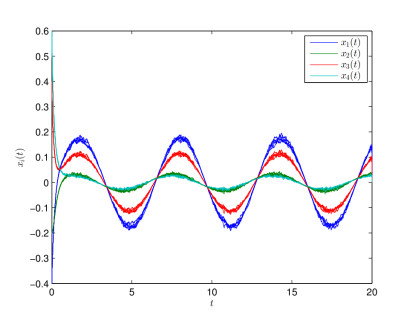
This paper discusses the exponential stability of periodic solutions for stochastic neural networks with multiple time-varying delays. For these networks, sufficient conditions in the linear matrix inequality forms are rare in the literature. We constructed an appropriate Lyapunov-Krasovskii functional to eliminate the items with multiple delays and establish some sufficient conditions in linear matrix inequality forms, to ensure exponential stability of the periodic solutions. Several examples are provided to demonstrate that our results are effective and less conservative than previous ones.
Citation: Zhigang Zhou, Li Wan, Qunjiao Zhang, Hongbo Fu, Huizhen Li, Qinghua Zhou. Exponential stability of periodic solution for stochastic neural networks involving multiple time-varying delays[J]. AIMS Mathematics, 2024, 9(6): 14932-14948. doi: 10.3934/math.2024723
[1] | Huahai Qiu, Li Wan, Zhigang Zhou, Qunjiao Zhang, Qinghua Zhou . Global exponential periodicity of nonlinear neural networks with multiple time-varying delays. AIMS Mathematics, 2023, 8(5): 12472-12485. doi: 10.3934/math.2023626 |
[2] | Qinghua Zhou, Li Wan, Hongbo Fu, Qunjiao Zhang . Exponential stability of stochastic Hopfield neural network with mixed multiple delays. AIMS Mathematics, 2021, 6(4): 4142-4155. doi: 10.3934/math.2021245 |
[3] | Li Wan, Qinghua Zhou, Hongbo Fu, Qunjiao Zhang . Exponential stability of Hopfield neural networks of neutral type with multiple time-varying delays. AIMS Mathematics, 2021, 6(8): 8030-8043. doi: 10.3934/math.2021466 |
[4] | Yanshou Dong, Junfang Zhao, Xu Miao, Ming Kang . Piecewise pseudo almost periodic solutions of interval general BAM neural networks with mixed time-varying delays and impulsive perturbations. AIMS Mathematics, 2023, 8(9): 21828-21855. doi: 10.3934/math.20231113 |
[5] | Nina Huo, Bing Li, Yongkun Li . Global exponential stability and existence of almost periodic solutions in distribution for Clifford-valued stochastic high-order Hopfield neural networks with time-varying delays. AIMS Mathematics, 2022, 7(3): 3653-3679. doi: 10.3934/math.2022202 |
[6] | Qian Cao, Xiaojin Guo . Anti-periodic dynamics on high-order inertial Hopfield neural networks involving time-varying delays. AIMS Mathematics, 2020, 5(6): 5402-5421. doi: 10.3934/math.2020347 |
[7] | Xiaofang Meng, Yongkun Li . Pseudo almost periodic solutions for quaternion-valued high-order Hopfield neural networks with time-varying delays and leakage delays on time scales. AIMS Mathematics, 2021, 6(9): 10070-10091. doi: 10.3934/math.2021585 |
[8] | Yijia Zhang, Tao Xie, Yunlong Ma . Robustness analysis of exponential stability of Cohen-Grossberg neural network with neutral terms. AIMS Mathematics, 2025, 10(3): 4938-4954. doi: 10.3934/math.2025226 |
[9] | Qinghua Zhou, Li Wan, Hongshan Wang, Hongbo Fu, Qunjiao Zhang . Exponential stability of Cohen-Grossberg neural networks with multiple time-varying delays and distributed delays. AIMS Mathematics, 2023, 8(8): 19161-19171. doi: 10.3934/math.2023978 |
[10] | Jin Gao, Lihua Dai . Weighted pseudo almost periodic solutions of octonion-valued neural networks with mixed time-varying delays and leakage delays. AIMS Mathematics, 2023, 8(6): 14867-14893. doi: 10.3934/math.2023760 |
This paper discusses the exponential stability of periodic solutions for stochastic neural networks with multiple time-varying delays. For these networks, sufficient conditions in the linear matrix inequality forms are rare in the literature. We constructed an appropriate Lyapunov-Krasovskii functional to eliminate the items with multiple delays and establish some sufficient conditions in linear matrix inequality forms, to ensure exponential stability of the periodic solutions. Several examples are provided to demonstrate that our results are effective and less conservative than previous ones.
In the past two decades, neural networks have received considerable attention, having been successfully applied in signal processing, parallel computing, and combinational optimization. In the implementation and application of neural networks, time delay is unavoidable, which may bring about instability, oscillations, bifurcation, and chaos for systems. In the application of neural networks with delays, it is often required that the networks have a unique and stable equilibrium point. Therefore, the stability analysis of equilibrium points for neural networks with delays is widely relevant [1,2,3,4,5,6,7,8,9].
Besides the stability analysis of equilibrium points, the research on neural networks with delays involves the stability analysis of periodic solutions. The properties of periodic solutions are of great interest, and these have been successfully applied in many biological and cognitive fields. For example, periodic solutions can be used to store acoustic characteristics in speech recognition, because they can contain information for a time series. In practice, a time series of audio signal is fed as input into the network if the weight of the network meet established criteria; then, the output is the periodic solution of the time series. We calculate the average power spectral density (PSD) of the periodic solution and compare it with the PSD of the original audio signal. If the difference between them is small, then the periodic solution can be considered as effectively storing the acoustic characteristics. In addition, an equilibrium point can be considered as a special periodic solution of neural networks with an arbitrary period. Therefore, the stability analysis of periodic solutions for neural networks is more general and interesting than that of equilibrium points. Recently, there have been a lot of work on the stabilization of periodic solutions; see [10,11,12,13,14,15,16,17,18].
