{a}_{\eta_{2}}\rangle \ldots , \sigma_{k +1} = \langle \mathfrak{a}_{\xi_{k+1}}, \; \mathfrak{a}_{\eta_{k+1}}\rangle using the suggested L q -ROFAAWA AgOp defined in Eq (3.5) is a L q -ROFN.
Proof. As the weights of L q -ROFNs \sigma_{1}, \sigma_{2}, \ldots, \sigma_{n} are \mathfrak{w}_{1}, \mathfrak{w}_{2}, \ldots, \mathfrak{w}_{n}, respectively, where \mathfrak{w}_{r}\in[0, \mathfrak{h}], r = 1, 2, \ldots, n with \sum_{r = 1}^{n}\mathfrak{w}_{r} = 1. If \sigma_{1} = \sigma_{2} = \ldots = \sigma_{n} = \sigma, then, we have
\begin{align*} Lq-ROFAAWA(\sigma_{1},\sigma_{2},\ldots,\sigma_{n}) = \; &\mathfrak{w}_{1}\sigma_{1}\bigoplus\mathfrak{w}_{2}\sigma_{2}\bigoplus\ldots\bigoplus\mathfrak{w}_{n}\sigma_{n},\\ = \; & \mathfrak{w}_{1}\sigma\bigoplus\mathfrak{w}_{2}\sigma\bigoplus\ldots\bigoplus\mathfrak{w}_{n}\sigma,\\ = \; &\sigma(\mathfrak{w}_{1}+\mathfrak{w}_{2}+\ldots+\mathfrak{w}_{n}),\\ = \; &\sigma. \end{align*} |
Proof. Since \sigma_{-} = \min\{\sigma_{1}, \sigma_{2}, \ldots, \sigma_{n}\} and \sigma_{+} = \max\{\sigma_{1}, \sigma_{2}, \ldots, \sigma_{n}\}, by implementing the suggested L q -ROFAAWA AgOp, we obtain:
\begin{align} Lq-ROFAAWA(\sigma_{1},\sigma_{2},\ldots,\sigma_{n}) = \; &\bigoplus_{r = 1}^{n}\mathfrak{w}_{r}\sigma_{r}\leq\bigoplus_{r = 1}^{n}\mathfrak{w}_{r}\sigma_{+} = \sigma_{+}\sum\limits_{r = 1}^{n}\mathfrak{w}_{r}, \end{align} | (D.1) |
\begin{align} Lq-ROFAAWA(\sigma_{1},\sigma_{2},\ldots,\sigma_{n}) = \; &\bigoplus_{r = 1}^{n}\mathfrak{w}_{r}\sigma_{r}\geq\bigoplus_{r = 1}^{n}\mathfrak{w}_{r}\sigma_{-} = \sigma_{-}\sum\limits_{r = 1}^{n}\mathfrak{w}_{r}. \end{align} | (D.2) |
By putting \sum_{r = 1}^{n}\mathfrak{w}_{r} = 1 in both Eqs (18.1) and (18.2), we get \sigma_{-}\leq L q -ROFAAWA(\sigma_{1}, \sigma_{2}, \ldots, \sigma_{n})\leq\sigma_{+}.
Proof. Using the suggested L q -ROFAAWA AgOp, we get
\begin{align*} Lq-ROFAAWA(\sigma_{1},\sigma_{2},\ldots,\sigma_{n}) = \; &\bigoplus_{r = 1}^{n}\mathfrak{w}_{r}\sigma_{r},\\ Lq-ROFAAWA(\sigma_{1}^{'},\sigma_{2}^{'},\ldots,\sigma_{n}^{'}) = \; &\bigoplus_{r = 1}^{n}\mathfrak{w}_{r}\sigma_{r}^{'}, \end{align*} |
since \sigma_{r}\leq\sigma_{r}^{'} with r = 1, 2, \ldots, n , and \sum_{r = 1}^{n}\mathfrak{w}_{r} = 1 , so, \bigoplus_{r = 1}^{n}\mathfrak{w}_{r}\sigma_{r}\leq\bigoplus_{r = 1}^{n}\mathfrak{w}_{r}\sigma_{r}^{'}. Therefore, we get L q -ROFAAWA(\sigma_{1}, \sigma_{2}, \ldots, \sigma_{n})\leq L q -ROFAAWA(\sigma_{1}^{'}, \sigma_{2}^{'}, \ldots, \sigma_{n}^{'}).
