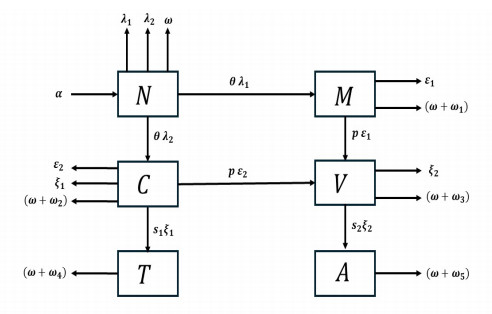
The relationship between cancer and the severe acute respiratory syndrome coronavirus 2 (SARS-CoV-2) infection is controversial. While SARS-CoV-2 can worsen the status of a cancer patient, many remission cases after SARS-CoV-2 infection have been recorded. It has been suggested that SARS-CoV-2 could have oncolytic properties, which needs further investigations. Mathematical modeling is a powerful tool that can significantly enhance experimental and medical studies. Our objective was to propose and analyze a mathematical model for oncolytic SARS-CoV-2 with immunity. The basic properties of this model, including existence, uniqueness, nonnegativity, and boundedness of the solutions, were confirmed. The equilibrium points were computed, and their existence conditions were determined. The global stability of the equilibria was proven using the Lyapunov theory. Numerical simulations were implemented to validate the theoretical results. It was found that the model has thirteen equilibrium points that reflect different infection states. Based on the model's results, the infection of cancer cells by SARS-CoV-2 can lead to a reduction in the concentration of cancer cells. Additionally, the induction of cytotoxic T lymphocytes (CTLs) decreases the number of cancer cells, potentially resulting in cancer remission or an improvement in the overall health of cancer patients. This theoretical result aligns with numerous studies highlighting the oncolytic role of SARS-CoV-2. In addition, given the limited availability of real data, further studies are essential to better comprehend the role of immune responses and their impact on the oncolytic role of SARS-CoV-2.
Citation: Afnan Al Agha, Hakim Al Garalleh. Oncolysis by SARS-CoV-2: modeling and analysis[J]. AIMS Mathematics, 2024, 9(3): 7212-7252. doi: 10.3934/math.2024351
[1] | Ahmed M. Elaiw, Amani S. Alsulami, Aatef D. Hobiny . Global properties of delayed models for SARS-CoV-2 infection mediated by ACE2 receptor with humoral immunity. AIMS Mathematics, 2024, 9(1): 1046-1087. doi: 10.3934/math.2024052 |
[2] | A. M. Elaiw, A. S. Shflot, A. D. Hobiny . Stability analysis of SARS-CoV-2/HTLV-I coinfection dynamics model. AIMS Mathematics, 2023, 8(3): 6136-6166. doi: 10.3934/math.2023310 |
[3] | Tahir Khan, Fathalla A. Rihan, Muhammad Bilal Riaz, Mohamed Altanji, Abdullah A. Zaagan, Hijaz Ahmad . Stochastic epidemic model for the dynamics of novel coronavirus transmission. AIMS Mathematics, 2024, 9(5): 12433-12457. doi: 10.3934/math.2024608 |
[4] | S. M. E. K. Chowdhury, J. T. Chowdhury, Shams Forruque Ahmed, Praveen Agarwal, Irfan Anjum Badruddin, Sarfaraz Kamangar . Mathematical modelling of COVID-19 disease dynamics: Interaction between immune system and SARS-CoV-2 within host. AIMS Mathematics, 2022, 7(2): 2618-2633. doi: 10.3934/math.2022147 |
[5] | Maryam Amin, Muhammad Farman, Ali Akgül, Mohammad Partohaghighi, Fahd Jarad . Computational analysis of COVID-19 model outbreak with singular and nonlocal operator. AIMS Mathematics, 2022, 7(9): 16741-16759. doi: 10.3934/math.2022919 |
[6] | Sara J Hamis, Fiona R Macfarlane . A single-cell mathematical model of SARS-CoV-2 induced pyroptosis and the effects of anti-inflammatory intervention. AIMS Mathematics, 2021, 6(6): 6050-6086. doi: 10.3934/math.2021356 |
[7] | Kai Zhang, Xinzhu Meng, Abdullah Khames Alzahrani . The ACE2 receptor protein-mediated SARS-CoV-2 infection: dynamic properties of a novel delayed stochastic system. AIMS Mathematics, 2024, 9(4): 8104-8133. doi: 10.3934/math.2024394 |
[8] | Murugesan Sivashankar, Sriramulu Sabarinathan, Vediyappan Govindan, Unai Fernandez-Gamiz, Samad Noeiaghdam . Stability analysis of COVID-19 outbreak using Caputo-Fabrizio fractional differential equation. AIMS Mathematics, 2023, 8(2): 2720-2735. doi: 10.3934/math.2023143 |
[9] | Oluwaseun F. Egbelowo, Justin B. Munyakazi, Manh Tuan Hoang . Mathematical study of transmission dynamics of SARS-CoV-2 with waning immunity. AIMS Mathematics, 2022, 7(9): 15917-15938. doi: 10.3934/math.2022871 |
[10] | C. W. Chukwu, Fatmawati . Modelling fractional-order dynamics of COVID-19 with environmental transmission and vaccination: A case study of Indonesia. AIMS Mathematics, 2022, 7(3): 4416-4438. doi: 10.3934/math.2022246 |
The relationship between cancer and the severe acute respiratory syndrome coronavirus 2 (SARS-CoV-2) infection is controversial. While SARS-CoV-2 can worsen the status of a cancer patient, many remission cases after SARS-CoV-2 infection have been recorded. It has been suggested that SARS-CoV-2 could have oncolytic properties, which needs further investigations. Mathematical modeling is a powerful tool that can significantly enhance experimental and medical studies. Our objective was to propose and analyze a mathematical model for oncolytic SARS-CoV-2 with immunity. The basic properties of this model, including existence, uniqueness, nonnegativity, and boundedness of the solutions, were confirmed. The equilibrium points were computed, and their existence conditions were determined. The global stability of the equilibria was proven using the Lyapunov theory. Numerical simulations were implemented to validate the theoretical results. It was found that the model has thirteen equilibrium points that reflect different infection states. Based on the model's results, the infection of cancer cells by SARS-CoV-2 can lead to a reduction in the concentration of cancer cells. Additionally, the induction of cytotoxic T lymphocytes (CTLs) decreases the number of cancer cells, potentially resulting in cancer remission or an improvement in the overall health of cancer patients. This theoretical result aligns with numerous studies highlighting the oncolytic role of SARS-CoV-2. In addition, given the limited availability of real data, further studies are essential to better comprehend the role of immune responses and their impact on the oncolytic role of SARS-CoV-2.
N: nutrient, it is produced from a source at a fixed rate, cells grow as a result of consuming nutrient; M: epithelial cells, the type of cells in the lungs that are infected by SARS-CoV-2; C cancer cells: the cells characterized by uncontrolled growth that become infected by SARS-CoV-2; V: the free SARS-CoV-2 particles, the virus responsible for COVID-19, these particles infect epithelial and cancer cells; T: anti-cancer CTLs, the immune cells that specifically target and eliminate cancer cells; A: antibodies, they are used by the immune system to eliminate virus particles from the body
COVID-19 is a disease caused by severe acute respiratory syndrome coronavirus 2 (SARS-CoV-2). While there has been a decline in reported cases and deaths globally, SARS-CoV-2 is still spreading in many countries [1]. SARS-CoV-2 passes into host cells by way of a transmembrane protein known as the angiotensin-converting enzyme 2 receptor [2]. It principally causes infection in alveolar epithelial type-II cells of the lungs [3,4]. However, other organs can be infected by SARS-CoV-2. The impact of COVID-19 on cancer patients has opened up a wide field of research. This group of patients is vulnerable to COVID-19 due to weakened immune system or ongoing anti-cancer treatments [5]. The question of whether SARS-CoV-2 induces remission in cancer patients or exacerbates the severity of the disease remains controversial [5,6].
The interconnection between cancer and viruses has become one of the most important topics in oncology and virology [7]. Some viruses, called oncolytic viruses (OVs), have the ability to infect cancer cells. These viruses can be found in nature or genetically modified to replicate in cancer cells without infecting normal cells [5,8]. OVs kill cancer cells after massive replication inside them and by inducing a specific antitumor immune response [5,8]. Examples of OVs include adenovirus, vaccinia virus, Coxsackievirus, and herpes simplex virus [8,9]. Talimogene laherparepvec is the only approved oncolytic virotherapy [6,10]. Talimogene laherparepvec is an engineered herpes virus used to treat advanced melanoma through immediate injection into the tumor [10].
The impact of COVID-19 on cancer patients is bidirectional. It has been indicated that SARS-CoV-2 infection can enhance cancer progression [5,7,8,11]. On the other hand, cancer remission after SARS-CoV-2 infection has been reported in many patients [6,12,13,14]. For example, Pasin et al. [15] reported the case of a patient with refractory natural killer (NK)/T-cell lymphoma who experienced a transient remission during SARS-CoV-2 infection. As angiotensin-converting enzyme 2 is expressed in NK cells, the authors in [15] proposed that SARS-CoV-2 could own some oncolytic properties. Challenor and Tucker [16] presented the case of a remission in a patient with classical Hodgkin lymphoma after SARS-CoV-2 infection. Another case was reported by Sollini et al. [17] involving a patient with follicular lymphoma who achieved full remission after SARS-CoV-2 infection. The authors in [16,17] supposed that the infection stimulated an antitumor immune response. Kandeel et al. [18] reported the remission of two cases with acute leukemia. Antwi-Amoabeng et al. [19] presented a case in which a patient with multiple myeloma had remission following SARS-CoV-2 infection. The patient received a single dose of chemotherapy. However, the authors mentioned that the remission in this case was parallel to the remission in patients who got four doses of chemotherapy. Ohadi et al. [20] reported a case of mycosis fungoides that went into remission after the coronavirus infection. Other cases of remission were reported in [6].
The above remission cases suggest that SARS-CoV-2 could have an oncolytic role in many types of cancer. It may infect and destroy cancer cells to expose the tumor-associated antigens. These antigens stimulate an immune response against cancer cells, leading to cancer remission [18]. Hence, there is an urgent need to understand the relationship between SARS-CoV-2 infection and cancer. This understanding may enable the engineering of SARS-CoV-2 for use as an efficient therapy against certain types of cancer. Mathematical modeling is a strong tool that is often employed to assist experiments and medical research [21,22]. Mathematical models have been used to understand the dynamics of many infectious diseases and test hypotheses that may be challenging to assess experimentally. The analysis of these models can offer predictions of outcomes and aid in identifying optimal treatment strategies.
