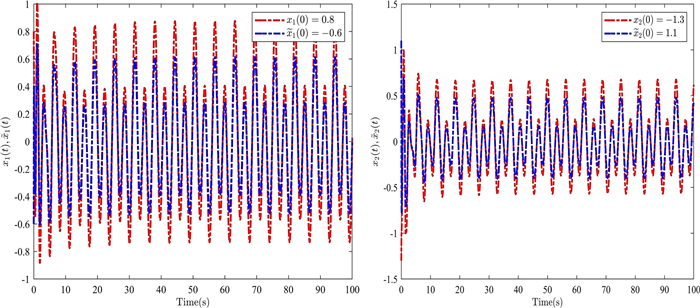
With the popularization of digital image technology, image information has inevitably developed to involved the disclosure of personal privacy; in this study, a color image encryption algorithm was designed to encrypt and decrypt images by using chaotic sequences of a class of memristor-based hybrid delayed bidirectional associative memory neural networks (MHDBAMNNs) to protect images from illegal acquisition and use. Additionally, the discontinuity problem of the right-hand side of the Filippov system due to the hopping property of the memristor has been treated by using differential inclusion and set-valued mapping theories, and a sufficient criterion for guaranteeing the synchronization of finite-time projections derived based on the drive-response concept, Lyppunov stability theorem, and inequality technique. To improve the security performance, a color image encryption algorithm based on a combination of Chen's hyperchaotic system and a DNA codec operation was adopted, also, the robustness and validity of our proposed approach was demonstrated through image performance analysis. Furthermore, the potential application of the model in secure transmission has been explored.
Citation: Meng Li, Gulijiamali Maimaitiaili. New results on finite-time projective synchronization for memristor-based hybrid delayed BAM neural networks with applications to DNA image encryption[J]. AIMS Mathematics, 2024, 9(4): 9822-9846. doi: 10.3934/math.2024481
[1] | N. Mohamed Thoiyab, Mostafa Fazly, R. Vadivel, Nallappan Gunasekaran . Stability analysis for bidirectional associative memory neural networks: A new global asymptotic approach. AIMS Mathematics, 2025, 10(2): 3910-3929. doi: 10.3934/math.2025182 |
[2] | Qingyi Cui, Changjin Xu, Wei Ou, Yicheng Pang, Zixin Liu, Jianwei Shen, Muhammad Farman, Shabir Ahmad . Further study on Hopf bifurcation and hybrid control strategy in BAM neural networks concerning time delay. AIMS Mathematics, 2024, 9(5): 13265-13290. doi: 10.3934/math.2024647 |
[3] | Jiaqing Zhu, Guodong Zhang, Leimin Wang . Quasi-projective and finite-time synchronization of fractional-order memristive complex-valued delay neural networks via hybrid control. AIMS Mathematics, 2024, 9(3): 7627-7644. doi: 10.3934/math.2024370 |
[4] | Yuzhen Zhou, Erxi Zhu . A new image encryption based on hybrid heterogeneous time-delay chaotic systems. AIMS Mathematics, 2024, 9(3): 5582-5608. doi: 10.3934/math.2024270 |
[5] | Ailing Li, Xinlu Ye . Finite-time anti-synchronization for delayed inertial neural networks via the fractional and polynomial controllers of time variable. AIMS Mathematics, 2021, 6(8): 8173-8190. doi: 10.3934/math.2021473 |
[6] | Chengqiang Wang, Xiangqing Zhao, Yang Wang . Finite-time stochastic synchronization of fuzzy bi-directional associative memory neural networks with Markovian switching and mixed time delays via intermittent quantized control. AIMS Mathematics, 2023, 8(2): 4098-4125. doi: 10.3934/math.2023204 |
[7] | Rakkiet Srisuntorn, Wajaree Weera, Thongchai Botmart . Modified function projective synchronization of master-slave neural networks with mixed interval time-varying delays via intermittent feedback control. AIMS Mathematics, 2022, 7(10): 18632-18661. doi: 10.3934/math.20221025 |
[8] | Shuang Li, Xiao-mei Wang, Hong-ying Qin, Shou-ming Zhong . Synchronization criteria for neutral-type quaternion-valued neural networks with mixed delays. AIMS Mathematics, 2021, 6(8): 8044-8063. doi: 10.3934/math.2021467 |
[9] | N. Jayanthi, R. Santhakumari, Grienggrai Rajchakit, Nattakan Boonsatit, Anuwat Jirawattanapanit . Cluster synchronization of coupled complex-valued neural networks with leakage and time-varying delays in finite-time. AIMS Mathematics, 2023, 8(1): 2018-2043. doi: 10.3934/math.2023104 |
[10] | Chengbo Yi, Rui Guo, Jiayi Cai, Xiaohu Yan . Pinning synchronization of dynamical neural networks with hybrid delays via event-triggered impulsive control. AIMS Mathematics, 2023, 8(10): 25060-25078. doi: 10.3934/math.20231279 |
With the popularization of digital image technology, image information has inevitably developed to involved the disclosure of personal privacy; in this study, a color image encryption algorithm was designed to encrypt and decrypt images by using chaotic sequences of a class of memristor-based hybrid delayed bidirectional associative memory neural networks (MHDBAMNNs) to protect images from illegal acquisition and use. Additionally, the discontinuity problem of the right-hand side of the Filippov system due to the hopping property of the memristor has been treated by using differential inclusion and set-valued mapping theories, and a sufficient criterion for guaranteeing the synchronization of finite-time projections derived based on the drive-response concept, Lyppunov stability theorem, and inequality technique. To improve the security performance, a color image encryption algorithm based on a combination of Chen's hyperchaotic system and a DNA codec operation was adopted, also, the robustness and validity of our proposed approach was demonstrated through image performance analysis. Furthermore, the potential application of the model in secure transmission has been explored.
Memristors used to replace resistors in artificial neural networks constitute memory neural networks (MNNs), which are steadily garnering more attention, particularly in the fields of brain-like simulation [1,2] and computer science. Owing to the hysteresis loop properties of memristors [3], memory-delayed neural network systems may exhibit special dynamics and produce novel chaotic phenomena [4]. These phenomena allow amnesic time-delay neural networks to be used as chaotic systems in applications such as pseudorandom number generators [5], secure communication [6], image transmission [7], and image encryption [8,9,10].
A novel flux-controlled memristor model containing a set of hyperbolic tangent functions has been developed by analyzing multiple double-scroll chaotic attractors, amplitude control features, and extreme multi-stability [11]. A five-dimensional grid-scroll memristive chaotic system has been effectively designed by employing two types of flux-controlled memristors and an equivalent circuit that is capable of generating grid-scroll attractors has been devised [12].
The efficiency of image transmission can be improved by incorporating finite-time synchronization. In a previous study on finite-time projection synchronization, aiming to address the unsynchronized oscillations caused by external noise of a nonlinear dynamic system of microgrid hierarchical control, a finite-time function projection synchronization control scheme was developed by combining complex networks and microgrids [13]. A finite-time robust feedback controller of surface-balanced systems with hidden attractors has been constructed by applying phase analysis, bifurcation, and a Poincaré section, and the synchronization of master-slave systems was realized in a finite time [14]. In [15], the finite-time projection synchronization problem for Caputo-type fractional-order complex-valued neural networks with time delay is proposed based on fractional calculus and inequality techniques.
The goodness of the chaotic system properties has been found to determine the effective encryption of chaotic images, and a four-dimensional multi-scroll chaotic system based on two types of flux-controlled non-volatile memristor models with a set of signum functions has been constructed, additionally, the chaotic sequence generated by the proposed system was demonstrated to effectively encrypt the image and have good robustness [16]. The equivalent simulation circuit has been constructed by using discrete components, and a commercial image encryption system for the Internet of Things has been implemented based on the proposed high-dimensional memristive Hopfield neural network model [17]. A 5D fractional-order memristive Hopfield neural network (FOMHNN) under electromagnetic radiation was developed by applying memristors as self-synaptic weights of a Hopfield neural network, and an extremely secure image encryption algorithm has been proposed based on the FOMHNN [18]. Kanwal et al. proposed an image encryption framework based on the integration of chaotic maps and color codes [19]. In [20], a color-image encryption scheme was designed by combining hyperchaotic maps, cross-plane operations, and gene theories.
DNA image encryption schemes based on a high-dimensional chaotic system have very broad development prospects and research value. In the study of Chen's hyperchaotic system, a new hyper-chaotic system based on the three-dimensional Chen system designed by using a smooth continuous nonlinear flux-controlled memristor model [21]. Regarding DNA image encryption schemes, a symmetric cipher based on the permutation substitution structure was comprehensively cryptanalyzed by combining quantum chaotic maps and DNA coding [22]. A chaotic image encryption scheme based on an improved foraging behavior of the artificial fish school and DNA coding has also been proposed [23].
Motivated by the aforementioned discussions, this study mainly involving investigating the finite-time projection synchronization of bidirectional associative MNNs with leakage delays, time-varying discrete delays, and time-varying distributed delays; the finite-time projection synchronization criterion has been derived under the condition that the negative feedback controller coefficient is related to the connection weight values of other layers; similarly, the color image encryption algorithm based on a combination of Chen's hyperchaotic system and a DNA codec operation has been designed to encrypt and decrypt images by using chaotic sequences of the proposed system, and good results have been achieved to show the superiority of the encryption algorithm.
The remainder of this paper is organized as follows. Section 2 describes the drive-response system model and preliminary preparations. Section 3 presents a time delay independent negative feedback controller that has been designed by using a feedback control strategy to provide a criterion for the finite-time projection synchronization of MHDBAMNNs. Section 4 provides numerical simulation examples to demonstrate the validity of the proposed method. Section 5 presents the color image encryption algorithm based on the combination of Chen's hyperchaotic system and the DNA codec operation that was developed by using chaotic sequences of the proposed system. Section 6 concludes the study and offers perspectives for future research.
Note: Ξ([−θ,0],Rn) is a Banach space consisting of all continuous function maps from [−θ,0] to Rn.
