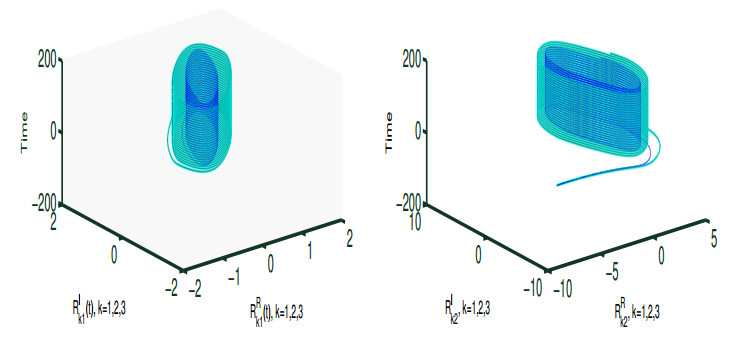
In cluster synchronization (CS), the constituents (i.e., multiple agents) are grouped into a number of clusters in accordance with a function of nodes pertaining to a network structure. By designing an appropriate algorithm, the cluster can be manipulated to attain synchronization with respect to a certain value or an isolated node. Moreover, the synchronization values among various clusters vary. The main aim of this study is to investigate the asymptotic and CS problem of coupled delayed complex-valued neural network (CCVNN) models along with leakage delay in finite-time (FT). In this paper, we describe several sufficient conditions for asymptotic synchronization by utilizing the Lyapunov theory for differential systems and the Filippov regularization framework for the realization of finite-time synchronization of CCVNNs with leakage delay. We also propose sufficient conditions for CS of the system under scrutiny. A synchronization algorithm is developed to indicate the usefulness of the theoretical results in case studies.
Citation: N. Jayanthi, R. Santhakumari, Grienggrai Rajchakit, Nattakan Boonsatit, Anuwat Jirawattanapanit. Cluster synchronization of coupled complex-valued neural networks with leakage and time-varying delays in finite-time[J]. AIMS Mathematics, 2023, 8(1): 2018-2043. doi: 10.3934/math.2023104
[1] | Zhifeng Lu, Fei Wang, Yujuan Tian, Yaping Li . Lag synchronization of complex-valued interval neural networks via distributed delayed impulsive control. AIMS Mathematics, 2023, 8(3): 5502-5521. doi: 10.3934/math.2023277 |
[2] | Călin-Adrian Popa . Synchronization of Clifford-valued neural networks with leakage, time-varying, and infinite distributed delays on time scales. AIMS Mathematics, 2024, 9(7): 18796-18823. doi: 10.3934/math.2024915 |
[3] | Chengqiang Wang, Xiangqing Zhao, Yang Wang . Finite-time stochastic synchronization of fuzzy bi-directional associative memory neural networks with Markovian switching and mixed time delays via intermittent quantized control. AIMS Mathematics, 2023, 8(2): 4098-4125. doi: 10.3934/math.2023204 |
[4] | Shuang Li, Xiao-mei Wang, Hong-ying Qin, Shou-ming Zhong . Synchronization criteria for neutral-type quaternion-valued neural networks with mixed delays. AIMS Mathematics, 2021, 6(8): 8044-8063. doi: 10.3934/math.2021467 |
[5] | Nayika Samorn, Kanit Mukdasai, Issaraporn Khonchaiyaphum . Analysis of finite-time stability in genetic regulatory networks with interval time-varying delays and leakage delay effects. AIMS Mathematics, 2024, 9(9): 25028-25048. doi: 10.3934/math.20241220 |
[6] | Hongguang Fan, Jihong Zhu, Hui Wen . Comparison principle and synchronization analysis of fractional-order complex networks with parameter uncertainties and multiple time delays. AIMS Mathematics, 2022, 7(7): 12981-12999. doi: 10.3934/math.2022719 |
[7] | Ailing Li, Xinlu Ye . Finite-time anti-synchronization for delayed inertial neural networks via the fractional and polynomial controllers of time variable. AIMS Mathematics, 2021, 6(8): 8173-8190. doi: 10.3934/math.2021473 |
[8] | Jiaqing Zhu, Guodong Zhang, Leimin Wang . Quasi-projective and finite-time synchronization of fractional-order memristive complex-valued delay neural networks via hybrid control. AIMS Mathematics, 2024, 9(3): 7627-7644. doi: 10.3934/math.2024370 |
[9] | Ankit Kumar, Subir Das, Vijay K. Yadav, Rajeev, Jinde Cao, Chuangxia Huang . Synchronizations of fuzzy cellular neural networks with proportional time-delay. AIMS Mathematics, 2021, 6(10): 10620-10641. doi: 10.3934/math.2021617 |
[10] | Yinjie Qian, Lian Duan, Hui Wei . New results on finite-/fixed-time synchronization of delayed memristive neural networks with diffusion effects. AIMS Mathematics, 2022, 7(9): 16962-16974. doi: 10.3934/math.2022931 |
In cluster synchronization (CS), the constituents (i.e., multiple agents) are grouped into a number of clusters in accordance with a function of nodes pertaining to a network structure. By designing an appropriate algorithm, the cluster can be manipulated to attain synchronization with respect to a certain value or an isolated node. Moreover, the synchronization values among various clusters vary. The main aim of this study is to investigate the asymptotic and CS problem of coupled delayed complex-valued neural network (CCVNN) models along with leakage delay in finite-time (FT). In this paper, we describe several sufficient conditions for asymptotic synchronization by utilizing the Lyapunov theory for differential systems and the Filippov regularization framework for the realization of finite-time synchronization of CCVNNs with leakage delay. We also propose sufficient conditions for CS of the system under scrutiny. A synchronization algorithm is developed to indicate the usefulness of the theoretical results in case studies.
In contrast to conventional neural networks (NNs) [1], coupled NNs (CNNs) are often susceptible to exhibit complex dynamical properties due to sub-network contact and cooperation [2,3,4,5]. CNNs are becoming important because of their potential in various application domains, e.g., electrical grid, medical science, image processing, and compression coding [6,7]. Although complex-valued signals are common in real-world applications, CNNs are unable to process them since real-valued signals are involved, e.g., in coupled real-valued neural networks (CRVNNs) [3,4,5,6].
In order to deal with complex-valued data [8,9,10,11], coupled complex-valued neural network (CCVNNs) are introduced. They can provide efficient and complex characteristics by incorporating complex variables as network elements [12,13,14,15,16,17,18]. The activation function selection poses the biggest challenge for a complex-valued neural network. There are two different activation functions in complex-valued neural networks (CVNNs): amplitude/phase activation and real/imaginary activation. The node activation function in a real case is often a smooth, bounded, nonconstant function. These constraints on the activation function are quite simple, so choosing a real function that satisfies them is not hard. In a complex valued neural network, any regular analytic function cannot be bounded unless it reduces to a constant. This is known as Liouville's theorem [19]. That is to say, activation functions in complex-valued neural networks cannot be both bounded and analytic. As a result, the primary difficulty for complex-valued neural networks is the activation functions. compared with CRVNNs, CCVNNs can solve various practical issues, e.g, a neuron with complex signal and orthogonal decision boundary can efficiently handle the XOR and symmetry detection problems [20,21]. In [22], CCVNNs can accurately represent optical wave fields of phase-conjugate resonators, since their phase and amplitude characteristics can be interpreted by complex-valued signals. Furthermore, CCVNNs have a number of advantages, including faster learning and reliability as well as powerful computing capabilities. Therefore, CCVNNs have attracted attention pertaining to their dynamic studies for real-world applications.
Many stability and synchronization studies have been reported, e.g., finite-time Mittag-Leffler stability [23], global stability [24,26], input-to-state stability [27] and lag synchronization, complete synchronization, anti-synchronization [28,29,30,31,32,33,34,35,36,37,38,39,40,41,42,43,44]. Among these, CNN synchronization has been popular due to its effectiveness in various applications, e.g, image encryption, image protection, and secure communication [45]. In cluster synchronization (CS), the elements in a network are grouped into various clusters. In this respect, the elements from the same cluster are completely synchronized, while those in different clusters are desynchronized. For example, two subgroups will be naturally formed in social networks when a crowd of people chooses to accept or reject an opinion according to their preference. Subtasks will divide the robot network into communities when a collection of robots is to complete a complex task, and consensus should be reached within each community. Many research studies on CS have been conducted since this phenomenon exists in many different systems, and it is applicable to different complex networks including cellular and metabolic networks, social networks, electrical power grid networks, biological NNs, and telephone cell graphs [2,3,46,47,48,49]. Liu et al. [50] considered the fractional-ordered linearly coupled system consisting of N NNs. Zhang et al. [51] explored CS of delayed CNNs with fixed and switching coupling topologies by employing Lyapunov theory and differential inequalities method. Yang et al. [52] discussed the CS issue of fractional-order networks subject to nonlinear coupling in finite time with complex variables based on the decomposition method. While CS of complex networks has received substantial attention, research on CS of complex-valued networks is new, despite its potential use. In [52], CS of a complex variable dynamical complex network was studied, but without considering time delays. Therefore, the problem of CS for complex variable networks in finite time requires further investigation.