As pointed out in [19], the synaptic transmission in real nervous systems is a noisy process brought on by random fluctuations in the release of neurotransmitters, and other probabilistic causes. Neural networks could be stabilized or destabilized by some stochastic inputs. Therefore, it is of prime importance to consider the stability of stochastic neural networks with delays [20,21,22,23,24,25,26].
The system discussed in this paper cannot be transformed into the vector-matrix form because of multiple delays τij(t). For neural networks with delays τij(t), the common methods in the literature include fixed point principles, differential inequalities, Lyapunov functional, and Halanay inequality. These methods do not include linear matrix inequalities. Therefore, to the best of our knowledge, for stochastic neural networks with delays τij(t), there is a lack of sufficient conditions in linear matrix inequality forms.
The innovations of this paper are listed as follows:
(1) By constructing an appropriate Lyapunov-Krasovskii functional and using linear matrix inequality, some sufficient conditions in the linear matrix inequality forms for the exponential stability of periodic solutions of stochastic neural networks with delays τij(t) are established.
(2) It is confirmed that the Lyapunov-Krasovskii functional and linear matrix inequality can be applied to stochastic neural networks with delays τij(t) that cannot be written in the vector-matrix form.
(3) Compared with the sufficient criteria established in [22,27,28], our sufficient criteria are delay-dependent and less conservative.
The rest of the paper is organized as follows. In Section 2, the stochastic neural networks with multiple time-varying delays and assumptions are introduced. In Sections 3, some sufficient conditions in the linear matrix inequality forms are given to ensure exponential stability of periodic solutions. In Section 4, several examples are given to demonstrate that our criteria are effective and less conservative. The conclusions are drawn in Section 5.
Notation:
(1) Let w(t)=(w1(t),⋯,wn(t))T be n-dimensional Brownian motion defined on a complete probability space (Ω,F,P) with a natural filtration {Ft}t≥0 generated by {w(t)}, in which Ω is the canonical space generated by w(t) and F is the associated σ-algebra generated by {w(s):0≤s≤t} with the probability measure P.
(2) A<0 means that matrix A is symmetric negative definite; AT denotes the transpose of the matrix A; ∗ means the symmetric terms of a symmetric matrix, and I denotes identity matrix.
(3) C([−τ,0];Rn) denotes the Banach space of all continuous Rn-valued functions defined on [−τ,0] satisfying sup−τ≤t≤0E‖ξ(t)‖2<∞; E denotes the mathematical expectation; ‖⋅‖ denotes the Euclidean norm.
The stochastic neural networks with multiple time-varying delays studied in this paper can be described as the following mathematical expression
dxi(t)=[−cixi(t)+n∑j=1aijfj(xj(t))+n∑j=1bijgj(xj(t−τij(t)))+ui(t)]dt+n∑j=1σij(xj(t),xj(t−τij(t)))dwj(t),i=1,⋯,n,t≥0, | (2.1) |
where aij,bij, and ci are some constants and ci>0, fi(⋅) and gi(⋅) are activation functions, σij(⋅,⋅) are diffusion functions. Obviously, the mathematical expression of system (2.1) cannot be transformed into a vector-matrix system.
The following assumption is added in order to discuss the stability of periodic solutions of the system (2.1):
There exist some constants F−i,F+i,G−i,G+i,Lij>0,Mij>0,τ and ˜τ such that for all z1,z2,z3,z4∈R, F−i≤F+i,G−i≤G+i and t≥0,
F−i≤fi(z1)−fi(z2)z1−z2≤F+i,G−i≤gi(z1)−gi(z2)z1−z2≤G+i,z1≠z2, | (2.2) |
0≤τij(t)≤τ,˙τij(t)≤˜τ<1, | (2.3) |
|σij(z1,z2)−σij(z3,z4)|≤Lij|z1−z3|+Mij|z2−z4|. | (2.4) |
The initial condition xi(s)=ξi(s),s∈[−τ,0], and ξ={(ξ1(s),⋯,ξ1(s))T:−τ≤s≤0} is C([−τ,0];Rn)-valued function and F0-measurable Rn-valued random variable.