In this study, the following bidirectional associative MNNs with mixed time delays (leakage time delays, time-varying discrete time delays, and time-varying distributed time delays) is considered:
{˙xi(t)=−δixi(t−σ)+∑mj=1aji(xi(t))fj(yj(t))+∑mj=1bji(xi(t))fj(yj(t−τ(t)))+∑mj=1cji(xi(t))∫tt−ρ(t)fj(yj(v))dv+Ii(t),˙yj(t)=−ωjyj(t−σ)+∑ni=1kij(yj(t))gi(xj(t))+∑ni=1lij(yj(t))gi(xj(t−τ(t)))+∑ni=1mij(yj(t))∫tt−ρ(t)gi(xj(v))dv+Hj(t), | (1) |
where t≥0,i=1,2,…,n,j=1,2,…,m; xi(t) and yj(t) denotes the states of the i -th neuron in the x -layer and j -th neuron in the y -layer, respectively; δi>0 and ωj>0 represent the rates of neuron self-inhibition; fj(·) and gi(·) are the neuron activation functions; σ is the leakage time delay, τ(t) denotes the time-varying discrete time delay, ρ(t) denotes the time-varying distributed time delay; σ>0, 0≤τ(t)≤τ0, ˙τ(t)≤τ1<1, and 0≤ρ(t)≤ρ0; Ii(t) and Hj(t) are the external input variables for the network system. The initial values of system (1) are given as xi(χ)=ϕi(χ), ϕ(χ)=[ϕ1(χ),ϕ2(χ),…,ϕn(χ)]∈Ξ([−θ,0],Rn); yj(χ)=ψj(χ), ψ(χ)=[ψ1(χ), ψ2(χ),…,ψm(χ)]∈Ξ([−θ,0],Rm), where θ=max{σ,τ0,ρ0}; aij(xi(t)), bij(xi(t)), cji(xi(t)), kij(yi(t)), lij(yi(t)), mij(yi(t)) describe the connection weights of the i -th and the j -th nodes, respectively, and they are given as follows:
aji(γ)={ˊaji,|γ|≤Ti,ˊaji,|γ|>Ti, |
bji(γ)={ˊbji,|γ|≤Ti,ˊbji,|γ|<Ti, |
cji(γ)={ˊcji,|γ|≤Ti,ˊcji,|γ|>Ti, |
kij(γ)={ˊkij,|γ|≤ˆTj,ˊkij,|γ|>ˆTj, |
lij(γ)={ˊlij,|γ|≤ˆTj,ˊlij,|γ|>ˆTj, |
mij(γ)={ˊmij,|γ|≤ˆTj,ˊmij,|γ|>ˆTj, |
where γ=xi(t) or yj(t); the switching jumps Ti,ˆTj are positive constants; and ˊaji,ˊaji,ˊbji,ˊbji,ˊcji, ˊcji,ˊkij,ˊkij,ˊlij,ˊlij,ˊmij´,mij are constants. To clearly present the proof of the theoretical process, the following representations are considered:
a+ji=max{ˊaji,ˊaji},a−ji=min{ˊaji,ˊaji},amji=max{|ˊaji|,|ˊaji|}, |
b+ji=max{ˊbji,ˊbji},b−ji=min{ˊbji,ˊbji},bmji=max{|ˊbji|,|ˊbji|}, |
c+ji=max{ˊcji,ˊcji},c−ji=min{ˊcji,ˊcji},cmji=max{|ˊcji|,|ˊcji|}, |
k+ij=max{ˊkij,ˊkij},k−ij=min{ˊkij,ˊkij},kmij=max{|ˊkij|,|ˊkij|}, |
l+ij=max{ˊlij,ˊlij},l−ij=min{ˊlij,ˊlij},lmij=max{|ˊlij|,|ˊlij|}, |
m+ij=max{ˊmij,ˊmij},m−ij=min{ˊmij,ˊmij},mmij=max{|ˊmij|,|ˊmij|}. |
Owing to the discontinuity problem of the system caused by the hopping property of the memristor, which must be treated by using the theory of differential inclusion and set-valued mapping, system (1) can be transformed into the following system:
{˙xi(t)∈−δixi(t−σ)+∑mj=1co[aji(xi(t))]fj(yj(t))+∑mj=1co[bji(xi(t))]fj(yj(t−τ(t)))+∑mj=1co[cji(xi(t))]∫tt−ρ(t)fj(yj(v))dv+Ii(t),˙yj(t)∈−ωjyj(t−σ)+∑ni=1co[kij(yj(t))]gi(xj(t))+∑ni=1co[lij(yj(t))]gi(xj(t−τ(t)))+∑ni=1co[mij(yj(t))]∫tt−ρ(t)gi(xj(v))dv+Hj(t), | (2) |
where co[aji(xi(t))], co[bji(xi(t))], co[cji(xi(t))], co[kij(yj(t))], co[lij(yj(t))], and co[mij(yj(t))] denote the convex closures of the assemblies [aji(xi(t))], [bji(xi(t))], [cji(xi(t))], [kij(yj(t))], [lij(yj(t))], and [mij(yj(t))], respectively, and they are given as follows:
co[aji(γ)]={ˊaji,|γ|<Ti,[a−ji,a+ji],|γ|=Ti,ˊaji,|γ|>Ti, |
co[bji(γ)]={ˊbji,|γ|<Ti,[b−ji,b+ji],|γ|=Ti,ˊbji,|γ|>Ti, |
co[cji(γ)]={ˊcji,|γ|<Ti,[c−ji,c+ji],|γ|=Ti,ˊcji,|γ|>Ti, |
co[kij(γ)]={ˊkij,|γ|<ˆTj,[k−ij,k+ij],|γ|=ˆTj,ˊkij,|γ|>ˆTj, |
co[lij(γ)]={ˊlij,|γ|<ˆTj,[l−ij,l+ij],|γ|=ˆTj,ˊlij,|γ|>ˆTj, |
co[mij(γ)]={ˊmij,|γ|<ˆTj,[m−ij,m+ij],|γ|=ˆTj,ˊmij,|γ|>ˆTj. |
Let axji(t)∈co[aji(xi(t))], bxji(t)∈co[bji(xi(t))], cxji(t)∈co[cji(xi(t))], kyij(t)∈co[kij(yj(t))], lyij(t)∈co[lij(yj(t))], and myij(t)∈co[mij(yj(t))] [24]. Then, the system (2) can be written as follows:
{˙xi(t)=−δixi(t−σ)+∑mj=1axji(t)fj(yj(t))+∑mj=1bxji(t)fj(yj(t−τ(t)))+∑mj=1cxji(t)∫tt−ρ(t)fj(yj(v))dv+Ii(t),˙yj(t)=−ωjyj(t−σ)+∑ni=1kyij(t)gi(xj(t))+∑ni=1lyij(t)gi(xj(t−τ(t)))+∑ni=1myij(t)∫tt−ρ(t)gi(xj(v))dv+Hj(t), | (3) |
The finite-time projection synchronization criterion is derived by using the drive-response concept, and system (1) is considered as a drive system; the response system of MHDBAMNNs is described as follows:
{˙ xi(t)=−δi xi(t−σ)+∑mj=1aji( xi(t))fj( yj(t))+∑mj=1bji( xi(t))fj( yj(t−τ(t)))+∑mj=1cji( xi(t))∫tt−ρ(t)fj( yj(v))dv+ui(t)+Ii(t),˙ yj(t)=−ωj yj(t−σ)+∑ni=1kij( yj(t))gi( xj(t))+∑ni=1lij( yj(t))gi( xj(t−τ(t)))+∑ni=1mij( yj(t))∫tt−ρ(t)gi( xj(v))dv+vj(t)+Hj(t), | (4) |
where ui(t) and vj(t) are the negative feedback controllers to be designed.
To obtain the criterion for finite-time projection synchronization, the following assumptions must be made.
Assumption 2.1. If the nonlinear activation functions fj(·) and gi(·) are Lipschitz continuous on a real number field R, then for any x,y∈R, the following inequality condition holds:
|fj(y)−fj(x)|≤ξj |y−x |, |
|gi(y)−gi(x)|≤ζi |y−x |, |
where ξj, ζi>0 are constants, with j=1,2,..,m,i=1,2,…,n.
Assumption 2.2. If fj(·) and gi(·) are continuous bounded functions, then for any xj、yi∈R, a constant pj,qi>0 exists such that
|fj(xj)|≤pj,j=1,2…..m, |
|gi(yi)|≤qi,i=1,2…..n. |
Assumption 2.3. If the functions Ii(t) and Hj(t) are bounded, then for any t, nonnegative constants Imi, Hmj exist such that the following conditions hold:
|Ii(t)|≤Imi,i=1,2,…,n, |
|Hj(t)|≤Hmj,j=1,2,…,m. |
Definition 2.1. [25] A constant t′>0 exists such that the error of the drive and response system satisfies that limt→t'exi(t)=limt→t'eyj(t)=0 for ∀t≥t′; also, exi(t)≡0, and eyj(t)≡0 hold. Thus, we claim that the drive and response system can achieve finite time synchronization, and t′ is the finite synchronization time of the system.
Definition 2.2. [26] Suppose that constant t0(e(0))≥0 satisfies the following conditions:
{limt→t0(e(0))‖exi(t)‖=limt→t0(e(0))‖ xi(t)−αixi(t)‖=0,limt→t0(e(0))‖eyj(t)‖=limt→t0(e(0))‖ yj(t)−βjyj(t)‖=0, |
where i = \mathrm{1, 2}, \dots, n;j = \mathrm{1, 2}, \dots, m;\left|{\alpha }_{i}\right|\le ℊ, \left|{\beta }_{j}\right|\le \mathcal{\hslash }; \mathcal{ }\mathcal{ }\mathcal{ }\mathcal{ }ℊ and \mathcal{\hslash } are constants; {t}_{0}\left(e\left(0\right)\right) depends on the initial value e\left(0\right) , and e\left(t\right) = ({e}_{1}^{x}\left(t\right), {e}_{2}^{x}\left(t\right), \dots, {e}_{n}^{x}\left(t\right), {e}_{1}^{y}\left(t\right), {e}_{2}^{y}\left(t\right), \dots, {e}_{m}^{y}\left(t\right){)}^{T} . Additionally, the finite-time projection synchronization is achieved between driving system (1) and the response system (4), and {\alpha }_{i} and {\beta }_{i} are the projection coefficients.
In this section, a study on the finite-time projection synchronization problem for bidirectional associative MNNs with leakage, discrete, and distributed delays is presented. Using MHDBAMNNSs (1) and (4), the form of error definition for system-corrected projection synchronization is derived as follows:
{e}_{i}^{x}\left(t\right) = {\stackrel{~}{x}}_{i}\left(t\right)-{\alpha }_{i}{x}_{i}\left(t\right), | (5) |
{e}_{j}^{y}\left(t\right) = {\stackrel{~}{y}}_{j}\left(t\right)-{\beta }_{i}{y}_{j}\left(t\right), | (6) |
where i = 1, \mathrm{ }2, \dots, n , j = \mathrm{1, 2}, \dots, m .