[1] |
L. A. Zadeh, Fuzzy sets, Information and Control, 8 (1965), 338–353. https://doi.org/10.1016/S0019-9958(65)90241-X doi: 10.1016/S0019-9958(65)90241-X
![]() |
[2] |
R. E. Bellman, L. A. Zadeh, Decision-making in a fuzzy environment, Manage. Sci., 17 (1970), 141–164. https://doi.org/10.1287/mnsc.17.4.B141 doi: 10.1287/mnsc.17.4.B141
![]() |
[3] |
Z. Pawlak, Rough sets, International Journal of Computer and Information Sciences, 11 (1982), 341–356. https://doi.org/10.1007/BF01001956 doi: 10.1007/BF01001956
![]() |
[4] |
J. Q. Wang, X. H. Zhang, Q. Shen, Choquet-like integrals with rough attribute fuzzy measures for data-driven decision-making, IEEE T. Fuzzy Syst., 32 (2024), 2825–2836. https://doi.org/10.1109/TFUZZ.2024.3363415 doi: 10.1109/TFUZZ.2024.3363415
![]() |
[5] |
J. Q. Wang, X. H. Zhang, Intuitionistic fuzzy granular matrix: novel calculation approaches for intuitionistic, fuzzy covering-based rough sets, Axioms, 13 (2024), 411. https://doi.org/10.3390/axioms13060411 doi: 10.3390/axioms13060411
![]() |
[6] |
K. T. Atanassov, Intuitionistic fuzzy sets, Fuzzy Set. Syst., 20 (1986), 87–96. https://doi.org/10.1016/S0165-0114(86)80034-3 doi: 10.1016/S0165-0114(86)80034-3
![]() |
[7] | R. R. Yager, Pythagorean fuzzy subsets, 2013 Joint IFSA World Congress and NAFIPS Annual Meeting (IFSA/NAFIPS), Edmonton, AB, Canada, 2013, 57–61. https://doi.org/10.1109/IFSA-NAFIPS.2013.6608375 |
[8] |
R. R. Yager, Generalized orthopair fuzzy sets, IEEE T. Fuzzy Syst., 25 (2017), 1222–1230. https://doi.org/10.1109/TFUZZ.2016.2604005 doi: 10.1109/TFUZZ.2016.2604005
![]() |
[9] |
L. A. Zadeh, The concept of a linguistic variable and its application to approximate reasoning–I, Inform. Sciences, 8 (1975), 199–249. https://doi.org/10.1016/0020-0255(75)90036-5 doi: 10.1016/0020-0255(75)90036-5
![]() |
[10] |
Z. C. Chen, P. H. Liu, Z. Pei, An approach to multiple attribute group decision making based on linguistic intuitionistic fuzzy numbers, Int. J. Comput. Int. Sys., 8 (2015), 747–760. https://doi.org/10.1080/18756891.2015.1061394 doi: 10.1080/18756891.2015.1061394
![]() |
[11] |
M. Akram, S. Naz, T. Abbas, Complex q-rung orthopair fuzzy 2-tuple linguistic group decision-making framework with Muirhead mean operators, Artif. Intell. Rev., 56 (2023), 10227–10274. https://doi.org/10.1007/s10462-023-10408-4 doi: 10.1007/s10462-023-10408-4
![]() |
[12] |
M. Akram, N. Ramzan, A. Luqman, G. Santos-García, An integrated MULTIMOORA method with 2-tuple linguistic Fermatean fuzzy sets: Urban quality of life selection application, AIMS Mathematics, 8 (2023), 2798–2828. https://doi.org/10.3934/math.2023147 doi: 10.3934/math.2023147
![]() |
[13] |
M. R. Seikh, U. Mandal, Interval-valued Fermatean fuzzy Dombi aggregation operators and SWARA based PROMETHEE II method to bio-medical waste management, Expert Syst. Appl., 226 (2023), 120082. https://doi.org/10.1016/j.eswa.2023.120082 doi: 10.1016/j.eswa.2023.120082
![]() |
[14] |