Let x(t,ξ) and y(t,ψ) be the solutions of system (2.1) with arbitrary initial conditions ξ and ψ, respectively. If it will not cause any misunderstanding, x(t,ξ) and y(t,ψ) are denoted by x(t) and y(t), respectively. Then, for i=1,⋯,n,t≥0, we have
d[xi(t)−yi(t)]=(−ci[xi(t)−yi(t)]+n∑j=1aij[fj(xj(t))−fj(yj(t))]+n∑j=1bij[gj(xj(t−τij(t)))−gj(yj(t−τij(t)))])dt+n∑j=1[σij(xj(t),xj(t−τij(t)))−σij(yj(t),yj(t−τij(t)))]dwj(t). | (2.5) |
Theorem 1. There exist two positive constants λ and K>1 such that
E‖x(t)−y(t)‖2≤Ke−λtE‖ξ−ψ‖2,t≥0, | (3.1) |
if there exist some positive constants p1,⋯,pn,ui1,⋯,uin (i=1,2) such that
Σ=(Σ1PA+U1¯FU2¯G∗−2U10∗∗−2U2+B21−˜τ)<0, | (3.2) |
where
Σ1=−2PC+PB1+L+11−˜τM−2U1˜F−2U2˜G,P=diag{p1,⋯,pn}, |
A=(aij)n×n,C=diag{c1,⋯,cn},Ui=diag{ui1,⋯,uin}(i=1,2), |
B1=diag{n∑j=1|b1j|,⋯,n∑j=1|bnj|},B2=diag{n∑j=1pj|bj1|,⋯,n∑j=1pj|bjn|}, |
L=diag{n∑j=12pjL2j1,⋯,n∑j=12pjL2jn},M=2diag{n∑j=12pjM2j1,⋯,n∑j=12pjM2jn}, |
˜F=diag{F−1F+1,⋯,F−nF+n},ˉF=diag{F−1+F+1,⋯,F−n+F+n}, |
˜G=diag{G−1G+1,⋯,G−nG+n},ˉG=diag{G−1+G+1,⋯,G−n+G+n}. |
Proof. It follows from (3.2) that there exists a constant λ>0 such that
¯Σ=(¯Σ1PA+U1¯FU2¯G∗−2U10∗∗−2U2+eλτ1−˜τB2)<0, | (3.3) |
where
ˉΣ1=λP−2PC+PB1+L+eλτ1−˜τM−2U1˜F−2U2˜G. |
The Lyapunov-Krasovskii functional is defined as follows:
V(t)=V1(t)+V2(t), | (3.4) |
where
V1(t)=eλtn∑i=1pi[xi(t)−yi(t)]2, |
V2(t)=n∑i=1n∑j=1∫tt−τij(t)eλ(s+τ)pi1−˜τ(|bij|[gj(xj(s))−gj(yj(s))]2+2M2ij[xj(s)−yj(s)]2)ds. |
Applying Itˆo formula in [20] to V(t) along with system (2.5), we obtain
dV1(t)=n∑i=1n∑j=1Wij(t)dwj(t)+λeλtn∑i=1pi[xi(t)−yi(t)]2dt+2eλtn∑i=1pi[xi(t)−yi(t)](−ci[xi(t)−yi(t)]+n∑j=1aij[fj(xj(t))−fj(yj(t))]+n∑j=1bij[gj(xj(t−τij(t)))−gj(yj(t−τij(t)))]+eλtn∑i=1pin∑j=1[σij(xj(t),xj(t−τij(t)))−σij(yj(t),yj(t−τij(t)))]2)dt≤n∑i=1n∑j=1Wij(t)dwj(t)+eλtn∑i=1(pi[λ−2ci][xi(t)−yi(t)]2+n∑j=12aijpi[xi(t)−yi(t)][fj(xj(t))−fj(yj(t))]+n∑j=1|bij|pi[xi(t)−yi(t)]2+n∑j=1|bij|pi[gj(xj(t−τij(t)))−gj(yj(t−τij(t)))]2+eλtn∑i=1n∑j=12piL2ij(xj(t)−yj(t))2+eλtn∑i=1n∑j=12piM2ij(xj(t−τij(t))−yj(t−τij(t)))2)dt, | (3.5) |
where
Wij(t)=2eλtpi[xi(t)−yi(t)][σij(xj(t),xj(t−τij(t)))−σij(yj(t),yj(t−τij(t)))], |
and
dV2(t)=n∑i=1n∑j=1eλ(t+τ)pi1−˜τ(|bij|[gj(xj(t))−gj(yj(t))]2+2M2ij[xj(t)−yj(t)]2)dt−(1−˙τij(t))n∑i=1n∑j=1eλ(t−τij(t))+τ)pi1−˜τ(|bij|[gj(xj(t−τij(t))))−gj(yj(t−τij(t))))]2+2M2ij[xj(t−τij(t)))−yj(t−τij(t)))]2)dt≤n∑i=1n∑j=1eλ(t+τ)pi1−˜τ(|bij|[gj(xj(t))−gj(yj(t))]2+2M2ij[xj(t)−yj(t)]2)dt−n∑i=1n∑j=1eλtpi(|bij|[gj(xj(t−τij(t))))−gj(yj(t−τij(t))))]2+2M2ij[xj(t−τij(t)))−yj(t−τij(t)))]2)dt. | (3.6) |
From (3.5) and (3.6), we have
dV(t)=dV1(t)+dV2(t)≤n∑i=1n∑j=1Wij(t)dwj(t)+eλtn∑i=1([λpi−2cipi+n∑j=1|bij|pi+n∑j=1pj(2L2ji+eλτ2M2ji1−˜τ)][xi(t)−yi(t)]2+n∑j=12aijpi[xi(t)−yi(t)][fj(xj(t))−fj(yj(t))])dt+n∑i=1n∑j=1eλ(t+τ)|bji|pj1−˜τ[gi(xi(t))−gi(yi(t))]2dt=n∑i=1n∑j=1Wij(t)dwj(t)+2[x(t)−y(t)]TPA[f(x(t))−f(y(t))]dt+eλt{[x(t)−y(t)]T(λP−2PC+PB1+L+eλτ1−˜τM)[x(t)−y(t)]dt+eλτ1−˜τ[g(x(t))−g(y(t))]TB2[g(x(t))−g(y(t))]dt}, | (3.7) |