Many mathematical models about SARS-CoV-2 [23,24,25], cancer [26], SARS-CoV-2/cancer [27], and oncolytic virotherapy [28,29,30,31,32] have been constructed and studied. However and to the best of our knowledge, no oncolytic SARS-CoV-2 models have been established yet. Such models are important to understand the effect of the infection of cancer cells by SARS-CoV-2 and the role of different immune responses during this coinfection. In this work, we propose an oncolytic SARS-CoV-2 virotherpy model. The construction of this model follows similar principles of those used in [33]. We conduct a comprehensive mathematical analysis of this model including assessments of boundedness, nonnegativity, and global stability of equilibrium points. In addition, we implement some numerical simulations.
This paper is structured as follows. Section 2 introduces the model under consideration. Section 3 demonstrates that all solutions are bounded and have zero or positive values. Furthermore, it computes the equilibrium solutions of the proposed model. Section 4 verifies the global properties of these solutions. Section 5 is dedicated to numerical simulations. The last section discusses the results and provides a glimpse of the future vision.
In formulating the model, we consider the following assumptions:
(i) The nutrient is produced from a source at a fixed rate, while it is depleted due to its consumption by epithelial cells and cancer cells. Additionally, depletion occurs as a result of natural death;
(ii) Epithelial cells proliferate as a result of nutrient consumption, and their numbers decrease due to either viral infection or natural death;
(iii) Cancer cells replicate as a result of nutrient utilization, and their numbers decline due to viral infection, attacks by cytotoxic T lymphocytes (CTLs), or natural cell death;
(iv) Free virus particles increase as a consequence of infecting epithelial cells and cancer cells, but they diminish due to the removal by antibodies or natural death;
(v) CTLs are stimulated by infected cancer cells, while antibodies are stimulated by free virus particles;
(vi) CTLs and antibodies undergo decay through natural processes;
(vii) The induction of CTLs by SARS-CoV-2 infection is implied in the stimulation rate of CTLs;
(viii) The model does not contain infected components for epithelial cells and cancer cells.
The proposed ordinary differential equation (ODE) model consists of six nonlinear equations and takes the form
{dN(t)dt=α−λ1N(t)M(t)−λ2N(t)C(t)−ωN(t),dM(t)dt=θλ1N(t)M(t)−ϵ1M(t)V(t)−(ω+ω1)M(t),dC(t)dt=θλ2N(t)C(t)−ϵ2C(t)V(t)−ξ1C(t)T(t)−(ω+ω2)C(t),dV(t)dt=pϵ1M(t)V(t)+pϵ2C(t)V(t)−ξ2V(t)A(t)−(ω+ω3)V(t),dT(t)dt=s1ξ1C(t)T(t)−(ω+ω4)T(t),dA(t)dt=s2ξ2V(t)A(t)−(ω+ω5)A(t), | (2.1) |
where N(t), M(t), C(t), V(t), T(t), and A(t) typify the concentrations of nutrient, epithelial cells, cancer cells, SARS-CoV-2 particles, anti-cancer CTLs, and antibodies. The nutrient is released from its source at rate α and declines at rate ωN. Epithelial cells expend nutrient at rate λ1NM, reproduce at rate θλ1NM, and get infected by SARS-CoV-2 at rate ϵ1MV. Cancer cells expend nutrient at rate λ2NC, grow at rate θλ2NC, and become infected at rate ϵ2CV. SARS-CoV-2 replicates as a result of infecting epithelial cells and cancer cells at rates pϵ1MV and pϵ2CV, respectively. CTLs kill cancer cells at rate ξ1CT and reproduce at rate s1ξ1CT. Antibodies eliminate SARS-CoV-2 at rate ξ2VA and get stimulated at rate s2ξ2VA. Epithelial cells, cancer cells, SARS-CoV-2 particles, CTLs, and antibodies die at natural rates ω1M, ω2C, ω3V, ω4T, and ω5A, respectively. The parameters in model (2.1) are postulated to take positive values. Figure 1 provides a schematic diagram of the model. For simplicity, we consider the following
a1≡ω+ω1, a2≡ω+ω2, a3≡ω+ω3, a4≡ω+ω4, a5≡ω+ω5. |
The following theorem demonstrates the existence and uniqueness of the solutions of model (2.1).
Theorem 1. Assume that the initial values (N0,M0,C0,V0,T0,A0)∈R6 are given. There exists t0>0 and continuously differentiable functions N,M,C,V,T,A: [0,t0)→R such that (N,M,C,V,T,A) satisfies model (2.1) and
(N(0),M(0),C(0),V(0),T(0),A(0))=(N0,M0,C0,V0,T0,A0). |
Proof. As the system of ODEs given in (2.1) is autonomous, it is enough to prove that the function f: R6→R6 defined by
f(z)=[α−λ1z1z2−λ2z1z3−ωz1θλ1z1z2−ϵ1z2z4−a1z2θλ2z1z3−ϵ2z3z4−ξ1z3z5−a2z3pϵ1z2z4+pϵ2z3z4−ξ2z4z6−a3z4s1ξ1z3z5−a4z5s2ξ2z4z6−a5z6] |
is locally Lipschitz in its z argument. We observe that the Jacobian matrix
∇f(z)=[−λ1z2−λ2z3−ω−λ1z1−λ2z1000θλ1z2θλ1z1−ϵ1z4−a10−ϵ1z200θλ2z30θλ2z1−ϵ2z4−ξ1z5−a2−ϵ2z3−ξ1z300pϵ1z4pϵ2z4pϵ1z2+pϵ2z3−ξ2z6−a30−ξ2z400s1ξ1z50s1ξ1z3−a40000s2ξ2z60s2ξ2z4−a5] |
is linear in z and consequently locally bounded for all z∈R6. Therefore, f has a continuous and bounded derivative on any compact subset of R6, and so f is locally Lipschitz in z. According to the classical Picard-Lindelöf theorem [34], there exists a unique solution z(t) to the ODE
dz(t)dt=f(z(t)) |
on the time interval [0,t0] for some t0>0 [35].
Next, we prove the nonnegativity and boundedness of the solutions of model (2.1).
Theorem 2. Let τi>0 (i=1,2,3,4,5), then the set
Ω={(N,M,C,V,T,A)∈R6+:0≤N(t)≤τ1,0≤M(t),C(t)≤τ2,0≤V(t)≤τ3,0≤T(t)≤τ4,0≤A(t)≤τ5} | (6) |
is positively invariant set for system (2.1).
Proof. For system (2.1), we obtain
dNdt|N=0=α>0,dMdt|M=0=0,dCdt|C=0=0,dVdt|V=0=0,dTdt|T=0=0,dAdt|A=0=0. |
This shows that
(N(t),M(t),C(t),V(t),T(t),A(t))∈R6+ |
for t≥0 whenever
(N(0),M(0),C(0),V(0),T(0),A(0))∈R6+. |
To prove the boundedness, we consider the function
χ(t)=N(t)+1θM(t)+1θC(t)+1θpV(t)+1θs1T(t)+1θps2A(t). |
By computing dχ(t)dt, we get
dχ(t)dt=α−ωN(t)−a1θM(t)−a2θC(t)−a3θpV(t)−a4θs1T(t)−a5θps2A(t)≤α−κ[N(t)+1θM(t)+1θC(t)+1θpV(t)+1θs1T(t)+1θps2A(t)]=α−κχ(t), |
where
κ=min{ω,a1,a2,a3,a4,a5}. |
This implicates that
0≤χ(t)≤τ1ifχ(0)≤τ1, for t≥0, |
where
τ1=ακ. |
Consequently, we have N(t)≤τ1, M(t)≤τ2, C(t)≤τ2, V(t)≤τ3, T(t)≤τ4, and A(t)≤τ5, where τ2=θτ1, τ3=θpτ1, τ4=θs1τ1, and τ5=θps2τ1. Thus, the set Ω is positively invariant [36].
Theorem 3. Model (2.1) has thirteen equilibrium points as follows:
(1) The trivial equilibrium E0 always exists;
(2) The uninfected-epithelial equilibrium E1 exists if R0>1;
(3) The uninfected-cancer equilibrium E2 exists if R1>1;
(4) The infected-epithelial equilibrium E3 exists if
R0>1+λ1a3ωpϵ1; |
(5) The infected-cancer equilibrium E4 exists if
R1>1+λ2a3ωpϵ2; |
(6) The uninfected-cancer equilibrium with CTLs E5 exists if
R1>1+λ2a4ωs1ξ1; |
(7) The infected epithelial-cancer equilibrium without immunity E6 exists if
R1+a1ϵ2a2ϵ1+a1λ2a3ωpϵ1a2<1+λ2a3ωpϵ2+αθλ1ϵ2ωϵ1a2, |
R0+a2ϵ1a1ϵ2+λ1a2a3ωpa1ϵ2<1+λ1a3ωpϵ1+αθϵ1λ2ωa1ϵ2, |
a1ϵ2a2ϵ1>1,λ1ϵ2λ2ϵ1>1,anda1λ2a2λ1>1; |
(8) The uninfected epithelial-cancer equilibrium with CTLs E7 exists if
R0>1+λ2a4ωs1ξ1anda1λ2a2λ1>1; |
(9) The infected-epithelial equilibrium with antibodies E8 exists if
R0>1+λ1a3ωpϵ1+ϵ1a5a1s2ξ2+λ1a3a5ωpa1s2ξ2; |
(10) The infected-cancer equilibrium with antibodies E9 exists if
R1>1+λ2a3ωpϵ2+ϵ2a5a2s2ξ2+λ2a3a5ωpa2s2ξ2; |
(11) The infected-cancer equilibrium with CTLs and antibodies E10 exists if
R1>1+λ2a4ωs1ξ1+ϵ2a5a2s2ξ2+ϵ2λ2a4a5ωs1ξ1a2s2ξ2andpϵ2a4s1ξ1a3>1; |
(12) The infected epithelial-cancer equilibrium with CTLs E11 exists if
λ2ϵ1λ1ϵ2+s1ξ1a3pϵ2a4+ωϵ1s1ξ1λ1ϵ2a4>1, |
R0+λ1ϵ2a4ωϵ1s1ξ1>1+λ2a4ωs1ξ1+λ1a3ωpϵ1 |
and
R1+a1ϵ2a2ϵ1+λ1ϵ2a4ωϵ1s1ξ1+a1λ1ϵ2a3ωpϵ21a2+a1ϵ2λ2a4ωϵ1s1ξ1a2>1+λ1a3ωpϵ1+λ2a4ωs1ξ1+a1λ1ϵ22a4ωϵ21s1ξ1a2+αθλ1ϵ2ωϵ1a2; |
(13) The infected epithelial-cancer equilibrium with CTLs and antibodies E12 exists if
R0>1+ϵ1a5a1s2ξ2+λ2a4ωs1ξ1+ϵ1λ2a4a5ωa1s1ξ1s2ξ2, |
a1λ2a2λ1+ϵ1λ2a5λ1a2s2ξ2>1+ϵ2a5a2s2ξ2 |
and
R0+λ1ϵ2a4ωϵ1s1ξ1+λ1ϵ2a4a5ωa1s1ξ1s2ξ2>1+ϵ1a5a1s2ξ2+λ1a3ωpϵ1+λ2a4ωs1ξ1+λ1a3a5ωpa1s2+ϵ1λ2a4a5ωa1s1ξ1s2ξ2. |
Proof. To get the equilibria of system (2.1), we solve the following system:
{0=α−λ1NM−λ2NC−ωN,0=θλ1NM−ϵ1MV−a1M,0=θλ2NC−ϵ2CV−ξ1CT−a2C,0=pϵ1MV+pϵ2CV−ξ2VA−a3V,0=s1ξ1CT−a4T,0=s2ξ2VA−a5A. |
Then, we obtain
(1) The trivial equilibrium
E0=(N0,0,0,0,0,0)=(αω,0,0,0,0,0). |
This point always exists. This point has no biological meaning as all components vanish except for the nutrient.