P. D. Liu, X. X. Liu, G. Y. Ma, Z. L. Liang, C. H. Wang, F. E. Alsaadi, A multi-attribute group decision-making method based on linguistic intuitionistic fuzzy numbers and Dempster-Shafer evidence theory, Int. J. Inf. Tech. Decis., 19 (2020), 499–524. https://doi.org/10.1142/S0219622020500042 doi: 10.1142/S0219622020500042
![]() |
[15] |
R. P. Yuan, J. Tang, F. Y. Meng, Linguistic intuitionistic fuzzy group decision making based on aggregation operators, Int. J. Fuzzy Syst., 21 (2019), 407–420. https://doi.org/10.1007/s40815-018-0582-4 doi: 10.1007/s40815-018-0582-4
![]() |
[16] |
X. J. Gou, X. R. Xu, F. M. Deng, W. Zhou, E. Herrera-Viedma, Medical health resources allocation evaluation in public health emergencies by an improved ORESTE method with linguistic preference orderings, Fuzzy Optim. Decis. Making, 23 (2024), 1–27. https://doi.org/10.1007/s10700-023-09409-3 doi: 10.1007/s10700-023-09409-3
![]() |
[17] |
X. T. Cheng, K. Zhang, T. Wu, Z. S. Xu, X. J. Gou, An opinions-updating model for large-scale group decision-making driven by autonomous learning, Inform. Sciences, 662 (2024), 120238. https://doi.org/10.1016/j.ins.2024.120238 doi: 10.1016/j.ins.2024.120238
![]() |
[18] |
M. R. Seikh, U. Mandal, q-Rung orthopair fuzzy Archimedean aggregation operators: application in the site selection for software operating units, Symmetry, 15 (2023), 1680. https://doi.org/10.3390/sym15091680 doi: 10.3390/sym15091680
![]() |
[19] |
M. R. Seikh, U. Mandal, Q-Rung orthopair fuzzy Frank aggregation operators and its application in multiple attribute decision-making with unknown attribute weights, Granul. Comput., 7 (2022), 709–730. https://doi.org/10.1007/s41066-021-00290-2 doi: 10.1007/s41066-021-00290-2
![]() |
[20] | H. Garg, K. Kumar, Group decision making approach based on possibility degree measures and the linguistic intuitionistic fuzzy aggregation operators using Einstein norm operations, J. Mult.-Valued Log. S., 31 (2018), 175–209. |
[21] |
H. Garg, K. Kumar, Some aggregation operators for linguistic intuitionistic fuzzy set and its application to group decision-making process using the set pair analysis, Arab. J. Sci. Eng., 43 (2018), 3213–3227. https://doi.org/10.1007/s13369-017-2986-0 doi: 10.1007/s13369-017-2986-0
![]() |
[22] |
H. Garg, T. Mahmood, U. U. Rehman, G. N. Nguyen, Multi-attribute decision-making approach based on Aczel-Alsina power aggregation operators under bipolar fuzzy information and its application to quantum computing, Alex. Eng. J., 82 (2023), 248–259. https://doi.org/10.1016/j.aej.2023.09.073 doi: 10.1016/j.aej.2023.09.073
![]() |
[23] |
K. Kumar, S. M. Chen, Group decision making based on improved linguistic interval-valued Atanassov intuitionistic fuzzy weighted averaging aggregation operator of linguistic interval-valued Atanassov intuitionistic fuzzy numbers, Inform. Sciences, 607 (2022), 884–900. https://doi.org/10.1016/j.ins.2022.05.082 doi: 10.1016/j.ins.2022.05.082
![]() |
[24] |
