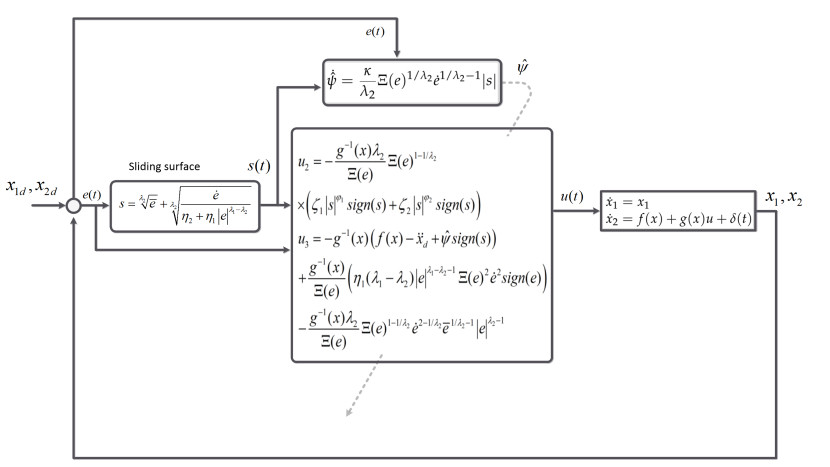
This work introduces a novel adaptive fixed-time control strategy for nonlinear systems subject to external disturbances. The focus pertains to the introduction of the fixed-time terminal sliding mode control (FxSMC) technique. The proposed scheme exhibits rapid convergence, chatter-free and smooth control inputs, and stability within a fixed time. The utilization of an adaptive methodology in combination with the FxSMC yields the proposed strategy. This approach is employed to address the dynamic system in the presence of external disturbances. The results obtained from the Lyapunov analysis will provide insights into the stability of the closed-loop system in a fixed time. In the end, the simulation results are presented in order to assess and demonstrate the effectiveness of the methodology.
Citation: Saim Ahmed, Ahmad Taher Azar, Ibraheem Kasim Ibraheem. Nonlinear system controlled using novel adaptive fixed-time SMC[J]. AIMS Mathematics, 2024, 9(4): 7895-7916. doi: 10.3934/math.2024384
[1] | Saim Ahmed, Ahmad Taher Azar, Ibraheem Kasim Ibraheem . Model-free scheme using time delay estimation with fixed-time FSMC for the nonlinear robot dynamics. AIMS Mathematics, 2024, 9(4): 9989-10009. doi: 10.3934/math.2024489 |
[2] | Zhiqiang Chen, Alexander Yurievich Krasnov . Disturbance observer based fixed time sliding mode control for a class of uncertain second-order nonlinear systems. AIMS Mathematics, 2025, 10(3): 6745-6763. doi: 10.3934/math.2025309 |
[3] | Honglei Yin, Bo Meng, Zhen Wang . Disturbance observer-based adaptive sliding mode synchronization control for uncertain chaotic systems. AIMS Mathematics, 2023, 8(10): 23655-23673. doi: 10.3934/math.20231203 |
[4] | Majid Roohi, Saeed Mirzajani, Ahmad Reza Haghighi, Andreas Basse-O'Connor . Robust stabilization of fractional-order hybrid optical system using a single-input TS-fuzzy sliding mode control strategy with input nonlinearities. AIMS Mathematics, 2024, 9(9): 25879-25907. doi: 10.3934/math.20241264 |
[5] | Hany Bauomy . Control and optimization mechanism of an electromagnetic transducer model with nonlinear magnetic coupling. AIMS Mathematics, 2025, 10(2): 2891-2929. doi: 10.3934/math.2025135 |
[6] | Miao Xiao, Zhe Lin, Qian Jiang, Dingcheng Yang, Xiongfeng Deng . Neural network-based adaptive finite-time tracking control for multiple inputs uncertain nonlinear systems with positive odd integer powers and unknown multiple faults. AIMS Mathematics, 2025, 10(3): 4819-4841. doi: 10.3934/math.2025221 |
[7] | Yebin Li, Dongshu Wang, Zuowei Cai . On asymptotic fixed-time controller design for uncertain nonlinear systems with pure state constraints. AIMS Mathematics, 2023, 8(11): 27151-27174. doi: 10.3934/math.20231389 |
[8] | Jiaojiao Li, Yingying Wang, Jianyu Zhang . Event-triggered sliding mode control for a class of uncertain switching systems. AIMS Mathematics, 2023, 8(12): 29424-29439. doi: 10.3934/math.20231506 |
[9] | Yifan Liu, Guozeng Cui, Ze Li . Fixed-time consensus control of stochastic nonlinear multi-agent systems with input saturation using command-filtered backstepping. AIMS Mathematics, 2024, 9(6): 14765-14785. doi: 10.3934/math.2024718 |
[10] | Bin Hang, Weiwei Deng . Finite-time adaptive prescribed performance DSC for pure feedback nonlinear systems with input quantization and unmodeled dynamics. AIMS Mathematics, 2024, 9(3): 6803-6831. doi: 10.3934/math.2024332 |
This work introduces a novel adaptive fixed-time control strategy for nonlinear systems subject to external disturbances. The focus pertains to the introduction of the fixed-time terminal sliding mode control (FxSMC) technique. The proposed scheme exhibits rapid convergence, chatter-free and smooth control inputs, and stability within a fixed time. The utilization of an adaptive methodology in combination with the FxSMC yields the proposed strategy. This approach is employed to address the dynamic system in the presence of external disturbances. The results obtained from the Lyapunov analysis will provide insights into the stability of the closed-loop system in a fixed time. In the end, the simulation results are presented in order to assess and demonstrate the effectiveness of the methodology.
Nonlinear systems subject to external disturbances are frequently encountered in various engineering domains, including but not limited to servo position control systems, interconnected mechanical systems, and robotic manipulators. These systems frequently encounter uncertainties and disturbances that have the potential to impact the system's performance and stability [1,2]. In order to tackle these challenges, researchers have devised a range of control techniques, such as sliding mode control (SMC) and adaptive control [3]. The controller that has been proposed integrates the benefits of sliding mode control and adaptive control to enhance transient performance and obtain the robustness of the overall dynamical system. Therefore, the disturbance does not affect, and the sliding mode converges in a fixed-time. The utilization of an adaptation tuning approach is employed to address system uncertainties and external disturbances that are unknown but bounded.