{\dot{e}}_{i}^{x}\left(t\right) = {\dot{\stackrel{~}{x}}}_{i}\left(t\right)-{\alpha }_{i}{\dot{x}}_{i}\left(t\right), | (7) |
{\dot{e}}_{j}^{y}\left(t\right) = {\dot{\stackrel{~}{y}}}_{j}\left(t\right)-{\beta }_{i}{\dot{y}}_{j}\left(t\right). | (8) |
Theorem 3.1. To realize the projection synchronization of a memristor-based neural network, under the condition that Assumptions 2.1-2.3 hold, a delayed independent negative feedback controller based on the systematic error can be designed as follows:
\left\{\begin{array}{c}{u}_{i}\left(t\right) = -{\lambda }_{1i}sign{(e}_{i}^{x}\left(t\right))-{\lambda }_{2i}{e}_{i}^{x}\left(t\right), \\ {v}_{j}\left(t\right) = -{\mu }_{1j}sign\left({e}_{j}^{y}\right(t\left)\right)-{\mu }_{2j}{e}_{j}^{y}\left(t\right), \end{array}\right. | (9) |
where i = \mathrm{1, 2}, \dots, n , j = \mathrm{1, 2}, \dots, m , {\lambda }_{1i} , {\lambda }_{2i} , {\mu }_{1j} , {\mu }_{2j} are parameters of the controller given by (9) and the following conditions are derived:
{\lambda }_{1i}\ge \left(1+ℊ\right)\sum _{j = 1}^{n}\left({a}_{ji}^{m}+{b}_{ji}^{m}+{\rho }_{0}{c}_{ji}^{m}\right){p}_{j}+\left(1+ℊ\right){I}_{i}^{m}, |
{\mu }_{1j}\ge \left(1+ℏ\right)\sum _{i = 1}^{n}\left({k}_{ij}^{m}+{l}_{ij}^{m}+{\rho }_{0}{m}_{ij}^{m}\right){q}_{i}-\left(1+ℏ\right){H}_{j}^{m}, |
{\lambda }_{2i} > {\delta }_{i}+\sum _{j = 1}^{n}{\zeta }_{j}({k}_{ji}^{m}+\frac{{l}_{ji}^{m}}{1-{\tau }_{1}}+{\rho }_{0}{m}_{ji}^{m}), |
{\mu }_{2j} > {\omega }_{j}+\sum _{i = 1}^{m}{{\xi }_{j}(a}_{ij}^{m}+\frac{{b}_{ij}^{m}}{1-{\tau }_{1}}+{\rho }_{0}{c}_{ij}^{m}), |
where i = \mathrm{1, 2}, \dots, n , j = \mathrm{1, 2}, \dots, m . Then, MHDBAMNNSs (1) and (4) under controller (9) can achieve projection synchronization in finite time, and the time can be calculated as follows:
{t}_{0}^{{{'}}}\le \frac{V\left(0\right)}{\varpi }, | (10) |
where
V\left(0\right) = \sum _{i = 1}^{n}\left|{e}_{i}^{x}\left(0\right)\right|+\sum _{i = 1}^{n}{\delta }_{i}{\int }_{-\sigma }^{0}\left|{e}_{i}^{x}\left(v\right)\right|dv+\sum _{i = 1}^{n}\sum _{j = 1}^{n}\frac{{\xi }_{j}{b}_{ji}^{m}}{1-{\tau }_{1}}{\int }_{-\tau \left(0\right)}^{0}\left|{e}_{j}^{y}\left(v\right)\right|dv |
+\sum _{i = 1}^{n}\sum _{j = 1}^{n}{\xi }_{j}{c}_{ji}^{m}{\int }_{-{\rho }_{0}}^{0}{\int }_{v}^{0}\left|{e}_{j}^{y}\left(w\right)\right|dwdv+\sum _{j = 1}^{m}\left|{e}_{j}^{y}\left(0\right)\right| |
+\sum _{j = 1}^{m}{\omega }_{j}{\int }_{-\sigma }^{0}\left|{e}_{j}^{y}\left(v\right)\right|dv+\sum _{j = 1}^{m}\sum _{i = 1}^{m}\frac{{\zeta }_{i}{l}_{ij}^{m}}{1-{\tau }_{1}}{\int }_{-\tau \left(0\right)}^{0}\left|{e}_{i}^{x}\left(v\right)\right|dv |
+\sum _{j = 1}^{m}\sum _{i = 1}^{m}{\zeta }_{i}{m}_{ij}^{m}{\int }_{-{\rho }_{0}}^{0}{\int }_{v}^{0}\left|{e}_{i}^{x}\left(w\right)\right|dwdv, |
\varpi = \underset{\begin{array}{c}1\le i\le n\\ 1\le j\le m\end{array}}{\mathit{min}}\{{\lambda }_{1i}-\left(1+ℊ\right)\sum _{j = 1}^{n}\left({a}_{ji}^{m}+{b}_{ji}^{m}+{\rho }_{0}{c}_{ji}^{m}\right){p}_{j}-\left(1+ℊ\right){I}_{i}^{m} , |
{\mu }_{1j}-\left(1+ℏ\right)\sum _{i = 1}^{n}\left({k}_{ij}^{m}+{l}_{ij}^{m}+{\rho }_{0}{m}_{ij}^{m}\right){q}_{i}-\left(1+ℏ\right){H}_{j}^{m} \} > 0. |
Proof of Theorem 3.1. Consider the following Lyapunov-Krasovsky function:
V\left(t\right) = \sum _{k = 1}^{8}{V}_{k}\left(t\right), |
where
{V}_{1}\left(t\right) = \sum _{i = 1}^{n}\left|{e}_{i}^{x}\left(t\right)\right|, |
{V}_{2}\left(t\right) = \sum _{i = 1}^{n}{\delta }_{i}{\int }_{t-\sigma }^{t}\left|{e}_{i}^{x}\left(v\right)\right|dv, |
{V}_{3}\left(t\right) = \sum _{i = 1}^{n}\sum _{j = 1}^{n}\frac{{\xi }_{j}{b}_{ji}^{m}}{1-{\tau }_{1}}{\int }_{t-\tau \left(t\right)}^{t}\left|{e}_{j}^{y}\left(v\right)\right|dv, |
{V}_{4}\left(t\right) = \sum _{i = 1}^{n}\sum _{j = 1}^{n}{\xi }_{j}{c}_{ji}^{m}{\int }_{-{\rho }_{0}}^{0}{\int }_{t+v}^{t}\left|{e}_{j}^{y}\left(w\right)\right|dwdv, |
{V}_{5}\left(t\right) = \sum _{j = 1}^{m}\left|{e}_{j}^{y}\left(t\right)\right|, |
{V}_{6}\left(t\right) = \sum _{j = 1}^{m}{\omega }_{j}{\int }_{t-\sigma }^{t}\left|{e}_{j}^{y}\left(v\right)\right|dv, |
{V}_{7}\left(t\right) = \sum _{j = 1}^{m}\sum _{i = 1}^{m}\frac{{\zeta }_{i}{l}_{ij}^{m}}{1-{\tau }_{1}}{\int }_{t-\tau \left(t\right)}^{t}\left|{e}_{i}^{x}\left(v\right)\right|dv, |
{V}_{8}\left(t\right) = \sum _{j = 1}^{m}\sum _{i = 1}^{m}{\zeta }_{i}{m}_{ij}^{m}{\int }_{-{\rho }_{0}}^{0}{\int }_{t+v}^{t}\left|{e}_{i}^{x}\left(w\right)\right|dwdv. |
Thus, the derivative of {V}_{1}\left(t\right) can be calculated as follows:
{\dot{V}}_{1}\left(t\right) = \sum _{i = 1}^{n}sign\left({e}_{i}^{x}\left(t\right)\right){\dot{e}}_{i}^{x}\left(t\right) = \sum _{i = 1}^{n}sign\left({e}_{i}^{x}\left(t\right)\right)\left[{\dot{\stackrel{~}{x}}}_{i}\left(t\right)-{\alpha }_{i}{\dot{x}}_{i}\left(t\right)\right] |
= \sum _{i = 1}^{n}sign\left({e}_{i}^{x}\left(t\right)\right)\{-{\delta }_{i}{\stackrel{~}{x}}_{i}\left(t-\sigma \right)+{\alpha }_{i}{\delta }_{i}{x}_{i}\left(t-\sigma \right) |
+\sum _{j = 1}^{m}{a}_{ji}\left({\stackrel{~}{x}}_{i}\left(t\right)\right){f}_{j}\left({\stackrel{~}{y}}_{j}\left(t\right)\right)-\sum _{j = 1}^{m}{a}_{ji}\left({x}_{i}\left(t\right)\right){f}_{j}\left({{\beta }_{j}y}_{j}\left(t\right)\right)+\sum _{j = 1}^{m}{a}_{ji}\left({x}_{i}\left(t\right)\right){f}_{j}\left({{\beta }_{j}y}_{j}\left(t\right)\right) |
-{\alpha }_{i}\sum _{j = 1}^{m}{a}_{ji}\left({x}_{i}\left(t\right)\right){f}_{j}\left({y}_{j}\left(t\right)\right)+\sum _{j = 1}^{m}{b}_{ji}\left({\stackrel{~}{x}}_{i}\left(t\right)\right)·{f}_{j}\left({\stackrel{~}{y}}_{j}\left(t-\tau \left(t\right)\right)\right) |
-\sum _{j = 1}^{m}{b}_{ji}\left({x}_{i}\left(t\right)\right){f}_{j}\left({{\beta }_{j}y}_{j}\left(t-\tau \left(t\right)\right)\right)+\sum _{j = 1}^{m}{b}_{ji}\left({x}_{i}\left(t\right)\right){f}_{j}\left({{\beta }_{j}y}_{j}\left(t-\tau \left(t\right)\right)\right) |
-{\alpha }_{i}\sum _{j = 1}^{m}{b}_{ji}\left({x}_{i}\left(t\right)\right){f}_{j}\left({y}_{j}\left(t-\tau \left(t\right)\right)\right)+\sum _{j = 1}^{m}{c}_{ji}\left({\stackrel{~}{x}}_{i}\left(t\right)\right){\int }_{t-\rho \left(t\right)}^{t}{f}_{j}\left({\stackrel{~}{y}}_{j}\left(v\right)\right)dv |
