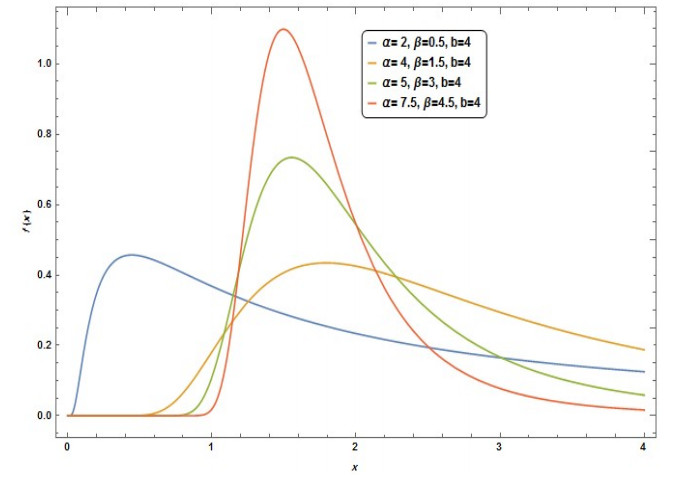
In this paper, we first constructed a semi-ring on a nexus and then defined a fuzzy sub-semi-ring associated with a nexus N. We investigated some properties and applications. Fuzzy versions of some well-known crisp concepts are provided over a nexus. We verified some applications of this fuzzy on semi-ring N. We obtained some relationships between sub-semi-ring and fuzzy sub-semi-ring of N. However, these relationships were not true for ideals. We put a condition on fuzzy sub-semi-ring so that these relationships were true for ideals. We defined strong fuzzy sub-semi-ring on N. For strong fuzzy sub-semi-ring on N and for every α∈[0,μ(0)], the level set μα was an ideal of N. For some strong fuzzy sub-semi-rings μ, we verified when μα was a prime ideal of N. In the following, for a semi-ring homomorphism f:N⟶M, we showed that if μ∈FSUBS(N), then f(μ)∈FSUBS(M) and if μ∈FSUBS(M) then f∘μ∈FSUBS(N). Finally, we verified some concepts of fuzzy quotient of a nexus semi-ring.
Citation: Vajiheh Nazemi Niya, Hojat Babaei, Akbar Rezaei. On fuzzy sub-semi-rings of nexuses[J]. AIMS Mathematics, 2024, 9(12): 36140-36157. doi: 10.3934/math.20241715
[1] | Mustafa Mudhesh, Hasanen A. Hammad, Eskandar Ameer, Muhammad Arshad, Fahd Jarad . Novel results on fixed-point methodologies for hybrid contraction mappings in Mb-metric spaces with an application. AIMS Mathematics, 2023, 8(1): 1530-1549. doi: 10.3934/math.2023077 |
[2] | Kumara Swamy Kalla, Sumati Kumari Panda, Thabet Abdeljawad, Aiman Mukheimer . Solving the system of nonlinear integral equations via rational contractions. AIMS Mathematics, 2021, 6(4): 3562-3582. doi: 10.3934/math.2021212 |
[3] | Niaz Ahmad, Nayyar Mehmood, Thabet Abdeljawad, Aiman Mukheimer . Krasnoselskii-type results for equiexpansive and equicontractive operators with application in radiative transfer equations. AIMS Mathematics, 2023, 8(6): 14592-14608. doi: 10.3934/math.2023746 |
[4] | Mohammed Shehu Shagari, Akbar Azam . Integral type contractions of soft set-valued maps with application to neutral differential equations. AIMS Mathematics, 2020, 5(1): 342-358. doi: 10.3934/math.2020023 |
[5] | Fatima M. Azmi . New fixed point results in double controlled metric type spaces with applications. AIMS Mathematics, 2023, 8(1): 1592-1609. doi: 10.3934/math.2023080 |
[6] | Ying Chang, Hongyan Guan . Generalized (αs,ξ,ℏ,τ)-Geraghty contractive mappings and common fixed point results in partial b-metric spaces. AIMS Mathematics, 2024, 9(7): 19299-19331. doi: 10.3934/math.2024940 |
[7] | Hasanen A. Hammad, Doha A. Kattan . Strong tripled fixed points under a new class of F-contractive mappings with supportive applications. AIMS Mathematics, 2025, 10(3): 5785-5805. doi: 10.3934/math.2025266 |
[8] | Saif Ur Rehman, Iqra Shamas, Shamoona Jabeen, Hassen Aydi, Manuel De La Sen . A novel approach of multi-valued contraction results on cone metric spaces with an application. AIMS Mathematics, 2023, 8(5): 12540-12558. doi: 10.3934/math.2023630 |
[9] | Mohamed Gamal, Tahair Rasham, Watcharaporn Cholamjiak, Fu-Gui Shi, Choonkil Park . New iterative scheme for fixed point results of weakly compatible maps in multiplicative GM−metric space via various contractions with application. AIMS Mathematics, 2022, 7(8): 13681-13703. doi: 10.3934/math.2022754 |
[10] | Shaoyuan Xu, Yan Han, Suzana Aleksić, Stojan Radenović . Fixed point results for nonlinear contractions of Perov type in abstract metric spaces with applications. AIMS Mathematics, 2022, 7(8): 14895-14921. doi: 10.3934/math.2022817 |
In this paper, we first constructed a semi-ring on a nexus and then defined a fuzzy sub-semi-ring associated with a nexus N. We investigated some properties and applications. Fuzzy versions of some well-known crisp concepts are provided over a nexus. We verified some applications of this fuzzy on semi-ring N. We obtained some relationships between sub-semi-ring and fuzzy sub-semi-ring of N. However, these relationships were not true for ideals. We put a condition on fuzzy sub-semi-ring so that these relationships were true for ideals. We defined strong fuzzy sub-semi-ring on N. For strong fuzzy sub-semi-ring on N and for every α∈[0,μ(0)], the level set μα was an ideal of N. For some strong fuzzy sub-semi-rings μ, we verified when μα was a prime ideal of N. In the following, for a semi-ring homomorphism f:N⟶M, we showed that if μ∈FSUBS(N), then f(μ)∈FSUBS(M) and if μ∈FSUBS(M) then f∘μ∈FSUBS(N). Finally, we verified some concepts of fuzzy quotient of a nexus semi-ring.