(2) The uninfected-epithelial equilibrium E1=(N1,M1,0,0,0,0). The components N1 and M1 are defined as:
N1=a1θλ1,M1=ωλ1(R0−1), |
where
R0=αθλ1ωa1. |
As N1>0, the equilibrium E1 exists if R0>1. This equilibrium represents a healthy individual without cancer or SARS-CoV-2 infection.
(3) The uninfected-cancer equilibrium E2=(N2,0,C2,0,0,0), where
N2=a2θλ2,C2=ωλ2(R1−1), |
where
R1=αθλ2ωa2. |
As N2>0, the point E2 exists if R1>1. This equilibrium represents the case of a person who has cancer, but without SARS-CoV-2 infection.
(4) The infected-epithelial equilibrium E3=(N3,M3,0,V3,0,0), where
N3=αpϵ1ωpϵ1+λ1a3,M3=a3pϵ1, |
and
V3=ωpa1ωpϵ1+λ1a3(R0−1−λ1a3ωpϵ1). |
Clearly, N3>0, M3>0, and V3>0 if
R0>1+λ1a3ωpϵ1. |
Hence, E3 exists if
R0>1+λ1a3ωpϵ1. |
The person here suffers from SARS-CoV-2 infection, but he is cancer-free.
(5) The infected-cancer equilibrium E4=(N4,0,C4,V4,0,0), where
N4=αpϵ2ωpϵ2+λ2a3,C4=a3pϵ2, |
and
V4=ωpa2ωpϵ2+λ2a3(R1−1−λ2a3ωpϵ2). |
Notably, N4>0, C4>0, and V4>0 if
R1>1+λ2a3ωpϵ2. |
Thus, E4 exists if
R1>1+λ2a3ωpϵ2. |
In this scenario, the cancer patient is experiencing a SARS-CoV-2 infection with the disappearance of healthy epithelial cells.
(6) The uninfected-cancer equilibrium with CTLs E5=(N5,0,C5,0,T5,0), where
N5=αs1ξ1λ2a4+ωs1ξ1,C5=a4s1ξ1, |
and
T5=ωs1a2λ2a4+ωs1ξ1(R1−1−λ2a4ωs1ξ1). |
Clearly, N5>0, C5>0, and T5>0 if
R1>1+λ2a4ωs1ξ1. |
Hence, E5 exists if
R1>1+λ2a4ωs1ξ1. |
CTLs are activated to eliminate cancer cells in a patient who has experienced the disappearance of healthy epithelial cells.
(7) The infected epithelial-cancer equilibrium without immunity E6=(N6,M6,C6,V6,0,0), where
N6=a2(a1ϵ2a2ϵ1−1)θλ2(λ1ϵ2λ2ϵ1−1),M6=ωϵ2(1+λ2a3ωpϵ2+αθλ1ϵ2ωϵ1a2−R1−a1ϵ2a2ϵ1−a1λ2a3ωpϵ1a2)ϵ1λ2(a1ϵ2a2ϵ1−1)(λ1ϵ2λ2ϵ1−1),C6=ωa1ϵ2(1+λ1a3ωpϵ1+αθϵ1λ2ωa1ϵ2−R0−a2ϵ1a1ϵ2−λ1a2a3ωpa1ϵ2)ϵ1a2λ2(a1ϵ2a2ϵ1−1)(λ1ϵ2λ2ϵ1−1),V6=λ1a2(a1λ2a2λ1−1)ϵ1λ2(λ1ϵ2λ2ϵ1−1). |
We note that the components are positive if
R1+a1ϵ2a2ϵ1+a1λ2a3ωpϵ1a2<1+λ2a3ωpϵ2+αθλ1ϵ2ωϵ1a2, |
R0+a2ϵ1a1ϵ2+λ1a2a3ωpa1ϵ2<1+λ1a3ωpϵ1+αθϵ1λ2ωa1ϵ2, |
a1ϵ2a2ϵ1>1,λ1ϵ2λ2ϵ1>1,anda1λ2a2λ1>1. |
Thus, E6 is defined when the above conditions are met. In this scenario, the cancer patient has SARS-CoV-2 infection with inactive immune responses.
(8) The uninfected epithelial-cancer equilibrium with CTLs E7=(N7,M7,C7,0,T7,0), where
N7=a1θλ1,M7=ωλ1(R0−1−λ2a4ωs1ξ1),C7=a4s1ξ1,T7=a2ξ1(a1λ2a2λ1−1). |
Accordingly, N7>0, C7>0, M7>0 if
R0>1+λ2a4ωs1ξ1, |
and T7>0 if
a1λ2a2λ1>1. |
Therefore, E7 exists when
R0>1+λ2a4ωs1ξ1anda1λ2a2λ1>1. |
Here, the cancer patient has active CTL immunity that works on killing cancer cells.
(9) The infected-epithelial equilibrium with antibodies E8=(N8,M8,0,V8,0,A8), where
N8=ϵ1a5+a1s2ξ2θλ1s2ξ2,M8=ωa1s2ξ2λ1(ϵ1a5+a1s2ξ2)(R0−1−ϵ1a5a1s2ξ2),V8=a5s2ξ2,A8=ωpa1ϵ1s2λ1(ϵ1a5+a1s2ξ2)(R0−1−λ1a3ωpϵ1−ϵ1a5a1s2ξ2−λ1a3a5ωpa1s2ξ2). |
Thus, the components are positive and E8 exists if
R0>1+ϵ1a5a1s2ξ2andR0>1+λ1a3ωpϵ1+ϵ1a5a1s2ξ2+λ1a3a5ωpa1s2ξ2. |
Notably, the first condition is naturally satisfied when the second condition is met.
The SARS-CoV-2 patient has active antibody immunity against the virus.
(10) The infected-cancer equilibrium with antibodies E9=(N9,0,C9,V9,0,A9), where
N9=ϵ2a5+a2s2ξ2θλ2s2ξ2,C9=ωa2s2ξ2λ2(ϵ2a5+a2s2ξ2)(R1−1−ϵ2a5a2s2ξ2),V9=a5s2ξ2,A9=ωpa2ϵ2s2λ2(ϵ2a5+a2s2ξ2)(R1−1−λ2a3ωpϵ2−ϵ2a5a2s2ξ2−λ2a3a5ωpa2s2ξ2). |
We note that E9 is defined when
R1>1+λ2a3ωpϵ2+ϵ2a5a2s2ξ2+λ2a3a5ωpa2s2ξ2, |
where the other condition
R1>1+ϵ2a5a2s2ξ2 |
is naturally met when the previous condition is satisfied.
The infected cancer patient who suffers from the disappearance of epithelial cells has active antibody immunity against the virus.
(11) The infected-cancer equilibrium with CTLs and antibodies E10=(N10,0,C10,V10,T10,A10), where
N10=αs1ξ1λ2a4+ωs1ξ1,C10=a4s1ξ1,V10=a5s2ξ2,T10=ωs1a2λ2a4+ωs1ξ1(R1−1−λ2a4ωs1ξ1−ϵ2a5a2s2ξ2−ϵ2λ2a4a5ωs1ξ1a2s2ξ2),A10=a3ξ2(pϵ2a4s1ξ1a3−1). |
Thus, E10 is biologically accepted when
R1>1+λ2a4ωs1ξ1+ϵ2a5a2s2ξ2+ϵ2λ2a4a5ωs1ξ1a2s2ξ2andpϵ2a4s1ξ1a3>1. |
This point represents the case of a cancer patient who is infected, with active immune responses, but experiencing the disappearance of epithelial cells.
(12) The infected epithelial-cancer equilibrium with CTLs E11=(N11,M11,C11,V11,T11,0), where
N11=αϵ1s1ξ1λ1ϵ2a4(λ2ϵ1λ1ϵ2+s1ξ1a3pϵ2a4+ωϵ1s1ξ1λ1ϵ2a4−1),M11=ϵ2a4ϵ1s1ξ1(s1ξ1a3pϵ2a4−1),C11=a4s1ξ1,V11=ωa1s1ξ1(R0+λ1ϵ2a4ωϵ1s1ξ1−1−λ2a4ωs1ξ1−λ1a3ωpϵ1)λ1ϵ2a4(λ2ϵ1λ1ϵ2+s1ξ1a3pϵ2a4+ωϵ1s1ξ1λ1ϵ2a4−1),T11=ωϵ1s1a2λ1ϵ2a4(λ2ϵ1λ1ϵ2+s1ξ1a3pϵ2a4+ωϵ1s1ξ1λ1ϵ2a4−1)(R1+a1ϵ2a2ϵ1+λ1ϵ2a4ωϵ1s1ξ1+a1λ1ϵ2a3ωpϵ21a2+a1ϵ2λ2a4ωϵ1s1ξ1a2−1−λ1a3ωpϵ1−λ2a4ωs1ξ1−a1λ1ϵ22a4ωϵ21s1ξ1a2−αθλ1ϵ2ωϵ1a2). |
It is easy to observe that N11, V11, and T11 are positive and E11 exists when
λ2ϵ1λ1ϵ2+s1ξ1a3pϵ2a4+ωϵ1s1ξ1λ1ϵ2a4>1, |
R0+λ1ϵ2a4ωϵ1s1ξ1>1+λ2a4ωs1ξ1+λ1a3ωpϵ1 |
and
R1+a1ϵ2a2ϵ1+λ1ϵ2a4ωϵ1s1ξ1+a1λ1ϵ2a3ωpϵ21a2+a1ϵ2λ2a4ωϵ1s1ξ1a2>1+λ1a3ωpϵ1+λ2a4ωs1ξ1+a1λ1ϵ22a4ωϵ21s1ξ1a2+αθλ1ϵ2ωϵ1a2. |
At this point, the infected cancer patient has active CTLs and inactive antibody immune response.