The majority of studies focused mostly on asymptotic or exponential synchronization of networks through traditional control techniques. However, in reality, the networks might always be expected to achieve synchronization as quickly as possible, particularly in engineering fields. To achieve a faster convergence rate in time-delay complex networks, an effective method is to use finite-time (FT) synchronization control techniques. FT synchronization means the optimality in convergence time. Investigation of finite-time CS (FTCS) of CCVNNs is useful from a practical perspective. FTCS denotes the ability of the controlled systems to achieve synchronization in a predetermined amount of time with the assistance of some suitable controllers. Comparing with asymptotic synchronization, which occurs as the time approaches omit infinity, in certain instances synchronization in FT not only improves synchronization speed but also has the advantage of low disruption and perseverance in the presence of uncertainty. Therefore, it is worthwhile to investigate the CS of CCVNNs in FT. Yu et al. [53] analyzed FTCS pertaining to coupled dynamical systems without time delays. Concerning the prescribed-time stability for nonlinear PWS systems, two novel lemmas are established in [42], where the stable time can be arbitrarily specified and is independent of any control settings and initial values. The global fixed-time convergence principle of nonlinear systems with semi-Markovian switching is developed in [49], to ensure the cluster synchronization in finite/fixed time for a semi-Markovian switching complex dynamical network with discontinuous dynamic nodes. He et al. [54] studied adaptive CS in FT based on a neutral type of CNN models with mixed delays. FTCS for a coupled fuzzy cellular NNs was investigated in [55]. These studies are generally based on the premise that the parameters of complex networks are in the real domain. There are very few results on the CS of CCVNNs in FT. Furthermore, it is well understood that time delays are unavoidable in NN models, which can result in oscillations or asynchronization [56]. As a result, it is essential to examine time delays affect on CNNs. Furthermore, several studies have reported that constant time delays and time-varying delays in NNs can result in chaotic behaviors. In many real-world problems, leakage delays appear as negative feedback terms in a system, which can have a substantial impact on the dynamics of NNs [57,58,59,60,61]. Because of the impact on many real-world systems such as automatic control systems, it is important to investigate synchronization of NNs with time-varying delays and leakage delays [62,63,64]. We investigate asymptotic and CS of CCVNNs in FT with leakage and time-varying delays, and provide several useful criteria in this paper. Our research contributions include:
(i) Examining CCVNN models with leakage and time-varying delays, which constitute a class of coupling systems. Finding a suitable activation function subject to complex functions is the primary goal of many studies on CVNNs. We employ the real and imaginary types of activation functions in this paper. In addition, it is useful to divide them into their corresponding real and imaginary parts of models for the analysis of FT synchronization of the drive-response CCVNN model with leakage time delays.
(ii) Deriving the sufficient conditions with respect to asymptotical synchronization of the drive-response CCVNN model with time delays. This is achieved by constructing some non-negative functions, along with inequality techniques. Moreover, the CS criteria are presented.
(iii) Formulating the conditions in terms of nonsingular M-matrices for determining asymptotic and FTCS of the CCVNN model. Comparing with other conditions such as the Linear Matrix Inequalities (LMIs), the main benefit of the adequate condition includes non-singularity of the M-matrix [65,66,67]. Unlike recent studies on CS in FT with nonlinear coupling and no time delay of CVNN models[52], our method is applicable to CVNN models with both leakage and time variable delays. As such, our results are more general, as compared with those in the existing literature.
Graph theory. Consider a graph G=(V,E,G) with a set of nodes V= {1,…,V}(V>2), E⊆V×V, and coupling matrix J=[ȷij]V×V∈RV×V with ȷii=−∑Vj=1,j≠iȷij for i=1,…,V, where ȷij>0 if there is an interaction between nodes i and j, or else ȷij=0. Denote {C1,C2,…,CM} with Ck= {lk−1+1,lk−1+2,…,lk} as a set of partitions of nodes V with non-empty subset M such that (2≤M<V).
Consider a linear CCVNN model consisting of V CVNNs with both leakage and time-varying delays. Referring to the ith node, its dynamics can be represented by:
˙zi(t)=−Aizi(t−δ)+Cif(zi(t))+Dif(zi(t−τ(t)))+ˆIi+V∑j=iȷijzj(t)+ui(t), | (2.1) |
where i∈Ck with k∈ {1,…,M}, zi(t)=(zi1(t),…,zin(t))T∈Cn denotes the ith CCVNN's state vector (2.1) at time t; τ(t) is the time-varying delay; δ is the leakage term; f(zi(t))= (f1(zi1(t)),…,fn(zin(t)))T denotes the vector of neuron activations; Ai=diag(ai1,ai2,…,ain)> 0;Ci=[cilr]n×n∈Cn×n and Di=[dilr]n×n∈Cn×n are the interconnection weight matrices, l,r=1,2,…,n; ˆIi=(ˆIi1,…,ˆIin)T∈Cn denotes an external input source. We will design the control law ui(t) later. The initial states that correspond to (2.1) are:
zi(s)=φi(s),s∈[t0−τ,t0],i=1,…,V, | (2.2) |
where φi(s)=(φi1(s),…,φin(s))T∈Cn is continuous.
Remark 2.1. The terms f(zi(t−τ(t))) and f(zi(t)) are referred to as activation functions with and without time delay, respectively. There are significant time delays in every biological process. Time delay occurs in the propagation of action potentials along the axon and transmitting signals across the synapse for connected neurons. Discrete delays reflect the system's centralized effects of delays, whereas distributed delays have effects on neural networks at some duration or period relative to the discrete point of delays. Further research using discrete and distributed delays on this model will be carried out in the near future.
Assumption 2.1. Let any given k=1,2,…,M, Alk−1+1= Alk−1+2=⋯=Alk=ˉAk,Clk−1+1=Clk−1+2=⋯=Clk=ˉCk,Dlk−1+1=Dlk−1+2=⋯= Dlk=ˉDk, and ˆIlk−1+1=ˆIlk−1+2=⋯=ˆIlk=ˉIk.
The activation function in (2.1) is Lipschitz continuous throughout this study.
Assumption 2.2. For z(t)=aR(t)+ibI(t)∈C,aR(t),bI(t)∈R,fk(zk(t)) is divided into its real and imaginary parts: fk(zk(t))=fRk(aRk(t))+ifIk(bIk(t)),k=1,2,…,n. With the existence of some constants ˆκ−k,ˇκ−k,ˆκ+k,ˇκ+k,k=1,…,n such that for any u,v∈R and u≠v, fRk(⋅),fIk(⋅),gRk(⋅),gIk(⋅) satisfies the following:
ˆκ−k≤fRk(u)−fRk(v)u−v≤ˆκ+k,ˇκ−k≤fIk(u)−fIk(v)u−v≤ˇκ+k. |
Remark 2.2. The proposed generalized activation function class in this paper can describe the activation functions more flexibly and more specifically. The constants ˆκ−k,ˇκ−k and ˆκ+k,ˇκ+k in Assumption 2.2 may be positive, negative, or zero, as illustrated in [68]. Especially, when ˆκ−k=ˇκ−k=0 and ˆκ+k>0,ˇκ+k>0 Assumption 2.2 describes the class of globally Lipschitz continuous and monotone nondecreasing activation functions. And when ˆκ+k>ˆκ−k>0 and ˇκ+k>ˇκ−k>0 Assumption 2.2 describes the class of globally Lipschitz continuous and monotone increasing activation functions. Obviously, sigmoid and piecewise linear neuron activation functions are special cases of the functions meeting Assumption 2.2.
Assumption 2.3. Assume that the coupling matrix J satisfies the following:
J=(J11J12⋯J1MJ21J22⋯J2M⋮⋮⋱⋮JM1JM2⋯JMM), |
where Jkk∈R(lk−lk−1)×(lk−lk−1) is a zero row-sum matrix consisting of non negative off-diagonal values. Jkr∈ R(lk−lk−1)×(lr−lr−1)(k≠r), k,r=1,…,M is a zero row-sum matrix.
Definition 2.1. The CCVNN model in (2.1) is said to achieve CS in FT with partition {C1,C2,…,CM}, if t∗>t0 such that for any i∈Ck,k=1,2,…,M
{limt→t∗‖zi(t)−Rk(t)‖=0,‖zi(t)−Rk(t)‖=0,∀t≥t∗, |
and the following equation is satisfied by Rk(t)=ˆaRk(t)+iˆbIk(t).
˙Rk(t)=−ˉAkRk(t−δ)+ˉCkf(Rk(t))+ˉDkf(Rk(t−τ(t)))+ˆˉIk. | (2.3) |
Assumption 2.3 and (2.3) are combined. As such, for any i∈Ck,k=1,2,…,M
∑j∈Ckȷijrk(t)=0. | (2.4) |
Lemma 2.1. [69] Consider the following differential inequality with time delay:
{dx(t)dt≤−ax(t)+bx(t−τ(t)),x(r)=s(r),r∈[−τ,0] | (2.5) |
where τ>0,x(t)∈R is continuously differential and non-negative function. If a>b>0, then limt→+∞x(t)=0.
Remark 2.3. In [70], different dynamical behaviors for RVNN models with time-varying delays were examined using Lyapunov stability theory. Here, we study CVNN models, i.e the states, connection weights and external inputs belong to the complex domain. Therefore, the derived theoretical results are complementary to those in [70].
The new criteria for asymptotic and CS in FT for CCVNN models are established. Let ei(t)=zi(t)−Rk(t) for i∈Ck. As such, the control laws UR(t) and UI(t) can be devised as:
URi(t)=−ˉλkeRi(t)+ˉAkeRi(t−δ),UIi(t)=−ˉλkeIi(t)+ˉAkeIi(t−δ). | (3.1) |
It follows that the error dynamical models are characterized by (2.1),(2.4) and Assumption 2.1
{˙eR(t)=−ˉAkeR(tδ)+ˉCRkfR(eR,eI)−ˉCIkfI(eR,eI)+ˉDRkfR(eRτ,eIτ)−ˉDIkfI(eRτ,eIτ)+V∑j=1ȷijeRj(t)−ˉλkeRi(t)+ˉAkeRi(tδ),˙eI(t)=−ˉAkeR(tδ)+ˉCRkfI(eR,eI)+ˉCIkfR(eR,eI)+ˉDRkfI(eRτ,eIτ)+ˉDIkfR(eRτ,eIτ)+V∑j=1ȷijeIj(t)−ˉλkeIi(t)+ˉAkeIi(tδ), | (3.2) |
where i∈Ck, fR(eR,eI),fI(eR,eI),fR(eRτ,eIτ),fI(eRτ,eIτ), eRi(tδ),eIi(tδ) are real and imaginary parts of f(ei(t))=f(zi(t)−f(Rk(t))), f(ei(t))=f(zi(t−τ(t))−f(Rk(t−τ(t)))), ei(t−δ(t)=zi(t−δ)−Rk(t−δ), respectively.
