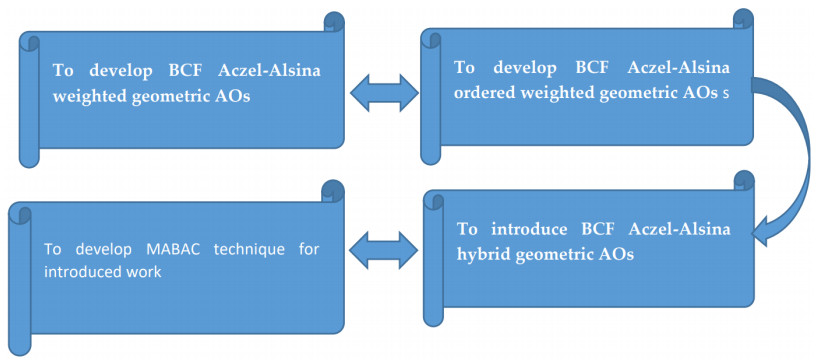
Aczel-Alsina t-norm and t-conorm are great substitutes for sum and product and recently various scholars developed notions based on the Aczel-Alsina t-norm and t-conorm. The theory of bipolar complex fuzzy set that deals with ambiguous and complex data that contains positive and negative aspects along with a second dimension. So, based on Aczel-Alsina operational laws and the dominant structure of the bipolar complex fuzzy set, we develop the notion of bipolar complex fuzzy Aczel-Alsina weighted geometric, bipolar complex fuzzy Aczel Alsina ordered weighted geometric and bipolar complex fuzzy Aczel Alsina hybrid geometric operators. Moreover, multi-attribute border approximation area comparison technique is a valuable technique that can cover many decision-making situations and have dominant results. So, based on bipolar complex fuzzy Aczel-Alsina aggregation operators, we demonstrate the notion of a multi-attribute border approximation area comparison approach for coping with bipolar complex fuzzy information. After that, we take a numerical example by taking artificial data for various types of operating systems and determining the finest operating system for a computer. In the end, we compare the deduced multi-attribute border approximation area comparison approach and deduced aggregation operators with numerous prevailing works.
Citation: Tahir Mahmood, Azam, Ubaid ur Rehman, Jabbar Ahmmad. Prioritization and selection of operating system by employing geometric aggregation operators based on Aczel-Alsina t-norm and t-conorm in the environment of bipolar complex fuzzy set[J]. AIMS Mathematics, 2023, 8(10): 25220-25248. doi: 10.3934/math.20231286
[1] | Saba Ijaz, Kifayat Ullah, Maria Akram, Dragan Pamucar . Approaches to multi-attribute group decision-making based on picture fuzzy prioritized Aczel–Alsina aggregation information. AIMS Mathematics, 2023, 8(7): 16556-16583. doi: 10.3934/math.2023847 |
[2] | Chunxiao Lu, Zeeshan Ali, Peide Liu . Selection of artificial neutral networks based on cubic intuitionistic fuzzy Aczel-Alsina aggregation operators. AIMS Mathematics, 2024, 9(10): 27797-27833. doi: 10.3934/math.20241350 |
[3] | Ghous Ali, Kholood Alsager, Asad Ali . Novel linguistic $ q $-rung orthopair fuzzy Aczel-Alsina aggregation operators for group decision-making with applications. AIMS Mathematics, 2024, 9(11): 32328-32365. doi: 10.3934/math.20241551 |
[4] | Wajid Ali, Tanzeela Shaheen, Iftikhar Ul Haq, Hamza Toor, Faraz Akram, Harish Garg, Md. Zia Uddin, Mohammad Mehedi Hassan . Aczel-Alsina-based aggregation operators for intuitionistic hesitant fuzzy set environment and their application to multiple attribute decision-making process. AIMS Mathematics, 2023, 8(8): 18021-18039. doi: 10.3934/math.2023916 |
[5] | Tehreem, Harish Garg, Kinza Ayaz, Walid Emam . Multi attribute decision-making algorithms using Hamacher Choquet-integral operators with complex intuitionistic fuzzy information. AIMS Mathematics, 2024, 9(12): 35860-35884. doi: 10.3934/math.20241700 |
[6] | Aziz Khan, Shahzaib Ashraf, Saleem Abdullah, Muhammad Ayaz, Thongchai Botmart . A novel decision aid approach based on spherical hesitant fuzzy Aczel-Alsina geometric aggregation information. AIMS Mathematics, 2023, 8(3): 5148-5174. doi: 10.3934/math.2023258 |
[7] | Muhammad Ali Khan, Saleem Abdullah, Alaa O. Almagrabi . Analysis of deep learning technique using a complex spherical fuzzy rough decision support model. AIMS Mathematics, 2023, 8(10): 23372-23402. doi: 10.3934/math.20231188 |
[8] | Muhammad Naeem, Younas Khan, Shahzaib Ashraf, Wajaree Weera, Bushra Batool . A novel picture fuzzy Aczel-Alsina geometric aggregation information: Application to determining the factors affecting mango crops. AIMS Mathematics, 2022, 7(7): 12264-12288. doi: 10.3934/math.2022681 |
[9] | Khawlah Alhulwah, Muhammad Azeem, Mehwish Sarfraz, Nasreen Almohanna, Ali Ahmad . Prioritized aggregation operators for Schweizer-Sklar multi-attribute decision-making for complex spherical fuzzy information in mobile e-tourism applications. AIMS Mathematics, 2024, 9(12): 34753-34784. doi: 10.3934/math.20241655 |
[10] | Muhammad Naeem, Aziz Khan, Shahzaib Ashraf, Saleem Abdullah, Muhammad Ayaz, Nejib Ghanmi . A novel decision making technique based on spherical hesitant fuzzy Yager aggregation information: application to treat Parkinson's disease. AIMS Mathematics, 2022, 7(2): 1678-1706. doi: 10.3934/math.2022097 |
Aczel-Alsina t-norm and t-conorm are great substitutes for sum and product and recently various scholars developed notions based on the Aczel-Alsina t-norm and t-conorm. The theory of bipolar complex fuzzy set that deals with ambiguous and complex data that contains positive and negative aspects along with a second dimension. So, based on Aczel-Alsina operational laws and the dominant structure of the bipolar complex fuzzy set, we develop the notion of bipolar complex fuzzy Aczel-Alsina weighted geometric, bipolar complex fuzzy Aczel Alsina ordered weighted geometric and bipolar complex fuzzy Aczel Alsina hybrid geometric operators. Moreover, multi-attribute border approximation area comparison technique is a valuable technique that can cover many decision-making situations and have dominant results. So, based on bipolar complex fuzzy Aczel-Alsina aggregation operators, we demonstrate the notion of a multi-attribute border approximation area comparison approach for coping with bipolar complex fuzzy information. After that, we take a numerical example by taking artificial data for various types of operating systems and determining the finest operating system for a computer. In the end, we compare the deduced multi-attribute border approximation area comparison approach and deduced aggregation operators with numerous prevailing works.
The first and most important product created by researchers at the dawn of modern technology is the computer. The computer is currently regarded as a high-level technology worldwide. Most of the technologies that humans develop will demand us to do things that are outside of our normal capabilities. Inventions like computers allowed for the quick automation of mathematical computations that were beyond the capabilities of even the smartest minds. Computers are a new tool being used in research. Regardless of whether it is being used for academic or commercial research, it is a crucial tool. Researchers in the fields of computing and information build creative applications for both new and old technologies. For use in business, science, health, and other disciplines, they research and address challenging computing-related issues. Computers are used to enter, update and manage data effectively, including taking follow-up activities, etc. Additionally, computers enable more flexibility in the data sets, allowing for easier data processing as well as more flexible data recording. In addition to having the ability to handle enormous amounts of data, it can also analyze data using a variety of statistical techniques. Statistics-based operations are performed by the computer. Computers process and analyze data quickly and accurately. The statistical analysis that used to take weeks or even months now just takes a few seconds or minutes. The operating system (OS) is crucial to the computer processing system, just as computers are essential in every aspect of life. The core of a computer is its OS. The computer cannot operate correctly and efficiently without it. A boot program loads an OS into the system, which then manages all other application programs in the computer. The OS is the most important application that runs on a computer. In a typical computing environment, many applications are running simultaneously and need access to the CPU, memory, and storage. So, OS is a key factor in the computer system. An OS is extremely beneficial to the creation of software and computer applications. Without an OS, each application would need to control all computer activities and other features, as well as its user interface and the whole amount of code necessary to do so. With the vast range of underlying technologies available, this would be challenging. Hansen [1] proposed the principal OS. Silberschatz et al. [2] introduced the applied OS concept. Comer [3] presented the idea of OS design. Klein and Sadhana [4] elaborated on an overview of OS verification. There are various applications of the OS which is used in many systems. Mercer [5] deduced OS support for multimedia. The idea of OS support for virtual machines was first suggested by King et al. [6]. The design and development of an OS to handle distributed multimedia applications were suggested by Leslie et al. [7]. Singh [8] provided a summary of the security features of the Android OS.
Among the most useful and trustworthy methods now employed in several fields is without a doubt the multi-attribute decision-making (MADM) methodology. A common real-world technique is the MADM technique, in which the decision-maker chooses the optimal opinion (alternative). MADM is widely used, has a wide range of applications and includes both qualitative and quantitative characteristic values. As complexity and uncertainty grow more and more every day. Therefore, it is challenging for experts or decision-makers to use crisp data to make the optimal judgment due to the complexity, ambiguity and uncertainty in the data. It is because, in a crisp set, there is only two types of option yes or no, include or not included, and true or false. This means that there are only two number options, 0 and 1. Yes, include and true means 1, and 0 means false, not included and no. So, due to these restrictions and limitations in crisp data, it is not suitable for experts and decision-makers to make the best solution using crisp information. To overcome these hurdles and complexities, vagueness and uncertainties in data, a fuzzy set (FS) was proposed by Zadeh [9]. Each element is given a degree of support (DS) in the range [0, 1] and an FS is an extension of the crisp set. The applications of FS were discussed by Zimmermann [10]. Riaz et al. [11] developed the concept of spherical linear Diophantine FS (LDFS)with modeling uncertainties in multi-criteria DM (MCDM). Ayub et al. [12] introduced the concept of LDF rough sets. A new rough set approach with DM Riaz and Hashmi. [13] Riaz et al. developed linear LDF and its applications toward MADM problems. Tolga et al. [14] investigated the OS selection process by employing the fuzzy AHP technique. Balli and Korukoglu [15] studied the OS selection process by employing the fuzzy TOPSIS approach. Kandel et al. [16] discussed fuzzy logic in OS. The aggregation operators (AOs) for fuzzy DM were deduced by Mardani et al. [17].
Each of the object's properties has a corresponding counter property, it was observed i.e., effect and side effect, positive and negative, fair and unfair, pass and fail, satisfactory and unsatisfactory, good and bad, healthy and unhealthy, pure and impure, correct and incorrect, etc. Thus, the concept of bipolar FS (BFS), which is a generalization of FS, was introduced by Zhang [18] by converting the range from [0, 1] to [-1, 1]. In BFS there are two types of membership for each element i.e., positive degree of support (PDS) and negative degree of support (NDS). The PDS and NDS both fall inside the enclosed range [0, 1] and [-1, 0] respectively. Computational psychiatry, biosystem control and bipolar quantum logic-based computing are several areas where BF is applied. Furthermore, the concept of BF Hamacher AOs in MADM was presented by Wei et al. [19]. Jana et al. [20] introduced the concept of BF Dombi AOs and their use in MADM processes. The BF graphs were deduced by Akram [21] and applications of BF graphs were also described by Akram [22]. Samanta and Pal [23] deduced irregular BF graphs and Rashmanlou et al. [24] discussed the product of BF graphs. The DM technique under BF information was deduced by Alghamdi et al. [25]. Akram et al. [26] proposed a method for solving BF complex linear systems with real and complex coefficients. Moreover, Akram et al. [27] established DM analysis based on BF N-soft information. Also, Akram and Al-Kenani [28] developed the concept of multi-criteria group DM (MCGDM) for the selection of green suppliers under the BF PROMETEE process. Akram et al. [29] introduced the concept of BF TOPSIS and BF ELECTRIC-I methods. Furthermore, Akram et al. [30] established group DM based on the VIKOR method with trapezoidal BF information. Akram and Arshad [31] proposed a novel trapezoidal BF TOPSIS method for group DM. Also, Akram et al. [32] provide the solution of a complex BF linear system, in progress in intelligence decision science. Jana et al. [33] proposed BF Dombi prioritized AOs in MADM. Riaz and Tehrim [34] introduced MAGDM based on cubic BF information using averaging AO.
Ramot et al. [35] deduced the model of complex FS (CFS) which is also the generalization of FS and transform in FS by setting the phase term in DS equal to zero. The DS of each element of CFS is in polar form and is a place in the unit disc of a complex plane. Subsequently, Tamir et al. [36] invented the CFS in a cartesian model and modified the range of the DS from a unit disc to a unit square in a complex plane. Moreover, Tamir et al. [37] interpreted CF logic. The CF geometric AOs were exhibited by Bi et al. [38]. The CF arithmetic AOs were prepared by Bi et al. [39].
The data analyst expertly employed BFS when they have to make decisions regarding both negative and positive features of an object or element and CFS when they have to make decisions on two-dimensional information. But when face two-dimensional information that has both good and bad features, what do data analysts and specialists do? So, motivated by the aforementioned theory, Mahmood and Ur Rehman [40] invented a structure of bipolar complex FS (BCFS). BCFS is more generalized, efficient and dominant as compared to FS, BFS and CFS. BCFS has the effective expertise to tackle the uncertainty, ambiguity and inconsistency which appear in genuine-life dilemmas. The Dombi AOs under the environment of BCFS were investigated by Mahmood and Ur Rehman [41]. Mahmood et al. [42] deduced Aczel-Alsina averaging AOs for BCFS.
Pamucar and Cirovic [43] deduced a technique named multi-attribute border approximation area comparison (MABAC) which is a unique technique for tackling MADM dilemmas. It relies on the distance among alternatives and the border approximation area (BAA) for every attribute. The biggest benefit of the MABAC technique is its straightforward calculation and stability of the solution. The technique of MABAC has more strength for the modification of the criterion measurement scale and the formulation of the criteria, compared to other techniques of MADM and is more sensitive to the modification of the criteria weights. The structure of MABAC takes into account the benefits merely of BAA to consider the immateriality of experts and decision-makers as well as the complexity of the decision-theoretic environment to establish more effective and practical aggregate data. The calculation based on the MABAC technique is reliable, uses a straightforward equation and considers inactive values of losses and profits. The MABAC method's main advantage is that it may be used in conjunction with several other strategies. Due to these advantages and superiority, researchers are paying a lot of attention to the MABAC technique, which has been widely used in numerous MADM dilemmas. As a result, the MABAC technique provides a useful model for the outcomes of DM. Verma [44] deduced the technique of MABAC for FS. Zhao et al. [45] investigated the MABAC technique of IFS. Jiang et al. [46] studied MABAC for picture FS (PFS). Jana [47] deduced the technique of MABAC for bipolar fuzzy information. Some other methods proposed by other researchers for MADM/MCDM such as the ELECTRIC II method based on the cosine similarity measures (CSMs) in the setting of a double hierarchy hesitant fuzzy (HF) linguistic environment were developed by Zhang et al. [48]. An improved VIKOR method in the setting of a probabilistic double hierarchy linguistic term set was introduced by Gou et al. [49]. Also, for MCDM in the setting of HF linguistic entropy and cross-entropy measures were established by Gou et al. [50]. If we talk about the ORESTE method, Gou et al. [51] proposed medical health resource allocation evaluation in public health emergencies by an improved ORESTE method with linguistic preferences.
Aczel and Alsina [52] modified the algebraic t-norm and t-conorm by providing Aczel-Alsina (AA) t-norm and t-conorm which are more flexible as compared to other t-norms and t-conorms. AA t-norm and t-conorm have a significant influence because of the variableness of the parameters and have great applications in the theory of FS. Numerous scholars employed AA t-norm and t-conorm to interpret AA AOs in various modifications of FS such as Senapati et al. [53] investigated AA AOs for IFS, Senapati et al. [54] deduced AA AOs for interval-valued IFS, AA AOs for PFS were investigated by Senapati [55], Hussain et al. [56] interpreted AA AOs for spherical FS. AA-based AOs for intuitionistic hesitant FS environment and their application to the MADM process were proposed by Ali et al. [57]. Palanikumar et al. [58] proposed a Fermatean normal different AOs framework for the robot sensors process. Some average AOs based on spherical fuzzy soft sets were proposed by Ahmmad et al. [59].
The point-wise motivation and contribution of the developed study are given by
1) As we know, BCFS is a more generalized, efficient, loyal and dominant version of information than FS, BFS and CFS.
2) There is less and a rare chance of losing the information in BCFS as compared to the prevailing sets and also data analysts and experts feel comfortable making better decisions in the environment of a BCF setting.
3) AA t-norm and t-conorm have a significant influence because of the variableness of the parameters and have great applications in the theory of FS.
4) Moreover, there is no research on AA geometric AOs in the setting of BCFS. So, based on the valuable feature of both BCFS and AA operations, we study geometric AOs by employing AA operations to diagnose AOs such as BCFAAWG, BCFAAOWG and BCFAAHA operators.
5) The calculation based on the MABAC technique is reliable, uses a straightforward equation and considers inactive values of losses and profits.
6) Moreover, there is no investigation of the MABAC technique for BCFNs in the prevailing literature to overcome MAGDM difficulties. Thus, we also interpret the MABAC technique in the setting of BCFNs. We solve a MAGDM numerical example associated with the OS of a computer and find the finest OS for a computer through the interpreted MABAC technique.
The remaining script is constructed as
● In Section 2, we study t-norm and t-conorm, AA t-norm and t-conorm, BCFS and associated definitions, AA operations and AA averaging operator for BCFS.
● In Section 3, we deduce the BCFAAWG, BCFAAOWG and BCFAAHA operators in the setting of BCFS.
● In Section 4, we create a technique of MABAC based on the investigated operators for BCF information and examine a numerical example related to the OS of a computer.
● In Section 5, the comparison of the proposed work with prevailing theories is discussed to reveal the dominance and benefits of the initiated work.
● Section 6 contains the concluding remarks.
● The pictorial representation of the introduced work is given in Figure 1.
To discuss the application given in Section 4.1, we need some mathematical tools and we will first discuss the basic notions that we will have to use to develop these tools. Here, we study AA t-norm and t-conorm, BCFS and associated definition and AA operations and AA averaging operator for BCFS.
The AA t-norm and t-conorm invented by Aczél and Alsina [52] in 1982 is demonstrated in the next definition.
Definition 1. [52] AA t-norm and t-conorm are deduced as
ΘÅE(b1,b2)={ΘÅ(b1,b2)if ℇ=0min(b1,b2)if ℇ=∞e−((−logb1)ℇ+(−logb2)ℇ)1ℇ,otherwise | (1) |
XÅE(b1,b2)={XÅ(b1,b2)if ℇ=0max(b1,b2)if ℇ=∞1−e−((−log(1−b1))ℇ+(−log(1−b2))ℇ)1ℇ,otherwise, | (2) |
where ℇ∈[0,∞].
Definition 2. [40] The theory of BCFS is deduced as
⊮={(o,β+(o),γ−(o))|o∈V} |
={(o,ζ+(o)+ις+(o),ζ−(o)+ις−(o))|o∈⋓}, | (3) |
where ⋓ is the universal set, β+(o)=ζ+(o)+ις+(o) represents the positive degree of support (PDS) and γ−(o)=ζ−(o)+ις−(o) represents the negative degree of support (NDS) along ζ+(o),ς+(o)∈[0,1] and ζ−(o),ς−(o)∈[−1,0]. The format ⊮={(ζ++ις+,ζ−+ις−)} represents the BCFNs, which will be applied to all manuscripts.