The family of probability distributions based on the Weibull distribution has been extensively used in numerous research papers. The Weibull distribution (WD) is a continuous probability distribution that was first studied by Fréchet [1] to describe the distribution of particle volume. Recently, it was noticed that the inverse Weibull distribution (IWD) is a very important distribution used in reliability analysis, such as, the depreciation of mechanical components, e.g., pistons and engines, see Balakrishnan[2].
Assume X is a random variable that follows the inverse Weibull distribution with two parameters, then the cumulative distribution function (CDF) and the probability density function (pdf) for x≥0 defined as
Q(x;α,β)=e−αx−β,x≥0,α>0,β>0 | (1.1) |
and
q(x;α,β)=αβx−(β+1)e−αx−β,x≥0,α>0,β>0, | (1.2) |
respectively, where α and β are the scale and shape parameters.
Corderio and Lemonte [3] proposed the parameter estimates for the inverse Weibull distribution using the maximum likelihood and least squares methods.
When we decrease the original distribution's domain to a smaller one, we get truncated distributions, which are conditional distributions. When it is impossible to determine what is happening above or below the phenomenon being examined, truncation is used. So, by setting the appropriate truncated value to the upper whisker limit, we employ a technique to exceed the outliers in the data. Behdani et al. [4] acknowledged that the Pareto distribution is a well-known long-tailed distribution and proposed a truncated variant to circumvent its long tail. Zaninetti [5] proposed a right and left truncated gamma distribution that serves as the distribution's upper and lower boundaries. The distribution's properties and features were studied. El-Din et al. [6] derived the probability density function of the mid-truncated Lindley distribution as a particular case. The failure of the components and units that make up an operating system's main structure was researched by statisticians in the fields of industrial and mechanical engineering. They employed statistical inference techniques to collect the data for their study, which also included examining the lifetime of the operational units until failure and predicting the reliability and risk functions for the entire system using data. The number of experimental units and the length of the lifetime experiment of some units must be limited because they are expensive and extremely valuable. Due to the time and cost constraints of the experiment, it is frequently necessary to remove items before they fail. In such cases, it is not possible for an experimenter to collect all of the sample data in a short amount of time during a lifetime test. This brings us to the subject of censoring, where some operating units are eliminated from the experiment due to a particular censoring scheme, see Aggarwala and Balakrishnan [7]. The progressive type-Ⅱ technique supports the demands of observing good estimators with no lifetime experimental lack. As the examiner assigns independent and identical units on the test, the progressive type-Ⅱ scheme can be observed and summarized as follows: Assume that n independent items are placed through a test with X1,X2,…,Xn failure times that are continuous and identically distributed. Assume that a censoring scheme (R1,R2,…,Rm) is predetermined so that immediately after the first failure X1,R1 surviving items are removed from the experiment at random, and immediately after the second failure X2,R2 surviving items are removed from the experiment at random. When the mth observed failure occurs, the remaining Rm surviving items are removed from the test, or Xm. The order statistics of size m that have been gradually type Ⅱ right-censored from a sample of size n using a progressive censoring scheme (R1,R2,…,Rm) are the m ordered observed failure times represented by X(R1,R2,…,Rm)1:m:n,X(R1,R2,…,Rm)2:m:n,…,X(R1,R2,…,Rm)m:m:n. There is no doubt that n=m+R1+R2+…+Rm. The particular situation of traditional type Ⅱ right-censored sampling occurs when R1=R2=…=Rm−1=0 and R=n−m. The progressive censoring scheme has been studied by many researchers, such as [8].
Many studies, like Meeker et al. [9], Mann et al. [10], Lawless [11], and Buzaridah et al. [12] have explored progressive type-Ⅱ censoring with varied failure time breakdowns. Balakrishnan [2] investigated the characteristics of progressively censored samples and reported that a collection of several advances in the inferential procedures depend on types-Ⅰ and -Ⅱ censored samples. A generalization of the progressive type-Ⅱ censoring was obtained, and it has more realistic applications, for example, Montanari et al. [13], as well as by Bairamov and Eryilmaz [14]. Additionally, Mahmoud et al. [15] presented a lifetime parameter estimation for a new extension of the exponential distribution with a mechanical application. Ramadan et al. [16] introduced the Bayesian estimation of some distributions under progressive type-Ⅱ censoring. Haj Ahmad et al. [17,18] used different models under a progressive type-Ⅱ censoring scheme and modeled water reservoir capacity, and the strength of aircraft's windows respectively. Shrahili et al. [19] derived some recurrence relations for the moments. Luo et al. [20] introduce modeling and estimation of a reliability system with a dynamic functioning area and some lifetime constraints. More work was achieved by many authors, for example, Buzaridah et al. [21] among others. This study's objective is to compare the two methods of inference with a new right-truncated Fréchet-inverted Weibull (RTFIW) distribution based on the progressive type-Ⅱ censoring scheme with an application.
The basic objective of creating these models is to truncate the original distribution's domain to a smaller one. Additionally, based on complete data, the asymptotic confidence intervals for the maximum likelihood estimator (MLE) of the unknown parameters were examined. In addition, the Markov chain Monte Carlo (MCMC) technique was derived for the Bayesian estimator. The purpose of this study is to present both traditional and Bayesian estimating methods for the unknown parameters of the RTFIW distribution under the progressive type-Ⅱ censoring scheme, where Bayesian methods are based on two different types of loss functions. In addition, a simulation study is conducted to evaluate the accuracy of the results and the quality of the loss function choice.