The SMC scheme has been used a lot in the field of nonlinear control systems because it can handle uncertainties and disturbances well, making the system robust [4]. However, chattering occurrence in the control input is a problem with this method. The act of chattering can result in the production of unwanted noise inside the system, hence potentially leading to instability of the system performance [5,6]. Several ways to stop chattering have been suggested, such as using a saturation function, adding a boundary layer, or using an integral sliding mode [7,8,9]. In addition, the developments of SMC over the years have been given as terminal SMC, nonsingular SMC, fast SMC, fast nonsingular SMC, and finite-time SMC to obtain better tracking, fast convergence, nonsingular and chatter-free control input [10,11,12,13,14,15]. However, the finite-time scheme is strongly influenced by the starting values of the states to converge. Hence, a fixed-time control is an alternate scheme that can be used to compute the convergence time and it is independent of the initial values [16].
Adaptive control is a technique that modifies the controller parameters based on the system's current state [17]. It is a well-known nonlinear control scheme and is often used in complex systems to compensate the unknown nonlinear dynamics. Therefore, this method can improve the performance and stability of a system by changing the parameters of the controller in real-time to counteract the effects of uncertainty, nonlinearity, and external disturbances [18]. Moreover, this control approach is extensively employed for controlling the behavior of both linear and nonlinear systems [17]. The combination of adaptive control schemes has been found with various classical and complex control schemes, including PI, PD, PID [19,20,21,22], sliding mode control [23,24], H∞ robust control [25], optimal control [26,27], time delay control [28], multi-agent system control [29], event-triggered control [30], fuzzy logic control [31,32], and conventional and radial basis function neural network (NN) scheme [33,34]. Thus, various adaptive control schemes have been designed with sliding mode control for the nonlinear dynamics to obtain robustness, improved tracking performance, fast convergence, non-singularity, reduced chattering, and adaptive tuning control as well [35].
Since the convergence speed varies as the initial value changes in a finite-time scheme, therefore, fixed-time control has been used in this work which does not rely on the initial conditions of the system states. A proposed approach is presented called novel adaptive fixed-time sliding mode control (NAFxSMC) for addressing the control of a class of nonlinear systems that are influenced by external disturbances. The proposed scheme integrates the benefits of sliding mode control and adaptive control techniques. The objective is to enhance the tracking and transient performances and to obtain the robustness of the closed-loop system. The proposed control method is designed to achieve fast convergence of the sliding mode within a fixed time. And the adaptive approach is utilized to compensate the unknown bounded external disturbances. Then, the verification of the fixed-time stability of the closed-loop nonlinear system is conducted using the Lyapunov theorem analysis.
This work is organized as follows: In Section 2, the related work is given in detail. Section 3 describes the main results including the proposed adaptive sliding mode control approach and the stability analyses. In Section 4, the numerical simulation results are illustrated. In Section 5, the discussion and analysis are presented. Finally, Section 6 closes the work and discusses future research directions.
Nonlinear systems benefit from fixed-time sliding mode control, which has convergence within a predetermined time frame and is unique in many respects. As a result, a considerable number of experts have recently focused their efforts on examining this particular subject [36]. To successfully manage the uncertainties and disturbances that are inherent in a nonlinear system, the authors propose the use of fixed-time fractional-order SMC [37]. The research findings were validated, demonstrating progress in fractional nonlinear chaotic systems using a novel synchronized fixed-time SMC technique [38]. A fast fixed-time SMC scheme using an extreme learning machine has been developed for the dynamics of robotic manipulator systems with uncertainties and disturbances [39]. Moreover, for a permanent-magnet synchronous motor, a SMC based on a fixed-time observer has been proposed. The fixed-time convergence SMC is designed to control both the inner loop, which regulates current, and the outer loop, which tracks speed [40]. A novel anti-saturation faster fixed-time adaptive NN-SMC has been developed for the uncertain dynamics of robotic system [41]. In [33], a robust fixed-time SMC control has been proposed for a class of nonlinear Euler-Lagrange systems under exogenous disturbances and uncertain dynamics. In which, a neural network is used as an adaptive estimation algorithm to approximate the continuous uncertain dynamics. An enhanced adaptive rapid integral sliding mode control technique has been developed with a specific focus on addressing the nonlinear dynamics of quadrotor applications [42]. The authors came up with a new way to predict unknown external disturbances that uses a high-order sliding mode observer [43]. When combined with exponential fixed-time SMC, this method successfully handles the control issues that come with nonlinear Euler-Lagrange systems [36]. Prescribed tracking performance has been obtained using proportional-integral funnel control for unknown lower-triangular nonlinear systems [44], and neural network control for underactuated surface vehicles [45]. This scheme enhances the control performance and allows the freely predefined convergence rate and accuracy.
Using the aforementioned procedures, one can develop effective adaptive schemes for the regulation of linear and nonlinear systems by utilizing a number of different types of adaptive control strategies. This enables the estimation and reduction of unknown dynamics [17]. In order to achieve fast responsiveness and get rid of chattering and singularity problems, the work used an adaptive finite-time terminal sliding mode control strategy to estimate uncertainties and external disturbances [18]. The researchers put forth a fuzzy adaptive sliding mode control methodology in order to address the existence of unfamiliar underactuated dynamics within a nonlinear system [46]. A robust adaptive fault-tolerant control circuit design has been developed for the disturbed continuous-time systems, converting them into analog circuits [47]. The approach is demonstrated using an adaptive compensation scheme against actuator faults and disturbances, implementing equivalent control circuits. The researchers proposed a novel adaptive fixed-time sliding mode control strategy to effectively handle unknown nonlinearities and faults in robot systems [48]. A sliding mode control technique was developed for a nonlinear robot with unknown and uncertain dynamics using an adaptive fixed-time control approach [9]. A novel adaptive strategy using the fast terminal sliding mode control technique was introduced in order to tackle the issue of accurately tracking the attitude of a spaceship with uncertain nonlinear dynamics while also considering the presence of saturation and actuator faults [49]. In [50], the design of a sliding mode controller reinforced by an adaptive law is discussed to control the desired state of three-story excited structure. An adaptive fractional-order sliding mode control strategy for uncertain fractional-order nonlinear systems with H∞ adaptive observer is proposed to estimate system states and unknown parameters [51]. Another adaptive nonlinear sliding mode control scheme for improving the performance of controllers in AC/DC and DC/DC converters. This control method improves the efficacy of controllers and provides reliable and efficient performance [52].
The present section begins with an analysis of nonlinear dynamical systems. The subsequent analysis designed a sliding surface and control scheme that are fixed-time and non-singular. Then, the investigation of stability analyses completes the development of the proposed control system.
The nonlinear system can be represented as [53]:
˙x1=x2,˙x2=f(x)+g(x)u+δ(t), | (3.1) |
where x=[x1,x2]T∈R, f(x) and g(x) are known functions, f(x) is a bounded continuous nonlinear function, and g(x) is a nonlinear function with g(x)≠0. δ(t)∈R is the unknown disturbance, and u∈R represents the control input.
To calculate the tracking error, (3.1) is employed in the following manner:
¨e=f(x)+g(x)u+δ(t)−¨xd, | (3.2) |
where xd is the desired state, e=x1−xd, ˙e=˙x1−˙xd and ¨e=¨x1−¨xd.