(13) The infected epithelial-cancer equilibrium with CTLs and antibodies E12=(N12,M12,C12,V12,T12,A12), where
N12=ϵ1a5+a1s2ξ2θλ1s2ξ2,M12=ωa1s2ξ2λ1(ϵ1a5+a1s2ξ2)(R0−1−ϵ1a5a1s2ξ2−λ2a4ωs1ξ1−ϵ1λ2a4a5ωa1s1ξ1s2ξ2),C12=a4s1ξ1,V12=a5s2ξ2,T12=a2s2s1ξ1(a1λ2a2λ1+ϵ1λ2a5λ1a2s2ξ2−1−ϵ2a5a2s2ξ2),A12=ωpa1ϵ1s2λ1(ϵ1a5+a1s2ξ2)(R0+λ1ϵ2a4ωϵ1s1ξ1+λ1ϵ2a4a5ωa1s1ξ1s2ξ2−1−ϵ1a5a1s2ξ2−λ1a3ωpϵ1−λ2a4ωs1ξ1−λ1a3a5ωpa1s2−ϵ1λ2a4a5ωa1s1ξ1s2ξ2). |
We observe that the components are positive and E12 is defined if
R0>1+ϵ1a5a1s2ξ2+λ2a4ωs1ξ1+ϵ1λ2a4a5ωa1s1ξ1s2ξ2, |
a1λ2a2λ1+ϵ1λ2a5λ1a2s2ξ2>1+ϵ2a5a2s2ξ2 |
and
R0+λ1ϵ2a4ωϵ1s1ξ1+λ1ϵ2a4a5ωa1s1ξ1s2ξ2>1+ϵ1a5a1s2ξ2+λ1a3ωpϵ1+λ2a4ωs1ξ1+λ1a3a5ωpa1s2+ϵ1λ2a4a5ωa1s1ξ1s2ξ2. |
This point imitates the case of cancer patient with SARS-CoV-2 infection and active immune responses.
In the next sections, we will focus our analysis on the equilibria E0, E1, E3, E6, E7, E8, E11, and E12 as we are interested in the points where the epithelial cells component (M) does not vanish.
The following theorems are aimed to establish the global stability of equilibria through nominating Lyapunov functions. Let Y′i be the largest invariant subset of
Yi={(N,M,C,V,T,A) | dΣidt=0}, |
where i=0,1,3,6,7,8,11,12.
Theorem 4. The equilibrium E0 is globally asymptotically stable (GS) when R0≤1 and R1≤1.
Proof. We consider
Σ0(t)=N0(NN0−1−lnNN0)+1θM+1θC+1θpV+1θs1T+1θps2A. |
Then, we get
dΣ0dt=(1−N0N)(α−λ1NM−λ2NC−ωN)+1θ(θλ1NM−ϵ1MV−a1M)+1θ(θλ2NC−ϵ2CV−ξ1CT−a2C)+1θp(pϵ1MV+pϵ2CV−ξ2VA−a3V)+1θs1(s1ξ1CT−a4T)+1θps2(s2ξ2VA−a5A)=(1−N0N)(α−ωN)+λ1N0M+λ2N0C−a1θM−a2θC−a3θpV−a5θps2A=−ω(N−N0)2N+a1θ(R0−1)M+a2θ(R1−1)C−a3θpV−a5θps2A. |
We see that
dΣ0dt≤0 |
if R0≤1 and R1≤1. Furthermore,
dΣ0dt=0 |
when N=N0 and M=C=V=A=0. This gives
dCdt=0. |
From the third equation of model (2.1), we obtain T=0. Hence, Y′0={E0} and by LaSalle's invariance principle (LP) [37], E0 is GS if R0≤1 and R1≤1.
Theorem 5. Let R0>1. Then, the equilibrium E1 is GS if
R0≤1+λ1a3ωpϵ1 |
and
a1λ2a2λ1≤1. |
Proof. We opt
Σ1(t)=N1(NN1−1−lnNN1)+1θM1(MM1−1−lnMM1)+1θC+1θpV+1θs1T+1θps2A. |
Then, we get
dΣ1dt=(1−N1N)(α−λ1NM−λ2NC−ωN)+1θ(1−M1M)(θλ1NM−ϵ1MV−a1M)+1θ(θλ2NC−ϵ2CV−ξ1CT−a2C)+1θp(pϵ1MV+pϵ2CV−ξ2VA−a3V)+1θs1(s1ξ1CT−a4T)+1θps2(s2ξ2VA−a5A). | (4.1) |
At equilibrium, E1 solves the system
{α=λ1N1M1+ωN1,λ1N1M1=a1θM1. |
Applying the above equations to collect (4.1) gives
dΣ1dt=(1−N1N)(ωN1−ωN)+λ1N1M1(2−N1N−NN1)+(λ2N1−a2θ)C+(ϵ1θM1−a3θp)V−a4θs1T−a5θps2A=−ω(N−N1)2N+λ1N1M1(2−N1N−NN1)+a2θ(a1λ2a2λ1−1)C+ωϵ1θλ1(R0−1−λ1a3ωpϵ1)V−a4θs1T−a5θps2A. |
Hence,
dΣ1dt≤0 |
if
R0≤1+λ1a3ωpϵ1anda1λ2a2λ1≤1. |
In addition,
dΣ1dt=0 |
if N=N1 and C=V=T=A=0. Consequently,
dNdt=0 |
and the first equation of (2.1) gives M=M1. Therefore, Y′1={E1} and E1 is GS when
R0>1,R0≤1+λ1a3ωpϵ1anda1λ2a2λ1≤1 |
according to LP [37].
Theorem 6. Let
R0>1+λ1a3ωpϵ1. |
Then, the equilibrium E3 is GS if
R0+a2ϵ1a1ϵ2+λ1a2a3ωpa1ϵ2≥1+λ1a3ωpϵ1+αθϵ1λ2ωa1ϵ2 |
and
R0≤1+λ1a3ωpϵ1+ϵ1a5a1s2ξ2+λ1a3a5ωpa1s2ξ2. |
Proof. We construct
Σ3(t)=N3(NN3−1−lnNN3)+1θM3(MM3−1−lnMM3)+1θC+1θpV3(VV3−1−lnVV3)+1θs1T+1θps2A. |
Then, we get
dΣ3dt=(1−N3N)(α−λ1NM−λ2NC−ωN)+1θ(1−M3M)(θλ1NM−ϵ1MV−a1M)+1θ(θλ2NC−ϵ2CV−ξ1CT−a2C)+1θp(1−V3V)(pϵ1MV+pϵ2CV−ξ2VA−a3V)+1θs1(s1ξ1CT−a4T)+1θps2(s2ξ2VA−a5A). | (4.2) |
The equilibrium conditions at E3 are
{α=λ1N3M3+ωN3,λ1N3M3=ϵ1θM3V3+a1θM3,ϵ1θM3V3=a3θpV3. | (4.3) |
By using (6), Eq (4.2) becomes
dΣ3dt=(1−N3N)(ωN3−ωN)+λ1N3M3(2−N3N−NN3)+(λ2N3−a2θ−ϵ2θV3)C+(ξ2θpV3−a5θps2)A−a4θs1T=−ω(N−N3)2N+λ1N3M3(2−N3N−NN3)+ωpa1ϵ2θ(λ1a3+ωpϵ1)(1+λ1a3ωpϵ1+αθϵ1λ2ωa1ϵ2−R0−a2ϵ1a1ϵ2−λ1a2a3ωpa1ϵ2)C+ωa1ξ2θ(λ1a3+ωpϵ1)(R0−1−λ1a3ωpϵ1−ϵ1a5a1s2ξ2−λ1a3a5ωpa1s2ξ2)A−a4θs1T. |
We observe that
dΣ3dt≤0 |
if
R0+a2ϵ1a1ϵ2+λ1a2a3ωpa1ϵ2≥1+λ1a3ωpϵ1+αθϵ1λ2ωa1ϵ2 |
and
R0≤1+λ1a3ωpϵ1+ϵ1a5a1s2ξ2+λ1a3a5ωpa1s2ξ2. |
In addition, dΣ3dt=0 when N=N3, M=M3, V=V3 and C=T=A=0. Thus, Y′3={E3} and LP [37] implies that E3 is GS when
R0>1+λ1a3ωpϵ1 |
with the above conditions.
Theorem 7. Let the existence conditions of E6 be satisfied. Then, the equilibrium E6 is GS if
R1+a1ϵ2a2ϵ1+λ1ϵ2a4ωϵ1s1ξ1+a1λ1ϵ2a3ωpϵ21a2+a1ϵ2λ2a4ωϵ1s1ξ1a2≤1+λ1a3ωpϵ1+λ2a4ωs1ξ1+a1λ1ϵ22a4ωϵ21s1ξ1a2+αθλ1ϵ2ωϵ1a2 |
and
a1λ2a2λ1+ϵ1λ2a5λ1a2s2ξ2≤1+ϵ2a5a2s2ξ2. |
Proof. See Appendix A.
Theorem 8. Let
R0>1+λ2a4ωs1ξ1anda1λ2a2λ1>1. |
Then, the equilibrium E7 is GS if
R0+λ1ϵ2a4ωϵ1s1ξ1≤1+λ2a4ωs1ξ1+λ1a3ωpϵ1. |
Proof. See Appendix B.
Theorem 9. Let
R0>1+λ1a3ωpϵ1+ϵ1a5a1s2ξ2+λ1a3a5ωpa1s2ξ2. |
Then, E8 is GS if
a1λ2a2λ1+ϵ1λ2a5λ1a2s2ξ2≤1+ϵ2a5a2s2ξ2. |
Proof. See Appendix C.
Theorem 10. Let
λ2ϵ1λ1ϵ2+s1ξ1a3pϵ2a4+ωϵ1s1ξ1λ1ϵ2a4>1, |
R0+λ1ϵ2a4ωϵ1s1ξ1>1+λ2a4ωs1ξ1+λ1a3ωpϵ1 |
and
R1+a1ϵ2a2ϵ1+λ1ϵ2a4ωϵ1s1ξ1+a1λ1ϵ2a3ωpϵ21a2+a1ϵ2λ2a4ωϵ1s1ξ1a2>1+λ1a3ωpϵ1+λ2a4ωs1ξ1+a1λ1ϵ22a4ωϵ21s1ξ1a2+αθλ1ϵ2ωϵ1a2. |
Then, E11 is GS if
R0+λ1ϵ2a4ωϵ1s1ξ1+λ1ϵ2a4a5ωa1s1ξ1s2ξ2≤1+ϵ1a5a1s2ξ2+λ1a3ωpϵ1+λ2a4ωs1ξ1+λ1a3a5ωpa1s2+ϵ1λ2a4a5ωa1s1ξ1s2ξ2. |
Proof. See Appendix D.