In the next two definitions, we review the definition of score and accuracy values for BCFN.
Definition 3. [41] The score function's value is described as follows:
ℷSF(⊮)=14(2+ζ+(o)+ς+(o)+ζ−(o)+ς−(o)),ℷSF∈[0,1]. | (4) |
Definition 4. [41] The accuracy function's value is described as follows:
ℸAF(⊮)=ζ+(o)+ς+(o)−ζ−(o)−ς−(o)4,ℸAF(⊮)∈[0,1]. | (5) |
We presented certain properties for Eqs (4) and (5), such as ifℷSF(⊮1)<ℷSF(⊮2),then⊮1<⊮2;ifℷSF(⊮1)>ℷSF(⊮2), then⊮1>⊮2;ifℷSF(⊮1)=ℷSF(⊮2),then⊮1=⊮2;ifℸAF(⊮1)<ℸAF(⊮2), then⊮1<⊮2;ifℸAF(⊮1)>ℸAF(⊮2),then⊮1>⊮2;ifℸAF(⊮1)=ℸAF(⊮2),then,⊮1=⊮2.
Definition 5. [41] For two BCFNs ⊮1=(ζ+1+ις+1,ζ−1+ις−1) and ⊮2=(ζ+2+ις+2,ζ−2+ις−2) along ∂>0 we have some basic binary operation of addition, multiplication, scalar multiplication and power for BCFNs which is stated as follows:
⊮1⨁⊮2=(ζ+1+ζ+2−+ι(ς+1+ς+2−ς+1ς+2)−(ζ−1ζ−2)+ι(−(ς−1ς−2))) | (6) |
⊮1⊗⊮2=(ζ+1ζ+2+ις+1ς+2,ζ−1+ζ−1ζ−2+ζ−2+ι(ς−1+ς−1ς−2+ς−2)) | (7) |
∂⊮1=(1−(1−ζ+1)∂+ι(1−(1−ς+1)∂,−|ζ−1|∂+ι(−|ς−1|∂))) | (8) |
⊮1∂=((ζ+1)∂+ι(ς+1)∂,−1+(1+ζ−1)∂+ι(−1+(1+ς−1)∂)). | (9) |
Underneath definition contains the operational laws for BCFNs based on AA t-norm and t-conorm invented by Mahmood et al. [42].
Definition 6. [42] For ⊮1=(ζ+1+ις+1,ζ−1+ις−1) and ⊮2=(ζ+2+ις+2,ζ−2+ις−2) along Ỿ≥1andk>0 respectively, the AA operations for BCFS are
⊮1⨁⊮2= |
(1−e−((−log(1−ζ+1))Ỿ+(−log(1−ζ+2))Ỿ)1Ỿ+ι(1−e−((−log(1−ς+1))Ỿ+(−log(1−ς+2))Ỿ)1Ỿ),−(e−((−log|ζ−1|)Ỿ+(−log|ζ−2|)Ỿ)1Ỿ)+ι(−(e−((−log|ς−1|)Ỿ+(−log|ς−2|)Ỿ)1Ỿ))) | (10) |
⊮1⊗⊮2= |
(e−((−log(ζ+1))Ỿ+(−log(ζ+2))Ỿ)1Ỿ+ι(e−((−log(ς+1))Ỿ+(−log(ς+2))Ỿ)1Ỿ)−1+e−((−log(1+ζ−1))Ỿ+(−log(1+ζ−2))Ỿ)1Ỿ+ι(−1+e−((−log(1+ς−1))Ỿ+(−log(1+ς−2))Ỿ)1Ỿ)) | (11) |
k⊮1=(1−e−(k(−log(1−ζ+1))Ỿ)1Ỿ+ι(1−e−(k(−log(1−ς+1))Ỿ)1Ỿ),−(e−(k(−log|ζ−1|)Ỿ)1Ỿ)+ι(−(e−(k(−log|ς−1|)Ỿ)1Ỿ))) | (12) |
(⊮1)k1=(e−(k1(−logζ+1)Ỿ)1Ỿ+ι(e−(k1(−logς+1)Ỿ)1Ỿ,(−1+e−(k1(−log(1+ζ−1))Ỿ)1Ỿ)+i(−1+(e−(k1(−log(1+ς−1))Ỿ)1Ỿ)). | (13) |
Underneath definition contains the BCF AA weighted averaging (BCFAAWA) operator for BCFNs invented by Mahmood et al. [42].
Definition 7. [42] For a set ⊮ρ=(ζ+ρ+ις+ρ,ζ−ρ+ις−ρ),ρ=(1,2,3,……..,n) of BCFNs, the BCFAAWA operator is gathered as
BCFAAWA(⊮1,⊮2,⊮3,……,⊮n)=⨁nρ=1(kρ⊮ρ)=k1⊮1⨁k2⊮2⨁…⨁kn⊮n |
=(1−e−(∑nρ=1kρ(−log(1−ζ+1))Ỿ)1Ỿ+ι(1−e−(∑nρ=1kρ(−log(1−ς+1))Ỿ)1Ỿ),−(e−(∑nρ=1kρ(−log|ζ−1|)Ỿ)1Ỿ)+ι(−(e−(∑nρ=1kρ(−log|ς−1|)Ỿ)1Ỿ))), | (14) |
where k = (k1,k2,k3,………,kn) is a weight vector with kjϵ[0,1] and ∑nρ=1kj=1.
By using AA operational laws and BCF data, the primary goal of this study is to identify the concept of the BCFAAWG, BCFAAOWG and BCFAAHA operators.
Definition 8. For a set ⊮ρ=(ζ+ρ+ις+ρ,ζ−ρ+ις−ρ),ρ=(1,2,3,……..,n) of BCFNs, the BCFAAWG operator is gathered as
BCFAAWG(⊮1,⊮2,⊮3,…….,⊮n)=⊗nρ=1=(⊮1)k1⊗(⊮2)k2⊗……⋯⊗(⊮n)kn, | (15) |
where k = (k1,k2,k3,………,kn) is a weight vector with kjϵ[0,1] and ∑nρ=1kj=1.
Theorem 1. For a set ⊮ρ=(ζ+ρ+ις+ρ,ζ−ρ+ις−ρ),ρ=(1,2,3,……..,n) of BCFNs, Eq (15) will again interpret a BCFN and
BCFAAWG(⊮1,⊮2,⊮3,…………,⊮n)= |
(e−(∑nρ=1kρ(−logζ+ρ)Ỿ)1Ỿ+ι(e−(∑nρ=1kρ(−logς+ρ)Ỿ)1Ỿ),(−1+e−(∑nρ=1kρ(−log(1+ζ−ρ))Ỿ)1Ỿ)+ι(−1+(e−(∑nρ=1kρ(−log(1+ς−ρ))Ỿ)1Ỿ))). | (16) |
Proof. For proof see Appendix A.
Theorem 2. (Idempotency) For a set ⊮ρ=(ζ+ρ+ις+ρ,ζ−ρ+ις−ρ),ρ=(1,2,3,……..,n) of BCFNs, if ⊮=⊮ρ∀ρ, then
BCFAAWG(⊮1,⊮2,⊮3,…………,⊮n)=⊮. |
Proof. For proof see Appendix A.
Theorem 3. (Monotonicity) For two sets ⊮ρ=(ζ+ρ+ις+ρ,ζ−ρ+ις−ρ) and ⊮∼ρ=(ζ+∼ρ+ις+∼ρ,ζ−∼ρ+ις−∼ρ),ρ=(1,2,3,……..,n) of BCFNs, if ⊮ρ≤⊮∼ρ∀ρ i.e., ζ+ρ≤ζ+ρ∼,ς+ρ≤ς+ρ∼,ζ−ρ≤ζ−ρ∼,ς−ρ≤ς−ρ∼, then
BCFAAWG(⊮1,⊮2,⊮3,…………,⊮n)≤BCFAAWG(⊮∼1,⊮∼2,⊮∼3,…………,⊮∼n). |
Proof. For proof see Appendix A.
Theorem 4. (Boundedness) For a set ⊮ρ=(ζ+ρ+ις+ρ,ζ−ρ+ις−ρ),ρ=(1,2,3,……..,n) of BCFNs, when
⊮−=(minρ{ζ+ρ}+ιminρ{ς+ρ},maxρ{ζ−ρ}+ιmaxρ{ς−ρ}) and ⊮+=(maxρ{ζ+ρ}+ιmaxρ{ς+ρ},minρ{ζ−ρ}+ιminρ{ς−ρ}),
then
⊮−≤BCFAAWG(⊮1,⊮2,⊮3,…………,⊮n)≤⊮+. |
Proof. For proof see Appendix A.
Definition 9. For a set ⊮ρ=(ζ+ρ+ις+ρ,ζ−ρ+ις−ρ),ρ=(1,2,3,……..,n) of BCFNs, the BCFAAOWG operator is gathered as
BCFAAOWG(⊮1,⊮2,⊮3,…………,⊮n)=⊗nρ=1(⊮(ρ))kρ=(⊮⊚(1))k1⨂(⊮⊚(2))k2⨂…(⊮⊚(n))kn, | (17) |
where (⊚(1),⊚(2),⊚(3),…⋯⊚(n)) are the permutation of (ρ=1,2,3,……..,n) for which ⊮⊚(ρ−1)≥⊮⊚(ρ)∀ρ and k = (k1,k2,k3,………,kn) is a weight vector with kjϵ[0,1] and ∑nρ=1kj=1.
Theorem 5. For a set ⊮ρ=(ζ+ρ+ις+ρ,ζ−ρ+ις−ρ),ρ=(1,2,3,……..,n) of BCFNs, Eq (17) will again interpret a BCFN and
BCFAAOWG(⊮1,⊮2,⊮3,…………,⊮n)= |
(e−(∑nρ=1wj(−logζ+⊚(ρ))Ỿ)1Ỿ+ι(e−(∑nρ=1wρ(−logς+⊚(ρ))Ỿ)1Ỿ,(−1+e−(∑nρ=1wj(−log(1+ζ−⊚(ρ)))Ỿ)1Ỿ)+i(−1+(e−(∑nρ=1wj(−log(1+ς−⊚(ρ)))Ỿ)1Ỿ)). | (18) |
Theorem 6. (Idempotency) For a set ⊮ρ=(ζ+ρ+ις+ρ,ζ−ρ+ις−ρ),ρ=(1,2,3,……..,n) of BCFNs, if ⊮=⊮ρ∀ρ, then
BCFAAOWG(⊮1,⊮2,⊮3,…………,⊮n)=⊮. |
Theorem 7. (Monotonicity) For two sets ⊮ρ=(ζ+ρ+ις+ρ,ζ−ρ+ις−ρ) and ⊮∼ρ=(ζ+∼ρ+ις+∼ρ,ζ−∼ρ+ις−∼ρ),ρ=(1,2,3,……..,n) of BCFNs, if ⊮ρ≤⊮∼ρ∀ρ i.e., ζ+ρ≤ζ+ρ∼,ς+ρ≤ς+ρ∼,ζ−ρ≤ζ−ρ∼,ς−ρ≤ς−ρ∼, then
BCFAAOWG(⊮1,⊮2,⊮3,…………,⊮n)≤BCFAAOWG(⊮∼1,⊮∼2,⊮∼3,…………,⊮∼n). |
Theorem 8. (Boundedness) For a set ⊮ρ=(ζ+ρ+ις+ρ,ζ−ρ+ις−ρ),ρ=(1,2,3,……..,n) of BCFNs, when
⊮−=(minρ{ζ+ρ}+ιminρ{ς+ρ},maxρ{ζ−ρ}+ιmaxρ{ς−ρ}), and ⊮+=(maxρ{ζ+ρ}+ιmaxρ{ς+ρ},minρ{ζ−ρ}+ιminρ{ς−ρ}),
then
⊮−≤BCFAAOWG(⊮1,⊮2,⊮3,…………,⊮n)≤⊮+. |
Definition 10. For a set ⊮ρ=(ζ+ρ+ις+ρ,ζ−ρ+ις−ρ),ρ=(1,2,3,……..,n) of BCFNs, the BCFAAHG operator is gathered as
BCFAAHG(⊮1,⊮2,⊮3,…………,⊮n) = ⊗nρ=1(⊮°⊚(ρ))kρ=(⊮°⊚(1))k1⊗(⊮°⊚(2))k2……⋯⊗(⊮°⊚(n))kn, | (19) |
where ⊮°⊚(ρ)=ne⊮ρ, (ρ=1,2,3,……..,n), (⊮°⊚(1),⊮°⊚(2),……,⊮°⊚(n)) is any permutation of an arrangement of the weighted BCFNs (⊮°(1),⊮°(2),……,⊮°(n)) in a way such that ⊮°⊚(ρ−1)≥⊮°⊚(ρ)∀ρ, e=(e1,e2,e3,……,en)T of ⊮ρ(ρ=1,2,3,……..,n) along the condition eρϵ[0,1] and ∑nρ=1eρ=1 and n is the balancing coefficient. Furthermore, k = (k1,k2,k3,………,kn) is a weight vector with kjϵ[0,1] and ∑nρ=1kj=1.
Theorem 9. For a set ⊮ρ=(ζ+ρ+ις+ρ,ζ−ρ+ις−ρ),ρ=(1,2,3,……..,n) of BCFNs, Eq (19) will again interpret a BCFN and
BCFAAHG(⊮1,⊮2,⊮3,…………,⊮n)= |
(e−(∑nρ=1wj(−logζ+⊚(ρ))Ỿ)1Ỿ+ι(e−(∑nρ=1wρ(−logς+⊚(ρ))Ỿ)1Ỿ,(−1+e−(∑nρ=1wj(−log(1+ζ−⊚(ρ)))Ỿ)1Ỿ)+i(−1+(e−(∑nρ=1wj(−log(1+ς−⊚(ρ)))Ỿ)1Ỿ)). | (20) |
Theorem 10. (Idempotency) For a set ⊮ρ=(ζ+ρ+ις+ρ,ζ−ρ+ις−ρ),ρ=(1,2,3,……..,n) of BCFNs, if ⊮=⊮ρ∀ρ, then
BCFAAOWG(⊮1,⊮2,⊮3,…………,⊮n)=⊮. |
Theorem 11. (Monotonicity) For two sets ⊮ρ=(ζ+ρ+ις+ρ,ζ−ρ+ις−ρ) and ⊮∼ρ=(ζ+∼ρ+ις+∼ρ,ζ−∼ρ+ις−∼ρ),ρ=(1,2,3,……..,n) of BCFNs, if ⊮ρ≤⊮∼ρ∀ρ i.e., ζ+ρ≤ζ+ρ∼,ς+ρ≤ς+ρ∼,ζ−ρ≤ζ−ρ∼,ς−ρ≤ς−ρ∼, then
BCFAAOWG(⊮1,⊮2,⊮3,…………,⊮n)≤BCFAAOWG(⊮∼1,⊮∼2,⊮∼3,…………,⊮∼n). |
Theorem 12. (Boundedness) For a set ⊮ρ=(ζ+ρ+ις+ρ,ζ−ρ+ις−ρ),ρ=(1,2,3,……..,n) of BCFNs, when
⊮−=(minρ{ζ+ρ}+ιminρ{ς+ρ},maxρ{ζ−ρ}+ιmaxρ{ς−ρ}) and ⊮+=(maxρ{ζ+ρ}+ιmaxρ{ς+ρ},minρ{ζ−ρ}+ιminρ{ς−ρ}),
then
⊮−≤BCFAAOWG(⊮1,⊮2,⊮3,…………,⊮n)≤⊮+. |
Definition 11. Let ⊮1=(ζ+1+ις+1,ζ−1+ις−1) and ⊮2=(ζ+2+ις+2,ζ−2+ις−2) be any two BCFNs. Then, the BCF normalized Hamming distance (BCFNHD) is initiated as
d(⊮1,⊮2)=14(|ζ+1−ζ+2|+|ς+1−ς+2|+|ζ−1−ζ−2+|+|ς−1−ς−2|), | (21) |
where d(⊮1,⊮2)∈[0,1].
Suppose there is a set of κ alternative {⊮1,⊮2,⊮3,…………,⊮κ} and η attributes {C1,C2,C3,…………,Cη} with an associated set of the weight vector {k1,k2,k3,………,kη} and ξ experts {P1,P2,P3,…………,Pξ} with associated weighted {e1,e2,e3,…………,eξ}. Then, BCF evaluation matrix M=[⊮ξρτ]κ×η=(ζ+ξρτ+ις+ξρτ,ζ−ξρτ+ις−ξρτ)κ×η,ρ=1,2,……,κ,τ=1,2,……,η,ζ+ξρ,ς+ρξ∈[0,1]andζ−ρξ,ς−ρξ∈[−1,0] Then, the BCF MABAC approach has the following steps:
Step 1: Analyzing the formulation of a BCF matrix M=[⊮ξρτ]κ×η=(ζ+ξρτ+ις+ξρτ,ζ−ξρτ+ις−ξρτ)κ×η,ρ=1,2,……,κ,τ=1,2,……,η given as
M=[⊮ξρτ]κ×η=[(ζ+ξ11+ις+ξ11,ζ+ξ11+ις+ξ11)(ζ+ξ12+ις+ξ12,ζ+ξ12+ις+ξ12)⋯(ζ+ξ1η+ις+ξ1η,ζ+ξ1η+ις+ξ1η)(ζ+ξ21+ις+ξ21,ζ+ξ21+ις+ξ21)(ζ+ξ22+ις+ξ22,ζ+ξ22+ις+ξ22)…(ζ+ξ2η+ις+ξ2η,ζ+ξ2η+ις+ξ2η)⋮⋱⋮(ζ+ξκ1+ις+ξκ1,ζ+ξκ1+ις+ξκ1)(ζ+ξκ2+ις+ξκ2,ζ+ξκ2+ις+ξκ2)⋯(ζ+ξκη+ις+ξκη,ζ+ξκη+ις+ξκη)], |
where ⊮ξρτ=(ζ+ξρτ+ις+ξρτ,ζ−ξρτ+ις−ξρτ)κ×η,ρ=1,2,……,κ,τ=1,2,……,η presented a formula given by the expert eξ and it is based on the attributes Cτ for BCF information.
Step 2: We may employ one of the developed operators that are BCFAAWG, BCFAAOWG or BCFAAHG operator to combine or merge the collected values of ⊮ξρτ to ⊮ρτ
M=[⊮ρτ]κ×η=[(ζ+11+ις+11,ζ+11+ις+11)(ζ+12+ις+12,ζ+12+ις+12)⋯(ζ+1η+ις+1η,ζ+1η+ις+1η)(ζ+21+ις+21,ζ+21+ις+21)(ζ+22+ις+22,ζ+22+ις+22)…(ζ+2η+ις+2η,ζ+2η+ις+2η)⋮⋱⋮(ζ+κ1+ις+κ1,ζ+κ1+ις+κ1)(ζ+κ2+ις+κ2,ζ+κ2+ις+κ2)⋯(ζ+κη+ις+κη,ζ+κη+ις+κη)]. |
Where ⊮ρτ=(ζ+ξρτ+ις+ξρτ,ζ−ξρτ+ις−ξρτ)κ×η,ρ=1,2,……,κ,τ=1,2,……,η presented a formula given by the expert eξ and it is based on the attributes Cτ for BCF information.