-\sum _{j = 1}^{m}{c}_{ji}\left({x}_{i}\left(t\right)\right){\int }_{t-\rho \left(t\right)}^{t}{f}_{j}\left({{\beta }_{j}y}_{j}\left(v\right)\right)dv+\sum _{j = 1}^{m}{c}_{ji}\left({x}_{i}\left(t\right)\right){\int }_{t-\rho \left(t\right)}^{t}{f}_{j}\left({{\beta }_{j}y}_{j}\left(v\right)\right)dv |
-{\alpha }_{i}\sum _{j = 1}^{m}{c}_{ji}\left({x}_{i}\left(t\right)\right){\int }_{t-\rho \left(t\right)}^{t}{f}_{j}\left({y}_{j}\left(v\right)\right)dv+\left(1-{\alpha }_{i}\right){I}_{i}\left(t\right)+{u}_{i}\left(t\right)\} |
= \sum _{i = 1}^{n}sign\left({e}_{i}^{x}\left(t\right)\right)·\{-{\delta }_{i}{e}_{i}^{x}\left(t-\sigma \right)+\sum _{j = 1}^{m}{a}_{ji}^{x}\left(t\right){\Lambda }_{j}\left({e}_{j}^{y}\left(t\right)\right)+\sum _{j = 1}^{m}{a}_{ji}^{x}\left(t\right){f}_{j}\left({{\beta }_{j}y}_{j}\left(t\right)\right) |
-{\alpha }_{i}\sum _{j = 1}^{m}{a}_{ji}^{x}\left(t\right){f}_{j}\left({y}_{j}\left(t\right)\right)+\sum _{j = 1}^{m}{b}_{ji}^{x}\left(t\right){\Lambda }_{j}\left({e}_{j}^{y}\left(t-\tau \left(t\right)\right)\right) |
+\sum _{j = 1}^{m}{b}_{ji}^{x}\left(t\right){f}_{j}\left({{\beta }_{j}y}_{j}\left(t-\tau \left(t\right)\right)\right)-{\alpha }_{i}\sum _{j = 1}^{m}{b}_{ji}^{x}\left(t\right)·{f}_{j}\left({y}_{j}\left(t-\tau \left(t\right)\right)\right) |
+\sum _{j = 1}^{m}{c}_{ji}^{x}\left(t\right){\int }_{t-\rho \left(t\right)}^{t}{\Lambda }_{j}\left({e}_{j}^{y}\left(v\right)\right)dv+\sum _{j = 1}^{m}{c}_{ji}^{x}\left(t\right){\int }_{t-\rho \left(t\right)}^{t}{f}_{j}\left({{\beta }_{j}y}_{j}\left(v\right)\right)dv |
-{\alpha }_{i}\sum _{j = 1}^{m}{c}_{ji}^{x}\left(t\right){\int }_{t-\rho \left(t\right)}^{t}{f}_{j}\left({y}_{j}\left(v\right)\right)dv+(1-{\alpha }_{i}){I}_{i}\left(t\right)+{u}_{i}\left(t\right)\}, |
where {\mathrm{\Lambda }}_{j}\left({e}_{j}^{y}\left(t\right)\right) = {f}_{j}\left({\stackrel{~}{y}}_{j}\left(t\right)\right)-{f}_{j}\left({{\beta }_{j}y}_{j}\left(t\right)\right) , i = \mathrm{1, 2}, \dots, n and j = \mathrm{1, 2}, \dots, m . Given that Assumptions 2.1-2.3 hold, each term of {\dot{V}}_{1}\left(t\right) can be computed separately by using the linear matrix inequality method, as follows:
sign\left({e}_{i}^{x}\left(t\right)\right)\left[-{\delta }_{i}{e}_{i}^{x}\left(t-\sigma \right)\right]\le {\delta }_{i}\left|{e}_{i}^{x}\left(t-\sigma \right)\right|, |
sign\left({e}_{i}^{x}\left(t\right)\right)[\sum _{j = 1}^{m}{a}_{ji}^{x}\left(t\right){\mathrm{\Lambda }}_{j}\left({e}_{j}^{y}\left(t\right)\right)]\le \sum _{j = 1}^{m}\left|{a}_{ji}^{x}\left(t\right)\right|{\xi }_{j}\left|{e}_{j}^{y}\right(t\left)\right|)\le \sum _{j = 1}^{m}{a}_{ji}^{m}{\xi }_{j}\left|{e}_{j}^{y}\left(t\right)\right|, |
sign\left({e}_{i}^{x}\left(t\right)\right)[\sum _{j = 1}^{m}{b}_{ji}^{x}\left(t\right){\mathrm{\Lambda }}_{j}\left({e}_{j}^{y}\left(t-\tau \left(t\right)\right)\right)]\le \sum _{j = 1}^{m}\left|{b}_{ji}^{x}\left(t\right)\right|{\xi }_{j}\left|{e}_{j}^{y}\right(t-\tau \left(t\right)\left)\right|) |
\le \sum _{j = 1}^{m}{b}_{ji}^{m}{\xi }_{j}\left|{e}_{j}^{y}\left(t-\tau \left(t\right)\right)\right|. |
The model precondition 0\le \rho \left(t\right)\le {\rho }_{0} , combined with Assumption 2.1, yields the following conclusion:
sign\left({e}_{i}^{x}\left(t\right)\right)\left[\sum _{j = 1}^{m}{c}_{ji}^{x}\left(t\right){\int }_{t-\rho \left(t\right)}^{t}{\mathrm{\Lambda }}_{j}\left({e}_{j}^{y}\left(v\right)\right)dv\right]\le \sum _{j = 1}^{m}\left|{c}_{ji}^{x}\left(t\right)\right|{\int }_{t-\rho \left(t\right)}^{t}{\xi }_{j}\left|{e}_{j}^{y}\left(v\right)\right|dv |
\le \sum _{j = 1}^{m}{c}_{ji}^{m}{\xi }_{j}{\int }_{t-{\rho }_{0}}^{t}\left|{e}_{j}^{y}\left(v\right)\right|dv. |
Under Assumption 2.2, the linear matrix inequality method yields
sign\left({e}_{i}^{x}\left(t\right)\right)\left[\sum _{j = 1}^{m}{a}_{ji}^{x}\left(t\right){f}_{j}\left({{\beta }_{j}y}_{j}\left(t\right)\right)\right]\le \sum _{j = 1}^{m}{\gamma }_{i}\left|{a}_{ji}^{x}\left(t\right)\right|\left|{f}_{j}\left({{\beta }_{j}y}_{j}\left(t\right)\right)\right| |
\le \sum _{j = 1}^{m}{\gamma }_{i}\left|{a}_{ji}^{x}\left(t\right)\right|{p}_{j}\le \sum _{j = 1}^{m}{a}_{ji}^{m}{p}_{j}{\gamma }_{i}, |
where {e}_{i}^{x}\left(t\right) = 0 at {\gamma }_{i} = 0 ; otherwise, {\gamma }_{i} = 1 . It can be deduced as follows:
sign\left({e}_{i}^{x}\left(t\right)\right)[-{\alpha }_{i}\sum _{j = 1}^{m}{a}_{ji}^{x}\left(t\right){f}_{j}\left({y}_{j}\left(t\right)\right)]\le \left|{\alpha }_{i}\right|\sum _{j = 1}^{m}{\gamma }_{i}\left|{a}_{ji}^{x}\left(t\right)\right|{p}_{j}\le \left|{\alpha }_{i}\right|\sum _{j = 1}^{m}{a}_{ji}^{m}{p}_{j}{\gamma }_{i}, |
sign\left({e}_{i}^{x}\left(t\right)\right)[\sum _{j = 1}^{m}{b}_{ji}^{x}\left(t\right){f}_{j}\left({{\beta }_{j}y}_{j}\left(t-\tau \left(t\right)\right)\right)]\le \sum _{j = 1}^{m}{\gamma }_{i}\left|{b}_{ji}^{x}\left(t\right)\right|{p}_{j}\le \sum _{j = 1}^{m}{b}_{ji}^{m}{p}_{j}{\gamma }_{i}, |
sign\left({e}_{i}^{x}\left(t\right)\right)[-{\alpha }_{i}\sum _{j = 1}^{m}{b}_{ji}^{x}\left(t\right)·{f}_{j}({y}_{j}(t-\tau \left(t\right))\left)\right]\le \left|{\alpha }_{i}\right|\sum _{j = 1}^{m}{\gamma }_{i}\left|{b}_{ji}^{x}\left(t\right)\right|{p}_{j}\le \left|{\alpha }_{i}\right|\sum _{j = 1}^{m}{b}_{ji}^{m}{p}_{j}{\gamma }_{i}, |
sign\left({e}_{i}^{x}\left(t\right)\right)\left[\sum _{j = 1}^{m}{c}_{ji}^{x}\left(t\right){\int }_{t-\rho \left(t\right)}^{t}{f}_{j}\left({{\beta }_{j}y}_{j}\left(v\right)\right)dv\right]\le \sum _{j = 1}^{m}{\gamma }_{i}\left|{c}_{ji}^{x}\left(t\right)\right|{\int }_{t-{\rho }_{0}}^{t}\left|{f}_{j}\left({{\beta }_{j}y}_{j}\left(t\right)\right)\right|dv |
\le {\rho }_{0}\sum _{j = 1}^{m}{c}_{ji}^{m}{p}_{j}{\gamma }_{i}, |
sign\left({e}_{i}^{x}\left(t\right)\right)[-{\alpha }_{i}\sum _{j = 1}^{m}{c}_{ji}^{x}\left(t\right){\int }_{t-\rho \left(t\right)}^{t}{f}_{j}\left({y}_{j}\left(v\right)\right)dv]\le {\rho }_{0}\left|{\alpha }_{i}\right|\sum _{j = 1}^{m}{\gamma }_{i}\left|{c}_{ji}^{x}\left(t\right)\right|{\int }_{t-{\rho }_{0}}^{t}\left|{f}_{j}\left({y}_{j}\left(v\right)\right)\right|dv |
\le {\rho }_{0}\left|{\alpha }_{i}\right|\sum _{j = 1}^{m}{c}_{ji}^{m}{p}_{j}{\gamma }_{i}. |