Theorem 11. Let
R0>1+ϵ1a5a1s2ξ2+λ2a4ωs1ξ1+ϵ1λ2a4a5ωa1s1ξ1s2ξ2, |
a1λ2a2λ1+ϵ1λ2a5λ1a2s2ξ2>1+ϵ2a5a2s2ξ2 |
and
R0+λ1ϵ2a4ωϵ1s1ξ1+λ1ϵ2a4a5ωa1s1ξ1s2ξ2>1+ϵ1a5a1s2ξ2+λ1a3ωpϵ1+λ2a4ωs1ξ1+λ1a3a5ωpa1s2+ϵ1λ2a4a5ωa1s1ξ1s2ξ2. |
Then, the equilibrium E12 is GS.
Proof. We nominate
Σ12(t)=N12(NN12−1−lnNN12)+1θM12(MM12−1−lnMM12)+1θC12(CC12−1−lnCC12)+1θpV12(VV12−1−lnVV12)+1θs1T12(TT12−1−lnTT12)+1θps2A12(AA12−1−lnAA12). |
Then, we get
dΣ12dt=(1−N12N)(α−λ1NM−λ2NC−ωN)+1θ(1−M12M)(θλ1NM−ϵ1MV−a1M)+1θ(1−C12C)(θλ2NC−ϵ2CV−ξ1CT−a2C)+1θp(1−V12V)(pϵ1MV+pϵ2CV−ξ2VA−a3V)+1θs1(1−T12T)(s1ξ1CT−a4T)+1θps2(1−A12A)(s2ξ2VA−a5A). | (4.4) |
By using the conditions of equilibrium state at E12
{α=λ1N12M12+λ2N12C12+ωN12,λ1N12M12=ϵ1θM12V12+a1θM12,λ2N12C12=ϵ2θC12V12+ξ1θC12T12+a2θC12,ϵ1θM12V12+ϵ2θC12V12=ξ2θpV12A12+a3θpV12,ξ1θC12T12=a4θs1T12,ξ2θpV12A12=a5θps2A12. |
Equation (4.4) is transformed into
dΣ12dt=−ω(N−N12)2N+λ1N12M12(2−N12N−NN12)+λ2N12C12(2−N12N−NN12). |
We note that dΣ12dt≤0 and dΣ12dt=0 at E12. Based on LP [37], E12 is GS when the existence conditions are met.
The ode45 solver of Matlab is utilized to effectuate the numerical simulations. ode45 is the default solver for ODEs in Matlab. It utilizes an explicit Runge-Kutta formula and generally performs well with a wide range of ODE problems. Nevertheless, when dealing with stiff problems or situations demanding high accuracy, alternative solvers like ode15s, ode23s, and ode23t may prove more efficient. We consider three different groups of initial conditions:
(1) (N(0),M(0),C(0),V(0),T(0),A(0))=(0.0001,0.01,0.03,0.01,0.001,0.001);
(2) (N(0),M(0),C(0),V(0),T(0),A(0))=(0.1,0.03,0.06,0.05,0.002,0.003);
(3) (N(0),M(0),C(0),V(0),T(0),A(0))=(0.6,0.06,0.1,0.06,0.03,0.01).
The chosen sets of initial conditions are arbitrary, as the global stability is guaranteed for any initial values. To affirm the global stability of E0, E1, E3, E6, E7, E8, E11, and E12, we partition the numerical simulations into eight classes. We change the values of λ1, λ2, s1, s2, ϵ1, ϵ2, ξ2, ω2, ω3, and ω5 to obtain the global stability of the equilibrium in each case. The other values are fixed and given in Table 1.
Parameter | Value | Source |
α | 0.02 | [33] |
λ1 | Varied | – |
λ2 | Varied | – |
ω | 0.02 | [33] |
ω1 | 0.01 | [38] |
ω2 | Varied | – |
ω3 | Varied | – |
ω4 | 0.1 | [38] |
ω5 | Varied | – |
θ | 0.8 | [33] |
ϵ1 | Varied | – |
ϵ2 | Varied | – |
p | 0.24 | [39] |
ξ1 | 0.5 | [38] |
ξ2 | Varied | – |
s1 | Varied | – |
s2 | Varied | – |
Thus, we have
(i) We opt λ1=0.03, λ2=0.03, s1=0.1, s2=0.2, ϵ1=0.55, ϵ2=0.55, ξ2=4.88×10−8, ω2=0.17, ω3=0.6, and ω5=0.05. This yields R0=0.8<1 and R1=0.1263<1. Thus, E0=(1,0,0,0,0,0) is GS as indicated in Theorem 4 (see Figure 2). This point has no significant biological interpretation as all populations, except the nutrient's component, tend to zero.
(ii) We choose λ1=0.05, λ2=0.03, s1=0.1, s2=0.2, ϵ1=1×10−5, ϵ2=0.55, ξ2=4.88×10−8, ω2=0.17, ω3=0.9, and ω5=0.05. The corresponding thresholds are
R0=1.33>1,R0<1+λ1a3ωpϵ1=9.58×105anda1λ2a2λ1=0.0947<1. |
Thus, E1=(0.75,0.1333,0,0,0,0) is GS which matches with Theorem 5 (see Figure 3). This simulates the case of an individual who neither has SARS-CoV-2 infection nor cancer.
(iii) We nominate λ1=0.07, λ2=0.03, s1=0.1, s2=0.2, ϵ1=0.5, ϵ2=0.55, ξ2=4.88×10−8, ω2=0.17, ω3=1×10−4, and ω5=0.2. This gives
R0=1.8667>1+λ1a3ωpϵ1=1.5863, |
R0+a2ϵ1a1ϵ2+λ1a2a3ωpa1ϵ2=10.9996>1+λ1a3ωpϵ1+αθϵ1λ2ωa1ϵ2=2.3135 |
and
R0<1+λ1a3ωpϵ1+ϵ1a5a1s2ξ2+λ1a3a5ωpa1s2ξ2=5.9593×108. |
This causes E3=(0.6304,0.1675,0,0.01061,0,0) to be GS as verified in Theorem 6 (see Figure 4). This imitates the case of a patient with SARS-CoV-2 infection but without cancer.
(iv) We pick out λ1=0.06, λ2=0.05, s1=0.1, s2=0.2, ϵ1=1×10−5, ϵ2=0.55, ξ2=4.88×10−8, ω2=0.001, ω3=0.001, and ω5=0.05. This corresponds to
R1+a1ϵ2a2ϵ1+a1λ2a3ωpϵ1a2=1.0982×105<1+λ2a3ωpϵ2+αθλ1ϵ2ωϵ1a2=1.2572×105, |
R0+a2ϵ1a1ϵ2+λ1a2a3ωpa1ϵ2=1.9341<1+λ1a3ωpϵ1+αθϵ1λ2ωa1ϵ2=2.6251×104, |
a1ϵ2a2ϵ1=7.8571×104>1,λ1ϵ2λ2ϵ1=66000>1,a1λ2a2λ1=1.1905>1, |
R1+a1ϵ2a2ϵ1+λ1ϵ2a4ωϵ1s1ξ1+a1λ1ϵ2a3ωpϵ21a2+a1ϵ2λ2a4ωϵ1s1ξ1a2=2.0634×109<1+λ1a3ωpϵ1+λ2a4ωs1ξ1+a1λ1ϵ22a4ωϵ21s1ξ1a2+αθλ1ϵ2ωϵ1a2=3.1114×1010 |
and
a1λ2a2λ1+ϵ1λ2a5λ1a2s2ξ2=2.8473×103<1+ϵ2a5a2s2ξ2=1.8784×108. |
In parallel with Theorem 7, E6=(0.625,0.06742,0.1591,0.0073,0,0) is GS (see Figure 5). This simulates the case of a cancer patient who has SARS-CoV-2 infection with inactive immune responses.
(v) We opt λ1=0.06, λ2=0.05, s1=1.2, s2=0.2, ϵ1=1×10−5, ϵ2=0.55, ξ2=4.88×10−8, ω2=1×10−4, ω3=0.9, and ω5=0.05. The resultant thresholds are
R0=1.6>1+λ2a4ωs1ξ1=1.5,a1λ2a2λ1=1.2438>1 |
and
R0+λ1ϵ2a4ωϵ1s1ξ1=3.3×104<1+λ2a4ωs1ξ1+λ1a3ωpϵ1=1.15×106. |
In agreement with Theorem 8, E7=(0.625,0.0333,0.2,0,0.0098,0) is GS (see Figure 6). In this scenario, the cancer patient does not have SARS-CoV-2 infection, and the CTL immunity against cancer cells is active.
(vi) We select λ1=0.07, λ2=0.03, s1=0.4, s2=1.6, ϵ1=0.5, ϵ2=0.55, ξ2=1.6, ω2=0.17, ω3=1×10−6, and ω5=1×10−8. This gives
R0=1.8667>1+λ1a3ωpϵ1+ϵ1a5a1s2ξ2+λ1a3a5ωpa1s2ξ2=1.7895 |
and
a1λ2a2λ1+ϵ1λ2a5λ1a2s2ξ2=0.0765<1+ϵ2a5a2s2ξ2=1.0226. |
As indicated in Theorem 9, E8=(0.6055,0.1862,0,0.0078,0,0.0015) is GS (see Figure 7). Here, the patient is solely affected by SARS-CoV-2 infection with an active immune response against the virus.
(vii) We consider λ1=0.06, λ2=0.06, s1=1.6, s2=0.2, ϵ1=1.4×10−1, ϵ2=0.55, ξ2=4.88×10−8, ω2=0.001, ω3=0.001, and ω5=0.05. This gives
λ2ϵ1λ1ϵ2+s1ξ1a3pϵ2a4+ωϵ1s1ξ1λ1ϵ2a4=1.8808>1, |
R0+λ1ϵ2a4ωϵ1s1ξ1=3.3679>1+λ2a4ωs1ξ1+λ1a3ωpϵ1=3.325, |
R1+a1ϵ2a2ϵ1+λ1ϵ2a4ωϵ1s1ξ1+a1λ1ϵ2a3ωpϵ21a2+a1ϵ2λ2a4ωϵ1s1ξ1a2=22.7143>1+λ1a3ωpϵ1+λ2a4ωs1ξ1+a1λ1ϵ22a4ωϵ21s1ξ1a2+αθλ1ϵ2ωϵ1a2=22.2262 |
and
R0+λ1ϵ2a4ωϵ1s1ξ1+λ1ϵ2a4a5ωa1s1ξ1s2ξ2=5.917×107<1+ϵ1a5a1s2ξ2+λ1a3ωpϵ1+λ2a4ωs1ξ1+λ1a3a5ωpa1s2+ϵ1λ2a4a5ωa1s1ξ1s2ξ2=1.1129×108. |
As proved in Theorem 10, E11=(0.6422,0.0357,0.15,0.0059,0.01316,0) is GS (Figure 8). The CTL immunity against cancer cells is activated in the cancer patient infected with SARS-CoV-2.