K. Kumar, S. M. Chen, Multiple attribute group decision making based on advanced linguistic intuitionistic fuzzy weighted averaging aggregation operator of linguistic intuitionistic fuzzy numbers, Inform. Sciences, 587 (2022), 813–824. https://doi.org/10.1016/j.ins.2021.11.014 doi: 10.1016/j.ins.2021.11.014
![]() |
[25] | Y. Liu, G. W. Wei, H. B. Liu, L. Xu, Group decision making for internet public opinion emergency based upon linguistic intuitionistic fuzzy information, Int. J. Mach. Learn. & Cyber., 13 (2022), 579–594. https://doi.org/10.1007/s13042-020-01262-9 |
[26] |
X. J. Gou, Z. S. Xu, H. C. Liao, F. Herrera, Probabilistic double hierarchy linguistic term set and its use in designing an improved VIKOR method: The application in smart healthcare, J. Oper. Res. Soc., 72 (2021), 2611–2630. https://doi.org/10.1080/01605682.2020.1806741 doi: 10.1080/01605682.2020.1806741
![]() |
[27] |
J. Tang, F. Y. Meng, Linguistic intuitionistic fuzzy Hamacher aggregation operators and their application to group decision making, Granul. Comput., 4 (2019), 109–124. https://doi.org/10.1007/s41066-018-0089-2 doi: 10.1007/s41066-018-0089-2
![]() |
[28] |
K. Kumar, S. M. Chen, Group decision making based on linguistic intuitionistic fuzzy Yager weighted arithmetic aggregation operator of linguistic intuitionistic fuzzy numbers, Inform. Sciences, 647 (2023), 119228. https://doi.org/10.1016/j.ins.2023.119228 doi: 10.1016/j.ins.2023.119228
![]() |
[29] |
R. Verma, N. Agarwal, Multiple attribute group decision-making based on generalized aggregation operators under linguistic interval-valued Pythagorean fuzzy environment, Granul. Comput., 7 (2022), 591–632. https://doi.org/10.1007/s41066-021-00286-y doi: 10.1007/s41066-021-00286-y
![]() |
[30] |
A. Fahmi, S. Abdullah, F. Amin, Aggregation operators on cubic linguistic hesitant fuzzy numbers and their application in group decision-making, Granul. Comput., 6 (2021), 303–320. https://doi.org/10.1007/s41066-019-00188-0 doi: 10.1007/s41066-019-00188-0
![]() |
[31] |
K. Kumar, S. M. Chen, Multiattribute decision making based on q-rung orthopair fuzzy Yager prioritized weighted arithmetic aggregation operator of q-rung orthopair fuzzy numbers, Inform. Sciences, 657 (2024), 119984. https://doi.org/10.1016/j.ins.2023.119984 doi: 10.1016/j.ins.2023.119984
![]() |
[32] |
X. T. Cheng, Z. S. Xu, X. J. Gou, A large-scale group decision-making model considering risk attitudes and dynamically changing roles, Expert Syst. Appl., 245 (2024), 123017. https://doi.org/10.1016/j.eswa.2023.123017 doi: 10.1016/j.eswa.2023.123017
![]() |
[33] |
F. X. Jin, F. Wang, K. Zhao, H. T. Chen, J. L. G. Guirao, The method of judging satisfactory consistency of linguistic judgment matrix based on adjacency matrix and 3-loop matrix, AIMS Mathematics, 9 (2024), 18944–18967. https://doi.org/10.3934/math.2024922 doi: 10.3934/math.2024922
![]() |
[34] |
M. W. Lin, X. M. Li, L. F. Chen, Linguistic q‐rung orthopair fuzzy sets and their interactional partitioned Heronian mean aggregation operators, Int. J. Intell. Syst., 35 (2019), 217–249. https://doi.org/10.1002/int.22136 doi: 10.1002/int.22136
![]() |
[35] |