Denote ˉΛ= diag(ˉλ1,…,ˉλM),ˉA=diag(a1min,…,aMmin) with akmin= min1≤j≤n{ail∣l=1,…,n,i∈Ck} for k=1,2,…,M,
ckmin=min1≤j≤n{cil∣l=1,…,n,i∈Ck} for k=1,…,M,L=diag(L1,…,Ln),ˉA=diag(‖ˉA1L‖1,…,‖ˉAML‖1),ˉCRR=diag{||ˉCR1μRR||1+||ˉCR1μRI||1,…,||ˉCRnμRR||+||ˉCRnμRI||1},ˉCII=diag{||ˉCI1μIR||1+||ˉCI1μII||1,…,||ˉCInμIR||+||ˉCInμII||1},ˉCRI=diag{||ˉCR1μIR||1+||ˉCR1μII||1,…,||ˉCRnμIR||+||ˉCRnμII||1},ˉCIR=diag{||ˉCI1μRR||1+||ˉCI1μRI||1,…,||ˉCInμRR||+||ˉCInμRI||1},ˉDRR=diag{||ˉDR1θRR||1+||ˉDR1θRI||1,…,||ˉDRnθRR||+||ˉDRnθRI||1},ˉDII=diag{||ˉDI1θIR||1+||ˉDI1θII||1,…,||ˉDInθIR||+||ˉDInθII||1},ˉDRI=diag{||ˉDR1θIR||1+||ˉDR1θII||1,…,||ˉDRnθIR||+||ˉDRnθII||1},ˉDIR=diag{||ˉDI1θRR||1+||ˉDI1θRI||1,…,||ˉDInθRR||+||ˉDInθRI||1}.ˉD=diag(dmax,…,dmax)∈RM×M, withdmax=max1≤k≤M{‖ˉDkL‖1},and ˉJ=[ˉJkp]M×M∈RM×M with |
ˉJkp={maxi∈Ck{∑j∈Ckȷji},if p=k,‖Jkp‖1,if p≠k. |
Theorem 3.1. If Assumptions 2.1–2.3 hold, the CCVNN model in (2.1) with t0=0 can achieve CS asymptotically under controller (3.1) if ˜Λ+˜A−˜C−˜D−˜J is a non-singular M-matrix.
Proof: Since ˜Λ+˜A−˜C−˜D−˜J is a non-singular M-matrix, there exists a positive vector J=(J1,…JM)T∈CM such that (˜Λ+˜A−˜C−˜D−˜J)J≥0, where,
˜Λ=(ˉΛ00ˉΛ),˜A=(ˉA00ˉA),˜C=(ˉCRR−ˉCIIˉCIRˉCRI),˜D=(ˉDRR−ˉDIIˉDIRˉDRI),˜J=(ˉJ00ˉJ). | (3.3) |
As most of the majority of neural network stability criteria are derived from the Lyapunov theory, they are all conservative. Reducing the conservatism has been the topic of much research. The reduction can be achieved with the Lyapunov stability theory primarily through two phases: a) choosing the suitable Lyapunov functional and b) estimating its derivative. Therefore, we constructed the following non-negative function as follows:
V(t)=n∑k=1∑i∈AkYk||eR(t)||+n∑k=1∑i∈AkYk||eI(t)||. | (3.4) |
The derivative of V(t) along the solution of model (3.2) gives
˙V(t)≤M∑k=1∑i∈CkYksgn(eRi(t))T˙eRi(t)+M∑k=1∑i∈CkYksgn(eIi(t))T˙eRi(t),=M∑k=1∑i∈CkYksgn(eRi(t))T[−ˉAkeR(tδ)+ˉCRkfR(eR,eI)−ˉCIkfI(eR,eI)+ˉDRkfR(eRτ,eIτ)−ˉDIkfI(eRτ,eIτ)+N∑j=1ȷijeRj(t)−ˉλkeRi(t)+ˉAkeRi(t−δ(t))]+M∑k=1∑i∈CkYksgn(eIi(t))T[−ˉAkeI(tδ)+ˉCRkfI(eR,eI)+ˉCIkfR(eR,eI)+ˉDRkfI(eRτ,eIτ)+ˉDIkfR(eRτ,eIτ)+N∑j=1ȷijeIj(t)−ˉλkeIi(t)+ˉAkeIi(t−δ(t))]. |
As such, from Assumption 2.2
M∑k=1∑i∈CkYksgn(eRi(t))TˉCRkfR(eR,eI)≤M∑k=1∑i∈Ckn∑s=1n∑r=1Yk|ckRrs|μRR|eRis(t)|+M∑k=1∑i∈Ckn∑s=1n∑r=1Yk|ckRrs|μRI|eIis(t)|≤M∑k=1∑i∈Akmax1≤s≤n{n∑r=1|ckRrs|μRR}Yk|eRis(t)|+M∑k=1∑i∈Ckmax1≤s≤n{n∑r=1|ckRrs|μRI}Yk|eIis(t)|=M∑k=1||ˉCRkμRR||1∑i∈CkYk||eRi(t)||+M∑k=1||ˉCRkμRI||1∑i∈CkYk||eIi(t)||. | (3.5) |
Similarly
M∑k=1∑i∈CkYksgn(eRi(t))TˉCIkfI(eR,eI)=M∑k=1||ˉCIkμIR||1∑i∈CkYk||eRi(t)||+M∑k=1||ˉCIkμII||1∑i∈CkYk||eIi(t)||,M∑k=1∑i∈CkYksgn(eIi(t))TˉCRkfI(eR,eI)=M∑k=1||ˉCRkμIR||1∑i∈CkYk||eRi(t)||+M∑k=1||ˉCRkμII||1∑i∈CkYk||eIi(t)||,M∑k=1∑i∈CkYksgn(eIi(t))TˉCIkfR(eR,eI)=M∑k=1||ˉCIkμRR||1∑i∈CkYk||eRi(t)||+M∑k=1||ˉCIkμRI||1∑i∈CkYk||eIi(t)||, |
and
M∑k=1∑i∈CYksgn(eRi(t))TˉDRkfR(eRτ,eIτ)=M∑k=1||ˉdRkθRR||1∑i∈CkYk||eRi(tτ)||δReRi(t)+M∑k=1||ˉdRkθRI||1∑i∈CkYk||eIi(tτ)||δIeIi(t),M∑k=1∑i∈CkYksgn(eRi(t))TˉDIkfI(eRτ,eIτ)=M∑k=1||ˉdIkθIR||1∑i∈CkYk||eRi(tτ)||δReRi(t)+M∑k=1||ˉdIkθII||1∑i∈CkYk||eIi(tτ)||δIeIi(t),M∑k=1∑i∈CkYksgn(eIi(t))TˉDRkfI(eRτ,eIτ)=M∑k=1||ˉDRkθIR||1∑i∈CkYk||eRi(tτ)||δReRi(t)+M∑k=1||ˉDRkθII||1∑i∈CkYk||eIi(tτ)||δIeIi(t),M∑k=1∑i∈CkYksgn(eIi(t))TˉDIkfR(eRτ,eIτ)=M∑k=1||ˉDIkθRR||1∑i∈CkYk||eRi(tτ)||δReRi(t)+M∑k=1||ˉDIkθRI||1∑i∈CkYk||eIi(tτ)||δIeIi(t). | (3.6) |
On the other hand, since
M∑k=1∑i∈CkYksgn(eRi(t))TN∑j=1ȷijeRj(t)=M∑k=1∑i∈Ckȷii‖eRi(t)‖1+M∑k=1∑i∈Ck∑j∈Ck,j≠iYksgn(eRi(t))TȷijeRj(t)+M∑k=1M∑p=1,p≠k∑i∈Ck∑j∈CpYksgn(eRi(t))TȷijeRj(t),≤M∑k=1∑i∈Ckȷii‖eRi(t)‖1+M∑k=1∑i∈Ck∑j∈Ck,j≠iYk|ȷij|||eRj(t)‖1+M∑k=1M∑p=1,p≠k∑i∈Ck∑j∈CpYk|ȷij|‖eRj(t)‖1, | (3.7) |
we obtain the following results based on the characteristics of matrix J,
M∑k=1∑i∈Ckȷii‖eRi(t)‖1+M∑k=1∑i∈Ck∑j∈Ck,j≠iYk∣ȷij‖eRj(t)‖1=M∑k=1∑i∈Ckȷii‖eRi(t)‖1+M∑k=1∑j∈Ck∑i∈Ck,i≠jYk|ȷji|||eRi(t)‖1=M∑k=1∑i∈Ckȷii‖eRi(t)‖1+M∑k=1∑i∈Ck∑j∈Ck,j≠iYk|ȷji|‖eRi(t)‖1=M∑k=1∑i∈Ck∑j∈CkYk|ȷji|||eRi(t)‖1≤M∑k=1maxi∈Ck{∑j∈Ck|ȷji|}∑i∈CkYk‖eRi(t)‖1, | (3.8) |
and
M∑k=1M∑p=1,p≠k∑i∈Ck∑j∈CpYk|ȷij|‖eRj(t)‖1=M∑p=1M∑k=1,k≠p∑j∈Cp∑i∈CkYp∣ȷji‖|eRi(t)‖1=M∑k=1M∑p=1,p≠k∑j∈Cp∑i∈CkYp|ȷji|‖eRi(t)‖1≤M∑k=1(M∑p=1,p≠kYpYkmaxi∈Ck{∑j∈Cp|ȷji|})∑i∈CkYk‖eRi(t)‖1=M∑k=1(M∑p=1,p≠kYpYk‖Jkp‖1)∑i∈CkYk‖eRi(t)‖1. | (3.9) |
As a result of (3.7), it follows that
M∑k=1∑i∈CkYksgn(eRi(t))TN∑j=1ȷijeRj(t)≤M∑k=1(maxi∈Ck{∑j∈Ckȷji}+M∑p=1,p≠kYpYk‖Jkp‖1)∑i∈CkYk‖eRi(t)‖1. | (3.10) |
Similarly, we can have
M∑k=1∑i∈CkYksgn(eIi(t))TN∑j=1ȷijeIj(t)≤M∑k=1(maxi∈Ck{∑j∈Ckȷji}+M∑p=1,p≠kYpYk‖Jkp‖1)∑i∈CkYk‖eIi(t)‖1. | (3.11) |
From (3.7)–(3.11) we have,
˙V(t)≤M∑k=11Yk[Yk(||ˉCRkμRR||1+||ˉCIkμIR||1+||ˉCRkμIR||1+||ˉCIkμRR||1+maxi∈Ck{∑i∈Ckȷij}−ˉdk)+∑p=1,p≠kYpYk||Jkp||1]∑i∈CkYk||eRi(t)||+M∑k=11Yk[(Yk(|ˉCRkμRI||1+||ˉCIkμII||1+||ˉCRkμII||1+‖|ˉCIkμRI||1)+maxi∈Ck{∑j∈Ckȷij}−ˉdk)+∑p=1,p≠kYpYk||Jkp||1]∑i∈CkYk||eIi(t)||+M∑k=11Yk[Yk(||ˉDRkθRR||1+||ˉDIkθIR||1+||ˉDRkθIR||1+||ˉDIkθRR||1)]∑i∈CkYk||eRi(tτ)||+M∑k=11Yk[Yk(||ˉDRkθRI||1+||ˉDIkθII||1+||ˉDRkθII||1+||ˉDIkθRI||1)]∑i∈CkYk||eIi(τ)||≤min{A,B}V(t)+max{AτB}τV(t−τ(t))≤−aminV(t)+bmaxV(t−τ(t)). | (3.12) |
where
A=M∑k=11Yk[Yk(||ˉCRkμRR||1+||ˉCIkμIR||1+||ˉCRkμIR||1+||ˉCIkμRR||1+maxi∈Ck{∑i∈Ckȷij}−ˉdk)+∑p=1,p≠kYpYk||Jkp||1]∑i∈CkYk,B=M∑k=11Yk[(Yk(|ˉCRkμRI||1+||ˉCIkμII||1+||ˉCRkμII||1+‖|ˉCIkμRI||1)+maxi∈Ck{∑j∈Ckȷij}−ˉdk)+∑p=1,p≠kYpYk||Jkp||1]∑i∈CkYk,Aτ=M∑k=11Yk[Yk(||ˉDRkθRR||1+||ˉDIkθIR||1+||ˉDRkθIR||1+||ˉDIkθRR||1)]∑i∈CkYk,Bτ=M∑k=11Yk[Yk(||ˉDRkθRI||1+||ˉDIkθII||1+||ˉDRkθII||1+||ˉDIkθRI||1)]∑i∈CkYk,amin=min{A,B},bmax=max{Aτ,Bτ}. |
As a result of (˜Λ+˜A−˜C−˜D−˜J)J≥0, amin>bmax>0. Then according to Lemma 2.1, one can obtain that limt→+∞V(t)=0, which implies that the coupled complex-valued neural networks (2.1) can achieve cluster synchronization asymptotically. This completes the proof of Theorem 8.