Additionally, Assumption 2.3 yields
sign\left({e}_{i}^{x}\left(t\right)\right)\left(1-{\alpha }_{i}\right){I}_{i}\left(t\right)\le \left|sign\left({e}_{i}^{x}\left(t\right)\right)\right|\left|\left(1-{\alpha }_{i}\right){I}_{i}\left(t\right)\right|\le \left|1-{\alpha }_{i}\right|{I}_{i}^{m}{\gamma }_{i}, |
sign\left({e}_{i}^{x}\left(t\right)\right){u}_{i}\left(t\right) = sign\left({e}_{i}^{x}\left(t\right)\right)\left[-{\lambda }_{1i}sign\left({e}_{i}^{x}\left(t\right)\right)-{\lambda }_{2i}{e}_{i}^{x}\left(t\right)\right] = -{\lambda }_{1i}{\gamma }_{i}-{\lambda }_{2i}\left|{e}_{i}^{x}\left(t\right)\right|. |
Thus, the derivatives of {V}_{2}\left(t\right) , {V}_{3}\left(t\right) , and {V}_{4}\left(t\right) can be calculated as follows:
{\dot{V}}_{2}\left(t\right) = \sum _{i = 1}^{n}{\delta }_{i}\left[\left|{e}_{i}^{x}\left(t\right)\right|-\left|{e}_{i}^{x}\left(t-\sigma \right)\right|\right], |
{\dot{V}}_{3}\left(t\right) = \sum _{i = 1}^{n}\sum _{j = 1}^{n}\frac{{\xi }_{j}{b}_{ji}^{m}}{1-{\tau }_{1}}\left[\left|{e}_{j}^{y}\left(t\right)\right|-\left(1-\dot{\tau }\left(t\right)\right)\left|{e}_{i}^{y}\left(t-\tau \left(t\right)\right)\right|\right] |
\le \sum _{i = 1}^{n}\sum _{j = 1}^{n}\frac{{\xi }_{j}{b}_{ji}^{m}}{1-{\tau }_{1}}\left|{e}_{j}^{y}\left(t\right)\right|-\sum _{i = 1}^{n}\sum _{j = 1}^{n}{\xi }_{j}{b}_{ji}^{m}\left|{e}_{j}^{y}\left(t-\tau \left(t\right)\right)\right|, |
{\dot{V}}_{4}\left(t\right) = \sum _{i = 1}^{n}\sum _{j = 1}^{n}{\xi }_{j}{c}_{ji}^{m}{\int }_{-{\rho }_{0}}^{0}\left[\left|{e}_{j}^{y}\left(t\right)\right|-\left|{e}_{j}^{y}\left(t+v\right)\right|\right]dv |
= {\rho }_{0}\sum _{i = 1}^{n}\sum _{j = 1}^{n}{\xi }_{j}{c}_{ji}^{m}\left|{e}_{j}^{y}\left(t\right)\right|-\sum _{i = 1}^{n}\sum _{j = 1}^{n}{\xi }_{j}{c}_{ji}^{m}{\int }_{t-{\rho }_{0}}^{t}\left|{e}_{j}^{y}\left(v\right)\right|dv. |
Given that \left|{\alpha }_{i}\right|\le ℊ and \left|{\beta }_{j}\right|\le ℏ , in summary, it can be obtained that
{\dot{V}}_{1}\left(t\right)+{\dot{V}}_{2}\left(t\right)+{\dot{V}}_{3}\left(t\right)+{\dot{V}}_{4}\left(t\right) |
\le {\sum }_{i = 1}^{n}\sum _{j = 1}^{n}{[\xi }_{j}({a}_{ji}^{m}+\frac{{b}_{ji}^{m}}{1-{\tau }_{1}}+{\rho }_{0}{c}_{ji}^{m})\left]\right|{e}_{j}^{y}\left(t\right)|+\sum _{i = 1}^{n}{(\delta }_{i}-{\lambda }_{2i}\left)\right|{e}_{i}^{x}\left(t\right)| |
-\sum _{i = 1}^{n}\left[{\lambda }_{1i}-\left(1+ℊ\right)\sum _{j = 1}^{n}({a}_{ji}^{m}+{b}_{ji}^{m}+{\rho }_{0}{c}_{ji}^{m}){p}_{j}-(1+ℊ)|{\mathrm{I}}_{\mathrm{i}}^{\mathrm{m}}\right]{\gamma }_{i}. |
Similarly, it can be determined that
{\dot{V}}_{4}\left(t\right)+{\dot{V}}_{5}\left(t\right)+{\dot{V}}_{6}\left(t\right)+{\dot{V}}_{7}\left(t\right) |
\le {\sum }_{j = 1}^{n}\sum _{i = 1}^{n}{[\zeta }_{i}({k}_{ij}^{m}+\frac{{l}_{ij}^{m}}{1-{\tau }_{1}}+{\rho }_{0}{m}_{ij}^{m})\left]\right|{e}_{i}^{x}\left(t\right)|+\sum _{j = 1}^{n}\left({\omega }_{j}-{\mu }_{2j}\right)\left|{e}_{j}^{y}\left(t\right)\right| |
-\sum _{j = 1}^{n}[{\mu }_{1j}-\left(1+ℏ\right)\sum _{i = 1}^{n}\left({k}_{ij}^{m}+{l}_{ij}^{m}+{\rho }_{0}{m}_{ij}^{m}\right){q}_{i}-\left(1+ℏ\right){H}_{j}^{m}]{\gamma }_{j}, |
where {e}_{j}^{y}\left(t\right) = 0 at {\gamma }_{j} = 0 ; otherwise, {\gamma }_{j} = 1 . Thus, we obtain
\dot{V}\left(t\right)\le {\sum }_{j = 1}^{n}\sum _{i = 1}^{n}{[{\delta }_{i}-{\lambda }_{2i}+\zeta }_{i}({k}_{ij}^{m}+\frac{{l}_{ij}^{m}}{1-{\tau }_{1}}+{\rho }_{0}{m}_{ij}^{m})\left]\right|{e}_{i}^{x}\left(t\right)| |
-\sum _{i = 1}^{n}[{\lambda }_{1i}-\left(1+ℊ\right)\sum _{j = 1}^{n}\left({a}_{ji}^{m}+{b}_{ji}^{m}+{\rho }_{0}{c}_{ji}^{m}\right){p}_{j}-\left(1+ℊ\right){I}_{i}^{m}]{\gamma }_{i} |
+{\sum }_{i = 1}^{n}\sum _{j = 1}^{n}{[{\omega }_{j}-{\mu }_{2j}+\xi }_{j}({a}_{ji}^{m}+\frac{{b}_{ji}^{m}}{1-{\tau }_{1}}+{\rho }_{0}{c}_{ji}^{m})\left]\right|{e}_{j}^{y}\left(t\right)| |
-\sum _{j = 1}^{n}[{\mu }_{1j}-\left(1+ℏ\right)\sum _{i = 1}^{n}\left({k}_{ij}^{m}+{l}_{ij}^{m}+{\rho }_{0}{m}_{ij}^{m}\right){q}_{i}-\left(1+ℏ\right){H}_{j}^{m}]{\gamma }_{j}. |
Given that {\lambda }_{1i} , {\lambda }_{2i} , {\mu }_{1j} , and {\mu }_{2j} satisfy the conditions in Theorem 3.1, V\left(t\right) can be derived as follows:
\dot{V}\left(t\right)\le -\sum _{i = 1}^{n}[{\lambda }_{1i}-\left(1+ℊ\right)\sum _{j = 1}^{n}\left({a}_{ji}^{m}+{b}_{ji}^{m}+{\rho }_{0}{c}_{ji}^{m}\right){p}_{j}-\left(1+ℊ\right){I}_{i}^{m}]{\gamma }_{i} |
-\sum _{j = 1}^{n}[{\mu }_{1j}-\left(1+ℏ\right)\sum _{i = 1}^{n}\left({k}_{ij}^{m}+{l}_{ij}^{m}+{\rho }_{0}{m}_{ij}^{m}\right){q}_{i}-\left(1+ℏ\right){H}_{j}^{m}]{\gamma }_{j} |
\le -\varpi (\sum _{i = 1}^{n}{\gamma }_{i}+\sum _{j = 1}^{m}{\gamma }_{j}), |
where \varpi = \underset{\begin{array}{c}1\le i\le n\\ 1\le j\le m\end{array}}{\mathit{min}}\{{\lambda }_{1i}-\left(1+ℊ\right)\sum _{j = 1}^{n}\left({a}_{ji}^{m}+{b}_{ji}^{m}+{\rho }_{0}{c}_{ji}^{m}\right){p}_{j}-\left(1+ℊ\right){I}_{i}^{m} ,
{\mu }_{1j}-\left(1+ℏ\right)\sum _{i = 1}^{n}\left({k}_{ij}^{m}+{l}_{ij}^{m}+{\rho }_{0}{m}_{ij}^{m}\right){q}_{i}-\left(1+ℏ\right){H}_{j}^{m} \} > 0 . |
When \left|\right|{e}_{i}^{x}\left(t\right)\left|\right|\ne 0 and \left|\right|{e}_{j}^{y}\left(t\right)\left|\right|\ne 0 , \dot{V}\left(t\right)\le -\varpi . Finite-time synchronization analysis of Definition 2.1 indicates that for \forall t\in \left[0, {t}_{0}\right] , V\left({t}_{0}\right)-V\left(0\right)\le -\varpi {t}_{0} . The above-mentioned properties of V\left(t\right) suggest that a constant {t}_{0} = V\left(0\right)/\varpi exists, which satisfies that \underset{t\to {t}_{0}}{\mathit{lim}}\left|\right|e\left(t\right)\left|\right| = 0 and \left|\right|e\left(t\right)\left|\right|\equiv 0 , \forall t\ge {t}_{0} .
By setting {t}_{0}^{{{'}}} = \mathrm{inf}\left\{t\in \left(0, {t}_{0}\right]:\left|\right|e\left({t}^{{{'}}}\right)\left|\right|\equiv 0, {t}^{{{'}}} > t\right\} , we obtain that \underset{t\to {t}_{0}^{\text{'}}}{\mathit{lim}}\left|\right|e\left(t\right)\left|\right| = 0 and \left|\right|e\left(t\right)\left|\right|\equiv 0 for \forall t\ge {t}_{0}^{{{'}}} . The MHDBAMNNs (1) and (4) can be synchronized with finite-time projections according to Definition 2.2. Thus, the proof of Theorem 3.1 is complete.