Assumption 1. The following equation presents a bounded condition for an unknown external disturbance:
|δ(t)|≤ψ, | (3.3) |
where ψ is an unknown positive constant.
This section presents the design of a fixed-time nonsingular sliding surface, that enables accurate and robust tracking performance for nonlinear systems within a fixed-time interval. Motivated by [16], the sliding manifold is given as
s=λ2√ˉe+λ2√˙eη2+η1|e|λ1−λ2, | (3.4) |
where ˉe=|e|λ2sign(e), constant values are λ1>1, 0<λ2<1, and η1,η2>0.
The above equation can be expressed as
s=λ2√ˉe+λ2√Ξ(e)˙e, | (3.5) |
where
Ξ(e)=1η2+η1|e|λ1−λ2. |
The derivative of (3.5) is obtained as follows:
˙s=ˉe1/λ2−1|e|λ2−1˙e+1λ2(Ξ(e)˙e)1/λ2−1[(η2+η1|e|λ1−λ2)¨e−η1(λ1−λ2)|e|λ1−λ2−1˙e2sign(e)(η2+η1|e|λ1−λ2)2]. | (3.6) |
Then it can be rewritten as
˙s=ˉe1/λ2−1|e|λ2−1˙e+1λ2(Ξ(e)˙e)1/λ2−1[Ξ(e)¨e−η1(λ1−λ2)|e|λ1−λ2−1Ξ(e)2˙e2sign(e)]. | (3.7) |
Substitution of error Eq (3.2) into (3.7), one gets
˙s=ˉe1/λ2−1|e|λ2−1˙e+1λ2(Ξ(e)˙e)1/λ2−1[Ξ(e){f(x)+g(x)u+δ(t)−¨xd}−η1(λ1−λ2)|e|λ1−λ2−1Ξ(e)2˙e2sign(e)]. | (3.8) |
Given the completion of the design of the sliding manifold, the forthcoming design of the NAFxSMC scheme for the nonlinear system aims to attain robust efficiency even in the presence of external disturbance.
The control FxSMC method can be formulated in the following manner, with the aim of efficiently controlling the nonlinear system in the presence of bounded disturbances:
u1=−g−1(x)(f(x)−¨xd+ψsign(s))+g−1(x)Ξ(e)(η1(λ1−λ2)|e|λ1−λ2−1Ξ(e)2˙e2sign(e))−g−1(x)λ2Ξ(e)Ξ(e)1−1/λ2˙e2−1/λ2ˉe1/λ2−1|e|λ2−1, | (3.9) |
u2=−g−1(x)λ2Ξ(e)Ξ(e)1−1/λ2(ζ1|s|φ1sign(s)+ζ2|s|φ2sign(s)), | (3.10) |
where u1+u2=u, u1 is nominal and u2 is switching control inputs with condition |e|λ2−1≠0 if e≠0. ζ1 and ζ2 are positive constants, φ1>1 and 0<φ2<1. Substitution of u1 and u2 into (3.8), one can have
˙s=−(Ξ(e)˙e)1/λ2−1[Ξ(e)1−1/λ2(ζ1|s|φ1sign(s)+ζ2|s|φ2sign(s))+1λ2Ξ(e)(−ψsign(s)+δ(t))]. | (3.11) |
In this subsection, stability analyses of the proposed control scheme have been established. The following important lemma will be used for the fixed-time calculation.
Lemma 1 ([9]). Considering the nonlinear system, we have
˙y(t)=f(t,y),y(0)=y0 | (3.12) |
with continuous function f(t,y). Therefore, the Lyapunov candidate V(y) that satisfies:
(i) V(y)=0⇔y=0,
(ii) ˙V(y)≤−a1Vb1(y)−a2V(y)b2,
with a1,a2>0,b1>1and0<b2<1. Subsequently, the fixed-time can be formulated as
T≤1a2(1−b2)+1a1(b1−1). | (3.13) |
For the stability of error dynamics, the Lyapunov function can be selected in the following manner:
V1=12e2. | (3.14) |
Then (3.14) can be derived as
˙V1=e˙e. | (3.15) |
When s=0, we can get the dynamics from (3.4) as
˙e=−η2|e|λ2sign(e)−η1|e|λ1sign(e). |
Thus, we can obtain (3.16) by the substitution of ˙e into (3.15) as
˙V1=e(−η2|e|λ2sign(e)−η1|e|λ1sign(e)), | (3.16) |
˙V1=−η1|e|λ1+1−η2|e|λ2+1. | (3.17) |
It can be expressed as
˙V1≤−η1(2V1)λ1+12−η2(2V1)λ2+12, | (3.18) |
˙V1≤−η12λ1+12V1λ1+12−η22λ2+12V1λ2+12. | (3.19) |
This expression represents that the error converges within a fixed-time, therefore, the convergence time Te can be computed using Lemma 1.
Now, the Lyapunov theorem analysis will be employed in Theorem 1 to determine the stability of a closed-loop system.
Theorem 1. The nonlinear system given in (3.1) is fixed-time stable with the suggested sliding surface (3.4), control method designed in (3.9) and (3.10).
Proof. The function for the Lyapunov candidate is as follows:
V2=12s2. | (3.20) |
Then ˙V2(t) can be computed as
˙V2=s˙s. | (3.21) |
Equation (3.11) is substituted into (3.21), one can get
˙V2=−s{(Ξ(e)˙e)1/λ2−1[Ξ(e)1−1/λ2(ζ1|s|φ1sign(s)+ζ2|s|φ2sign(s))+1λ2Ξ(e)(−ψsign(s)+δ(t))]}. | (3.22) |
Simplifying the above equation with (3.3), one can formulate
˙V2≤−˙e1/λ2−1(ζ1|s|φ1+1+ζ2|s|φ2+1). | (3.23) |
There are two possible cases:
1) ˙e1/λ2−1>0when˙e≠0,
2) ˙e1/λ2−1=0when˙e=0.
For ˙e≠0, the condition for Lyapunov fixed-time stability is always satisfied as ˙e1/λ2−1>0. For ˙e=0, by substituting (3.9) and (3.10) into (3.2), we get
¨e=−ψsign(s)+δ(t)−λ2Ξ(e)Ξ(e)1−1/λ2(ζ1|s|φ1sign(s)+ζ2|s|φ2sign(s)). |
As can be easily observed, when s>0, ¨e is negative, and when s<0, ¨e is positive. This implies that ˙e=0 cannot be an attractor. Thus, no trajectory is able to remain on the ˙e=0, every trajectory will intersect ˙e=0 and reach the sliding surface in fixed time. Based on Lemma 1, the fixed time Ts1 can easily be calculated as
Ts1≤1ζ12φ1+12(φ1+12−1)+1ζ22φ2+12(1−φ2+12) |
and then total time can be obtained as T1=Ts1+Te.