where
x(t)−y(t)=(x1(t)−y1(t),⋯,xn(t)−yn(t))T, |
f(x(t))−f(y(t))=(f1(x1(t))−f1(y1(t)),⋯,fn(xn(t))−fn(yn(t)))T, |
g(x(t))−g(y(t))=(g1(x1(t))−g1(y1(t)),⋯,gn(xn(t))−gn(yn(t)))T. |
Simultaneously, by using (2.2), we can deduce
0≤−2n∑i=1u1i[(fi(xi(t))−fi(yi(t)))−F+i(xi(t)−yi(t))][(fi(xi(t))−fi(yi(t)))−F−i(xi(t)−yi(t))]=−2n∑i=1u1i(fi(xi(t))−fi(yi(t)))2−2n∑i=1u1iF+iF−i(xi(t)−yi(t))2+2(F+i+F−i)n∑i=1u1i(fi(xi(t))−fi(yi(t)))(xi(t)−yi(t))=−2[f(x(t))−f(y(t))]TU1[f(x(t))−f(y(t))]−2[x(t)−y(t)]TU1˜F[x(t)−y(t)]+2[f(x(t))−f(y(t))]TU1ˉF[x(t)−y(t)], | (3.8) |
and
0≤−2n∑i=1u2i[(gi(xi(t))−gi(yi(t)))−G+i(xi(t)−yi(t))][(gi(xi(t))−gi(yi(t)))−G−i(xi(t)−yi(t))]=−2[g(x(t))−g(y(t))]TU2[g(x(t))−g(y(t))]−2[x(t)−y(t)]TU2˜G[x(t)−y(t)]+2[g(x(t))−g(y(t))]TU2ˉG[x(t)−y(t)]. | (3.9) |
From (3.4) and (3.7)–(3.9), we deduce
eλtmin1≤i≤n{pi}‖x(t)−y(t)‖2≤V(t)≤V(0)+∫t0eλshT(s)ˉΣ1h(s)ds+∫t0n∑i=1n∑j=1Wij(s)dwj(s)≤max1≤i≤n{pi}‖x(0)−y(0)‖2+∫t0n∑i=1n∑j=1Wij(s)dwj(s)+n∑i=1n∑j=1∫0−τeλ(s+τ)pi1−˜τ(|bij|[gj(xj(s))−gj(yj(s))]2+2M2ij[xj(s)−yj(s)]2)ds≤max1≤i≤n{pi}‖x(0)−y(0)‖2+∫t0n∑i=1n∑j=1Wij(s)dwj(s)+n∑i=1n∑j=1∫0−τeλ(s+τ)pi1−˜τ(|bij|G2j+2M2ij)[xj(s)−yj(s)]2ds, | (3.10) |
where h(t)=([x(t)−y(t)]T,[f(x(t))−f(y(t))]T,[g(x(t))−g(y(t))]T)T,Gi=max{|G−i|,|G+i|},i=1,2,⋯,n.
Taking mathematical expectations for both sides of (3.10), we obtain
eλtmin1≤i≤n{pi}E‖x(t)−y(t)‖2≤EV(t)≤(max1≤i≤n{pi}+eλττ1−˜τmax1≤i≤n{n∑j=1pj[|bji|G2i+2M2ji]})sup−τ≤t≤0E‖x(t)−y(t)‖2, | (3.11) |
which can deduce (3.1).
Remark 1. Obviously, the right-hand side of (3.10) is a non-negative semimartingale. From the non-negative semimartingale convergence theorem [20] and (3.10), we obtain
lim supt→∞eλtmin1≤i≤n{pi}‖x(t)−y(t)‖2<+∞,P−almostsurely, |
which implies
lim supt→∞ln‖x(t)−y(t)‖2t<−λ,P−almostsurely. |
Remark 2. Inequality (3.2) is the sufficient condition in the linear matrix inequality form. However, the matrices P, U1, and U2 in (3.2) cannot be solved by MATLAB because the matrices B2,L, and M contain the constants p1,⋯,pn. In order to obtain the easily verified condition, we take pi=p(i=1,⋯,n), then (3.2) transforms into (3.12).
Corollary 1. Inequality (3.1) holds if there exist some positive constants p,ui1,⋯,uin (i=1,2) such that
Σ=(Σ1pA+U1¯FU2¯G∗−2U10∗∗−2U2+p1−˜τ¯B2)<0, | (3.12) |
where
Σ1=−2pC+pB1+pˉL+p1−˜τˉM−2U1˜F−2U2˜G,ˉB2=diag{n∑j=1|bj1|,⋯,n∑j=1|bjn|}, |
ˉL=diag{n∑j=12L2j1,⋯,n∑j=12L2jn},ˉM=diag{n∑j=12M2j1,⋯,n∑j=12M2jn}, |
the other symbols are the same as Theorem 1.
Certainly, we can also slightly modify the proof of Theorem 1 so that these matrices B2,L, and M do not contain the constants p1,⋯,pn.
Theorem 2. Inequality (3.1) holds if there exist some positive constants p1,⋯,pn,q,ui1,⋯,uin (i=1,2) such that q>max1≤i≤n{pi} and
Σ=(Σ1PA+U1¯FU2¯G∗−2U10∗∗−2U2+11−˜τ¯B2)<0, | (3.13) |
where
Σ1=−2PC+P2B1+qˉL+q1−˜τˉM−2U1˜F−2U2˜G, |
the other symbols are the same as Theorem 1 and Corollary 1.