The contents of this paper are organized as follows: The structural characteristics of the RTFIW distribution are introduced in Section 2. In Section 3, we introduce order statistics, extreme values, the quantile function, the moments, and the moment-generating function. In Section 4, asymptotic confidence intervals based on the MLE are computed along with the point estimation of the parameters, the hazard rate, and reliability functions. In Section 5, methods are detailed to estimate the unknown parameters using Bayes estimation under the square error and the linear exponential (LINEX) loss function estimators. A simulation study is undertaken in Section 6, to evaluate the effectiveness of the different estimation methods developed in this study. In Section 7, two real data are examined. Finally, concluding remarks are summarized in Section 8.
The right truncated Fréchet-inverted Weibull (RTFIW) distribution has a CDF F(x) that can be obtained by
F(x;α,β,b)=Q(x;α,β)Q(b;α,β)=e−α(x−β−b−β),0<x≤b,α>0,β>0. | (2.1) |
If the cumulative distribution function of a random variable X is given by Eq (2.1), it can be given as
F(x;α,β,b)=e−α(x−β−b−β),0<x≤b,α>0,β>0, | (2.2) |
where α and β are the scale and shape parameters respectively. The value of b is a constant that represents the truncated part.
The probability density function pdf for RTFIW is given by
f(x;α,β,b)=ddxF(x)=αβxβ+1e−α(x−β−b−β),0<x≤b,α>0,β>0. | (2.3) |
The survival function S(x) and the hazard rate function h(t) for the RTFIW distribution are given by
S(x;α,β,b)=P(X>x)=1−F(x;α,β,b)=1−e−α(x−β−b−β),0<x≤b,α>0,β>0 | (2.4) |
and,
h(x;α,β,b)=f(x;α,β,b)S(x;α,β,b)=αβe−α(x−β−b−β)xβ+1(1−e−α(x−β−b−β)),0<x≤b,α>0,β>0, | (2.5) |
respectively.
Figures 1–4 depict the RTFIW distribution's density function, the cumulative distribution function, hazard rate, and survival function for various parameter choices. Figure 1 shows the behavior of the pdf when changing the parameters α,β where b=4. Figure 2 shows how the CDF behaves, affected by the change in parameters α,β where b=4. The behavior of CDF is increasing depending on the values of the parameters. Figure 3 demonstrates the behavior of S(x) when the parameters α,β are changed, where b=4, and how it decreases based on the parameter values. Figure 4 depicts how changing the settings affects the behavior of h(x). The behavior of h(x) may exhibit unimodality. Depending on the values of the parameters, it is either increasing or decreasing.
Now, we will propose several statistical characteristics of the RTFIW distribution.
The Quantile function has a variety of significant applications, including calculating the median, skewness, and kurtosis, as well as generating random variables, see Moors [22]. The quantile function of X is given by
x=Q(z)=F−1(z)=F−1(e−α(x−β−b−β)), | (3.1) |
where z∼uniform(0,1), it is simple to obtain random numbers using
x=(1b−β−1αlog(z))1β. | (3.2) |
By inputting z=0.5, the median of the RTEIW distribution from Eq (3.2) is defined as
median=(1b−β−1αlog(0.5))1β. | (3.3) |
By differentiating the probability density function of the RTEIW distribution at x>0 and making it equal to zero. The mode is defined as:
ddx(αβxβ+1e−α(x−β−b−β))=0 |
and
αβeαb−β[(−β−1)x−β−2e−αx−β+αβx−2β−2e−αx−β]=0. | (3.4) |
The moments of distributions are used to characterize several of the most essential model properties, such as dispersion, skewness, and kurtosis. As a result, the rth moment of the RTFIW distribution is given by
μ′r=E(xr)=∫b0xrf(x;α,β,b)dx, | (3.5) |
μ′r=∫b0xrαβxβ+1e−α(x−β−b−β)dx |
=αβeαb−β∫b0xr−β−1e−αx−βdx. |
Let −αx−β=t, we get
μ′r=αβeαb−β∫αb−β0(1αt)r−β−1e−t(1α)−1β−1βt−1β−1dt =αβeαb−β∫αb−β0(1α)r−β−1−1β(t)r−β−1−1β−1e−tdt, | (3.6) |
where γ(r−β−1β−1,αb−β) is the lower incomplete gamma function. Then,
μ′r=αβeαb−β(αb−β)r−β−1β−1Γ(r−β−1β−1)e−αb−β∞∑k=0(αb−β)kΓ(r−β−1β−1+αb−β)=αβ(αb−β)r−β−1β−1Γ(r−β−1β−1)∞∑k=0(αb−β)kΓ(r−β−1β+αb−β). | (3.7) |
The moment generating function (MGF) of the RTFIW distribution is the following:
MX(t)=∫∞0etxf(x;α,β,b)dx. | (3.8) |
As a result, using Taylor series to extend MX(t) produces
MX(t)=∫b0αβxβ+1etxe−α(x−β−b−β)dx=αβ∞∑r=0∫b0trxrr!x−β−1e−α(x−β−b−β)dx=∞∑r=0trr!μ′r. | (3.9) |
Hence the MGF of the RTFIWD is
MX(t)=∞∑r=0trr!αβ(αb−β)r−β−1β−1Γ(r−β−1β−1)∞∑k=0(αb−β)kΓ(r−β−1β+αb−β). |
The fields of reliability and testing provide several applications for order statistics. Numerous areas of statistical reasoning include the use of order statistics. Let X1, X2,....Xn represent a random sample of size n from the RTFIW with cumulative distribution function F(x) and probability density function f(x). Following that, order statistics are represented by the notations X (1)≤X(2)≤....X(n), X(1)=min(X1,X2,....Xn), and X(n)=max(X1,X2,....Xn). The pdf of the RTFIW's kth-order statistics is
f(k:n)(x)=n!(k−1)!(n−k)![αβxβ+1e−α(x−β−b−β)]×[e−α(x−β−b−β)]k−1×[1−e−α(x−β−b−β)]n−k. |
The pdf of f(1:n)(x)-order statistics when k=1 is
f(1:n)(x)=n[αβxβ+1e−α(x−β−b−β)]×[1−e−α(x−β−b−β)]n−1. |
The pdf of f(n:n)(x)-order statistics when k=n is
f(n:n)(x)=n[αβxβ+1e−α(x−β−b−β)]×[e−α(x−β−b−β)]n−1. |
Distribution of maximum, minimum, and median
Assume that X1,X2,...,Xn are samples selected at random from a continuous distribution with PDF and CDF. Now we will compute the PDF for X(1), the smallest value of the sample. In order statistics, it is usually easier to begin by considering the CDF. Then, the pdf for the minimum value from distribution is given by
fX(1)(x)=n[1−e−α(x−β−b−β)]n−1[αβxβ+1e−α(x−β−b−β)]. | (3.10) |
Then, the pdf for the maximum and median from the RTFIW distribution is given by
fX(n)(x)=n[e−α(x−β−b−β)]n−1[αβxβ+1e−α(x−β−b−β)] | (3.11) |
and
fmed(x)=(2m+1)!m!m!(e−α(x−β−b−β))m(1−e−α(x−β−b−β))m[αβxβ+1e−α(x−β−b−β)]. | (3.12) |
According to a progressive type-Ⅱ censored sample, see [23], with the RTFIW failures times X1:m:n,X2:m:n,....,Xm:m:n,1≤m≤n and the removal units are R1,R2,...,Rm, m<n, the likelihood function is expressed by
L(α,β,b∣x)=Cm∏i=1f(xi:m:n;α,β)[1−F(xi:m:n;α,β)]Ri,m<n, | (4.1) |
where C=n(n−R1−1)....(n−m−1∑i=1(Ri−1)), is a constant.
