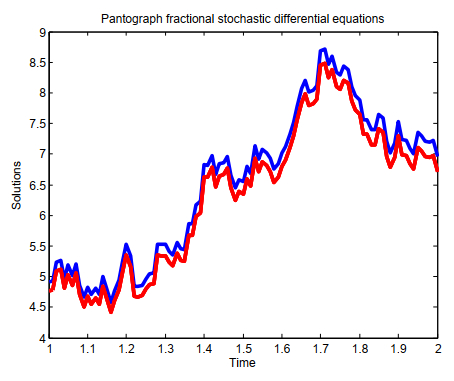
In this paper, we review the underappreciated theorem by Lotz that tells us that every strongly continuous operator semigroup on a Grothendieck space with the Dunford-Pettis property is automatically uniformly continuous. A large class of spaces that carry these geometric properties are L∞(Ω,Σ,μ) for non-negative measure spaces. This shows once again that L∞-spaces have to be treated differently.
Citation: Christian Budde. Another special role of L∞-spaces-evolution equations and Lotz' theorem[J]. AIMS Mathematics, 2024, 9(12): 36158-36166. doi: 10.3934/math.20241716
[1] | J. Vanterler da C. Sousa, E. Capelas de Oliveira, F. G. Rodrigues . Ulam-Hyers stabilities of fractional functional differential equations. AIMS Mathematics, 2020, 5(2): 1346-1358. doi: 10.3934/math.2020092 |
[2] | Souad Ayadi, Ozgur Ege, Manuel De la Sen . On a coupled system of generalized hybrid pantograph equations involving fractional deformable derivatives. AIMS Mathematics, 2023, 8(5): 10978-10996. doi: 10.3934/math.2023556 |
[3] | Ahmed M. A. El-Sayed, Wagdy G. El-Sayed, Kheria M. O. Msaik, Hanaa R. Ebead . Riemann-Liouville fractional-order pantograph differential equation constrained by nonlocal and weighted pantograph integral equations. AIMS Mathematics, 2025, 10(3): 4970-4991. doi: 10.3934/math.2025228 |
[4] | Sabri T. M. Thabet, Sa'ud Al-Sa'di, Imed Kedim, Ava Sh. Rafeeq, Shahram Rezapour . Analysis study on multi-order ϱ-Hilfer fractional pantograph implicit differential equation on unbounded domains. AIMS Mathematics, 2023, 8(8): 18455-18473. doi: 10.3934/math.2023938 |
[5] | Songkran Pleumpreedaporn, Chanidaporn Pleumpreedaporn, Weerawat Sudsutad, Jutarat Kongson, Chatthai Thaiprayoon, Jehad Alzabut . On a novel impulsive boundary value pantograph problem under Caputo proportional fractional derivative operator with respect to another function. AIMS Mathematics, 2022, 7(5): 7817-7846. doi: 10.3934/math.2022438 |
[6] | Qun Dai, Shidong Liu . Stability of the mixed Caputo fractional integro-differential equation by means of weighted space method. AIMS Mathematics, 2022, 7(2): 2498-2511. doi: 10.3934/math.2022140 |
[7] | Abdelkader Moumen, Ramsha Shafqat, Zakia Hammouch, Azmat Ullah Khan Niazi, Mdi Begum Jeelani . Stability results for fractional integral pantograph differential equations involving two Caputo operators. AIMS Mathematics, 2023, 8(3): 6009-6025. doi: 10.3934/math.2023303 |
[8] | Saeed M. Ali, Mohammed S. Abdo, Bhausaheb Sontakke, Kamal Shah, Thabet Abdeljawad . New results on a coupled system for second-order pantograph equations with ABC fractional derivatives. AIMS Mathematics, 2022, 7(10): 19520-19538. doi: 10.3934/math.20221071 |
[9] | Sabri T. M. Thabet, Miguel Vivas-Cortez, Imed Kedim . Analytical study of ABC-fractional pantograph implicit differential equation with respect to another function. AIMS Mathematics, 2023, 8(10): 23635-23654. doi: 10.3934/math.20231202 |
[10] | Weerawat Sudsutad, Chatthai Thaiprayoon, Sotiris K. Ntouyas . Existence and stability results for ψ-Hilfer fractional integro-differential equation with mixed nonlocal boundary conditions. AIMS Mathematics, 2021, 6(4): 4119-4141. doi: 10.3934/math.2021244 |
In this paper, we review the underappreciated theorem by Lotz that tells us that every strongly continuous operator semigroup on a Grothendieck space with the Dunford-Pettis property is automatically uniformly continuous. A large class of spaces that carry these geometric properties are L∞(Ω,Σ,μ) for non-negative measure spaces. This shows once again that L∞-spaces have to be treated differently.
A generalization of classical calculus that permits the differentiation and integration of any order is known as fractional calculus (FC). FC serves as a suitable tool for modeling systems with memory effects, where past actions impact current behavior. Fractional-order derivatives better capture memory effects in biological systems, diffusion processes, and viscoelastic materials. Among the noteworthy applications are[1,2,3,4,5,6,7,8,9,10,11,12,13]:
● Complex physical systems like viscoelastic materials, diffusion processes, electrical circuits, and fluid dynamics are all being modeled and analyzed using FC. Systems that exhibit anomalous diffusion, non-local behaviors, and memory effects are better described using fractional differential equations.
● In control systems, fractional-order controllers, fractional-order observers, and systems with temporal delays are all being modeled using FC. In some applications, fractional-order controllers perform better, are more robust, and are more stable than integer-order controllers.
● In signal processing, FC is used for applications including image processing, time series analysis, noise reduction, and signal denoising. Long-range dependence, fractal features, and handling non-stationary signals are all benefits of fractional-order filters and transforms.
● FC is used to model physiological processes, biological systems, and medical imaging procedures. Applications include neuronal dynamics modeling, biological signal analysis, drug kinetics, and gene expression.
● Fractional-order damping, nonlinear dynamics, and viscoelastic features of mechanical systems are all being modeled using FC. Applications include vibration analysis, control of flexible structures, and structural health monitoring.
● The dynamics of batteries, electrochemical systems, and materials with unusual transport properties are all being modeled using FC. Mass and charge transport processes are more accurately described by fractional diffusion and reaction-diffusion equations.
● Precipitation patterns, pollutant transfer, and groundwater movement are all being modeled using FC. In hydrological systems, long-term memory effects and anomalous transport processes are better described by fractional-order differential equations.
● In robotics, FC is used to model robot dynamics and to create motion and control algorithms. The benefits of fractional-order controllers include robustness against shocks, trajectory tracking, and adaptive control.
● Within its own domain, FC finds use in the analysis of differential equations involving fractional operators, integral transforms, and special functions. Strong tools for deciphering intricate mathematical and physical systems and phenomena are offered by FC.
● FC is employed to analyze economic time series data, which often exhibits long-range dependence and non-stationary behavior. Fractional processes can capture the memory effects and persistence observed in economic variables over time. Economic models incorporating fractional calculus better capture the dynamics of financial markets, including stock price movements, trading volume, and market volatility.
Researchers utilize FC to find applications in various fields, including the study of synchronization phenomena in complex networks [14,15,16,17]. Global bipartite synchronization refers to the phenomenon where two distinct groups of nodes in a network synchronize their dynamics while remaining unsynchronized with nodes within their group. This phenomenon is crucial in understanding the collective behavior of complex systems and has applications in various domains such as neuroscience, biology, and engineering. The combination of fractional calculus and global bipartite synchronization allows researchers to model and analyze complex network dynamics with memory effects and long-range dependencies. Fractional-order differential equations capture the non-local interactions and memory effects present in real-world systems, while the concept of global bipartite synchronization provides insights into the emergent collective behavior of networked systems. By integrating FC with the study of global bipartite synchronization, researchers develop more accurate models and algorithms for understanding and controlling the dynamics of complex networks. This interdisciplinary approach has the potential to advance our understanding of synchronization phenomena in networked systems and facilitates the design of robust and efficient communication and control strategies.
Fractional order derivative (FOD) is defined in different ways; some definitions are conformable, Riemann-Liouville (R-L), Caputo-Fabrizio, Atangana-Baleanu, Caputo-Hadamard, and Grunuwald Letnikov [18,19,20,21,22]. There are various definitions for FOD, in contrast to integer-order derivatives. In general, these definitions do not match each other. The conformable fractional derivative (CFD), which provides a unique description of the FOD in contrast to previous versions, is developed by Khalil et al. [23].
Regarding a mapping Ψ(τ):[0,∞[→R, the CFD is:
TℓτΨ(τ)=limϵ→0Ψ⌈ℓ⌉−1(τ+ϵτ⌈ℓ⌉−ℓ)−Ψ⌈ℓ⌉−1(τ)ϵ, |
where Tℓτ is the CFD with respect to time and u−1<ℓ≤u,τ>0, u∈N and ⌈ℓ⌉, the lowest integer that is equal to or larger than ℓ. In a certain instance, if 0<ℓ≤1, we acquire
TℓτΨ(τ)=limϵ→0Ψ(τ+ϵτ1−ℓ)−Ψ(τ)ϵ,τ>0. |
If Ψ(τ) is ℓ-differentiable in some (0,Ξ), Ξ>0 and limτ→0+Ψℓ(τ) exists, so we have Ψ(ℓ)(0)=limτ→0+Ψ(ℓ)(τ). The fractional integral in the sense of conformable of a function Ψ(τ) starting from τ0≥0 is defined as:
Iτ0ℓ(Ψ)(τ)=∫ττ0Ψ(χ)(χ−τ0)1−ℓdχ,ℓ∈(0,1]. |
Differential equations (DEs) with a random or stochastic component are known as stochastic differential equations (SDEs). In the natural and social sciences, including physics, chemistry, biology, ecology, economics, finance, and engineering, SDEs are extensively employed to model a wide range of phenomena. These are only a few of the several scientific and engineering domains where SDEs are used [24,25,26].