Proof. From (3.13), we can obtain a constant λ>0 such that
ˉΣ=(ˉΣ1PA+U1ˉFU2ˉG∗−2U10∗∗−2U2+eλτ1−˜τˉB2)<0, | (3.14) |
where
ˉΣ1=λP−2PC+P2B1+qˉL+qeλτ1−˜τˉM−2U1˜F−2U2˜G. |
The Lyapunov-Krasovskii functional is defined as follows:
V(t)=eλtn∑i=1pi[xi(t)−yi(t)]2+n∑i=1n∑j=1∫tt−τij(t)eλ(s+τ)1−˜τ(|bij|[gj(xj(s))−gj(yj(s))]2+2qM2ij[xj(s)−yj(s)]2)ds. |
From (3.5) and q>max1≤i≤n{pi}, we have
dV(t)≤n∑i=1n∑j=1Wij(t)dwj(t)+eλtn∑i=1(pi[λ−2ci][xi(t)−yi(t)]2+n∑j=12aijpi[xi(t)−yi(t)][fj(xj(t))−fj(yj(t))]+n∑j=1|bij|p2i[xi(t)−yi(t)]2+n∑j=1|bij|[gj(xj(t−τij(t)))−gj(yj(t−τij(t)))]2+eλtn∑i=1n∑j=12qL2ij(xj(t)−yj(t))2+eλtn∑i=1n∑j=12qM2ij(xj(t−τij(t))−yj(t−τij(t)))2)dt+n∑i=1n∑j=1eλ(t+τ)1−˜τ(|bij|[gj(xj(t))−gj(yj(t))]2+2qM2ij[xj(t)−yj(t)]2)dt−n∑i=1n∑j=1eλt(|bij|[gj(xj(t−τij(t))))−gj(yj(t−τij(t))))]2+2qM2ij[xj(t−τij(t)))−yj(t−τij(t)))]2)dt≤n∑i=1n∑j=1Wij(t)dwj(t)+eλtn∑i=1([λpi−2cipi+n∑j=1|bij|p2i+n∑j=1q(2L2ji+2M2jieλτ1−˜τ)][xi(t)−yi(t)]2+n∑j=12aijpi[xi(t)−yi(t)][fj(xj(t))−fj(yj(t)))+n∑j=1eλτ|bji|1−˜τ[gi(xi(t))−gi(yi(t))]2)dt=n∑i=1n∑j=1Wij(t)dwj(t)+eλt{[x(t)−y(t)]T(λP−2PC+P2B1+qˉL+qeλτ1−˜τˉM)[x(t)−y(t)]+2[x(t)−y(t)]TPA[f(x(t))−f(y(t))]+eλτ1−˜τ[g(x(t))−g(y(t))]TˉB2[g(x(t))−g(y(t))]}dt. |
The rest of the proof is similar to that of Theorem 1 and so is omitted.
Remark 3. When pi=p(i=1,⋯,n), (3.3) becomes
ˉΣ=(ˉΣ1pA+U1ˉFU2ˉG∗−2U10∗∗−2U2+eλτ1−˜τpˉB2)<0, | (3.15) |
where
ˉΣ1=λpI−2pC+pB1+pˉL+eλτ1−˜τpˉM−2U1˜F−2U2˜G. |
It is easy to see that the stability criteria (3.12)–(3.15) are delay-dependent.
Remark 4. In [22,27,28], delay-independent stability criteria of periodic solutions are established by using the fixed-point principle, Gronwall-Bellman inequality, Lyapunov functional, Halanay inequality, and differential inequality technique (see Remarks 5–7). It is generally agreed that delay-dependent stability criteria are less conservative than delay-independent ones [7]. Therefore, our sufficient criteria are less conservative than those in [22,27,28].
Theorem 3. Let ui(t) and τij(t)(i,j=1,⋯,n) be ω-periodic functions. If the condition (3.12) (or (3.13)) holds, then system (2.1) has a unique periodic solution, which is globally exponentially stable in the mean square.
Proof. If the condition (3.12) (or (3.13)) holds, then inequality (3.1) holds. We can choose a positive integer m such that
Ke−λmω≤116. | (3.16) |
We define a Poincare mapping P:C([−τ,0];Rn)→C([−τ,0];Rn) by Pξ=xω(ξ)=x(ω+θ,ξ),θ∈[−τ,0),t≥0. Then, from (3.1) and (3.16), we have
E‖x(t)−y(t)‖2=E‖Pmξ−Pmψ‖2≤18E‖ξ−ψ‖2. |
By the integral property of measurable functions, we have
‖Pmξ−Pmψ‖2≤116‖ξ−ψ‖2,a.e. |
that is,
‖Pmξ−Pmψ‖≤14‖ξ−ψ‖,a.e. |
So Pm is a contraction mapping and there exists a unique fixed point ξ∗∈C([−τ,0];Rn) such that Pmξ∗=ξ∗,a.e. Note that
Pm(Pξ∗)=P(Pmξ∗)=Pξ∗,a.e. |
We know that Pξ∗∈C([−τ,0];Rn) is also a fixed point of Pm and obtain
xω(ξ∗)=Pξ∗=ξ∗,a.e. |
Let x(t,ξ∗) be the solution of (2.1) through (0,ξ∗). Then, x(t+ω,ξ∗) is also a solution of (2.1) and for t≥0,
xt+ω(ξ∗)=xt(xω(ξ∗))=xt(ξ∗),a.e. |
So we have x(t+ω,ξ∗)=x(t,ξ∗),a.e. which shows x(t,ξ∗) is one ω-periodic solution of (2.1). From (3.1), we know all other solutions converge exponentially to x(t,ξ∗) in the mean square as t→∞.
Example 1. Consider system (2.1) with the following parameters and functions:
A=(1−1−11−11−1−111−11−1−1−1−1),B=(−11−11−1−11−11−1−1−1−1−1−11), |
C=diag{6,6,5,5},fi(x)=tanh(xi),gi(xi)=0.8tanh(xi),ui=sint,τii(t)=0.2sint+0.2, τij(t)=0.2cost+0.2(i≠j),σij(xj(t),xj(t−τij(t)))=0.05xj(t)+0.05xj(t−τij(t)),i,j=1,2,3,4.
We calculate that B1=ˉB2=4I,˜F=˜G=0,ˉF=I,ˉG=0.8I,Lij=Mij=0.005,ˉL=ˉM=0.02I,˜τ=0.2.
By using MATLAB LMI Control Toolbox, we obtain P=0.6044I,U1=diag{1.5261,1.5243,1.5127,1.6004},U2=diag{2.9135,2.9018,2.9970,2.9887} to satisfy the condition (3.12) of Corollary 1, and obtain P=diag{0.5191,0.5209,0.5726,0.5705},q=1.7100,U1=diag{1.2485,1.2521,1.2398,1.3223},U2=diag{2.3854,2.3835,2.6177,2.6148} to satisfy the condition (3.13) of Theorem 2. Therefore, Corollary 1 and Theorem 2 are effective. Figure 1 shows the solution trajectories of system (2.1) with the initial value (−0.4,−0.2,0.4,0.6)T appearing as periodic motions after a few seconds.