The subsequent equations present a comprehensive description of the control input, incorporating an adaptive law to address the challenges encountered by an unknown disturbance
u(t)=u2(t)+u3(t) | (3.24) |
where u2(t) is similar to (3.10), and adaptive control input is designed as
u3=−g−1(x)(f(x)−¨xd+ˆψsign(s))+g−1(x)Ξ(e)(η1(λ1−λ2)|e|λ1−λ2−1Ξ(e)2˙e2sign(e))−g−1(x)λ2Ξ(e)Ξ(e)1−1/λ2˙e2−1/λ2ˉe1/λ2−1|e|λ2−1 | (3.25) |
whereas ˆψ is the estimation of ψ.
The resulting adaptive law is proposed as a solution for solving unknown dynamics
˙ˆψ=κλ2Ξ(e)1/λ2˙e1/λ2−1|s|, | (3.26) |
where κ>0 is known constant. Substitution of (3.24) into (3.8), one can obtain
˙s=−(Ξ(e)˙e)1/λ2−1[Ξ(e)1−1/λ2(ζ1|s|φ1sign(s)+ζ2|s|φ2sign(s))+1λ2Ξ(e)(−ˆψsign(s)+δ(t))]. | (3.27) |
The use of Eq (3.24) can successfully solve the problem relating to the compensation of unknown dynamics. Consequently, the NAFxTSM technique is employed to achieve tracking performance in nonlinear systems under the influence of disturbances.
Theorem 2. The nonlinear dynamics in (3.1) are fixed-time stable with the proposed sliding surface (3.4), the control scheme given in (3.24), and the adaptive control laws (3.26).
Proof. Here is the chosen Lyapunov function:
V3=12s2+12κ˜ψ2, | (3.28) |
where ˜ψ=ˆψ−ψ is the estimation error. The ˙V3(t) is obtained as follows
˙V3=s˙s+1κ˜ψ˙ˆψ. | (3.29) |
By substituting the derivative of ˙s(t) obtained from Eq (3.27) into Eq (3.29), we may construct the following expression
˙V3=−s(Ξ(e)˙e)1/λ2−1[Ξ(e)1−1/λ2(ζ1|s|φ1sign(s)+ζ2|s|φ2sign(s))+1λ2Ξ(e)(−ˆψsign(s)+δ(t))]+1κ˜ψ˙ˆψ. | (3.30) |
Based on the findings presented in (3.26) and the condition (3.3), it is possible to represent (3.30) as
˙V3≤−˙e1/λ2−1(ζ1|s|φ1+1+ζ2|s|φ2+1)−1λ2(Ξ(e)˙e)1/λ2−1Ξ(e)˜ψ|s|+1κ˜ψ˙ˆψ≤−˙e1/λ2−1[ζ1|s|φ1+1+ζ2|s|φ2+1]. | (3.31) |
The above equation can be represented as
˙V3≤−˙e1/λ2−1[ζ1(2(V3−12κ˜ψ2))φ1+12+ζ2(2(V3−12κ˜ψ2))φ2+12]=−˙e1/λ2−1[ζ12φ1+12(1−12κV3˜ψ2)φ1+12V3φ1+12+ζ22φ2+12(1−12κV3˜ψ2)φ2+12V3φ2+12]. | (3.32) |
Then (3.32) can be expressed as
˙V3≤−˙e1/λ2−1[L1V3φ1+12+L2V3φ2+12], | (3.33) |
where
L1=ζ12φ1+12(1−12κV3˜ψ2)φ1+12, |
L2=ζ22φ2+12(1−12κV3˜ψ2)φ2+12. |
The sliding surface will reach in fixed-time Ts2. The settling time Ts2 is calculated using Lemma 1 as
Ts2≤1L1(φ1+12−1)+1L2(1−φ2+12). |
the equation for the overall settling time can be determined as T2=Te+Ts2. Therefore, the nonlinear system is employed to accurately control states and maintain the stability of the system in a fixed time. Moreover, a thorough examination of stability analyses is conducted.
Remark 1. By applying the proposed NAFxSMC method, which incorporates the fixed-time sliding surface (3.4), adaptive SMC scheme (3.24), and adaptive rule (3.26), to the dynamics of the nonlinear system (3.1), it is formulated that the convergence of tracking error within a fixed-time interval is obtained. The complete designed model is shown in Figure 1. The reason for this is that the strategy being given utilizes a technique of adaptive fixed-time sliding mode control in order to attain enhanced performance. The findings of the computational model will be provided in the subsequent section. Lemma 1 implies that the selection of the parameters as ζi and ηi can significantly influence the fixed-time, as specified by T2. When assigning significant values to these parameters, the rate of convergence will be enhanced accordingly.
To evaluate the simulation efficacy and validate the proposed NAFxSMC technique, two applications are employed to execute the nonlinear system in the presence of disturbances. The provided simulations aim to show the efficacy of the NAFxSMC under unknown disturbance. To validate the performance of the proposed scheme, two examples are given, and the consequences of these investigations are elucidated through the utilization of MATLAB/Simulink simulations.
To validate the efficacy of the proposed scheme, the NAFxSMC has been applied to the nonlinear model given as [16]:
˙x=δ(t)+u(t). | (4.1) |
The main objective of the proposed control is to ensure that the trajectories of the system align with the desired signal xd=sin(t). The proposed control parameters are chosen as: η1=100,η2=30,ζ1=300,ζ2=100,λ1=1.95,λ2=0.9,φ1=1.5 and φ2=0.7. Then, the initial conditions of states are given as x1(0)=0.1 and x2(0)=0. Moreover, the parameter of adaptive law is κ=1, its initial value is ψ(0)=0.3 and the disturbance is δ(t)=30sin(t)+15sin(10t). The states' tracking is given in Figures 2 and 3, tracking errors e and ˙e are depicted in Figures 4 and 5, control input applied to the system is shown in Figure 6, and adaptive estimation of the unknown dynamics is depicted in Figure 7.
From Figures 2–5, it is shown that the proposed scheme provides high trajectory tracking and small error with fast convergence. Moreover, the performance of the control input is given in Figure 6, and it clearly seems that the suggested scheme gives less chatter in the control input.
The description encompasses detailed information regarding the nonlinear model and its parameters, external disturbance, and desired trajectory. Therefore, the subsequent second-order nonlinear dynamical system is expressed as [53]:
˙x1=x2,˙x2=(3+cos(x1))θ−x21−1.5x2+2sin(x2)+(3+cos(x1))u(t)+δ(t), | (4.2) |
where the parameters are given as θ = 2. The unknown external disturbance is represented by the δ(t) and gaussian noise is used as a disturbance. And the control parameters are selected as follows:
η1=100,η2=30,ζ1=300, |
ζ2=100,λ1=1.95,λ2=0.9,φ1=1.5,φ2=0.7. |
Furthermore, the initial values of states are defined as x1(0)=0.5 and x2(0)=0. The primary aim of control is to ensure that the trajectories of the system align with the designated reference signal
xd=0.6e−4t−1.4e−t+1.45. |
Moreover, the parameter of the adaptive law is κ=1 and the initial value is ψ(0)=2.