Step 3: In Step 3, we standardized the merge resultant matrix M=[⊮ρτ]κ×η, ρ=1,2,……,κ,τ=1,2,……,η based on the nature of each character using the following formula:
For benefit attributes:
M=⊮ρτ=(ζ+ρτ+ις+ρτ,ζ−ρτ+ις−ρτ) |
ρ=1,2,……,κ,τ=1,2,……,η. |
For cost attributes:
M=(⊮ρτ)c=(1−ζ+ρτ+ι(1−ς+ρτ),−1−ζ−ρτ+ι(−1−ς−ρτ)) |
ρ=1,2,……,κ,τ=1,2,……,η. |
Step 4: Now for standardized matrix =M=(⊮ρτ)c=((ζ+ρ+ις+ρ),(ζ−ρ+ις−ρ))(ρ=1,2,……,κ,τ=1,2,……,η) and the weight of attributes kη,(τ=1,2,……,η), compute standardized BCF weighting matrix wMρτ=((ζ+ρ+ις+ρ)∘,(ζ−ρ+ις−ρ)∘), ρ=1,2,……,κ,τ=1,2,……,η by the following formula:
wMρτ=kη⨁Mρτ,ρ=1,2,……,κ,τ=1,2,……,η |
=(1−e−(∑ητ=1kτ(−log(1−ζ+ρτ))Ỿ)1Ỿ+ι(1−e−(∑ητ=1kτ(−log(1−ς+ρτ))Ỿ)1Ỿ),−(e−(∑ητ=1kτ(−log|ζ−ρτ|)Ỿ)1Ỿ)+ι(−(e−(∑ητ=1kτ(−log|ς−ρτ|)Ỿ)1Ỿ))). | (22) |
Step 5: The BAA values are evaluated in Step 5, the BAA matrix T=[tτ]1×η maybe obtained as;
tτ=(κ∑ρ=1Mρτ)1κ,ρ=1,2,……,κ,τ=1,2,……,η |
=(e−(∑κρ=11κ(−log(ζ+ρτ))Ỿ)1Ỿ+ι(e−(∑κρ=11κ(−log(ς+ρτ))Ỿ)1Ỿ),(−1+e−(∑ηρ=11κ(−log(1+ζ−ρτ))Ỿ)1Ỿ)+ι(−1+(e−(∑ηρ=11κ(−log(1+ς−ρτ))Ỿ)1Ỿ))). | (23) |
Step 6: The distance O=[dρτ]κ×η between each alternative and BAA, as determined by the following equation, is evaluated in Step 6:
dρτ={d(wMρτ,tτ),ifwMρτ>tτ0,ifwMρτ=tτ−d(wMρτ,tτ),ifwMρτ<tτ}, |
where d(wMρτ,tτ) is the mean distance between wMρτ and tτ.
Step 7: Finally, using the following formula we add the values of each alternative's dρτ:
Sρ=∑ητ=1dρτ. |
To better illustrate the above algorithm, we provided a flow chart for the process of the MABAC method which is shown in Figure 2.
Application
Computers were invented to automate mathematical calculations that far exceeded that which even the brightest brains were capable of. Innovative applications for both new and old technology are created by computer and information scientists. Thus, computer assistance is needed for data entry, editing and administration, including follow-up activities, etc. Regardless of whether it is being used for academic or commercial research, it is a crucial tool. The OS is the central element of a computer. The computer cannot be successfully used without an OS. An OS is software that controls all of the other applications. It manages the hardware and software, as well as the computer's memory and processes. Normally, several computer applications operate concurrently, requiring access to the computer's CPU, memory and storage. OSs are crucial to the computer processing system, just as computers are essential in every aspect of life. Computer applications and software development benefit greatly from the OS. Each application would need to have both its user interface and the vast code necessary to govern all low-level computer processes in the absence of an OS. Because of this, it takes substantially less effort and code to create and debug an application while still enabling users to control, modify and manage the system hardware through an intuitive interface. The many OSs that are utilized in computer processing systems will be covered in this section. Some of these operating systems are discussed as follows:
1) Single-user single-tasking OS (SU-OS)
SU-OS refers to an OS that only permits a single user to accomplish one job at a time. This approach allows each user to execute a single application at a time. This OS is created for usage with personal computers or home computers. It permits one user at a time to have access to your personal computer. Certain tasks, such as printing paper, downloading photographs, using MS Word or Palm OS and playing movies, can be completed in a single frame.
2) Multiprocessing operating system (M-Pro OS)
The M-Pro OS to a single computer system that employs two or more CPUs. This OS refers to a system's capacity to accommodate several processors or to divide duties among them. M-Pro is the term used to describe processing carried out by two or more computers or processors connected in parallel. It enables several computers to run two or more applications simultaneously.
3) Multi-programming operating system (M-Prog OS)
An OS that supports many programs running simultaneously on a single processor unit is known as M-Prog OS. It offers the ability to switch between processes and can enable more than one program to be prepared for execution. The idea of M-Prog depends on a computer's capacity to store the program for future use. Several programs are stored in the memory during M-prog.
4) Multi-tasking operating system (M-Tas OS)
An OS known as M-Tas OS enables a user to carry out many computer tasks simultaneously, including running application software. This OS can move between tasks without losing data while keeping track of where you are in multiple processes. M-Tas is the process of running two or more applications simultaneously by one user on the same computer while using the same CPU.
By utilizing the MABAC method based on BCFAAWG operators in the scenario of BCFS to tackle MAGDM difficulties, the aforementioned application is resolved. The various OS types in the following numerical examples are viewed as alternatives.
Numerical example
Say an IT Organization wants to evaluate the performance of four OS kinds to get the best and better OS. These 4 OS are underneath
⊮1: SU-OS
⊮2: M-Pro OS
⊮3: M-Prog OS
⊮4: M-Tas OS.
Which would be evaluated by the underneath attributes
C1: Licensing
C2: Software compatibility
C3: Complexity
C4: Resource management.
For this evaluation, the IT organization hire a team of three experts Pξ(ξ=1,2,3) and the weight of these experts is (0.4,0.35,0.25). Further, the weight of the attributes of these 4 OS is (0.4,0.3,0.2,0.1). The evaluated values of OSs are in the model of BCFN.
Step 1: The BCF matrices of every expert is revealed in Tables 1–3.
C1 | C2 | C3 | C4 | |
⊮1 | (0.19+ι0.45−0.69−ι0.51) | (0.45+ι0.59−0.89−ι0.90) | (0.23+ι0.49−0.39−ι0.57) | (0.76+ι0.90−0.69−ι0.51) |
⊮2 | (0.13+ι0.49−0.89−ι0.56) | (0.45+ι0.54−0.60−ι0.15) | (0.04+ι0.50−0.99−ι0.88) | (0.11+ι0.15−0.79−ι0.71) |
⊮3 | (0.21+ι0.42−0.83−ι0.67) | (0.17+ι0.49−0.69−ι0.71) | (0.17+ι0.09−0.58−ι0.21) | (0.33+ι0.46−0.29−ι0.59) |
⊮4 | (0.35+ι0.44−0.67−ι0.59) | (0.28+ι0.55−0.42−ι0.58) | (0.36+ι0.48−0.89−ι0.66) | (0.88+ι0.12−0.29−ι0.31) |
C1 | C2 | C3 | C4 | |
⊮1 | (0.34+ι0.460.88−ι0.01) | (0.67+ι0.09−0.06−ι0.98) | (0.22+ι0.41−0.32−ι0.53) | (0.15+ι0.91−0.65−ι0.52) |
⊮2 | (0.23+ι0.79−0.49−ι0.06) | (0.78+ι0.24−0.20−ι0.55) | (0.23+ι0.54−0.89−ι0.38) | (0.17+ι0.65−0.89−ι0.51) |
⊮{\text{}}_{\bf{3}} | \left(\begin{array}{c}0.22+\iota 0.47\\ -0.55-\iota 0.31\end{array}\right) | \left(\begin{array}{c}0.14+\iota 0.20\\ -0.29-\iota 0.11\end{array}\right) | \left(\begin{array}{c}0.17+\iota 0.33\\ -0.58-\iota 0.24\end{array}\right) | \left(\begin{array}{c}0.20+\iota 0.49\\ -0.29-\iota 0.51\end{array}\right) |
⊮{\text{}}_{\bf{4}} | \left(\begin{array}{c}0.19+\iota 0.56\\ -0.60-\iota 0.90\end{array}\right) | \left(\begin{array}{c}0.28+\iota 0.25\\ -0.92-\iota 0.68\end{array}\right) | \left(\begin{array}{c}0.48+\iota 0.44\\ -0.63-\iota 0.66\end{array}\right) | \left(\begin{array}{c}0.88+\iota 0.18\\ -0.59-\iota 0.33\end{array}\right) |
\mathbb{C}{\text{}}_{\bf{1}} | \mathbb{C}{\text{}}_{\bf{2}} | \mathbb{C}{\text{}}_{\bf{3}} | \mathbb{C}{\text{}}_{\bf{4}} | |
⊮{\text{}}_{\bf{1}} | \left(\begin{array}{c}0.16+\iota 0.45\\ -0.79-\iota 0.51\end{array}\right) | \left(\begin{array}{c}0.12+\iota 0.19\\ -0.59-\iota 0.93\end{array}\right) | \left(\begin{array}{c}0.56+\iota 0.33\\ -0.39-\iota 0.59\end{array}\right) | \left(\begin{array}{c}0.60+\iota 0.70\\ -0.13-\iota 0.22\end{array}\right) |
⊮{\text{}}_{\bf{2}} | \left(\begin{array}{c}0.70+\iota 0.89\\ -0.89-\iota 0.26\end{array}\right) | \left(\begin{array}{c}0.45+\iota 0.30\\ -0.50-\iota 0.75\end{array}\right) | \left(\begin{array}{c}0.05+\iota 0.59\\ -0.94-\iota 0.32\end{array}\right) | \left(\begin{array}{c}0.15+\iota 0.61\\ -0.99-\iota 0.11\end{array}\right) |
⊮{\text{}}_{\bf{3}} | \left(\begin{array}{c}0.22+\iota 0.23\\ -0.85-\iota 0.27\end{array}\right) | \left(\begin{array}{c}0.74+\iota 0.69\\ -0.69-\iota 0.71\end{array}\right) | \left(\begin{array}{c}0.11+\iota 0.19\\ -0.58-\iota 0.71\end{array}\right) | \left(\begin{array}{c}0.66+\iota 0.46\\ -0.49-\iota 0.39\end{array}\right) |
⊮{\text{}}_{\bf{4}} | \left(\begin{array}{c}0.27+\iota 0.45\\ -0.77-\iota 0.54\end{array}\right) | \left(\begin{array}{c}0.78+\iota 0.15\\ -0.22-\iota 0.59\end{array}\right) | \left(\begin{array}{c}0.36+\iota 0.83\\ -0.77-\iota 0.90\end{array}\right) | \left(\begin{array}{c}0.81+\iota 0.37\\ -0.69-\iota 0.71\end{array}\right) |
Step 2: In Step 2, we aggregate the values of {\mathfrak{M}}_{1}, {\mathfrak{M}}_{2}, {\mathfrak{M}}_{3} to \mathfrak{M} by using the BCFAAWG operator i.e., we obtain {⊮}_{\rho \tau }^{\xi } to {⊮}_{\rho \tau } . Table 4 revealed the aggregated matrix.
Alternatives/attributes | \mathbb{C}{\text{}}_{\bf{1}} | \mathbb{C}{\text{}}_{\bf{2}} | \mathbb{C}{\text{}}_{\bf{3}} | \mathbb{C}{\text{}}_{\bf{4}} |
⊮{\text{}}_{\bf{1}} | \left(\begin{array}{c}0.8+\iota 0.94\\ -0.23-\iota 0.03\end{array}\right) | \left(\begin{array}{c}0.87+\iota 0.77\\ -0.18-\iota 0.58\end{array}\right) | \left(\begin{array}{c}0.85+\iota 0.93\\ -0.02-\iota 0.06\end{array}\right) | \left(\begin{array}{c}0.88+\iota 0.99\\ -0.08-\iota 0.04\end{array}\right) |
⊮{\text{}}_{\bf{2}} | \left(\begin{array}{c}0.79+\iota 0.98\\ -0.27-\iota 0.03\end{array}\right) | \left(\begin{array}{c}0.96+\iota 0.89\\ -0.04-\iota 0.07\end{array}\right) | \left(\begin{array}{c}0.51+\iota 0.96\\ -0.68-\iota 0.17\end{array}\right) | \left(\begin{array}{c}0.69+\iota 0.86\\ -0.53-\iota 0.07\end{array}\right) |
⊮{\text{}}_{\bf{3}} | \left(\begin{array}{c}0.08+\iota 0.91\\ -0.20-\iota 0.05\end{array}\right) | \left(\begin{array}{c}0.78+\iota 0.90\\ -0.08-\iota 0.09\end{array}\right) | \left(\begin{array}{c}0.71+\iota 0.72\\ -0.07-\iota 0.04\end{array}\right) | \left(\begin{array}{c}0.87+\iota 0.95\\ -0.02-\iota 0.05\end{array}\right) |
⊮{\text{}}_{\bf{4}} | \left(\begin{array}{c}0.84+\iota 0.95\\ -0.12-\iota 0.20\end{array}\right) | \left(\begin{array}{c}0.89+\iota 0.85\\ -0.20-\iota 0.09\end{array}\right) | \left(\begin{array}{c}0.92+\iota 0.96\\ -0.23-\iota 0.19\end{array}\right) | \left(\begin{array}{c}0.99+\iota 0.75\\ -0.06-\iota 0.05\end{array}\right) |
Step 3: As the data given in matrix \mathfrak{M} = {\left[{\mathfrak{M}}_{\rho \tau }\right]}_{\kappa \times \eta }, \rho = \mathrm{1, 2}, \dots \dots, \kappa \tau = \mathrm{1, 2}, \dots \dots, \eta is of benefit attributes. So, the standardized matrix would be the same as given in Table 4.
Step 4: Now, using Eq (22), we calculate a standardized BCF weighting matrix for standardized matrix \mathfrak{M} = {\left[{\mathfrak{M}}_{\rho \tau }\right]}_{\kappa \times \eta }, \left(\rho = \mathrm{1, 2}, \dots \dots, \kappa, \tau = \mathrm{1, 2}, \dots \dots, \eta \right) and attribute's weight {\mathfrak{k}}_{\tau }, \left(\tau = \mathrm{1, 2}, \dots \dots, \eta \right), which is interpreted in Table 5.
Alternatives/attributes | \mathbb{C}{\text{}}_{\bf{1}} | \mathbb{C}{\text{}}_{\bf{2}} | \mathbb{C}{\text{}}_{\bf{3}} | \mathbb{C}{\text{}}_{\bf{4}} |
⊮{\text{}}_{\bf{1}} | \left(\begin{array}{c}0.09+\iota 0.26\\ -0.92-\iota 0.63\end{array}\right) | \left(\begin{array}{c}0.11+\iota 0.06\\ -0.92-\iota 0.99\end{array}\right) | \left(\begin{array}{c}0.07+\iota 0.12\\ -0.75-\iota 0.86\end{array}\right) | \left(\begin{array}{c}0.04+\iota 0.18\\ -0.94-\iota 0.91\end{array}\right) |
⊮{\text{}}_{\bf{2}} | \left(\begin{array}{c}0.09+\iota 0.44\\ -0.94-\iota 0.63\end{array}\right) | \left(\begin{array}{c}0.25+\iota 0.13\\ -0.75-\iota 0.82\end{array}\right) | \left(\begin{array}{c}0.01+\iota 0.18\\ -0.99-\iota 0.94\end{array}\right) | \left(\begin{array}{c}0.01+\iota 0.04\\ -0.99-\iota 0.94\end{array}\right) |
⊮{\text{}}_{\bf{3}} | \left(\begin{array}{c}0.09+\iota 0.02\\ -0.91-\iota 0.71\end{array}\right) | \left(\begin{array}{c}0.06+\iota 0.14\\ -0.83-\iota 0.85\end{array}\right) | \left(\begin{array}{c}0.03+\iota 0.03\\ -0.88-\iota 0.82\end{array}\right) | \left(\begin{array}{c}0.04+\iota 0.08\\ -0.87-\iota 0.92\end{array}\right) |
⊮{\text{}}_{\bf{4}} | \left(\begin{array}{c}0.12+\iota 0.29\\ -0.84-\iota 0.91\end{array}\right) | \left(\begin{array}{c}0.13+\iota 0.10\\ -0.93-\iota 0.85\end{array}\right) | \left(\begin{array}{c}0.11+\iota 0.18\\ -0.96-\iota 0.95\end{array}\right) | \left(\begin{array}{c}0.18+\iota 0.02\\ -0.93-\iota 0.92\end{array}\right) |
Step 5: We assess the BAA values. The BAA matrix \mathbb{T} = {\left[{\mathfrak{t}}_{\tau }\right]}_{1\times \eta } may be obtained using Eq (23).
{\mathfrak{t}}_{1} = \left(0.66+\iota 0.88, -0.36-\iota 0.16\right), {\mathfrak{t}}_{2} = \left(0.70+\iota 0.67, -0.29-\iota 0.45\right), {\mathfrak{t}}_{3} = \left(0.42+ \\ \iota 0.65, -0.51-\iota 0.36\right), {\mathfrak{t}}_{4} = \left(0.41+\iota 0.52, -0.53-\iota 0.39\right) . |
Step 6: We evaluate the distance \mathfrak{O} = {\left[{\mathcal{d}}_{\rho \tau }\right]}_{\kappa \times \eta } between each OS and BAA and the outcome is displayed in Table 6.
Alternatives | \mathbb{C}{\text{}}_{\bf{1}} | \mathbb{C}{\text{}}_{\bf{2}} | \mathbb{C}{\text{}}_{\bf{3}} | \mathbb{C}{\text{}}_{\bf{4}} |
⊮{\text{}}_{\bf{1}} | −0.60 | −0.60 | −0.40 | −0.41 |
⊮{\text{}}_{\bf{2}} | −0.50 | −0.50 | −0.50 | −0.47 |
⊮{\text{}}_{\bf{3}} | −0.60 | −0.50 | −0.50 | −0.42 |
⊮{\text{}}_{\bf{4}} | −0.60 | −0.50 | −0.50 | −0.43 |
Step 7: In this step, we have
{\mathcal{S}}_{1} = \left(-0.60\right)+\left(-0.60\right)+\left(-0.40\right)+\left(-0.41\right) = -2.01 |
{\mathcal{S}}_{2} = -1.97, {\mathcal{S}}_{3} = -2.02, {\mathcal{S}}_{4} = -2.03 . |
The following order list is derived from the aforementioned evaluation to help us choose the best decision:
⊮{\text{}}_{2}\succ ⊮{\text{}}_{1}\succ ⊮{\text{}}_{3}\succ ⊮{\text{}}_{4} .. |
Because ⊮{\text{}}_{2} has a value larger than all other alternatives. Hence, ⊮{\text{}}_{2} is the best and better OS.
First, we compared our considered work with several prevalent studies, including those by Wei et al. [19], Jana et al. [20], Jana et al. [33], Riaz and Tehrim. [34], Bi et al. [38] and Bi et al. [39], to ascertain the influence and supremacy of the proposed work. Using the information in Table 4 as a starting point, Table 7 compares the diagnostic work with the existing work.
