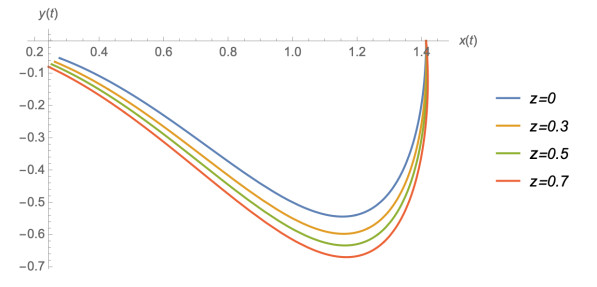
This paper examines the propagation dynamics of a T-lymphoblastic leukemia virus type Ⅰ (HTLV-1) infection model in a stochastic environment combined with an Ornstein-Uhlenbeck process. In conjunction with the theory of Lyapunov functions, we initially demonstrate the existence of a unique global solution to the model when initial values are positive. Subsequently, we establish a sufficient condition for the existence of a stochastic model stationary distribution. Based on this condition, the local probability density function expression of the model near the quasi-equilibrium point is solved by combining it with the Fokker-Planck equation. Subsequently, we delineate the pivotal conditions that precipitate the extinction of the disease. Finally, we select suitable data for numerical simulation intending to corroborate the theorem previously established.
Citation: Yan Ren, Yan Cheng, Yuzhen Chai, Ping Guo. Dynamics and density function of a HTLV-1 model with latent infection and Ornstein-Uhlenbeck process[J]. AIMS Mathematics, 2024, 9(12): 36444-36469. doi: 10.3934/math.20241728
[1] | Stelios Arvanitis, Michalis Detsis . Mild explocivity, persistent homology and cryptocurrencies' bubbles: An empirical exercise. AIMS Mathematics, 2024, 9(1): 896-917. doi: 10.3934/math.2024045 |
[2] | Zhe Su, Yiying Tong, Guo-Wei Wei . Persistent de Rham-Hodge Laplacians in Eulerian representation for manifold topological learning. AIMS Mathematics, 2024, 9(10): 27438-27470. doi: 10.3934/math.20241333 |
[3] | Li Shen, Jian Liu, Guo-Wei Wei . Evolutionary Khovanov homology. AIMS Mathematics, 2024, 9(9): 26139-26165. doi: 10.3934/math.20241277 |
[4] | Mohamed Said Mohamed, Najwan Alsadat, Oluwafemi Samson Balogun . Continuous Tsallis and Renyi extropy with pharmaceutical market application. AIMS Mathematics, 2023, 8(10): 24176-24195. doi: 10.3934/math.20231233 |
[5] | Yunan He, Jian Liu . Multi-scale Hochschild spectral analysis on graph data. AIMS Mathematics, 2025, 10(1): 1384-1406. doi: 10.3934/math.2025064 |
[6] | Sergio Iglesias-Perez, Alberto Partida, Regino Criado . The advantages of k-visibility: A comparative analysis of several time series clustering algorithms. AIMS Mathematics, 2024, 9(12): 35551-35569. doi: 10.3934/math.20241687 |
[7] | Ana Lazcano de Rojas, Miguel A. Jaramillo-Morán, Julio E. Sandubete . EMDFormer model for time series forecasting. AIMS Mathematics, 2024, 9(4): 9419-9434. doi: 10.3934/math.2024459 |
[8] | Ana Lazcano de Rojas . Data augmentation in economic time series: Behavior and improvements in predictions. AIMS Mathematics, 2023, 8(10): 24528-24544. doi: 10.3934/math.20231251 |
[9] | J. F. Torres, M. Martinez-Ballesteros, A. Troncoso, F. Martinez-Alvarez . Advances in time series forecasting: innovative methods and applications. AIMS Mathematics, 2024, 9(9): 24163-24165. doi: 10.3934/math.20241174 |
[10] | Sunil Kumar, Amit Kumar , Zaid Odibat, Mujahed Aldhaifallah, Kottakkaran Sooppy Nisar . A comparison study of two modified analytical approach for the solution of nonlinear fractional shallow water equations in fluid flow. AIMS Mathematics, 2020, 5(4): 3035-3055. doi: 10.3934/math.2020197 |
This paper examines the propagation dynamics of a T-lymphoblastic leukemia virus type Ⅰ (HTLV-1) infection model in a stochastic environment combined with an Ornstein-Uhlenbeck process. In conjunction with the theory of Lyapunov functions, we initially demonstrate the existence of a unique global solution to the model when initial values are positive. Subsequently, we establish a sufficient condition for the existence of a stochastic model stationary distribution. Based on this condition, the local probability density function expression of the model near the quasi-equilibrium point is solved by combining it with the Fokker-Planck equation. Subsequently, we delineate the pivotal conditions that precipitate the extinction of the disease. Finally, we select suitable data for numerical simulation intending to corroborate the theorem previously established.
In a seminal paper in 1964, Buchdahl considered [1] a class of metrics on a (3+1)-dimensional spherically symmetric static spacetime in a general relativity framework [2]. In terms of the so-called isotropic coordinates (t,r,θ,ϕ), this family of metrics is given by
ds2=(1−f(r))2(1+f(r))2dt2−(1+f(r))4[dr2+r2(dθ2+sin2θdϕ2)], | (1.1) |
where the function f(r) had to be determined and units are chosen such that the speed of light is normalized to c=1. This equation was shown to correspond to a relativistic fluid sphere. Under some additional requirements, the field equations gave rise to the following scalar second-order nonlinear ordinary differential equation (ODE):
d2fdr2−3f(dfdr)2−1rdfdr=0, | (1.2) |
which admits the exact solution (see [1])
f(r)=±1k1√1+k2r2, | (1.3) |
where k1, k2 are the two integration constants. Note that Eq (1.2) can be written alternatively in terms of total derivatives as
ddr(1rddr(1f2))=0. |
The Buchdahl equation (1.1) has also been considered in a different context, namely that of nonlinear ODEs with time- and position-dependent coefficients, by replacing the radial function f(r) by x(t) and interpreting t as the time [3,4,5,6], leading to the modified Buchdahl equation
d2xdt2=3x(dxdt)2+1tdxdt. | (1.4) |
In this form, the equation has been studied in the aforementioned works using several different methods in [3, Example 2], [5, Example 1], and [6, Example 3.6].
In the general context of nonlinear ODEs, Eq (1.4) is nothing but a special case of the generic equation
d2xdt2=a(x)(dxdt)2+b(t)dxdt, | (1.5) |
where a(x) and b(t) are arbitrary functions of their arguments. This generalization can also be regarded as the equation of the motion of a dynamical system with variable coefficients a(x) and b(t), depending on the velocity/momentum terms. It is not difficult to see that, by dividing by
˙x=dxdt, |
the equation* can be rewritten as
*In other terms, the equation admits an integrating factor.
ddtln|˙x|=ddt(∫xa(ξ)dξ)+ddt(∫tb(τ)dτ), | (1.6) |
showing that (1.5) is an exact equation, the solution of which is recovered from the expression
ln|˙x|=∫xa(ξ)dξ+∫tb(τ)dτ+k1 | (1.7) |
using quadratures, where k1 is a constant of integration [7,8,9]. It can be further shown that for any choices of a(x) and b(t), Eq (1.5) is linearizable by a point transformation, i.e., it admits a maximal Lie algebra of point symmetries isomorphic to sl(3,R) [10]†. However, as the point transformation is not canonical, it may change the physical meaning of the dependent and independent variables, and thus this does not constitute the most appropriate strategy for some applications. On the other hand, the first-order equation (1.7) may not always be explicitly integrable, in the sense that x can be expressed in terms of the elementary functions of the independent variable t.
†In Appendix, the symmetry generators are explicitly given.
As already mentioned, in various physical applications, the use of (1.6) may not be computationally satisfactory, and an alternative approach to derive exact solutions (whenever possible) is required. In this context, it was observed in [11,12] that the Eq (1.4), when interpreted as a first-order system of ODEs, possesses the supplementary structure of a Lie–Hamilton (LH) system associated with the so-called "book algebra" b2 (see [12,13,14] and references therein). It was further shown in [14] that any LH system based on this Lie algebra can be integrated by quadratures, a property that also holds, under certain constraints, for its quantum deformation [15].
The structure of the paper is as follows. In Section 2, we reconsider the generalized Buchdahl equation (1.5) from the point of view of LH systems, determining the general solution by means of the associated book Lie algebra b2, following the general ansatz proposed in [14]. The procedure is illustrated through some special types of the generalized Buchdahl equations in Section 3. In particular, it is shown that no further extension of the generalized Buchdahl equation as an LH system based on b2 is possible. In Section 4, we study the Poisson–Hopf deformation of the generalized Buchdahl equations associated with the quantum book algebra [15], showing that the corresponding equations can also be solved exactly, and then apply such results in Section 5 to the special cases considered before in Section 3, with special emphasis on the first-order approximation in the quantum deformation parameter z. It is shown that these equations can also be solved exactly. In Section 6, we extrapolate the results thus obtained to the oscillator algebra h4 extending b2, finding that the corresponding systems can still be solved exactly. The procedure is illustrated by considering the extension of the special cases studied in Section 3. In Section 7, their Poisson–Hopf deformation, based on the "nonstandard" quantum deformation of h4, is analyzed, also obtaining its general solution, which is a completely novel result. In addition, it is applied to the systems in Section 5, arriving at their extended version. In Section 8, the physical and mathematical significance of the quantum deformation parameter z is considered in more detail. Specifically, it is shown that for higher-dimensional deformations, the corresponding Hamiltonian equations exhibit interaction terms that are intrinsic to the deformation, meaning that the equations of the motion are no longer copies of the original one-dimensional system. Finally, in a concluding section, we summarize the results obtained concerning the (deformed) generalized Buchdahl equations based on b2 and h4, also highlighting how these systems are related by a limiting process. Moreover, the existence of a Lagrangian formalism for any (quantum deformed) equation obtained from (1.4) is indicated. Some conclusions are drawn, and possible future developments of the method in connection with the embedding of the book algebra into either the simple Lie algebra sl(2,R)⊃b2 or the two-photon algebra h6⊃h4⊃b2 are briefly discussed.
We call (1.5) the generalized Buchdahl equation, as considered in [6]. For the special choice
a(x)=3/xandb(t)=1/t, |
the classical Buchdahl equation is recovered. As shown in [6], Eq (1.5) admits both a standard and reciprocal Lagrangian description for arbitrary choices of the parameter functions. The generalized equation, besides its applications in the context of general relativity, can also be interpreted as the equation of the motion for nonlinear systems with variable coefficients.
We first reinterpret the generalized Buchdahl equation in the context of the LH system framework, deriving its general solution in appropriate coordinates after a canonical transformation, and we analyze some relevant particular cases in Section 3. Although this approach does not supply essential new information (the equation is solved by (1.6)), it is relevant for the study of perturbations or quantum deformations of the equation, which are, in general, no longer exact and linearizable by a point transformation, and where the application of the traditional direct integration methods may be too cumbersome.
Considering the variable
y≡dxdt, |
Equation (1.5) is equivalent to the first-order nonlinear system of ODEs
dxdt=y,dydt=a(x)y2+b(t)y. | (2.1) |
This system can further be expressed in terms of the t-dependent vector field
Xt=X1+b(t)X2, | (2.2) |
where the vector fields X1 and X2 are explicitly given by
X1=y∂∂x+a(x)y2∂∂y,X2=y∂∂y. | (2.3) |
These vector fields satisfy the Lie bracket
[X2,X1]=X1; | (2.4) |
hence they span a Lie algebra isomorphic to the book algebra b2‡. The generator X2 can be seen as a dilation, while X1 corresponds to a translation. Formally, (2.1) and (2.2) define a Lie system [16,17,18] with a noninvariance Lie algebra (called Vessiot–Guldberg Lie algebra) isomorphic to the "book" Lie algebra b2. It can actually be shown (see e.g., [9]) that the ODE (1.5) is the most general second-order scalar equation that admits b2 as a Vessiot–Guldberg algebra. However, the generalized Buchdahl equation is more than a mere Lie system, as it admits a symplectic form ω such that the vector fields Xi are Hamiltonian vector fields with respect to the appropriate Hamiltonian functions hi (i=1,2). The compatibility conditions
‡This is the same as the affine algebra in two dimensions.
LXiω=0,ιXiω=dhi, | (2.5) |
with ι denoting the contraction or inner product of ω, determine the structure of an LH system (see [13] and the references therein)§.