P. D. Liu, W. Q. Liu, Multiple-attribute group decision-making based on power Bonferroni operators of linguistic q-rung orthopair fuzzy numbers, Int. J. Intell. Syst., 34 (2018), 652–689. https://doi.org/10.1002/int.22071 doi: 10.1002/int.22071
![]() |
[36] |
P. D. Liu, W. Q. Liu, Multiple‐attribute group decision‐making method of linguistic q‐rung orthopair fuzzy power Muirhead mean operators based on entropy weight, Int. J. Intell. Syst., 34 (2019), 1755–1794. https://doi.org/10.1002/int.22114 doi: 10.1002/int.22114
![]() |
[37] |
M. J. Ranjan, B. P. Kumar, T. D. Bhavani, A. V. Padmavathi, V. Bakka, Probabilistic linguistic q-rung orthopair fuzzy Archimedean aggregation operators for group decision-making, Decision Making: Applications in Management and Engineering, 6 (2023), 639–667. https://doi.org/10.31181/dmame622023527 doi: 10.31181/dmame622023527
![]() |
[38] |
H. J. Wang, Y. Liu, Y. Rong, Group decision making approach based on linguistic q-rung orthopair fuzzy generalized shapley bi-direction Choquet-copula operators, Granul. Comput., 8 (2023), 1837–1856. https://doi.org/10.1007/s41066-023-00404-y doi: 10.1007/s41066-023-00404-y
![]() |
[39] |
G. Ali, M. Afzal, M. Asif, A. Shazad, Attribute reduction approaches under interval-valued q-rung orthopair fuzzy soft framework, Appl. Intell., 52 (2022), 8975–9000. https://doi.org/10.1007/s10489-021-02853-x doi: 10.1007/s10489-021-02853-x
![]() |
[40] |
N. Deb, A. Sarkar, A. Biswas, Linguistic q-rung orthopair fuzzy prioritized aggregation operators based on Hamacher t-norm and t-conorm and their applications to multicriteria group decision making, Arch. Control Sci., 32 (2022), 451–484. https://doi.org/10.24425/acs.2022.141720 doi: 10.24425/acs.2022.141720
![]() |
[41] | P. D. Liu, S. Naz, M. Akram, M. Muzammal, Group decision-making analysis based on linguistic q-rung orthopair fuzzy generalized point weighted aggregation operators, Int. J. Mach. Learn. & Cyber., 13 (2022), 883–906. https://doi.org/10.1007/s13042-021-01425-2 |
[42] |
M. Akram, S. Naz, S. A. Edalatpanah, R. Mehreen, Group decision-making framework under linguistic q-rung orthopair fuzzy Einstein models, Soft Comput., 25 (2021), 10309–10334. https://doi.org/10.1007/s00500-021-05771-9 doi: 10.1007/s00500-021-05771-9
![]() |
[43] |
C. Jana, M. Dobrodolac, V. Simic, M. Pal, B. Sarkar, Ž. Stević, Evaluation of sustainable strategies for urban parcel delivery: Linguistic q-rung orthopair fuzzy Choquet integral approach, Eng. Appl. Artif. Intel., 126 (2023), 106811. https://doi.org/10.1016/j.engappai.2023.106811 doi: 10.1016/j.engappai.2023.106811
![]() |
[44] | S. Naz, M. Akram, A. Shafiq, K. Akhtar, Optimal airport selection utilizing power Muirhead mean based group decision model with 2-tuple linguistic q-rung orthopair fuzzy information, Int. J. Mach. Learn. & Cyber., 15 (2024), 303–340. https://doi.org/10.1007/s13042-023-01911-9 |
[45] |
J. Aczél, C. Alsina, Characterizations of some classes of quasilinear functions with applications to triangular norms and to synthesizing judgements, Aeq. Math., 25 (1982), 313–315. https://doi.org/10.1007/BF02189626 doi: 10.1007/BF02189626