Now a thorough comparison between the proposed NAFxSMC and FTSMC is conducted [35]; therefore, the parameters are appropriately modified. Thus, upon analyzing Figures 8–10, it becomes evident that the proposed scheme exhibits better tracking, reduces state error, and achieves a faster convergence time. Additionally, the control performance is illustrated in Figure 11, which clearly demonstrates that the proposed method produces a mitigated chattering problem in the control input.
In order to illustrate the benefits of the proposed robust adaptive fixed-time SMC scheme under unknown external disturbance, a comparison is made between its findings and those obtained from the fast finite-time sliding mode controller [35]. Given that the focus of this study pertains to the development of a robust controller for a second-order nonlinear system, it is appropriate to conduct a comparative analysis to establish the superiority of the suggested methodology over the findings presented in [35].
The graphs presented in Figures 12–14 illustrate x1, x2, and tracking error e. The attainment of satisfactory tracking performance is clearly seen. Both controllers have the capability to rapidly minimize the tracking error to zero. However, the controller described in [35] is unable to achieve exact tracking, and it is seen that the suggested law results in rapid convergence. The control input is depicted in Figure 15, and the suggested control law exhibits both smoothness and acceptable tracking performance, effectively mitigating external disturbance.
Furthermore, the control law given in this study requires less effort in comparison to the control law presented in the work of [35]. The compensation of the unknown external disturbance with the nonlinear system may be noticed in Figure 16. It is noticed that the proposed adaptive parameter shows that the drifting issue does not affect it, and the comparative scheme is influenced by the drifting problem. Hence, the simulation provides a clear demonstration that supports the theoretical analysis.
The simulated outcomes of the suggested NAFxSMC approach have been demonstrated. This part now provides a brief analysis of the constraints imposed by the suggested controller. The present study engages in a comprehensive examination of the constraints associated with the suggested controller gain amounts and stability proofs. In addition, this theoretical discussion will explore potential applications of the proposed methodology to a nonlinear system. Therefore, two nonlinear systems have been employed to assess the efficacy of the proposed scheme. Both provided examples demonstrate the validation of the proposed NAFxSMC, and the analysis also indicates superior performance to the compared scheme.
The suitable parameters of the suggested method are those that have been selected in accordance with the given range as η1>0, η2>0, ζ1>0, ζ2>0, 0<λ2<1, λ1>1, 0<φ2<1, and φ1>1. In this case, the suggested strategy is going to stay unaltered, but the closed-loop stability of the system could be gone if these conditions are ignored. Based on T1 and T2, it becomes evident that there is inverse relation between ηi and T1, ζi and T2, and the direct relationship between ηi,ζi and u(t). In order to accomplish both convergence of error within a fixed time and closed-loop stability, it is required to appropriately modify the values of ηi and ζi. Therefore, these values will serve as the deciding factor in establishing the stability of the system. Given the availability of information regarding the specific ranges within which each individual parameter falls, it becomes feasible to select a suitable value to a certain degree. This facilitates the process of selecting an appropriate value.
An NAFxSMC has been designed to obtain the enhanced tracking performance of a nonlinear system in the existence of an external disturbance. The utilization of the designed adaptive scheme is necessary for the capability to compensate for the unknown bound of system dynamics. And the FxSMC approach exhibits convergence within a fixed time and achieves satisfactory tracking performance. To show the efficacy of the devised method, we employed two applications of a nonlinear system and showed how it may be controlled using NAFxSMC, which has unknown dynamics. The findings suggest that the NAFxSMC strategy, when compared to the FTSMC method, demonstrates superior performance in terms of faster response, lesser tracking error, and enhanced ability to control unknown dynamics. For further studies, the non-smooth nonlinearities can be incorporated into the field of research on nonlinear systems. This suggested development would represent a substantial increase in the scope of the original effort.
The authors declare they have not used Artificial Intelligence (AI) tools in the creation of this article.
The authors would like to thank Prince Sultan University, Riyadh, Saudi Arabia for supporting APC of this work. Special acknowledgment to Automated Systems & Soft Computing Lab (ASSCL), Prince Sultan University, Riyadh, Saudi Arabia.
All authors declare no conflicts of interest in this paper.
[1] |
W. Chen, Disturbance observer based control for nonlinear systems, IEEE/ASME Trans. Mech., 9 (2004), 706–710. https://doi.org/10.1109/TMECH.2004.839034 doi: 10.1109/TMECH.2004.839034
![]() |
[2] |
H. Tajadodi, A. Khan, J. Francisco Gómez-Aguilar, H. Khan, Optimal control problems with atangana-baleanu fractional derivative, Optim. Contr. Appl. Met., 42 (2021), 96–109. https://doi.org/10.1002/oca.2664 doi: 10.1002/oca.2664
![]() |
[3] |