§According to the classification of LH systems on R2 [11,12], the book Lie algebra b2 is locally diffeomorphic to the imprimitive class I14A with r=1 and η1(x)=ex.
In this case, the symplectic form is given by
ω=exp(−∫xa(ξ)dξ)ydx∧dy. | (2.6) |
Hereafter, it will be assumed that y≠0. The Hamiltonian functions associated with the Hamiltonian vector fields Xi are
h1=yexp(−∫xa(ξ)dξ),h2=−∫xexp(−∫ξa(ξ′)dξ′)dξ, | (2.7) |
which satisfy the following Poisson bracket with respect to ω (see (2.4)):
{h2,h1}ω=−h1. |
Hence, the LH system (2.2) has a t-dependent Hamiltonian given by
ht=h1+b(t)h2, | (2.8) |
whose Hamilton equations with respect to the noncanonical symplectic form ω (2.6) give rise to Eq (2.1)
dxdt={x,ht}ω=yexp(−∫xa(ξ)dξ)∂ht∂y,dydt={y,ht}ω=−yexp(−∫xa(ξ)dξ)∂ht∂x. |
We observe that all the structures above are properly defined on R2y≠0.
LH systems related to the book algebra were generically studied in [11], with nonlinear superposition rules being given in [12], using an appropriate embedding of b2 into a higher-dimensional LH algebra. The generalized Buchdahl equation is one particular case of an ample class of b2-LH systems that comprise, among others, complex Bernoulli differential equations (with t-dependent real coefficients) [14], some Lotka–Volterra systems, and various types of time-dependent epidemic models with stochastic fluctuations [19], for which an exact solution can be found using an explicit diffeomorphism (corresponding to an appropriate change of variables) that leads to a canonical realization of the LH algebra (see [14,19] for further details).
As a shorthand notation, we define the function
Ξ(x):=exp(−∫xa(ξ)dξ), |
so that the expressions (2.6) and (2.7) read
ω=Ξ(x)ydx∧dy,h1=yΞ(x),h2=−∫xΞ(ξ)dξ. | (2.9) |
Now, under the change of variables
q=−yΞ(x),p=1yΞ(x)−1∫xΞ(ξ)dξ, | (2.10) |
the Buchdahl equation, as presented above, can be reformulated in terms of the b2-LH algebra in the canonical form introduced in [20] (see also [14,19])), which differs considerably from that given in the works [11,12], although both are (locally) diffeomorphic. In this context, the coordinates (q,p) are canonical, and the symplectic form (2.6) and Hamiltonian functions (2.7) become
ω≡ωcan=dq∧dp, | (2.11) |
h1=−q,h2=qp,{h2,h1}ωcan=−h1, | (2.12) |
while the t-dependent Hamiltonian (2.8) reduces to
ht=h1+b(t)h2=−q+b(t)qp. | (2.13) |
The corresponding equations of the motion are given by
dqdt={q,ht}ωcan=b(t)q,dpdt={p,ht}ωcan=1−b(t)p, | (2.14) |
which is a linear, uncoupled system. We observe that a(x) does not appear explicitly in (2.14) (compare this with (2.1)), as it is "hidden" within the transformation (2.10) through Ξ(x). The Hamiltonian vector fields (2.3), when expressed in the canonical variables, are
X1=∂∂p,X2=q∂∂q−p∂∂p, | (2.15) |
which satisfy the Lie commutator (2.4) and provide the same LH system (2.14). As follows from (2.14), the generalized Buchdahl LH system (2.1) is separable in the coordinates (q,p), and can be easily solved by quadratures as follows:
q(t)=c1eγ(t),γ(t):=∫tb(τ)dτ,p(t)=(c2+∫teγ(τ)dτ)e−γ(t), | (2.16) |
where c1 and c2 are the two constants of integration determined by the initial conditions. These results are summarized in the following statement.
Proposition 2.1. The general solution of the generalized second-order Buchdahl equation (1.5) and its associated first-order system of ODEs (2.1) is given by
∫xΞ(ξ)dξ=−c1(c2+∫teγ(τ)dτ),Ξ(x):=exp(−∫xa(ξ)dξ),y(t)=dxdt=−c1eγ(t)Ξ(x), γ(t):=∫tb(τ)dτ, | (2.17) |
where c1 and c2 are the two constants of integration determined by the initial conditions.
Observe, in particular, that the first equation of (2.17) can also be expressed as
ddt(e−γ(t)ddt∫xΞ(ξ)dξ)=0 ≡ ddt(e−γ(t)Ξ(x)dxdt)=0, |
which, taking into account that
ddte−γ(t)=−e−γ(t)b(t),ddxΞ(x)=−Ξ(x)a(x),ddtΞ(x)=−Ξ(x)a(x)dxdt, |
reproduces the initial generalized second-order Buchdahl equation (1.5).
In the context of nonlinear dynamical systems with variable coefficients, the previous result can be applied directly by means of appropriate choices for the parameter functions a(x) and b(t). In this situation, the functions Ξ and γ are obtained from (2.17), reducing the problem to the integration of x(t), while y(t) follows directly by insertion of x(t) into the second equation, providing the solution of system (2.1).
We illustrate the procedure in terms of LH systems by studying some particular cases corresponding to explicit choices for the parameter functions a(x) and b(t).
The equation arises from the specific choices
a(x)=3x,b(t)=1t,x∈R∗,t∈R∗ | (3.1) |
in (2.1), so that the functions Ξ(x) and γ(t) in (2.17) are given by
Ξ(x)=x−3,γ(t)=lnt. |
Note that
eγ(t)=t, |
which in (2.17), is therefore always well-defined. It follows that the symplectic form and Hamiltonian functions (2.9) are given by
ω=1x3ydx∧dy,h1=yx3,h2=12x2. |
Substituting these expressions into the first equation of (2.17), we find that
1x2(t)=2c1c2+c1t2, |
from which the general solution
x(t)=±1√2c1c2+c1t2,y(t)=∓c1t(2c1c2+c1t2)3/2 | (3.2) |
is obtained, recovering the expression (1.3) of the Buchdahl equation (1.4) with
c1=k21k2,c2=12k2andf(r)≡x(t). |
As a first natural generalization in (2.1), we consider the function
a(x)=x−1 |
and an arbitrary b(t). It follows that
Ξ(x)=x−1,x>0, |
with γ(t) as given in (2.17). The symplectic form and Hamiltonian functions (2.9) read
ω=1xydx∧dy,h1=yx,h2=−lnx. |
The first equation in (2.17) provides
lnx(t)=−c1(c2+∫teγ(τ)dτ), |
which can be easily solved, leading to the exact solution
x(t)=exp(−c1c2−c1∫teγ(τ)dτ),y(t)=−c1eγ(t)exp(−c1c2−c1∫teγ(τ)dτ). |
For instance, choosing b(t)=1/t with t∈R∗ (i.e., eγ(t)=t), we find that
x(t)=exp(−c1c2−c1t22),y(t)=−c1texp(−c1c2−c1t22), | (3.3) |
which can be compared with (3.2).
We now choose
a(x)=αx−1 |
with α∈R∗ and α≠1, keeping b(t) arbitrary, so that
Ξ(x)=x−α,x∈R∗. |
Hence the expression (2.9) gives rise to
ω=1xαydx∧dy,h1=yxα,h2=−x1−α1−α,α≠1. |
The first equation in (2.17) reads
x1−α(t)1−α=−c1(c2+∫teγ(τ)dτ), |
providing the following exact solution:
x(t)=((α−1)c1(c2+∫teγ(τ)dτ))11−α,y(t)=−c1eγ(t)((α−1)c1(c2+∫teγ(τ)dτ))α1−α. |
As a particular case, taking b(t)=1/t with t∈R∗, we find that
x(t)=((α−1)c1(c2+t22))11−α,y(t)=−c1t((α−1)c1(c2+t22))α1−α, |
which, as expected, reduces for α=3 to the solution (3.2) of the classical Buchdahl equation.
Despite the previous particular cases determined by Proposition 2.1, for which exact solutions have been presented in an explicit form, it is worth observing that, in general, the first equation in (2.17) does not provide an explicit expression for x(t), as it may not be expressible in terms of the usual elementary functions.
The simplest choice for which this pattern is observed corresponds to polynomial choices of a(x); more precisely
a(x)=αxr,x∈R∗,α∈R∗,r∈R∗,r≠−1. |
Then we find that
Ξ(x)=exp(−αxr+1r+1) |
along with
ω=1yexp(−αxr+1r+1)dx∧dy,h1=yexp(−αxr+1r+1),h2=1r+1(αr+1)−1r+1Γ(1r+1,αxr+1r+1),r≠−1, |
where Γ(u,v) denotes the incomplete Gamma function [21]. The first equation in (2.17), which provides the general solution x(t), adopts the cumbersome implicit form
1r+1(αr+1)−1r+1Γ(1r+1,αxr+1r+1)=c1(c2+∫teγ(τ)dτ). |
For general values of α, this expression cannot be solved explicitly with respect to x(t), and alternative methods, like the Lie series [22], have to be applied to determine the solution of the system.
Taking into account the b2-LH algebra's symmetry (see (2.1)–(2.8)) of the generalized Buchdahl system, it is rather natural to analyze whether it is possible to extend the system adding a second t-dependent arbitrary function, i.e., to consider two coefficient functions b1(t) and b2(t). We start with the first-order ODE system
dxdt=b1(t)y,dydt=b1(t)a(x)y2+b2(t)y, | (3.4) |
such that b1(t) and b2(t) are arbitrary. These equations determine a Lie system with the t-dependent vector field
Xt=b1(t)X1+b2(t)X2, |
where the t-independent vector fields Xi are defined as in (2.3). It is straightforward to verify that (3.4) also determines a b2-LH system with the Hamiltonian
ht=b1(t)h1+b2(t)h2 |
and the same Hamiltonian functions (2.7) and symplectic form (2.6).
Assuming that b1(t)≠0, we consider the following change in the coordinate y:
˜y(t)=b1(t)y(t). |
It follows that
d˜ydt=b1dydt+db1dty=b21a(x)y2+b1b2y+db1dty=a(x)˜y2+b(t)˜y, |
where
b(t)=b2(t)+1b1(t)db1dt. |
In this case, the system (3.4) is equivalent to (2.1), showing that the latter cannot be generalized as an LH system based on the LH algebra b2, consistent with the results derived in [9].
Starting from the quantum deformation of the book algebra, which is briefly recalled, in this section, we obtain the deformed counterpart of the generalized Buchdahl system (2.1), together with its corresponding general solution, thus extending Proposition 2.1 to an arbitrary quantum deformation parameter z, while keeping the parameter functions b(t) and a(x) arbitrary.
The quantum algebra deformation (see [23,24] for details) of the book Lie algebra b2 is denoted by
Uz(b2)≡bz,2, |
where z is the quantum deformation real parameter (usually q=ez). Its Hopf structure is defined by the following deformed coproduct map Δz and compatible commutation relation in an "abstract" basis (v1,v2):
Δz(v1)=v1⊗1+1⊗v1,Δz(v2)=v2⊗e−zv1+1⊗v2,[v2,v1]z=−1−e−zv1z, | (4.1) |
such that Δz is an algebra homomorphism and satisfies the coassociativity condition
(Id⊗Δz)Δz=(Δz⊗Id)Δz. |
The pair (bz,2,Δz) thus defines a coalgebra structure (see [14,15] and references therein for more details). In the following, we apply to b2 the formalism of Poisson–Hopf deformations of LH systems introduced in [20,25], from which a deformation of the generalized Buchdahl system (2.1) will be derived.
A deformed symplectic representation Dz of bz,2 (4.1) in terms of the canonical variables (q,p) of Section 2.1 and the canonical symplectic form (2.11) is given by
hz,1:=Dz(v1)=−q,hz,2:=Dz(v2)=(ezq−1z)p, | (4.2) |
where the corresponding deformed Poisson bracket with respect to ωcan is
{hz,2,hz,1}ωcan=e−zhz,1−1z. |
From the relation
ιXz,iωcan=dhz,i, |
we compute the corresponding deformation of the vector fields (2.15)
Xz,1=∂∂p,Xz,2=(ezq−1z)∂∂q−ezqp∂∂p, |
which span a smooth distribution in the sense of Stefan–Sussmann [26,27,28] through the commutator
[Xz,2,Xz,1]=ezqXz,1. |
The invariance condition of ωcan in (2.11) under the Lie derivative (2.5) is trivially satisfied.