(viii) We pick up λ1=0.06, λ2=0.06, s1=1.6, s2=1.9, ϵ1=1.4×10−1, ϵ2=1.2, ξ2=1.9, ω2=0.001, ω3=0.001, and ω5=0.000001. The corresponding thresholds are
R0=1.6>1+ϵ1a5a1s2ξ2+λ2a4ωs1ξ1+ϵ1λ2a4a5ωa1s1ξ1s2ξ2=1.4875, |
a1λ2a2λ1+ϵ1λ2a5λ1a2s2ξ2=1.4655>1+ϵ2a5a2s2ξ2=1.3166 |
and
R0+λ1ϵ2a4ωϵ1s1ξ1+λ1ϵ2a4a5ωa1s1ξ1s2ξ2=5.5569>1+ϵ1a5a1s2ξ2+λ1a3ωpϵ1+λ2a4ωs1ξ1+λ1a3a5ωpa1s2+ϵ1λ2a4a5ωa1s1ξ1s2ξ2=3.4110. |
As indicated in Theorem 11, E12=(0.641,0.03657,0.1499,0.006,0.006,0.0123) is GS (see Figure 9). The CTL and antibody immune responses are activated in the cancer patient infected with SARS-CoV-2.
To observe the impact of ϵ2 (the infection rate of cancer cells by SARS-CoV-2) on the concentration of cancer cells before stimulating any immune responses, we increase the value of ϵ2 in case (iv). When we set ϵ2=0.7, we get C6=0.125. Additionally, if we raise ϵ2 to 0.9, we find C6=0.097. Figure 10 shows the impact of increasing ϵ2 on the decrease in the concentration of cancer cells for other values of ϵ2. Thus, the infection of cancer cells by SARS-CoV-2 can lead to a reduction in cancer cells concentration, consequently resulting in a remission or an improvement in the patient's situation. Similarly, when we increase the value of ξ1 (the killing rate of cancer cells by CTLs) in case (viii), the concentration of cancer cells decreases to lower values (See Figure 11). In fact, these results align with many studies that suggest the ability of SARS-CoV-2 to infect cancer cells and induce immune responses, leading to cancer remission [15,16,17,18,19].
Cancer remission after SARS-CoV-2 infection has been observed in many patients. This remission has been transient or complete, and it has been recorded with various types of cancer such as NK/T-cell lymphoma [15], Hodgkin lymphoma [16], follicular lymphoma [17], acute leukemia [18], and other types of cancer [6]. This has raised an urgent need to understand the relationship between cancer and SARS-CoV-2. This paper proposes and analyzes an oncolytic SARS-CoV-2 model. The model has 13 equilibrium points, and we focused our analysis on the points with the most important biological significance as follows:
(1) The trivial equilibrium E0 which is GS if R0≤1 and R1≤1. At this point, all populations disappear except for the nutrient.
(2) The uninfected-epithelial equilibrium E1 is GS if R0>1, R0≤1+λ1a3ωpϵ1, and a1λ2a2λ1≤1. Here, the patient is free from both SARS-CoV-2 infection and cancer.
(3) The infected-epithelial equilibrium E3 is GS if
R0>1+λ1a3ωpϵ1,R0+a2ϵ1a1ϵ2+λ1a2a3ωpa1ϵ2≥1+λ1a3ωpϵ1+αθϵ1λ2ωa1ϵ2 |
and
R0≤1+λ1a3ωpϵ1+ϵ1a5a1s2ξ2+λ1a3a5ωpa1s2ξ2. |
The patient here has only SARS-CoV-2 infection.
(4) The infected epithelial-cancer equilibrium without immunity E6 is GS when
R1+a1ϵ2a2ϵ1+a1λ2a3ωpϵ1a2<1+λ2a3ωpϵ2+αθλ1ϵ2ωϵ1a2, |
R0+a2ϵ1a1ϵ2+λ1a2a3ωpa1ϵ2<1+λ1a3ωpϵ1+αθϵ1λ2ωa1ϵ2, |
a1ϵ2a2ϵ1>1,λ1ϵ2λ2ϵ1>1,a1λ2a2λ1>1, |
R1+a1ϵ2a2ϵ1+λ1ϵ2a4ωϵ1s1ξ1+a1λ1ϵ2a3ωpϵ21a2+a1ϵ2λ2a4ωϵ1s1ξ1a2≤1+λ1a3ωpϵ1+λ2a4ωs1ξ1+a1λ1ϵ22a4ωϵ21s1ξ1a2+αθλ1ϵ2ωϵ1a2 |
and
a1λ2a2λ1+ϵ1λ2a5λ1a2s2ξ2≤1+ϵ2a5a2s2ξ2. |
Here, the cancer patient has SARS-CoV-2 infection with inactive immunity.
(5) The uninfected epithelial-cancer equilibrium with CTLs E7 is GS when
R0>1+λ2a4ωs1ξ1,a1λ2a2λ1>1 |
and
R0+λ1ϵ2a4ωϵ1s1ξ1≤1+λ2a4ωs1ξ1+λ1a3ωpϵ1. |
Here, the cancer patient with active CTL immunity does not have SARS-CoV-2 infection.
(6) The infected-epithelial equilibrium with antibodies E8 is GS if
R0>1+λ1a3ωpϵ1+ϵ1a5a1s2ξ2+λ1a3a5ωpa1s2ξ2 |
and
a1λ2a2λ1+ϵ1λ2a5λ1a2s2ξ2≤1+ϵ2a5a2s2ξ2. |
The patient is cancer-free and suffers only from SARS-CoV-2 infection with active immunity against the virus.
(7) The infected epithelial-cancer equilibrium with CTLs E11 is GS when
λ2ϵ1λ1ϵ2+s1ξ1a3pϵ2a4+ωϵ1s1ξ1λ1ϵ2a4>1, |
R0+λ1ϵ2a4ωϵ1s1ξ1>1+λ2a4ωs1ξ1+λ1a3ωpϵ1, |
R1+a1ϵ2a2ϵ1+λ1ϵ2a4ωϵ1s1ξ1+a1λ1ϵ2a3ωpϵ21a2+a1ϵ2λ2a4ωϵ1s1ξ1a2>1+λ1a3ωpϵ1+λ2a4ωs1ξ1+a1λ1ϵ22a4ωϵ21s1ξ1a2+αθλ1ϵ2ωϵ1a2 |
and
R0+λ1ϵ2a4ωϵ1s1ξ1+λ1ϵ2a4a5ωa1s1ξ1s2ξ2≤1+ϵ1a5a1s2ξ2+λ1a3ωpϵ1+λ2a4ωs1ξ1+λ1a3a5ωpa1s2+ϵ1λ2a4a5ωa1s1ξ1s2ξ2. |
In this case, the cancer patient has SARS-CoV-2 with active CTLs against the cancer cells.
(8) The infected epithelial-cancer equilibrium with CTLs and antibodies E12 is GS if
R0>1+ϵ1a5a1s2ξ2+λ2a4ωs1ξ1+ϵ1λ2a4a5ωa1s1ξ1s2ξ2, |
a1λ2a2λ1+ϵ1λ2a5λ1a2s2ξ2>1+ϵ2a5a2s2ξ2 |
and
R0+λ1ϵ2a4ωϵ1s1ξ1+λ1ϵ2a4a5ωa1s1ξ1s2ξ2>1+ϵ1a5a1s2ξ2+λ1a3ωpϵ1+λ2a4ωs1ξ1+λ1a3a5ωpa1s2+ϵ1λ2a4a5ωa1s1ξ1s2ξ2. |
The cancer patient has SARS-CoV-2 infection with active immune responses against the cancer cells and virus particles.
We found complete agreement between the theoretical contributions and numerical simulations. The global stability conditions of equilibrium points determine various infection scenarios, such as patients having only SARS-CoV-2, cancer, both SARS-CoV-2 and cancer, or no infections. These conditions are dependent on the parameters of model (2.1), emphasizing the importance of carefully selecting their values. Furthermore, our findings indicate that the infection rate of cancer cells by SARS-CoV-2 (ϵ2) and the killing rate of these cells by CTLs (ξ1) contribute to the reduction in the concentration of cancer cells. Based on these results, SARS-CoV-2 has the potential to lead to cancer remission or improve health conditions by either infecting cancer cells or inducing an anti-cancer immune response. This outcome aligns with recent studies suggesting an oncolytic role of SARS-CoV-2 [15,16,17,18,19]. In comparison to existing works, our model is the first to propose and analyze the oncolytic effect of SARS-CoV-2 in cancer patients. As such, these results warrant further investigation and comparison with the outcomes of experimental studies. Then, the model can be utilized in studies aiming to employ SARS-CoV-2 as oncolytic virotherapy to target cancer cells. However, a main limitation of this work is the absence of real data to estimate the values of the parameters in model (2.1), given the limited availability of such data in this direction. We utilized values from the literature and made assumptions for some parameters. Consequently, model (1) can be developed by:
(i) Estimating parameter values through fitting with real data once sufficient information becomes available;
(ii) Testing the model results against real data;
(iii) Studying the effect of immune responses on the oncolytic role of SARS-CoV-2 and when they can be supportive;
(iv) Including the direct induction of CTLs by SARS-CoV-2;
(v) Adding more components to the model, such as infected cancer cells and infected epithelial cells, for a deeper understanding of the model's dynamics;
(vi) Considering time delays that occur during different biological processes;
(vii) Accounting for parameters and model uncertainties by performing sensitivity analysis and other methods once experimental or real data becomes available.
These enhancements would contribute to a better understanding of the model and facilitate improved predictions.
The authors declare they have not used artificial intelligence (AI) tools in the creation of this article.
The authors acknowledge that this project was funded by the Deanship of Scientific Research (DSR), University of Business and Technology, Jeddah 21361, Saudi Arabia. The authors, therefore, gratefully acknowledge the DSR technical and financial support.
The authors declare that there are no conflicts of interest.