The likelihood function follows as
L(α,β,b∣x)=Cm∏i=1(αβxβ+1i:m:ne−α(x−βi:m:n−b−β))(1−e−α(x−βi:m:n−b−β))Ri=Cαmβmm∏i=1e−α(x−βi:m:n−b−β)m∏i=1xβ+1i:m:nm∏i=1(1−e−α(x−βi:m:n−b−β))Ri. | (4.2) |
The log-likelihood function is obtained as
ℓ∝mlogα+mlogβ−m∑i=1α(x−βi:m:n−b−β)−(β+1)m∑i=1log(xi:m:n)+m∑i=1Rilog(1−e−α(x−βi:m:n−b−β)). | (4.3) |
Notice that b is a constant. By computing the partial derivatives of ℓ with respect to α and β and equating each one to zero, we get,
∂ℓ∂α=mα−∑mi=1(x−βi:m:n−b−β)+∑mi=1Ri(xi:m:ne−α(x−βi:m:n−b−β)−b−βe−α(x−βi:m:n−b−β)1−e−α(x−βi:m:n−b−β)) | (4.4) |
and
∂ℓ∂β=mβ−m∑i=1log(xi:m:n)−m∑i=1α(b−βlog(b)−log(xi:m:n)x−βi:m:n)+m∑i=1Ri[αb−βe−α(x−βi:m:n−b−β)log(b)−αlog(xi:m:n)xi:m:ne−α(x−βi:m:n−b−β)1−e−α(x−βi:m:n−b−β)]. | (4.5) |
The estimates for Eqs (4.4) and (4.5) without a closed-form solution are obtained using the Newton-Raphson iteration method. Additionally, by substituting α and β with ˆα and ˆβ as shown, the MLEs of S(t) and h(t) can be determined using the invariance property of MLEs. The entries of the Fisher information matrix Iij= E{−[∂2ℓ(Ψ)/∂ψi∂ψj]}, where i,j=1,2 and Ψ=(ψ1,ψ2)=(α,β), represent the asymptotic variances and covariances of the MLEs, ˆα and ˆβ. Consequently, it is challenging to find the precise closed forms for the aforementioned demands. The observed Fisher information matrix ˆIij= {−[∂2ℓ(Ψ)/∂ψi∂ψj]}Ψ=ˆΨ, which is produced by eliminating the expectation operator E, which will be used to generate confidence intervals (CIs) for the parameters. This is represented by
ˆI(ˆα,ˆβ)=(−∂2ℓ∂α2−∂2ℓ∂α∂β−∂2ℓ∂β∂α−∂2ℓ∂β2)(α,β)=(ˆα,ˆβ), | (4.6) |
where
∂2ℓ∂α2=−mα2+m∑i=1[−Ri(−x−βi:m:n+b−β)2e−α(x−βi:m:n−b−β)1−e−α(x−βi:m:n−b−β)−Ri(−x−βi:m:n+b−β)2(e−α(x−βi:m:n−b−β))2(1−e−α(x−βi:m:n−b−β))2] | (4.7) |
∂2ℓ∂β2=−mβ2+mαb−βlog(b)2−m∑i=1αx−βi:m:nlog(xi:m:n)2+m∑i=1[Riα(x−βi:m:nlog(xi:m:n)2−b−βlog(b)2)e−α(x−βi:m:n−b−β)1−e−α(x−βi:m:n−b−β)−Riα2(−x−βi:m:nlog(xi:m:n)+b−βlog(b))2e−α(x−βi:m:n−b−β)1−e−α(x−βi:m:n−b−β)−Riα2(−x−βi:m:nlog(xi:m:n)+b−βlog(b))2(e−α(x−βi:m:n−b−β))2(1−e−α(x−βi:m:n−b−β))2] | (4.8) |
∂2ℓ∂α∂β=−mb−βlog(b)+m∑i=1x−βi:m:nlog(xi:m:n)+m∑i=1[Ri(−x−βi:m:nlog(xi:m:n)+b−βlog(b))e−α(x−βi:m:n−b−β)1−e−α(x−βi:m:n−b−β)+Riα(−x−βi:m:nlog(xi:m:n)+b−βlog(b))(−x−βi:m:n+b−β)e−α(x−βi:m:n−b−β)1−e−α(x−βi:m:n−b−β)+Riα(−x−βi:m:nlog(xi:m:n)+b−βlog(b))(−x−βi:m:n+b−β)(e−α(x−βi:m:n−b−β))2(1−e−α(x−βi:m:n−b−β))2] | (4.9) |
and
∂2ℓ∂β∂α=−mb−βlog(b)+m∑i=1x−βi:m:nlog(xi:m:n)+m∑i=1[Ri(−x−βi:m:nlog(xi:m:n)+b−βlog(b))e−α(x−βi:m:n−b−β)1−e−α(x−βi:m:n−b−β)+Riα(−x−βi:m:nlog(xi:m:n)+b−βlog(b))(−x−βi:m:n+b−β)e−α(x−βi:m:n−b−β)1−e−α(x−βi:m:n−b−β)+Riα(−x−βi:m:nlog(xi:m:n)+b−βlog(b))(−x−βi:m:n+b−β)(e−α(x−βi:m:n−b−β))2(1−e−α(x−βi:m:n−b−β))2]. | (4.10a) |
The observed information matrix ˆI(α,β) is inverted in order to derive the approximate asymptotic variance-covariance matrix [ˆV] for the MLEs, therefore, it is defined as
[ˆV]=ˆI−1(ˆα,ˆβ)=(Var(ˆα)cov(ˆα,ˆβ)cov(ˆβ,ˆα)Var(ˆβ)). | (4.11) |
A multivariate normal distribution with a mean (α,β) and covariance matrix I−1(α,β) is believed to be similar to the Normal distribution under certain regularity conditions, see Lawless [11]. The two-sided 100(1−γ)% confidence interval of α and β and can be obtained by
ˆα±Zγ2√Var(ˆα) | (4.12) |
and
ˆβ±Zγ2√Var(ˆβ), |