This leads to the deformed t-dependent Hamiltonian and t-dependent vector field (compare this with Eq (2.13))
hz,t=hz,1+b(t)hz,2=−q+b(t)(ezq−1z)p,Xz,t=Xz,1+b(t)Xz,2=∂∂p+b(t)((ezq−1z)∂∂q−ezqp∂∂p), |
where b(t) is an arbitrary real parameter function. The associated first-order system of nonautonomous nonlinear and coupled ODEs on R2 is given by
dqdt=b(t)(ezq−1z),dpdt=1−b(t)ezqp. | (4.3) |
For the limit z→0, the system (2.14) is recovered¶. As the first of the equations above is separable, it can be easily solved, and substitution into the second equation yields, after a quadrature, the general solution (see [14,15])
¶All the deformed expressions above reduce to (2.12)–(2.16) concerning the classical b2-LH algebra.
q(t)=−ln(1−zc1eγ(t))z,γ(t):=∫tb(τ)dτ,p(t)=(e−γ(t)−zc1)(c2+∫t1e−γ(τ)−zc1dτ), | (4.4) |
where c1 and c2 are the two constants of integration determined by the initial conditions. Observe that the first equation in (4.4) can be expressed alternatively as
1−e−zq(t)z=c1eγ(t). |
It is worth observing that the presence of the quantum deformation parameter z can be regarded as the introduction of a perturbation in the classical b2-LH system (2.14), in such a manner that a nonlinear interaction or coupling between the variables (q,p) in the deformed b2-LH system (4.3) arises through the term ezqp. This fact can be clearly appreciated by taking a power series expansion in z of (4.3) and truncating at the first order, leading to the system
dqdt=b(t)(q+12zq2)+o[z2],dpdt=1−b(t)(p+zqp)+o[z2], | (4.5) |
which hold for a small value of z. In this approximation, we find that z introduces a quadratic term q2 in the first equation of (4.5), leading to a real Bernoulli equation, while the second equation is linear in p, once the value of q has been obtained. This enables us to integrate the system by quadratures, allowing us to obtain the general solution.
By introducing the change of variables (2.10) into the expressions of (4.2) to (4.3) with the initial canonical variables (q,p), we directly obtain the deformation of the generalized Buchdahl equation presented in Section 2 in the appropriate coordinates (x,y). This result is summarized as follows.
Proposition 4.1. (i) The deformation of the t-dependent generalized Buchdahl Hamiltonian (2.8) in terms of the variables (x,y) is defined by
hz,t=hz,1+b(t)hz,2,hz,1=yΞ(x),Ξ(x):=exp(−∫xa(ξ)dξ),hz,2=exp(−zyΞ(x))−1zyΞ(x)∫xΞ(ξ)dξ, | (4.6) |
such that
{hz,2,hz,1}ω=e−zhz,1−1z |
with respect to the noncanonical symplectic form ω (2.9).
(ii) The deformation of the generalized Buchdahl system (2.1) is given by
dxdt={x,hz,t}ω=y+b(t)(exp(zyΞ(x))−1−zyΞ(x)zyΞ2(x))exp(−zyΞ(x))∫xΞ(ξ)dξ,dydt={y,hz,t}ω=a(x)y2+b(t)exp(−zyΞ(x))×(exp(zyΞ(x))−1zΞ(x)+exp(zyΞ(x))−1−zyΞ(x)zΞ2(x)a(x)∫xΞ(ξ)dξ), | (4.7) |
for any arbitrary a(x), b(t), and z.
It follows that the introduction of the Poisson–Hopf deformation of the book algebra leads to the appearance of strong nonlinear interaction terms in the initial generalized Buchdahl system (2.1) determined by the quantum deformation parameter z. In this sense, note that the first equation in (4.7) is no longer equal to
dxdt=y, |
and additional functions depending on (x,y) and z, as well as the coefficient b(t) itself, enter into the deformation. At the first-order approximation in z, the system (4.7) reduces to
dxdt=y+12zyb(t)∫xΞ(ξ)dξ+o[z2],dydt=a(x)y2+b(t)y(1−12zy(Ξ(x)−a(x)∫xΞ(ξ)dξ))+o[z2], | (4.8) |
which nontrivially couples the coordinate functions.
The deformed vector fields corresponding to the Hamiltonian (4.6) are given by
Xz,t=Xz,1+b(t)Xz,2,Xz,1=y∂∂x+a(x)y2∂∂y,Xz,2=(exp(zyΞ(x))−1−zyΞ(x)zyΞ2(x))exp(−zyΞ(x))∫xΞ(ξ)dξ∂∂x+exp(−zyΞ(x))(exp(zyΞ(x))−1zΞ(x)+exp(zyΞ(x))−1−zyΞ(x)zΞ2(x)a(x)∫xΞ(ξ)dξ)∂∂y, |
which fulfill the relation (2.5) with respect to ω in (2.6) and provide the same deformed generalized Buchdahl system (4.7). These vector fields span a distribution with the commutator
[Xz,2,Xz,1]=exp(−zyΞ(x))Xz,1. |
Despite the complicated expressions of the deformed generalized Buchdahl system (4.7), we emphasize that a general solution can be derived from the exact solution for deformed book LH systems (4.4) with canonical variables and the change of coordinates (2.10). This is achieved as follows.
Proposition 4.2. The general solution of the first-order system of deformed generalized Buchdahl equations (4.7) is given by
∫xΞ(ξ)dξ=ln(1−zc1eγ(t))z(e−γ(t)−zc1)(c2+∫t1e−γ(τ)−zc1dτ),y(t)=ln(1−zc1eγ(t))zΞ(x),Ξ(x):=exp(−∫xa(ξ)dξ),γ(t):=∫tb(τ)dτ, | (4.9) |
where c1 and c2 are the two integration constants determined by the initial conditions.
Notice that, in contrast to Proposition 2.1, now y≠dx/dt (see (4.7)). As expected, under the limit z→0, we recover the undeformed/classical solution presented in Proposition 2.1. As in the classical case, one has to choose explicit expressions for a(x) and b(t) in the first equation of (4.9) and try to derive the solution x(t). The solution y(t) can be deduced from the second equation. As already mentioned, despite the algorithmic procedure, an explicit integration differs from being a trivial task in the general case.
We illustrate the results established in Propositions 4.1 and 4.2 by constructing the deformed counterpart of the particular generalized Buchdahl equations described in Sections 3.1–3.3.
We choose the functions
a(x)=3x−1 (x∈R∗) |
and
b(t)=t−1 (t∈R∗) |
as in (3.1), giving rise to
Ξ(x)=x−3 |
and
γ(t)=lnt. |
Thus the symplectic form and the deformed Hamiltonian vector fields (4.6) read
ω=1x3ydx∧dy,hz,1=yx3,hz,2=x1−exp(−zyx3)2zy. |
The deformed system of Buchdahl equation (4.7) is given by
dxdt=y+12t(xexp(−zyx3)−x41−exp(−zyx3)zy),dydt=3xy2+12t(3yexp(−zyx3)−x31−exp(−zyx3)z). | (5.1) |
The corresponding exact solution is obtained by the application of Proposition 4.2. In particular, the first equation of (4.9) directly yields the exact solution for x(t), namely
1x2(t)=2(zc1t−1)ln(1−zc1t)zt(c2−zc1t+ln(1−zc1t)z2c21). | (5.2) |
The second equation gives the following solution y(t) in terms of the result above:
y(t)=x3(t)ln(1−zc1t)z. |
The exact solution (3.2) of the proper Buchdahl equation is recovered under the undeformed limit z→0; the limit of the first factor in (5.2) leads to 2c1, while the second factor gives c2+t2/2.
Furthermore, we stress that the quantum deformation parameter z can be regarded as a small integrable perturbation parameter, similarly to (4.8). Under such a first-order approximation in z, the system (5.1) reduces to
dxdt≃y−zy4tx2,dydt≃3xy2+yt−z5y24tx3, | (5.3) |
where, for simplicity, we omit the term o[z2] for such approximations. From (5.3), and taking into account that only terms that are at most linear in z are considered, it follows that the first-order deformation of the Buchdahl equation (1.4) adopts the form
d2xdt2≃3x(dxdt)2+1tdxdt+z14t2x2dxdt. | (5.4) |
In contrast to the nondeformed equation, Eq (5.4) only admits one Lie point symmetry
Y=t∂∂t−x2∂∂x, |
showing that the maximal symmetry has been broken, i.e., the equation is no longer linearizable [10]. According to the previous discussion, the solution of (5.3) and (5.4) is given by
x(t)≃±1√2c1c2+c1t2(1+zc1t(6c2−t2)12(2c2+t2)),y(t)≃∓c1t(2c1c2+c1t2)3/2(1+zc1t(10c2+t2)4(2c2+t2)), | (5.5) |
which can be compared with Eq (3.2).
To illustrate the effect of the real deformation parameter, in Figure 1, we plot several solutions (5.5) for positive values of z, whereas Figure 2 shows the corresponding negative values.
If
a(x)=x−1, |
then
Ξ(x)=x−1 (x>0). |
From (4.6) we find that
ω=1xydx∧dy,hz,1=yx,hz,2=xlnxexp(−zyx)−1zy. |
Proposition 4.1 leads to the corresponding deformed system of Buchdahl equations for any b(t), namely
dxdt=y+b(t)xlnx(xexp(zyx)−1zy−1)exp(−zyx),dydt=y2x+b(t)(x1−exp(−zyx)z(1+lnx)−yexp(−zyx)lnx). | (5.6) |
Its exact solution, provided by Proposition 4.2, reads as
lnx(t)=ln(1−zc1eγ(t))z(e−γ(t)−zc1)(c2+∫t1e−γ(τ)−zc1dτ),y(t)=x(t)ln(1−zc1eγ(t))z. | (5.7) |
This yields a family of deformed Buchdahl-type systems, together with their exact solution, depending on the function b(t). Once the latter is fixed, it is possible to study the first-order approximation in z, analogous to the discussion in Section 5.1.
Let us choose, for example,
b(t)=1/t |
with t∈R∗ (so eγ(t)=t), as in Section 3.2. The exact solution (5.7) for Eq (5.6) reduces to
lnx(t)=(1−zc1t)ln(1−zc1t)zt(c2−zc1t+ln(1−zc1t)z2c21),y(t)=x(t)ln(1−zc1t)z. |
At the first-order in z, the system (5.6) becomes
dxdt≃y+zylnx2t,dydt≃y2x+yt+zy2(lnx−1)2tx. | (5.8) |
After some algebraic manipulation, we arrive at the approximation of the deformed second-order generalized Buchdahl equation (1.5) in the form
d2xdt2≃1x(dxdt)2+1tdxdt−zlnx2t2dxdt. | (5.9) |
As expected, the deformed equation only admits one Lie point symmetry
Y=t∂∂t+xlnx∂∂x, |
and the solution of (5.8) and (5.9) turns out to be
x(t)≃exp(−c1c2−c1t22)(1+zc21t2(c2−t26)),y(t)≃−c1texp(−c1c2−c1t22)(1+zc1t2(1+c1c2−c1t26)), |
which can be compared with the nondeformed Eq (3.3).
As a final example, we consider the function
Ξ(x)=x−α (x∈R∗) |
with α≠1. The expression (4.6) yields
ω=1xαydx∧dy,hz,1=yxα,hz,2=xexp(−zyx−α)−1zy(1−α),α≠1, |
in such a manner that the deformed system of the Buchdahl equation (4.7) is now given by
dxdt=y+b(t)x1−α(xαexp(zyx−α)−1zy−1)exp(−zyx−α),dydt=αy2x+b(t)11−α(xαexp(zyx−α)−1z−αy)exp(−zyx−α). | (5.10) |
The corresponding exact solution, obtained by the previous prescription, turns out to be
x1−α(t)=(1−α)ln(1−zc1eγ(t))z(e−γ(t)−zc1)(c2+∫t1e−γ(τ)−zc1dτ),y(t)=xα(t)ln(1−zc1eγ(t))z. | (5.11) |
As in the preceding cases, we can obtain an approximation of the deformed system at the first order in z, once an explicit function b(t) has been chosen. To compare these with the previous results, we again set
b(t)=1/t (t∈R∗). |
Under such an approximation, Eq (5.10) reads
dxdt≃y+zyx1−α2(1−α)t,dydt≃αy2x+yt−zy2x−α(1−2α)2(1−α)t, | (5.12) |
which leads to the following deformed second-order Buchdahl equation
d2xdt2≃αx(dxdt)2+1tdxdt+zx1−α2(α−1)t2dxdt. | (5.13) |
This ODE again only possesses one Lie point symmetry
Y=(1−α)t∂∂t+x∂∂x, |
showing that it is not linearizable by point transformations [10]. A solution of (5.12) and (5.13) can, however, be deduced from (5.11), showing that
x(t)≃((α−1)c1(c2+t22))11−α+z12c21t(6c2−t2)((α−1)c1(c2+t22))α1−α,y(t)≃−z12c31t2(6c2(2α−1)+t2(2α−3))((α−1)c1(c2+t22))2α−11−α−c1t((α−1)c1(c2+t22))α1−α. |
Many other particular equations and their solutions can be analyzed by applying the general results described in Propositions 4.1 and 4.2.