Remark 3.1. CS is a more feasible collective behavior than complete synchronization, is thought to be important in biological research and communication engineering [71]. Generally, if all of the neurons in neural networks are separated into many clusters, nodes within the same cluster can achieve complete synchronization, but there is no uniform behavior between various clusters. In this paper, we investigate cluster synchronization of the model (2.1) using the synchronization manifold method described in [72]. It should be noted that we do not assume the coupling matrix is symmetric or diagonal. However, the majority of previous works on network synchronization are predicated on this assumption [73,74]. Moreover, only the simple Lyapunov function is used in this paper. As a result, the conditions of Theorems are strict. To further reduce the conservativeness and improve the conditions for this cluster synchronization problem, a delay partitioning approach or the free-weight matrix method [75,76] could be used. However, these methods will significantly increase the complexity of the proof procedure. Therefore, it is omitted here. In the near future, this model will be subjected to additional research using the delay partitioning approach or the free-weight matrix method.
In the following, the solution of model (2.1) is designed in the sense of Filippov. As such, the control inputs UR(t),UI(t) to be constructed is discontinuous and the differential inclusion can be written as:
˙zi(t)∈−Aizi(t−δ)+Cif(zi(t))+Dif(zi(t−τ(t)))+Ii+n∑j=igijzj(t)+KUi(t), | (3.13) |
where K[Ui(t)] represents the closure of the convex hull of Ui(t). There exists a measurable function Si(t)∈K[Ui(t)] when the measurable selection theorem is applied such that
˙zi(t)=−Aizi(t−δ)+Cif(zi(t))+Dif(zi(t−τ(t)))+Ii+n∑j=igijzj(t)+Si(t). | (3.14) |
From [77,78], it is straightforward to establish that if Assumption 2.2 holds, then there exists a solution z(t) for the IVP combined with (2.1) on [t0,∞) with controller inputs URi(t),UIi(t) for given initial condition (2.2). Consider the controller inputs in the following form:
URi(t)=−ˉmkeRi(t)+AkeRi(t−δ)−(αk+βRk||eRi(t−τ)||1)sgn(eRi(t)),UIi(t)=−ˉmkeIi(t)+AkeIi(t−δ)−(αk+βIk||eIi(t−τ)||1)sgn(eIi(t)). | (3.15) |
From Assumption 2.1 as well as (2.1) and (2.4), the synchronization errors become
{˙eR(t)=−ˉAkeR(tδ)+ˉCRkfR(eR,eI)−ˉCIkfI(eR,eI)+ˉDRkfR(eRτ,eIτ)−ˉDIkfI(eRτ,eIτ)+n∑j=1ȷijeRj(t)−ˉmkeRi(t)+AkeRi(t−δ)−(αk+βRk||eRi(t−τ)||1)sgn(eRi(t)),˙eI(t)=−ˉAkeR(tδ)+ˉCRkfI(eR,eI)+ˉCIkfR(eR,eI)+ˉDRkfI(eRτ,eIτ)+ˉDIkfR(eRτ,eIτ)+n∑j=1ȷijeRj(t)−ˉmkeIi(t)+AkeIi(t−δ)−(αk+βIk||eIi(t−τ)||1)sgn(eIi(t)), | (3.16) |
and the set valued map Ui(t) is denoted by
K[URi(t)]=−ˉmkeRi(t)+AkeRi(t−δ)−(αk+βRk||eRi(t−τ)||1))Ksgn(eRi(t)),K[URi(t)]=−ˉmkeIi(t)+AkeIi(t−δ)−(αk+βIk||eIi(t−τ)||1))Ksgn(eIi(t)). | (3.17) |
Then there exists Oi(t)∈K[sgn(ei(t))] such that t≥t0
{˙eR(t)=−ˉAkeR(tδ)+ˉCRkfR(eR,eI)−ˉCIkfI(eR,eI)+ˉDRkfR(eRτ,eIτ)−ˉDIkfI(eRτ,eIτ)+n∑j=1ȷijeRj(t)−ˉmkeRi(t)+AkeRi(t−δ)−(αk+ηR1k||eRi(tτ)||1+ηI1k||eIi(tτ)||1)ORi(t),˙eI(t)=−ˉAkeR(tδ)+ˉCRkfI(eR,eI)+ˉCIkfR(eR,eI)+ˉDRkfI(eRτ,eIτ)+ˉDIkfR(eRτ,eIτ)+n∑j=1ȷijeRj(t)−ˉmkeIi(t)+AkeIi(t−δ)−(αk+ηR2k||eRi(tτ)||1+ηI2k||eIi(tτ)||1)OIi(t). | (3.18) |
Definition 3.1. [79] The response model of (2.3) is said to be synchronized with the drive model of (2.1) in FT under a suitable designed controller (3.15), if a constant T∗>0 exists such that ‖e(T∗)‖1=0 and ‖e(t)‖1≡0 for t>T∗, where ‖e(t)‖1=∑ni=1|ei(t)|,e(t)=(eR(t),eI(t))T. Note that T is denoted as the settling time of synchronization if T=inf{T∗≥0 for t≥T∗}.
Based on the above account, a theorem is put forward, as follows.
Theorem 3.2. Based on Assumptions 2.1 and 2.2, if ηR1k≥||ˉdRkθRR||1−||ˉdIkθIR||1,ηI1k≥||ˉdRkθRI||1−||ˉdIkθII||1,ηR2k≥||ˉdRkθIR||1+||ˉdIkθRR||1,ηI2k≥||ˉdRkθII||1+||ˉdIkθRI||1,ˉY≥0, for k=1,…,M and ˜Λ+˜A−˜C−˜J is a non singular M−matrix, i.e, there exists a positive vector Y=(Y1,…,Y2M)T∈R2M such that (˜Λ+˜A−˜C−˜J)Y>0∈R2M, then the coupled CVNN model of (3.13) can realize FTCS under controller (3.15).