● Physics: Systems that are sensitive to random forces, noise, or temperature changes have their motion described by SDEs. One SDE that simulates the Brownian motion of a particle in a fluid is the Langevin equation.
● Chemistry: The dynamics of chemical reactions involving random events, including collisions, transitions, or catalysis, are captured by SDEs. The intermolecular forces and thermodynamic fluctuations are taken into consideration in the molecular dynamics equation, which explains the motion of individual molecules in a system.
● Biology: Genetic mutations, environmental variations, and population interactions are examples of random events that can affect the behavior of biological systems, and SDEs simulate their behavior. An example of an SDE that depicts the dynamics of predator-prey relationships in an ecosystem is the Lotka-Volterra equation.
● Ecology: SDEs simulate the growth and decline of populations that are affected by stochastic events, such as births, deaths, migrations, or competitions. For example, the logistic equation is a SDE that describes the growth of a population with a carrying capacity.
● Economics: Random shock-driven economic variables, such as interest rates, prices, and exchange rates, are analyzed using SDEs. One SDE that establishes the value of a European option in a financial market is the Black-Scholes equation.
● Finance: Financial assets like stocks, bonds, and derivatives that are susceptible to uncertainty have their risk and return estimated by SDEs. One SDE that simulates the price of a stock that moves in a random walk with drift and volatility is the geometric Brownian motion.
● Engineering: Engineering systems subject to random disturbances, including signal processing, control, or communication systems, have their performance and dependability improved by SDEs. One SDE that approximates a system's state from noisy observations is the Kalman filter.
A DE with a FOD and a stochastic process is known as a fractional stochastic differential equation (FSDE). Systems with long-range dependencies, turbulence, economics, and biology are being modeled by these equations. The idea of FSDEs still faces many questions and challenges that need to be addressed. The existence and uniqueness of solutions, stability and convergence, numerical techniques, and applications to physics, biology, finance, and other domains are a few of the interesting subjects.
Some authors have been actively researching the FSDEs lately. Li and Xu developed exponential stability in the mean square of delay FSDEs [27]. This study endeavored to provide additional conditions for the exponential stability of the mean square of the scenarios it investigated. Sadovskii's fixed-point theory was applied by Li and Peng [28] and worked on the important concept of the controllability of FSDEs. Cui and Yan [29] established results for delay integrodifferential equations with stochastic terms with respect to the existence of solutions in Hilbert spaces with the help of Sadovskii's fixed-point. Specifically, in [30], Niu and Xie investigated the existence, uniqueness, and regularity of FSDEs. With the help of the Schauder theorem, Chen and Li [31] worked on some characteristics of solutions to FSDEs. Under different assumptions, the authors of the articles [32,33] showed that solutions to FSDEs exist. Karczewska and Lizama reported a number of findings in [34] on the solutions to FSDEs. Schnaubelt and Veraar [35] have worked on path-wise continuous features for solutions to stochastic problems. The stopping time technique was used by Xiao and Wang [36] to investigate the stability of FSDEs of the Caputo type. Saifullah et al. [37] investigated the existence and uniqueness of solutions for FSDEs with R-L. The authors applied the fixed point theory (FPT) for uniqueness and the Móach condition for the existence of a solution. The writers also present the Hyers-Ulam stability of these equations. Moumen et al. [38] subjected FSDEs with nonlocal conditions to an approximate controllability analysis. The authors derived the approximate controllability of nonlinear FSDEs. For the desired results, the FPT for multi-valued operators was employed.
Dynamic systems depend on states other than their present ones from the past; these occurrences are often simulated using pantograph differential equations (PDEs) [39,40,41,42,43,44]. PDEs, FC, and stochastic calculus are combined in stochastic fractional pantograph differential equations (PFSDEs). They are useful in many domains where systems show memory effects in addition to random fluctuations. Among the noteworthy uses of PFSDEs are [45,46,47,48,49,50]:
● PFSDEs are used to simulate stochastic volatility and memory effects in financial processes, including option pricing models, asset price dynamics, and risk management techniques. These models aid in the development of successful investing strategies as well as the comprehension of the intricate behavior of financial markets.
● PFSDEs are used in the modeling and analysis of random delay and memory effect communication systems, including packet-switched networks, wireless networks, and data transfer protocols. These models help maximize dependability, reduce latency, and optimize network performance.
● Stochastic dynamical systems, random walks in fractal media, diffusion processes, and other physical systems with random fluctuations and memory effects are among the systems that PFSDEs simulate. These models aid in forecasting the long-term evolution of complex physical systems and in understanding their behavior.
● PFSDEs are used to represent biological systems, including gene expression pathways, neuronal dynamics, and physiological responses to external stimuli, that exhibit both stochastic variability and memory effects. These models aid in the development of strategies for the management and treatment of disease as well as the comprehension of the fundamental mechanisms driving biological processes.
● PFSDEs are useful for simulating stochastic variability and memory effects in environmental processes, including pollution dispersion, ecological systems, and climate models. These models are helpful for forecasting changes in the environment, evaluating the effects of human activity on ecosystems, and formulating conservation plans for the environment.
● PFSDEs are employed in the modeling and analysis of control systems, including time-delay systems, networked control systems, and adaptive control algorithms, that have both stochastic disturbances and memory effects. These models aid in the development of reliable control schemes that successfully manage disturbances and uncertainty.
● PFSDEs are used to create stochastic models for optimization issues, reinforcement learning, and machine learning methods. These models make it possible for learning algorithms to identify long-term dependencies as well as short-term oscillations in the data, resulting in learning algorithms that are more reliable and effective.
The existence and uniqueness of solutions to FSDEs are one of the main subjects covered in mathematics. Knowing whether or not a certain FSDE has a unique solution is essential. One of the most important qualitative ideas in dynamical systems is stability theory. Subsequently, the concept of stability garners increased interest across several fields of study and practical uses. In mathematics, Ulam-Hyers stability refers to the approximations of functional equation solutions. In the 1940s, Ulam and Hyers introduced it, and Rassias and others later generalized it.
Inspired by these findings, we first demonstrated the existence and uniqueness of solutions of PFSDEs in the framework of CFD by using the Banach fixed point theorem; after that, we demonstrated the continuous dependency (Con-D) of solutions on both the initial value (In-V) and the fractional exponent ℓ. The second section was devoted to examining the Ulam-Hyers stability (UHS) of PFSDE using the generalized Gronwall inequalities. The main part of the proof involved the use of the Cauchy-Schwartz inequality (CS-Ineq), Itˆo isometry formula (I-IsF), generalized Gronwall's inequality (GG-Ineq), and temporally weighted norm.
Compared with the research results of [39,40,41,42,43,44,45,46,47,48,49,50], the major contributions of this paper include at least the following three aspects:
(ⅰ) In contrast to [39,40,41,42,43,44], the system we studied was more generalized because it included the stochastic term.
(ⅱ) In contrast to [45,46,47,48,49,50], in our research work, we established results regarding the existence, uniqueness, continuous dependency on both the initial condition and fractional component, and Ulam-Hyers stability in the sense of CFD.
(ⅲ) In contrast to [45,46,47,48,49,50,51], in which continuous dependency was not discussed, we have evaluated this factor in our research work. We presented continuous dependency of solutions of PFSDEs on the initial value and fractional exponent in the sense of CFD.
We examined the following PFSDEs of order 12<ℓ<1:
TℓτZ(τ)=A1(τ,Z(τ),Z(ητ))+A2(τ,Z(τ),Z(ητ))dWτdτ, | (1.1) |
where ητ represents the past state and η∈(0,1) and ℓ represent the CFD, A1:[τ0,ℑ]×Rm×Rm→Rm, A2:[τ0,ℑ]×Rm×Rm→Rm are measurable and on an underlying complete filtered probability space (Ω,F,P), and (Wτ)τ∈[0,∞) is a scalar Brownian motion, with the filtration Fτ=(Fτ)τ0≤τ≤ℑ.
Fractional orders in the interval (12,1) are a popular choice [52,53,54,55]; the precise range may differ based on the situation, the nature of the issue, and the intended trade-off between correctly capturing the dynamics of the system and maintaining mathematical tractability. For instance, the fractional order in viscoelastic materials may indicate the ratio of elasticity to viscosity. Values between 12 and 1 more closely represent the behavior of the system in such circumstances. When compared to fractional-order systems with orders below 12, those with orders in the interval (12,1) frequently show superior stability and regularity features. Systems having orders smaller than 12 can result in non-unique solutions or a deficiency of solutions in mathematics.
Extending the fractional order range from (12,1) to (0,1) poses challenges due to the inclusion of the point 0. However, we have provided unified criteria for selecting fractional orders within the extended range (0,1) by considering the following factors:
(ⅰ) We established criteria based on stability and regularity properties of solutions. Fractional orders within the range (0,1) yield stable and well-behaved solutions for the systems under consideration. Analyzing stability and regularity conditions guided the selection of suitable fractional orders.