So far, we have obtained (deformed) generalized Buchdahl equations from the (quantum) book algebra b2 in terms of the arbitrary functions a(x) and b(t). Moreover, we have proven, in Section 3.5, that there is no possible extension of the generalized Buchdahl equation (2.1) from b2, i.e., from this underlying symmetry, no additional t-dependent coefficient can be considered in a nontrivial way.
Nevertheless, as b2 has arisen as a subalgebra of other higher-dimensional Lie algebras, it is natural to extend the method of exact solutions to other LH systems (and their corresponding Poisson–Hopf deformations) that keep b2 as an LH subalgebra. From the classification of LH systems on the plane (see [11,12]), it follows that the relevant candidates are the oscillator h4, sl(2,R) and the so-called two-photon h6 LH algebras.
Taking into account the canonical representation (2.12), we find that the simplest extension is provided by the oscillator LH algebra, corresponding to the imprimitive class I8 in [11,12], which entails the introduction of an additional nontrivial arbitrary t-dependent coefficient. Furthermore, the corresponding exact solution is straightforward, allowing us to apply this result to the framework of Buchdahl equations, which we develop in what follows. We recall that the embedding b2⊂h4 has already been used in the context of t-dependent epidemic models in [19]. However, we stress that an exact solution can also be deduced for the deformed counterpart from a quantum oscillator algebra, which, to best of our knowledge, has been lacking but will be addressed in the next section.
Thus, we start with the oscillator h4-LH algebra with Hamiltonian functions, expressed in canonical coordinates (q,p) as [11,12]
h1=−q,h2=qp,h3=p,h0=1, | (6.1) |
obeying the commutation relations
{h2,h1}ωcan=−h1,{h2,h3}ωcan=h3,{h3,h1}ωcan=h0,{h0,⋅}ωcan=0, | (6.2) |
with respect to the canonical symplectic form (2.11). Hence, h2 can be regarded as the number generator, h1, h3 as lowering/raising generators, and h0 as the central element (which is necessary to close the brackets). The extension of the t-dependent Hamiltonian (2.13) in terms of two arbitrary t-dependent functions
b1(t)≡b(t) |
and b2(t) yields
ht=h1+b1(t)h2+b2(t)h3=−q+b1(t)qp+b2(t)p, | (6.3) |
giving rise to the Hamilton equations
dqdt={q,ht}ωcan=b1(t)q+b2(t),dpdt={p,ht}ωcan=1−b1(t)p, | (6.4) |
which again form a linear and uncoupled system.
The associated Hamiltonian vector fields are obtained from (6.1) through the relation (2.5) and read as
X1=∂∂p,X2=q∂∂q−p∂∂p,X3=∂∂q, | (6.5) |
which satisfy the Lie commutators
[X2,X1]=X1,[X2,X3]=−X3,[X1,X3]=0. | (6.6) |
Therefore, the Vessiot–Guldberg Lie algebra is isomorphic to the (1+1)-dimensional Poincaré algebra on a light-cone basis, with X2 playing the role of the boost generator, and X1, X3 as translations. In fact, if (q,p) are identified with the light-like coordinates (x+,x−), the vector fields (6.5) are just the Killing vector fields of the metric
ds2=dx+dx− |
in Minkowskian spacetime.
The oscillator LH system (6.4) can then be solved directly, leading to the following exact solution in terms of the two integration constants c1 and c2:
q(t)=(c1+∫te−γ(τ)b2(τ)dτ)eγ(t),γ(t):=∫tb1(τ)dτ,p(t)=(c2+∫teγ(τ)dτ)e−γ(t). | (6.7) |
The results above can be applied in a straightforward manner to the generalized Buchdahl equations by applying the change of coordinates (2.10). The Hamiltonian function (6.1) and the vector fields (6.5) turn out to be
h1=yΞ(x),h2=−∫xΞ(ξ)dξ,h3=1yΞ(x)∫xΞ(ξ)dξ,h0=1, | (6.8) |
and
X1=y∂∂x+a(x)y2∂∂y,X2=y∂∂y,X3=−1yΞ2(x)∫xΞ(ξ)dξ∂∂x−1Ξ2(x)(Ξ(x)+a(x)∫xΞ(ξ)dξ)∂∂y, | (6.9) |
with Ξ(x) as given in (2.17). They fulfill the same commutation rules (6.2) and (6.6) (the former is with respect to the symplectic form (2.6)). Observe that, as a byproduct, the t-independent vector fields Xi in (6.9) are Killing vector fields of the Minkowskian metric, now reading as
ds2=a(x)(Ξ(x)+a(x)∫xΞ(ξ)dξ)dx2+1y2∫xΞ(ξ)dξdy2−1y(Ξ(x)+2a(x)∫xΞ(ξ)dξ)dxdy. |
From ht (6.3) or
Xt=X1+b1(t)X2+b2(t)X3, |
we arrive at the extended generalized Buchdahl equations as the first-order nonlinear system of ODEs given by
dxdt=y−b2(t)1yΞ2(x)∫xΞ(ξ)dξ,dydt=a(x)y2+b1(t)y−b2(t)1Ξ2(x)(Ξ(x)+a(x)∫xΞ(ξ)dξ), | (6.10) |
(compare this with the system (2.1)). In this respect, it should be observed that consideration of the h4-LH algebra implies the introduction of a "naïve" term b2(t)p in the Hamiltonian ht (6.3), whose effect becomes quite strong in the context of the Buchdahl equations above. In particular, the coefficient b2(t) introduces complicated terms in both equations with
dxdt≠y. |
Their corresponding exact solution is provided by (6.7) and is characterized by the following "extended" version of Proposition 2.1.
Proposition 6.1. The general solution of the extended generalized Buchdahl equations (6.10), determined by the oscillator h4-LH algebra, is given by
∫xΞ(ξ)dξ=−(c1+∫te−γ(τ)b2(τ)dτ)(c2+∫teγ(τ)dτ),y(t)=−eγ(t)Ξ(x)(c1+∫te−γ(τ)b2(τ)dτ),Ξ(x):=exp(−∫xa(ξ)dξ),γ(t):=∫tb1(τ)dτ, | (6.11) |
where c1 and c2 are the two constants of integration determined by the initial conditions.
Once the coefficients b1(t), b2(t), and a(x) have been chosen, the procedure used to obtain the solution (x(t),y(t)) from Proposition 6.1 consists of calculating Ξ(x) and γ(t), introducing them in the first equation trying to compute x(t), and substituting it into the second equation, finding y(t).
We point out that the particular case with the constant
b2(t)=b0, |
covered by Proposition 6.1, is also endowed with the h4-LH algebra. Although ht (6.3) is thus composed of only two Hamiltonian functions, a third function is needed to close the Poisson brackets, leading to an LH algebra isomorphic to h4. Explicitly, if we define
h±:=h1±b0h3, |
then the commutation rules (6.2) are transformed into
{h2,h±}ω=−h∓,{h+,h−}ω=2b0h0,{h0,⋅}ω=0, |
with respect to the symplectic form (2.6), and
ht=h++b1(t)h2. |
Likewise, this particular system comes from the t-dependent vector field
Xt=X++b1(t)X2, |
where
X±:=X1±b0X3, |
such that
[X2,X±]=X∓ |
and
[X+,X−]=0, |
which is isomorphic to the Poincaré Lie algebra.
We illustrate the h4-LH systems (6.10) together with their general solution (6.11) by constructing the extensions of the particular generalized Buchdahl equations studied previously in Sections 3.1–3.3, the final results of which are summarized in Table 1.
● Case Ⅰ Extended Buchdahl equation a(x)=3x−1 b1(t)=t−1 Ξ(x)=x−3 γ(t)=lnt |
ω=1x3ydx∧dyh1=yx3h2=12x2h3=−x2y |
dxdt=y+b2(t)x42ydydt=3y2x+yt+b2(t)x32 |
x(t)=±{(c1+∫tb2(τ)τdτ)(2c2+t2)}−1/2 |
y(t)=∓t(c1+∫tb2(τ)τdτ)−1/2(2c2+t2)−3/2 |
● Case Ⅱ a(x)=x−1 arbitrary b1(t) Ξ(x)= x−1 |
ω=1xydx∧dyh1=yxh2=−lnxh3=xlnxy |
dxdt=y−b2(t)x2lnxydydt=y2x+b1(t)y−b2(t)x(1+lnx) |
x(t)=exp{−(c1+∫te−γ(τ)b2(τ)dτ)(c2+∫teγ(τ)dτ)} |
y(t)=−eγ(t)(c1+∫te−γ(τ)b2(τ)dτ)exp{−(c1+∫te−γ(τ)b2(τ)dτ)(c2+∫teγ(τ)dτ)} |
Subcase Ⅱ a(x)=x−1 b1(t)=t−1 Ξ(x)= x−1 γ(t)=lnt |
x(t)=exp{−(c1+∫tb2(τ)τdτ)(c2+t22)} |
y(t)=−t(c1+∫tb2(τ)τdτ)exp{−(c1+∫tb2(τ)τdτ)(c2+t22)} |
● Case Ⅲ a(x)=αx−1 (α≠1) arbitrary b1(t) Ξ(x)= x−α |
ω=1xαydx∧dyh1=yxαh2=−x1−α1−αh3=xy(1−α) |
dxdt=y−b2(t)x1+α(1−α)ydydt=αy2x+b1(t)y−b2(t)xα1−α |
x(t)={(α−1)(c1+∫te−γ(τ)b2(τ)dτ)(c2+∫teγ(τ)dτ)}11−α |
y(t)=−eγ(t)(c1+∫te−γ(τ)b2(τ)dτ){(α−1)(c1+∫te−γ(τ)b2(τ)dτ)(c2+∫teγ(τ)dτ)}α1−α |
Subcase Ⅲ a(x)=αx−1 (α≠1) b1(t)=t−1 Ξ(x)= x−α γ(t)=lnt |
x(t)={(α−1)(c1+∫tb2(τ)τdτ)(c2+t22)}11−α |
y(t)=−t(c1+∫tb2(τ)τdτ){(α−1)(c1+∫tb2(τ)τdτ)(c2+t22)}α1−α |
∗Note: For each case, we indicate the choice of the coefficients a(x) and b1(t) for an arbitrary b2(t), the symplectic form (2.6), the functions Ξ(x) and γ(t), the Hamiltonian functions (6.8), the system (6.10), and its corresponding general solution (6.11). |
Among all possible quantum deformations of the oscillator Lie algebra h4 [29], only the so-called nonstandard deformation, namely
Uz(h4)≡hz,4, |
enables the Hopf algebra embedding
bz,2⊂hz,4. |
In fact, hz,4 is a central extension of the nonstandard quantum (1+1) Poincaré algebra [30,31] on the light-cone basis (6.6). In what follows, we first recall the role of hz,4 in the framework of LH systems and then apply these results to the context of Buchdahl equations, arriving at the extension of the deformed generalized equations obtained in Sections 4 and 5.