![]() |
[46] | F. Farahbod, M. Efekhari, Comparison of different t-norm operators in classification problems, 2012, arXiv: 1208.1955. https://doi.org/10.48550/arXiv.1208.1955 |
[47] |
M. Tahir, Azam, U. ur Rehman, J. Ahmmad, Prioritization and selection of operating system by employing geometric aggregation operators based on Aczel-Alsina t-norm and t-conorm in the environment of bipolar complex fuzzy set, AIMS Mathematics, 8 (2023), 25220–25248. https://doi.org/10.3934/math.20231286 doi: 10.3934/math.20231286
![]() |
[48] |
M. Tahir, Z. Ali, Multi-attribute decision-making methods based on Aczel-Alsina power aggregation operators for managing complex intuitionistic fuzzy sets, Comp. Appl. Math., 42 (2023), 87. https://doi.org/10.1007/s40314-023-02204-1 doi: 10.1007/s40314-023-02204-1
![]() |
[49] |
H. Garg, Tehreem, G. N. Nguyen, T. Alballa, H. A. E. W. Khalifa, Choquet integral-based Aczel–Alsina aggregation operators for interval-valued intuitionistic fuzzy information and their application to human activity recognition, Symmetry, 15 (2023), 1438. https://doi.org/10.3390/sym15071438 doi: 10.3390/sym15071438
![]() |
[50] |
P. D. Liu, Z. Ali, T. Mahmood, Y. S. Geng, Prioritized aggregation operators for complex intuitionistic fuzzy sets based on Aczel-Alsina t-norm and t-conorm and their applications in decision-making, Int. J. Fuzzy Syst., 25 (2023), 2590–2608. https://doi.org/10.1007/s40815-023-01541-x doi: 10.1007/s40815-023-01541-x
![]() |
[51] |
Z. U. Rehman, G. Ali, M. Asif, Y. F. Chen, M. Z. U. Abidin, Identification of desalination and wind power plants sites using m-polar fuzzy Aczel-Alsina aggregation information, Sci. Rep., 14 (2024), 409. https://doi.org/10.1038/s41598-023-50397-6 doi: 10.1038/s41598-023-50397-6
![]() |
[52] |
G. Ali, A. Farooq, M. M. A. Al-Shamiri, Novel multiple criteria decision-making analysis under m-polar fuzzy aggregation operators with application, Math. Biosci. Eng., 20 (2023), 3566–3593. https://doi.org/10.3934/mbe.2023166 doi: 10.3934/mbe.2023166
![]() |
[53] |
A. Ali, K. Ullah, A. Hussain. An approach to multi-attribute decision-making based on intuitionistic fuzzy soft information and Aczel-Alsina operational laws, Journal of Decision Analytics and Intelligent Computing, 3 (2023), 80–89. https://doi.org/10.31181/jdaic10006062023a doi: 10.31181/jdaic10006062023a
![]() |
[54] |
M. Akram, K. Ullah, G. Cirovic, D Pamucar, Algorithm for energy resource selection using priority degree-based aggregation operators with generalized orthopair fuzzy information and Aczel-Alsina aggregation operators, Energies, 16 (2023), 2816. https://doi.org/10.3390/en16062816 doi: 10.3390/en16062816
![]() |
[55] |
X. M. Shi, Z. Ali, T. Mahmood, P. D. Liu, Power aggregation operators of interval-valued Atanassov-intuitionistic fuzzy sets based on Aczel-Alsina t-norm and t-conorm and their applications in decision making, Int. J. Comput. Intell. Syst., 16 (2023), 43. https://doi.org/10.1007/s44196-023-00208-7 doi: 10.1007/s44196-023-00208-7