where Zγ2 represents the right-tail probability γ2 of the standard normal distribution.
It is also important to determine their variances in order to calculate the asymptotic CI of S(t) and h(t), which are functions in the parameters α and β. The approximation of estimation of the variance of ˆS(t) and ˆh(t) are computed using the delta approach, see Greene [24]. The variance of ˆS(t) and ˆh(t) can be estimated using this method, respectively, by
ˆσ2ˆS(t)=[∇ˆS(t)]T[ˆV][∇ˆS(t)]and ˆσ2ˆh(t)=[∇ˆh(t)]T[ˆV][∇ˆh(t)], |
where the gradient of ˆS(t)and ˆh(t) with respect to αand β are denoted by ∇ˆS(t)and ∇ˆh(t), respectively. Then, the (1−γ)100% two-sided confidence intervals of S(t) and h(t) can be provided by
ˆS(t)±Zγ2√ˆσ2ˆS(t) and ˆh(t)±Zγ2√ˆσ2ˆh(t). | (4.13) |
The Bayesian estimation of the RTFIW distribution based on progressive type-Ⅱ censoring is obtained. According to Zellner et al., [25], the following gamma prior distributions are applied when α and β are independent parameters.
{π1(α)=αa1−1exp(−b1α), α>0,π2(β)=βa2−1exp(−b2β), β>0, | (5.1) |
where, the hyper-parameters ai and bi, i=1,2 are non negative and known. Combining the likelihood function from Eq (4.3) and the priors from Eq (5.1) to get the posterior distribution of the parameters α and β, which is represented by π∗(α,β∣x)
π∗(α,β∣x)∝π1(α)π2(β)L(α,β∣x)∞∫0∞∫0π1(α)π2(β)L(α,β∣x)dαdβ. | (5.2) |
The square error loss function (SEL) is a symmetry-identical loss function that is frequently used. It is defined as the squared difference between the actual parameter ϕ and an estimator ˆϕ.
L(ϕ,ˆϕ)=(ˆϕ−ϕ)2. | (5.3) |
As a result, under the SEL function the Bayes estimator of any function of α and β, say g(α,β), can be calculated as follows
ˆgBS(α,β∣x)=Eα,β∣x_(g(α,β)). | (5.4) |
Hence,
Eα,β∣x_(g(α,β))=∞∫0∞∫0g(α,β)π1(α)π2(β)L(α,β∣x_)dαdβ∞∫0∞∫0π1(α)π2(β)L(α,β∣x_)dαdβ. | (5.5) |
For a parameter ϕ, the LINEX loss function (L(△)) is denoted by
L(△)=(ec△−c△−1), c≠0, △=ˆϕ−ϕ. | (5.6) |
LINEX loss is used to discuss Bayesian estimation and prediction. As a result, under the LINEX loss function, the Bayes estimate of a function g(α,β) is
ˆgBL(α,β∣x_)=−1clog[E(e−cg(α,β)∣x_)],c≠0, | (5.7) |
where
E(e−cg(α,β)∣x_)=∞∫0∞∫0e−cg(α,β)π1(α)π2(β)L(α,β∣x_)dαdβ∞∫0∞∫0π1(α)π2(β)L(α,β∣x_)dαdβ. | (5.8) |
where the calculations in Eqs (5.5) and (5.8) cannot be evaluated analytically. Thus, in Eq (5.2), the Markov chain Monte Carlo MCMC technique is employed to produce samples from the joint posterior density function. The Metropolis-Hasting (M-H) and Gibbs sampling methods are two popular MCMC approaches in statistics. From Eq (5.2), the joint posterior distribution is
π∗(α,β∣x)∝αm+a1−1βm+a2−1e−b1α−b2βm∏i=1xβ+1i:m:nm∏i=1e−α(x−βi:m:n−b−β)∏mi=1(1−e−α(x−βi:m:n−b−β))Ri. | (5.9) |
The posterior conditionals for α and β are
π∗1(α∣β,x)∝αm+a1−1e−b1αm∏i=1e−α(x−βi:m:n−b−β)m∏i=1(1−e−α(x−βi:m:n−b−β))Ri | (5.10) |
and
π∗2(β∣α,x)∝βm+a2−1e−b2βm∏i=1x−(β+1)i:m:nm∏i=1e−α(x−βi:m:n−b−β)∏mi=1(1−e−α(x−βi:m:n−b−β))Ri. | (5.11) |
However, the conditional posteriors of α and β in Eqs (5.10) and (5.11) do not have standard forms. Consequently, it is difficult to sample directly by standard methods; however, the plots presented in Figures 5 and 6 display that they have curves that are symmetric and hence, similar to the normal distribution.