(ⅱ) Unified criteria ensure the existence and uniqueness of solutions for fractional differential equations within the extended range. We developed mathematical conditions that guarantee the existence and uniqueness of solutions, considering the fractional order's impact on these properties.
(ⅲ) Criteria were based on the physical interpretation of fractional orders within the range (0,1). We ensured that selected fractional orders aligned with the underlying dynamics of the systems being modeled. This involved considering the physical meaning of fractional orders in specific applications, such as viscoelasticity or diffusion processes.
(ⅳ) Unified criteria address the numerical stability and efficiency of methods used to solve fractional differential equations with fractional orders in the range (0,1). We established guidelines for selecting numerical methods that accurately and efficiently handle fractional order equations while maintaining stability and convergence properties.
(ⅴ) We conducted comparative analyses between fractional orders within the range (0,1) and those within the range (12,1). By comparing the behavior and performance of systems modeled with different fractional orders, the authors identified criteria that effectively differentiated between suitable fractional orders.
By considering these factors and establishing unified criteria, we provided guidance for selecting fractional orders within the extended range (0,1) that ensured the stability, regularity, and physical relevance of solutions in various applications.
The study is structured as follows: We use some fundamental ideas in the next section to provide the framework for the PFSDE conclusions. We first demonstrate the well-posedness of the PFSDE solution in the first subsection of Section 3, and we demonstrate the UHS and offer two instances to support our findings in the second section. Section 4 then presents the conclusion.
In this section, we will go over some basic concepts, assumptions, characteristics, and definitions that will be useful in this paper.
First, we present some useful characteristics of CFD that make it distinguishable from other fractional derivatives. The primary advantages of CFD are outlined in the following order [56,57,58,59,60,61,62,63,64,65,66,67]:
(ⅰ) In contrast to the other fractional formulations, it satisfies every need and regulation of an ordinary derivative, such as the product, quotient, and chain rules; Rolle's theorem; and mean-value theorem.
(ⅱ) It reduces the complexity of well-known transforms, such as the Sumudu and Laplace transforms, which are used as instruments to solve some DEs.
(ⅲ) It may be quickly and simply modified to solve systems, analytical, and numerical DEs.
(ⅳ) Fuzzy generalized CFD, Katugampola fractional derivatives, M-CFD, deformable fractional derivatives, and class CFD are just a few of the new concepts that may be created and expanded upon thanks to it.
(ⅴ) In many different applications, it produces new comparisons between CFD and previous fractional definitions.
(ⅵ) CFD draws a lot of interest from researchers since it can be used to depict a wide range of phenomena and applications that need to be addressed.
The following are some significant aspects of CFD that are relevant to differentiation processes.
Theorem 2.1. [68] Let 0<ℓ≤1, Ψ1(τ) and Ψ2(τ) be ℓ-differentiable at a point τ>0. Then
(i) Tℓτ(ℏ1Ψ1(τ)+ℏ2Ψ2(τ))=ℏ1TℓτΨ1(τ)+ℏ2TℓτΨ2(τ),∀ℏ1,ℏ2∈R.
(ii) Tℓτ(τℏ)=ℏτℏ−ℓ,∀ℏ∈R.
(iii) Tℓτ(Υ)=0,Υ∈R.
(iv) TℓτΨ1(τ)Ψ2(τ))=Ψ1(τ)TℓτΨ2(τ)+Ψ2(τ)TℓτΨ1(τ).
(v) TℓτΨ1(τ)Ψ2(τ)=Ψ2(τ)TℓτΨ1(τ)−Ψ1(τ)TℓτΨ2(τ)Ψ22(τ).
Denote by (Ω,F,(Fτ)τ0≤τ≤ℑ,P) the complete probability space and W(τ) is the standard Brownian motion. Suppose Zτ=L2(Ω,Fτ,P) be the space of all Fτ-measurable, integrable, and mean square functions Z=(Z1,Z2,⋯,Zm)T:Ω→Rm with
‖Z‖MS=(m∑ȷ=1E[|Zȷ|2])12=(E[‖Z‖2])12, |
making use of the usual Euclidian norm, ‖⋅‖.
Definition 2.2. To prove the desired results, we assume that coefficients A1 and A2 meet the following assumptions:
(H1): There is L>0 such that ∀(τ,ν1,ν2,˜ν1,˜ν2)∈[τ0,ℑ]×Rm×Rm×Rm×Rm:
‖A1(τ,ν1,ν2)−A1(τ,˜ν1,˜ν2)‖2≤L2(‖ν1−˜ν1‖2+‖ν2−˜ν2‖2), |
‖A2(τ,ν1,ν2)−A2(τ,˜ν1,˜ν2)‖2≤L2(‖ν1−˜ν1‖2+‖ν2−˜ν2‖2). |
(H2): A1(.,0,0) and A2(.,0,0) are bounded, i.e,
‖A1(.,0,0)‖∞=esssupτ∈[τ0,ℑ]‖A1(τ,0,0)‖<∞, |
‖A2(.,0,0)‖∞=esssupτ∈[τ0,ℑ]‖A2(τ,0,0)‖<∞, |
∫ℑτ0‖A1(χ,0,0)‖2dχ<∞, |
∫ℑτ0‖A2(χ,0,0)‖2dχ<∞. |
We give now the definition of UHS.
Definition 2.3. The Eq (1.1) is UHS with respect to ϵ if there is a constant ℵ>0 such that for each ϵ>0 and N(τ)∈H2([ητ0,ℑ]) of the following inequality: ∀τ∈[τ0,ℑ]
E[‖N(τ)−N(τ0)−(∫ττ0(χ−τ0)ℓ−1(A1(χ,N(χ),N(ηχ))dχ+A2(χ,N(χ),N(ηχ))dW(χ)))‖2]≤ϵ, | (2.1) |
there exists a solution Z(τ)∈H2([ητ0,ℑ]) of Eq (1.1), with Z(τ)=N(τ) for τ∈[ητ0,τ0], satisfies E[‖N(τ)−Z(τ)‖2]≤ℵϵ, ∀τ∈[τ0,ℑ].
In this part of the paper, we present our results for the well-posedness solutions of PFSDE.
Assume ˜H2(ητ0,ℑ) is the space where all processes Z(τ) which are measurable Fℑ−adapted, with Fℑ=(Fτ)τ∈[ητ0,ℑ] and satisfy the following:
‖Z‖˜H2=supητ0≤τ≤ℑ‖Z(τ)‖MS<∞. |
It is easy to show that (˜H2([ητ0,ℑ]),‖⋅‖˜H2) is a Banach space. For ϖ∈H2([ητ0,τ0]), we consider the operator Λϖ : ˜H2([ητ0,ℑ])→˜H2([ητ0,ℑ]) defined by
Λϖ(Z(τ))=ϖ(τ0)+∫ττ0(χ−τ0)ℓ−1A1(χ,Z(χ),Z(ηχ))dχ+∫ττ0(χ−τ0)ℓ−1A2(χ,Z(χ),Z(ηχ))dW(χ), | (3.1) |
for τ∈[τ0,ℑ] and Λϖ(Z(τ))=ϖ(τ) for τ∈[ητ0,τ0].
The subsequent lemma shows this operator's well-defined property. It is essential to demonstrate the well-definedness of an operator in the context of DEs because a well-defined operator in DEs guarantees a clear, consistent mapping from the domain to the range that is independent of the representation of the domain's elements. In the study of DEs, this ensures the accuracy and consistency of mathematical operations and solutions.
The elementary inequality below is employed in the proof of this result as well as multiple others that follow:
‖Z1+Z2+⋯+Zȷ‖2≤ȷ(‖Z1‖2+‖Z2‖2+⋯+‖Zȷ‖2),∀Z1,Z2,⋯,Zȷ∈Rm. | (3.2) |
Lemma 3.1. Assume that assumptions A1 and A2 are true, then operator Λϖ is well-defined for all ϖ∈˜H2([ητ0,τ0]).
Proof. Let Z∈˜H2([ητ0,ℑ]). By utilizing Eqs (3.1) and (3.2), we get the following result:
‖Λϖ(Z(τ))‖2MS≤3‖ϖ(τ0)‖2MS+3E[‖∫ττ0(χ−τ0)ℓ−1A1(χ,Z(χ),Z(ηχ))dχ‖2]+3E[‖∫ττ0(χ−τ0)ℓ−1A2(χ,Z(χ),Z(ηχ))dW(χ)‖2]. | (3.3) |
With CS-ineq, we derive
E[‖∫ττ0(χ−τ0)ℓ−1A1(χ,Z(χ),Z(ηχ))dχ‖2]≤(∫ττ0(χ−τ0)2ℓ−2dχ)E[∫ττ0‖A1(χ,Z(χ),Z(ηχ))‖2dχ]. | (3.4) |
Via the use of (A1), we conclude that
‖A1(χ,Z(χ),Z(ηχ))‖2≤2L2(‖Z(χ)‖+‖Z(ηχ)‖)2+2‖A1(χ,0,0)‖2∞. | (3.5) |
Then, from Eq (3.5), we get
E[∫ττ0‖A1(χ,Z(χ),Z(ηχ))‖2dχ]≤4L2(ℑ−τ0)supτ∈[τ0,ℑ]E[‖Z(τ)‖2+‖Z(ητ)‖2]+2∫ℑτ0‖A1(χ,0,0)‖2dχ≤8L2(ℑ−τ0)supτ∈[ητ0,ℑ]E[‖Z(τ)‖2]+2∫ℑτ0‖A1(χ,0,0)‖2∞dχ. | (3.6) |
By utilizing Eq (3.6) in Eq (3.4), we acquire the following outcomes:
E[‖∫ττ0(χ−τ0)ℓ−1A1(χ,Z(χ),Z(ηχ))dχ‖2]≤12ℓ−1(ℑ−τ0)2ℓ−1(8L2(ℑ−τ0)supτ∈[ητ0,ℑ]E[‖Z(τ)‖2]+2∫ℑτ0‖A1(χ,0,0)‖2dχ). | (3.7) |
Considering the use of I-IsF, we can determine that
E[‖∫ττ0(χ−τ0)ℓ−1A2(χ,Z(χ),Z(ηχ))dW(χ)‖2]=E[∫ττ0(χ−τ0)2ℓ−2‖A2(χ,Z(χ),Z(ηχ))‖2dχ]. | (3.8) |
Applying (A1), we may deduce that
‖A2(χ,Z(χ),Z(ηχ))‖2≤4L2(‖Z(χ)‖2+‖Z(ηχ)‖2)+2‖A2(⋅,0,0)‖2∞. | (3.9) |
Thus, using Eq (3.9), we derive the next outcomes of our findings from Eq (3.8).