![]() |
[56] |
M. M. A. Al-Shamiri, A. Farooq, M. Nabeel, G. Ali, D. Pamucar, Integrating TOPSIS and ELECTRE-I methods with cubic m-polar fuzzy sets and its application to the diagnosis of psychiatric disorders, AIMS Mathematics, 8 (2023), 11875–11915. https://doi.org/10.3934/math.2023601 doi: 10.3934/math.2023601
![]() |
[57] |
P. D. Liu, P. Wang, Some improved linguistic intuitionistic fuzzy aggregation operators and their applications to multiple-attribute decision making, Int. J. Inf. Tech. Decis., 16 (2017), 817–850. https://doi.org/10.1142/S0219622017500110 doi: 10.1142/S0219622017500110
![]() |
[58] |
R. Verma, J. M. Merigo, Multiple attribute group decision making based on 2-dimension linguistic intuitionistic fuzzy aggregation operators, Soft Comput., 24 (2020), 17377–17400. https://doi.org/10.1007/s00500-020-05026-z doi: 10.1007/s00500-020-05026-z
![]() |
[59] |
Z. S. Xu, A method based on linguistic aggregation operators for group decision making with linguistic preference relations, Inform. Sciences, 166 (2004), 19–30. https://doi.org/10.1016/j.ins.2003.10.006 doi: 10.1016/j.ins.2003.10.006
![]() |
[60] |
M. R. Khan, K. Ullah, H. Karamti, Q. Khan, T. Mahmood, Multi-attribute group decision-making based on q-rung orthopair fuzzy Aczel-Alsina power aggregation operators, Eng. Appl. Artif. Intel., 126 (2023), 106629. https://doi.org/10.1016/j.engappai.2023.106629 doi: 10.1016/j.engappai.2023.106629
![]() |
[61] |
M. R. Seikh, U. Mandal, Multiple attribute group decision making based on quasirung orthopair fuzzy sets: Application to electric vehicle charging station site selection problem, Eng. Appl. Artif. Intel., 115 (2022), 105299. https://doi.org/10.1016/j.engappai.2022.105299 doi: 10.1016/j.engappai.2022.105299
![]() |
[62] |
M. R. Seikh, U. Mandal, Multiple attribute decision-making based on 3, 4-quasirung fuzzy sets, Granul. Comput., 7 (2022), 965–978, https://doi.org/10.1007/s41066-021-00308-9 doi: 10.1007/s41066-021-00308-9
![]() |
1 ^{\rm st} -GDM method | Ranking orders |
Liu and Wang's GDM technique [57] | \mathcal{R}_{4} > \mathcal{R}_{3} > \mathcal{R}_{2} > \mathcal{R}_{1} |
Chen et al.'s GDM method [10] | \mathcal{R}_{4} > \mathcal{R}_{3} > \mathcal{R}_{1} > \mathcal{R}_{2} |
Kumar and Chen's GDM technique [24] | \mathcal{R}_{4} > \mathcal{R}_{3} > \mathcal{R}_{1} > \mathcal{R}_{2} |
Garg and Kumar's GDM method [20] | \mathcal{R}_{4} > \mathcal{R}_{1} > \mathcal{R}_{3} > \mathcal{R}_{2} |
Nayana et al. GDM technique [40] | \mathcal{R}_{4} > \mathcal{R}_{1} > \mathcal{R}_{3} > \mathcal{R}_{2} |
Kumar and Chen's GDM method [28] | \mathcal{R}_{4} > \mathcal{R}_{3} > \mathcal{R}_{1} > \mathcal{R}_{2} |
The suggested first GDM approach | \mathcal{R}_{4} > \mathcal{R}_{3} > \mathcal{R}_{1} > \mathcal{R}_{2} |
Parameter ( q ) | \mathcal{Y}(\varpi_{1}) | \mathcal{Y}(\varpi_{2}) | \mathcal{Y}(\varpi_{3}) | \mathcal{Y}(\varpi_{4}) | Ranking orders (or \mathcal{RO}s ) |