By changing some functions in system (2.1), we obtain the following systems considered in [22,27,28] :
dxi(t)=[−cixi(t)+n∑j=1aijfj(xj(t))+n∑j=1bijgj(xj(t−τij(t)))+ui(t)]dt+n∑j=1σij(xj(t))dwj(t), | (4.1) |
˙xi(t)=−cixi(t)+n∑j=1aijfj(xj(t))+n∑j=1bijgj(xj(t−τj(t)))+ui(t), | (4.2) |
and
˙xi(t)=−cixi(t)+n∑j=1aijfj(xj(t))+n∑j=1bijfj(xj(t−τij(t)))+ui(t). | (4.3) |
Remark 5. In [22], fixed-point principle and Gronwall-Bellman inequality are used to establish the stability condition of the periodic solutions of system (4.1), that is, ρ=max1≤i≤n{3θi/c2i}<1, where θi=(∑nj=1|aij|Fj+|bij|Gj)2+(∑nj=1Lij)2+(∑nj=1˜Lij)2, Fj=max{|F−j|,|F+j|},Gj=max{|G−j|,|G+j|},|σij(⋅)|≤˜Lij in this paper. However, for system (4.1) in Example 2, we calculate ρ>1, which implies that the condition in [22] is not applicable.
Example 2. Consider system (4.1) with σij(xj(t))=0.1+0.05sin(xj(t)),i,j=1,2,3,4, other symbols are the same as in Example 1.
We calculate that B1=ˉB2=4I,˜F=˜G=0,ˉF=I,ˉG=0.8I,Lij=0.05,Mij=0,ˉL=0.02I,ˉM=0,˜τ=0.2.
By using MATLAB LMI Control Toolbox, we obtain P=0.1563I,U1=diag{0.4515,0.4500,0.4772,0.4761},U2=diag{0.7289,0.7291,0.7314,0.7315} to satisfy the condition (3.12) of Corollary 1, and obtain P=diag{0.1765,0.1751,0.1993,0.1970},q=0.8439,U1=diag{0.4678,0.4659,0.4929,0.4932},U2=diag{0.7652,0.7624,0.8485,0.8434} to satisfy the condition (3.13) of Theorem 2. Therefore, Corollary 1 and Theorem 2 are effective. Figure 2 shows the solution trajectories of system (4.1) with the initial value (−0.4,−0.2,0.4,0.6)T appearing as periodic motions after a few seconds.
On the other hand, based on Theorem 3.1 in [22], we calculate that Fj=1,Gj=0.8,|aij|=|bij|=1,Lij=0.05,˜Lij=0.15 θi=52.24 and ρ=52.24×3/25=6.2688>1. Therefore, Theorem 3.1 in [22] is not applicable to system (4.1) in this example.
Remark 6. In [27], fixed-point principle and differential inequality technique are used to establish the stability condition of the periodic solutions of system (4.2), that is, F=ξ||A||2+η||B||2c0<1, where ξ=max1≤i≤n{supxi≠0fi(xi)xi},η=max1≤i≤n{supxi≠0gi(xi)xi},c0=min1≤i≤n{ci},||A||2 denotes the square root of the largest eigenvalue of ATA. However, for system (4.2) in Example 3, we calculate F>1, which implies the condition in [27] is not applicable.
Example 3. Consider system (4.2) with τj(t)=0.2sint+0.2,j=1,2,3,4, other symbols are the same as in Example 1.
We calculate that B1=ˉB2=4I,˜F=˜G=0,ˉF=I,ˉG=0.8I,ˉL=ˉM=0,˜τ=0.2.
By using MATLAB LMI Control Toolbox, we obtain P=0.1564I,U1=diag{0.4524,0.4509,0.4781,0.4770},U2=diag{0.7300,0.7301,0.7325,0.7326} to satisfy the condition (3.12) of Corollary 1, and obtain P=diag{0.1829,0.1814,0.2069,0.2045},U1=diag{0.4908,0.4887,0.5160,0.5162},U2=diag{0.7981,0.7951,0.8849,0.8795} to satisfy the condition (3.13) of Theorem 2. Therefore, Corollary 1 and Theorem 2 are effective. Figure 3 shows the solution trajectories of system (4.2) with the initial value (1,−1,−0.5,0.5)T appearing as periodic motions after a few seconds.
On the other hand, according to Theorem 3 in [27], we calculate ξ=1,η=0.8,||A||2=√8,||B||2=√7.4641,c0=min1≤i≤4{ci}=5 and
F=ξ||A||2+η||B||2c0=5.0145>1. |
Therefore, Theorem 3 in [27] is not applicable to system (4.2) in this example.
Remark 7. In [28], Lyapunov functional and Halanay inequality are used to establish the stability conditions of the periodic solutions of system (4.3) : di−Li∑nj=1(|aji|+|bji|)>0 or di−∑nj=1(|aij|+|bij|)Lj>0,i=1,⋯,n, where di=ci and Lj=max{|F−j|,|F+j|} in this paper. However, for system (4.3) in Example 4, we calculate di−Li∑nj=1(|aji|+|bji|)=di−∑nj=1(|aij|+|bij|)Lj=0(i=1,⋯,n), which implies the criteria in [28] are not applicable.
Example 4. Consider system (4.3) with C=diag{4,4,4,4},fi(xi)=0.5tanh(xi),τii(t)=0.2sint+0.2,τij(t)=0.2cost+0.2(i≠j),i,j=1,2,3,4, the matrices A and B are the same as in Example 1.
We calculate that B1=ˉB2=4I,˜F=˜G=ˉL=ˉM=0,ˉF=ˉG=0.5I,˜τ=0.2.