Methods | {\mathit{\gimel }}_{\mathbb{S}\mathbb{F}}\left(⊮{\text{}}_{\bf{1}}\right) | {\mathit{\gimel }}_{\mathbb{S}\mathbb{F}}\left(⊮{\text{}}_{\bf{2}}\right) | {\mathit{\gimel }}_{\mathbb{S}\mathbb{F}}\left(⊮{\text{}}_{\bf{3}}\right) | {\mathit{\gimel }}_{\mathbb{S}\mathbb{F}}\left(⊮{\text{}}_{\bf{4}}\right) | Ranking |
Wei et al. [19] method | ⤰\rightsquigarrow ⤰\rightsquigarrow ⤰ | ⤰\rightsquigarrow ⤰\rightsquigarrow ⤰ | ⤰\rightsquigarrow ⤰\rightsquigarrow ⤰ | ⤰\rightsquigarrow ⤰\rightsquigarrow ⤰ | ⤰\rightsquigarrow ⤰\rightsquigarrow ⤰ |
Jana et al. [20] method | ⤰\rightsquigarrow ⤰\rightsquigarrow ⤰ | ⤰\rightsquigarrow ⤰\rightsquigarrow ⤰ | ⤰\rightsquigarrow ⤰\rightsquigarrow ⤰ | ⤰\rightsquigarrow ⤰\rightsquigarrow ⤰ | ⤰\rightsquigarrow ⤰\rightsquigarrow ⤰ |
Jana et al. [33] method | ⤰\rightsquigarrow ⤰\rightsquigarrow ⤰ | ⤰\rightsquigarrow ⤰\rightsquigarrow ⤰ | ⤰\rightsquigarrow ⤰\rightsquigarrow ⤰ | ⤰\rightsquigarrow ⤰\rightsquigarrow ⤰ | ⤰\rightsquigarrow ⤰\rightsquigarrow ⤰ |
Riaz and Tehrim. [34] | ⤰\rightsquigarrow ⤰\rightsquigarrow ⤰ | ⤰\rightsquigarrow ⤰\rightsquigarrow ⤰ | ⤰\rightsquigarrow ⤰\rightsquigarrow ⤰ | ⤰\rightsquigarrow ⤰\rightsquigarrow ⤰ | ⤰\rightsquigarrow ⤰\rightsquigarrow ⤰ |
Bi et al. [38] method | ⤰\rightsquigarrow ⤰\rightsquigarrow ⤰ | ⤰\rightsquigarrow ⤰\rightsquigarrow ⤰ | ⤰\rightsquigarrow ⤰\rightsquigarrow ⤰ | ⤰\rightsquigarrow ⤰\rightsquigarrow ⤰ | ⤰\rightsquigarrow ⤰\rightsquigarrow ⤰ |
Bi et al. [39] method | ⤰\rightsquigarrow ⤰\rightsquigarrow ⤰ | ⤰\rightsquigarrow ⤰\rightsquigarrow ⤰ | ⤰\rightsquigarrow ⤰\rightsquigarrow ⤰ | ⤰\rightsquigarrow ⤰\rightsquigarrow ⤰ | ⤰\rightsquigarrow ⤰\rightsquigarrow ⤰ |
BCFAAWG | 0.985 | 0.977 | 0.994 | 0.995 | ⊮{\text{}}_{4}\succ ⊮{\text{}}_{3}\succ ⊮{\text{}}_{1}\succ ⊮{\text{}}_{2} |
Table 7's data demonstrate existing theories, such as those by Wei et al. [19], Jana et al. [20], Jana et al. [33] Riaz and Tehrim [34], Bi et al. [38] and Bi et al. [39], were unable to handle the types of data given in Table 4 because they all had flaws. The point-wise discussion is given by
1) We start with Wei et al. [19]. We noticed that the Wei et al. [19] method although covering the positive and negative aspects but the data given in Table 4 has positive and negative aspects along with 2nd dimension. Thus, the theory invented by Wei et al. [19] can't cope with such data. Merely, the invented work can cope with such information. So, we can see that our proposed method can cover all information in Table 4, so the developed approach is the more advanced method.
2) We can observe that Jana et al. [20] identified Dombi AOs operators and techniques of DM for BFS which deal with data that includes both positive and negative evaluations of people in one dimension. However, Table 4's data is two dimensional, making it impossible for the efforts of Jana et al. [20] to overcome this type of data. This means that the developed notion is more advanced because it can cover more advanced data.
3) Notice that the developments made by Jana et al. [33] can only consider the bipolar fuzzy information, while the proposed data consist of bipolar complex fuzzy information. So, no result was found in this case because bipolar complex fuzzy cannot be covered by Jana et al. [35] method.
4) For Riaz and Tehrim [34], the structure is more general and based on cubic bipolar fuzzy information, but this method is not capable of considering the bipolar complex fuzzy information that is two-dimensional data.
5) Bi et al. [38] and Bi et al. [39] have identified geometric and arithmetic AOs respectively for CF set to deal with two-dimensional data, but these AOs are unable to reflect the positive and negative aspects of human opinion. They were unable to resolve the data in Table 4. But our proposed work does not fail in any condition and handles two-dimensional data with positive and negative aspects. First of all, we aggregated the data given in Table 4 using the BCFAAWG operator. After aggregating the different values given in Table 4, we used score and accuracy functions to rank different values. After ranking, we see that the value of ⊮{\text{}}_{4} is greater than all the other alternative values and hence we get our optimal alternative which is ⊮{\text{}}_{4} . That is why our proposed work is superior to all of the existing work and identifies the best alternative which is the ⊮{\text{}}_{4} , demonstrating the flexibility of the proposed AOs. The proposed AOs are quite skilled compared to the existing operators. As a consequence, the suggested work is superior to the earlier attempts in terms of success and dominance.
Moreover, the graphical representation of the developed theory is given in Figure 3.
If we consider the data given in numerical Example 4.2, we now compared our considered work along several other prevalent works i.e., our BCF MABAC approach will now be compared to the fuzzy MABAC method and BF MABAC method, which was proposed by Varma [44] and Jana [47] respectively. Using the data in numerical Example 4.2 as a starting point, Table 8 compares the diagnosed work with the existing work.
Sources | Method | The distance between alternative and BAA added together | Ranking |
Varma [44] | Fuzzy MABAC | ⤰\rightsquigarrow ⤰\rightsquigarrow ⤰ | ⤰\rightsquigarrow ⤰\rightsquigarrow ⤰ |
Jana [47] | BF MABAC | ⤰\rightsquigarrow ⤰\rightsquigarrow ⤰ | ⤰\rightsquigarrow ⤰\rightsquigarrow ⤰ |
Invented work | BCF MABAC | {\mathcal{S}}_{1}=-2.01, {\mathcal{S}}_{2}=-1.97 {\mathcal{S}}_{3}=-2.02, {\mathcal{S}}_{4}=-2.03 |
⊮{\text{}}_{2}\succ ⊮{\text{}}_{1}\succ ⊮{\text{}}_{3}\succ ⊮{\text{}}_{4} |
Moreover, a graphical depiction of the data given in Table 8 is given in Figure 4.
According to the data in numerical Example 4.2, the dominant theories, such as Varma [44] and Jana [42] were unable to explain this type of data because each of them had flaws. Varma [44] diagnosed the fuzzy MABAC approach that deals with information comprising simply a positive degree of support. The known work of Varma [44] cannot resolve the data provided in numerical Example 4.2. It is because the fuzzy MABAC technique does not deal with the information that includes positive and negative degrees of support nor does it deal with two-dimensional information, and the data in numerical Example 4.2 is two-dimensional with positive and negative degrees of support. Similarly, the data in numerical Example 4.2 is two dimensional and the Jana [47] diagnostic BFMABAC approach works with data that incorporates both positive and negative human viewpoints in one dimension. Therefore, the diagnostic work of Jana [47] cannot resolve such data. The existing practices only cover a tiny amount of information and are comparatively out-of-date, ineffective and simple. In light of this, the proposed work differs from previous work in the sense that it is novel, more potent, more dominating, covers more regions and tackles a wide range of difficult real-world problems.
An operating system is a piece of software that controls a computer's hardware and software resources and offers standard services to other software applications. It serves as an interface between the hardware and software of the computer, facilitating communication between programs and the computer. As AOs are the fundamental tools that convert the overall information into a single value that can help with DM problems. So, based on the notion of BCFSs and Aczel-Alsina t-norm and t-conorm, we have developed the notion of geometric AOs like BCFAAWG, BCFAAOWG and BCFAAHG operators. We also invented the linked properties of BCFAAWG, BCFAAOWG and BCFAAHG operators. The MABAC is the dominant technique to deal with ambiguous and complex information and it proves more dominant results. So, we have interpreted the technique of MABAC based on BCF Aczel-Alsina geometric AOs. Moreover, we have provided a numerical example that shows the uses of developed techniques in the field of OSs. At the end of this article, to portray the dominance and superiority of the invented work, we interpreted the comparative analysis of our invented work with various prevailing theories.
Structures like bipolar complex IFS, bipolar complex PFS and bipolar complex spherical FS use more advanced conditions than that of our developed notion. So, when the decision maker comes up with bipolar complex IF data, bipolar complex PF data and bipolar complex spherical fuzzy data, the existing notion fails.
In the future, we would examine various other theories such as three-way DM [60,61] and would try to do this invented work in these notions.
Moreover, some important abbreviation used throughout the article is given in Table 9.
Name | Abbreviation |
Fuzzy set | FS |
Bipolar fuzzy set | BFS |
Complex fuzzy set | CFS |
Bipolar complex fuzzy sets | BCFSs |
Decision making | DM |
Multi-attribute decision making | MADM |
Multi-Criteria decision making | MCDM |
Aczel Alsina | AA |
Operating System | OS |
Multi-attribute border approximation area comparison | MABAC |
Aggregation operators | AOs |
Bipolar complex fuzzy Aczel Alsina weighted averaging | BCFAAWA |
Bipolar complex fuzzy Aczel Alsina weighted geometric | BCFAAWG |
Bipolar complex fuzzy Aczel Alsina ordered weighted geometric | BCFAAOWG |
Bipolar complex fuzzy Aczel Alsina hybrid geometric | BCFAAHG |
The authors declare they have not used Artificial Intelligence (AI) tools in the creation of this article.
The authors declare that they do not have conflict of interest regarding this manuscript.
Proof of Theorem 1. We utilize a mathematical induction to assess the aforementioned problem, therefore first we solve it for n = 2 such that
\mathrm{B}\mathrm{C}\mathrm{F}\mathrm{A}\mathrm{A}\mathrm{W}\mathrm{G} ( ⊮{\text{}}_{1}, ⊮{\text{}}_{2}) = {\left(⊮{\text{}}_{1}\right)}^{{\mathfrak{k}}_{1}}\otimes {\left(⊮{\text{}}_{2}\right)}^{{\mathfrak{k}}_{2}} |
{\left(⊮{\text{}}_{1}\right)}^{{\mathfrak{k}}_{1}} = \left(\begin{array}{c}{\mathbb{e}}^{-{\left({\mathfrak{k}}_{1}{\left(-\mathrm{log}{\zeta }_{1}^{+}\right)}^{Ỿ}\right)}^{\frac{1}{Ỿ}}}+\iota ({\mathbb{e}}^{-{\left({{\mathfrak{k}}_{1}\left(-\mathrm{log}{\varsigma }_{1}^{+}\right)}^{Ỿ}\right)}^{\frac{1}{Ỿ}}}, \\ \left(-1+{\mathbb{e}}^{-{\left({\mathfrak{k}}_{1}{\left(-\mathrm{log}(1+{\zeta }_{1}^{\text{}-})\right)}^{Ỿ}\right)}^{\frac{1}{Ỿ}}}\right)+\iota (-1+\left({\mathbb{e}}^{-{\left({\mathfrak{k}}_{1}{\left(-\mathrm{log}(1+{\varsigma }_{1}^{-})\right)}^{Ỿ}\right)}^{\frac{1}{Ỿ}}}\right)\end{array}\right) |
{\left(⊮{\text{}}_{2}\right)}^{{\mathfrak{k}}_{2}} = \left(\begin{array}{c}{\mathbb{e}}^{-{\left({\mathfrak{k}}_{2}{\left(-\mathrm{log}{\zeta }_{2}^{+}\right)}^{Ỿ}\right)}^{\frac{1}{Ỿ}}}+\iota ({\mathbb{e}}^{-{\left({{\mathfrak{k}}_{2}\left(-\mathrm{log}{\varsigma }_{2}^{+}\right)}^{Ỿ}\right)}^{\frac{1}{Ỿ}}}, \\ \left(-1+{\mathbb{e}}^{-{\left({\mathfrak{k}}_{2}{\left(-\mathrm{log}(1+{\zeta }_{2}^{\text{}-})\right)}^{Ỿ}\right)}^{\frac{1}{Ỿ}}}\right)+\iota (-1+\left({\mathbb{e}}^{-{\left({\mathfrak{k}}_{2}{\left(-\mathrm{log}(1+{\varsigma }_{2}^{-})\right)}^{Ỿ}\right)}^{\frac{1}{Ỿ}}}\right)\end{array}\right) |
{\left(⊮{\text{}}_{1}\right)}^{{\mathfrak{k}}_{1}}\otimes {\left(⊮{\text{}}_{2}\right)}^{{\mathfrak{k}}_{2}} = \left(\begin{array}{c}{\mathbb{e}}^{-{\left({\mathfrak{k}}_{1}{\left(-\mathrm{log}{\zeta }_{1}^{+}\right)}^{Ỿ}\right)}^{\frac{1}{Ỿ}}}+\iota ({\mathbb{e}}^{-{\left({{\mathfrak{k}}_{1}\left(-\mathrm{log}{\varsigma }_{1}^{+}\right)}^{Ỿ}\right)}^{\frac{1}{Ỿ}}}, \\ \left(-1+{\mathbb{e}}^{-{\left({\mathfrak{k}}_{1}{\left(-\mathrm{log}(1+{\zeta }_{1}^{\text{}-})\right)}^{Ỿ}\right)}^{\frac{1}{Ỿ}}}\right)+\iota (-1+\left({\mathbb{e}}^{-{\left({\mathfrak{k}}_{1}{\left(-\mathrm{log}\left(1+{\varsigma }_{1}^{-}\right)\right)}^{Ỿ}\right)}^{\frac{1}{Ỿ}}}\right)\end{array}\right) |
\otimes \left(\begin{array}{c}{\mathbb{e}}^{-{\left({\mathfrak{k}}_{2}{\left(-\mathrm{log}{\zeta }_{2}^{+}\right)}^{Ỿ}\right)}^{\frac{1}{Ỿ}}}+\iota ({\mathbb{e}}^{-{\left({{\mathfrak{k}}_{2}\left(-\mathrm{log}{\varsigma }_{2}^{+}\right)}^{Ỿ}\right)}^{\frac{1}{Ỿ}}}, \\ \left(-1+{\mathbb{e}}^{-{\left({\mathfrak{k}}_{2}{\left(-\mathrm{log}(1+{\zeta }_{2}^{\text{}-})\right)}^{Ỿ}\right)}^{\frac{1}{Ỿ}}}\right)+\iota (-1+\left({\mathbb{e}}^{-{\left({\mathfrak{k}}_{2}{\left(-\mathrm{log}(1+{\varsigma }_{2}^{-})\right)}^{Ỿ}\right)}^{\frac{1}{Ỿ}}}\right)\end{array}\right) |
= \left(\begin{array}{c}\begin{array}{c}{{\mathbb{e}}^{-\left({\left(-\mathrm{log}{\left({\mathbb{e}}^{-\left({\mathfrak{k}}_{1}{\left(-\mathrm{log}{\zeta }_{1}^{+}\right)}^{Ỿ}\right)}\right)}^{\frac{1}{Ỿ}}\right)}^{Ỿ}+{\left(-\mathrm{log}{\left({\mathbb{e}}^{-\left({\mathfrak{k}}_{2}{\left(-\mathrm{log}{\zeta }_{2}^{+}\right)}^{Ỿ}\right)}\right)}^{\frac{1}{Ỿ}}\right)}^{Ỿ}\right)}}^{\frac{1}{Ỿ}}\\ +\iota {{\mathbb{e}}^{-\left({\left(-\mathrm{log}{\left({\mathbb{e}}^{-\left({\mathfrak{k}}_{1}{\left(-\mathrm{log}{\varsigma }_{1}^{+}\right)}^{Ỿ}\right)}\right)}^{\frac{1}{Ỿ}}\right)}^{Ỿ}+{\left(-\mathrm{log}{\left({\mathbb{e}}^{-\left({\mathfrak{k}}_{2}{\left(-\mathrm{log}{\varsigma }_{2}^{+}\right)}^{Ỿ}\right)}\right)}^{\frac{1}{Ỿ}}\right)}^{Ỿ}\right)}}^{\frac{1}{Ỿ}}, \end{array}\\ \left(\begin{array}{c}-1+{{\mathbb{e}}^{-\left(\begin{array}{c}{\left(-\mathrm{log}\left(1+\left(-1+{\mathbb{e}}^{-{\left({\mathfrak{k}}_{1}{\left(-\mathrm{log}\left(1+{\zeta }_{1}^{-}\right)\right)}^{Ỿ}\right)}^{\frac{1}{Ỿ}}}\right)\right)\right)}^{Ỿ}\\ +{\left(-\mathrm{log}\left(1+\left(-1+{\mathbb{e}}^{-{\left({\mathfrak{k}}_{2}{\left(-\mathrm{log}\left(1+{\zeta }_{2}^{-}\right)\right)}^{Ỿ}\right)}^{\frac{1}{Ỿ}}}\right)\right)\right)}^{Ỿ}\end{array}\right)}}^{\frac{1}{Ỿ}}\\ +\iota \left(-1+{{\mathbb{e}}^{-\left(\begin{array}{c}{\left(-\mathrm{log}\left(1+\left(-1+{\mathbb{e}}^{-{\left({\mathfrak{k}}_{1}{\left(-\mathrm{log}\left(1+{\varsigma }_{1}^{-}\right)\right)}^{Ỿ}\right)}^{\frac{1}{Ỿ}}}\right)\right)\right)}^{Ỿ}\\ +{\left(-\mathrm{log}\left(1+\left(-1+{\mathbb{e}}^{-{\left({\mathfrak{k}}_{2}{\left(-\mathrm{log}\left(1+{\varsigma }_{2}^{-}\right)\right)}^{Ỿ}\right)}^{\frac{1}{Ỿ}}}\right)\right)\right)}^{Ỿ}\end{array}\right)}}^{\frac{1}{Ỿ}}\right)\end{array}\right)\end{array}\right) |