As a result, Gibbs sampling is not an obvious choice, meaning the Metropolis-Hastings sampler is necessary for the MCMC approach implementations. Within Gibbs sampling, the Metropolis-Hastings method is as follows:
(1) Begin by including an accurate assumption (α(0),β(0)).
(2) Let k=1.
(3) Create α(k) and β(k) by using the M-H technique from π∗1(α(k−1)∣β(k),x_) and π∗2(β(k−1)∣α(k),x_) with standard proposed distributions N(α(k−1),var(α)) and N(β(k−1),var(β)), where var(α) and var(β) are the variance of parameters α and β respectively.
(4) Create a proposed α∗ from N(α(k−1),var(α)) and β∗ from N(β(k−1),var(α)).
(i) Compute the possibilities of acceptance.
ωα=min[1,π∗1(α∗∣β(k−1),x_)π∗1(α(j−1)∣β(k−1),x_)],ωβ=min[1,π∗2(β∗∣α(k),x_)π∗2(β(k−1)∣α(k),x_)],} |
(ii) Produce a Z1 and Z2 from a uniform distribution.
(iii) If Z1<ωα, accept the suggestion and put α(k)=α∗, else put α(k)=α(k−1).
(iv) If Z2<ωβ, accept the suggestion and put β(k)=β∗, else put β(k)=β(k−1).
(5) Evaluate h(k)(t) and S(k)(t).
(6) k=k+1.
(7) We can get α(l),β(l),S(l)(t) and h(l)(t),l=1,2,...N by repeating steps 3 to 6, N times.
(8) The 100(1−γ)% CRIs of ψj are (ψj(Nγ/2),ψj(N(1−γ/2))), that computes the CRs of α, β, S(t) and h(t) ψ(l)j,j=1,2,3,4,(ψ1,ψ2,ψ3,ψ4)=(α,β,S(t),h(t)) as ψ(1)j<ψ(2)j...<ψ(N)j.
The chosen samples are ψ(i)j,k=M+1,...N, for sufficiently large N. The Bayesian estimations of ψj based on the SEL function are given by
ˆψj=1N−MN∑k=M+1ψ(k). | (5.12) |
The Bayesian estimations ofψk from Eq (5.8) under the LINEX loss function are
ˆψj=−1clog[1N−MN∑k=M+1e−cφ(k)],j=1,2,3,4. | (5.13) |
For each simulation, 1000 progressive type-Ⅱ censored samples are used to evaluate parameter estimators, some lifetime parameters, and the RTFIW distribution's hazard and reliability functions. To create a sample size of progressive type-Ⅱ censored from the RTFIW distribution with initial values of α∘ = 0.5 and β∘ = 0.05. The mean square error (MSE) of the estimators of the results α,β,S(t) and h(t), at t=0.4, has been estimated based on a comparison of the various methods. The various approaches are used to estimate the 95% confidence intervals CIs derived from asymptotic distributions of the MLEs and CRIs. The coverage probability (CP) and the average confidence interval lengths (ACLs) are developed to assess the performance of estimation methods under study. The percentage of times the interval contains the interest-generating beginning value is known as a coverage probability (CP). In this study, the following progressive schemes are introduced:
● Scheme Ⅰ: R1=n−m,Rj=0for j≠1.
● Scheme Ⅱ: Rm2=Rm2+1=n−m2,Rj=0 for j≠m2 and j≠m2+1.
● Scheme Ⅲ: Rm=n−m,Rj=0for j≠m.
The results of the estimated parameters and their MSE are presented in Tables 1–4. Tables 5 and 6 present the results of the ACL and CP of the 95% CIs.
LINEX | ||||||
(n, m) | CS | MLE | SEL | c = - 2 | c = 0.0001 | c = 2 |
(30, 20) | Ⅰ | 0.3853 | 0.3843 | 0.3843 | 0.3842 | 0.3843 |
(0.0727) | (0.0739) | (0.0739) | (0.0738) | (0.0737) | ||
Ⅱ | 0.4046 | 0.4036 | 0.4037 | 0.4036 | 0.4036 | |
(0.063) | (0.0626) | (0.0626) | (0.0625) | (0.0624) | ||
Ⅲ | 0.4238 | 0.4237 | 0.4236 | 0.4237 | 0.4236 | |
(0.641) | (0.064) | (0.064) | (0.063) | (0.0639) | ||
(40, 15) | Ⅰ | 0.3794 | 0.3793 | 0.3794 | 0.3793 | 0.3792 |
(0.0783) | (0.0789) | (0.079) | (0.0785) | (0.0784) | ||
Ⅱ | 0.4256 | 0.4264 | 0.4265 | 0.4264 | 0.4263 | |