The Hopf structure of hz,4 is determined by the following coproduct map and compatible commutation relations in a basis (v1,v2,v3,v0) [29]:
Δz(v1)=v1⊗1+1⊗v1,Δz(v2)=v2⊗e−zv1+1⊗v2,Δz(v3)=v3⊗e−zv1+1⊗v3+zv2⊗e−zv1v0,Δz(v0)=v0⊗1+1⊗v0,[v2,v1]z=e−zv1−1z,[v2,v3]z=v3,[v3,v1]z=e−zv1v0,[v0,⋅]z=0. | (7.1) |
In canonical variables (q,p), a deformed symplectic representation Dz of hz,4 turns out to be [31]
hz,1:=Dz(v1)=−q, hz,2:=Dz(v2)=(ezq−1z)p,hz,3:=Dz(v3)=ezqp,hz,0:=Dz(v0)=1, | (7.2) |
which fulfill the deformed Poisson brackets given by
{hz,2,hz,1}ωcan=e−zhz,1−1z,{hz,2,hz,3}ωcan=hz,3,{hz,3,hz,1}ωcan=e−zhz,1hz,0, {hz,0,⋅}ωcan=0, | (7.3) |
with respect to the canonical symplectic form (2.11).
The relation
ιXz,iωcan=dhz,i |
leads to the associated deformed Hamiltonian vector fields
Xz,1=∂∂p,Xz,2=(ezq−1z)∂∂q−ezqp∂∂p,Xz,3=ezq∂∂q−zezqp∂∂p, |
which do not close on the nonstandard quantum Poincaré algebra [30] but on a smooth Stefan–Sussmann distribution [26,27,28] given by the commutation relations [20]
[Xz,2,Xz,1]=e−zhz,1Xz,1,[Xz,2,Xz,3]=−Xz,3,[Xz,3,Xz,1]=ze−zhz,1hz,0Xz,1. | (7.4) |
Thus, we obtain a deformed t-dependent Hamiltonian and a vector field in terms of two coefficients
b1(t)≡b(t) |
and b2(t) as follows
hz,t=hz,1+b1(t)hz,2+b2(t)hz,3=−q+b1(t)(ezq−1z)p+b2(t)ezqp,Xz,t=Xz,1+b1(t)Xz,2+b2(t)Xz,3=∂∂p+b1(t)((ezq−1z)∂∂q−ezqp∂∂p)+b2(t)(ezq∂∂q−zezqp∂∂p), | (7.5) |
yielding the first-order system of nonlinear and coupled ODEs on R2 given by
dqdt=b1(t)(ezq−1z)+b2(t)ezq,dpdt=1−b1(t)ezqp−zb2(t)ezqp. | (7.6) |
The first equation can be solved directly, and by substituting into the second one, we obtain the general solution
q(t)=−1zln{1−zeγ(t)(c1+∫te−γ(τ)b2(τ)dτ)},γ(t):=∫tb1(τ)dτ,p(t)={e−γ(t)−z(c1+∫te−γ(τ)b2(τ)dτ)}×{c2+∫t(e−γ(τ)−z(c1+∫τe−γ(τ′)b2(τ′)dτ′))−1dτ}, | (7.7) |
where c1 and c2 are the two integration constants. We note that, to best of our knowledge, this exact solution has not yet been considered in the framework of LH systems. On the contrary, the usual approach is to deduce "deformed" superposition rules from t-independent constants of the motion [20]. Note also that, as expected, the expressions (6.1)–(6.7) are recovered from (7.2)–(7.7) under the limit z→0.
The first-order of the power series expansion in z of (7.6) yields
dqdt=b2(t)+b1(t)q+z(b2(t)q+12b1(t)q2)+o[z2],dpdt=1−b1(t)p−z(b2(t)p+b1(t)qp)+o[z2], |
which can be compared with (4.5).
Similarly to Section 4.2, we apply the change of variables (2.10) to the expressions (7.2) and (7.6), obtaining the deformation of the extended generalized Buchdahl equation in Section 6 from the quantum oscillator algebra hz,4.
Proposition 7.1. (i) The deformation of the t-dependent extended generalized Buchdahl Hamiltonian (6.3) is defined, in terms of the variables (x,y), by the Hamiltonian functions
hz,t=hz,1+b1(t)hz,2+b2(t)hz,3,hz,1=yΞ(x),hz,2=exp(−zyΞ(x))−1zyΞ(x)∫xΞ(ξ)dξ,hz,3=exp(−zyΞ(x))yΞ(x)∫xΞ(ξ)dξ,Ξ(x):=exp(−∫xa(ξ)dξ), | (7.8) |
which, together with
hz,0=1, |
verify the same deformed Poisson brackets (7.3), now with respect to the (noncanonical) symplectic form ω (2.9).
(ii) The Poisson–Hopf deformation of the extended generalized Buchdahl system (6.10) reads as
dxdt={x,hz,t}ω=y+b1(t)(exp(zyΞ(x))−1−zyΞ(x)zyΞ2(x))exp(−zyΞ(x))∫xΞ(ξ)dξ−b2(t)(1+zyΞ(x)yΞ2(x))exp(−zyΞ(x))∫xΞ(ξ)dξ,dydt={y,hz,t}ω=a(x)y2+b1(t)exp(−zyΞ(x))×(exp(zyΞ(x))−1zΞ(x)+exp(zyΞ(x))−1−zyΞ(x)zΞ2(x)a(x)∫xΞ(ξ)dξ)−b2(t)exp(−zyΞ(x))Ξ2(x)(Ξ(x)+(1+zyΞ(ξ))a(x)∫xΞ(ξ)dξ), | (7.9) |
for any arbitrary a(x), b1(t), b2(t), and z.
The deformed vector fields associated with the Hamiltonian functions (7.8) turn out to be
Xz,t=Xz,1+b1(t)Xz,2+b2(t)Xz,3,Xz,1=y∂∂x+a(x)y2∂∂y,Xz,2=(exp(zyΞ(x))−1−zyΞ(x)zyΞ2(x))exp(−zyΞ(x))∫xΞ(ξ)dξ∂∂x+exp(−zyΞ(x))(exp(zyΞ(x))−1zΞ(x)+exp(zyΞ(x))−1−zyΞ(x)zΞ2(x)a(x)∫xΞ(ξ)dξ)∂∂y,Xz,3=−(1+zyΞ(x)yΞ2(x))exp(−zyΞ(x))∫xΞ(ξ)dξ∂∂x−exp(−zyΞ(x))Ξ2(x)(Ξ(x)+(1+zyΞ(ξ))a(x)∫xΞ(ξ)dξ), |
that span a distribution with the same commutation rules given by (7.4).
From the general solution of the deformed extended generalized Buchdahl equations in canonical variables (7.7), we arrive at the one corresponding to the deformed system in Proposition 7.1.
Proposition 7.2. The general solution of the first-order system of deformed extended generalized Buchdahl equation (7.9) is given by
∫xΞ(ξ)dξ=1zln{1−zeγ(t)(c1+∫te−γ(τ)b2(τ)dτ)}{e−γ(t)−z(c1+∫te−γ(τ)b2(τ)dτ)}×{c2+∫t(e−γ(τ)−z(c1+∫τe−γ(τ′)b2(τ′)dτ′))−1dτ},y(t)=1zΞ(x)ln{1−zeγ(t)(c1+∫te−γ(τ)b2(τ)dτ)},Ξ(x):=exp(−∫xa(ξ)dξ),γ(t):=∫tb(τ)dτ, | (7.10) |
where c1 and c2 are the two integration constants provided by the initial conditions.
Propositions 7.1 and 7.2 state the most general results of this work, covering all the previous systems obtained so far. They can be applied to particular cases, along the same lines as in Section 5, by selecting precise functions a(x), b1(t), and b2(t). The main results concerning the extensions of the systems in Sections 5.1–5.3 are presented in Table 2, which correspond to the deformation of the h4-LH systems shown in Table 1, which are recovered when z→0. It is clear that a further analysis of the perturbations at the first order in z can be performed in a similar way to that in Section 5.
● Case Ⅰ Deformed extended Buchdahl equation a(x)=3x−1 b1(t)=t−1 Ξ(x)=x−3 γ(t)=lnt |
ω=1x3ydx∧dyhz,1=yx3hz,2=x1−exp(−zyx3)2zyhz,3=−x2yexp(−zyx3) |
dxdt=y+12t(xexp(−zyx3)−x41−exp(−zyx3)zy)+b2(t)x4+zxy2yexp(−zyx3) |
dydt=3y2x+12t(3yexp(−zyx3)−x31−exp(−zyx3)z)+12b2(t)(x3+3zy)exp(−zyx3) |
1x2(t)=2zt(zt(c1+∫tb2(τ)τdτ)−1)ln{1−zt(c1+∫tb2(τ)τdτ)} |
×(c2+∫tt(1−zt(c1+∫τb2(τ′)τ′dτ′))−1dτ) |
y(t)=x3(t)1zln{1−zt(c1+∫tb2(τ)τdτ)} |
● Case Ⅱ a(x)=x−1 arbitrary b1(t) Ξ(x)= x−1 |
ω=1xydx∧dyhz,1=yxhz,2=xlnxexp(−zyx)−1zyhz,3=xlnxyexp(−zyx) |
dxdt=y+b1(t)xlnx(xexp(zyx)−1zy−1)exp(−zyx)−b2(t)(x2+zxy)lnxyexp(−zyx) |
dydt=y2x+b1(t)(x1−exp(−zyx)z(1+lnx)−yexp(−zyx)lnx)−b2(t)(x+(x+zy)lnx)exp(−zyx) |
lnx(t)=1zln{1−zeγ(t)(c1+∫te−γ(τ)b2(τ)dτ)}(e−γ(t)−z(c1+∫te−γ(τ)b2(τ)dτ)) |
×{c2+∫t(e−γ(τ)−z(c1+∫τe−γ(τ′)b2(τ′)dτ′))−1dτ} |
y(t)=x(t)1zln(1−zeγ(t)(c1+∫te−γ(τ)b2(τ)dτ)) |
● Case Ⅲ a(x)=αx−1 (α≠1) arbitrary b1(t) Ξ(x)= x−α |
ω=1xαydx∧dyhz,1=yxαhz,2=xexp(−zyx−α)−1zy(1−α)hz,3=xy(1−α)exp(−zyx−α) |
dxdt=y+b1(t)x1−α(xαexp(zyx−α)−1zy−1)exp(−zyx−α)−b2(t)x1+α+zxy(1−α)yexp(−zyx−α) |
dydt=αy2x+b1(t)11−α(xαexp(zyx−α)−1z−αy)exp(−zyx−α)−b2(t)xα+zαy1−αexp(−zyx−α) |
x1−α(t)=(1−α)1zln{1−zeγ(t)(c1+∫te−γ(τ)b2(τ)dτ)}(e−γ(t)−z(c1+∫te−γ(τ)b2(τ)dτ)) |
×{c2+∫t(e−γ(τ)−z(c1+∫τe−γ(τ′)b2(τ′)dτ′))−1dτ} |
y(t)=xα(t)1zln(1−zeγ(t)(c1+∫te−γ(τ)b2(τ)dτ)) |
∗Note: For each case we show the coefficients a(x) and b1(t), always with an arbitrary b2(t), the symplectic form (2.6), the functions Ξ(x) and γ(t), the Hamiltonian functions (7.8), the system (7.9), and its general solution (7.10). |
As a final stage, it seems pertinent to discuss the mathematical and physical roles played by the quantum deformation parameter z in more detail.
In general, given a system, considering a quantum deformation induced by introducing z (or q=ez) implies dealing with an additional degree of freedom which, in turn, can be regarded as a modification of the initial system. One approach is to interpret this presence as a (integrable) perturbation of the initial system, as we have considered here, but, in addition, it also allows the construction of analytical models from experimental results. In other words, in some cases, it would be possible to fix a certain value of z in some quantum algebra that matches with the data for some model, arriving at an underlying quantum group symmetry and, therefore, analytical expressions. For instance, this was exactly the procedure used in [32] to determine the spectrum in quantum optical models and in [33] to describe fermion–boson interactions in a nuclear physics context.
Furthermore, the introduction of z usually leads to a coupling of the differential equations of the initial system, as shown by the explicit expressions in Sections 5 and 7; this has consequences with respect to linearization and maximal symmetry which are broken.
Beyond these comments, a quantum deformation, i.e., a Poisson–Hopf deformation of LH systems in our framework, has profound implications when constructing higher-dimensional systems. In particular, let us address this point by taking the oscillator h4-LH algebra of Section 6 and its quantum deformation hz,4 from Section 7 in canonical variables (q,p), as they give rise to the most general systems of this work.
The tool which enables one to obtain higher-dimensional systems is the coproduct map, which, for any LH algebra, is always (trivial) primitive and denoted Δ. Let (v1,v2,v3,v0) be a basis of h4, fulfilling the Lie brackets
[v2,v1]=−v1,[v2,v3]=v3,[v3,v1]=v0,[v0,⋅]=0, |
and thus they are formally similar to (6.2).