E[‖∫ττ0(χ−τ0)ℓ−1A2(χ,Z(χ),Z(ηχ))dW(χ)‖2]≤E[∫ττ0(χ−τ0)2ℓ−2(4L2(‖Z(χ)‖2+‖Z(ηχ)‖2)+2‖A2(⋅,0,0)‖2)dχ]≤8L22ℓ−1(ℑ−τ0)2ℓ−1‖Z(τ)‖2˜H2+22ℓ−1(ℑ−τ0)2ℓ−1‖A2(⋅,0,0)‖2∞. | (3.10) |
From Eqs (3.7) and (3.10) consequently, we extract our intended conclusions.
To establish the EU, we have to prove the following lemma.
Lemma 3.2. For any ℓ>12 and τ>0, the inequality that follows is valid:
XΓ(2ℓ−1)∫τ0χ2ℓ−2E2ℓ−1(Xχ2ℓ−1)dχ≤E2ℓ−1(Xτ2ℓ−1), | (3.11) |
where E2ℓ−1(.) is a Mittag-Leffler function which is defined as
E(2ℓ−1)(τ)=∑∞ȷ=0τȷΓ((2ℓ−1)ȷ+1). | (3.12) |
Proof. Let X>0 be arbitrary. First, we interchange integral and sum, and then we apply the procedures that follow identity.
∫τ0χ2ℓ−2χȷ(2ℓ−1)dχ=τ(ȷ+1)(2ℓ−1)B(2ℓ−1,ȷ(2ℓ−1)+1),ȷ=0,1,2,⋯. |
So, we get
XΓ(2ℓ−1)∫τ0χ2ℓ−2E2ℓ−1(Xχ2ℓ−1)dχ=X∑∞ȷ=0XȷΓ(ȷ(2ℓ−1)+1)∫τ0χ2ℓ−2χȷ(2ℓ−1)dχ=∑∞ȷ=0Xȷ+1τ(ȷ+1)(2ℓ−1)Γ(2ℓ−1)Γ(ȷ(2ℓ−1)+1)=∑∞ȷ=1Xȷτȷ(2ℓ−1)Γ(ȷ(2ℓ−1)+1)=E2ℓ−1(Xτ2ℓ−1)−1≤E2ℓ−1(Xτ2ℓ−1). |
In this case, the beta function is B. This brings the proof to an end.
Theorem 3.3. Assume that the assumptions (A1) and (A2) are fulfilled, then the problem Eq (1.1) with In.C ϖ has unique solution.
Proof. In the beginning, proceed to select a fixed positive constant X:
X>8L2(ℑ−τ0+1)Γ(2τ−1). | (3.13) |
We establish a weighted norm ‖⋅‖X over the space ˜H2([ητ0,ℑ]) as:
‖Z(τ)‖X=Supτ∈[ητ0,ℑ](E[‖Z(τ)‖2]Y(τ))12,∀Z(τ)∈˜H2([ητ0,ℑ]), | (3.14) |
Y(τ)=E2ℓ−1(X(τ−τ0)2ℓ−1) when τ∈[τ0,ℑ] and Y(τ)=1 for τ∈[ητ0,τ0]. ‖⋅‖˜H2 and ‖⋅‖X, two norms, are equivalent. For this reason, (˜H2([ητ0,ℑ]),‖⋅‖X) is a Banach space. Let Z1,Z2∈˜H2([ητ0,ℑ]), we have ∀τ∈[ητ0,τ0],Λϖ(Z1(τ))−Λϖ(Z2(τ))=0. For τ∈[τ0,ℑ], we get
E[‖Λϖ(Z1(τ))−Λϖ(Z2(τ))‖2]≤2E[‖∫ττ0(χ−τ0)ℓ−1(A1(χ,Z1(χ),Z1(ηχ))−A1(χ,Z2(χ),Z2(ηχ)))dχ‖2]+2E[‖∫ττ0(χ−τ0)ℓ−1(A2(χ,Z1(χ),Z1(ηχ))−A2(χ,Z2(χ),Z2(ηχ)))dW(χ)‖2]. | (3.15) |
Employing the CS-ineq and (A1), we acquire
E[‖∫ττ0(χ−τ0)ℓ−1(A1(χ,Z1(χ),Z1(ηχ))−A1(χ,Z2(χ),Z2(ηχ)))dχ‖2]≤2L2(ℑ−τ0)∫ττ0(χ−τ0)2ℓ−2(E[‖Z1(χ)−Z2(χ)‖2]+E[‖Z1(ηχ)−Z2(ηχ)‖2])dχ. | (3.16) |
By applying I-IsF and (A1), we reach the subsequent outcome:
E[‖∫ττ0(χ−τ0)ℓ−1(A2(χ,Z1(χ),Z1(ηχ))−A2(χ,Z2(χ),Z2(ηχ)))dW(χ)‖2]=E[∫ττ0(χ−τ0)2ℓ−2‖A2(χ,Z1(χ),Z1(ηχ))−A2(χ,Z2(χ),Z2(ηχ))‖2dχ]≤2L2∫ττ0(χ−τ0)2ℓ−2(E[‖Z1(χ)−Z2(χ)‖2]+E[‖Z1(ηχ)−Z2(ηχ)‖2])dχ. | (3.17) |
Then,
E[‖Λϖ(Z1(τ))−Λϖ(Z2(τ))‖2]≤δ∫ττ0(χ−τ0)2ℓ−2(E[‖Z1(χ)−Z2(χ)‖2]+E[‖Z1(ηχ)−Z2(ηχ)‖2])dχ, | (3.18) |
where δ=4L2(ℑ−τ0+1)Γ(ℓ)2. Then,
E[‖Λϖ(Z1(τ))−Λϖ(Z2(τ))‖2]≤δ∫ττ0(χ−τ0)2ℓ−2(Y(χ)E[‖Z1(χ)−Z2(χ)‖2]Y(χ)+Y(ηχ)E[‖Z1(ηχ)−Z2(ηχ)‖2]Y(ηχ))dχ≤δ‖Z1(τ)−Z2(τ)‖2X∫ττ0(χ−τ0)2ℓ−2(Y(χ)+Y(ηχ))dχ≤2δ‖Z1(τ)−Z2(τ)‖2X∫ττ0(χ−τ0)2ℓ−2Y(χ)dχ≤2δΓ(2ℓ−1)XE2ℓ−1(X(τ−τ0)2ℓ−1)‖Z1(τ)−Z2(τ)‖2X. |
Therefore,
‖Λϖ(Z1(τ))−Λϖ(Z2(τ))‖X≤√2δΓ(2ℓ−1)X‖Z1(τ)−Z2(τ)‖X. |
Then, there is a unique solution to Eq (1.1).
We will demonstrate the Con-D of solutions on In-V that are ϖ and Θ to PFSDEs in the following theorem.
Theorem 3.4. (A1) and (A2) are assumed to be valid. The solution Zℓ(.,ϖ) of Eq (1.1) is always dependent on ϖ, i.e.,
limΘ→ϖsupτ∈[ητ0,ℑ]‖Zℓ(τ,ϖ)−Zℓ(τ,Θ)‖MS=0. | (3.19) |
Proof. Because Zℓ(.,ϖ) and Zℓ(.,Θ) are solutions of Eq (1.1), employing Eq (3.2), the CS-Ineq, I-ISF, (A1), (A2), and Lemma 3.2, we yield
supτ∈[ητ0,ℑ]E[‖Zℓ(τ,ϖ)−Zℓ(τ,Θ)‖2]E2ℓ−1(X(τ−τ0)2ℓ−1)≤3E[‖ϖ−Θ‖2]+Γ(2ℓ−1)X6L2(τ+1)×supτ∈[ητ0,ℑ]E[‖Zℓ(τ,ϖ)−Zℓ(τ,Θ)‖2]E2ℓ−1(X(τ−τ0)2ℓ−1). | (3.20) |
By virtue of definition of ‖⋅‖X, we have
(1−Γ(2ℓ−1)X6L2(τ+1))‖Zℓ(τ,ϖ)−Zℓ(τ,Θ)‖2X≤3‖ϖ−Θ‖2MS, | (3.21) |
which, together with Eq (3.13), we derive
limϖ→Θsupτ∈[ητ0,τ]‖Zℓ(τ,ϖ)−Zℓ(τ,Θ)‖2MS=0. | (3.22) |
We will demonstrate the Con-D of solutions on fractional exponent ℓ to PFSDEs in the following theorem.