= \left(\begin{array}{c}\begin{array}{c}{\mathbb{e}}^{-\left(\left({\left({\left({\mathfrak{k}}_{1}{\left(-\mathrm{log}{\zeta }_{1}^{+}\right)}^{Ỿ}\right)}^{\frac{1}{Ỿ}}\right)}^{Ỿ}\right)+{\left({\left({\left({\mathfrak{k}}_{2}{\left(-\mathrm{log}{\zeta }_{2}^{+}\right)}^{Ỿ}\right)}^{\frac{1}{Ỿ}}\right)}^{Ỿ}\right)}^{\frac{1}{Ỿ}}\right)}\\ +\iota \left({\mathbb{e}}^{-}\left(\left({\left({\left({\mathfrak{k}}_{1}{\left(-\mathrm{log}{\varsigma }_{1}^{+}\right)}^{Ỿ}\right)}^{\frac{1}{Ỿ}}\right)}^{Ỿ}\right)+{\left({\left({\left({\mathfrak{k}}_{2}{\left(-\mathrm{log}{\varsigma }_{2}^{+}\right)}^{Ỿ}\right)}^{\frac{1}{Ỿ}}\right)}^{Ỿ}\right)}^{\frac{1}{Ỿ}}\right)\right), \end{array}\\ -1+{{\mathbb{e}}^{-\left({\left({\left({\mathfrak{k}}_{1}{\left(-\mathrm{log}\left(1+{\zeta }_{1}^{-}\right)\right)}^{Ỿ}\right)}^{\frac{1}{Ỿ}}\right)}^{Ỿ}+\left({\left({\left({\mathfrak{k}}_{2}{\left(-\mathrm{log}\left(1+{\zeta }_{2}^{-}\right)\right)}^{Ỿ}\right)}^{\frac{1}{Ỿ}}\right)}^{Ỿ}\right)\right)}}^{\frac{1}{Ỿ}}\\ +\iota \left(-1+{{\mathbb{e}}^{-\left({\left({\left({\mathfrak{k}}_{1}{\left(-\mathrm{log}\left(1+{\varsigma }_{1}^{-}\right)\right)}^{Ỿ}\right)}^{\frac{1}{Ỿ}}\right)}^{Ỿ}+\left({\left({\left({\mathfrak{k}}_{2}{\left(-\mathrm{log}\left(1+{\varsigma }_{2}^{-}\right)\right)}^{Ỿ}\right)}^{\frac{1}{Ỿ}}\right)}^{Ỿ}\right)\right)}}^{\frac{1}{Ỿ}}\right)\end{array}\right) |
= \left(\begin{array}{c}{{\mathbb{e}}^{-\left(\left({\mathfrak{k}}_{1}{\left(-\mathrm{log}{\zeta }_{1}^{+}\right)}^{Ỿ}\right)+\left({\mathfrak{k}}_{2}{\left(-\mathrm{log}{\zeta }_{2}^{+}\right)}^{Ỿ}\right)\right)}}^{\frac{1}{Ỿ}}\\ +\iota \left({{\mathbb{e}}^{-\left(\left({\mathfrak{k}}_{1}{\left(-\mathrm{log}{\varsigma }_{1}^{+}\right)}^{Ỿ}\right)+\left({\mathfrak{k}}_{2}{\left(-\mathrm{log}{\varsigma }_{2}^{+}\right)}^{Ỿ}\right)\right)}}^{\frac{1}{Ỿ}}\right), \\ -1+{{\mathbb{e}}^{-\left(\left({\mathfrak{k}}_{1}{\left(-\mathrm{log}\left(1+{\zeta }_{1}^{-}\right)\right)}^{Ỿ}\right)+\left({\mathfrak{k}}_{2}{\left(-\mathrm{log}\left(1+{\zeta }_{2}^{-}\right)\right)}^{Ỿ}\right)\right)}}^{\frac{1}{Ỿ}}\\ +\iota \left(-1+{{\mathbb{e}}^{-\left(\left({\mathfrak{k}}_{1}{\left(-\mathrm{log}\left(1+{\varsigma }_{1}^{-}\right)\right)}^{Ỿ}\right)+\left({\mathfrak{k}}_{2}{\left(-\mathrm{log}\left(1+{\varsigma }_{2}^{-}\right)\right)}^{Ỿ}\right)\right)}}^{\frac{1}{Ỿ}}\right)\end{array}\right) |
{\left(⊮{\text{}}_{1}\right)}^{{\mathfrak{k}}_{1}}\otimes {\left(⊮{\text{}}_{2}\right)}^{{\mathfrak{k}}_{2}} = \left(\begin{array}{c}{\mathbb{e}}^{-{\left(\sum _{\rho = 1}^{2}\left({\mathfrak{k}}_{\rho }{\left(-\mathrm{log}{\zeta }_{\rho }^{+}\right)}^{Ỿ}\right)\right)}^{\frac{1}{Ỿ}}}+\iota {\mathbb{e}}^{-{\left(\sum _{\rho = 1}^{2}\left({\mathfrak{k}}_{\rho }{\left(-\mathrm{log}{\varsigma }_{\rho }^{+}\right)}^{Ỿ}\right)\right)}^{\frac{1}{Ỿ}}}, \\ -1+{\mathbb{e}}^{{-\left(\sum _{\rho = 1}^{2}\left({\mathfrak{k}}_{\rho }{\left(-\mathrm{log}\left(1+{\zeta }_{\rho }^{-}\right)\right)}^{Ỿ}\right)\right)}^{\frac{1}{Ỿ}}}+\iota \left(-1+{\mathbb{e}}^{{-\left(\sum _{\rho = 1}^{2}\left({\mathfrak{k}}_{\rho }{\left(-\mathrm{log}\left(1+{\varsigma }_{\rho }^{-}\right)\right)}^{Ỿ}\right)\right)}^{\frac{1}{Ỿ}}}\right)\end{array}\right) . |
The aforementioned equation is therefore true for n = 2 . If we now suppose that n = q is true, then we have
\mathrm{B}\mathrm{C}\mathrm{F}\mathrm{A}\mathrm{A}\mathrm{W}\mathrm{G} ( ⊮{\text{}}_{1}, \mathrm{ }\mathrm{ }\mathrm{ }\mathrm{ }⊮{\text{}}_{2}, \mathrm{ }\mathrm{ }\mathrm{ }\mathrm{ }⊮{\text{}}_{3}, \dots \dots \dots \dots , \mathrm{ }\mathrm{ }\mathrm{ }\mathrm{ }⊮\mathfrak{q} ) = {\otimes }_{\rho = 1}^{\mathfrak{q}}{\left(⊮{\text{}}_{\rho }\right)}^{{\mathfrak{k}}_{\rho }} |
= \left(\begin{array}{c}{\mathbb{e}}^{-{\left(\sum _{\rho = 1}^{\mathfrak{q}}\left({\mathfrak{k}}_{\rho }{\left(-\mathrm{log}{\zeta }_{\rho }^{+}\right)}^{\mathrm{Ỿ}}\right)\right)}^{\frac{1}{\mathrm{Ỿ}}}}+\iota {\mathbb{e}}^{-{\left(\sum _{\rho = 1}^{\mathfrak{q}}\left({\mathfrak{k}}_{\rho }{\left(-\mathrm{log}{\varsigma }_{\rho }^{+}\right)}^{\mathrm{Ỿ}}\right)\right)}^{\frac{1}{\mathrm{Ỿ}}}}, \\ -1+{\mathbb{e}}^{{-\left(\sum _{\rho = 1}^{\mathfrak{q}}\left({\mathfrak{k}}_{\rho }{\left(-\mathrm{log}\left(1+{\zeta }_{\rho }^{-}\right)\right)}^{\mathrm{Ỿ}}\right)\right)}^{\frac{1}{\mathrm{Ỿ}}}}+\iota \left(-1+{\mathbb{e}}^{{-\left(\sum _{\rho = 1}^{\mathfrak{q}}\left({\mathfrak{k}}_{\rho }{\left(-\mathrm{log}\left(1+{\varsigma }_{\rho }^{-}\right)\right)}^{\mathrm{Ỿ}}\right)\right)}^{\frac{1}{\mathrm{Ỿ}}}}\right)\end{array}\right) . |
Next, we must demonstrate it for n = q+1 , that is,
\mathrm{B}\mathrm{C}\mathrm{F}\mathrm{A}\mathrm{A}\mathrm{W}\mathrm{G} ( ⊮{\text{}}_{1}, \mathrm{ }\mathrm{ }\mathrm{ }\mathrm{ }⊮{\text{}}_{2}, \mathrm{ }\mathrm{ }\mathrm{ }\mathrm{ }⊮{\text{}}_{3}, \dots \dots \dots \dots , {⊮}_{\mathfrak{q}}, {⊮}_{\mathfrak{q}+1} ) = {\otimes }_{\rho = 1}^{\mathfrak{q}}{\left(⊮{\text{}}_{\rho }\right)}^{{\mathfrak{k}}_{\rho }}\otimes {\left(⊮{\text{}}_{\mathfrak{q}+1}\right)}^{{\mathfrak{k}}_{\mathfrak{q}+1}} |
= \left(\begin{array}{c}{\mathbb{e}}^{-{\left(\sum _{\rho = 1}^{\mathfrak{q}}\left({\mathfrak{k}}_{\rho }{\left(-\mathrm{log}{\zeta }_{\rho }^{+}\right)}^{\mathrm{Ỿ}}\right)\right)}^{\frac{1}{\mathrm{Ỿ}}}}+\iota {\mathbb{e}}^{-{\left(\sum _{\rho = 1}^{\mathfrak{q}}\left({\mathfrak{k}}_{\rho }{\left(-\mathrm{log}{\varsigma }_{\rho }^{+}\right)}^{\mathrm{Ỿ}}\right)\right)}^{\frac{1}{\mathrm{Ỿ}}}}, \\ -1+{\mathbb{e}}^{{-\left(\sum _{\rho = 1}^{\mathfrak{q}}\left({\mathfrak{k}}_{\rho }{\left(-\mathrm{log}\left(1+{\zeta }_{\rho }^{-}\right)\right)}^{\mathrm{Ỿ}}\right)\right)}^{\frac{1}{\mathrm{Ỿ}}}}+\iota \left(-1+{\mathbb{e}}^{{-\left(\sum _{\rho = 1}^{\mathfrak{q}}\left({\mathfrak{k}}_{\rho }{\left(-\mathrm{log}\left(1+{\varsigma }_{\rho }^{-}\right)\right)}^{\mathrm{Ỿ}}\right)\right)}^{\frac{1}{\mathrm{Ỿ}}}}\right)\end{array}\right) |
\otimes \left(\begin{array}{c}{{\mathbb{e}}^{-\left(\left({\mathfrak{k}}_{\mathfrak{q}+1}{\left(-\mathrm{log}{\zeta }_{\mathfrak{q}+1}^{+}\right)}^{\mathrm{Ỿ}}\right)\right)}}^{\frac{1}{\mathrm{Ỿ}}}+\iota \left({{\mathbb{e}}^{-\left(\left({\mathfrak{k}}_{\mathfrak{q}+1}{\left(-\mathrm{log}{\varsigma }_{\mathfrak{q}+1}^{+}\right)}^{\mathrm{Ỿ}}\right)\right)}}^{\frac{1}{\mathrm{Ỿ}}}\right), \\ \left(-1+{{\mathbb{e}}^{-\left(\left({\mathfrak{k}}_{\mathfrak{q}+1}{\left(-\mathrm{log}\left(1+{\zeta }_{\mathfrak{q}+1}^{-}\right)\right)}^{\mathrm{Ỿ}}\right)\right)}}^{\frac{1}{\mathrm{Ỿ}}}+\iota \left(-1+{{\mathbb{e}}^{-\left(\left({\mathfrak{k}}_{\mathfrak{q}+1}{\left(-\mathrm{log}\left(1+{\varsigma }_{\mathfrak{q}+1}^{-}\right)\right)}^{\mathrm{Ỿ}}\right)\right)}}^{\frac{1}{\mathrm{Ỿ}}}\right)\right)\end{array}\right) |
= \left(\begin{array}{c}{\mathbb{e}}^{-{\left(\sum _{\rho = 1}^{\mathfrak{q}+1}\left({\mathfrak{k}}_{\rho }{\left(-\mathrm{log}{\zeta }_{\rho }^{+}\right)}^{\mathrm{Ỿ}}\right)\right)}^{\frac{1}{\mathrm{Ỿ}}}}+\iota {\mathbb{e}}^{-{\left(\sum _{\rho = 1}^{\mathfrak{q}+1}\left({\mathfrak{k}}_{\rho }{\left(-\mathrm{log}{\varsigma }_{\rho }^{+}\right)}^{\mathrm{Ỿ}}\right)\right)}^{\frac{1}{\mathrm{Ỿ}}}}, \\ -1+{\mathbb{e}}^{{-\left(\sum _{\rho = 1}^{\mathfrak{q}+1}\left({\mathfrak{k}}_{\rho }{\left(-\mathrm{log}\left(1+{\zeta }_{\rho }^{-}\right)\right)}^{\mathrm{Ỿ}}\right)\right)}^{\frac{1}{\mathrm{Ỿ}}}}+\iota \left(-1+{\mathbb{e}}^{{-\left(\sum _{\rho = 1}^{\mathfrak{q}+1}\left({\mathfrak{k}}_{\rho }{\left(-\mathrm{log}\left(1+{\varsigma }_{\rho }^{-}\right)\right)}^{\mathrm{Ỿ}}\right)\right)}^{\frac{1}{\mathrm{Ỿ}}}}\right)\end{array}\right) . |
Finally, we discovered that the preceding equation is true for n = q+1 . Hence, all (non-negative integer) values of n were fulfilled by the aforementioned equation.
Proof of Theorem 2. Consider {⊮}_{\rho } = ⊮ = \left({\zeta }_{\rho }^{+}+{\varsigma }_{\rho }^{+}, {\zeta }_{\rho }^{\text{}-}+{\varsigma }_{\rho }^{-}\right) . Then, we have
\mathrm{B}\mathrm{C}\mathrm{F}\mathrm{A}\mathrm{A}\mathrm{W}\mathrm{G} ( ⊮{\text{}}_{1}, \mathrm{ }\mathrm{ }\mathrm{ }\mathrm{ }⊮{\text{}}_{2}, \mathrm{ }\mathrm{ }\mathrm{ }\mathrm{ }⊮{\text{}}_{3}, \dots \dots \dots \dots , \mathrm{ }\mathrm{ }\mathrm{ }\mathrm{ }⊮{\text{}}_{n} ) = \\ \left(\begin{array}{c}{\mathbb{e}}^{-{\left(\sum _{\rho = 1}^{n}{\mathfrak{k}}_{\rho }{\left(-\mathrm{log}{\zeta }^{+}\right)}^{\mathrm{Ỿ}}\right)}^{\frac{1}{\mathrm{Ỿ}}}}+\iota ({\mathbb{e}}^{-{\left(\sum _{\rho = 1}^{n}{{\mathfrak{k}}_{\rho }\left(-\mathrm{log}{\varsigma }^{+}\right)}^{\mathrm{Ỿ}}\right)}^{\frac{1}{\mathrm{Ỿ}}}}, \\ \left(-1+{\mathbb{e}}^{-{\left(\sum _{\rho = 1}^{n}{\mathfrak{k}}_{\rho }{\left(-\mathrm{log}(1+{\zeta }^{-})\right)}^{\mathrm{Ỿ}}\right)}^{\frac{1}{\mathrm{Ỿ}}}}\right)+\iota (-1+\left({\mathbb{e}}^{-{\left(\sum _{\rho = 1}^{n}{\mathfrak{k}}_{\rho }{\left(-\mathrm{log}(1+{\varsigma }^{+})\right)}^{\mathrm{Ỿ}}\right)}^{\frac{1}{\mathrm{Ỿ}}}}\right)\end{array}\right) |
= \left(\begin{array}{c}{\mathbb{e}}^{-{\left({\left(-\mathrm{log}{\zeta }^{+}\right)}^{\mathrm{Ỿ}}\right)}^{\frac{1}{\mathrm{Ỿ}}}}+\iota ({\mathbb{e}}^{-{\left({\left(-\mathrm{log}{\varsigma }^{+}\right)}^{\mathrm{Ỿ}}\right)}^{\frac{1}{\mathrm{Ỿ}}}}, \\ \left(-1+{\mathbb{e}}^{-{\left({\left(-\mathrm{log}(1+{\zeta }^{-})\right)}^{\mathrm{Ỿ}}\right)}^{\frac{1}{\mathrm{Ỿ}}}}\right)+\iota (-1+\left({\mathbb{e}}^{-{\left({\left(-\mathrm{log}(1+{\varsigma }^{+})\right)}^{\mathrm{Ỿ}}\right)}^{\frac{1}{\mathrm{Ỿ}}}}\right)\end{array}\right) |
= \left({\mathbb{e}}^{-\left(-\mathrm{log}{\zeta }^{+}\right)}+\iota \left({\mathbb{e}}^{-\left(-\mathrm{log}{\varsigma }^{+}\right)}\right), -1+{\mathbb{e}}^{-\left(-\mathrm{log}(1+{\zeta }^{-})\right)}+\iota \left(-1+{\mathbb{e}}^{-\left(-\mathrm{log}(1+{\varsigma }^{-})\right)}\right)\right) |
= \left({\zeta }_{\rho }^{+}+{\varsigma }_{\rho }^{+}, {\zeta }_{\rho }^{\text{}-}+{\varsigma }_{\rho }^{-}\right) = ⊮ . |
Thus, we obtain the ultimate result.
Proof of Theorem 3. Observing the analysis above, we have {⊮}_{\rho }\le {⊮}_{\rho }^{\sim }\forall \rho i.e., {\zeta }_{\rho }^{+}\le {\zeta }_{\rho \sim }^{+}, {\varsigma }_{\rho }^{+}\le {\varsigma }_{\rho \sim }^{+}, {\zeta }_{\rho }^{\text{}-}\le {\zeta }_{\rho \sim }^{\text{}-}, {\varsigma }_{\rho }^{-}\le {\varsigma }_{\rho \sim }^{-} . Subsequently, along with further data, we obtain
{\zeta }_{\rho }^{+}\le {\zeta }_{\rho \sim }^{+}\Rightarrow \mathrm{log}{\zeta }_{\rho }^{+}\le \mathrm{log}{\zeta }_{\rho \sim }^{+} |
\Rightarrow \mathrm{log}{\zeta }_{\rho }^{+}\le \mathrm{log}{\zeta }_{\rho \sim }^{+}\Rightarrow -\mathrm{log}{\zeta }_{\rho }^{+}\ge -\mathrm{log}{\zeta }_{\rho \sim }^{+} |
\Rightarrow \sum\limits_{\rho = 1}^{n}{\mathfrak{k}}_{\rho }{\left(-\mathrm{log}{\zeta }_{\rho }^{+}\right)}^{\mathrm{Ỿ}}\ge \sum\limits_{\rho = 1}^{n}{\mathfrak{k}}_{\rho }{\left(-\mathrm{log}{\zeta }_{\rho \sim }^{+}\right)}^{\mathrm{Ỿ}} |
\Rightarrow -\sum\limits_{\rho = 1}^{n}{\mathfrak{k}}_{\rho }{\left(-\mathrm{log}{\zeta }_{\rho }^{+}\right)}^{\mathrm{Ỿ}}\le -\sum\limits_{\rho = 1}^{n}{\mathfrak{k}}_{\rho }{\left(-\mathrm{log}{\zeta }_{\rho \sim }^{+}\right)}^{\mathrm{Ỿ}} |
\Rightarrow {\mathbb{e}}^{-{\left(\sum _{\rho = 1}^{n}{\mathfrak{k}}_{\rho }{\left(-\mathrm{log}{\zeta }_{\rho }^{+}\right)}^{\mathrm{Ỿ}}\right)}^{\frac{1}{\mathrm{Ỿ}}}}\le {\mathbb{e}}^{-{\left(\sum _{\rho = 1}^{n}{\mathfrak{k}}_{\rho }{\left(-\mathrm{log}{\zeta }_{\rho \sim }^{+}\right)}^{\mathrm{Ỿ}}\right)}^{\frac{1}{\mathrm{Ỿ}}}} . |
Similar to this, we have established the theory for the imaginary part along a positive membership grade, such as
{\mathbb{e}}^{-{\left(\sum _{\rho = 1}^{n}{\mathfrak{k}}_{\rho }{\left(-\mathrm{log}{\varsigma }_{\rho }^{+}\right)}^{Ỿ}\right)}^{\frac{1}{Ỿ}}}\le {\mathbb{e}}^{-{\left(\sum _{\rho = 1}^{n}{\mathfrak{k}}_{\rho }{\left(-\mathrm{log}{\varsigma }_{\rho \sim }^{+}\right)}^{Ỿ}\right)}^{\frac{1}{Ỿ}}} . |
Based on the aforementioned data, we can now further discuss:
1+{\zeta }_{\rho }^{\text{}-}\le 1+{\zeta }_{\rho \sim }^{\text{}-}\Rightarrow \mathrm{log}\left(1+{\zeta }_{\rho }^{\text{}-}\right)\le \mathrm{log}\left(1+{\zeta }_{\rho \sim }^{\text{}-}\right) |
\Rightarrow -\mathrm{log}\left(1+{\zeta }_{\rho }^{\text{}-}\right)\ge -\mathrm{log}\left(1+{\zeta }_{\rho \sim }^{\text{}-}\right) |
\Rightarrow \sum\limits_{\rho = 1}^{n}{\mathfrak{k}}_{\rho }{\left(-\mathrm{log}\left(1+{\zeta }_{\rho }^{\text{}-}\right)\right)}^{\mathrm{Ỿ}}\ge \sum\limits_{\rho = 1}^{n}{\mathfrak{k}}_{\rho }{\left(-\mathrm{log}\left(1+{\zeta }_{\rho \sim }^{\text{}-}\right)\right)}^{\mathrm{Ỿ}} |
\Rightarrow -\sum\limits_{\rho = 1}^{n}{\mathfrak{k}}_{\rho }{\left(-\mathrm{log}\left(1+{\zeta }_{\rho }^{\text{}-}\right)\right)}^{\mathrm{Ỿ}}\le -\sum\limits_{\rho = 1}^{n}{\mathfrak{k}}_{\rho }{\left(-\mathrm{log}\left(1+{\zeta }_{\rho \sim }^{\text{}-}\right)\right)}^{\mathrm{Ỿ}} |
\Rightarrow {\mathbb{e}}^{-{\left(\sum _{\rho = 1}^{n}{\mathfrak{k}}_{\rho }{\left(-\mathrm{log}\left(1+{\zeta }_{\rho }^{\text{}-}\right)\right)}^{\mathrm{Ỿ}}\right)}^{\frac{1}{\mathrm{Ỿ}}}}\le {\mathbb{e}}^{-{\left(\sum _{\rho = 1}^{n}{\mathfrak{k}}_{\rho }{\left(-\mathrm{log}\left(1+{\zeta }_{\rho \sim }^{\text{}-}\right)\right)}^{\mathrm{Ỿ}}\right)}^{\frac{1}{\mathrm{Ỿ}}}} |
\Rightarrow {-1+\mathbb{e}}^{-{\left(\sum _{\rho = 1}^{n}{\mathfrak{k}}_{\rho }{\left(-\mathrm{log}\left(1+{\zeta }_{\rho }^{\text{}-}\right)\right)}^{\mathrm{Ỿ}}\right)}^{\frac{1}{\mathrm{Ỿ}}}}\le {-1+\mathbb{e}}^{-{\left(\sum _{\rho = 1}^{n}{\mathfrak{k}}_{\rho }{\left(-\mathrm{log}\left(1+{\zeta }_{\rho \sim }^{\text{}-}\right)\right)}^{\mathrm{Ỿ}}\right)}^{\frac{1}{\mathrm{Ỿ}}}} . |
Likewise, we obtain
{-1+\mathbb{e}}^{-{\left(\sum _{\rho = 1}^{n}{\mathfrak{k}}_{\rho }{\left(-\mathrm{log}\left(1+{\varsigma }_{\rho }^{\text{}-}\right)\right)}^{Ỿ}\right)}^{\frac{1}{Ỿ}}}\le {-1+\mathbb{e}}^{-{\left(\sum _{\rho = 1}^{n}{\mathfrak{k}}_{\rho }{\left(-\mathrm{log}\left(1+{\varsigma }_{\rho \sim }^{\text{}-}\right)\right)}^{Ỿ}\right)}^{\frac{1}{Ỿ}}} . |
We may easily arrive at the following conclusion by applying the score and accuracy function, which is
\mathrm{B}\mathrm{C}\mathrm{F}\mathrm{A}\mathrm{A}\mathrm{W}\mathrm{G}\left(⊮{\text{}}_{1}, ⊮{\text{}}_{2}, ⊮{\text{}}_{3}, \dots \dots \dots \dots , ⊮{\text{}}_{n}\right)\le \mathrm{B}\mathrm{C}\mathrm{F}\mathrm{A}\mathrm{A}\mathrm{W}\mathrm{G}\left({⊮}_{1}^{\sim }, {⊮}_{2}^{\sim }, {⊮}_{3}^{\sim }, \dots \dots \dots \dots , {⊮}_{n}^{\sim }\right) . |
Proof of Theorem 4. Using the properties of idempotency and monotonicity, we can quickly and easily demonstrate the necessary theorem.