The Hopf structure is determined by the coproduct (i=0,1,2,3)
Δ(vi)=vi⊗1+1⊗vi. |
If we denote the "one-particle" symplectic representation of h4 (6.1) as
D(vi)=hi(q1,p1):=h(1)i, |
then the "two-particle" representation is obtained as [12]
(D⊗D)(Δ(v1))=h1(q1,p1)+h1(q2,p2)=−q1−q2:=h(2)1,(D⊗D)(Δ(v2))=h2(q1,p1)+h2(q2,p2)=q1p1+q2p2:=h(2)2,(D⊗D)(Δ(v3))=h3(q1,p1)+h3(q2,p2)=p1+p2:=h(2)3,(D⊗D)(Δ(v0))=h0(q1,p1)+h0(q2,p2)=1+1:=h(2)0. |
These Hamiltonian functions satisfy the same commutation relations (6.2) with respect to the canonical symplectic form
ω(2)can=dq1∧dp1+dq2∧dp2. |
In the same way, one can construct higher-dimensional representations. The relevant point is that any system with h4-LH algebra symmetry in any dimension is equivalent to considering several copies of the initial system, which is a well-known property in Lie systems; in other words, they can trivially be reduced to the initial "one-particle" one. In our case, the two-particle version of the t-dependent Hamiltonian ht (6.3) reads as
h(2)t=h(2)1+b1(t)h(2)2+b2(t)h(2)3=ht(q1,p1)+ht(q2,p2)=(−q1+b1(t)q1p1+b2(t)p1)+(−q2+b1(t)q2p2+b2(t)p2). |
This situation changes drastically when a Poisson–Hopf deformation is introduced, since the deformed coproduct Δz naturally entails a coupling of the representation [20,25]. From the deformed coproduct Δz (7.1) and representation Dz (7.2) of hz,4, we obtain its "two-particle" representation in the form
(Dz⊗Dz)(Δz(v1))=hz,1(q1,p1)+hz,1(q2,p2)=−q1−q2:=h(2)z,1,(Dz⊗Dz)(Δz(v2))=hz,2(q1,p1)e−zhz,1(q2,p2)+hz,2(q2,p2)=(ezq1−1z)p1ezq2+(ezq2−1z)p2:=h(2)z,2,(Dz⊗Dz)(Δz(v3))=hz,3(q1,p1)e−zhz,1(q2,p2)+hz,3(q2,p2)+zhz,2(q1,p1)e−zhz,1(q2,p2)hz,0(q2,p2)=ezq1p1ezq2+ezq2p2+z(ezq1−1z)p1ezq2:=h(2)z,3,(Dz⊗Dz)(Δz(v0))=hz,0(q1,p1)+hz,0(q2,p2)=1+1:=h(2)z,0. |
They fulfill the deformed commutation relations (7.3) with respect to ω(2)can. The "two-particle" version of the Hamiltonian hz,t (7.5) turns out to be
h(2)z,t=h(2)z,1+b1(t)h(2)z,2+b2(t)h(2)z,3, |
and, consequently, is no longer the sum of two copies of (7.5) as hz,t(q1,p1)+hz,t(q2,p2). The corresponding Hamilton equations are given by
dq1dt=b1(t)(ezq1−1z)ezq2+b2(t)(2ezq1−1)ezq2,dp1dt=1−b1(t)ezq1ezq2p1−2zb2(t)ezq1ezq2p1,dq2dt=b1(t)(ezq2−1z)+b2(t)ezq2,dp2dt=1−b1(t)ezq2((ezq1−1)p1+p2)−zb2(t)ezq2((2ezq1−1)p1+p2), |
showing that there are coupling terms and that the resulting system is intrinsic in the sense that it is completely different from the initial one (7.6), and hence finding that the corresponding solutions should be seen as a new problem.
In this work, using the general approach proposed in [14], the generalized Buchdahl equation has been revisited from the perspective of LH systems. Although the Buchdahl equation can be solved directly by means of integrating factors (and is further linearizable by point transformations), its reformulation in terms of a book b2-LH system is of interest, as the solution method can be extended to Poisson–Hopf deformations from the quantum algebra bz,2, providing a systematic procedure to determine the general solution of differential equations that, in general, do not admit more than one Lie point symmetry and are not related to exact equations. Further, truncation of the series expansion in the quantum deformation parameter z provides perturbations of the equation of an arbitrary order, which under certain circumstances, can also be solved explicitly. Certain special cases of the generalized Buchdahl equation and their quantum deformations have been analyzed, as well as the first-order approximations in the deformation parameter z. The next natural step, namely extending the underlying Lie algebra to the oscillator algebra h4⊃b2, provides additional generalizations of the Buchdahl equation that still preserve the property of allowing an explicit solution, including the quantum deformations hz,4⊃bz,2. An interesting question in this context is whether equivalence criteria for the first-order approximation of the deformed equations can be obtained, eventually making possible the obtainment of canonical forms of such perturbations and simplifying the computation of exact solutions.
To summarize the results obtained in this paper, the most general cases have been presented in Propositions 7.1 and 7.2, by considering the deformed oscillator algebra hz,4. From them, the different generalized systems of Buchdahl equations can be recovered through the nondeformed limit z→0 or by setting the additional t-dependent coefficient b2(t)=0, as shown in Figure 3:
As a general remark, it is worth observing that any perturbation at an arbitrary order in z of the generalized Buchdahl equation (in particular, (5.13)) always admits a Lagrangian formulation, as actually happens with any scalar second-order ODE [34]. For example, restricting it to the case of perturbations of the type
d2xdt2≃3x(dxdt)2+1tdxdt+ϕz(t,x)dxdt, | (9.1) |
such that
limz→0ϕz(t,x)=0, |
setting
u=∂L∂˙x2, |
a (nonstandard) Lagrangian L can be obtained as a solution of the first-order linear partial differential equation
∂u∂t+˙x∂u∂x+(3x˙x2+(1t+ϕz(t,x))˙x)∂u∂˙x+(6x˙x+1t+ϕz(t,x))u=0, | (9.2) |
which is deeply connected with the Jacobi multipliers [35]. Clearly, an admissible solution must satisfy the constraint that
L0=limz→0L(z,t,x,˙x) |
provides a (nonstandard) Lagrangian for the undeformed Buchdahl equation (1.4). It can be easily verified that the Lagrangian
L0=t3x6˙x−2 |
given in [6] is a particular solution of (9.2) for
ϕz(t,x)=0, |
as well as the alternative Lagrangian
L1=t3x6˙x2+kt2x6, |
where k is an arbitrary nonzero constant||.
||In this context, we observe that the second Lagrangian L2=(k˙x2t3x6+t)−1 given in [6] does not provide Eq (1.4) but the equation d2xdt2=−(3x(dxdt)2+1tdxdt).
The solution procedure is valid for other types of scalar ordinary differential equations that can be expressed as LH systems based on the book algebra b2, encompassing, among others, complex Bernoulli equations with real parameter functions, some types of Lotka–Volterra systems and various oscillator systems, as well as their corresponding quantum deformations [14,15]. The same holds for equations leading to LH systems governed by the oscillator algebra h4. In this context, a physically relevant class of differential equations that deserves a deeper analysis in connection with the LH formalism is given by the three-dimensional Hamiltonians associated with metric tensors in (3+1) dimensions, in the specific context of integrable cosmological models [36].
Finally, concerning the extension of the method of exact solutions to other LH systems (and their corresponding quantum deformations) based on Lie algebras that contains b2 as a subalgebra, let us mention that the remaining possibilities in the classification of [11,12] are the simple Lie algebra sl(2,R) and the two-photon one h6⊃h4⊃b2. However, no exact solution is yet known to be obtainable for such LH systems, so that the LH approach considered here merely provides t-independent constants of the motion and superposition rules [12,20,25], from which, eventually, the generic solution can be derived once a sufficient number of particular solutions has been determined. A detailed analysis of these further generalizations is currently in progress.
Rutwig Campoamor-Stursberg: conceptualization, methodology, investigation, and writing; Eduardo Fernández-Saiz: conceptualization, methodology, investigation, writing–review and editing; Francisco J. Herranz: methodology, investigation, software, and writing. All authors have read and agreed to the published version of the manuscript.
The authors declare they have not used Artificial Intelligence (AI) tools in the creation of this article.
Rutwig Campoamor-Stursberg and Francisco J. Herranz have been partially supported by Agencia Estatal de Investigación (Spain) under the grant PID2023-148373NB-I00 funded by MCIN/AEI/10.13039/501100011033/FEDER, UE. Francisco J. Herranz acknowledges support by the Q-CAYLE Project funded by the Regional Government of Castilla y León (Junta de Castilla y León, Spain) and by the Spanish Ministry of Science and Innovation (MCIN) through the European Union funds NextGenerationEU (PRTR C17.I1). The authors also acknowledge the contribution of RED2022-134301-T funded by MCIN/AEI/10.13039/501100011033 (Spain).
Rutwig Campoamor-Stursberg and Francisco J. Herranz are the Guest Editors of the special issue "Applications of Lie group analysis to integrability and superintegrability" for AIMS Mathematics. Rutwig Campoamor-Stursberg and Francisco J. Herranz were not involved in the editorial review and the decision to publish this article. All authors declare no conflicts of interest in this paper.
Symmetry generators of Eq (1.5)
The Lie point symmetries of the generalized Buchdahl equations are obtained using the standard Lie symmetry method [37].
Let us write
A(x)=∫xa(ξ)dξ |
and
B(t)=∫tb(τ)dτ. |
The Lie symmetry generators are given by
Y1=eA(x)∂∂x,Y2=eA(x)(∫e−A(x)dx)∂∂x,Y3=e−B(t)∂∂t,Y4=e−B(t)(∫eB(t)dt)∂∂t,Y5=eA(x)(∫eB(t)dt)∂∂x,Y6=e−B(t)(∫e−A(x)dx)∂∂t,Y7=e−B(t)(∫e−A(x)dx)(∫eB(t)dt)∂∂t+eA(x)(∫e−A(x)dx)2∂∂x,Y8=e−B(t)(∫eB(t)dt)2∂∂t+eA(x)(∫e−A(x)dx)(∫eB(t)dt)∂∂x. |
The corresponding nontrivial commutators are
[Y1,Y2]=Y1,[Y1,Y6]=Y3,[Y1,Y7]=Y4+2Y2,[Y1,Y8]=Y5,[Y2,Y5]=−Y5,[Y2,Y6]=Y6,[Y2,Y7]=Y7,[Y3,Y4]=Y3,[Y3,Y5]=Y1,[Y3,Y7]=Y6,[Y3,Y8]=2Y4+Y2,[Y4,Y5]=Y5,[Y4,Y6]=−Y6,[Y4,Y8]=Y8,[Y5,Y6]=Y4−Y2, [Y5,Y7]=Y8,[Y6,Y8]=Y7, |
which are easily seen to generate a Lie algebra isomorphic to sl(3,R), implying that the equation is linearizable via a point transformation [10,37]. We further observe that two-dimensional Lie point symmetry algebras of the canonical types LI2,1, LII2,1, LI2,2, and LII2,2 (see [10] for details) that also imply linearization are, respectively, generated by the following vector fields:
LI2,1:Y1, Y3,[Y1,Y3]=0, δ=−eA(t)e−B(t)≠0,LII2,1:Y1, Y5,[Y1,Y5]=0, δ=0,LI2,2:Y2, Y6,[Y2,Y6]=Y6,δ=−eA(x)e−B(t)(∫e−A(x)dx)2≠0,LII2,2:Y1, Y2,[Y1,Y2]=Y1,δ=0, |
where
δ=det(ξ1η1ξ2η2) |
and
Zi=ξi∂∂t+ηi∂∂x |
are the infinitesimal symmetry generators for i=1,2.