q=1 | 4.08 | 3.60 | 4.21 | 5.50 | \mathcal{R}_{4} > \mathcal{R}_{3} > \mathcal{R}_{1} > \mathcal{R}_{2} |
q=2 | 4.39 | 4.01 | 4.54 | 4.76 | \mathcal{R}_{4} > \mathcal{R}_{3} > \mathcal{R}_{1} > \mathcal{R}_{2} |
q=3 | 4.44 | 4.03 | 4.57 | 5.23 | \mathcal{R}_{4} > \mathcal{R}_{3} > \mathcal{R}_{1} > \mathcal{R}_{2} |
q=4 | 4.45 | 4.04 | 4.56 | 5.12 | \mathcal{R}_{4} > \mathcal{R}_{3} > \mathcal{R}_{1} > \mathcal{R}_{2} |
q=5 | 4.51 | 4.11 | 4.60 | 5.10 | \mathcal{R}_{4} > \mathcal{R}_{3} > \mathcal{R}_{1} > \mathcal{R}_{2} |
q=6 | 4.75 | 4.30 | 4.82 | 5.31 | \mathcal{R}_{4} > \mathcal{R}_{3} > \mathcal{R}_{1} > \mathcal{R}_{2} |
q=7 | 4.71 | 4.27 | 4.77 | 5.23 | \mathcal{R}_{4} > \mathcal{R}_{3} > \mathcal{R}_{1} > \mathcal{R}_{2} |
q=8 | 4.65 | 4.22 | 4.71 | 5.14 | \mathcal{R}_{4} > \mathcal{R}_{3} > \mathcal{R}_{1} > \mathcal{R}_{2} |
q=9 | 4.73 | 4.29 | 4.78 | 5.22 | \mathcal{R}_{4} > \mathcal{R}_{3} > \mathcal{R}_{1} > \mathcal{R}_{2} |
q=10 | 4.69 | 4.27 | 4.75 | 5.18 | \mathcal{R}_{4} > \mathcal{R}_{3} > \mathcal{R}_{1} > \mathcal{R}_{2} |
1 ^{\rm st} -GDM method | Ranking orders |
Liu and Wang's GDM technique [57] | \mathcal{R}_{4} > \mathcal{R}_{3} > \mathcal{R}_{2} > \mathcal{R}_{1} |
Chen et al.'s GDM method [10] | \mathcal{R}_{4} > \mathcal{R}_{3} > \mathcal{R}_{1} > \mathcal{R}_{2} |
Kumar and Chen's GDM technique [24] | \mathcal{R}_{4} > \mathcal{R}_{3} > \mathcal{R}_{1} > \mathcal{R}_{2} |
Garg and Kumar's GDM method [20] | \mathcal{R}_{4} > \mathcal{R}_{1} > \mathcal{R}_{3} > \mathcal{R}_{2} |
Nayana et al. GDM technique [40] | \mathcal{R}_{4} > \mathcal{R}_{1} > \mathcal{R}_{3} > \mathcal{R}_{2} |
Kumar and Chen's GDM method [28] | \mathcal{R}_{4} > \mathcal{R}_{3} > \mathcal{R}_{1} > \mathcal{R}_{2} |
The suggested first GDM approach | \mathcal{R}_{4} > \mathcal{R}_{3} > \mathcal{R}_{1} > \mathcal{R}_{2} |
Parameter ( q ) | \mathcal{Y}(\varpi_{1}) | \mathcal{Y}(\varpi_{2}) | \mathcal{Y}(\varpi_{3}) | \mathcal{Y}(\varpi_{4}) | Ranking orders (or \mathcal{RO}s ) |
q=1 | 4.08 | 3.60 | 4.21 | 5.50 | \mathcal{R}_{4} > \mathcal{R}_{3} > \mathcal{R}_{1} > \mathcal{R}_{2} |
q=2 | 4.39 | 4.01 | 4.54 | 4.76 | \mathcal{R}_{4} > \mathcal{R}_{3} > \mathcal{R}_{1} > \mathcal{R}_{2} |
q=3 | 4.44 | 4.03 | 4.57 | 5.23 | \mathcal{R}_{4} > \mathcal{R}_{3} > \mathcal{R}_{1} > \mathcal{R}_{2} |
q=4 | 4.45 | 4.04 | 4.56 | 5.12 | \mathcal{R}_{4} > \mathcal{R}_{3} > \mathcal{R}_{1} > \mathcal{R}_{2} |
q=5 | 4.51 | 4.11 | 4.60 | 5.10 | \mathcal{R}_{4} > \mathcal{R}_{3} > \mathcal{R}_{1} > \mathcal{R}_{2} |
q=6 | 4.75 | 4.30 | 4.82 | 5.31 | \mathcal{R}_{4} > \mathcal{R}_{3} > \mathcal{R}_{1} > \mathcal{R}_{2} |
q=7 | 4.71 | 4.27 | 4.77 | 5.23 | \mathcal{R}_{4} > \mathcal{R}_{3} > \mathcal{R}_{1} > \mathcal{R}_{2} |
q=8 | 4.65 | 4.22 | 4.71 | 5.14 | \mathcal{R}_{4} > \mathcal{R}_{3} > \mathcal{R}_{1} > \mathcal{R}_{2} |
q=9 | 4.73 | 4.29 | 4.78 | 5.22 | \mathcal{R}_{4} > \mathcal{R}_{3} > \mathcal{R}_{1} > \mathcal{R}_{2} |
q=10 | 4.69 | 4.27 | 4.75 | 5.18 | \mathcal{R}_{4} > \mathcal{R}_{3} > \mathcal{R}_{1} > \mathcal{R}_{2} |