S. Mobayen, K. A. Alattas, A. Fekih, F. F. M. El-Sousy, M. Bakouri, Barrier function-based adaptive nonsingular sliding mode control of disturbed nonlinear systems: a linear matrix inequality approach, Chaos Soliton. Fract., 157 (2022), 111918. https://doi.org/10.1016/j.chaos.2022.111918 doi: 10.1016/j.chaos.2022.111918
![]() |
[4] |
D. Tong, C. Xu, Q. Chen, W. Zhou, Sliding mode control of a class of nonlinear systems, J. Franklin Inst., 357 (2020), 1560–1581. https://doi.org/10.1016/j.jfranklin.2019.11.004 doi: 10.1016/j.jfranklin.2019.11.004
![]() |
[5] |
B. B. Musmade, B. M. Patre, Robust sliding mode control of uncertain nonlinear systems with chattering alleviating scheme, Int. J. Mod. Phys. B, 35 (2021), 2140042. https://doi.org/10.1142/S0217979221400427 doi: 10.1142/S0217979221400427
![]() |
[6] |
S. Singh, A. T. Azar, A. Ouannas, Q. Zhu, W. Zhang, J. Na, Sliding mode control technique for multi-switching synchronization of chaotic systems, 2017 9th International Conference on Modelling, Identification and Control (ICMIC), 2017,880–885. https://doi.org/10.1109/ICMIC.2017.8321579 doi: 10.1109/ICMIC.2017.8321579
![]() |
[7] |
N. Cheng, L. Guan, L. Wang, J. Han, Chattering reduction of sliding mode control by adopting nonlinear saturation function, Adv. Mater. Res., 143-144 (2011), 53–61. https://doi.org/10.4028/www.scientific.net/AMR.143-144.53 doi: 10.4028/www.scientific.net/AMR.143-144.53
![]() |
[8] |
P. V. Suryawanshi, P. D. Shendge, S. B. Phadke, A boundary layer sliding mode control design for chatter reduction using uncertainty and disturbance estimator, Int. J. Dynam. Control, 4 (2016), 456–465. https://doi.org/10.1007/s40435-015-0150-9 doi: 10.1007/s40435-015-0150-9
![]() |
[9] |
S. Ahmed, A. T. Azar, Adaptive fractional tracking control of robotic manipulator using fixed-time method, Complex Intell. Syst., 10 (2023), 369–382. https://doi.org/10.1007/s40747-023-01164-7 doi: 10.1007/s40747-023-01164-7
![]() |
[10] |
Y. Feng, X. Yu, Z. Man, Non-singular terminal sliding mode control of rigid manipulators, Automatica, 38 (2002), 2159–2167. https://doi.org/10.1016/S0005-1098(02)00147-4 doi: 10.1016/S0005-1098(02)00147-4
![]() |
[11] |
L. Yang, J. Yang, Nonsingular fast terminal sliding-mode control for nonlinear dynamical systems, Int. J. Robust Nonlinear Control, 21 (2011), 1865–1879. https://doi.org/10.1002/rnc.1666 doi: 10.1002/rnc.1666
![]() |
[12] |
C. Ton, C. Petersen, Continuous fixed-time sliding mode control for spacecraft with flexible appendages, IFAC-PapersOnLine, 51 (2018), 1–5. https://doi.org/10.1016/j.ifacol.2018.07.079 doi: 10.1016/j.ifacol.2018.07.079
![]() |
[13] |
Z. Hou, P. Lu, Z. Tu, Nonsingular terminal sliding mode control for a quadrotor UAV with a total rotor failure, Aerosp. Sci. Technol., 98 (2020), 105716. https://doi.org/10.1016/j.ast.2020.105716 doi: 10.1016/j.ast.2020.105716
![]() |
[14] |
X. Zhao, Y. Gong, H. Jin, C. Xu, Adaptive super-twisting-based nonsingular fast terminal sliding mode control of permanent magnet linear synchronous motor, Trans. Inst. Meas. Control, 45 (2023), 3057–3066. https://doi.org/10.1177/01423312231162782 doi: 10.1177/01423312231162782
![]() |
[15] |
H. Khan, S. Ahmed, J. Alzabut, A. T. Azar, A generalized coupled system of fractional differential equations with application to finite time sliding mode control for Leukemia therapy, Chaos Soliton. Fract., 174 (2023), 113901. https://doi.org/10.1016/j.chaos.2023.113901 doi: 10.1016/j.chaos.2023.113901
![]() |
[16] | J. Mishra, Finite-time sliding mode control strategies and their applications, Ph.D. Thesis, RMIT University, 2019. |
[17] |
G. Tao, Multivariable adaptive control: a survey, Automatica, 50 (2014), 2737–2764. https://doi.org/10.1016/j.automatica.2014.10.015 doi: 10.1016/j.automatica.2014.10.015
![]() |
[18] | E. Lavretsky, K. A. Wise, Robust adaptive control, In: Robust and adaptive control: with aerospace applications, London: Springer, 2012,317–353. https://doi.org/10.1007/978-1-4471-4396-3_11 |
[19] | M. A. Attia, A. Y. Abdelaziz, K. Sarita, A. S. S. Vardhan, A. S. S. Vardhan, S. Singh, et al., AVR performance enhancement by using adaptive PI controller, In: A. P. Pandian, R. Palanisamy, M. Narayanan, T. Senjyu, Proceedings of Third International Conference on Intelligent Computing, Information and Control Systems, Singapore: Springer, 1415 (2022), 249–260. https://doi.org/10.1007/978-981-16-7330-6_19 |
[20] |
J. M. R. Chintu, R. K. Sahu, S. Panda, Adaptive differential evolution tuned hybrid fuzzy PD-PI controller for automatic generation control of power systems, Int. J. Ambient Energy, 43 (2022), 515–530. https://doi.org/10.1080/01430750.2019.1653986 doi: 10.1080/01430750.2019.1653986
![]() |
[21] |
M. Y. Silaa, O. Barambones, A. Bencherif, A novel adaptive PID controller design for a PEM fuel cell using stochastic gradient descent with momentum enhanced by whale optimizer, Electronics, 11 (2022), 2610. https://doi.org/10.3390/electronics11162610 doi: 10.3390/electronics11162610
![]() |
[22] | T. S. Gorripotu, H. Samalla, C. J. M. Rao, A. T. Azar, D. Pelusi, TLBO algorithm optimized fractional-order PID controller for AGC of interconnected power system, In: J. Nayak, A. Abraham, B. Krishna, G. Chandra Sekhar, A. Das, Soft computing in data analytics, Proceedings of International Conference on SCDA 2018, Singapore: Springer, 758 (2019), 847–855. https://doi.org/10.1007/978-981-13-0514-6_80 |
[23] |
H. Yin, B. Meng, Z. Wang, Disturbance observer-based adaptive sliding mode synchronization control for uncertain chaotic systems, AIMS Math., 8 (2023), 23655–23673. https://doi.org/10.3934/math.20231203 doi: 10.3934/math.20231203
![]() |
[24] |
Z. Liu, O. Zhang, Y. Gao, Y. Zhao, Y. Sun, J. Liu, Adaptive neural network-based fixed-time control for trajectory tracking of robotic systems, IEEE Trans. Circuits Syst. II, 70 (2022), 241–245. https://doi.org/10.1109/TCSII.2022.3194917 doi: 10.1109/TCSII.2022.3194917