The point symmetries of Eqs (5.4), (5.9), and (5.13) are computed similarly. On the other hand, equations of the type in (9.1) admit point symmetries of the type
Z=β1∂∂t+(α1x3+α2x)∂∂x |
for perturbation terms of the form
ϕz(t,x)=1tΦ(z,1α2t−4α2β1(α2x4+α1)). |
[1] |
E. L. Murphy, HTLV-1 and blood donation, British J. Haemat., 204 (2023), 29–30. https://doi.org/10.1111/bjh.19051 doi: 10.1111/bjh.19051
![]() |
[2] |
N. Legrand, S. McGregor, R. Bull, S. Bajis, B. Mark Valencia, A. Ronnachit, et al. Clinical and public health implications of human t-lymphotropic virus type 1 infection, Clinical Microb. Rev., 35 (2022), e0007821. https://doi.org/10.1128/cmr.00078-21 doi: 10.1128/cmr.00078-21
![]() |
[3] |
C. R. M. Bangham, HTLV-1 infections, J. Clinic. Path., 53 (2000), 581–586. https://doi.org/10.1136/jcp.53.8.581 doi: 10.1136/jcp.53.8.581
![]() |
[4] |
P. Katri, S. Ruan, Dynamics of human T-cell lymphotropic virus Ⅰ (HTLV-Ⅰ) infection of CD4+ T-cells, Compt. Rend.-Biologies, 327 (2004), 1009–1016. https://doi.org/10.1016/j.crvi.2004.05.011 doi: 10.1016/j.crvi.2004.05.011
![]() |
[5] |
N. Ramesh, B. Cockbain, G. P. Taylor, C. Rosadas, How do socioeconomic determinants of health affect the likelihood of living with HTLV-1 globally? A systematic review with meta-analysis, Front. Public Heal., 12 (2024), 1298308. https://doi.org/10.3389/fpubh.2024.1298308 doi: 10.3389/fpubh.2024.1298308
![]() |
[6] |
G. C. L. Sampaio, J. R. Ribeiro, C. N. de Almeida, N. Boa-Sorte, B. Galvao-Castro, M. F. R. Grassi, et al. Human T cell lymphotropic virus type 1 global prevalence associated with the human development index: Systematic review with meta-analysis, Aids Res. Human Retrov., 39 (2023), 145–165. https://doi.org/10.1089/aid.2021.0230 doi: 10.1089/aid.2021.0230
![]() |
[7] |
V. Soriano, C. de Mendoza, S. H. Network, Screening for HTLV-1 infection should be expanded in Europe, Internat. J. Infect. Disease, 140 (2024), 99–101. https://doi.org/10.1016/j.ijid.2024.01.015 doi: 10.1016/j.ijid.2024.01.015
![]() |
[8] |
M. Y. Li, A. G. Lim, Modelling the role of tax expression in HTLV-Ⅰ persistence in vivo, Bull. Math. Bio., 73 (2011), 3008–3029. https://doi.org/10.1007/s11538-011-9657-1 doi: 10.1007/s11538-011-9657-1
![]() |
[9] |
X. Zhang, M. Liu, Dynamical analysis of a stochastic delayed SIR epidemic model with vertical transmission and vaccination, Adv. Cont. Discr. Models, 2022 (2022), 35. https://doi.org/10.1186/s13662-022-03707-7 doi: 10.1186/s13662-022-03707-7
![]() |
[10] |
S. He, Y. Peng, K. Sun, SEIR modeling of the COVID-19 and its dynamics, Nonlinear Dyn., 1 (2020), 1–14. https://doi.org/10.1007/s11071-020-05743-y doi: 10.1007/s11071-020-05743-y
![]() |
[11] |
K. Wang, H. Fan, Y. Zhu, Dynamics and application of a generalized SIQR epidemic model with vaccination and treatment, Appl. Math. Modell., 120 (2023), 382–399. https://doi.org/10.1016/j.apm.2023.03.036 doi: 10.1016/j.apm.2023.03.036
![]() |
[12] |
W. Wang, W. B. Ma, Global dynamics of a reaction and diffusion model for an htlv-i infection with mitotic division of actively infected cells, J. Appl. Anal. Comput., 7 (2017), 899–930. https://doi.org/10.11948/2017057 doi: 10.11948/2017057
![]() |
[13] |
S. Khajanchi, S. Bera, T. K. Roy, Mathematical analysis of the global dynamics of a HTLV-Ⅰ infection model, considering the role of cytotoxic T-lymphocytes, Math. Comput. Simul., 180 (2021), 354–378. https://doi.org/10.1016/j.matcom.2020.09.009 doi: 10.1016/j.matcom.2020.09.009
![]() |
[14] |
Y. Wang, J. Liu, J. M. Heffernan, Viral dynamics of an HTLV-Ⅰ infection model with intracellular delay and CTL immune response delay, J. Math. Anal. Appl., 459 (2018), 506–527. https://doi.org/10.1016/j.jmaa.2017.10.027 doi: 10.1016/j.jmaa.2017.10.027
![]() |
[15] |
V. E. Papageorgiou, P. Kolias, A novel epidemiologically informed particle filter for assessing epidemic phenomena. Application to the monkeypox outbreak of 2022, Inverse Prob., 40 (2024), 035006. https://doi.org/10.1088/1361-6420/ad1e2f doi: 10.1088/1361-6420/ad1e2f
![]() |
[16] |
D. Calvetti, A. Hoover, J. Rose, E. Somersalo, Bayesian particle filter algorithm for learning epidemic dynamics, Inverse Prob., 37 (2021), 115008. https://doi.org/10.1088/1361-6420/ac2cdc doi: 10.1088/1361-6420/ac2cdc
![]() |
[17] |
J. Elfring, E. Torta, R. van de Molengraft, Particle filters: A Hands-On tutorial, Sensors, 21 (2021), 438. https://doi.org/10.3390/s21020438 doi: 10.3390/s21020438
![]() |
[18] |
J. R. Artalejo, A. Economou, M. Lopez-Herrero, The stochastic SEIR model before extinction: Computational approaches, Appl. Math. Comput., 265 (2015), 1026–1043. https://doi.org/10.1016/j.amc.2015.05.141 doi: 10.1016/j.amc.2015.05.141
![]() |
[19] |
D. Li, F. Wei, X. Mao, Stationary distribution and density function of a stochastic SVIR epidemic model, J. Franklin Inst., 359 (2022), 9422–9449. https://doi.org/10.1016/j.jfranklin.2022.09.026 doi: 10.1016/j.jfranklin.2022.09.026
![]() |
[20] |
S. Li, S. Guo, Persistence and extinction of a stochastic sis epidemic model with regime switching and levy jumps, Disc. Contin. Dyn. Syst. Series B, 26 (2021), 5101–5134. https://doi.org/10.3934/dcdsb.2020335 doi: 10.3934/dcdsb.2020335
![]() |
[21] |
Z. Ni, D. Jiang, Z. Cao, X. Mu, Analysis of stochastic SIRC model with cross immunity based on Ornstein-Uhlenbeck process, Qual. Theory Dyn. Syst., 22 (2023). https://doi.org/10.1007/s12346-023-00782-3 doi: 10.1007/s12346-023-00782-3
![]() |
[22] |
Edward Allen, Environmental variability and mean-reverting processes, Disc. Contin. Dyn. Syst. Series B, 21 (2016), 2073–2089. https://doi.org/10.3934/dcdsb.2016037 doi: 10.3934/dcdsb.2016037
![]() |
[23] |
B. Zhou, D. Jiang, B. Han, T. Hayat, Threshold dynamics and density function of a stochastic epidemic model with media coverage and mean-reverting Ornstein-Uhlenbeck process, Math. Comput. Simulation, 196 (2022), 15–44. https://doi.org/10.1016/j.matcom.2022.01.014 doi: 10.1016/j.matcom.2022.01.014
![]() |
[24] |
Y. Cai, J. Jiao, Z. Gui, Y. Liu, Y. Liu, Environmental variability in a stochastic epidemic model, Appl. Math. Comput., 329 (2018), 210–226. https://doi.org/10.1016/j.amc.2018.02.009 doi: 10.1016/j.amc.2018.02.009
![]() |
[25] |
T. Su, Q. Yang, X. Zhang, D. Jiang, Stationary distribution, extinction and probability density function of a stochastic SEIV epidemic model with general incidence and Ornstein-Uhlenbeck process, Phys. A: Stat. Mech. Appl., 615 (2023), 128605. https://doi.org/10.1016/j.physa.2023.128605 doi: 10.1016/j.physa.2023.128605
![]() |
[26] |
Z. Shi, D. Jiang, Environmental variability in a stochastic HIV infection model, Commun. Nonlinear Sci. Numer. Simul., 120 (2023), 107201. https://doi.org/10.1016/j.cnsns.2023.107201 doi: 10.1016/j.cnsns.2023.107201
![]() |
[27] |
Q. Liu, D. Jiang, Analysis of a stochastic logistic model with diffusion and Ornstein-Uhlenbeck process, J. Math. Phys., 63 (2022), 53505. https://doi.org/10.1063/5.0082036 doi: 10.1063/5.0082036
![]() |
[28] |
J. Shang, W. Li, Dynamical behaviors of a stochastic SIRV epidemic model with the Ornstein-Uhlenbeck process, Adv. Contin. Discrete Model., 2024 (2024), 9. https://doi.org/10.1186/s13662-024-03807-6 doi: 10.1186/s13662-024-03807-6
![]() |
[29] |
Q. Liu, Dynamical analysis of a stochastic maize streak virus epidemic model with logarithmic Ornstein-Uhlenbeck process, J. Math. Bio., 89 (2024), 30. https://doi.org/10.1007/s00285-024-02127-3 doi: 10.1007/s00285-024-02127-3
![]() |
[30] | X. Mao, Stochastic differential equations and applications, Elsevier, 2007. https://doi.org/10.1533/9780857099402 |
[31] |
C. W. Gardiner, Handbook of stochastic methods for physics, chemistry and the natural sciences, Springer Ser. Syn., 1985. https://doi.org/10.1002/phbl.19860420812 doi: 10.1002/phbl.19860420812
![]() |
[32] |