By using MATLAB LMI Control Toolbox, we obtain P=2.2433I,U1=diag{9.8971,9.8971,10.7128,10.7128},U2=14.3836I to satisfy the condition (3.12) of Corollary 1, and obtain P=diag{1.6333,1.6333,2.2619,2.2619},U1=diag{7.6665,7.6665,8.3747,8.3747},U2=diag{10.9331,10.9331,12.0760,12.0760} to satisfy the condition (3.13) of Theorem 2. Therefore, Corollary 1 and Theorem 2 are effective. Figure 4 shows that the solution trajectories of system (4.3) with the initial value (−0.6,0.4,−0.4,0.8)T appearing as periodic motions after a few seconds.
On the other hand, based on the results proposed in [28], we calculate that di=4,Li=0.5,di−Li∑4j=1(|aji|+|bji|)=di−∑4j=1(|aij|+|bij|)Lj=0,i=1,2,3,4. Therefore, the sufficient criteria in [28] are not applicable to system (4.3) in this example.
This paper has investigated the global exponential periodicity of stochastic neural networks with multiple time-varying delays τij(t). Such stochastic neural networks cannot be transformed into the vector-matrix form because of the multiple time-varying delays τij(t). For neural networks with delays τij(t), the commonly used methods do not include linear matrix inequality. Therefore, the stability conditions in the linear matrix inequality forms are rare for networks with delays τij(t). In this paper, we establish several sets of easily verified conditions in the linear matrix inequality forms to ensure that the stochastic networks have a unique and exponentially stable periodic solution. Several examples are given to demonstrate that our sufficient criteria are effective and less conservative than those in the existing references.
The authors declare they have not used Artificial Intelligence (AI) tools in the creation of this article.
The authors would like to thank the editor and the reviewers for their detailed comments and valuable suggestions.
This work was supported by the National Natural Science Foundation of China (No: 12271416, 11971367, 11826209, and 11271295), the Guiding Project of Natural Science Foundation of Hubei Province under Grant (2022CFC065), the Natural Science Foundation of Guangdong Province (2018A030313536).
The authors declare no conflict of interest.
[1] |
Y. H. Zhou, C. D. Li, H. Wang, Stability analysis on state-dependent impulsive Hopfield neural networks via fixed-time impulsive comparison system method, Neurocomputing, 316 (2018), 20–29. https://doi.org/10.1016/j.neucom.2018.07.047 doi: 10.1016/j.neucom.2018.07.047
![]() |
[2] |
S. Arik, A modified Lyapunov functional with application to stability of neutral-type neural networks with time delays, J. Franklin I., 356 (2019), 276–291. https://doi.org/10.1016/j.jfranklin.2018.11.002 doi: 10.1016/j.jfranklin.2018.11.002
![]() |
[3] |
B. Sun, Y. T. Cao, Z. Y. Guo, Z. Yan, S. P. Wen, Synchronization of discrete-time recurrent neural networks with time-varying delays via quantized sliding mode control, Appl. Math. Comput., 375 (2020), 125093. https://doi.org/10.1016/j.amc.2020.125093 doi: 10.1016/j.amc.2020.125093
![]() |
[4] |
Y. X. Wang, Y. T. Cao, Z. Y. Guo, S. P. Wen, Passivity and passification of memristive recurrent neural networks with multi-proportional delays and impulse, Appl. Math. Comput., 369 (2020), 124838. https://doi.org/10.1016/j.amc.2019.124838 doi: 10.1016/j.amc.2019.124838
![]() |
[5] |
Q. K. Song, Y. X. Chen, Z. J. Zhao, Y. R. Liu, F. E. Alsaadi, Robust stability of fractional-order quaternion-valued neural networks with neutral delays and parameter uncertainties, Neurocomputing, 420 (2021), 70–81. https://doi.org/10.1016/j.neucom.2020.08.059 doi: 10.1016/j.neucom.2020.08.059
![]() |
[6] |
J. Chen, M. H. Jiang, Stability of memristor-based fractional-order neural networks with mixed time-delay and impulsive, Neural Process. Lett., 55 (2023), 4697–4718. https://doi.org/10.1007/s11063-022-11061-8 doi: 10.1007/s11063-022-11061-8
![]() |
[7] |
X. Zhang, Z. J. Zhang, T. T. Yu, X. Wang, Global results on exponential stability of neutral Cohen-Grossberg neural networks involving multiple neutral and discrete time-varying delays: A method based on system solutions, Neural Process. Lett., 55 (2023), 11273–11291. https://doi.org/10.1007/s11063-023-11375-1 doi: 10.1007/s11063-023-11375-1
![]() |
[8] |
Z. L. Zhai, H. C. Yan, S. M. Chen, Y. F. Chang, J. Zhou, Novel stability criteria of generalized neural networks with time-varying delay based on the same augmented LKF and bounding technique, Appl. Math. Comput., 460 (2024), 128289. https://doi.org/10.1016/j.amc.2023.128289 doi: 10.1016/j.amc.2023.128289
![]() |
[9] |
Y. Ni, Z. Wang, X. Huang, Q. Ma, H. Shen, Intermittent sampled-data control for local stabilization of neural networks subject to actuator saturation: A work-interval-dependent functional approach, IEEE T. Neur. Net. Lear., 35 (2024), 1087–1097. https://doi.org/10.1109/TNNLS.2022.3180076 doi: 10.1109/TNNLS.2022.3180076
![]() |
[10] |