![]() |
[25] |
S. Ahmed, Robust model reference adaptive control for five-link robotic exoskeleton, Int. J. Model. Identif. Control, 39 (2021), 324–331. https://doi.org/10.1504/IJMIC.2021.123799 doi: 10.1504/IJMIC.2021.123799
![]() |
[26] |
B. Meghni, D. Dib, A. T. Azar, A. Saadoun, Effective supervisory controller to extend optimal energy management in hybrid wind turbine under energy and reliability constraints, Int. J. Dynam. Control, 6 (2018), 369–383. https://doi.org/10.1007/s40435-016-0296-0 doi: 10.1007/s40435-016-0296-0
![]() |
[27] | B. Meghni, D. Dib, A. T. Azar, S. Ghoudelbourk, A. Saadoun, Robust adaptive supervisory fractional order controller for optimal energy management in wind turbine with battery storage, In: A. Azar, S. Vaidyanathan, A. Ouannas, Fractional order control and synchronization of chaotic systems, Cham: Springer, 688 (2017), 165–202. https://doi.org/10.1007/978-3-319-50249-6_6 |
[28] |
Y. Wang, B. Li, F. Yan, B, Chen, Practical adaptive fractional-order nonsingular terminal sliding mode control for a cable-driven manipulator, Int. J. Robust Nonlinear Control, 29 (2019), 1396–1417. https://doi.org/10.1002/rnc.4441 doi: 10.1002/rnc.4441
![]() |
[29] |
X. Zhang, J. Wu, X. Zhan, T. Han, H. Yan, Observer-based adaptive time-varying formation-containment tracking for multiagent system with bounded unknown input, IEEE Trans. Syst. Man Cybern., 53 (2022), 1479–1491. https://doi.org/10.1109/TSMC.2022.3199410 doi: 10.1109/TSMC.2022.3199410
![]() |
[30] |
Y. Zhao, F. Tang, G. Zong, X. Zhao, N. Xu, Event-based adaptive containment control for nonlinear multiagent systems with periodic disturbances, IEEE Trans. Circuits Syst. II, 69 (2022), 5049–5053. https://doi.org/10.1109/TCSII.2022.3200053 doi: 10.1109/TCSII.2022.3200053
![]() |
[31] |
C. Li, Y. Wang, X. Yang, Adaptive fuzzy control of a quadrotor using disturbance observer, Aerosp. Sci. Technol., 128 (2022), 107784. https://doi.org/10.1016/j.ast.2022.107784 doi: 10.1016/j.ast.2022.107784
![]() |
[32] |
M. Naeem, A. Khan, S. Ashraf, S. Abdullah, M. Ayaz, N. Ghanmi, A novel decision making technique based on spherical hesitant fuzzy Yager aggregation information: application to treat Parkinson's disease, AIMS Math., 7 (2022), 1678–1706. https://doi.org/10.3934/math.2022097 doi: 10.3934/math.2022097
![]() |
[33] |
Z. Zhao, X. Jin, X. Wu, H. Wang, J. Chi, Neural network-based fixed-time sliding mode control for a class of nonlinear Euler-Lagrange systems, Appl. Math. Comput., 415 (2022), 126718. https://doi.org/10.1016/j.amc.2021.126718 doi: 10.1016/j.amc.2021.126718
![]() |
[34] |
A. Khan, T. Abdeljawad, M. Alqudah, Neural networking study of worms in a wireless sensor model in the sense of fractal fractional, AIMS Math., 8 (2023), 26406–26424. https://doi.org/10.3934/math.20231348 doi: 10.3934/math.20231348
![]() |
[35] |
S. Ahmed, H. Wang, Y. Tian, Fault tolerant control using fractional-order terminal sliding mode control for robotic manipulators, Stud. Inform. Control, 27 (2018), 55–64. https://doi.org/10.24846/V27I1Y201806 doi: 10.24846/V27I1Y201806
![]() |
[36] |
J. Zhai, Z. Li, Fast-exponential sliding mode control of robotic manipulator with super-twisting method, IEEE Trans. Circuits Syst. II, 69 (2021), 489–493. https://doi.org/10.1109/TCSII.2021.3081147 doi: 10.1109/TCSII.2021.3081147
![]() |
[37] |
S. Ahmed, A. T. Azar, M. Tounsi, I. K. Ibraheem, Adaptive control design for Euler-Lagrange systems using fixed-time fractional integral sliding mode scheme, Fractal Fract., 7 (2023), 712. https://doi.org/10.3390/fractalfract7100712 doi: 10.3390/fractalfract7100712
![]() |
[38] |
J. Ni, L. Liu, C. Liu, X. Hu, Fractional order fixed-time nonsingular terminal sliding mode synchronization and control of fractional order chaotic systems, Nonlinear Dyn., 89 (2017), 2065–2083. https://doi.org/10.1007/s11071-017-3570-6 doi: 10.1007/s11071-017-3570-6
![]() |
[39] |
M. Gao, L. Ding, X. Jin, ELM-based adaptive faster fixed-time control of robotic manipulator systems, IEEE Trans. Neur. Net. Lear. Syst., 34 (2021), 4646–4658. https://doi.org/10.1109/TNNLS.2021.3116958 doi: 10.1109/TNNLS.2021.3116958
![]() |
[40] |
X. Lin, C. Wu, W. Yao, Z. Liu, X. Shen, R. Xu, et al., Observer-based fixed-time control for permanent-magnet synchronous motors with parameter uncertainties, IEEE Trans. Power Electron., 38 (2022), 4335–4344. https://doi.org/10.1109/TPEL.2022.3226033 doi: 10.1109/TPEL.2022.3226033
![]() |
[41] |
Z. Liu, Y. Zhao, O. Zhang, W. Chen, J. Wang, Y. Gao, et al., A novel faster fixed-time adaptive control for robotic systems with input saturation, IEEE Trans. Ind. Electron., 71 (2023), 5215–5223. https://doi.org/10.1109/TIE.2023.3281701 doi: 10.1109/TIE.2023.3281701
![]() |
[42] |
M. Labbadi, H. El Moussaoui, An improved adaptive fractional-order fast integral terminal sliding mode control for distributed quadrotor, Math. Comput. Simul., 188 (2021), 120–134. https://doi.org/10.1016/j.matcom.2021.03.039 doi: 10.1016/j.matcom.2021.03.039
![]() |
[43] |
Z. Anjum, H. Zhou, S. Ahmed, Y. Guo, Fixed time sliding mode control for disturbed robotic manipulator, J. Vib. Control, 2023. https://doi.org/10.1177/10775463231165094 doi: 10.1177/10775463231165094
![]() |
[44] |
J. Zhang, T. Chai, Proportional-integral funnel control of unknown lower-triangular nonlinear systems, IEEE Trans. Autom. Control, 2023, 1–7. https://doi.org/10.1109/TAC.2023.3330900 doi: 10.1109/TAC.2023.3330900
![]() |
[45] |
J. Zhang, T. Yang, T. Chai, Neural network control of underactuated surface vehicles with prescribed trajectory tracking performance, IEEE Trans. Neural Neural Learn. Syst., 2022, 1–14. https://doi.org/10.1109/TNNLS.2022.3223666 doi: 10.1109/TNNLS.2022.3223666
![]() |
[46] |