B. Zhou, D. Jiang, Y. Dai, T. Hayat, Stationary distribution and density function expression for a stochastic SIQRS epidemic model with temporary immunit, Nonlinear Dyn., 105 (2021), 931–955. https://doi.org/10.1007/s11071-020-06151-y doi: 10.1007/s11071-020-06151-y
![]() |
[33] |
D. J. Higham, An algorithmic introduction to numerical simulation of stochastic differential equations, SIAM Rev., 43 (2001), 525–546. https://doi.org/10.1137/s0036144500378302 doi: 10.1137/s0036144500378302
![]() |
[34] | J. Duan, An introduction to stochastic dynamics, Cambridge University Press, 2015. |
[35] |
A. Yang, H. Wang, T. Zhang, S. Yuan, Stochastic switches of eutrophication and oligotrophication: Modeling extreme weather via non-Gaussian Levy noise, Chaos, 32 (2022), 1–15. https://doi.org/10.1063/5.0085560 doi: 10.1063/5.0085560
![]() |
[36] |
Z. Shi, D. Jiang, Dynamical behaviors of a stochastic HTLV-Ⅰ infection model with general infection form and Ornstein-Uhlenbeck process, Chaos Solit. Fract., 165 (2022), 112789. https://doi.org/10.1016/j.chaos.2022.112789 doi: 10.1016/j.chaos.2022.112789
![]() |
[37] |
C. Song, R. Xu, Mathematical analysis of an htlv-i infection model with the mitosis of cd4+ t cells and delayed ctl immune response(Article), Nonlinear Anal.: Modell. Control, 26 (2021), 1–20. https://doi.org/10.15388/namc.2021.26.21050 doi: 10.15388/namc.2021.26.21050
![]() |
[38] |
A. M. Elaiw, N. H. AlShamrani, Analysis of a within-host HIV/HTLV-Ⅰ co-infection model with immunity, Virus Res., 295 (2021), 198204. https://doi.org/10.1016/j.virusres.2020.198204 doi: 10.1016/j.virusres.2020.198204
![]() |
[39] |
Q. Liu, Stationary distribution and probability density for a stochastic SISP respiratory disease model with Ornstein-Uhlenbeck process, Commun. Nonlinear Sci. Num. Simul., 119 (2023), 107128. https://doi.org/10.1016/j.cnsns.2023.107128 doi: 10.1016/j.cnsns.2023.107128
![]() |
[40] |
D. Kuang, Q. Yin, J. Li, Dynamics of stochastic HTLV-Ⅰ infection model with nonlinear CTL immune response, Math. Meth. Appl. Sci., 44 (2021), 14059–14078. https://doi.org/10.1002/mma.7674 doi: 10.1002/mma.7674
![]() |
● Case Ⅰ Extended Buchdahl equation a(x)=3x−1 b1(t)=t−1 Ξ(x)=x−3 γ(t)=lnt |
ω=1x3ydx∧dyh1=yx3h2=12x2h3=−x2y |
dxdt=y+b2(t)x42ydydt=3y2x+yt+b2(t)x32 |
x(t)=±{(c1+∫tb2(τ)τdτ)(2c2+t2)}−1/2 |
y(t)=∓t(c1+∫tb2(τ)τdτ)−1/2(2c2+t2)−3/2 |
● Case Ⅱ a(x)=x−1 arbitrary b1(t) Ξ(x)= x−1 |
ω=1xydx∧dyh1=yxh2=−lnxh3=xlnxy |
dxdt=y−b2(t)x2lnxydydt=y2x+b1(t)y−b2(t)x(1+lnx) |
x(t)=exp{−(c1+∫te−γ(τ)b2(τ)dτ)(c2+∫teγ(τ)dτ)} |
y(t)=−eγ(t)(c1+∫te−γ(τ)b2(τ)dτ)exp{−(c1+∫te−γ(τ)b2(τ)dτ)(c2+∫teγ(τ)dτ)} |
Subcase Ⅱ a(x)=x−1 b1(t)=t−1 Ξ(x)= x−1 γ(t)=lnt |
x(t)=exp{−(c1+∫tb2(τ)τdτ)(c2+t22)} |
y(t)=−t(c1+∫tb2(τ)τdτ)exp{−(c1+∫tb2(τ)τdτ)(c2+t22)} |
● Case Ⅲ a(x)=αx−1 (α≠1) arbitrary b1(t) Ξ(x)= x−α |
ω=1xαydx∧dyh1=yxαh2=−x1−α1−αh3=xy(1−α) |
dxdt=y−b2(t)x1+α(1−α)ydydt=αy2x+b1(t)y−b2(t)xα1−α |
x(t)={(α−1)(c1+∫te−γ(τ)b2(τ)dτ)(c2+∫teγ(τ)dτ)}11−α |
y(t)=−eγ(t)(c1+∫te−γ(τ)b2(τ)dτ){(α−1)(c1+∫te−γ(τ)b2(τ)dτ)(c2+∫teγ(τ)dτ)}α1−α |
Subcase Ⅲ a(x)=αx−1 (α≠1) b1(t)=t−1 Ξ(x)= x−α γ(t)=lnt |
x(t)={(α−1)(c1+∫tb2(τ)τdτ)(c2+t22)}11−α |
y(t)=−t(c1+∫tb2(τ)τdτ){(α−1)(c1+∫tb2(τ)τdτ)(c2+t22)}α1−α |
∗Note: For each case, we indicate the choice of the coefficients a(x) and b1(t) for an arbitrary b2(t), the symplectic form (2.6), the functions Ξ(x) and γ(t), the Hamiltonian functions (6.8), the system (6.10), and its corresponding general solution (6.11). |
● Case Ⅰ Deformed extended Buchdahl equation a(x)=3x−1 b1(t)=t−1 Ξ(x)=x−3 γ(t)=lnt |
ω=1x3ydx∧dyhz,1=yx3hz,2=x1−exp(−zyx3)2zyhz,3=−x2yexp(−zyx3) |
dxdt=y+12t(xexp(−zyx3)−x41−exp(−zyx3)zy)+b2(t)x4+zxy2yexp(−zyx3) |
dydt=3y2x+12t(3yexp(−zyx3)−x31−exp(−zyx3)z)+12b2(t)(x3+3zy)exp(−zyx3) |
1x2(t)=2zt(zt(c1+∫tb2(τ)τdτ)−1)ln{1−zt(c1+∫tb2(τ)τdτ)} |
×(c2+∫tt(1−zt(c1+∫τb2(τ′)τ′dτ′))−1dτ) |
y(t)=x3(t)1zln{1−zt(c1+∫tb2(τ)τdτ)} |
● Case Ⅱ a(x)=x−1 arbitrary b1(t) Ξ(x)= x−1 |
ω=1xydx∧dyhz,1=yxhz,2=xlnxexp(−zyx)−1zyhz,3=xlnxyexp(−zyx) |
dxdt=y+b1(t)xlnx(xexp(zyx)−1zy−1)exp(−zyx)−b2(t)(x2+zxy)lnxyexp(−zyx) |
dydt=y2x+b1(t)(x1−exp(−zyx)z(1+lnx)−yexp(−zyx)lnx)−b2(t)(x+(x+zy)lnx)exp(−zyx) |
lnx(t)=1zln{1−zeγ(t)(c1+∫te−γ(τ)b2(τ)dτ)}(e−γ(t)−z(c1+∫te−γ(τ)b2(τ)dτ)) |
×{c2+∫t(e−γ(τ)−z(c1+∫τe−γ(τ′)b2(τ′)dτ′))−1dτ} |
y(t)=x(t)1zln(1−zeγ(t)(c1+∫te−γ(τ)b2(τ)dτ)) |
● Case Ⅲ a(x)=αx−1 (α≠1) arbitrary b1(t) Ξ(x)= x−α |
ω=1xαydx∧dyhz,1=yxαhz,2=xexp(−zyx−α)−1zy(1−α)hz,3=xy(1−α)exp(−zyx−α) |
dxdt=y+b1(t)x1−α(xαexp(zyx−α)−1zy−1)exp(−zyx−α)−b2(t)x1+α+zxy(1−α)yexp(−zyx−α) |
dydt=αy2x+b1(t)11−α(xαexp(zyx−α)−1z−αy)exp(−zyx−α)−b2(t)xα+zαy1−αexp(−zyx−α) |
x1−α(t)=(1−α)1zln{1−zeγ(t)(c1+∫te−γ(τ)b2(τ)dτ)}(e−γ(t)−z(c1+∫te−γ(τ)b2(τ)dτ)) |
×{c2+∫t(e−γ(τ)−z(c1+∫τe−γ(τ′)b2(τ′)dτ′))−1dτ} |
y(t)=xα(t)1zln(1−zeγ(t)(c1+∫te−γ(τ)b2(τ)dτ)) |
∗Note: For each case we show the coefficients a(x) and b1(t), always with an arbitrary b2(t), the symplectic form (2.6), the functions Ξ(x) and γ(t), the Hamiltonian functions (7.8), the system (7.9), and its general solution (7.10). |
● Case Ⅰ Extended Buchdahl equation a(x)=3x−1 b1(t)=t−1 Ξ(x)=x−3 γ(t)=lnt |
ω=1x3ydx∧dyh1=yx3h2=12x2h3=−x2y |
dxdt=y+b2(t)x42ydydt=3y2x+yt+b2(t)x32 |
x(t)=±{(c1+∫tb2(τ)τdτ)(2c2+t2)}−1/2 |
y(t)=∓t(c1+∫tb2(τ)τdτ)−1/2(2c2+t2)−3/2 |
● Case Ⅱ a(x)=x−1 arbitrary b1(t) Ξ(x)= x−1 |
ω=1xydx∧dyh1=yxh2=−lnxh3=xlnxy |
dxdt=y−b2(t)x2lnxydydt=y2x+b1(t)y−b2(t)x(1+lnx) |
x(t)=exp{−(c1+∫te−γ(τ)b2(τ)dτ)(c2+∫teγ(τ)dτ)} |
y(t)=−eγ(t)(c1+∫te−γ(τ)b2(τ)dτ)exp{−(c1+∫te−γ(τ)b2(τ)dτ)(c2+∫teγ(τ)dτ)} |
Subcase Ⅱ a(x)=x−1 b1(t)=t−1 Ξ(x)= x−1 γ(t)=lnt |
x(t)=exp{−(c1+∫tb2(τ)τdτ)(c2+t22)} |
y(t)=−t(c1+∫tb2(τ)τdτ)exp{−(c1+∫tb2(τ)τdτ)(c2+t22)} |
● Case Ⅲ a(x)=αx−1 (α≠1) arbitrary b1(t) Ξ(x)= x−α |
ω=1xαydx∧dyh1=yxαh2=−x1−α1−αh3=xy(1−α) |
dxdt=y−b2(t)x1+α(1−α)ydydt=αy2x+b1(t)y−b2(t)xα1−α |
x(t)={(α−1)(c1+∫te−γ(τ)b2(τ)dτ)(c2+∫teγ(τ)dτ)}11−α |
y(t)=−eγ(t)(c1+∫te−γ(τ)b2(τ)dτ){(α−1)(c1+∫te−γ(τ)b2(τ)dτ)(c2+∫teγ(τ)dτ)}α1−α |
Subcase Ⅲ a(x)=αx−1 (α≠1) b1(t)=t−1 Ξ(x)= x−α γ(t)=lnt |
x(t)={(α−1)(c1+∫tb2(τ)τdτ)(c2+t22)}11−α |
y(t)=−t(c1+∫tb2(τ)τdτ){(α−1)(c1+∫tb2(τ)τdτ)(c2+t22)}α1−α |
∗Note: For each case, we indicate the choice of the coefficients a(x) and b1(t) for an arbitrary b2(t), the symplectic form (2.6), the functions Ξ(x) and γ(t), the Hamiltonian functions (6.8), the system (6.10), and its corresponding general solution (6.11). |
● Case Ⅰ Deformed extended Buchdahl equation a(x)=3x−1 b1(t)=t−1 Ξ(x)=x−3 γ(t)=lnt |
ω=1x3ydx∧dyhz,1=yx3hz,2=x1−exp(−zyx3)2zyhz,3=−x2yexp(−zyx3) |
dxdt=y+12t(xexp(−zyx3)−x41−exp(−zyx3)zy)+b2(t)x4+zxy2yexp(−zyx3) |
dydt=3y2x+12t(3yexp(−zyx3)−x31−exp(−zyx3)z)+12b2(t)(x3+3zy)exp(−zyx3) |
1x2(t)=2zt(zt(c1+∫tb2(τ)τdτ)−1)ln{1−zt(c1+∫tb2(τ)τdτ)} |
×(c2+∫tt(1−zt(c1+∫τb2(τ′)τ′dτ′))−1dτ) |
y(t)=x3(t)1zln{1−zt(c1+∫tb2(τ)τdτ)} |
● Case Ⅱ a(x)=x−1 arbitrary b1(t) Ξ(x)= x−1 |
ω=1xydx∧dyhz,1=yxhz,2=xlnxexp(−zyx)−1zyhz,3=xlnxyexp(−zyx) |
dxdt=y+b1(t)xlnx(xexp(zyx)−1zy−1)exp(−zyx)−b2(t)(x2+zxy)lnxyexp(−zyx) |
dydt=y2x+b1(t)(x1−exp(−zyx)z(1+lnx)−yexp(−zyx)lnx)−b2(t)(x+(x+zy)lnx)exp(−zyx) |
lnx(t)=1zln{1−zeγ(t)(c1+∫te−γ(τ)b2(τ)dτ)}(e−γ(t)−z(c1+∫te−γ(τ)b2(τ)dτ)) |
×{c2+∫t(e−γ(τ)−z(c1+∫τe−γ(τ′)b2(τ′)dτ′))−1dτ} |
y(t)=x(t)1zln(1−zeγ(t)(c1+∫te−γ(τ)b2(τ)dτ)) |
● Case Ⅲ a(x)=αx−1 (α≠1) arbitrary b1(t) Ξ(x)= x−α |
ω=1xαydx∧dyhz,1=yxαhz,2=xexp(−zyx−α)−1zy(1−α)hz,3=xy(1−α)exp(−zyx−α) |
dxdt=y+b1(t)x1−α(xαexp(zyx−α)−1zy−1)exp(−zyx−α)−b2(t)x1+α+zxy(1−α)yexp(−zyx−α) |
dydt=αy2x+b1(t)11−α(xαexp(zyx−α)−1z−αy)exp(−zyx−α)−b2(t)xα+zαy1−αexp(−zyx−α) |
x1−α(t)=(1−α)1zln{1−zeγ(t)(c1+∫te−γ(τ)b2(τ)dτ)}(e−γ(t)−z(c1+∫te−γ(τ)b2(τ)dτ)) |
×{c2+∫t(e−γ(τ)−z(c1+∫τe−γ(τ′)b2(τ′)dτ′))−1dτ} |
y(t)=xα(t)1zln(1−zeγ(t)(c1+∫te−γ(τ)b2(τ)dτ)) |
∗Note: For each case we show the coefficients a(x) and b1(t), always with an arbitrary b2(t), the symplectic form (2.6), the functions Ξ(x) and γ(t), the Hamiltonian functions (7.8), the system (7.9), and its general solution (7.10). |