\mathrm{B}\mathrm{C}\mathrm{F}\mathrm{A}\mathrm{A}\mathrm{W}\mathrm{G}\left(⊮{\text{}}_{1}, ⊮{\text{}}_{2}, ⊮{\text{}}_{3}, \dots \dots \dots \dots , ⊮{\text{}}_{n}\right)\le \mathrm{B}\mathrm{C}\mathrm{F}\mathrm{A}\mathrm{A}\mathrm{W}\mathrm{G}\left({⊮}^{+}, {⊮}^{+}, {⊮}^{+}, \dots \dots \dots \dots , {⊮}^{+}\right) = {⊮}^{+} |
\mathrm{B}\mathrm{C}\mathrm{F}\mathrm{A}\mathrm{A}\mathrm{W}\mathrm{G}\left(⊮{\text{}}_{1}, \mathrm{ }⊮{\text{}}_{2}, \mathrm{ }⊮{\text{}}_{3}, \dots \dots \dots \dots , \mathrm{ }⊮{\text{}}_{n}\right)\ge \mathrm{B}\mathrm{C}\mathrm{F}\mathrm{A}\mathrm{A}\mathrm{W}\mathrm{G}\left({⊮}^{-}, {⊮}^{-}, {⊮}^{-}, \dots \dots \dots \dots , {⊮}^{-}\right) = {⊮}^{-} . |
Hence, using the equations above, we arrive at
{⊮}^{-}\le \mathrm{B}\mathrm{C}\mathrm{F}\mathrm{A}\mathrm{A}\mathrm{W}\mathrm{G}\left(⊮{\text{}}_{1}, ⊮{\text{}}_{2}, ⊮{\text{}}_{3}, \dots \dots \dots \dots , ⊮{\text{}}_{n}\right)\le {⊮}^{+}. |
[1] | P. B. Hansen, Operating system principles, United States: Prentice-Hall, Inc., 1973. Available from: https://dl.acm.org/doi/abs/10.5555/540365. |
[2] | A. Silberschatz, P. B. Galvin, G. Gagne, Applied operating system concepts, United States: John Wiley and Sons, Inc., 1999. Available from: https://dl.acm.org/doi/abs/10.5555/330796. |
[3] | D. Comer, Operating system design, New York: CRC Press, 2011. |
[4] | G. Klein, Operating system verification—an overview, Springer, 34 (2009), 27–69. https://doi.org/10.1007/s12046-009-0002-4 |
[5] | C. W. Mercer, Operating system support for multimedia applications, In: Proceedings of the second ACM international conference on Multimedia, 1994,492–493. https://doi.org/10.1145/192593.197424 |
[6] | S. T. King, G. W. Dunlap, P. M. Chen, Operating system support for virtual machines, In: USENIX Annual Technical Conference, General Track, 2003, 71–84. |
[7] | I. M. Leslie, D. McAuley, R. Black, T. Roscoe, P. Barham, D. Evers, et al., The design and implementation of an operating system to support distributed multimedia applications, IEEE J. Sel. Area. Comm., 14 (1996), 1280–1297. https://doi.org/10.1109/49.536480 |
[8] | R. Singh, An overview of the android operating system and its security, Int. J. Eng. Res. Appl., 4 (2014), 519–521. |
[9] | L. A. Zadeh, Fuzzy sets, Inf. Control, 8 (1965), 338–353. https://doi.org/10.1016/S0019-9958(65)90241-X |
[10] | H. J. Zimmermann, Fuzzy set theory—and its applications, 4 Eds, Springer Sci., 2011. |
[11] |
M. Riaz, M. R. Hashmi, D. Pamucar, Y. M. Ch, Spherical linear Diophantine fuzzy sets with modeling uncertainties in MCDM, Comput. Model. Eng. Sci., 126 (2021), 1125–1164. https://doi.org/10.32604/cmes.2021.013699 doi: 10.32604/cmes.2021.013699
![]() |
[12] |
S. Ayub, M. Shabir, M. Riaz, W. Mahmood, D. Bozanic, D. Marinkovic, Linear Diophantine fuzzy rough sets: A new rough set approach with decision making, Symmetry, 14 (2022), 525. https://doi.org/10.3390/sym14030525 doi: 10.3390/sym14030525
![]() |
[13] |
M. Riaz, M. R. Hashmi, Linear Diophantine fuzzy set and its applications towards multi-attribute decision-making problems, J. Intell. Fuzzy Syst., 37 (2019), 5417–5439. https://doi.org/10.3233/JIFS-190550 doi: 10.3233/JIFS-190550
![]() |
[14] |
E. Tolga, M. L. Demircan, C. Kahraman, Operating system selection using fuzzy replacement analysis and analytic hierarchy process, Int. J. Prod. Econ., 97 (2005), 89–117. https://doi.org/10.1016/j.ijpe.2004.07.001 doi: 10.1016/j.ijpe.2004.07.001
![]() |
[15] |
S. Ballı, S. Korukoğlu, Operating system selection using fuzzy AHP and TOPSIS methods, Math. Comput. Appl., 14 (2009), 119–130. https://doi.org/10.3390/mca14020119 doi: 10.3390/mca14020119
![]() |
[16] |
A. Kandel, Y. Q. Zhang, M. Henne, On the use of fuzzy logic technology in operating systems, Fuzzy Set. Syst., 99 (1998), 241–251. https://doi.org/10.1016/S0165-0114(96)00392-2 doi: 10.1016/S0165-0114(96)00392-2
![]() |
[17] |
A. Mardani, M. Nilashi, E. K. Zavadskas, S. R. Awang, H. Zare, N. M. Jamal, Decision making methods based on fuzzy aggregation operators: Three decades review from 1986 to 2017, Int. J. Inf. Technol. Decisi. Mak., 17 (2018), 391–466. https://doi.org/10.1142/S021962201830001X doi: 10.1142/S021962201830001X
![]() |
[18] | W. R. Zhang, Bipolar fuzzy sets and relations: A computational framework for cognitive modeling and multiagent decision analysis, In: NAFIPS/IFIS/NASA'94, Proceedings of the First International Joint Conference of The North American Fuzzy Information Processing Society Biannual Conference, The Industrial Fuzzy Control and Intellige, 1994,305–309. https://doi.org/10.1109/IJCF.1994.375115 |
[19] |
G. Wei, F. E. Alsaadi, T. Hayat, A. Alsaedi, Bipolar fuzzy Hamacher aggregation operators in multiple attribute decision making, Int. J. Fuzzy Syst., 20 (2018), 1–12. https://doi.org/10.1007/s40815-017-0338-6 doi: 10.1007/s40815-017-0338-6
![]() |
[20] |
C. Jana, M. Pal, J. Q. Wang, Bipolar fuzzy Dombi aggregation operators and its application in multiple-attribute decision-making process, J. Amb. Intel. Hum. Comp., 10 (2019), 3533–3549. https://doi.org/10.1007/s12652-018-1076-9 doi: 10.1007/s12652-018-1076-9
![]() |
[21] | M. Akram, Bipolar fuzzy graphs, Inf. Sci., 181 (2011), 5548–5564. https://doi.org/10.1016/j.ins.2011.07.037 |
[22] |
M. Akram, Bipolar fuzzy graphs with applications, Knowl.-Based Syst., 39 (2013), 1–8. https://doi.org/10.1016/j.knosys.2012.08.022 doi: 10.1016/j.knosys.2012.08.022
![]() |
[23] | S. Samanta, M. Pal, Irregular bipolar fuzzy graphs, arXiv preprint, 2012. https://doi.org/10.48550/arXiv.1209.1682 |
[24] |
H. Rashmanlou, S. Samanta, M. Pal, R. A. Borzooei, Product of bipolar fuzzy graphs and their degree, Int. J. Gen. Syst., 45 (2016), 1–14. https://doi.org/10.1080/03081079.2015.1072521 doi: 10.1080/03081079.2015.1072521
![]() |
[25] |
M. A. Alghamdi, N. O. Alshehri, M. Akram, Multi-criteria decision-making methods in bipolar fuzzy environment, Int. J. Fuzzy Syst., 20 (2018), 2057–2064. https://doi.org/10.1007/s40815-018-0499-y doi: 10.1007/s40815-018-0499-y
![]() |
[26] |
M. Akram, M. Ali, T. Allahviranloo, A method for solving bipolar fuzzy complex linear systems with real and complex coefficients, Soft Comput., 26 (2022), 2157–2178. https://doi.org/10.1007/s00500-021-06672-7 doi: 10.1007/s00500-021-06672-7
![]() |
[27] |
M. Akram, U. Amjad, B. Davvaz, Decision-making analysis based on bipolar fuzzy N-soft information, Comput. Appl. Math., 40 (2021), 182. https://doi.org/10.1007/s40314-021-01570-y doi: 10.1007/s40314-021-01570-y
![]() |
[28] | M. Akram, A. N. Al-Kenani, Multi-criteria group decision-making for selection of green suppliers under bipolar fuzzy PROMETHEE process, Symmetry, 12 (2020), 77. https://doi.org/10.3390/sym12010077 |
[29] |
M. Akram, Shumaiza, M. Arshad, Bipolar fuzzy TOPSIS and bipolar fuzzy ELECTRE-I methods to diagnosis, Comput. Appl. Math., 39 (2020), 1–21. https://doi.org/10.1007/s40314-019-0980-8 doi: 10.1007/s40314-019-0980-8
![]() |
[30] |
M. Akram, A. N. Al-Kenani, J. C. R. Alcantud, Group decision-making based on the VIKOR method with trapezoidal bipolar fuzzy information, Symmetry, 11 (2019), 1313. https://doi.org/10.3390/sym11101313 doi: 10.3390/sym11101313
![]() |
[31] |
M. Akram, M. Arshad, A novel trapezoidal bipolar fuzzy TOPSIS method for group decision-making, Group Decis. Negot., 28 (2019), 565–584. https://doi.org/10.1007/s10726-018-9606-6 doi: 10.1007/s10726-018-9606-6
![]() |
[32] | M. Akram, M. Ali, T. Allahviranloo, Solution of the complex bipolar fuzzy linear system, In: Progress in Intelligent Decision Science, Springer, Cham, 1301 (2021), 899–927. https://doi.org/10.1007/978-3-030-66501-2-73 |
[33] |
C. Jana, M. Pal, J. Q. Wang, Bipolar fuzzy Dombi prioritized aggregation operators in multiple attribute decision making, Soft Comput., 24 (2020), 3631–3646. https://doi.org/10.1007/s00500-019-04130-z doi: 10.1007/s00500-019-04130-z
![]() |
[34] |
M. Riaz, S. T. Tehrim, Multi-attribute group decision making based on cubic bipolar fuzzy information using averaging aggregation operators, J. Intell. Fuzzy Syst., 37 (2019), 2473–2494. https://doi.org/10.3233/JIFS-182751 doi: 10.3233/JIFS-182751
![]() |
[35] | D. Ramot, R. Milo, M. Friedman, A. Kandel, Complex fuzzy sets, IEEE T. Fuzzy Syst. 10 (2002), 171–186. https://doi.org/10.1109/91.995119 |
[36] |
D. E. Tamir, L. Jin, A. Kandel, A new interpretation of complex membership grade, Int. J. Intell. Syst., 26 (2011), 285–312. https://doi.org/10.1002/int.20454 doi: 10.1002/int.20454
![]() |
[37] | D. E. Tamir, N. D. Rishe, A. Kandel, Complex fuzzy sets and complex fuzzy logic an overview of theory and applications, In: Fifty years of fuzzy logic and its applications, Springer, Cham, 326 (2015), 661–681. https://doi.org/10.1007/978-3-319-19683-1-31 |
[38] | L. Bi, S. Dai, B. Hu, Complex fuzzy geometric aggregation operators, Symmetry, 10 (2018), 251. https://doi.org/10.3390/sym10070251 |
[39] |
L. Bi, S. Dai, B. Hu, S. Li, Complex fuzzy arithmetic aggregation operators, J. Intell. Fuzzy Syst., 36 (2019), 2765–2771. https://doi.org/10.3233/JIFS-18568 doi: 10.3233/JIFS-18568
![]() |
[40] | T. Mahmood, U. Ur Rehman, A novel approach towards bipolar complex fuzzy sets and their applications in generalized similarity measures, Int. J. Intell. Syst., 37 (2022), 535–567. https://doi.org/10.1002/int.22639 |
[41] | T. Mahmood, U. Ur Rehman, A method to multi-attribute decision making technique based on Dombi aggregation operators under bipolar complex fuzzy information, Comput. Appl. Math., 41 (2022), 1–23. https://doi.org/10.1007/s40314-021-01735-9 |
[42] |
T. Mahmood, U. Ur Rehman, Z. Ali, Analysis and application of Aczel-Alsina aggregation operators based on bipolar complex fuzzy information in multiple attribute decision making, Inf. Sci., 619 (2023), 817–833. https://doi.org/10.1016/j.ins.2022.11.067 doi: 10.1016/j.ins.2022.11.067
![]() |
[43] | D. Pamučar, G. Ćirović, The selection of transport and handling resources in logistics centers using Multi-Attributive Border Approximation Area Comparison (MABAC), Expert Syst. Appl., 42 (2015), 3016–3028. https://doi.org/10.1016/j.eswa.2014.11.057 |
[44] |
R. Verma, Fuzzy MABAC method based on new exponential fuzzy information measures, Soft Comput., 25 (2021), 9575–9589. https://doi.org/10.1007/s00500-021-05739-9 doi: 10.1007/s00500-021-05739-9
![]() |
[45] |
M. Zhao, G. Wei, X. Chen, Y. Wei, Intuitionistic fuzzy MABAC method based on cumulative prospect theory for multiple attribute group decision making, Int. J. Intel. Syst., 36 (2021), 6337–6359. https://doi.org/10.1002/int.22552 doi: 10.1002/int.22552
![]() |
[46] |
Z. Jiang, G. Wei, Y. Guo, Picture fuzzy MABAC method based on prospect theory for multiple attribute group decision making and its application to suppliers' selection, J. Intel. Fuzzy. Syst., 42 (2022), 3405–3415. https://doi.org/10.3233/JIFS-211359 doi: 10.3233/JIFS-211359
![]() |
[47] |
C. Jana, Multiple attribute group decision-making method based on extended bipolar fuzzy MABAC approach, Comput. Appl. Math., 40 (2021), 1–17. https://doi.org/10.1007/s40314-021-01606-3 doi: 10.1007/s40314-021-01606-3
![]() |
[48] |
R. Zhang, Z. Xu, X. Gou, ELECTRE Ⅱ method based on the cosine similarity to evaluate the performance of financial logistics enterprises under a double hierarchy hesitant fuzzy linguistic environment, Fuzzy Optim. Decis. Ma., 22 (2023), 23–49. https://doi.org/10.1007/s10700-022-09382-3 doi: 10.1007/s10700-022-09382-3
![]() |
[49] |
X. Gou, Z. Xu, H. Liao, F. Herrera, Probabilistic double hierarchy linguistic term set and its use in designing an improved VIKOR method: The application in smart healthcare, J. Oper. Res. Soc., 72 (2021), 2611–2630. https://doi.org/10.1080/01605682.2020.1806741 doi: 10.1080/01605682.2020.1806741
![]() |
[50] |
X. Gou, Z. Xu, H. Liao, Hesitant fuzzy linguistic entropy and cross-entropy measures and alternative queuing method for multiple criteria decision making, Inf. Sci., 388 (2017), 225–246. https://doi.org/10.1016/j.ins.2017.01.033 doi: 10.1016/j.ins.2017.01.033
![]() |
[51] | X. Gou, X. Xu, F. Deng, W. Zhou, E. Herrera-Viedma, Correction: Medical health resources allocation evaluation in public health emergencies by an improved ORESTE method with linguistic preference orderings, Fuzzy Optim. Decis. Ma., 2023. https://doi.org/10.1007/s10700-023-09409-3 |
[52] |
J. Aczel, C. Alsina, Characterizations of some classes of quasilinear functions with applications to triangular norms and synthesizing judgments, Aequationes Math., 25 (1982), 313–315. https://doi.org/10.1007/BF02189626 doi: 10.1007/BF02189626
![]() |
[53] |