Theorem 3.5. Let us suppose that both (A1) and (A2) are valid. After that, the solution Zℓ(.,ϖ) to Eq (1.1) is Con-D on ℓ, i.e.,
lim˜ℓ→ℓsupτ∈[ητ0,ℑ]‖Zℓ(τ,ϖ)−Z˜ℓ(τ,ϖ)‖MS=0. | (3.23) |
Proof. Let ℓ,˜ℓ be arbitrary but fixed. As Zℓ(.,ϖ) and Z˜ℓ(.,ϖ) are solutions of Eq (1.1) and we implement Eq (3.2), the CS-Ineq, I-IsF, (A1), (A2), and Lemma 3.2, we achieve
E[‖Zℓ(τ,ϖ)−Z˜ℓ(τ,ϖ)‖2]E2ℓ−1(X(τ−τ0)2ℓ−1)≤supτ∈[ητ0,ℑ]E[‖Zℓ(τ,ϖ)−Z˜ℓ(τ,ϖ)‖2]E2ℓ−1(X(τ−τ0)2ℓ−1)8(τ+1)L2∫ττ0χ2ℓ−2E2ℓ−1(X(χ−τ0)2ℓ−1)dχE2ℓ−1(X(χ−τ0)2ℓ−1)+4(τ+1)(4L2supτ∈[ητ0,ℑ]‖Z˜τ(τ,ϖ)‖2+2L2‖ϖ‖2MS | (3.24) |
+‖A1(.,0,0)‖2∞+‖A2(.,0,0)‖2∞)×∫ττ0(λ(χ,τ0,ℓ,˜ℓ))2dχ, | (3.25) |
where
λ(χ,τ0,ℓ,˜ℓ)=|(χ−τ0)ℓ−1−(χ−τ0)˜ℓ−1|. | (3.26) |
By virtue of Lemma 3.2, we attain
(1−8(ℑ+1)L2Γ(2ℓ−1)X)‖Zℓ(τ,ϖ)−Z˜ℓ(τ,ϖ)‖2X≤4(ℑ+1)(4L2supτ∈[ητ0,ℑ]‖Z˜ℓ(τ,ϖ)‖2+2L2‖ϖ‖2MS+‖A1(.,0,0)‖2∞+‖A2(.,0,0)‖2∞)×∫ℓτ0(λ(χ,τ0,ℓ,˜ℓ))2dχ. | (3.27) |
However, we yield
∫ττ0(λ(χ,τ0,ℓ,˜ℓ))2dχ=∫ττ0(χ−τ0)2ℓ−2dχ+∫ττ0(χ−τ0)2˜ℓ−2dχ+∫ττ0(χ−τ0)ℓ+˜ℓ−2dχ=(τ−τ0)2ℓ−12ℓ−1+(τ−τ0)2˜ℓ−12˜ℓ−1+(τ−τ0)ℓ+˜ℓ−1ℓ+˜ℓ−1. | (3.28) |
As a result,
\begin{equation*} \lim\limits_{\tilde{\ell}\to\ell}\underset{\chi\in[\eta\tau_{\tau_{0}},\Im]}{sup}\int_{\tau_{0}}^{\tau}\big(\lambda(\chi,\tau_{0},\ell,\tilde{\ell})\big)^{2}d\chi = 0. \end{equation*} |
This suggests the completion of the proof when combined with Eq (1.1).
In the next subsection, we will prove that Eq (1.1) is Ulam-Hyers stable.
Theorem 3.6. Under ( \mathscr{A}_{1} ) and ( \mathscr{A}_{2} ), the PFSDE Eq (1.1) is UHS on \left[\tau_{0}, \Im\right] .
Proof. Set \epsilon > 0 and \mathscr{N}(\tau)\in\mathscr{H}^{2}\left(\left[\eta \tau_{0}, \Im\right]\right) satisfies Eq (2.1). Let \mathbb{Z}(\tau) \in \mathscr{H}^{2}\left(\left[\eta \tau_{0}, \Im\right]\right) be the unique solution of Eq (1.1) with In.V \mathbb{Z}(\tau) = \mathscr{N}(\tau) for \tau\in\left[\eta \tau_{0}, \tau_{0}\right] , then
\begin{align} \mathbb{Z}(\tau) = &\mathscr{N}(\tau_{0}\big)+\Bigg(\int_{\tau_{0}}^{\tau}(\chi-\tau_{0})^{\ell-1} \mathcal{A}_{1}\big(\chi,\mathbb{Z}(\chi),\mathbb{Z}(\eta\chi)\big)d\chi \\& +\int_{\tau_{0}}^{\tau}(\chi-\tau_{0})^{\ell-1}\mathcal{A}_{2}\big(\chi,\mathbb{Z}(\chi),\mathbb{Z}(\eta\chi)\big)d\mathscr{W}(\chi)\Bigg). \end{align} | (3.29) |
Thus,
\begin{align} \mathscr{E}\big[\Vert\mathscr{N}(\tau)-\mathbb{Z}(\tau)\Vert^{2}\big]\leq&2 \mathscr{E}\Bigg[\bigg\Vert\mathscr{N}(\tau)-\mathscr{N}\big(\tau_{0}\big)- \Bigg(\int_{\tau_{0}}^{\tau} (\chi-\tau_{0})^{\ell-1}\mathcal{A}_{1}\big(\chi,\mathscr{N}(\chi),\mathscr{N}(\eta\chi)\big)d\chi \\& +\int_{\tau_{0}}^{\tau}(\chi-\tau_{0})^{\ell-1}\mathcal{A}_{2}\big(\chi,\mathscr{N}(\chi),\mathscr{N}(\eta\chi)\big)d\mathscr{W}(\chi)\Bigg)\bigg\Vert^{2}\Bigg] \\& +2\mathscr{E}\Bigg[\bigg\Vert\Bigg(\int_{\tau_{0}}^{\tau}(\chi-\tau_{0})^{\ell-1}\bigg(\mathcal{A}_{1}\big(\chi,\mathscr{N}(\chi), \mathscr{N}(\eta\chi)\big)-\mathcal{A}_{1}\big(\chi,\mathbb{Z}(\chi),\mathbb{Z}(\eta\chi)\big)\bigg)d\chi \\&+ \int_{\tau_{0}}^{\tau}(\chi-\tau_{0})^{\ell-1} \bigg(\mathcal{A}_{2}\big(\chi,\mathscr{N}(\chi),\mathscr{N}(\eta\chi)\big)\\&-\mathcal{A}_{2}\big(\chi,\mathbb{Z}(\chi), \mathbb{Z}(\eta\chi)\big)\bigg)d\mathscr{W}(\chi)\Bigg)\bigg\Vert^{2}\Bigg]. \end{align} | (3.30) |
Thus, using CS-Ineq and (\mathscr{A}_{1}) , (\mathscr{A}_{2}) , we can derive that
\begin{align*} \mathscr{E}\big[\Vert\mathscr{N}(\tau)-\mathbb{Z}(\tau)\Vert^{2}\big]\leq&2\epsilon +4\mathscr{E}\Bigg[\bigg\Vert \int_{\tau_{0}}^{\tau}(\chi-\tau_{0})^{\ell-1}\bigg(\mathcal{A}_{1}\big(\chi,\mathscr{N}(\chi),\mathscr{N}(\eta\chi)\big) -\mathcal{A}_{1}\big(\chi,\mathbb{Z}(\chi),\mathbb{Z}(\eta\chi)\big)\bigg)d\chi\bigg\Vert^{2}\Bigg] \\&+ 4\mathscr{E}\Bigg\Vert\int_{\tau_{0}}^{\tau}(\chi-\tau_{0})^{\ell-1} \bigg(\mathcal{A}_{2}\big(\chi,\mathscr{N}(\chi),\mathscr{N}(\eta\chi)\big) -\mathcal{A}_{2}\big(\chi,\mathbb{Z}(\chi),\mathbb{Z}(\eta\chi)\big)\bigg)d\mathscr{W}(\chi)\bigg\Vert^{2}\Bigg] \\\leq& 2\epsilon+\frac{8\mathscr{L}^{2}\big(\Im-\tau_{0}\big)^{2\ell-1}}{(2\ell-1)} \mathscr{E}\Bigg[\int_{\tau_{0}}^{\tau}\bigg(\Vert\mathscr{N}(\chi)-\mathbb{Z}(\chi)\Vert^{2}+\Vert\mathscr{N}(\eta\chi)- \mathbb{Z}(\eta\chi)\Vert^{2}\bigg)d\chi\Bigg] \\& +8\mathscr{L}^{2}\mathscr{E}\Bigg[\int_{\tau_{0}}^{\tau}(\chi-\tau_{0})^{2\ell-2} \bigg(\Vert\mathscr{N}(\chi)-\mathbb{Z}(\chi)\Vert^{2}+ \Vert\mathscr{N}(\eta\chi)-\mathbb{Z}(\eta\chi)\Vert^{2}\bigg)d\chi\Bigg]. \end{align*} |
Let \digamma(\tau) = \sup _{\chi\in\left[\eta \tau_{0}, \tau\right]} \mathscr{E}\big[\Vert\mathscr{N}(\chi)-\mathbb{Z}(\chi)\Vert^{2}\big] for \tau \in\left[\tau_{0}, \Im\right] .