Appendix A
Proof of Theorem 7. We nominate
Σ6(t)=N6(NN6−1−lnNN6)+1θM6(MM6−1−lnMM6)+1θC6(CC6−1−lnCC6)+1θpV6(VV6−1−lnVV6)+1θs1T+1θps2A. |
By evaluating dΣ6dt, we get
dΣ6dt=(1−N6N)(α−λ1NM−λ2NC−ωN)+1θ(1−M6M)(θλ1NM−ϵ1MV−a1M)+1θ(1−C6C)(θλ2NC−ϵ2CV−ξ1CT−a2C)+1θp(1−V6V)(pϵ1MV+pϵ2CV−ξ2VA−a3V)+1θs1(s1ξ1CT−a4T)+1θps2(s2ξ2VA−a5A). | (A.1) |
At equilibrium, E6 fulfills the equations:
{α=λ1N6M6+λ2N6C6+ωN6,λ1N6M6=ϵ1θM6V6+a1θM6,λ2N6C6=ϵ2θC6V6+a2θC6,ϵ1θM6V6+ϵ2θC6V6=a3θpV6. |
Thus, Eq (A.1) can be collected as
dΣ6dt=(1−N6N)(ωN6−ωN)+λ1N6M6(2−N6N−NN6)+λ2N6C6(2−N6N−NN6)+(ξ1θC6−a4θs1)T+(ξ2θpV6−a5θps2)A=−ω(N−N6)2N+λ1N6M6(2−N6N−NN6)+λ2N6C6(2−N6N−NN6)+ωξ1θλ2(a1ϵ2a2ϵ1−1)(λ1ϵ2λ2ϵ1−1)(R1+a1ϵ2a2ϵ1+λ1ϵ2a4ωϵ1s1ξ1+a1λ1ϵ2a3ωpϵ21a2+a1ϵ2λ2a4ωϵ1s1ξ1a2−1−λ1a3ωpϵ1−λ2a4ωs1ξ1−a1λ1ϵ22a4ωϵ21s1ξ1a2−αθλ1ϵ2ωϵ1a2)T+λ1a2ξ2θpϵ1λ2(λ1ϵ2λ2ϵ1−1)(a1λ2a2λ1+ϵ1λ2a5λ1a2s2ξ2−1−ϵ2a5a2s2ξ2)A. |
Thus,
dΣ6dt≤0 |
if
R1+a1ϵ2a2ϵ1+λ1ϵ2a4ωϵ1s1ξ1+a1λ1ϵ2a3ωpϵ21a2+a1ϵ2λ2a4ωϵ1s1ξ1a2≤1+λ1a3ωpϵ1+λ2a4ωs1ξ1+a1λ1ϵ22a4ωϵ21s1ξ1a2+αθλ1ϵ2ωϵ1a2 |
and
a1λ2a2λ1+ϵ1λ2a5λ1a2s2ξ2≤1+ϵ2a5a2s2ξ2. |
Also, it is easy to observe that dΣ6dt=0 when (N,M,C,V,T,A)=(N6,M6,C6,V6,0,0). Hence, Y′6={E6} and E6 is GS when the existence conditions and the global stability conditions are met based on LP [37].
Appendix B
Proof of Theorem 8. We select
Σ7(t)=N7(NN7−1−lnNN7)+1θM7(MM7−1−lnMM7)+1θC7(CC7−1−lnCC7)+1θpV+1θs1T7(TT7−1−lnTT7)+1θps2A. |
Then, we obtain
dΣ7dt=(1−N7N)(α−λ1NM−λ2NC−ωN)+1θ(1−M7M)(θλ1NM−ϵ1MV−a1M)+1θ(1−C7C)(θλ2NC−ϵ2CV−ξ1CT−a2C)+1θp(pϵ1MV+pϵ2CV−ξ2VA−a3V)+1θs1(1−T7T)(s1ξ1CT−a4T)+1θps2(s2ξ2VA−a5A). | (B.1) |
By applying the equilibrium conditions at E7
{α=λ1N7M7+λ2N7C7+ωN7,λ1N7M7=a1θM7,λ2N7C7=ξ1θC7T7+a2θC7,ξ1θC7T7=a4θs1T7. |
Equation (B.1) can be collected as
dΣ7dt=(1−N7N)(ωN7−ωN)+λ1N7M7(2−N7N−NN7)+λ2N7C7(2−N7N−NN7)+(ϵ1θM7+ϵ2θC7−a3θp)V−a5θps2A=−ω(N−N7)2N+λ1N7M7(2−N7N−NN7)+λ2N7C7(2−N7N−NN7)+ωϵ1θλ1(R0+λ1ϵ2a4ωϵ1s1ξ1−1−λ2a4ωs1ξ1−λ1a3ωpϵ1)V−a5θps2A. |
Thus,
dΣ7dt≤0 |
if
R0+λ1ϵ2a4ωϵ1s1ξ1≤1+λ2a4ωs1ξ1+λ1a3ωpϵ1. |
Also, dΣ7dt=0 when (N,M,C,V,T,A)=(N7,M7,C7,0,T7,0). Hence, Y′7={E7} and by LP [37], E7 is GS if
R0>1+λ2a4ωs1ξ1,a1λ2a2λ1>1 |
and
R0+λ1ϵ2a4ωϵ1s1ξ1≤1+λ2a4ωs1ξ1+λ1a3ωpϵ1. |
Appendix C
Proof of Theorem 9. We nominate
Σ8(t)=N8(NN8−1−lnNN8)+1θM8(MM8−1−lnMM8)+1θC+1θpV8(VV8−1−lnVV8)+1θs1T+1θps2A8(AA8−1−lnAA8). |
Then, we have
dΣ8dt=(1−N8N)(α−λ1NM−λ2NC−ωN)+1θ(1−M8M)(θλ1NM−ϵ1MV−a1M)+1θ(θλ2NC−ϵ2CV−ξ1CT−a2C)+1θp(1−V8V)(pϵ1MV+pϵ2CV−ξ2VA−a3V)+1θs1(s1ξ1CT−a4T)+1θps2(1−A8A)(s2ξ2VA−a5A). | (C.1) |
At equilibrium, E8 fulfills the following:
{α=λ1N8M8+ωN8,λ1N8M8=ϵ1θM8V8+a1θM8,ϵ1θM8V8=ξ2θpV8A8+a3θpV8,ξ2θpV8A8=a5θps2A8. |
Thus, Eq (C.1) can be collected as
dΣ8dt=(1−N8N)(ωN8−ωN)+λ1N8M8(2−N8N−NN8)+(λ2N8−a2θ−ϵ2θV8)C−a4θs1T=−ω(N−N8)2N+λ1N8M8(2−N8N−NN8)+a2θ(a1λ2a2λ1+ϵ1λ2a5λ1a2s2ξ2−1−ϵ2a5a2s2ξ2)C−a4θs1T. |
Hence,
dΣ8dt≤0 |
if
a1λ2a2λ1+ϵ1λ2a5λ1a2s2ξ2≤1+ϵ2a5a2s2ξ2anddΣ8dt=0 |
when (N,M,C,V,T,A)=(N8,M8,0,V8,0,A8). Therefore, Y′8={E8} and based on LP [37], E8 is GS when it exists and
a1λ2a2λ1+ϵ1λ2a5λ1a2s2ξ2≤1+ϵ2a5a2s2ξ2. |
Appendix D
Proof of Theorem 10. We select
Σ11(t)=N11(NN11−1−lnNN11)+1θM11(MM11−1−lnMM11)+1θC11(CC11−1−lnCC11)+1θpV11(VV11−1−lnVV11)+1θs1T11(TT11−1−lnTT11)+1θps2A. |
Then, we have
dΣ11dt=(1−N11N)(α−λ1NM−λ2NC−ωN)+1θ(1−M11M)(θλ1NM−ϵ1MV−a1M)+1θ(1−C11C)(θλ2NC−ϵ2CV−ξ1CT−a2C)+1θp(1−V11V)(pϵ1MV+pϵ2CV−ξ2VA−a3V)+1θs1(1−T11T)(s1ξ1CT−a4T)+1θps2(s2ξ2VA−a5A). | (D.1) |
At equilibrium, E11 fulfills the system
{α=λ1N11M11+λ2N11C11+ωN11,λ1N11M11=ϵ1θM11V11+a1θM11,λ2N11C11=ϵ2θC11V11+ξ1θC11T11+a2θC11,ϵ1θM11V11+ϵ2θC11V11=a3θpV11,ξ1θC11T11=a4θs1T11. |
Thus, Eq (D.1) can be collected as
dΣ11dt=(1−N11N)(ωN11−ωN)+λ1N11M11(2−N11N−NN11)+λ2N11C11(2−N11N−NN11)+(ξ2θpV11−a5θps2)A=−ω(N−N11)2N+λ1N11M11(2−N11N−NN11)+λ2N11C11(2−N11N−NN11)+ωa1s1ξ1ξ2θpλ1ϵ2a4(λ2ϵ1λ1ϵ2+s1ξ1a3pϵ2a4+ωϵ1s1ξ1λ1ϵ2a4−1)(R0+λ1ϵ2a4ωϵ1s1ξ1+λ1ϵ2a4a5ωa1s1ξ1s2ξ2−1−ϵ1a5a1s2ξ2−λ1a3ωpϵ1−λ2a4ωs1ξ1−λ1a3a5ωpa1s2−ϵ1λ2a4a5ωa1s1ξ1s2ξ2)A. |
Thus,
dΣ11dt≤0 |
if
R0+λ1ϵ2a4ωϵ1s1ξ1+λ1ϵ2a4a5ωa1s1ξ1s2ξ2≤1+ϵ1a5a1s2ξ2+λ1a3ωpϵ1+λ2a4ωs1ξ1+λ1a3a5ωpa1s2+ϵ1λ2a4a5ωa1s1ξ1s2ξ2. |
Also,
dΣ11dt=0 |
when (N,M,C,V,T,A)=(N11,M11,C11,V11,T11,0). Therefore, Y′11={E11} and by LP [37], E11 is GS when the existence and stability conditions are met.