Proof: Leveraging on the non-singularity of J matrix
˙V(t)≤M∑k=1∑i∈CkYksgn(eRi(t))T˙eRi(t)+M∑k=1∑i∈CkYksgn(eIi(t))T˙eRi(t) | (3.19) |
=M∑k=1∑i∈CkYksgn(eRi(t))T[−ˉAkeR(tδ)+ˉCRkfR(eR,eI)−ˉCIkfI(eR,eI)+ˉDRkfR(eRτ,eIτ)−ˉDIkfI(eRτ,eIτ)+N∑j=1ȷijeRj(t)−ˉλkeRi(t)+ˉAkeRi(t−δ(t))−(αk+ηR1k||eRi(tτ)||1+ηI1k||eIi(tτ)||1)ORi(t)]+M∑k=1∑i∈CkYksgn(eIi(t))T[−ˉAkeI(tδ)+ˉCRkfI(eR,eI)+ˉCIkfR(eR,eI)+ˉDRkfI(eRτ,eIτ)+ˉDIkfR(eRτ,eIτ)+N∑j=1ȷijeIj(t)−ˉλkeIi(t)+ˉAkeIi(t−δ(t))−(αk+ηR2k||eRi(tτ)||1+ηI2k||eIi(tτ)||1)OIi(t)]. | (3.20) |
We can obtain
−M∑k=1∑i∈CkYksgn(eRi(t))T(αk+ηR1k||eRi(tτ)||1+ηI1k||eIi(tτ)||1)ORi(t)=−M∑k=1∑i∈CkYk(αk+ηR1k||eRi(tτ)||1+ηI1k||eIi(tτ)||1)δR(eRi(t)), | (3.21) |
−M∑k=1∑i∈CkYksgn(eIi(t))T(αk+ηR2k||eRi(tτ)||1+ηI2k||eIi(tτ)||1)OIi(t)=−M∑k=1∑i∈CkYk(αk+ηR2k||eRi(tτ)||1+ηI2k||eIi(tτ)||1)δI(eIi(t)). | (3.22) |
According to ηR1k≥||ˉdRkθRR||1−||ˉdIkθIR||1,ηI1k≥||ˉdRkθRI||1−||ˉdIkθII||1,ηR2k≥||ˉdRkθIR||1+||ˉdIkθRR||1,ηI2k≥||ˉdRkθII||1+||ˉdIkθRI||1 for k=1,…,M, (3.6), (3.21) and (3.22), one can obtain
M∑k=1∑i∈CkYksgn(eRi(t))T[ˉDRkfR(eRτ,eIτ)−ˉDIkfI(eRτ,eIτ)]−M∑k=1∑i∈CkYk(ηR1k||eRi(tτ)||1+ηI1k||eIi(tτ)||1)δR(eRi(t))≤0, | (3.23) |
M∑k=1∑i∈CkYksgn(eIi(t))T[ˉDRkfI(eRτ,eIτ)+ˉDIkfR(eRτ,eIτ)]−M∑k=1∑i∈CkYk(ηR2k||eRi(tτ)||1+ηI2k||eIi(tτ)||1)δI(eIi(t))≤0. | (3.24) |
The above equations yield
˙V(t)≤M∑k=11Yk[Yk(||ˉCRkμRR||1+||ˉCIkμIR||1+||ˉCRkμIR||1+||ˉCIkμRR||1+maxi∈Ck{∑i∈Ckȷij}−ˉdk)+∑p=1,p≠kYpYk||Jkp||1]∑i∈CkYk||eRi(t)||+M∑k=11Yk[(Yk(|ˉCRkμRI||1+||ˉCIkμII||1+||ˉCRkμII||1+‖|ˉCIkμRI||1)+maxi∈Ck{∑j∈Ckȷij}−ˉdk)+∑p=1,p≠kYpYk||Jkp||1]∑i∈CkYk||eIi(t)||−M∑k=1∑i∈CkYk(αkδR(eRi(t))+αkδI(eIi(t))),≤ˉYV(t)−M∑k=1∑i∈Ck2Ykˉαki,≤−ˉYV(t)−min1≤k≤M2Ykαk, | (3.25) |
where ˉαki=αk if eRi(t)≠0, eIi(t)≠0 otherwise ˉαki=0.
˙V(t)≤−min1≤k≤M2Ykαk,t∈(0,+∞]. | (3.26) |
Integrating both sides of the above inequality from 0 to t gives
V(t)−V(0)≤−min1≤k≤M2Ykαkt,t≥0. | (3.27) |
Note that as ||e(t)||1≥0, it indicates that
min1≤k≤M2Ykαk≥0. |
It then follows from (3.25) that limt→+∞V(t)=−∞, which contradicts V(t)>0, for t∈(0,+∞). Hence there exists T∗∈(0,+∞) such that V(T∗)=0, which implies that
‖e(T∗)‖1=0. | (3.28) |
Next, we show that ‖e(t)‖1≡0 for ∀t≥T∗. Otherwise, suppose that there exists T1>T∗ such that ‖e(T1)‖1>0. Let
Ts=sup{t∈[T∗,T1]:‖e(t)‖1=0}. |
It then follows from the fact ‖e(T∗)‖1=0 that Ts is nonempty. Notice that Ts<T1,‖e(Ts)‖1=0 and ‖e(t)‖1>0 for all t∈(Ts,T1]. As such, there exists T2∈[Ts,T1] such that ˙V(t)|t=T2>0, otherwise, ∀t∈[Ts,T1],˙V(t)≤0. Then V(t) is monotone increasing, we obtain 0=V(Ts)≥V(T1)>0, which is a contradiction. Therefore, there exists T2∈(Ts,T1] such that ˙V(t)|t=T2>0. On the other hand, note that ‖e(T2)‖1>0 implies that
min1≤k≤M2Ykαk≥0, |
It follows from inequality (3.25) that ˙V(T2)<0, which is also a contradiction. As such, ‖e(t)‖1≡0,∀t≥T∗. Consequently, based on Definition 3.1, response model (2.1) is synchronized with drive model (2.3) in FT under controller (3.15). The proof of is completed.
Remark 3.2. Different from [70] in this study, 1-norm of vectors is used to construct the real and imaginary types of Lyapunov functions. The necessary sufficient criterion is derived in the form of a non-singular M-matrix using the constructed Lyapunov function (3.4), which can be easily verified. In addition, other Lyapunov functions that prove CS of different NN models are available, e.g., Euclidean norm or positive-definite quadratic form. Nonetheless, the resulting adequate conditions are in the form of LMIs or eigenvalues, which are more difficult to be verified.
Remark 3.3. Referring to Theorems 8 and 10, when aRi(t)=bIi(t),ˆaRk(t)=ˆbIk(t),
n∑k=1∑i∈AkYk||eR(t)||=n∑k=1∑i∈AkYk||eI(t)||, |
we can establish new adequate conditions for asymptotic CS and FTCS for NN models described by differential equations. Therefore, the results can be used for analyzing FTCS of NN models with a differential equation as well as with two differential equations.
Remark 3.4. In [52], the dynamical properties of ordinary difference equations in the real domain were studied. However, very few results on coupled network models in the complex domain are available. Different from the existing literature, we investigate CCVNN models and analyze their dynamical behaviours in this study.
A simulation example to demonstrate the usefulness of the theoretical results is presented.
Example 4.1. Consider a two-neuron coupled CVNN model of (2.1) with leakage and time varying delays, where f(⋅)= (f1(⋅),f2(⋅))T=(tanh(⋅),tanh(⋅))T,τ(t)=2,M=3 and
ˉC1=(1.95+i20.1+i0.3−5−i4.53+i2.5),ˉC2=(2+i20.3+i0.3−5−i4.52.8+i3),ˉC3=(2+i20.3+i0.3−5−i4.52.8+i3),ˉD1=(2+i20.3+i0.3−5−i4.52.8+i3),ˉD2=(2+i20.3+i0.3−5−i4.52.8+i3),ˉD3=(2+i20.3+i0.3−5−i4.52.8+i3),ˉA1=ˉA2=ˉA3=(1001),ˆI1=.ˆI2=ˆI3=(00). |
The initial values for k=1,2,3 are Rk(t)=(0.8,−8)T for t∈[−1,0]. The phase portraits are shown in Figure 1, demonstrating their chaotic behaviors.
The coupling matrix G for CCVNN model (2.1) is
G=(−6330.5−0.5−0.40.43−300.2−0.20.2−0.222−40.3−0.30.5−0.50.50.5−1−11−0.10.10.8−0.801−1−0.20.20.20.2−0.40.1−0.1−110.10.1−0.2−0.20.22−2). |
Then, model (2.1) can be separated into three clusters C1= {1,2,3},C2={4,5}, and C3={6,7}. With the above-mentioned settings, we can obtain A= diag(1,1,1),μRR=μRI=μIR=μII=θRR=θRI=θIR=θII=diag(1,1,1),ˉCRR=ˉCRI=ˉCIR=ˉCII=diag(14,14.2,14),ˉDRR=ˉDRI=ˉDIR=ˉDII=diag(5.2,5.1,5), and
ˉJ=(211.11.300.30.60.33). |
Case 1 : Let ˉΛ=diag(20,19,20), one can achieve
˜Λ+˜A−˜C−˜D−˜J=(−0.2−1−19−19.200−1.30.7−0.30−19.30−0.6−0.3−100−19−19.200−0.2−1−1.10−19.30−1.30.7−0.300−19−0.6−0.3−1), |
which is a non-singular M-matrix. Based on Theorem 8, CCVNN model (2.1) can realize CS asymptotically under (3.1) with respect to C1,C2,C3. To analyze the CS process, the error in each cluster is
ek(t)=∑i,j∈Ck‖zi(t)−Rk(t)‖1, k=1,2,3. |
For k=1,2,3 in Figures 2–4, we have ek(t)→0 when t→+∞, which implies that all states achieve CS asymptotically.
Case 2: Let ˉΛ=diag(19,18,18),β1=3,β2=2.73,β3= 2.5, αR1=αR2=αR3=1,αI1=αI2=αI3=1. As such, the control law (3.15) follows that
˜Λ+˜A−˜C−˜J==(4−1−1.1−1400−1.34.8−0.30−14.20−0.6−0.3200−14−14004−1−1.10−14.20−1.34.8−0.300−14−0.6−0.32) |
implying that ˜Λ+˜A−˜C−˜J= is a strictly diagonally dominant matrix.
Case 3: In this case, we take three neurons instead of two neurons in above two cases, the three dimensional interconnection weight matrices are taken as follows:
ˉC1=(1.9+i0.0121+i0.040.1+i0.30.2+i0.11.9+i0.010.2+i0.10.2+i00+i0.010.1+i0.1),ˉC2=(1.9+i0.0121+i0.040.1+i0.30.2+i0.11.9+i0.010.2+i0.10.2+i00+i0.010.1+i0.1),ˉD1=(−1.4−i0.120.21+i00.1+0.21i0.11+i0.01−1.4−0i0.1+i0.030.22+i0.007−0.1−i0.090.1+i0.01),ˉD2=(−1.4−i0.120.21+i00.1+0.21i0.11+i0.01−1.4−0i0.1+i0.030.22+i0.007−0.1−i0.090.1+i0.01),ˉA1=ˉA2=(100010001),ˆI1=ˆI2=(000). |
Then activation functions f(zi(t)) and f(zi(t−τ(t))) in (2.1) can be selected as f(zi(t))=tanh(zi(t)),f(zi(t−τ(t)))=tanh(zi(t−τ(t))) and the transmission time-varying delay is τ(t)=etet+1.