T. Senapati, G. Chen, R. R. Yager, Aczel-Alsina aggregation operators and their application to intuitionistic fuzzy multiple attribute decision making, Intel. J. Fuzzy. Syst., 37 (2022), 1529–1551. https://doi.org/10.1002/int.22684 doi: 10.1002/int.22684
![]() |
[54] |
T. Senapati, G. Chen, R. Mesiar, R. R. Yager, Novel Aczel-Alsina operations-based interval-valued intuitionistic fuzzy aggregation operators and their applications in multiple attribute decision-making process, Intel. J. Fuzzy Syst., 37 (2022), 5059–5081. https://doi.org/10.1002/int.22751 doi: 10.1002/int.22751
![]() |
[55] |
T. Senapati, Approaches to multi-attribute decision-making based on picture fuzzy Aczel-Alsina average aggregation operators, Comput. Appl. Math., 41 (2022), 40. https://doi.org/10.1007/s40314-021-01742-w doi: 10.1007/s40314-021-01742-w
![]() |
[56] | A. Hussain, K. Ullah, M. S. Yang, D. Pamucar, Aczel-Alsina aggregation operators on T-spherical fuzzy (TSF) information with application to TSF multi-attribute decision making, IEEE Access, 10 (2022), 26011–26023. |
[57] | W. Ali, T. Shaheen, I. U. Haq, H. Toor, F. Akram, H. Garg, et al., Aczel-Alsina-based aggregation operators for intuitionistic hesitant fuzzy set environment and their application to multiple attribute decision-making process, AIMS Math., 8 (2023), 18021–18039. https://doi.org/10.3934/math.2023916 |
[58] |
M. Palanikumar, N. Kausar, H. Garg, S. F. Ahmed, C. Samaniego, Robot sensors process based on generalized Fermatean normal different aggregation operator's framework, AIMS Math., 8 (2023), 16252–16277. https://doi.org/10.3934/math.2023832 doi: 10.3934/math.2023832
![]() |
[59] |
J. Ahmmad, T. Mahmood, R. Chinram, A. Iampan, Some average aggregation operators based on spherical fuzzy soft sets and their applications in multi-criteria decision making, AIMS Math., 6 (2021), 7798–7833. https://doi.org/10.3934/math.2021454 doi: 10.3934/math.2021454
![]() |
[60] |
J. Zhan, J. Deng, Z. Xu, L. Martínez, A three-way decision methodology with regret theory via triangular fuzzy numbers in incomplete multi-scale decision information systems, IEEE T. Fuzzy Syst., 31 (2023), 2773–2787. https://doi.org/10.1109/TFUZZ.2023.3237646 doi: 10.1109/TFUZZ.2023.3237646
![]() |
[61] |
J. Zhu, X. Ma, G. Kou, E. Herrera-Viedma, J. Zhan, A three-way consensus model with regret theory under the framework of probabilistic linguistic term sets, Inform. Fusion, 95 (2023), 250–274. https://doi.org/10.1016/j.inffus.2023.02.029 doi: 10.1016/j.inffus.2023.02.029
![]() |
1. | Zeeshan Ali, Khumara Ashraf, Naila Siddique, Sarbast Moslem, Tapan Senapati, The Selection of Industry 4.0 Technologies Through Aczel-Alsina Information Based on Circular Bipolar Complex Fuzzy Uncertainty: An Operational Perspective, 2024, 12, 2169-3536, 85027, 10.1109/ACCESS.2024.3407929 | |
2. | Nazmiye Gonul Bilgin, Gurel Bozma, Muhammad Riaz, Location selection criteria for a military base in border region using N-AHP method, 2024, 9, 2473-6988, 7529, 10.3934/math.2024365 | |
3. | Bibhuti Bhusana Meher, Jeevaraj S, Interval-valued fermatean fuzzy Aczel-Alsina geometric aggregation operators and their applications to group decision-making, 2024, 99, 0031-8949, 095027, 10.1088/1402-4896/ad6fda | |
4. | Ghous Ali, Kholood Alsager, Asad Ali, Novel linguistic $ q $-rung orthopair fuzzy Aczel-Alsina aggregation operators for group decision-making with applications, 2024, 9, 2473-6988, 32328, 10.3934/math.20241551 | |
5. | Zia Ur Rahman, Ghous Ali, Muhammad Asif, Yufeng Chen, Muhammad Zain Ul Abidin, Identification of desalination and wind power plants sites using m-polar fuzzy Aczel–Alsina aggregation information, 2024, 14, 2045-2322, 10.1038/s41598-023-50397-6 | |
6. | Lijun Ma, Zeeshan Ali, Shi Yin, Van Thanh Tien Nguyen, Implementation of Learning Management Systems (LMS) in higher education systems through bipolar complex hesitant fuzzy Aczel-Alsina power aggregation operators: A case review for China, 2024, 19, 1932-6203, e0300317, 10.1371/journal.pone.0300317 | |
7. | Ghous Ali, Kholood Alsager, Novel Heronian mean based $ m $-polar fuzzy power geometric aggregation operators and their application to urban transportation management, 2024, 9, 2473-6988, 34109, 10.3934/math.20241626 |
\mathbb{C}{\text{}}_{\bf{1}} | \mathbb{C}{\text{}}_{\bf{2}} | \mathbb{C}{\text{}}_{\bf{3}} | \mathbb{C}{\text{}}_{\bf{4}} | |
⊮{\text{}}_{\bf{1}} | \left(\begin{array}{c}0.19+\iota 0.45\\ -0.69-\iota 0.51\end{array}\right) | \left(\begin{array}{c}0.45+\iota 0.59\\ -0.89-\iota 0.90\end{array}\right) | \left(\begin{array}{c}0.23+\iota 0.49\\ -0.39-\iota 0.57\end{array}\right) | \left(\begin{array}{c}0.76+\iota 0.90\\ -0.69-\iota 0.51\end{array}\right) |
⊮{\text{}}_{\bf{2}} | \left(\begin{array}{c}0.13+\iota 0.49\\ -0.89-\iota 0.56\end{array}\right) | \left(\begin{array}{c}0.45+\iota 0.54\\ -0.60-\iota 0.15\end{array}\right) | \left(\begin{array}{c}0.04+\iota 0.50\\ -0.99-\iota 0.88\end{array}\right) | \left(\begin{array}{c}0.11+\iota 0.15\\ -0.79-\iota 0.71\end{array}\right) |
⊮{\text{}}_{\bf{3}} | \left(\begin{array}{c}0.21+\iota 0.42\\ -0.83-\iota 0.67\end{array}\right) | \left(\begin{array}{c}0.17+\iota 0.49\\ -0.69-\iota 0.71\end{array}\right) | \left(\begin{array}{c}0.17+\iota 0.09\\ -0.58-\iota 0.21\end{array}\right) | \left(\begin{array}{c}0.33+\iota 0.46\\ -0.29-\iota 0.59\end{array}\right) |
⊮{\text{}}_{\bf{4}} | \left(\begin{array}{c}0.35+\iota 0.44\\ -0.67-\iota 0.59\end{array}\right) | \left(\begin{array}{c}0.28+\iota 0.55\\ -0.42-\iota 0.58\end{array}\right) | \left(\begin{array}{c}0.36+\iota 0.48\\ -0.89-\iota 0.66\end{array}\right) | \left(\begin{array}{c}0.88+\iota 0.12\\ -0.29-\iota 0.31\end{array}\right) |
\mathbb{C}{\text{}}_{\bf{1}} | \mathbb{C}{\text{}}_{\bf{2}} | \mathbb{C}{\text{}}_{\bf{3}} | \mathbb{C}{\text{}}_{\bf{4}} | |
⊮{\text{}}_{\bf{1}} | \left(\begin{array}{c}0.34+\iota 0.46\\ 0.88-\iota 0.01\end{array}\right) | \left(\begin{array}{c}0.67+\iota 0.09\\ -0.06-\iota 0.98\end{array}\right) | \left(\begin{array}{c}0.22+\iota 0.41\\ -0.32-\iota 0.53\end{array}\right) | \left(\begin{array}{c}0.15+\iota 0.91\\ -0.65-\iota 0.52\end{array}\right) |
⊮{\text{}}_{\bf{2}} | \left(\begin{array}{c}0.23+\iota 0.79\\ -0.49-\iota 0.06\end{array}\right) | \left(\begin{array}{c}0.78+\iota 0.24\\ -0.20-\iota 0.55\end{array}\right) | \left(\begin{array}{c}0.23+\iota 0.54\\ -0.89-\iota 0.38\end{array}\right) | \left(\begin{array}{c}0.17+\iota 0.65\\ -0.89-\iota 0.51\end{array}\right) |
⊮{\text{}}_{\bf{3}} | \left(\begin{array}{c}0.22+\iota 0.47\\ -0.55-\iota 0.31\end{array}\right) | \left(\begin{array}{c}0.14+\iota 0.20\\ -0.29-\iota 0.11\end{array}\right) | \left(\begin{array}{c}0.17+\iota 0.33\\ -0.58-\iota 0.24\end{array}\right) | \left(\begin{array}{c}0.20+\iota 0.49\\ -0.29-\iota 0.51\end{array}\right) |
⊮{\text{}}_{\bf{4}} | \left(\begin{array}{c}0.19+\iota 0.56\\ -0.60-\iota 0.90\end{array}\right) | \left(\begin{array}{c}0.28+\iota 0.25\\ -0.92-\iota 0.68\end{array}\right) | \left(\begin{array}{c}0.48+\iota 0.44\\ -0.63-\iota 0.66\end{array}\right) | \left(\begin{array}{c}0.88+\iota 0.18\\ -0.59-\iota 0.33\end{array}\right) |
\mathbb{C}{\text{}}_{\bf{1}} | \mathbb{C}{\text{}}_{\bf{2}} | \mathbb{C}{\text{}}_{\bf{3}} | \mathbb{C}{\text{}}_{\bf{4}} | |
⊮{\text{}}_{\bf{1}} | \left(\begin{array}{c}0.16+\iota 0.45\\ -0.79-\iota 0.51\end{array}\right) | \left(\begin{array}{c}0.12+\iota 0.19\\ -0.59-\iota 0.93\end{array}\right) | \left(\begin{array}{c}0.56+\iota 0.33\\ -0.39-\iota 0.59\end{array}\right) | \left(\begin{array}{c}0.60+\iota 0.70\\ -0.13-\iota 0.22\end{array}\right) |
⊮{\text{}}_{\bf{2}} | \left(\begin{array}{c}0.70+\iota 0.89\\ -0.89-\iota 0.26\end{array}\right) | \left(\begin{array}{c}0.45+\iota 0.30\\ -0.50-\iota 0.75\end{array}\right) | \left(\begin{array}{c}0.05+\iota 0.59\\ -0.94-\iota 0.32\end{array}\right) | \left(\begin{array}{c}0.15+\iota 0.61\\ -0.99-\iota 0.11\end{array}\right) |
⊮{\text{}}_{\bf{3}} | \left(\begin{array}{c}0.22+\iota 0.23\\ -0.85-\iota 0.27\end{array}\right) | \left(\begin{array}{c}0.74+\iota 0.69\\ -0.69-\iota 0.71\end{array}\right) | \left(\begin{array}{c}0.11+\iota 0.19\\ -0.58-\iota 0.71\end{array}\right) | \left(\begin{array}{c}0.66+\iota 0.46\\ -0.49-\iota 0.39\end{array}\right) |
⊮{\text{}}_{\bf{4}} | \left(\begin{array}{c}0.27+\iota 0.45\\ -0.77-\iota 0.54\end{array}\right) | \left(\begin{array}{c}0.78+\iota 0.15\\ -0.22-\iota 0.59\end{array}\right) | \left(\begin{array}{c}0.36+\iota 0.83\\ -0.77-\iota 0.90\end{array}\right) | \left(\begin{array}{c}0.81+\iota 0.37\\ -0.69-\iota 0.71\end{array}\right) |
Alternatives/attributes | \mathbb{C}{\text{}}_{\bf{1}} | \mathbb{C}{\text{}}_{\bf{2}} | \mathbb{C}{\text{}}_{\bf{3}} | \mathbb{C}{\text{}}_{\bf{4}} |
⊮{\text{}}_{\bf{1}} | \left(\begin{array}{c}0.8+\iota 0.94\\ -0.23-\iota 0.03\end{array}\right) | \left(\begin{array}{c}0.87+\iota 0.77\\ -0.18-\iota 0.58\end{array}\right) | \left(\begin{array}{c}0.85+\iota 0.93\\ -0.02-\iota 0.06\end{array}\right) | \left(\begin{array}{c}0.88+\iota 0.99\\ -0.08-\iota 0.04\end{array}\right) |
⊮{\text{}}_{\bf{2}} | \left(\begin{array}{c}0.79+\iota 0.98\\ -0.27-\iota 0.03\end{array}\right) | \left(\begin{array}{c}0.96+\iota 0.89\\ -0.04-\iota 0.07\end{array}\right) | \left(\begin{array}{c}0.51+\iota 0.96\\ -0.68-\iota 0.17\end{array}\right) | \left(\begin{array}{c}0.69+\iota 0.86\\ -0.53-\iota 0.07\end{array}\right) |
⊮{\text{}}_{\bf{3}} | \left(\begin{array}{c}0.08+\iota 0.91\\ -0.20-\iota 0.05\end{array}\right) | \left(\begin{array}{c}0.78+\iota 0.90\\ -0.08-\iota 0.09\end{array}\right) | \left(\begin{array}{c}0.71+\iota 0.72\\ -0.07-\iota 0.04\end{array}\right) | \left(\begin{array}{c}0.87+\iota 0.95\\ -0.02-\iota 0.05\end{array}\right) |
⊮{\text{}}_{\bf{4}} | \left(\begin{array}{c}0.84+\iota 0.95\\ -0.12-\iota 0.20\end{array}\right) | \left(\begin{array}{c}0.89+\iota 0.85\\ -0.20-\iota 0.09\end{array}\right) | \left(\begin{array}{c}0.92+\iota 0.96\\ -0.23-\iota 0.19\end{array}\right) | \left(\begin{array}{c}0.99+\iota 0.75\\ -0.06-\iota 0.05\end{array}\right) |
Alternatives/attributes | \mathbb{C}{\text{}}_{\bf{1}} | \mathbb{C}{\text{}}_{\bf{2}} | \mathbb{C}{\text{}}_{\bf{3}} | \mathbb{C}{\text{}}_{\bf{4}} |
⊮{\text{}}_{\bf{1}} | \left(\begin{array}{c}0.09+\iota 0.26\\ -0.92-\iota 0.63\end{array}\right) | \left(\begin{array}{c}0.11+\iota 0.06\\ -0.92-\iota 0.99\end{array}\right) | \left(\begin{array}{c}0.07+\iota 0.12\\ -0.75-\iota 0.86\end{array}\right) | \left(\begin{array}{c}0.04+\iota 0.18\\ -0.94-\iota 0.91\end{array}\right) |
⊮{\text{}}_{\bf{2}} | \left(\begin{array}{c}0.09+\iota 0.44\\ -0.94-\iota 0.63\end{array}\right) | \left(\begin{array}{c}0.25+\iota 0.13\\ -0.75-\iota 0.82\end{array}\right) | \left(\begin{array}{c}0.01+\iota 0.18\\ -0.99-\iota 0.94\end{array}\right) | \left(\begin{array}{c}0.01+\iota 0.04\\ -0.99-\iota 0.94\end{array}\right) |
⊮{\text{}}_{\bf{3}} | \left(\begin{array}{c}0.09+\iota 0.02\\ -0.91-\iota 0.71\end{array}\right) | \left(\begin{array}{c}0.06+\iota 0.14\\ -0.83-\iota 0.85\end{array}\right) | \left(\begin{array}{c}0.03+\iota 0.03\\ -0.88-\iota 0.82\end{array}\right) | \left(\begin{array}{c}0.04+\iota 0.08\\ -0.87-\iota 0.92\end{array}\right) |
⊮{\text{}}_{\bf{4}} | \left(\begin{array}{c}0.12+\iota 0.29\\ -0.84-\iota 0.91\end{array}\right) | \left(\begin{array}{c}0.13+\iota 0.10\\ -0.93-\iota 0.85\end{array}\right) | \left(\begin{array}{c}0.11+\iota 0.18\\ -0.96-\iota 0.95\end{array}\right) | \left(\begin{array}{c}0.18+\iota 0.02\\ -0.93-\iota 0.92\end{array}\right) |
Alternatives | \mathbb{C}{\text{}}_{\bf{1}} | \mathbb{C}{\text{}}_{\bf{2}} | \mathbb{C}{\text{}}_{\bf{3}} | \mathbb{C}{\text{}}_{\bf{4}} |
⊮{\text{}}_{\bf{1}} | −0.60 | −0.60 | −0.40 | −0.41 |
⊮{\text{}}_{\bf{2}} | −0.50 | −0.50 | −0.50 | −0.47 |
⊮{\text{}}_{\bf{3}} | −0.60 | −0.50 | −0.50 | −0.42 |
⊮{\text{}}_{\bf{4}} | −0.60 | −0.50 | −0.50 | −0.43 |
Methods | {\mathit{\gimel }}_{\mathbb{S}\mathbb{F}}\left(⊮{\text{}}_{\bf{1}}\right) | {\mathit{\gimel }}_{\mathbb{S}\mathbb{F}}\left(⊮{\text{}}_{\bf{2}}\right) | {\mathit{\gimel }}_{\mathbb{S}\mathbb{F}}\left(⊮{\text{}}_{\bf{3}}\right) | {\mathit{\gimel }}_{\mathbb{S}\mathbb{F}}\left(⊮{\text{}}_{\bf{4}}\right) | Ranking |
Wei et al. [19] method | ⤰\rightsquigarrow ⤰\rightsquigarrow ⤰ | ⤰\rightsquigarrow ⤰\rightsquigarrow ⤰ | ⤰\rightsquigarrow ⤰\rightsquigarrow ⤰ | ⤰\rightsquigarrow ⤰\rightsquigarrow ⤰ | ⤰\rightsquigarrow ⤰\rightsquigarrow ⤰ |
Jana et al. [20] method | ⤰\rightsquigarrow ⤰\rightsquigarrow ⤰ | ⤰\rightsquigarrow ⤰\rightsquigarrow ⤰ | ⤰\rightsquigarrow ⤰\rightsquigarrow ⤰ | ⤰\rightsquigarrow ⤰\rightsquigarrow ⤰ | ⤰\rightsquigarrow ⤰\rightsquigarrow ⤰ |