(0.0768) | (0.767) | (0.0767) | (0.0767) | (0.766) | ||
Ⅲ | 0.4564 | 0.4567 | 0.4569 | 0.4567 | 0.4565 | |
(0.071) | (0.0719) | (0.72) | (0.0716) | (0.714) | ||
(40, 20) | Ⅰ | 0.3784 | 0.3781 | 0.3781 | 0.3780 | 0.3779 |
(0.0689) | (0.0686) | (0.0689) | (0.0685) | (0.0684) | ||
Ⅱ | 0.3988 | 0.3989 | 0.399 | 0.43988 | 0.3987 | |
(0.0636) | (0.0639) | (0.0639) | (0.0631) | (0.0630) | ||
Ⅲ | 0.4364 | 0.4363 | 0.4364 | 0.4363 | 0.4362 | |
(0.0608) | (0.0613) | (0.0613) | (0.0611) | (0.0610) | ||
(50, 15) | Ⅰ | 0.3817 | 0.3815 | 0.3814 | 0.3810 | 0.3809 |
(0.0759) | (0.0748) | (0.0759) | (0.0749) | (0.0741) | ||
Ⅱ | 0.4338 | 0.4344 | 0.4346 | 0.4344 | 0.4342 | |
(0.081) | (0.0813) | (0.0814) | (0.0810) | (0.0807) | ||
Ⅲ | 0.4499 | 0.4496 | 0.4497 | 0.4496 | 0.4495 | |
(0.0683) | (0.0682) | (0.0683) | (0.0680) | (0.0677) | ||
(60, 15) | Ⅰ | 0.406 | 0.4058 | 0.406 | 0.4058 | 0.4057 |
(0.0883) | (0.0889) | (0.0889) | (0.0884) | (0.0880) | ||
Ⅱ | 0.4198 | 0.4197 | 0.4197 | 0.4196 | 0.4195 | |
(0.0783) | (0.0785) | (0.0786) | (0.0784) | (0.0779) | ||
Ⅲ | 0.4474 | 0.448 | 0.4482 | 0.4481 | 0.4477 | |
(0.0673) | (0.0684) | (0.0686) | (0.0683) | (0.0680) | ||
(60, 20) | Ⅰ | 0.407 | 0.4059 | 0.407 | 0.4059 | 0.4058 |
(0.0884) | (0.0890) | (0.0890) | (0.0885) | (0.0881) | ||
Ⅱ | 0.4199 | 0.4198 | 0.4198 | 0.4197 | 0.4196 | |
(0.0784) | (0.0784) | (0.0787) | (0.0785) | (0.0780) | ||
Ⅲ | 0.4475 | 0.449 | 0.4483 | 0.4482 | 0.4478 | |
(0.0675) | (0.0686) | (0.0687) | (0.0684) | (0.0683) |
LINEX | ||||||
(n, m) | CS | MLE | SEL | c = - 2 | c = 0.0001 | c = 2 |
(30, 20) | Ⅰ | 0.0633 | 0.0632 | 0.0632 | 0.0631 | 0.0630 |
(0.0008) | (0.0006) | (0.0008) | (0.0005) | (0.0004) | ||
Ⅱ | 0.0614 | 0.0613 | 0.0614 | 0.0612 | 0.0611 | |
(0.0006) | (0.0005) | (0.0005) | (0.0004) | (0.0003) | ||
Ⅲ | 0.06 | 0.06 | 0.063 | 0.062 | 0.060 | |
(0.0005) | (0.0004) | (0.0004) | (0.0003) | (0.0002) | ||
(40, 15) | Ⅰ | 0.0634 | 0.0633 | 0.0634 | 0.0633 | 0.0631 |
(0.0007) | (0.0006) | (0.0006) | (0.0005) | (0.0004) | ||
Ⅱ | 0.0608 | 0.0607 | 0.0607 | 0.0606 | 0.0605 | |
(0.0006) | (0.0005) | (0.0005) | (0.0004) | (0.0003) | ||
Ⅲ | 0.0582 | 0.0581 | 0.0581 | 0.0580 | 0.0579 | |
(0.0005) | (0.0004) | (0.0004) | (0.0003) | (0.0002) | ||
(40, 20) | Ⅰ | 0.0632 | 0.0628 | 0.0628 | 0.0627 | 0.0625 |
(0.0006) | (0.0005) | (0.0005) | (0.0004) | (0.0003) | ||
Ⅱ | 0.0617 | 0.0614 | 0.0614 | 0.0613 | 0.0612 | |
(0.0005) | (0.0004) | (0.0004) | (0.0003) | (0.0002) | ||
Ⅲ | 0.0586 | 0.0584 | 0.0584 | 0.0583 | 0.0582 | |
(0.0004) | (0.0003) | (0.0003) | (0.0002) | (0.0001) | ||
(50, 15) | Ⅰ | 0.063 | 0.062 | 0.062 | 0.061 | 0.060 |
(0.0006) | (0.0005) | (0.0005) | (0.0004) | (0.0003) | ||
Ⅱ | 0.0598 | 0.0597 | 0.0597 | 0.40596 | 0.0595 | |
(0.0006) | (0.0005) | (0.0005) | (0.0004) | (0.0003) | ||
Ⅲ | 0.0577 | 0.0577 | 0.0577 | 0.0576 | 0.0574 | |
(0.0004) | (0.0003) | (0.0003) | (0.0002) | (0.0001) | ||
(60, 15) | Ⅰ | 0.0608 | 0.0607 | 0.0607 | 0.0606 | 0.0675 |
(0.0004) | (0.0003) | (0.0003) | (0.0002) | (0.0001) | ||
Ⅱ | 0.0608 | 0.0607 | 0.0607 | 0.0606 | 0.40605 | |
(0.0005) | (0.0004) | (0.0004) | (0.0003) | (0.0002) | ||
Ⅲ | 0.0578 | 0.40579 | 0.0579 | 0.0578 | 0.0577 | |
(0.0005) | (0.0004) | (0.0004) | (0.0003) | (0.0002) | ||
(60, 20) | Ⅰ | 0.0609 | 0.0608 | 0.0608 | 0.0607 | 0.06076 |
(0.0005) | (0.0004) | (0.0004) | (0.0003) | (0.0002) | ||