For the existence of the constants ˆκ−k=0.5,ˇκ−k=0.5,ˆκ+k=1,ˇκ+k=1 Assumption 3.2 is satisfies for the activation functions f(zi(t))=tanh(zi(t)),f(zi(t−τ(t)))=tanh(zi(t−τ(t))).
The leakage term is selected as δ=1.2. The initial conditions associated with model (2.1) is chosen as z1(0)=(0.51−i0.32,−0.29−i0.31,0.01+0.01)T,z2(0)=(−0.32−i0.3,−0.3−i0.31,0.01+i0.01)T,z3(0)=(−0.311−i0.3,−0.31−i0.32,0.01+i0.01)T,z4(0)=(−0.2−i0.3,−0.32−i0.31,0.01+i0.01)T. The coupling matrix G for CCVNN model (2.1) is
G=(1−1−1001000−1100001). |
Then, model (2.1) can be separated into two clusters C1= {1,2} and C2={3,4}. From the above findings we can easily show that the matrix ˜Λ+˜A−˜C−˜D−˜J is a non-singular matrix for suitable ˜Λ. Based on Theorem 8, CCVNNs (2.1) can realize CS asymptotically under the controller (3.1) with respect to C1,C2. To analyze the CS process, the error in each cluster is
ek(t)=∑i,j∈Ck‖zi(t)−Rk(t)‖1, k=1,2. |
For k=1,2 in Figures 5–6, we have ek(t)→0 when t→+∞, which implies that all states achieve CS asymptotically.
This study has examined the FT asymptotic and CS problem of delayed CVNN models with leakage delay. By utilizing the Lyapunov theory for differential systems and the Filippov regularization framework, new sufficient conditions to ensure the FT asymptotic and the CS for the considered drive-response coupled complex-valued models have been obtained. The conditions are formulated in terms of non-singular M-matrices for determining FT asymptotic and CS of CCVNN models. A simulation example with two cases has been given to validate the theoretical results. Although there exist many studies on CS [2,3,47,50] of CNNs and network control methods such as adaptive control [50], investigations on adaptive control schemes to realize adaptive and CS CCVNN models are limited. In this study, asymptotic CS of CCVNN models has been realized under adaptive control. The obtained results can extend further to those in the existing literature [2,3,47,50]. For further research, the dynamics of coupled delayed CVNN models with stochastic disturbances and impulsive effects will be investigated.
This research is made possible through financial support from the Rajamangala University of Technology Suvarnabhumi, Thailand. The authors are grateful to the Rajamangala University of Technology Suvarnabhumi, Thailand for supporting this research.
The authors declares no conflicts of interest.
[1] |
R. Manivannan, S. Panda, K. T. Chong, J. Cao, An Arcak-type state estimation design for time-delayed static neural networks with leakage term based on unified criteria, Neural Networks, 106 (2018), 110–126. https://doi.org/10.1016/j.neunet.2018.06.015 doi: 10.1016/j.neunet.2018.06.015
![]() |
[2] |
X. Zhang, C. Li, Z. He, Cluster synchronization of delayed coupled neural networks: Delay-dependent distributed impulsive control, Neural Networks, 142 (2021), 34–43. https://doi.org/10.1016/j.neunet.2021.04.026 doi: 10.1016/j.neunet.2021.04.026
![]() |
[3] |
W. Zhou, Y. Sun, X. Zhang, P. Shi, Cluster synchronization of coupled neural networks with Lévy noise via event-triggered pinning control, IEEE Transl. Neural Networks Learn. Syst., 106 (2021), 1–14. https://doi.org/10.1109/TNNLS.2021.3072475 doi: 10.1109/TNNLS.2021.3072475
![]() |
[4] |
X. Qi, H. Bao, J. Cao, Synchronization criteria for quaternion-valued coupled neural networks with impulses, Neural Networks, 128 (2020), 150–157. https://doi.org/10.1016/j.neunet.2020.04.027 doi: 10.1016/j.neunet.2020.04.027
![]() |
[5] |
A. Pratap, R. Raja, R. Agarwal, J. Cao, O. Bagdasar, Multi-weighted complex structure on fractional order coupled neural networks with linear coupling delay: A robust synchronization problem, Neural Process. Lett., 51 (2020), 2453–2479. https://doi.org/10.1007/s11063-019-10188-5 doi: 10.1007/s11063-019-10188-5
![]() |
[6] |
H. E. Elzain, S. Y. Chung, V. Senapathi, S. Sekar, N. Park, A. A. Mahmoud, Modeling of aquifer vulnerability index using deep learning neural networks coupling with optimization algorithms, Environ. Sci. Pollut. Res., 28 (2021), 57030–57045. https://doi.org/10.1007/s11356-021-14522-0 doi: 10.1007/s11356-021-14522-0
![]() |
[7] |
J. Xia, Y. Lu, L. Tan, Research of multimodal medical image fusion based on parameter-adaptive pulse-coupled neural network and convolutional sparse representation, Comput. Math. Methods Med., 2020 (2020), 3290136. https://doi.org/10.1155/2020/3290136 doi: 10.1155/2020/3290136
![]() |
[8] |
P. Chanthorn, G. Rajchakit, J. Thipcha, C. Emharuethai, R. Sriraman, C. P. Lim, R. Ramachandran, Robust stability of complex-valued stochastic neural networks with time-varying delays and parameter uncertainties, Mathematics, 8 (2020), 742. https://doi.org/10.3390/math8050742 doi: 10.3390/math8050742
![]() |
[9] |
G. Rajchakit, R. Sriraman, Robust passivity and stability analysis of uncertain complex-valued impulsive neural networks with time-varying delays, Neural Process. Lett., 53 (2021), 581–606. https://doi.org/10.1007/s11063-020-10401-w doi: 10.1007/s11063-020-10401-w
![]() |
[10] |
P. Chanthorn, G. Rajchakit, U. Humphries, P. Kaewmesri, R. Sriraman, C. P. Lim, A delay-dividing approach to robust stability of uncertain stochastic complex-valued hopfield delayed neural networks, Symmetry, 12 (2020), 683. https://doi.org/10.3390/sym12050683 doi: 10.3390/sym12050683
![]() |
[11] |
P. Chanthorn, G. Rajchakit, S. Ramalingam, C. P. Lim, R. Ramachandran, Robust dissipativity analysis of hopfield-type complex-valued neural networks with time-varying delays and linear fractional uncertainties, Mathematics, 8 (2020), 595. https://doi.org/10.3390/math8040595 doi: 10.3390/math8040595
![]() |
[12] |
L. Li, X. Shi, J. Liang, Synchronization of impulsive coupled complex-valued neural networks with delay: the matrix measure method, Neural Networks, 117 (2019), 285–294. https://doi.org/10.1016/j.neunet.2019.05.024 doi: 10.1016/j.neunet.2019.05.024
![]() |
[13] |
M. Hymavathi, G. Muhiuddin, M. Syed Ali, J. F. Al-Amri, N. Gunasekaran, R. Vadivel, Global exponential stability of fractional order complex-valued neural networks with leakage delay and mixed time varying delays, Fractal Fract., 6 (2022), 140. https://doi.org/10.3390/fractalfract6030140 doi: 10.3390/fractalfract6030140
![]() |
[14] |
N. Gunasekaran, G. Zhai, Sampled-data state-estimation of delayed complex-valued neural networks, Int. J. Syst. Sci., 51 (2020), 303–312. https://doi.org/10.1080/00207721.2019.1704095 doi: 10.1080/00207721.2019.1704095
![]() |
[15] |
R. Samidurai, R. Sriraman, J. Cao, Z. Tu, Effects of leakage delay on global asymptotic stability of complex‐valued neural networks with interval time‐varying delays via new complex‐valued Jensen's inequality, Int. J. Adapt. Control Signal Process., 32 (2018), 1294–312. https://doi.org/10.1002/acs.2914 doi: 10.1002/acs.2914
![]() |
[16] |
N. Gunasekaran, G. Zhai, Stability analysis for uncertain switched delayed complex-valued neural networks, Neurocomputing, 367 (2019), 198-206. https://doi.org/10.1016/j.neucom.2019.08.030 doi: 10.1016/j.neucom.2019.08.030
![]() |
[17] |
Y. Huang, J. Hou, E. Yang, Passivity and synchronization of coupled reaction-diffusion complex-valued memristive neural networks, Appl. Math. Comput., 379 (2020), 125271. https://doi.org/10.1016/j.amc.2020.125271 doi: 10.1016/j.amc.2020.125271
![]() |
[18] |
L. Feng, C. Hu, J. Yu, H. Jiang, S. Wen, Fixed-time synchronization of coupled memristive complex-valued neural networks, Chaos Solitons Fract., 148 (2021), 110993. https://doi.org/10.1016/j.chaos.2021.110993 doi: 10.1016/j.chaos.2021.110993
![]() |
[19] | W. Rudin, Real and complex analysis, McGraw-Hill, 1987. |
[20] |
N. Benvenuto, F. Piazza, On the complex backpropagation algorithm, IEEE Trans. Signal Process., 40 (1992), 967–969. https://doi.org/10.1109/78.127967 doi: 10.1109/78.127967
![]() |
[21] |
T. Nitta, Solving the XOR problem and the detection of symmetry using a single complex-valued neuron, Neural Networks, 16 (2003), 1101–1105. https://doi.org/10.1016/S0893-6080(03)00168-0 doi: 10.1016/S0893-6080(03)00168-0
![]() |
[22] |
M. Takeda, T. Kishigami, Complex neural fields with a hopfield-like energy function and an analogy to optical fields generated in phase-conjugate resonators, J. Opt. Soc. Am., 9 (1992), 2182–2191. https://doi.org/10.1364/JOSAA.9.002182 doi: 10.1364/JOSAA.9.002182