Jana et al. [33] method | ⤰\rightsquigarrow ⤰\rightsquigarrow ⤰ | ⤰\rightsquigarrow ⤰\rightsquigarrow ⤰ | ⤰\rightsquigarrow ⤰\rightsquigarrow ⤰ | ⤰\rightsquigarrow ⤰\rightsquigarrow ⤰ | ⤰\rightsquigarrow ⤰\rightsquigarrow ⤰ |
Riaz and Tehrim. [34] | ⤰\rightsquigarrow ⤰\rightsquigarrow ⤰ | ⤰\rightsquigarrow ⤰\rightsquigarrow ⤰ | ⤰\rightsquigarrow ⤰\rightsquigarrow ⤰ | ⤰\rightsquigarrow ⤰\rightsquigarrow ⤰ | ⤰\rightsquigarrow ⤰\rightsquigarrow ⤰ |
Bi et al. [38] method | ⤰\rightsquigarrow ⤰\rightsquigarrow ⤰ | ⤰\rightsquigarrow ⤰\rightsquigarrow ⤰ | ⤰\rightsquigarrow ⤰\rightsquigarrow ⤰ | ⤰\rightsquigarrow ⤰\rightsquigarrow ⤰ | ⤰\rightsquigarrow ⤰\rightsquigarrow ⤰ |
Bi et al. [39] method | ⤰\rightsquigarrow ⤰\rightsquigarrow ⤰ | ⤰\rightsquigarrow ⤰\rightsquigarrow ⤰ | ⤰\rightsquigarrow ⤰\rightsquigarrow ⤰ | ⤰\rightsquigarrow ⤰\rightsquigarrow ⤰ | ⤰\rightsquigarrow ⤰\rightsquigarrow ⤰ |
BCFAAWG | 0.985 | 0.977 | 0.994 | 0.995 | ⊮{\text{}}_{4}\succ ⊮{\text{}}_{3}\succ ⊮{\text{}}_{1}\succ ⊮{\text{}}_{2} |
Sources | Method | The distance between alternative and BAA added together | Ranking |
Varma [44] | Fuzzy MABAC | ⤰\rightsquigarrow ⤰\rightsquigarrow ⤰ | ⤰\rightsquigarrow ⤰\rightsquigarrow ⤰ |
Jana [47] | BF MABAC | ⤰\rightsquigarrow ⤰\rightsquigarrow ⤰ | ⤰\rightsquigarrow ⤰\rightsquigarrow ⤰ |
Invented work | BCF MABAC | {\mathcal{S}}_{1}=-2.01, {\mathcal{S}}_{2}=-1.97 {\mathcal{S}}_{3}=-2.02, {\mathcal{S}}_{4}=-2.03 |
⊮{\text{}}_{2}\succ ⊮{\text{}}_{1}\succ ⊮{\text{}}_{3}\succ ⊮{\text{}}_{4} |
Name | Abbreviation |
Fuzzy set | FS |
Bipolar fuzzy set | BFS |
Complex fuzzy set | CFS |
Bipolar complex fuzzy sets | BCFSs |
Decision making | DM |
Multi-attribute decision making | MADM |
Multi-Criteria decision making | MCDM |
Aczel Alsina | AA |
Operating System | OS |
Multi-attribute border approximation area comparison | MABAC |
Aggregation operators | AOs |
Bipolar complex fuzzy Aczel Alsina weighted averaging | BCFAAWA |
Bipolar complex fuzzy Aczel Alsina weighted geometric | BCFAAWG |
Bipolar complex fuzzy Aczel Alsina ordered weighted geometric | BCFAAOWG |
Bipolar complex fuzzy Aczel Alsina hybrid geometric | BCFAAHG |
\mathbb{C}{\text{}}_{\bf{1}} | \mathbb{C}{\text{}}_{\bf{2}} | \mathbb{C}{\text{}}_{\bf{3}} | \mathbb{C}{\text{}}_{\bf{4}} | |
⊮{\text{}}_{\bf{1}} | \left(\begin{array}{c}0.19+\iota 0.45\\ -0.69-\iota 0.51\end{array}\right) | \left(\begin{array}{c}0.45+\iota 0.59\\ -0.89-\iota 0.90\end{array}\right) | \left(\begin{array}{c}0.23+\iota 0.49\\ -0.39-\iota 0.57\end{array}\right) | \left(\begin{array}{c}0.76+\iota 0.90\\ -0.69-\iota 0.51\end{array}\right) |
⊮{\text{}}_{\bf{2}} | \left(\begin{array}{c}0.13+\iota 0.49\\ -0.89-\iota 0.56\end{array}\right) | \left(\begin{array}{c}0.45+\iota 0.54\\ -0.60-\iota 0.15\end{array}\right) | \left(\begin{array}{c}0.04+\iota 0.50\\ -0.99-\iota 0.88\end{array}\right) | \left(\begin{array}{c}0.11+\iota 0.15\\ -0.79-\iota 0.71\end{array}\right) |
⊮{\text{}}_{\bf{3}} | \left(\begin{array}{c}0.21+\iota 0.42\\ -0.83-\iota 0.67\end{array}\right) | \left(\begin{array}{c}0.17+\iota 0.49\\ -0.69-\iota 0.71\end{array}\right) | \left(\begin{array}{c}0.17+\iota 0.09\\ -0.58-\iota 0.21\end{array}\right) | \left(\begin{array}{c}0.33+\iota 0.46\\ -0.29-\iota 0.59\end{array}\right) |
⊮{\text{}}_{\bf{4}} | \left(\begin{array}{c}0.35+\iota 0.44\\ -0.67-\iota 0.59\end{array}\right) | \left(\begin{array}{c}0.28+\iota 0.55\\ -0.42-\iota 0.58\end{array}\right) | \left(\begin{array}{c}0.36+\iota 0.48\\ -0.89-\iota 0.66\end{array}\right) | \left(\begin{array}{c}0.88+\iota 0.12\\ -0.29-\iota 0.31\end{array}\right) |
\mathbb{C}{\text{}}_{\bf{1}} | \mathbb{C}{\text{}}_{\bf{2}} | \mathbb{C}{\text{}}_{\bf{3}} | \mathbb{C}{\text{}}_{\bf{4}} | |
⊮{\text{}}_{\bf{1}} | \left(\begin{array}{c}0.34+\iota 0.46\\ 0.88-\iota 0.01\end{array}\right) | \left(\begin{array}{c}0.67+\iota 0.09\\ -0.06-\iota 0.98\end{array}\right) | \left(\begin{array}{c}0.22+\iota 0.41\\ -0.32-\iota 0.53\end{array}\right) | \left(\begin{array}{c}0.15+\iota 0.91\\ -0.65-\iota 0.52\end{array}\right) |
⊮{\text{}}_{\bf{2}} | \left(\begin{array}{c}0.23+\iota 0.79\\ -0.49-\iota 0.06\end{array}\right) | \left(\begin{array}{c}0.78+\iota 0.24\\ -0.20-\iota 0.55\end{array}\right) | \left(\begin{array}{c}0.23+\iota 0.54\\ -0.89-\iota 0.38\end{array}\right) | \left(\begin{array}{c}0.17+\iota 0.65\\ -0.89-\iota 0.51\end{array}\right) |
⊮{\text{}}_{\bf{3}} | \left(\begin{array}{c}0.22+\iota 0.47\\ -0.55-\iota 0.31\end{array}\right) | \left(\begin{array}{c}0.14+\iota 0.20\\ -0.29-\iota 0.11\end{array}\right) | \left(\begin{array}{c}0.17+\iota 0.33\\ -0.58-\iota 0.24\end{array}\right) | \left(\begin{array}{c}0.20+\iota 0.49\\ -0.29-\iota 0.51\end{array}\right) |
⊮{\text{}}_{\bf{4}} | \left(\begin{array}{c}0.19+\iota 0.56\\ -0.60-\iota 0.90\end{array}\right) | \left(\begin{array}{c}0.28+\iota 0.25\\ -0.92-\iota 0.68\end{array}\right) | \left(\begin{array}{c}0.48+\iota 0.44\\ -0.63-\iota 0.66\end{array}\right) | \left(\begin{array}{c}0.88+\iota 0.18\\ -0.59-\iota 0.33\end{array}\right) |
\mathbb{C}{\text{}}_{\bf{1}} | \mathbb{C}{\text{}}_{\bf{2}} | \mathbb{C}{\text{}}_{\bf{3}} | \mathbb{C}{\text{}}_{\bf{4}} | |
⊮{\text{}}_{\bf{1}} | \left(\begin{array}{c}0.16+\iota 0.45\\ -0.79-\iota 0.51\end{array}\right) | \left(\begin{array}{c}0.12+\iota 0.19\\ -0.59-\iota 0.93\end{array}\right) | \left(\begin{array}{c}0.56+\iota 0.33\\ -0.39-\iota 0.59\end{array}\right) | \left(\begin{array}{c}0.60+\iota 0.70\\ -0.13-\iota 0.22\end{array}\right) |
⊮{\text{}}_{\bf{2}} | \left(\begin{array}{c}0.70+\iota 0.89\\ -0.89-\iota 0.26\end{array}\right) | \left(\begin{array}{c}0.45+\iota 0.30\\ -0.50-\iota 0.75\end{array}\right) | \left(\begin{array}{c}0.05+\iota 0.59\\ -0.94-\iota 0.32\end{array}\right) | \left(\begin{array}{c}0.15+\iota 0.61\\ -0.99-\iota 0.11\end{array}\right) |
⊮{\text{}}_{\bf{3}} | \left(\begin{array}{c}0.22+\iota 0.23\\ -0.85-\iota 0.27\end{array}\right) | \left(\begin{array}{c}0.74+\iota 0.69\\ -0.69-\iota 0.71\end{array}\right) | \left(\begin{array}{c}0.11+\iota 0.19\\ -0.58-\iota 0.71\end{array}\right) | \left(\begin{array}{c}0.66+\iota 0.46\\ -0.49-\iota 0.39\end{array}\right) |
⊮{\text{}}_{\bf{4}} | \left(\begin{array}{c}0.27+\iota 0.45\\ -0.77-\iota 0.54\end{array}\right) | \left(\begin{array}{c}0.78+\iota 0.15\\ -0.22-\iota 0.59\end{array}\right) | \left(\begin{array}{c}0.36+\iota 0.83\\ -0.77-\iota 0.90\end{array}\right) | \left(\begin{array}{c}0.81+\iota 0.37\\ -0.69-\iota 0.71\end{array}\right) |
Alternatives/attributes | \mathbb{C}{\text{}}_{\bf{1}} | \mathbb{C}{\text{}}_{\bf{2}} | \mathbb{C}{\text{}}_{\bf{3}} | \mathbb{C}{\text{}}_{\bf{4}} |
⊮{\text{}}_{\bf{1}} | \left(\begin{array}{c}0.8+\iota 0.94\\ -0.23-\iota 0.03\end{array}\right) | \left(\begin{array}{c}0.87+\iota 0.77\\ -0.18-\iota 0.58\end{array}\right) | \left(\begin{array}{c}0.85+\iota 0.93\\ -0.02-\iota 0.06\end{array}\right) | \left(\begin{array}{c}0.88+\iota 0.99\\ -0.08-\iota 0.04\end{array}\right) |
⊮{\text{}}_{\bf{2}} | \left(\begin{array}{c}0.79+\iota 0.98\\ -0.27-\iota 0.03\end{array}\right) | \left(\begin{array}{c}0.96+\iota 0.89\\ -0.04-\iota 0.07\end{array}\right) | \left(\begin{array}{c}0.51+\iota 0.96\\ -0.68-\iota 0.17\end{array}\right) | \left(\begin{array}{c}0.69+\iota 0.86\\ -0.53-\iota 0.07\end{array}\right) |
⊮{\text{}}_{\bf{3}} | \left(\begin{array}{c}0.08+\iota 0.91\\ -0.20-\iota 0.05\end{array}\right) | \left(\begin{array}{c}0.78+\iota 0.90\\ -0.08-\iota 0.09\end{array}\right) | \left(\begin{array}{c}0.71+\iota 0.72\\ -0.07-\iota 0.04\end{array}\right) | \left(\begin{array}{c}0.87+\iota 0.95\\ -0.02-\iota 0.05\end{array}\right) |
⊮{\text{}}_{\bf{4}} | \left(\begin{array}{c}0.84+\iota 0.95\\ -0.12-\iota 0.20\end{array}\right) | \left(\begin{array}{c}0.89+\iota 0.85\\ -0.20-\iota 0.09\end{array}\right) | \left(\begin{array}{c}0.92+\iota 0.96\\ -0.23-\iota 0.19\end{array}\right) | \left(\begin{array}{c}0.99+\iota 0.75\\ -0.06-\iota 0.05\end{array}\right) |
Alternatives/attributes | \mathbb{C}{\text{}}_{\bf{1}} | \mathbb{C}{\text{}}_{\bf{2}} | \mathbb{C}{\text{}}_{\bf{3}} | \mathbb{C}{\text{}}_{\bf{4}} |
⊮{\text{}}_{\bf{1}} | \left(\begin{array}{c}0.09+\iota 0.26\\ -0.92-\iota 0.63\end{array}\right) | \left(\begin{array}{c}0.11+\iota 0.06\\ -0.92-\iota 0.99\end{array}\right) | \left(\begin{array}{c}0.07+\iota 0.12\\ -0.75-\iota 0.86\end{array}\right) | \left(\begin{array}{c}0.04+\iota 0.18\\ -0.94-\iota 0.91\end{array}\right) |
⊮{\text{}}_{\bf{2}} | \left(\begin{array}{c}0.09+\iota 0.44\\ -0.94-\iota 0.63\end{array}\right) | \left(\begin{array}{c}0.25+\iota 0.13\\ -0.75-\iota 0.82\end{array}\right) | \left(\begin{array}{c}0.01+\iota 0.18\\ -0.99-\iota 0.94\end{array}\right) | \left(\begin{array}{c}0.01+\iota 0.04\\ -0.99-\iota 0.94\end{array}\right) |
⊮{\text{}}_{\bf{3}} | \left(\begin{array}{c}0.09+\iota 0.02\\ -0.91-\iota 0.71\end{array}\right) | \left(\begin{array}{c}0.06+\iota 0.14\\ -0.83-\iota 0.85\end{array}\right) | \left(\begin{array}{c}0.03+\iota 0.03\\ -0.88-\iota 0.82\end{array}\right) | \left(\begin{array}{c}0.04+\iota 0.08\\ -0.87-\iota 0.92\end{array}\right) |
⊮{\text{}}_{\bf{4}} | \left(\begin{array}{c}0.12+\iota 0.29\\ -0.84-\iota 0.91\end{array}\right) | \left(\begin{array}{c}0.13+\iota 0.10\\ -0.93-\iota 0.85\end{array}\right) | \left(\begin{array}{c}0.11+\iota 0.18\\ -0.96-\iota 0.95\end{array}\right) | \left(\begin{array}{c}0.18+\iota 0.02\\ -0.93-\iota 0.92\end{array}\right) |
Alternatives | \mathbb{C}{\text{}}_{\bf{1}} | \mathbb{C}{\text{}}_{\bf{2}} | \mathbb{C}{\text{}}_{\bf{3}} | \mathbb{C}{\text{}}_{\bf{4}} |
⊮{\text{}}_{\bf{1}} | −0.60 | −0.60 | −0.40 | −0.41 |
⊮{\text{}}_{\bf{2}} | −0.50 | −0.50 | −0.50 | −0.47 |
⊮{\text{}}_{\bf{3}} | −0.60 | −0.50 | −0.50 | −0.42 |
⊮{\text{}}_{\bf{4}} | −0.60 | −0.50 | −0.50 | −0.43 |
Methods | {\mathit{\gimel }}_{\mathbb{S}\mathbb{F}}\left(⊮{\text{}}_{\bf{1}}\right) | {\mathit{\gimel }}_{\mathbb{S}\mathbb{F}}\left(⊮{\text{}}_{\bf{2}}\right) | {\mathit{\gimel }}_{\mathbb{S}\mathbb{F}}\left(⊮{\text{}}_{\bf{3}}\right) | {\mathit{\gimel }}_{\mathbb{S}\mathbb{F}}\left(⊮{\text{}}_{\bf{4}}\right) | Ranking |
Wei et al. [19] method | ⤰\rightsquigarrow ⤰\rightsquigarrow ⤰ | ⤰\rightsquigarrow ⤰\rightsquigarrow ⤰ | ⤰\rightsquigarrow ⤰\rightsquigarrow ⤰ | ⤰\rightsquigarrow ⤰\rightsquigarrow ⤰ | ⤰\rightsquigarrow ⤰\rightsquigarrow ⤰ |
Jana et al. [20] method | ⤰\rightsquigarrow ⤰\rightsquigarrow ⤰ | ⤰\rightsquigarrow ⤰\rightsquigarrow ⤰ | ⤰\rightsquigarrow ⤰\rightsquigarrow ⤰ | ⤰\rightsquigarrow ⤰\rightsquigarrow ⤰ | ⤰\rightsquigarrow ⤰\rightsquigarrow ⤰ |
Jana et al. [33] method | ⤰\rightsquigarrow ⤰\rightsquigarrow ⤰ | ⤰\rightsquigarrow ⤰\rightsquigarrow ⤰ | ⤰\rightsquigarrow ⤰\rightsquigarrow ⤰ | ⤰\rightsquigarrow ⤰\rightsquigarrow ⤰ | ⤰\rightsquigarrow ⤰\rightsquigarrow ⤰ |
Riaz and Tehrim. [34] | ⤰\rightsquigarrow ⤰\rightsquigarrow ⤰ | ⤰\rightsquigarrow ⤰\rightsquigarrow ⤰ | ⤰\rightsquigarrow ⤰\rightsquigarrow ⤰ | ⤰\rightsquigarrow ⤰\rightsquigarrow ⤰ | ⤰\rightsquigarrow ⤰\rightsquigarrow ⤰ |
Bi et al. [38] method | ⤰\rightsquigarrow ⤰\rightsquigarrow ⤰ | ⤰\rightsquigarrow ⤰\rightsquigarrow ⤰ | ⤰\rightsquigarrow ⤰\rightsquigarrow ⤰ | ⤰\rightsquigarrow ⤰\rightsquigarrow ⤰ | ⤰\rightsquigarrow ⤰\rightsquigarrow ⤰ |
Bi et al. [39] method | ⤰\rightsquigarrow ⤰\rightsquigarrow ⤰ | ⤰\rightsquigarrow ⤰\rightsquigarrow ⤰ | ⤰\rightsquigarrow ⤰\rightsquigarrow ⤰ | ⤰\rightsquigarrow ⤰\rightsquigarrow ⤰ | ⤰\rightsquigarrow ⤰\rightsquigarrow ⤰ |
BCFAAWG | 0.985 | 0.977 | 0.994 | 0.995 | ⊮{\text{}}_{4}\succ ⊮{\text{}}_{3}\succ ⊮{\text{}}_{1}\succ ⊮{\text{}}_{2} |
Sources | Method | The distance between alternative and BAA added together | Ranking |
Varma [44] | Fuzzy MABAC | ⤰\rightsquigarrow ⤰\rightsquigarrow ⤰ | ⤰\rightsquigarrow ⤰\rightsquigarrow ⤰ |
Jana [47] | BF MABAC | ⤰\rightsquigarrow ⤰\rightsquigarrow ⤰ | ⤰\rightsquigarrow ⤰\rightsquigarrow ⤰ |
Invented work | BCF MABAC | {\mathcal{S}}_{1}=-2.01, {\mathcal{S}}_{2}=-1.97 {\mathcal{S}}_{3}=-2.02, {\mathcal{S}}_{4}=-2.03 |
⊮{\text{}}_{2}\succ ⊮{\text{}}_{1}\succ ⊮{\text{}}_{3}\succ ⊮{\text{}}_{4} |
Name | Abbreviation |
Fuzzy set | FS |
Bipolar fuzzy set | BFS |
Complex fuzzy set | CFS |
Bipolar complex fuzzy sets | BCFSs |
Decision making | DM |
Multi-attribute decision making | MADM |
Multi-Criteria decision making | MCDM |
Aczel Alsina | AA |
Operating System | OS |
Multi-attribute border approximation area comparison | MABAC |
Aggregation operators | AOs |
Bipolar complex fuzzy Aczel Alsina weighted averaging | BCFAAWA |
Bipolar complex fuzzy Aczel Alsina weighted geometric | BCFAAWG |
Bipolar complex fuzzy Aczel Alsina ordered weighted geometric | BCFAAOWG |
Bipolar complex fuzzy Aczel Alsina hybrid geometric | BCFAAHG |