In this section, numerical simulation examples are provided to demonstrate the validity of the obtained results and their potential applications in image encryption. Here, MHDBAMNNs based on Eqs (11) and (12) as the drive system and its corresponding response system with m = 2 and n = 2 , respectively, are considered as follows:
\left\{\begin{array}{c}{\dot{x}}_{i}\left(t\right) = -{\delta }_{i}{x}_{i}\left(t-\sigma \right)+\sum _{j = 1}^{2}{a}_{ji}\left({x}_{i}\left(t\right)\right){f}_{j}\left({y}_{j}\left(t\right)\right)+\sum _{j = 1}^{2}{b}_{ji}\left({x}_{i}\left(t\right)\right)·{f}_{j}\left({y}_{j}\left(t-\tau \left(t\right)\right)\right)\\ +\sum _{j = 1}^{2}{c}_{ji}\left({x}_{i}\left(t\right)\right){\int }_{t-\rho \left(t\right)}^{t}{f}_{j}\left({y}_{j}\left(v\right)\right)dv+{I}_{i}\left(t\right), \\ {\dot{y}}_{j}\left(t\right) = -{\omega }_{j}{y}_{j}\left(t-\sigma \right)+\sum _{i = 1}^{2}{k}_{ij}\left({y}_{j}\left(t\right)\right){g}_{j}\left({x}_{j}\left(t\right)\right)+\sum _{i = 1}^{2}{l}_{ij}\left({y}_{j}\left(t\right)\right)· {g}_{j}\left({x}_{j}\left(t-\tau \left(t\right)\right)\right)\\ +\sum _{i = 1}^{2}{m}_{ij}\left({y}_{j}\left(t\right)\right){\int }_{t-\rho \left(t\right)}^{t}{g}_{j}\left({x}_{j}\left(v\right)\right)dv+{H}_{j}\left(t\right), \end{array}\right. | (11) |
\left\{\begin{array}{c}{\dot{\stackrel{~}{x}}}_{i}\left(t\right) = -{\delta }_{i}{\stackrel{~}{x}}_{i}\left(t-\sigma \right)+\sum _{j = 1}^{2}{a}_{ji}\left({\stackrel{~}{x}}_{i}\left(t\right)\right){f}_{j}\left({\stackrel{~}{y}}_{j}\left(t\right)\right)+\sum _{j = 1}^{2}{b}_{ji}\left({\stackrel{~}{x}}_{i}\left(t\right)\right)·{f}_{j}\left({\stackrel{~}{y}}_{j}\left(t-\tau \left(t\right)\right)\right)\\ +\sum _{j = 1}^{2}{c}_{ji}\left({\stackrel{~}{x}}_{i}\left(t\right)\right){\int }_{t-\rho \left(t\right)}^{t}{f}_{j}\left({\stackrel{~}{y}}_{j}\left(v\right)\right)dv+{u}_{i}\left(t\right)+{I}_{i}\left(t\right), \\ {\dot{\stackrel{~}{y}}}_{j}\left(t\right) = -{\omega }_{j}{\stackrel{~}{y}}_{j}\left(t-\sigma \right)+\sum _{i = 1}^{2}{k}_{ij}\left({\stackrel{~}{y}}_{j}\left(t\right)\right){g}_{j}\left({\stackrel{~}{x}}_{j}\left(t\right)\right)+\sum _{i = 1}^{2}{l}_{ij}\left({\stackrel{~}{y}}_{j}\left(t\right)\right)· {g}_{j}\left({\stackrel{~}{x}}_{j}\left(t-\tau \left(t\right)\right)\right)\\ +\sum _{i = 1}^{2}{m}_{ij}\left({\stackrel{~}{y}}_{j}\left(t\right)\right){\int }_{t-\rho \left(t\right)}^{t}{g}_{j}\left({\stackrel{~}{x}}_{j}\left(v\right)\right)dv+{v}_{i}\left(t\right)+{H}_{j}\left(t\right), \end{array}\right. | (12) |
where {\delta }_{1} = 2.5, {\delta }_{2} = 2.2, {\omega }_{1} = 2.3 , and {\omega }_{2} = 2.1 , with the following memristor parameters:
{a}_{11}\left(\gamma \right) = \left\{\begin{array}{c}0.1, \left|\gamma \right| < 1, \\ -0.8, \left|\gamma \right| > 1, \end{array}\right. {a}_{21}\left(\gamma \right) = \left\{\begin{array}{c}0.9, \left|\gamma \right| < 1, \\ -0.5, \left|\gamma \right| > 1, \end{array}\right. |
{a}_{12}\left(\gamma \right) = \left\{\begin{array}{c}1.8, \left|\gamma \right| < 1, \\ 0.9, \left|\gamma \right| > 1, \end{array}\right. {a}_{22}\left(\gamma \right) = \left\{\begin{array}{c}-0.3, \left|\gamma \right| < 1, \\ 0.6, \left|\gamma \right| > 1, \end{array}\right. |
{b}_{11}\left(\gamma \right) = \left\{\begin{array}{c}2.3, \left|\gamma \right| < 1, \\ 1.7, \left|\gamma \right| > 1, \end{array}\right. {b}_{21}\left(\gamma \right) = \left\{\begin{array}{c}1.2, \left|\gamma \right| < 1, \\ -1.9, \left|\gamma \right| > 1, \end{array}\right. |
{b}_{12}\left(\gamma \right) = \left\{\begin{array}{c}2.8, \left|\gamma \right| < 1, \\ -1.2, \left|\gamma \right| > 1, \end{array}\right. {b}_{22}\left(\gamma \right) = \left\{\begin{array}{c}-0.3, \left|\gamma \right| < 1, \\ 0.5, \left|\gamma \right| > 1, \end{array}\right. |
{c}_{11}\left(\gamma \right) = \left\{\begin{array}{c}0.6, \left|\gamma \right| < 1, \\ -1.5, \left|\gamma \right| > 1, \end{array}\right. {c}_{21}\left(\gamma \right) = \left\{\begin{array}{c}-0.7, \left|\gamma \right| < 1, \\ 1.3, \left|\gamma \right| > 1, \end{array}\right. |
{c}_{12}\left(\gamma \right) = \left\{\begin{array}{c}-1.5, \left|\gamma \right| < 1,\\ -0.9, \left|\gamma \right| > 1,\end{array}\right. {c}_{22}\left(\gamma \right) = \left\{\begin{array}{c}1, \left|\gamma \right| < 1,\\ -0.3, \left|\gamma \right| > 1,\end{array}\right. |
{k}_{11}\left(\gamma \right) = \left\{\begin{array}{c}-2.2, \left|\gamma \right| < 1, \\ 0.7, \left|\gamma \right| > 1, \end{array}\right. {k}_{21}\left(\gamma \right) = \left\{\begin{array}{c}-2.7, \left|\gamma \right| < 1, \\ -0.8, \left|\gamma \right| > 1, \end{array}\right. |
{k}_{12}\left(\gamma \right) = \left\{\begin{array}{c}1, \left|\gamma \right| < 1, \\ -0.6, \left|\gamma \right| > 1, \end{array}\right. {k}_{22}\left(\gamma \right) = \left\{\begin{array}{c}1.2, \left|\gamma \right| < 1, \\ 0.5, \left|\gamma \right| > 1, \end{array}\right. |
{l}_{11}\left(\gamma \right) = \left\{\begin{array}{c}0.75, \left|\gamma \right| < 1, \\ -1, \left|\gamma \right| > 1, \end{array}\right. {l}_{21}\left(\gamma \right) = \left\{\begin{array}{c}2.7, \left|\gamma \right| < 1, \\ 2, \left|\gamma \right| > 1, \end{array}\right. |
{l}_{12}\left(\gamma \right) = \left\{\begin{array}{c}-1.4, \left|\gamma \right| < 1, \\ 0.5, \left|\gamma \right| > 1, \end{array}\right. {l}_{22}\left(\gamma \right) = \left\{\begin{array}{c}2.8, \left|\gamma \right| < 1, \\ -1, \left|\gamma \right| > 1, \end{array}\right. |
{m}_{11}\left(\gamma \right) = \left\{\begin{array}{c}-0.3, \left|\gamma \right| < 1, \\ 1.5, \left|\gamma \right| > 1, \end{array}\right. {m}_{21}\left(\gamma \right) = \left\{\begin{array}{c}-1.8, \left|\gamma \right| < 1, \\ 0.7, \left|\gamma \right| > 1, \end{array}\right. |
{m}_{12}\left(\gamma \right) = \left\{\begin{array}{c}0.2, \left|\gamma \right| < 1, \\ 1.1, \left|\gamma \right| > 1, \end{array}\right. {m}_{22}\left(\gamma \right) = \left\{\begin{array}{c}0.1, \left|\gamma \right| < 1, \\ -1.9, \left|\gamma \right| > 1.\end{array}\right. |
Given these parameters, the activation functions are denoted by {f}_{1}\left(\gamma \right) = {{f}_{2}\left(\gamma \right) = g}_{1}\left(\gamma \right) = {g}_{2}\left(\gamma \right) = (\left|\gamma +1\right|-\left|\gamma -1\right|)/2 ; \sigma = 0.2 , \tau \left(t\right) = 0.3\mathit{cos}\left(t\right)+0.5 , \rho \left(t\right) = 0.6\mathit{sin}\left(t\right)+0.9 ; {I}_{1}\left(t\right) = \mathit{sin}\left(t\right) , {I}_{2}\left(t\right) = \mathit{cos}\left(t\right) , {H}_{1}\left(t\right) = \mathit{cos}\left(2t\right) , and {H}_{2}\left(t\right) = \mathit{sin}\left(2t\right) are the external inputs. Thus, the constraints were computed to obtain {\tau }_{0} = 0.8 , {\rho }_{0} = 1.5 , and {\tau }_{1} = 0.8 . According to Assumptions 2.2 and 2.3, {p}_{1} = {p}_{2} = {q}_{1} = {q}_{2} = 1 , {I}_{1} = {I}_{2} = {H}_{1} = {H}_{2} = 1 , the initial values of the system are given by \phi \left(\chi \right) = {\left(0.8, -1.3\right)}^{T} , \psi \left(\chi \right) = {\left(-\mathrm{1, 0.5}\right)}^{T} , \stackrel{~}{\phi }\left(\chi \right) = {\left(-\mathrm{0.6, 1.1}\right)}^{T} , and \stackrel{~}{\psi }\left(\chi \right) = {\left(0.5, -1\right)}^{T} , and the controller coefficients were calculated as {\lambda }_{11}\ge 18.87 , {\lambda }_{12}\ge 17.765 , {\mu }_{11}\ge 24.735 , {\mu }_{12}\ge 20.23 , and {\lambda }_{21} > 30.85, {\lambda }_{22} > 29.9, {\mu }_{21} > 29.2, {\mu }_{22} > 24.75.
The dynamic trajectories of states x and y in the system are shown in Figures 1 and 2, respectively.
Figures 3(b) and 4(b) show the synchronization errors curves {e}^{x}\left(t\right) and {e}^{y}\left(t\right) , respectively, with projection coefficients {\alpha }_{1} = 0.7 , {\alpha }_{2} = 0.7 , {\beta }_{1} = 0.7 , and {\beta }_{2} = 0.7 . The controller coefficients {\lambda }_{11} = 20 , {\lambda }_{12} = 19 , {\mu }_{11} = 26 , {\mu }_{12} = 21 and {\lambda }_{21} = 32 , {\lambda }_{22} = 31.2 , {\mu }_{21} = 30.5 , {\mu }_{22} = 26 were applied under the control of (9). A comparison of the state trajectories of the synchronization errors {e}^{x}\left(t\right) and {e}^{y}\left(t\right) are shown in Figure 4(a) without controller (9), respectively, revealing the uncontrolled error system is unstable. Accordingly, the driving system and response system were not synchronized. The synchronization simulation results presented in Figures 3(b) and 4(b) indicate that the synchronization errors {e}^{x}\left(t\right) and {e}^{y}\left(t\right) converge to zero within a finite time period of 0.32 seconds. Additionally, the two error systems can be quickly stabilized to the zero point; thus, a finite time projection synchronization could be achieved between systems (11) and (12). Furthermore, the theoretical estimation of the upper bound of the time was determined to be {t}_{0}^{\text{'}} > 5.72 seconds to reach synchronization, and the time of the experimental synchronization was found to be less than the theoretically estimated time {t}_{0}^{\text{'}} , which verifies the validity of the finite-time synchronization theory adopted in Theorem 3.1.
The chaotic attractors of the systems are illustrated in Figure 5. Evidently, Eqs (11) and (12) can be effectively applied to chaotic image algorithms.
Since MHDBAMNNs have large chaotic attractors, the above simulation results can be applied to image encryption experiments. Consider the color image P as the experimental object, whose size is m\times n\times 3 . First, P is divided into two-dimensional matrices comprising red, green and blue components, which greatly reduces the required time and space resources. Second, DNA coding and operations are performed on each 2D matrix chunk; also, after encryption, row and column substitution is performed again to enhance the anti-cropping property, and finally the three channels are merged to obtain the encrypted image. By implementing the MHDBAMNN synchronous simulation algorithm in the drive system (11), we generated three chaotic sequences {x}_{1}, {y}_{1} and {y}_{2} , where the product of {x}_{1} and the average gray value of the three channels is used as a random chaos matrix for the DNA encryption operation on original image, The index sequences obtained by multiplying the average grayscale values of channels {y}_{1} and {y}_{2} with the red and green channels, respectively, determine the permutation of rows and columns after encryption to enhance the scrambling effect of the ciphertext image and resist differential attacks. The initial values of Chen's hyperchaotic system are associated with the original image. The generated four chaotic sequences determine the DNA encoding, decoding, and computational rules for each small block of the image, significantly enhancing the system's resistance to attacks.
The encryption steps are as follows:
1) Split P into three 2D pixel matrices, denoted as {P}_{R}(i, j), {P}_{G}(i, j) and {P}_{B}(i, j) , according to Eq (11), where i\in \{\mathrm{1, 2}, \dots, n\} and j\in \{\mathrm{1, 2}, \dots, m\} . Divide the matrices {P}_{R}(i, j), {P}_{G}(i, j) and {P}_{B}(i, j) into evenly sized blocks of t\times t , where t = 4 .