[1] | World Health Organization, COVID-19 epidemiological update - 27 October 2023, 2023. Available from: https://www.who.int/publications/m/item/covid-19-epidemiological-update---27-october-2023. |
[2] |
S. A. Gold, V. Margulis, Uncovering a link between COVID-19 and renal cell carcinoma, Nat. Rev. Urol., 20 (2023), 330–331. https://doi.org/10.1038/s41585-023-00749-8 doi: 10.1038/s41585-023-00749-8
![]() |
[3] |
M. Shariq, J. A. Sheikh, N. Quadir, N. Sharma, S. E. Hasnain, N. Z. Ehtesham, COVID-19 and tuberculosis: the double whammy of respiratory pathogens, Eur. Respir. Rev., 31 (2022), 210264. https://doi.org/10.1183/16000617.0264-2021 doi: 10.1183/16000617.0264-2021
![]() |
[4] |
A. Tahmasebzadeh, R. Paydar, H. Kaeidi, Lifetime attributable breast cancer risk related to lung CT scan in women with Covid19, Front. Biomed. Technol., 11 (2024), 69–74. https://doi.org/10.18502/fbt.v11i1.14513 doi: 10.18502/fbt.v11i1.14513
![]() |
[5] |
Y. S. Li, H. C. Ren, J. H. Cao, Correlation of SARS‑COV‑2 to cancer: carcinogenic or anticancer? (review), Int. J. Oncolytics, 60 (2022), 42. https://doi.org/10.3892/ijo.2022.5332 doi: 10.3892/ijo.2022.5332
![]() |
[6] |
C. Meo, G. Palma, F. Bruzzese, A. Budillon, C. Napoli, F. de Nigris, Spontaneous cancer remission after COVID-19: insights from the pandemic and their relevance for cancer treatment, J. Transl. Med., 21 (2023), 273. https://doi.org/10.1186/s12967-023-04110-w doi: 10.1186/s12967-023-04110-w
![]() |
[7] |
M. Costanzo, M. A. R. de Giglio, G. N. Roviello, Deciphering the relationship between SARS-COV-2 and cancer, Int. J. Mol. Sci., 24 (2023), 7803. https://doi.org/10.3390/ijms24097803 doi: 10.3390/ijms24097803
![]() |
[8] |
H. Goubran, J. Stakiw, J. Seghatchian, G. Ragab, T. Burnouf, SARS-COV-2 and cancer: the intriguing and informative cross-talk, Transfus. Apher. Sci., 61 (2022), 103488. https://doi.org/10.1016/j.transci.2022.103488 doi: 10.1016/j.transci.2022.103488
![]() |
[9] |
D. Barh, S. Tiwari, L. G. R. Gomes, M. E. Weener, K. J. Alzahrani, K. Alsharif, et al., Potential molecular mechanisms of rare anti-tumor immune response by SARS-COV-2 in isolated cases of lymphomas, Viruses, 13 (2021), 1927. https://doi.org/10.3390/v13101927 doi: 10.3390/v13101927
![]() |
[10] |
O. K. Choong, R. Jakobsson, A. G. Bergdahl, S. Brunet, A. Kärmander, J. Waldenström, et al., SARS-COV-2 replicates and displays oncolytic properties in clear cell and papillary renal cell carcinoma, PLoS One, 18 (2023), e0279578. https://doi.org/10.1371/journal.pone.0279578 doi: 10.1371/journal.pone.0279578
![]() |
[11] |
E. J. Schafer, F. Islami, X. Han, L. M. Nogueira, N. S. Wagle, K. R. Yabroff, et al., Changes in cancer incidence rates by stage during the COVID-19 pandemic in the US, Int. J. Cancer, 154 (2024), 786–792. https://doi.org/10.1002/ijc.34758 doi: 10.1002/ijc.34758
![]() |
[12] |
J. P. Bounassar-Filho, L. Boeckler-Troncoso, J. Cajigas-Gonzalez, M. G. Zavala-Cerna, SARS-COV-2 as an oncolytic virus following reactivation of the immune system: a review, Int. J. Mol. Sci., 24 (2023), 2326. https://doi.org/10.3390/ijms24032326 doi: 10.3390/ijms24032326
![]() |
[13] |
D. H. Shin, A. Gillard, A. V. Wieren, C. Gomez-Manzano, J. Fueyo, Remission of liquid tumors and SARS-COV-2 infection: a literature review, Mol. Ther. Oncolytics, 26 (2022), 135–140. https://doi.org/10.1016/j.omto.2022.06.006 doi: 10.1016/j.omto.2022.06.006
![]() |
[14] | A. Donia, R. Shahid, M. Nawaz, T. Yaqub, H. Bokhari, Can we develop oncolytic SARSCOV-2 to specifically target cancer cells? Ther. Adv. Med. Oncolytics, 13 (2021), 1988. https://doi.org/10.1177/17588359211061988 |
[15] |
F. Pasin, M. M. Calveri, A. Calabrese, G. Pizzarelli, I. Bongiovanni, M. Andreoli, et al., Oncolytic effect of SARS-CoV-2 in a patient with NK lymphoma, Acta Biomed., 91 (2020), 10141. https://doi.org/10.23750/abm.v91i3.10141 doi: 10.23750/abm.v91i3.10141
![]() |
[16] |
S. Challenor, D. Tucker, SARS‐COV‐2‐induced remission of Hodgkin lymphoma, Br. J. Haematol., 192 (2021), 415. https://doi.org/10.1111/bjh.17116 doi: 10.1111/bjh.17116
![]() |
[17] |
M. Sollini, F. Gelardi, C. Carlo-Stella, A. Chiti, Complete remission of follicular lymphoma after SARS-COV-2 infection: from the "flare phenomenon" to the "abscopal effect", Eur. J. Nucl. Med. Mol. Imaging, 48 (2021), 2652–2654. https://doi.org/10.1007/s00259-021-05275-6 doi: 10.1007/s00259-021-05275-6
![]() |
[18] | E. Z. Kandeel, L. Refaat, R. Abdel-Fatah, M. Samra, A. Bayoumi, M. S. Abdellateif, et al., Could COVID-19 induce remission of acute leukemia? Hematology, 26 (2021), 870–873. https://doi.org/10.1080/16078454.2021.1992117 |
[19] |
D. Antwi-Amoabeng, M. B. Ulanja, B. D. Beutler, S. V. Reddy, Multiple myeloma remission following COVID-19: an observation in search of a mechanism (a case report), Pan Afr. Med. J., 39 (2021), 117. https://doi.org/10.11604/pamj.2021.39.117.30000 doi: 10.11604/pamj.2021.39.117.30000
![]() |
[20] |
L. Ohadi, F. Hosseinzadeh, S. Dadkhahfar, S. Nasiri, Oncolytic effect of SARS-CoV-2 in a patient with mycosis fungoides: a case report, Clin. Case Rep., 10 (2022), e05682. https://doi.org/10.1002/ccr3.5682 doi: 10.1002/ccr3.5682
![]() |
[21] | P. Agarwal, J. Nieto, D. Torres, Mathematical analysis of infectious diseases, Academic Press, 2022. |
[22] |
A. Debbouche, J. J. Nieto, D. F. M. Torres, Focus point: cancer and HIV/AIDS dynamics-from optimality to modellin, Eur. Phys. J. Plus, 136 (2021), 165. https://doi.org/10.1140/epjp/s13360-021-01154-z doi: 10.1140/epjp/s13360-021-01154-z
![]() |
[23] |
O. Nave, I. Hartuv, U. Shemesh, Θ-SEIHRD mathematical model of Covid19-stability analysis using fast-slow decomposition, PeerJ, 8 (2020), e10019. https://doi.org/10.7717/peerj.10019 doi: 10.7717/peerj.10019
![]() |
[24] |
A. Atifa, M. A. Khan, K. Iskakova, F. S. Al-Duais, I. Ahmad, Mathematical modeling and analysis of the SARS-Cov-2 disease with reinfection, Comput. Biol. Chem., 98 (2022), 107678. https://doi.org/10.1016/j.compbiolchem.2022.107678 doi: 10.1016/j.compbiolchem.2022.107678
![]() |
[25] | J. H. Rojas, M. Paredes, M. Banerjee, O. Akman, A. Mubayi, Mathematical modeling and dynamics of SARS-COV-2 in Colombia, Lett. Biomath., 9 (2022), 41-–56. |
[26] |
O. Nave, M. Sigron, A mathematical model for cancer treatment based on combination of anti-angiogenic and immune cell therapies, Results Appl. Math., 16 (2022), 10030. https://doi.org/10.1016/j.rinam.2022.100330 doi: 10.1016/j.rinam.2022.100330
![]() |
[27] |
A. M. Elaiw, A. D. A. Agha, Global dynamics of SARS-CoV-2/cancer model with immune responses, Appl. Math. Comput., 408 (2021), 126364. https://doi.org/10.1016/j.amc.2021.126364 doi: 10.1016/j.amc.2021.126364
![]() |
[28] |
A. M. Elaiw, A. D. Hobiny, A. D. A. Agha, Global dynamics of reaction-diffusion oncolytic M1 virotherapy with immune response, Appl. Math. Comput., 367 (2020), 124758. https://doi.org/10.1016/j.amc.2019.124758 doi: 10.1016/j.amc.2019.124758
![]() |
[29] |
J. Malinzi, P. Sibanda, H. Mambili-Mamboundou, Analysis of virotherapy in solid tumor invasion, Math. Biosci., 263 (2015), 102–110. https://doi.org/10.1016/j.mbs.2015.01.015 doi: 10.1016/j.mbs.2015.01.015
![]() |
[30] |
T. Alzahrani, R. Eftimie, D. Trucu, Multiscale modelling of cancer response to oncolytic viral therapy, Math. Biosci., 310 (2019), 76–95. https://doi.org/10.1016/j.mbs.2018.12.018 doi: 10.1016/j.mbs.2018.12.018
![]() |
[31] | K. W. Okamoto, P. Amarasekare, I. T. D. Petty, Modeling oncolytic virotherapy: is complete tumor-tropism too much of a good thing? J. Theor. Biol., 358 (2014), 166–178. https://doi.org/10.1016/j.jtbi.2014.04.030 |
[32] |
J. Zhao, J. P. Tian, Spatial model for oncolytic virotherapy with lytic cycle delay, Bull. Math. Biol., 81 (2019), 2396–2427. https://doi.org/10.1007/s11538-019-00611-2 doi: 10.1007/s11538-019-00611-2
![]() |
[33] |
Z. Wang, Z. Guo, H. Peng, A mathematical model verifying potent oncolytic efficacy of M1 virus, Math. Biosci., 276 (2016), 19–27. https://doi.org/10.1016/j.mbs.2016.03.001 doi: 10.1016/j.mbs.2016.03.001
![]() |
[34] | R. G. Bartle, D. R. Sherbert, Introduction to real analysis, John Wiley & Sons, Inc., 2000. |
[35] | E. Jones, P. Romemer, M. Raghupathi, S. Pankavich, Analysis and simulation of the three-component model of HIV dynamics, arXiv, 2013. https://doi.org/10.48550/arXiv.1312.3671 |
[36] | H. L. Smith, P. Waltman, The theory of the chemostat: dynamics of microbial competition, Cambridge University Press, 1995. https://doi.org/10.1017/CBO9780511530043 |
[37] | H. K. Khalil, Nonlinear Systems, Prentice-Hall, 1996. |
[38] |
T. Sumi, K. Harada, Immune response to SARS-CoV-2 in severe disease and long COVID-19, iScience, 25 (2022), 104723. https://doi.org/10.1016/j.isci.2022.104723 doi: 10.1016/j.isci.2022.104723
![]() |
[39] |
C. Li, J. Xu, J. Liu, Y. Zhou, The within-host viral kinetics of SARS-CoV-2, Math. Biosci. Eng., 17 (2020), 2853–2861. https://doi.org/10.3934/mbe.2020159 doi: 10.3934/mbe.2020159
![]() |