F. C. Kong, Q. X. Zhu, K. Wang, J. J. Nieto, Stability analysis of almost periodic solutions of discontinuous BAM neural networks with hybrid time-varying delays and D operator, J. Franklin I., 356 (2019), 11605–11637. https://doi.org/10.1016/j.jfranklin.2019.09.030 doi: 10.1016/j.jfranklin.2019.09.030
![]() |
[11] |
M. Abdelaziz, F. Cherif, Piecewise asymptotic almost periodic solutions for impulsive fuzzy Cohen-Grossberg neural networks, Chaos Soliton. Fract., 132 (2020), 109575. https://doi.org/10.1016/j.chaos.2019.109575 doi: 10.1016/j.chaos.2019.109575
![]() |
[12] |
Y. X. Wang, Y. T. Cao, Z. Y. Guo, T. W. Huang, S. P. Wen, Event-based sliding-mode synchronization of delayed memristive neural networks via continuous/periodic sampling algorithm, Appl. Math. Comput., 383 (2020), 125379. https://doi.org/10.1016/j.amc.2020.125379 doi: 10.1016/j.amc.2020.125379
![]() |
[13] |
Q. D. Jiang, Q. R. Wang, Almost periodic solutions for quaternion-valued neural networks with mixed delays on time scales, Neurocomputing, 439 (2021), 363–373. https://doi.org/10.1016/j.neucom.2020.09.063 doi: 10.1016/j.neucom.2020.09.063
![]() |
[14] |
Z. W. Cai, L. H. Huang, Z. Y. Wang, X. M. Pan, S. K. Liu, Periodicity and multi-periodicity generated by impulses control in delayed Cohen-Grossberg-type neural networks with discontinuous activations, Neural Networks, 143 (2021), 230–245. https://doi.org/10.1016/j.neunet.2021.06.013 doi: 10.1016/j.neunet.2021.06.013
![]() |
[15] |
F. C. Kong, Y. Ren, R. Sakthivel, Delay-dependent criteria for periodicity and exponential stability of inertial neural networks with time-varying delays, Neurocomputing, 419 (2021), 261–272. https://doi.org/10.1016/j.neucom.2020.08.046 doi: 10.1016/j.neucom.2020.08.046
![]() |
[16] |
Y. Zhang, Y. H. Qiao, L. J. Duan, J. Miao, Multistability of almost periodic solution for Clifford-valued Cohen-Grossberg neural networks with mixed time delays, Chaos Soliton. Fract., 176 (2023), 114100. https://doi.org/10.1016/j.chaos.2023.114100 doi: 10.1016/j.chaos.2023.114100
![]() |
[17] |
J. Gao, L. H. Dai, H. Y. Jiang, Stability analysis of pseudo almost periodic solutions for octonion-valued recurrent neural networks with proportional delay, Chaos Soliton. Fract., 175 (2023), 4061–4078. https://doi.org/10.1016/j.chaos.2023.114061 doi: 10.1016/j.chaos.2023.114061
![]() |
[18] |
J. X. Cheng, W. D. Liu, Stability analysis of anti-periodic solutions for Cohen-Grossberg neural networks with inertial term and time delays, Mathematics, 12 (2024), 198. https://doi.org/10.3390/math12020198 doi: 10.3390/math12020198
![]() |
[19] | S. Haykin, Neural networks: A comprehensive foundation, Englewood Cliffs: Prentice-Hall, 1998. |
[20] |
S. Blythe, X. R. Mao, X. X. Liao, Stability of stochastic delay neural networks, J. Franklin I., 338 (2001), 481–495. https://doi.org/10.1016/S0016-0032(01)00016-3 doi: 10.1016/S0016-0032(01)00016-3
![]() |
[21] |
X. D. Li, Existence and global exponential stability of periodic solution for delayed neural networks with impulsive and stochastic effects, Neurocomputing, 73 (2010), 749–758. https://doi.org/10.1016/j.neucom.2009.10.016 doi: 10.1016/j.neucom.2009.10.016
![]() |
[22] |
W. Q. Wu, L. Yang, Y. P. Ren, Periodic solutions for stochastic Cohen-Grossberg neural networks with time-varying delays, Int. J. Nonlin. Sci. Num., 22 (2021), 13–21. https://doi.org/10.1515/ijnsns-2019-0142 doi: 10.1515/ijnsns-2019-0142
![]() |
[23] |
Y. Y. Hou, L. H. Dai, Square-mean pseudo almost periodic solutions for quaternion-valued stochastic neural networks with time-varying delays, Math. Probl. Eng., 2021 (2021), 6679326. https://doi.org/10.1155/2021/6679326 doi: 10.1155/2021/6679326
![]() |
[24] |
L. Yao, Z. Wang, X. Huang, Y. Li, Q. Ma, H. Shen, Stochastic sampled-data exponential synchronization of markovian jump neural networks with time-varying delays, IEEE T. Neur. Net. Lear., 34 (2023), 909–920. https://doi.org/10.1109/TNNLS.2021.3103958 doi: 10.1109/TNNLS.2021.3103958
![]() |
[25] |
J. Xiang, M. Tan, Existence and stability of Stepanov-almost periodic solution in distribution for quaternion-valued memristor-based stochastic neural networks with delays, Nonlinear Dynam., 111 (2023), 1715–1732. https://doi.org/10.1007/s11071-022-07877-7 doi: 10.1007/s11071-022-07877-7
![]() |
[26] |
Y. K. Li, X. H. Wang, Besicovitch almost periodic stochastic processes and almost periodic solution of Clifford-valued stochastic neural networks, Discrete Cont. Dyn.-B, 28 (2023), 2154. https://doi.org/10.3934/dcdsb.2022162 doi: 10.3934/dcdsb.2022162
![]() |
[27] |
H. Y. Zhao, Global exponential stability and periodicity of cellular neural networks with variable delays, Phys. Lett. A, 336 (2005), 331–341. https://doi.org/10.1016/j.physleta.2004.12.001 doi: 10.1016/j.physleta.2004.12.001
![]() |
[28] |
L. Q. Zhou, G. D. Hu, Global exponential periodicity and stability of cellular neural networks with variable and distributed delays, Appl. Math. Comput., 195 (2008), 402–411. https://doi.org/10.1016/j.amc.2007.04.114 doi: 10.1016/j.amc.2007.04.114
![]() |