2) In the synchronous simulation example of the MHDBAMNN, the chaotic sequence {x}_{1} generated by the drive system (11) is multiplied by the average grayscale values {I}_{1} of the three channels. This result is then transformed into a random chaotic matrix Q of size m\times n , where the initial value of sequence m\times n and {I}_{1} serve as one of the encryption keys.
{I}_{1} = sum({P}_{R}\left(:\right)+{P}_{G}\left(:\right)+{P}_{B}(:\left)\right)/(255\times 3\times m\times n) . | (13) |
3) Set the initial values and parameters for the Chen hyperchaotic system. Utilize Matlab built-in functions to compute four sequences \left\{{X}_{i}\right\} , \left\{{Y}_{i}\right\} , \left\{{Z}_{i}\right\} , \left\{{H}_{i}\right\} , where i\in \{\mathrm{1, 2}, \dots, m\times n/{t}^{2}\} . Specifically, the initial value X\left(0\right) is obtained by the bitand operation between {P}_{R}(i, j) and the 1st and 5th bit planes, Y\left(0\right) is derived from {P}_{G}(i, j) with the 2nd and 6th, Z\left(0\right) is calculated by using {P}_{B}(i, j) with the 3rd and 7th, and H\left(0\right) is derived from {P}_{R}(i, j) with the 4th and 8th, as specified in Eqs (14)-(17). Different plaintext images have different initial values; hence, these four initial values also serve as one of the encryption keys.
X\left(0\right) = sum\left(sum\right(bitand({P}_{R}, 17)\left)\right)⁄(17\times m\times n), | (14) |
Y\left(0\right) = sum\left(sum\right(bitand({P}_{G}, 34)\left)\right)⁄(34\times m\times n), | (15) |
Z\left(0\right) = sum\left(sum\right(bitand({P}_{B}, 68)\left)\right)⁄(68\times m\times n), | (16) |
H\left(0\right) = sum\left(sum\right(bitand({P}_{R}, 136)\left)\right)⁄(136\times m\times n). | (17) |
4) Apply the same DNA coding scheme to the corresponding sub-blocks of {P}_{R}(i, j) , {P}_{G}(i, j) and {P}_{B}(i, j) at the same positions to enhance encryption efficiency. The coding is represented by A, G, C, and T, determined by the sequence \left\{{X}_{i}\right\} . The encoding scheme for each sub-block of the Q matrix is determined by \left\{{Y}_{i}\right\} . Apply the same operation rules, determined by \left\{{Z}_{i}\right\} , between the coded {P}_{R}(i, j) , {P}_{G}(i, j) and {P}_{B}(i, j) and the corresponding blocks of Q. Here, {Z}_{i} = 1 represents addition, {Z}_{i} = 2 represents subtraction, {Z}_{i} = 3 represents bitxor operation, and {Z}_{i} = 4 represents the bitand operation. The specific algorithm is outlined in Eqs (18)-(20).
X = mod\left(round\left(X\times {10}^{4}\right), 8\right)+1, | (18) |
Y = mod\left(round\left(Y\times {10}^{4}\right), 8\right)+1, | (19) |
Z = mod\left(round\left(Z\times {10}^{4}\right), 3\right)+1. | (20) |
5) In addition to the first sub-block, the encryption results of the previous sub-block and the current sub-block are again subjected to DNA operations according to \left\{{Z}_{i}\right\} to obtain better diffusion results.
6) Decoding is the inverse process of encoding, where the matrix chunks after DNA operations are DNA decoded by the sequence \left\{{H}_{i}\right\} , i.e., A, G, C, T are decoded into specific values.
7) To perform row substitution and column substitution on the matrix of the three channels after DNA decoding, the index sequence {K}_{1} after multiplying the chaotic sequence {y}_{1} generated by the driving system (11) in the synchronous simulation algorithm of the MHDBAMNNs with the average grey value {I}_{2} of the red channel is multiplied by the index sequence {K}_{1} ; furthermore, the exchange coordinates of {K}_{1} and its corresponding index as the rows are used to determine the post-cryptographic row substitution, and the index sequence {K}_{2} after multiplying the chaotic sequence {y}_{1} generated by the driving system (11) in the synchronous simulation algorithm of the MHDBAMNNs is multiplied by the average grey value {I}_{3} of the green channel. The index sequence {K}_{2} after multiplication, with {K}_{2} and its corresponding index as the exchange coordinates of columns is used to determine the encrypted column replacement; the lengths {K}_{1} and {K}_{2} are denoted by m and n , respectively, as shown in Eqs (21)-(26), where the initial values of the sequences {y}_{1} and {y}_{2} and {I}_{2} and {I}_{3} constitute one of the keys.
{I}_{2} = sum\left({P}_{R}\right(:\left)\right)/(255\times m\times n), | (21) |
{I}_{3} = sum\left({P}_{G}\right(:\left)\right)/(255\times m\times n), | (22) |
{U}_{1} = {y}_{1}\times {I}_{2}, | (23) |
{U}_{2} = {y}_{2}\times {I}_{3}, | (24) |
[\sim, {K}_{1}] = sort({U}_{1}, \text{'}descend\text{'}), | (25) |
\left[\sim , {K}_{2}\right] = sort\left({U}_{2}{, }^{\text{'}}descen{d}^{\text{'}}\right). | (26) |
8) Combine the three two-dimensional matrices obtained after row and column substitution into a three-dimensional matrix to obtain the ciphertext image.
The encryption algorithm flowchart is as shown in Figure 6.
With the help of the key, we encrypted the color baboon image, the encryption result and decryption result are shown in Figure 7.
The original, encrypted and decrypted images are shown in Figure 8. Evidently, the uniform distribution of the encrypted image indicates that it does not display any information about the original image.
Correlation analysis is a very important image evaluation index; the calculation method for the correlation coefficient was employed as described in [16].
The correlation between neighboring pixels in the original and encrypted images in different directions (horizontal, vertical, and diagonal) is shown in Figure 9. A strong correlation between neighboring pixels in the original image can be observed in Figure 9(a-c), whereas the correlation between neighboring pixels in the encrypted image, shown in Figure 9(d-e), was found to be weak. This is further evidenced by the data in Table 1. The weak correlation of encrypted images demonstrates the potential application of MHDBAMNNs for image encryption; thus, the validity of Theorem 3.1 is verified.
Original image | Encrypted image | |
Horizontal direction | 0.9237 | 0.0012 |
Vertical direction | 0.8792 | 0.0076 |
Diagonal direction | 0.8437 | -0.0034 |
Information entropy represents the degree of confusion of image pixel values, and the mathematical definition is as follows:
H = -{\sum }_{i = 0}^{{2}^{N}-1}p\left({x}_{i}\right)lo{g}_{2}p\left({x}_{i}\right) | (27) |
where p\left({x}_{i}\right) represents the proportion of the gray value {x}_{i} in an image, and {2}^{N} is the gray level of the image. The information entropy results for different channels of the original and encrypted images are shown in Table 2. It can be ascertained from the results that the information entropy of each channel is very close to the theoretical maximum of 8, and that the encryption algorithm can effectively resist the attack based on the image information entropy.
Original image | Encrypted image | |
R | 7.7063 | 7.9994 |
G | 7.4105 | 7.9993 |
B | 7.7285 | 7.9994 |
If a small change in the key causes the decrypted image to be completely unrelated to the original image, this characteristic is called strong key sensitivity. The key X\left(0\right) was changed from 0.5146 to 0.5146000000000001 during decryption, and the decrypted image under the wrong key was obtained as shown in Figure 10. It can be ascertained from the results that the key changed by {10}^{-16} , but the decrypted image cannot be recovered, demonstrating the strong key sensitivity of the algorithm.
One or several pixel values of the original image was changed and encrypted according to the encryption algorithm by the attacker; some relationships existing between the images were obtained by comparing the pixel values of the encrypted and the original encrypted images, which can be represented by the change rate of the number of pixels (NPCR) and uniform average intensity of change (UACI); the corresponding formulas are as follows:
NPCR = \frac{{\sum }_{i, j}D(i, j)}{m\times n}\times 100, | (28) |
UACI = \frac{1}{m\times n}\left[{\sum }_{i, j}\frac{\left|{C}_{1}\right(i, j)-{C}_{2}(i, j\left)\right|}{255}\right]\times 100, | (29) |
D\left(i, j\right) = \left\{\begin{array}{c}1, {C}_{1}\left(i, j\right)\ne {C}_{2}\left(i, j\right)\\ 0, {C}_{1}\left(i, j\right) = {C}_{2}\left(i, j\right)\end{array}\right., | (30) |
where m and n are the height and width of the original image, respectively, {C}_{1} and {C}_{2} are the two encrypted images mentioned above, and the ideal values of NPCR and UACI are 99.6094% and 33.4635%, respectively. The pixel value in the baboon image with the coordinate of (158, 164) was added by 1, and the specific numerical results were obtained according to Eqs (28)-(30) as shown in Table 3. The results show that the scheme can resist differential attacks.
Image | NPCR | UACI |
Baboon | 99.6102% | 33.4547% |
[16] Lena | 99.6063% | 33.4607% |
[16] 5.1.09.tiff | 99.6124% | 33.4790% |
[16] 5.1.10.tiff | 99.6109% | 33.4533% |
Some information may be lost due to noise during transmission. Salt and pepper noise with an intensity of 10% and a center clipping attack with an intensity of 20% were introduced into the encrypted image. The encrypted images after disturbance, as well as the corresponding decrypted images are shown in Figure 11, which shows that the decrypted images can be recognized, and that the proposed scheme is robust against noise.
The difference between the encrypted image F and the original image P of a good encryption algorithm should be huge. We applied the peak signal-to-noise ratio (PSNR) and the mean square error (MSE) as two image quality evaluation indexes, where the better effect of the encryption is a larger MSE value and a smaller PNSR value. The PSNR and MSE can be calculated as shown below:
MSE = \frac{1}{m\times n}{\sum }_{i = 1}^{m}{\sum }_{j = 1}^{n}{\left[P\right(i, j)-F(i, j\left)\right]}^{2}, | (31) |
PSNR = 10\times {log}_{10}\left(\frac{{255}^{2}}{MSE}\right), | (32) |
where m and n are the height and width of the original image, respectively, and the MSE and PSNR results for the original image, as compared to those of [16], are shown in Table 4. The results show that the proposed algorithm can effectively encrypt images.