We have
\begin{equation} \mathscr{E}\big[\Vert\mathscr{N}(\chi)-\mathbb{Z}(\chi)\Vert^{2}\big]\leq\digamma(\chi), \end{equation} | (3.31) |
and
\begin{equation} \mathscr{E}\big[\Vert\mathscr{N}(\eta\chi)-\mathbb{Z}(\eta\chi)\Vert^{2}\big] \leq \digamma(\chi),\; \forall\chi\in\left[\tau_{0},\Im\right]. \end{equation} | (3.32) |
Then, for \tau\in\left[\tau_{0}, \Im\right] , we obtain
\begin{align*} \mathscr{E}\big[\|\mathscr{N}(\tau)-\mathbb{Z}(\tau)\|^{2}\big] \leq 2\epsilon+\frac{16\mathscr{L}^{2}\left(\Im-\tau_{0}\right)^{2\ell-1}}{(2 \ell-1)} \int_{\tau_{0}}^{\tau}\digamma(\chi)d\chi +16\mathscr{L}^{2}\int_{\tau_{0}}^{\tau}(\chi-\tau_{0})^{2 \ell-2}\digamma(\chi)d\chi. \end{align*} |
Hence, for all \tau\in\left[\tau_{0}, \tau\right] ,
\begin{align*} \mathscr{E}\big[\|\mathscr{N}(\chi)-\mathbb{Z}(\chi)\|^{2}\big]\leq2\epsilon+\frac{16 \mathscr{L}^{2}\left(\Im-\tau_{0}\right)^{2\ell-1}}{(2\ell-1)} \int_{\tau_{0}}^{\tau}\digamma(\chi)d\chi +16\mathscr{L}^{2}\int_{\tau_{0}}^{\tau}(\chi-\tau_{0})^{2\ell-2}\digamma(\chi)d\chi. \end{align*} |
Then,
\begin{equation} \digamma(\tau)\leq2\epsilon+\delta_{1}\int_{\tau_{0}}^{\tau}\digamma(\chi)d\chi+\delta_{2}\int_{\tau_{0}}^{\tau}(\chi-\tau_{0})^{2\ell-2}\digamma(\chi)d\chi, \end{equation} | (3.33) |
for all \tau\in\left[\tau_{0}, \Im\right] , where \delta_{1} = \frac{16\mathscr{L}^{2}\left(\Im-\tau_{0}\right)^{2\ell-1}}{(2\ell-1)} and \delta_{2} = 16 \mathscr{L}^{2} .
Using GG-Ineq, we get
\begin{align*} \digamma(\tau) &\leq \left(2\epsilon+\delta_{1}\int_{\tau_{0}}^{\tau}\digamma(\chi)d\chi\right)\mathbb{E}_{2\ell-1}\left(\delta_{2}\Gamma(2 \ell-1)\left(\tau-\tau_{0}\right)^{2\ell-1}\right)\\ &\leq \delta_{3}\epsilon+\delta_{4}\int_{\tau_{0}}^{\tau}\digamma(\chi)d\chi, \end{align*} |
where \delta_{3} = 2\mathbb{E}_{2\ell-1}\left(\delta_{2} \Gamma(2\ell-1)\left(\Im-\tau_{0}\right)^{2\ell-1}\right) and \delta_{4} = \delta_{1}\mathbb{E}_{2\ell-1}\left(\delta_{2}\Gamma(2 \ell-1)\left(\Im-\tau_{0}\right)^{2 \ell-1}\right) .
Using GG-Ineq, we get
\begin{equation} \digamma(\tau)\leq\delta_{3}\epsilon e^{\delta_{4}\big(\tau-\tau_{0}\big)}. \end{equation} | (3.34) |
Hence,
\begin{equation} \mathscr{E}[\|\mathscr{N}(\tau)-\mathbb{Z}(\tau)\|^{2}]\leq\aleph\epsilon,\; \forall\tau\in\big[\tau_{0},\Im\big], \end{equation} | (3.35) |
where \aleph = \delta_{3} e^{\delta_{4}\left(\Im-\tau_{0}\right)} . Thus, Eq (1.1) is UHS.
The purpose of this section is to understand the results that we established in the above section. For this purpose, we present two numerical examples of PFSDEs.
The PFSDEs find applications in various fields where systems exhibit random behavior and are influenced by both deterministic and stochastic factors. One physical application of PFSDEs is in modeling the dynamics of certain types of mechanical systems subject to random perturbations. In such cases, PFSDEs can be used to model the dynamics of the system. The pantograph term arises when there are delays in the system's response, for instance, due to the time it takes for vibrations to propagate through the structure. The stochastic component captures the randomness inherent in the external forcing or system parameters.
A suspension bridge subjected to random wind loads can be modeled using PFSDEs. The deterministic part of the equation accounts for the bridge's structural properties, such as stiffness and damping, while the stochastic part represents the random nature of wind loads. The pantograph term may account for delays in the bridge's response to wind gusts due to factors like the propagation speed of vibrations along its structure.
Example 4.1. Let \tau\in[1, 2] and the PFSDE for each \epsilon > 0 be provided by
\begin{align} \left\{\begin{array}{l} \mathcal{T}_{\tau}^{\ell}\mathbb{Z}(\tau) = \mathcal{A}_{1}\big(\tau, \mathbb{Z}(\tau),\mathbb{Z}(0.5 \tau)\big)+\mathcal{A}_{2}\big(\tau,\mathbb{Z}(\tau),\mathbb{Z}(0.5 \tau)\big) \frac{d\mathscr{W}(\tau)}{d\tau},\\ \mathscr{E}\bigg[\big\Vert\mathbb{Z}(\tau)-\mathbb{Z}(1)-\Bigg(\int_{1}^{\tau}(\chi-\tau_{0})^{\ell-1} \bigg(\mathcal{A}_{1}(\chi,\mathbb{Z}(\chi),\mathbb{Z}(0.5\chi))d\chi \\+\mathcal{A}_{2}(\chi,\mathbb{Z}(\chi),\mathbb{Z}(0.5\chi))d\mathscr{W}(\chi)\big)\bigg)\Bigg)\big\Vert^{2}\bigg]\leq\epsilon, \\ \mathbb{Z}(\tau) = \varpi(\tau),\tau\in[0.5,1], \end{array}\right. \end{align} | (4.1) |
where
\begin{align*} &\mathbb{Z}(\tau)\in\mathscr{H}^{2}\big([0.5,2],\mathscr{R}\big), \\ &\mathcal{A}_{1}\big(\tau,\mathbb{Z}(\tau),\mathbb{Z}(0.5\tau)\big) = e^{0.5 \tau} \cos (\mathbb{Z}(\tau)), \\ &\mathcal{A}_{2}\big(\tau, \mathbb{Z}(\tau),\mathbb{Z}(0.5 \tau)\big) = \frac{\cos\big(\mathbb{Z}(0.5 \tau)\big)}{\sqrt{1+0.5 \tau}}. \end{align*} |
Where, the deterministic and stochastic parts of Eq (4.1) are e^{0.5 \tau} \cos (\mathbb{Z}(\tau)) and \frac{\cos\big(\mathbb{Z}(0.5 \tau)\big)}{\sqrt{1+0.5 \tau}} respectively. Now we demonstrate that, with relation to \epsilon , Eq (4.1) is UHS. Let \left(\tau, \mathbb{Z}_{1}, \mathbb{Z}_{2}\right) \in[1, 2] \times \mathscr{R} \times \mathscr{R} , therefore,
\begin{align*} &\big\Vert\mathcal{A}_{1}\big(\tau,\mathbb{Z}_{1},\widetilde{\mathbb{Z}}_{1}\big)-\mathcal{A}_{1}\big(\tau, \mathbb{Z}_{2}, \widetilde{\mathbb{Z}}_{2}\big)\big\Vert+\big\Vert\mathcal{A}_{2}\big(\tau,\mathbb{Z}_{1},\widetilde{\mathbb{Z}}_{1}\big)- \mathcal{A}_{2}\big(\tau, \mathbb{Z}_{2},\widetilde{\mathbb{Z}}_{2}\big)\big\Vert \\ \leq& e^{1}\bigg(\big\Vert\mathbb{Z}_{1}-\mathbb{Z}_{2}\big\Vert+\big\Vert\widetilde{\mathbb{Z}}_{1}-\widetilde{\mathbb{Z}}_{2}\big\Vert\bigg). \end{align*} |
This means that ( \mathscr{A}_{1} ) is satisfied. Furthermore,
\begin{equation*} \left\Vert\mathcal{A}_{2}(\cdot,0,0)\right\Vert_{\infty}\leq 1, \end{equation*} |
and
\begin{equation*} \int_{1}^{2}\left\Vert\mathcal{A}_{1}(\tau,0,0)\right\Vert^{2}d\tau \leq e^{2}. \end{equation*} |
The assumptions ( \mathscr{A}_{1} ) and ( \mathscr{A}_{2} ) are therefore met. Thus, with respect to \epsilon on [1, 2], Eq (4.1) is UHS utilizing Theorem 3.6. For Systems Eqs (4.1) and (4.2), we conduct a simulation based on the Euler-Maruyama scheme with a step size of 10^{-3} . We can see from Figure 1 that the distance between \mathscr{N}(\tau) and \mathbb{Z}(\tau) is less than a constant, and according to Definition 2.3, the solution of Eq (4.1) is UHS.