![]() |
[23] |
A. Pratap, R. Raja, J. Alzabut, J. Dianavinnarasi, J. Cao, G. Rajchakit, Finite-time Mittag-Leffler stability of fractional-order quaternion-valued memristive neural networks with impulses, Neural Process. Lett., 51 (2020), 1485–1526. https://doi.org/10.1007/s11063-019-10154-1 doi: 10.1007/s11063-019-10154-1
![]() |
[24] |
G. Rajchakit, P. Chanthorn, P. Kaewmesri, R. Sriraman, C.P. Lim, Global Mittag-Leffler stability and stabilization analysis of fractional-order quaternion-valued memristive neural networks, Mathematics, 8 (2020), 422. https://doi.org/10.3390/math8030422 doi: 10.3390/math8030422
![]() |
[25] |
N. Gunasekaran, N. M. Thoiyab, P. Muruganantham, G. Rajchakit, B. Unyong, Novel results on global robust stability analysis for dynamical delayed neural networks under parameter uncertainties, IEEE Access, 8 (2020), 178108–178116. https://doi.org/10.1109/ACCESS.2020.3016743 doi: 10.1109/ACCESS.2020.3016743
![]() |
[26] |
U. Humphries, G. Rajchakit, P. Kaewmesri, P. Chanthorn, R. Sriraman, R. Samidurai, et al., Global stability analysis of fractional-order quaternion-valued bidirectional associative memory neural networks, Mathematics, 8 (2020), 801. https://doi.org/10.3390/math8050801 doi: 10.3390/math8050801
![]() |
[27] | U. Humphries, G. Rajchakit, P. Kaewmesri, P. Chanthorn, R. Sriraman, R. Samidurai et al., Stochastic memristive quaternion-valued neural networks with time delays: An analysis on mean square exponential input-to-state stability, Mathematics, 8 (2020) 815. https://doi.org/10.3390/math8050815 |
[28] |
W. W. Zhang, H. Zhang, J. D. Cao, H. M. Zhang, D. Y. Chen, Synchronization of delayed fractional-order complex-valued neural networks with leakage delay, Phys. A Stat. Mech. Appl., 556 (2020), 124710. https://doi.org/10.1016/j.physa.2020.124710 doi: 10.1016/j.physa.2020.124710
![]() |
[29] |
P. Anbalagan, R. Ramachandran, J. Cao, G. Rajchakit, C. P. Lim, Global robust synchronization of fractional order complex valued neural networks with mixed time varying delays and impulses, Int. J. Control Autom. Syst., 17 (2019), 509–520. https://doi.org/10.1007/s12555-017-0563-7 doi: 10.1007/s12555-017-0563-7
![]() |
[30] |
N. Gunasekaran, G. Zhai, Q. Yu, Sampled-data synchronization of delayed multi-agent networks and its application to coupled circuit, Neurocomputing, 413 (2020), 499–511. https://doi.org/10.1016/j.neucom.2020.05.060 doi: 10.1016/j.neucom.2020.05.060
![]() |
[31] |
R. Vadivel, P. Hammachukiattikul, N. Gunasekaran, R. Saravanakumar, H. Dutta, Strict dissipativity synchronization for delayed static neural networks: An event-triggered scheme, Chaos Solitons Fract., 150 (2021), 111212. https://doi.org/10.1016/j.chaos.2021.111212 doi: 10.1016/j.chaos.2021.111212
![]() |
[32] |
M. Syed Ali, N. Gunasekaran, R. Agalya, Y. H. Joo, Non-fragile synchronisation of mixed delayed neural networks with randomly occurring controller gain fluctuations, Int. J. Syst. Sci., 49 (2018), 3354–3364. https://doi.org/10.1080/00207721.2018.1540730 doi: 10.1080/00207721.2018.1540730
![]() |
[33] |
R. Guo, S. Xu, J. Guo, Sliding-mode synchronization control of complex-valued inertial neural networks with leakage delay and time-varying delays, IEEE Trans. Syst. Man Cybern. Syst., 2022, 1–9. https://doi.org/10.1109/TSMC.2022.3193306 doi: 10.1109/TSMC.2022.3193306
![]() |
[34] |
N. Jayanthi, R. Santhakumari, Synchronization of time-varying time delayed neutral-type neural networks for finite-time in complex field, Math. Model. Comput., 8 (2021), 486–498. https://doi.org/10.23939/mmc2021.03.486 doi: 10.23939/mmc2021.03.486
![]() |
[35] |
N. Jayanthi, R. Santhakumari, Synchronization of time invariant uncertain delayed neural networks in finite time via improved sliding mode control, Math. Model. Comput., 8 (2021), 228–240. https://doi.org/10.23939/mmc2021.02.228 doi: 10.23939/mmc2021.02.228
![]() |
[36] |
R. Anbuvithya, S. Dheepika Sri, R. Vadivel, P. Hammachukiattikul, C. Park, G. Nallappan, Extended dissipativity synchronization for Markovian jump recurrent neural networks via memory sampled-data control and its application to circuit theory, Int. J. Nonlinear Anal. Appl., 13 (2022), 2801–2820. https://doi.org/10.22075/IJNAA.2021.25114.2919 doi: 10.22075/IJNAA.2021.25114.2919
![]() |
[37] |
J. Bai, H. Wu, J. Cao, Secure synchronization and identification for fractional complex networks with multiple weight couplings under DoS attacks, Comput. Appl. Math., 41 (2022), 187. https://doi.org/10.1007/s40314-022-01895-2 doi: 10.1007/s40314-022-01895-2
![]() |
[38] |
Z. Ruan, Y. Li, J. Hu, J. Mei, D. Xia, Finite-time synchronization of the drive-response networks by event-triggered aperiodic intermittent control, Neurocomputing, 485 (2022), 89–102 https://doi.org/10.1016/j.neucom.2022.02.037 doi: 10.1016/j.neucom.2022.02.037
![]() |
[39] |
N. Gunasekaran, R. Saravanakumar, Y. H. Joo, H. S. Kim, Finite-time synchronization of sampled-data T–S fuzzy complex dynamical networks subject to average dwell-time approach, Fuzzy Sets Syst., 374 (2019), 40–59. https://doi.org/10.1016/j.fss.2019.01.007 doi: 10.1016/j.fss.2019.01.007
![]() |
[40] |
C. Wang, H. Zhang, I. Stamova, J. Cao, Global synchronization for BAM delayed reaction-diffusion neural networks with fractional partial differential operator, J. Franklin Inst., 2022. https://doi.org/10.1016/j.jfranklin.2022.08.038 doi: 10.1016/j.jfranklin.2022.08.038
![]() |
[41] |
H. Zhang, Y. Cheng, H. Zhang, W. Zhang, J. Cao, Hybrid control design for Mittag-Leffler projective synchronization on FOQVNNs with multiple mixed delays and impulsive effects, Math. Comput. Simul., 197 (2022), 341–357. https://doi.org/10.1016/j.matcom.2022.02.022 doi: 10.1016/j.matcom.2022.02.022
![]() |
[42] |
X. Li, H. Wu, J. Cao, Prescribed-time synchronization in networks of piecewise smooth systems via a nonlinear dynamic event-triggered control strategy, Math. Comput. Simul., 203 (2023), 647-668. https://doi.org/10.1016/j.matcom.2022.07.010 doi: 10.1016/j.matcom.2022.07.010
![]() |
[43] |
M. Syed Ali, M. Hymavathi, G. Rajchakit, S. Saroha, L. Palanisamy, P. Hammachukiattikul, Synchronization of fractional order fuzzy BAM neural networks with time varying delays and reaction diffusion terms, IEEE Access, 8 (2020), 186551–186571. https://doi.org/10.1109/ACCESS.2020.3029145 doi: 10.1109/ACCESS.2020.3029145
![]() |
[44] |
D. Liu, S. Zhu, K. Sun, Global anti-synchronization of complex-valued memristive neural networks with time delays, IEEE Trans. Cybern., 49 (2019), 1735–1747. https://doi.org/10.1109/TCYB.2018.2812708 doi: 10.1109/TCYB.2018.2812708
![]() |
[45] |
B. Hu, Z. Guan, N. Xiong, H. Chao, Intelligent impulsive synchronization of nonlinear interconnected neural networks for image protection, IEEE Trans. Ind. Inf., 14 (2018), 3775–3787. https://doi.org/10.1109/TII.2018.2808966 doi: 10.1109/TII.2018.2808966
![]() |
[46] |
L. V. Gambuzza, M. Frasca, A criterion for stability of cluster synchronization in networks with external equitable partitions, Automatica, 100 (2019), 212–218. https://doi.org/10.1016/j.automatica.2018.11.026 doi: 10.1016/j.automatica.2018.11.026
![]() |
[47] |
J. Qin, W. Fu, Y. Shi, H. Gao, Y. Kang, Leader-following practical cluster synchronization for networks of generic linear systems: An event-based approach, IEEE Trans. Neural Networks Learn. Syst., 30 (2018), 215–224. https://doi.org/10.1109/TNNLS.2018.2817627 doi: 10.1109/TNNLS.2018.2817627
![]() |
[48] |
N. A. Lai, W. Xiang, Y. Zhou, Global instability of multi-dimensional plane shocks for isothermal flow, Acta Math. Sci., 42 (2022), 887–902. https://doi.org/10.1007/s10473-022-0305-7 doi: 10.1007/s10473-022-0305-7
![]() |
[49] |
Z. Zhang, H. Wu, Cluster synchronization in finite/fixed time for semi-Markovian switching TS fuzzy complex dynamical networks with discontinuous dynamic nodes, AIMS Math., 7 (2022), 11942–11971. https://doi.org/10.3934/math.2022666 doi: 10.3934/math.2022666
![]() |
[50] |
P. Liu, Z. Zeng, J. Wang, Asymptotic and finite-time cluster synchronization of coupled fractional order neural networks with time delay, IEEE Trans. Neural Networks Learn. Syst., 31 (2020), 4956–4967. https://doi.org/10.1109/TNNLS.2019.2962006 doi: 10.1109/TNNLS.2019.2962006
![]() |
[51] |