Image | MSE | PSNR |
Baboon | 9741.2 | 8.2447 |
[16] Lena | 8861.4 | 8.6558 |
[16] 5.1.09.tiff | 6233.4 | 10.1836 |
[16] 5.1.10.tiff | 7696.4 | 9.2680 |
In this study, an MHDBAMNN was investigated for finite-time projection synchronization, along with its application to image encryption. To achieve secure image transmission, a time delay independent negative feedback controller was constructed by using a feedback control strategy and the criterion for finite-time projection synchronization of MHDBAMNN was derived. Numerical simulation examples demonstrated that the drive-response system realized finite-time projection synchronization, thus validating the finite-time synchronization theory proposed in this study. Given that the MHDBAMNN model has good chaotic properties; in the diffusion stage, the combination of Chen's hyperchaotic system and the DNA method was used to achieve a better diffusion effect. The experimental results show that the proposed algorithm can effectively encrypt images and has good robustness. The security of the proposed algorithm and the potential application of the model in secure image transmission were demonstrated by analyzing the encryption effect. All of these satisfactory characteristics make the proposed scheme a potential candidate for multimedia data encryption, and studying the dynamics of fractional order neural networks with hidden hyperchaos or hidden multi-scrolls is our future work.
The authors declare they have not used Artificial Intelligence (AI) tools in the creation of this article.
This research was funded by the NSFC, grant numbers 61462087 and 61751316, and the Special Education Project of Xinjiang, grant number 2022D03029.
The authors declare no conflict of interest.
[1] |
Z. Wang, X. Wang, Z. Zeng, Memristive circuit design of brain-like emotional learning and generation, IEEE Trans. Cybernet, 53 (2023), 222-235. https://doi.org/10.1109/TCYB.2021.3090811 doi: 10.1109/TCYB.2021.3090811
![]() |
[2] |
B. Gao, Y. Zhou, Q. Zhang, S. Zhang, P. Yang, Y. Xi, et al., Memristor-based analogue computing for brain-inspired sound localization with in situ training, Nat. Commun., 13 (2022), 2026. https://doi.org/10.1038/s41467-022-29712-8 doi: 10.1038/s41467-022-29712-8
![]() |
[3] |
A. A. Kausani, M. Anwar, Behavioral modeling of the pinched hysteresis loop of a Pt/TiO2/Pt memristor, Int. J. High Speed Electronics Syst., 32 (2023), 2350008. https://doi.org/10.1142/S0129156423500088 doi: 10.1142/S0129156423500088
![]() |
[4] |
F. Min, L. Xue, Routes toward chaos in a memristor-based Shinriki circuit, Chaos, 33 (2023), 023122. https://doi.org/10.1063/5.0126900 doi: 10.1063/5.0126900
![]() |
[5] |
R. B. Naik, U. Singh, A review on applications of chaotic maps in pseudo-random number generators and encryption, Ann. Data Sci., 11 (2022), 25-50. https://doi.org/10.1007/s40745-021-00364-7 doi: 10.1007/s40745-021-00364-7
![]() |
[6] |
I. Ahmad, M. Shafiq, B. Naderi, Finite-time synchronization of four-dimensional memristor-based chaotic oscillator and applied to secure communication systems, Franklin Open, 3 (2023), 100015. https://doi.org/10.1016/j.fraope.2023.100015 doi: 10.1016/j.fraope.2023.100015
![]() |
[7] |
B. Alhayani, A. A. Abdallah, Manufacturing intelligent Corvus corone module for a secured two way image transmission under WSN, Eng. Comput., 38 (2021), 1751-1788. https://doi.org/10.1108/EC-02-2020-0107 doi: 10.1108/EC-02-2020-0107
![]() |
[8] |
S. Sun, H. Shi, S. Duan, L. Wang, Memristor-based time-delay hyperchaotic system with circuit simulation and image encryption, Phys. Scr., 97 (2022), 035204. https://doi.org/10.1088/1402-4896/ac4cfb doi: 10.1088/1402-4896/ac4cfb
![]() |
[9] |
D. Ding, H. Xiao, Z. Yang, H. Luo, Y. Hu, X. Zhang, et al., Coexisting multi-stability of Hopfield neural network based on coupled fractional-order locally active memristor and its application in image encryption, Nonlinear Dyn., 108 (2022), 4433-4458. https://doi.org/10.1007/s11071-022-07371-0 doi: 10.1007/s11071-022-07371-0
![]() |
[10] |
L. Chen, H. Yin, T. Huang, L. Yuan, S. Zheng, L. Yin, Chaos in fractional-order discrete neural networks with application to image encryption, Neural Netw., 125 (2020), 174-184. https://doi.org/10.1016/j.neunet.2020.02.008 doi: 10.1016/j.neunet.2020.02.008
![]() |
[11] |
Q. Lai, Z. Wan, H. Zhang, G. Chen, Design and analysis of multiscroll memristive hopfield neural network with adjustable memductance and application to image encryption, IEEE Trans. Neural Netw. Learn Syst., 34 (2022), 7824-7837. https://doi.org/10.1109/TNNLS.2022.3146570 doi: 10.1109/TNNLS.2022.3146570
![]() |
[12] |
Q. Lai, Z. Chen, Dynamical analysis and finite-time synchronization of grid-scroll memristive chaotic system without equilibrium, Chaos Solitons Fractals, 176 (2023), 114118. https://doi.org/10.1016/j.chaos.2023.114118 doi: 10.1016/j.chaos.2023.114118
![]() |
[13] |
X. Yang, W. Chen, L. Wu, N. Qiu, Synchronous oscillation characteristic and finite-time function projection synchronization control method for microgrids, Electr. Power Syst. Res., 221 (2023), 109492. https://doi.org/10.1016/j.epsr.2023.109492 doi: 10.1016/j.epsr.2023.109492
![]() |
[14] |
R. Zhang, X. Xi, H. Tian, Z. Wan, Dynamical analysis and finite-time synchronization for a chaotic system with hidden attractor and surface equilibrium, Axioms, 11 (2022), 579. https://doi.org/10.3390/axioms11110579 doi: 10.3390/axioms11110579
![]() |
[15] |
S. Wang, H. Zhang, W. Zhang, H. Zhang, Finite-time projective synchronization of Caputo type fractional complex-valued delayed neural networks, Mathematics, 9 (2021), 1406. https://doi.org/10.3390/math9121406 doi: 10.3390/math9121406
![]() |
[16] |
Q. Lai, Z. Chen, Grid-scroll memristive chaotic system with application to image encryption, Chaos Solitons Fractals, 170 (2023), 113341. https://doi.org/10.1016/j.chaos.2023.113341 doi: 10.1016/j.chaos.2023.113341
![]() |
[17] |
C. Wang, D. Tang, H. Lin, F. Yu, Y. Sun, High-dimensional memristive neural network and its application in commercial data encryption communication, Expert Syst. Appl., 242 (2024), 122513. https://doi.org/10.1016/j.eswa.2023.122513 doi: 10.1016/j.eswa.2023.122513
![]() |
[18] |
X. Kong, F. Yu, W. Yao, S. Cai, J. Zhang, H. Lin, Memristor-induced hyperchaos, multiscroll and extreme multistability in fractional-order HNN: Image encryption and FPGA implementation, Neural Netw., 171 (2024), 85-103. https://doi.org/10.1016/j.neunet.2023.12.008 doi: 10.1016/j.neunet.2023.12.008
![]() |
[19] |
S. Kanwal, S. Inam, O. Cheikhrouhou, K. Mahnoor, A. Zaguia, H. Hamam, Analytic study of a novel color image encryption method based on the chaos system and color codes, Complexity, 2021 (2021), 5499538. https://doi.org/10.1155/2021/5499538 doi: 10.1155/2021/5499538
![]() |
[20] |
X. Gao, B. Sun, Y. Cao, S. Banerjee, B. Mou, A color image encryption algorithm based on hyperchaotic map and DNA mutation, Chinese Phys. B, 32 (2023), 030501. https://doi.org/10.1088/1674-1056/ac8cdf doi: 10.1088/1674-1056/ac8cdf
![]() |
[21] |
X. Xie, S. Wen, Y. Feng, B. O. Onasanya, Three-stage-impulse control of memristor-based chen hyper-chaotic system, Mathematics, 10 (2022), 4560. https://doi.org/10.3390/math10234560 doi: 10.3390/math10234560
![]() |
[22] |
H. Wen, Y. Lin, Cryptanalysis of an image encryption algorithm using quantum chaotic map and DNA coding, Expert Syst. Appl., 237 (2024), 121514. https://doi.org/10.1016/j.eswa.2023.121514 doi: 10.1016/j.eswa.2023.121514
![]() |
[23] |
Y. Zhu, C. Wang, J. Sun, F. Yu, A chaotic image encryption method based on the artificial fish swarms algorithm and the DNA coding, Mathematics, 11 (2023), 767. https://doi.org/10.3390/math11030767 doi: 10.3390/math11030767
![]() |
[24] |
C. Xu, X. Yang, J. Lu, J. Feng, F. E. Alsaadi, T. Hayat, Finite-time synchronization of networks via quantized intermittent pinning control, IEEE Trans. Cybern., 48 (2018), 3021-3027. https://doi.org/10.1109/TCYB.2017.2749248 doi: 10.1109/TCYB.2017.2749248
![]() |
[25] |
X. Yang, Can neural networks with arbitrary delays be finite-timely synchronized? Neurocomputing, 143 (2014), 275-281. https://doi.org/10.1016/j.neucom.2014.05.064 doi: 10.1016/j.neucom.2014.05.064
![]() |
[26] |
H. Wu, L. Wang, P. Niu, Y. Wang, Global projective synchronization in finite time of nonidentical fractional-order neural networks based on sliding mode control strategy, Neurocomputing, 235 (2017), 264-273. https://doi.org/10.1016/j.neucom.2017.01.022 doi: 10.1016/j.neucom.2017.01.022
![]() |
1. | N. Mohamed Thoiyab, Saravanan Shanmugam, Rajarathinam Vadivel, Nallappan Gunasekaran, Frobenius Norm-Based Global Stability Analysis of Delayed Bidirectional Associative Memory Neural Networks, 2025, 17, 2073-8994, 183, 10.3390/sym17020183 |
Original image | Encrypted image | |
Horizontal direction | 0.9237 | 0.0012 |
Vertical direction | 0.8792 | 0.0076 |
Diagonal direction | 0.8437 | -0.0034 |
Original image | Encrypted image | |
R | 7.7063 | 7.9994 |
G | 7.4105 | 7.9993 |
B | 7.7285 | 7.9994 |
Original image | Encrypted image | |
Horizontal direction | 0.9237 | 0.0012 |
Vertical direction | 0.8792 | 0.0076 |
Diagonal direction | 0.8437 | -0.0034 |
Original image | Encrypted image | |
R | 7.7063 | 7.9994 |
G | 7.4105 | 7.9993 |
B | 7.7285 | 7.9994 |
Image | NPCR | UACI |
Baboon | 99.6102% | 33.4547% |
[16] Lena | 99.6063% | 33.4607% |
[16] 5.1.09.tiff | 99.6124% | 33.4790% |
[16] 5.1.10.tiff | 99.6109% | 33.4533% |
Image | MSE | PSNR |
Baboon | 9741.2 | 8.2447 |
[16] Lena | 8861.4 | 8.6558 |
[16] 5.1.09.tiff | 6233.4 | 10.1836 |
[16] 5.1.10.tiff | 7696.4 | 9.2680 |