Example 4.2. Let \tau \in[1, 4] and the PFSDE for each \epsilon > 0 be provided by
\begin{align} \left\{\begin{array}{l} \mathcal{T}_{\tau}^{\ell} \mathbb{Z}(\tau) = \mathcal{A}_{1}(\tau,\mathbb{Z}(\tau),\mathbb{Z}(0.25 \tau))+\mathcal{A}_{2}(\tau,\mathbb{Z}(\tau),\mathbb{Z}(0.25 \tau)) \frac{d\mathscr{W}(\tau)}{d\tau}, \\ \mathscr{E}\Bigg[\bigg\Vert\mathbb{Z}(\tau)-\mathbb{Z}(1)- \Bigg(\int_{1}^{\tau}(\chi-1)^{\ell-1}\bigg(\mathcal{A}_{1}\big(\chi,\mathbb{Z}(\chi),\mathbb{Z}(0.25\chi)\big)d\chi \\ + \mathcal{A}_{2}\big(\chi,\mathbb{Z}(\chi),\mathbb{Z}(0.25\chi)\big)d\mathscr{W}(\chi)\bigg)\Bigg)\bigg\Vert^{2}\Bigg]\leq\epsilon,\\ \mathbb{Z}(\tau) = \varpi(\tau), \tau \in[0.25,1], \end{array}\right. \end{align} | (4.2) |
where
\begin{align*} &\mathbb{Z}(\tau) \in \mathscr{H}^{2}\big([0.25,4],\mathscr{R}\big),\\ &\mathcal{A}_{1}\big(\tau, \mathbb{Z}(\tau),\mathbb{Z}(0.25 \tau)\big) = \frac{\cos\mathbb{Z}(\tau)}{\sqrt{1+(0.25\tau)^{2}}}, \\ &\mathcal{A}_{2}\big(\tau, \mathbb{Z}(\tau),\mathbb{Z}(0.25 \tau)\big) = \frac{\mathbb{Z}(0.25 \tau)}{1+\tau^{2}}. \end{align*} |
Where, the deterministic and stochastic parts of the Eq (4.2) are \frac{\cos\mathbb{Z}(\tau)}{\sqrt{1+(0.25\tau)^{2}}} and \frac{\mathbb{Z}(0.25 \tau)}{1+\tau^{2}} respectively. Now, with regard to \epsilon , we demonstrate that Eq (4.2) is UHS. Assume that \left(\tau, \mathbb{Z}_{1}, \mathbb{Z}_{2}\right) \in[1, 4] \times \mathscr{R} \times \mathscr{R} , hence
\begin{align*} \big\Vert\mathcal{A}_{1}\big(\tau,\mathbb{Z}_{1},\widetilde{\mathbb{Z}}_{1}\big)-\mathcal{A}_{1}\big(\tau,\mathbb{Z}_{2}, \widetilde{\mathbb{Z}}_{2}\big)\big\Vert+\big\Vert\mathcal{A}_{2}\big(\tau, \mathbb{Z}_{1},\widetilde{\mathbb{Z}}_{1}\big)- \mathcal{A}_{2}\big(\tau,\mathbb{Z}_{2},\widetilde{\mathbb{Z}}_{2}\big)\big\Vert \leq \big\Vert\mathbb{Z}_{1}-\mathbb{Z}_{2}\big\Vert+\big\Vert\widetilde{\mathbb{Z}}_{1}-\widetilde{\mathbb{Z}}_{2}\big\Vert. \end{align*} |
Consequently, ( \mathscr{A}_{1} ) is met. Likewise
\begin{equation*} \left|\mathcal{A}_{2}(\cdot, 0)\right|_{\infty} = 0, \end{equation*} |
and
\begin{equation*} \int_{1}^{4}\left|\mathcal{A}_{1}(\tau, 0)\right|^{2} d \tau \leq \pi. \end{equation*} |
The assumptions ( \mathscr{A}_{1}) and ( \mathscr{A}_{2} ) are therefore met. Thus, with respect to \epsilon on [1, 4], Eq (4.2) is UHS employing Theorem 3.6. We can see from Figure 2 that the distance between \mathscr{N}(\tau) and \mathbb{Z}(\tau) is less than a constant, and according to Definition 2.3, the solution of Eq (4.2) is UHS.
Continuous dependency is a key property that contributes to the well-posedness of systems of differential equations, ensuring stability in the solutions by guaranteeing that small changes in parameters or initial conditions lead to correspondingly small changes in the solutions. Therefore, continuous dependency is closely tied to the overall stability and reliability of mathematical models described by differential equations. As both models presented in Examples 4.1 and 4.2 satisfy the criteria of continuous dependency, we conclude that both models are stable.
In the present paper, we examined the existence and uniqueness of the pantograph fractional stochastic differential equations using the Banach fixed point theorem. Second, we demonstrated that solutions continuously depend on the fractional order \ell and initial value. The fractional-order derivative is considered in the conformable sense. Using the generalized Gronwall inequalities and stochastic analytic methods, we examined the Ulam-Hyers stability of the considered problem. We demonstrated our findings with two examples in the final section.
We will use numerical techniques in our future work to solve various kinds of real-world challenges modeled with pantograph fractional stochastic differential equations.
The authors declare they have not used Artificial Intelligence (AI) tools in the creation of this article.
This work was supported by Princess Nourah bint Abdulrahman University Researchers Supporting Project number (PNURSP2024R157), Princess Nourah bint Abdulrahman University, Riyadh, Saudi Arabia. This study was supported via funding from Prince Sattam bin Abdulaziz University project number (PSAU/2024/R/1445). The authors are thankful to the Deanship of Graduate Studies and Scientific Research at the University of Bisha for supporting this work through the Fast-Track Research Support Program.
The authors declare that there are no conflicts of interest.
[1] | Y. A. Abramovich, C. D. Aliprantis, An invitation to operator theory, American Mathematical Society, 50 (2002). https://doi.org/10.1090/gsm/050 |
[2] |
A. A. Albanese, J. Bonet, W. J. Ricker, Grothendieck spaces with the Dunford-Pettis property, Positivity, 14 (2010), 145–164. https://doi.org/10.1007/s11117-009-0011-x doi: 10.1007/s11117-009-0011-x
![]() |
[3] | N. Alon, K. Makarychev, Y. Makarychev, A. Naor, Quadratic forms on graphs, Invent. Math., 163 (2006), 499–522. https://doi.org/10.1007/s00222-005-0465-9 |
[4] |
N. Alon, A. Naor, Approximating the cut-norm via Grothendieck's inequality, SIAM J. Comput., 35 (2006), 787–803. https://doi.org/10.1137/S0097539704441629 doi: 10.1137/S0097539704441629
![]() |
[5] | W. Arendt, A. Grabosch, G. Greiner, U. Moustakas, R. Nagel, U. Schlotterbeck, et al., One-parameter semigroups of positive operators, Lecture Notes in Mathematics, 1184 (1986). https://doi.org/10.1007/BFb0074922 |
[6] | S. V. Astashkin, L. Maligranda, L_p +L_{\infty} and L_p \cap L_{\infty} are not isomorphic for all 1 \leq p < \infty, p \neq 2. Proc. Amer. Math. Soc., 146 (2018), 2181–2194. https://doi.org/10.1090/proc/13928 |
[7] |
M. J. Beltrán-Meneu, M. C. Gómez-Collado, E. Jordá, D. Jornet, Mean ergodic composition operators on Banach spaces of holomorphic functions, J. Funct. Anal., 270 (2016), 4369–4385. https://doi.org/10.1016/j.jfa.2016.03.003 doi: 10.1016/j.jfa.2016.03.003
![]() |
[8] | T. F. Bewley, A very weak theorem on the existence of equilibria in atomless economies with infinitely many commodities, Equilibrium theory in infinite dimensional spaces, 1991,224–232. https://doi.org/10.1007/978-3-662-07071-0_9 |
[9] |
C. Bombach, D. Gallaun, C. Seifert, M. Tautenhahn, Observability and null-controllability for parabolic equations in L_p-spaces, Math. Control Relat. F., 13 (2023), 1484–1499. https://doi.org/10.3934/mcrf.2022046 doi: 10.3934/mcrf.2022046
![]() |
[10] |
J. Bonet, E. Jordá, A. Rodríguez, Mean ergodic multiplication operators on weighted spaces of continuous functions, Mediterr. J. Math., 15 (2018), 108. https://doi.org/10.1007/s00009-018-1150-8 doi: 10.1007/s00009-018-1150-8
![]() |
[11] |
J. Bourgain, On the Dunford-Pettis property, Proc. Amer. Math. Soc., 81 (1981), 265–272. https://doi.org/10.2307/2044207 doi: 10.2307/2044207
![]() |
[12] |
J. Bourgain, H^{\infty} is a Grothendieck space, Stud. Math., 75 (1983), 193–216. https://doi.org/10.4064/sm-75-2-193-216 doi: 10.4064/sm-75-2-193-216
![]() |
[13] |
J. Bourgain, The Dunford-Pettis property for the ball-algebras, the polydisc-algebras and the Sobolev spaces, Stud. Math., 77 (1984), 245–253. https://doi.org/10.4064/sm-77-3-246-253 doi: 10.4064/sm-77-3-246-253
![]() |
[14] | C. Budde, M. K. Fijavž, Bi-continuous semigroups for flows on infinite networks, 16 (2021), 553–567. https://doi.org/10.3934/nhm.2021017 |