G. E. M. Abro, S. A. B. M. Zulkifli, V. S. Asirvadam, Z. A. Ali, Model-free-based single-dimension fuzzy SMC design for underactuated quadrotor UAV, Actuators, 10 (2021), 191. https://doi.org/10.3390/act10080191 doi: 10.3390/act10080191
![]() |
[47] |
X. Jin, W. Che, Z. Wu, H. Wang, Analog control circuit designs for a class of continuous-time adaptive fault-tolerant control systems, IEEE Trans. Cybern., 52 (2020), 4209–4220. https://doi.org/10.1109/TCYB.2020.3024913 doi: 10.1109/TCYB.2020.3024913
![]() |
[48] |
S. Ahmed, A. T. Azar, M. Tounsi, Adaptive fault tolerant non-singular sliding mode control for robotic manipulators based on fixed-time control law, Actuators, 11 (2022), 353. https://doi.org/10.3390/act11120353 doi: 10.3390/act11120353
![]() |
[49] |
Z. Han, K. Zhang, T. Yang, M. Zhang, Spacecraft fault-tolerant control using adaptive non-singular fast terminal sliding mode, IET Control Theory Appl., 10 (2016), 1991–1999. https://doi.org/10.1049/iet-cta.2016.0044 doi: 10.1049/iet-cta.2016.0044
![]() |
[50] | F. Leyla, Z. Khaled, S. Abdelkrim, B. I. Khalil, D. Mohamed, Adaptive sliding mode control vibrations of structures, In: C. Fischer, J. Náprstek, Vibration control of structures, IntechOpen, 2021. https://doi.org/10.5772/intechopen.98193 |
[51] |
J. Huang, G. Xie, S. Gao, F. Qian, Adaptive H∞ observer-based sliding mode control for uncertain fractional-order nonlinear systems, IEEJ Trans. Electr. Electron. Eng., 18 (2023), 1127–1135. https://doi.org/10.1002/tee.23811 doi: 10.1002/tee.23811
![]() |
[52] |
R. M. Arasi, S. Padma, Adaptive nonlinear sliding mode control for DC power distribution in commercial buildings, Intell. Autom. Soft Comput., 36 (2023), 997–1012. https://doi.org/10.32604/iasc.2023.032645 doi: 10.32604/iasc.2023.032645
![]() |
[53] |
M. Golestani, S. Mobayen, H. Richter, Fast robust adaptive tracker for uncertain nonlinear second-order systems with time-varying uncertainties and unknown parameters, Int. J. Adapt. Control Signal Process., 32 (2018), 1764–1781. https://doi.org/10.1002/acs.2943 doi: 10.1002/acs.2943
![]() |
1. | Omayma Mansouri, Ali Ben Brahim, Fayçal Ben Hmida, Anis Sellami, Sliding Mode Fault-Tolerant Control for Nonlinear LPV Systems with Variable Time-Delay, 2024, 29, 2297-8747, 96, 10.3390/mca29060096 | |
2. | Daniel Centeno-Barreda, Sergio Salazar-Cruz, Ricardo López-Gutiérrez, Yukio Rosales-Luengas, Rogelio Lozano, Lower limb exoskeleton for gait rehabilitation with adaptive nonsingular sliding mode control, 2024, 0263-5747, 1, 10.1017/S0263574724001668 | |
3. | Saim Ahmed, Ahmad Taher Azar, Haoping Wang, Jun Ma, Adaptive fixed-time TSM for uncertain nonlinear dynamical system under unknown disturbance, 2024, 19, 1932-6203, e0304448, 10.1371/journal.pone.0304448 | |
4. | Chong Li, Jianwei Xia, Jing Zhang, Hao Shen, Event-triggered adaptive finite-time synchronization control for dual-motor servo systems with uncertainties and torque disturbance, 2024, 0924-090X, 10.1007/s11071-024-10618-7 | |
5. | Xin Xiong, Changzhuang Chen, Bing Zhu, Yaming Liu, Zhihong Li, Fei Xu, Research on robust backstepping sliding mode control strategy for magnetorheological semi-active suspension based on quantized input signals, 2024, 0954-4062, 10.1177/09544062241286301 | |
6. | Abdelatif Boutiara, Jehad Alzabut, Hasib Khan, Saim Ahmed, Ahmad Taher Azar, Qualitative analytical results of complex order nonlinear fractional differential equations with robust control scheme, 2024, 9, 2473-6988, 20692, 10.3934/math.20241006 | |
7. | Xuejuan Shao, Zhentao Tao, Zhimei Chen, Jinggang Zhang, Zhicheng Zhao, Liangliang Zhou, Positioning anti-sway control method for tower crane based on improved MFAC, 2024, 1077-5463, 10.1177/10775463241292118 | |
8. | Saim Ahmed, Ahmad Taher Azar, Ibraheem Kasim Ibraheem, Model-free scheme using time delay estimation with fixed-time FSMC for the nonlinear robot dynamics, 2024, 9, 2473-6988, 9989, 10.3934/math.2024489 | |
9. | Laohu Yuan, Jinxin Zheng, Xiaoguang Wang, Le Ma, Attitude Control of a Mass-Actuated Fixed-Wing UAV Based on Adaptive Global Fast Terminal Sliding Mode Control, 2024, 8, 2504-446X, 305, 10.3390/drones8070305 | |
10. | Majid Roohi, Saeed Mirzajani, Ahmad Reza Haghighi, Andreas Basse-O'Connor, Robust stabilization of fractional-order hybrid optical system using a single-input TS-fuzzy sliding mode control strategy with input nonlinearities, 2024, 9, 2473-6988, 25879, 10.3934/math.20241264 | |
11. | Wei Chen, Zekai Wang, Zebin Zhou, Optimized interval type-2 fuzzy global sliding mode control for quadrotor robot, 2025, 0025-6455, 10.1007/s11012-024-01922-y | |
12. | Saim Ahmed, Ahmad Taher Azar, Adaptive PD sliding mode control for robot dynamics using predefined-time approach, 2025, 13, 2195-268X, 10.1007/s40435-025-01603-y | |
13. | Van-Cuong Nguyen, Seong Han Kim, A novel fixed-time prescribed performance sliding mode control for uncertain wheeled mobile robots, 2025, 15, 2045-2322, 10.1038/s41598-025-89126-6 | |
14. | Taoyu Wang, Shiyan Sun, Qijie Chen, Non-singular terminal super-twitsing control of servo systems with backlash, 2025, 15, 2045-2322, 10.1038/s41598-025-88795-7 | |
15. | Junqi Wu, Hao Xiong, Zhenhua Pan, Hongbin Deng, Fractional-order finite-time attitude control for underactuated quadrotors with dynamic event-triggered mechanism, 2025, 0924-090X, 10.1007/s11071-025-10973-z | |
16. | Shunjing Hu, Yi Wan, Xichang Liang, Adaptive nonsingular fast terminal sliding mode trajectory tracking control for robotic manipulators with model feedforward compensation, 2025, 0924-090X, 10.1007/s11071-025-11008-3 | |
17. | Taha Etem, 2025, The Bagged Tree Ensemble Model for Predicting Steel Industry Energy Consumption Profile, 979-8-3315-3969-6, 43, 10.1109/CDMA61895.2025.00013 |