X. Zhang, C. Li, Z. He, Cluster synchronization of delayed coupled neural networks: Delay-dependent distributed impulsive control, Neural Networks, 142 (2021), 34–43. https://doi.org/10.1016/j.neunet.2021.04.026 doi: 10.1016/j.neunet.2021.04.026
![]() |
[52] |
S. Yang, C. Hu, J. Yu, H. Jiang, Finite-time cluster synchronization in complex-variable networks with fractional-order and nonlinear coupling, Neural Networks, 135 (2021), 212–224. https://doi.org/10.1016/j.neunet.2020.12.015 doi: 10.1016/j.neunet.2020.12.015
![]() |
[53] |
T. Yu, J. Cao, C. Huang, Finite-time cluster synchronization of coupled dynamical systems with impulsive effects, Discrete Contin. Dyn. Syst. B, 26 (2021), 3595–3620. https://doi.org/10.3934/dcdsb.2020248 doi: 10.3934/dcdsb.2020248
![]() |
[54] |
J. J. He, Y. Q. Lin, M. F. Ge, C. D. Liang, T. F. Ding, L. Wang, Adaptive finite-time cluster synchronization of neutral-type coupled neural networks with mixed delays, Neurocomputing, 384 (2020), 11–20. https://doi.org/10.1016/j.neucom.2019.11.046 doi: 10.1016/j.neucom.2019.11.046
![]() |
[55] |
R. Tang, X. Yang, X. Wan, Finite-time cluster synchronization for a class of fuzzy cellular neural networks via non-chattering quantized controllers, Neural Networks, 113 (2019), 79–90. https://doi.org/10.1016/j.neunet.2018.11.010 doi: 10.1016/j.neunet.2018.11.010
![]() |
[56] |
D. Liu, Y. Du, New results of stability analysis for a class of neutral-type neural network with mixed time delays, Int. J. Mach. Learn. Cybern., 6 (2015), 555–566. https://doi.org/10.1007/s13042-014-0302-9 doi: 10.1007/s13042-014-0302-9
![]() |
[57] |
Y. Cao, R. Samidurai, R. Sriraman, Robust passivity analysis for uncertain neural networks with leakage delay and additive time-varying delays by using general activation function, Math. Comput. Simul., 155 (2019), 57–77. https://doi.org/10.1016/j.matcom.2017.10.016 doi: 10.1016/j.matcom.2017.10.016
![]() |
[58] |
H. Zhang, J. Cheng, H. Zhang, W. Zhang, J. Cao, Quasi-uniform synchronization of Caputo type fractional neural networks with leakage and discrete delays, Chaos Solitons Fract., 152 (2021), 111432. https://doi.org/10.1016/j.chaos.2021.111432 doi: 10.1016/j.chaos.2021.111432
![]() |
[59] |
X. Wei, Z. Zhang, M. Liu, Z. Wang, J. Chen, Anti-synchronization for complex-valued neural networks with leakage delay and time-varying delays, Neurocomputing, 412 (2020), 312–319. https://doi.org/10.1016/j.neucom.2020.06.080 doi: 10.1016/j.neucom.2020.06.080
![]() |
[60] |
L. Wang, Q. Song, Z. Zhao, Y. Liu, F. E. Alsaadi, Synchronization of two nonidentical complex-valued neural networks with leakage delay and time-varying delays, Neurocomputing, 356 (2019), 52–59. https://doi.org/10.1016/j.neucom.2019.04.068 doi: 10.1016/j.neucom.2019.04.068
![]() |
[61] |
M. S. Ali, N. Gunasekaran, C. K. Ahn, P. Shi, Sampled-data stabilization for fuzzy genetic regulatory networks with leakage delays, IEEE/ACM Trans. Comput. Biol. Bioinf., 15 (2016), 271–285. https://doi.org/10.1109/TCBB.2016.2606477 doi: 10.1109/TCBB.2016.2606477
![]() |
[62] |
A. Pratap, R. Raja, J. Cao, G. Rajchakit, F. E. Alsaadi, Further synchronization in finite time analysis for time-varying delayed fractional order memristive competitive neural networks with leakage delay, Neurocomputing, 317 (2018), 110–126. https://doi.org/10.1016/j.neucom.2018.08.016 doi: 10.1016/j.neucom.2018.08.016
![]() |
[63] |
Q. Song, Z. Zhao, Stability criterion of complex-valued neural networks with both leakage delay and time-varying delays on time scales, Neurocomputing, 171 (2016), 179–184. https://doi.org/10.1016/j.neucom.2015.06.032 doi: 10.1016/j.neucom.2015.06.032
![]() |
[64] |
R. Samidurai, R. Sriraman, S. Zhu, Leakage delay-dependent stability analysis for complex-valued neural networks with discrete and distributed time-varying delays, Neurocomputing, 338 (2016), 262–273. https://doi.org/10.1016/j.neucom.2019.02.027 doi: 10.1016/j.neucom.2019.02.027
![]() |
[65] |
N. F. Rulkov, Images of synchronized chaos: Experiments with circuits, Chaos, 6 (1996), 262–279. https://doi.org/10.1063/1.166174 doi: 10.1063/1.166174
![]() |
[66] |
J. Zhou, Y. Zhao, Z. Wu, Cluster synchronization of fractional-order directed networks via intermittent pinning control, Physica A Stat. Mech. Appl., 519 (2019), 22–33. https://doi.org/10.1016/j.physa.2018.12.032 doi: 10.1016/j.physa.2018.12.032
![]() |
[67] |
S. Lakshmanan, J. H. Park, H. Y. Jung, P. Balasubramaniam, Design of state estimator for neural networks with leakage, discrete and distributed delays, Appl. Math. Comput., 218 (2012), 11297–11310. https://doi.org/10.1016/j.amc.2012.05.022 doi: 10.1016/j.amc.2012.05.022
![]() |
[68] |
T. Li, W. X. Zheng, C. Lin, Delay-slope-dependent stability results of recurrent neural networks, IEEE Trans. Neural Networks, 22 (2011), 2138–2143. https://doi.org/10.1109/TNN.2011.2169425 doi: 10.1109/TNN.2011.2169425
![]() |
[69] |
L. Wen, Y. Yu, W. Wang, Generalized Halanay inequalities for dissipativity of Volterra functional differential equations, J. Math. Anal. Appl., 347 (2008), 169–178. https://doi.org/10.1016/j.jmaa.2008.05.007 doi: 10.1016/j.jmaa.2008.05.007
![]() |
[70] |
X. Li, X. Fu, Effect of leakage time-varying delay on stability of nonlinear differential systems, J. Franklin Inst., 350 (2013), 1335–1344. https://doi.org/10.1016/j.jfranklin.2012.04.007 doi: 10.1016/j.jfranklin.2012.04.007
![]() |
[71] |
K. Kaneko, Relevance of dynamic clustering to biological networks, Phys. D Nonlinear Phenom., 75 (1994), 55–73. https://doi.org/10.1016/0167-2789(94)90274-7 doi: 10.1016/0167-2789(94)90274-7
![]() |
[72] |
W. Yu, J. Cao, J. Lü, Global synchronization of linearly hybrid coupled networks with time-varying delay, SIAM J. Appl. Dyn. Syst., 7 (2008), 108–133. https://doi.org/10.1137/070679090 doi: 10.1137/070679090
![]() |
[73] |
J. Cao, G. Chen, P. Li, Global synchronization in an array of delayed neural networks with hybrid coupling, IEEE Trans. Syst. Man Cybern., 38 (2008), 488–498. https://doi.org/10.1109/TSMCB.2007.914705 doi: 10.1109/TSMCB.2007.914705
![]() |
[74] |
J. Cao, W. Yu, Y. Qu, A new complex network model and convergence dynamics for reputation computation in virtual organizations, Phys. lett. A, 356 (2006), 414–425 https://doi.org/10.1016/j.physleta.2006.04.005 doi: 10.1016/j.physleta.2006.04.005
![]() |
[75] |
L. Hu, H. Gao, W. Zheng, Novel stability of cellular neural networks with interval time-varying delay, Neural Networks, 21 (2008), 1458–1463. https://doi.org/10.1016/j.neunet.2008.09.002 doi: 10.1016/j.neunet.2008.09.002
![]() |
[76] |
S. Mou, H. Gao, W. Qiang, K. Chen, New delay-dependent exponential stability for neural networks with time delay, IEEE Trans. Syst. Man Cybern., 38 (2008), 571–576. https://doi.org/10.1109/TSMCB.2007.913124 doi: 10.1109/TSMCB.2007.913124
![]() |
[77] |
X. Peng, H. Wu, K. Song, J. Shi, Global synchronization in finite time for fractional-order neural networks with discontinuous activations and time delays, Neural Networks, 94 (2017), 46–54. https://doi.org/10.1016/j.neunet.2017.06.011 doi: 10.1016/j.neunet.2017.06.011
![]() |
[78] |
Z. Ding, Z. Zeng, L. Wang, Robust finite-time stabilization of fractional-order neural networks with discontinuous and continuous activation functions under uncertainty, IEEE Trans. Neural Networks Learn. Syst., 29 (2017), 1477–1490. https://doi.org/10.1109/TNNLS.2017.2675442 doi: 10.1109/TNNLS.2017.2675442
![]() |
[79] |
X. Yang, Can neural networks with arbitrary delays be finite-timely synchronized, Neurocomputing, 143 (2014), 275–281. https://doi.org/10.1016/j.neucom.2014.05.064 doi: 10.1016/j.neucom.2014.05.064
![]() |
1. | G. Soundararajan, G. Nagamani, Ardak Kashkynbayev, Exponential H∞ filtering for complex-valued uncertain discrete-time neural networks with time-varying delays, 2024, 128, 10075704, 107595, 10.1016/j.cnsns.2023.107595 | |
2. | Muhammad Marwan, Maoan Han, Rizwan Khan, Muhammad Aqeel, Generalized external synchronization of networks based on clustered pandemic systems—The approach of Covid-19 towards influenza, 2023, 18, 1932-6203, e0288796, 10.1371/journal.pone.0288796 | |
3. | Jie Liu, Jian-Ping Sun, Pinning clustering component synchronization of nonlinearly coupled complex dynamical networks, 2024, 9, 2473-6988, 9311, 10.3934/math.2024453 |