[15] | J. Diestel, Grothendieck spaces and vector measures, Vector Operator Valued Meas. Appl., 1973, 97–108. https://doi.org/10.1016/B978-0-12-702450-9.50015-4 |
[16] | J. Diestel, A survey of results related to the Dunford-Pettis property, In: Proceedings of the Conference on Integration, Topology, and Geometry in Linear Spaces, 2 (1980), 15–60. https://doi.org/10.1090/conm/002/621850 |
[17] |
N. Dunford, B. J. Pettis, Linear operations on summable functions, Trans. Amer. Math. Soc., 47 (1940), 323–392. https://doi.org/10.1090/S0002-9947-1940-0002020-4 doi: 10.1090/S0002-9947-1940-0002020-4
![]() |
[18] | N. Dunford, J. T. Schwartz, Linear operators, I. General theory, New York and London: Interscience Publishers, 1988. |
[19] |
E. Emel'yanov, N. Erkursun, Lotz-Räbiger's nets of Markov operators in L^1-spaces, J. Math. Anal. Appl., 371 (2010), 777–783. https://doi.org/10.1016/j.jmaa.2010.05.060 doi: 10.1016/j.jmaa.2010.05.060
![]() |
[20] | K. J. Engel, R. Nagel, One-parameter semigroups for linear evolution equations, Graduate Texts in Mathematics, 194 (2000). https://doi.org/10.1007/b97696 |
[21] |
B. Farkas, Perturbations of bi-continuous semigroups, Stud. Math., 161 (2004), 147–161. https://doi.org/10.4064/sm161-2-3 doi: 10.4064/sm161-2-3
![]() |
[22] | D. H. Fremlin, Measure theory, Colchester: Torres Fremlin, 2003. |
[23] | J. A. Goldstein, Semigroups of linear operators and applications, Oxford: Oxford University Press, 1987. https://doi.org/10.1137/1029026 |
[24] |
M. González, T. Kania, Grothendieck spaces: The landscape and perspectives, Jpn. J. Math., 16 (2021), 247–313. https://doi.org/10.1007/s11537-021-2116-3 doi: 10.1007/s11537-021-2116-3
![]() |
[25] |
A. Grothendieck, Sur les applications linéaires faiblement compactes d'espaces du type C(K), Can. J. Math., 5 (1953), 129–173. https://doi.org/10.4153/CJM-1953-017-4 doi: 10.4153/CJM-1953-017-4
![]() |
[26] |
B. Jacob, F. L. Schwenninger, J. Wintermayr, A refinement of Baillon's theorem on maximal regularity, Stud. Math., 263 (2022), 141–158. https://doi.org/10.4064/sm200731-20-3 doi: 10.4064/sm200731-20-3
![]() |
[27] |
H. Keshavarzi, Mean ergodic composition operators on H^{\infty} (\mathbb{B}_n), Positivity, 26 (2022), 30. https://doi.org/10.1007/s11117-022-00901-5 doi: 10.1007/s11117-022-00901-5
![]() |
[28] |
A. Kishimoto, D. W. Robinson, Subordinate semigroups and order properties, J. Aust. Math. Soc. Ser. A, 31 (1981), 59–76. https://doi.org/10.1017/S1446788700018486 doi: 10.1017/S1446788700018486
![]() |
[29] |
F. Kühnemund, A Hille-Yosida theorem for bi-continuous semigroups, Semigroup Forum, 67 (2003), 205–225. https://doi.org/10.1007/s00233-002-5000-3 doi: 10.1007/s00233-002-5000-3
![]() |
[30] | H. P. Lotz, Tauberian theorems for operators on L^{\infty} and similar spaces, North-Holland Math. Stud., 90 (1984), 117–133. https://doi.org/10.1016/S0304-0208(08)71470-1 |
[31] |
H. P. Lotz, Uniform convergence of operators on L^{\infty} and similar spaces, Math. Z., 190 (1985), 207–220. https://doi.org/10.1007/BF01160459 doi: 10.1007/BF01160459
![]() |
[32] | G. Lumer, Evolution equations and their applications in physical and life sciences, Proceeding of the Bad Herrenalb (Karlsruhe) conference, New York, NY: Marcel Dekker, 2001. https://doi.org/10.1201/9780429187810 |
[33] | P. Meyer-Nieberg, Banach lattices, Berlin: Springer-Verlag, Universitext, 1991. https://doi.org/10.1007/978-3-642-76724-1 |
[34] | A. Pazy, Semigroups of linear operators and applications to partial differential equations, Applied Mathematical Sciences, 44 (1983). https://doi.org/10.1007/978-1-4612-5561-1 |
[35] | G. Pisier, Grothendieck's theorem, past and present, Bull. Amer. Math. Soc., 49 (2012), 237–323. https://doi.org/10.1090/S0273-0979-2011-01348-9 |
[36] | F. Räbiger, Beiträge zur Strukturtheorie der Grothendieck-Räume, Heidelberger: Sitzungsber, 1985. https://doi.org/10.1007/978-3-642-45612-1 |
[37] |
C. L. Rogers, Complete L_\infty -algebras and their homotopy theory, J. Pure Appl. Algebra, 227 (2023), 107403. https://doi.org/10.1016/j.jpaa.2023.107403 doi: 10.1016/j.jpaa.2023.107403
![]() |
[38] | H. H. Schaefer, Banach lattices and positive operators, New York-Heidelberg: Springer-Verlag, 1974. https://doi.org/10.1007/978-3-642-65970-6 |
[39] |
W. Seyoum, T. Mengestie, J. Bonet, Mean ergodic composition operators on generalized Fock spaces, RACSAM, 114 (2020), 6. https://doi.org/10.1007/s13398-019-00738-w doi: 10.1007/s13398-019-00738-w
![]() |
[40] |
S. Y. Shaw, Uniform convergence of ergodic limits and approximate solutions, Proc. Amer. Math. Soc., 114 (1992), 405–411. https://doi.org/10.2307/2159662 doi: 10.2307/2159662
![]() |
[41] |
J. M. A. M. van Neerven, A converse of Lotz's theorem on uniformly continuous semigroups, Proc. Amer. Math. Soc., 116 (1992), 525–527. https://doi.org/10.2307/2159762 doi: 10.2307/2159762
![]() |
[42] |
J. von Below, J. A. Lubary, The eigenvalues of the Laplacian on locally finite networks, Results Math., 47 (2005), 199–225. https://doi.org/10.1007/BF03323026 doi: 10.1007/BF03323026
![]() |
[43] |
J. von Below, J. A. Lubary, The eigenvalues of the Laplacian on locally finite networks under generalized node transition, Results Math., 54 (2009), 15–39. https://doi.org/10.1007/s00025-009-0376-y doi: 10.1007/s00025-009-0376-y
![]() |
[44] |
R. Wittmann, Schwach irreduzible Markoff-operatoren, Monatsh. Math., 105 (1988), 319–334. https://doi.org/10.1007/BF01318808 doi: 10.1007/BF01318808
![]() |
[45] |
A. J. Wrobel, A sufficient condition for a singular functional on L^\infty [0, 1] to be represented on \mathcal{C} [0, 1] by a singular measure, Indagat. Math. New Ser., 29 (2018), 746–751. https://doi.org/10.1016/j.indag.2017.12.005 doi: 10.1016/j.indag.2017.12.005
![]() |
[46] | K. Yosida, E. Hewitt, Finitely additive measures, Trans. Amer. Math. Soc., 72 (1952), 46–66. https://doi.org/10.2307/1990654 |
[47] |
Y. Zhang, C. C. Chen, Stochastic asymptotical regularization for linear inverse problems, Inverse Probl., 39 (2022), 015007. https://doi.org/10.1088/1361-6420/aca70f doi: 10.1088/1361-6420/aca70f
![]() |
[48] |
Y. Zhang, B. Hofmann, On the second-order asymptotical regularization of linear ill-posed inverse problems, Appl. Anal., 99 (2020), 1000–1025. https://doi.org/10.1080/00036811.2018.1517412 doi: 10.1080/00036811.2018.1517412
![]() |
1. |
Wedad Albalawi, Muhammad Imran Liaqat, Fahim Ud Din, Kottakkaran Sooppy Nisar, Abdel-Haleem Abdel-Aty,
The analysis of fractional neutral stochastic differential equations in |
|
2. | Abdelhamid Mohammed Djaouti, Muhammad Imran Liaqat, Qualitative Analysis for the Solutions of Fractional Stochastic Differential Equations, 2024, 13, 2075-1680, 438, 10.3390/axioms13070438 | |
3. | Akhtar Jan, Rehan Ali Shah, Hazrat Bilal, Bandar Almohsen, Rashid Jan, Bhupendra K. Sharma, Dynamics and stability analysis of enzymatic cooperative chemical reactions in biological systems with time-delayed effects, 2024, 11, 26668181, 100850, 10.1016/j.padiff.2024.100850 | |
4. | Zareen A. Khan, Muhammad Imran Liaqat, Ali Akgül, J. Alberto Conejero, Qualitative Analysis of Stochastic Caputo–Katugampola Fractional Differential Equations, 2024, 13, 2075-1680, 808, 10.3390/axioms13110808 |