Ⅱ | 0.0609 | 0.40608 | 0.40608 | 0.0607 | 0.0606 | |
(0.0006) | (0.0005) | (0.0005) | (0.0004) | (0.0003) | ||
Ⅲ | 0.0579 | 0.0580 | 0.0580 | 0.0579 | 0.0578 | |
(0.0006) | (0.0005) | (0.0005) | (0.0004) | (0.0003) |
LINEX | ||||||
(n, m) | CS | MLE | SEL | c = - 2 | c = 0.0001 | c = 2 |
(30, 20) | Ⅰ | 0.0457 | 0.0455 | 0.0455 | 0.0454 | 0.0453 |
(0.0003) | (0.0004) | (0.0004) | (0.0003) | (0.0002) | ||
Ⅱ | 0.0475 | 0.0474 | 0.0474 | 0.0472 | 0.0471 | |
(0.0004) | (0.0003) | (0.0003) | (0.0002) | (0.0001) | ||
Ⅲ | 0.0489 | 0.0488 | 0.0488 | 0.0487 | 0.0486 | |
(0.0004) | (0.0003) | (0.0003) | (0.0002) | (0.0001) | ||
(40, 15) | Ⅰ | 0.0449 | 0.0448 | 0.0448 | 0.0447 | 0.0446 |
(0.005) | (0.004) | (0.004) | (0.003) | (0.002) | ||
Ⅱ | 0.0479 | 0.0478 | 0.0478 | 0.0477 | 0.0476 | |
(0.0005) | (0.0004) | (0.0004) | (0.0003) | (0.0001) | ||
Ⅲ | 0.0505 | 0.0504 | 0.0504 | 0.0503 | 0.0502 | |
(0.0005) | (0.0004) | (0.0004) | (0.0003) | (0.0001) | ||
(40, 20) | Ⅰ | 0.0455 | 0.0454 | 0.0454 | 0.0453 | 0.0452 |
(0.0004) | (0.0003) | (0.0003) | (0.0002) | (0.0001) | ||
Ⅱ | 0.048 | 0.047 | 0.047 | 0.046 | 0.045 | |
(0.0004) | (0.0003) | (0.0003) | (0.0002) | (0.0001) | ||
Ⅲ | 0.0495 | 0.0494 | 0.0494 | 0.0493 | 0.0492 | |
(0.0004) | (0.0003) | (0.0003) | (0.0002) | (0.0001) | ||
(50, 15) | Ⅰ | 0.0446 | 0.0445 | 0.0445 | 0.0444 | 0.0443 |
(0.0004) | (0.0004) | (0.0004) | (0.0004) | (0.0004) | ||
Ⅱ | 0.048 | 0.0479 | 0.0479 | 0.0478 | 0.0477 | |
(0.0006) | (0.0005) | (0.0005) | (0.0004) | (0.0002) | ||
Ⅲ | 0.0499 | 0.0498 | 0.0498 | 0.0497 | 0.0495 | |
(0.0006) | (0.0004) | (0.0004) | (0.0002) | (0.0001) | ||
(60, 15) | Ⅰ | 0.0457 | 0.0456 | 0.0456 | 0.0455 | 0.0453 |
(0.0006) | (0.0006) | (0.0004) | (0.0003) | (0.0002) | ||
Ⅱ | 0.0473 | 0.0472 | 0.0472 | 0.0471 | 0.0470 | |
(0.0007) | (0.0006) | (0.0006) | (0.0004) | (0.0003) | ||
Ⅲ | 0.0497 | 0.0496 | 0.0496 | 0.0495 | 0.0494 | |
(0.0004) | (0.0003) | (0.0003) | (0.0002) | (0.0001) | ||
(60, 20) | Ⅰ | 0.0458 | 0.0457 | 0.0457 | 0.0456 | 0.0454 |
(0.0007) | (0.0006) | (0.0006) | (0.0004) | (0.0002) | ||
Ⅱ | 0.0474 | 0.0473 | 0.0473 | 0.0472 | 0.0471 | |
(0.0008) | (0.0007) | (0.0007) | (0.0005) | (0.0004) | ||
Ⅲ | 0.0498 | 0.0497 | 0.0497 | 0.0496 | 0.0495 | |
(0.0005) | (0.0004) | (0.0004) | (0.0003) | (0.0002) |
LINEX | ||||||
(n, m) | CS | MLE | SEL | c = - 2 | c = 0.0001 | c = 2 |
(30, 20) | Ⅰ | 1.1289 | 1.1288 | 1.1288 | 1.1287 | 1.1286 |
(0.0019) | (0.0016) | (0.0016) | (0.0014) | (0.0012) | ||
Ⅱ | 1.1253 | 1.1252 | 1.1252 | 1.1251 | 1.1250 | |
(0.0015) | (0.0013) | (0.0013) | (0.0012) | (0.0011) | ||
Ⅲ | 1.1229 | 1.1229 | 1.1229 | 1.1227 | 1.1226 | |
(0.0013) | (0.0012) | (0.0012) | (0.0011) | (0.0010) | ||
(40, 15) | Ⅰ | 1.1295 | 1.1294 | 1.1294 | 1.1293 | 1.1292 |
(0.0017) | (0.0016) | (0.0016) | (0.0014) | (0.0013) | ||
Ⅱ | 1.1242 | 1.1241 | 1.1241 | 1.1240 | 1.1239 | |
(0.0015) | (0.0013) | (0.0013) | (0.0011) | (0.0010) | ||
Ⅲ | 1.1193 | 1.1192 | 1.1192 | 1.1191 | 1.1190 | |
(0.0011) | (0.0009) | (0.0009) | (0.0008) | (0.0007) | ||
(40, 20) | Ⅰ | 1.1283 | 1.1282 | 1.1282 | 1.1281 | 1.1280 |
(0.0015) | (0.0014) | (0.0014) | (0.0013) | (0.0011) | ||
Ⅱ | 1.1256 | 1.1255 | 1.1255 | 1.1254 | 1.1252 | |
(0.0013) | (0.0012) | (0.0012) | (0.0011) | (0.0010) | ||
Ⅲ | 1.1203 | 1.1202 | 1.1202 | 1.1201 | 1.1200 | |
(0.004) | (0.003) | (0.003) | (0.002) | (0.001) | ||
(50, 15) | Ⅰ | 1.1279 | 1.1278 | 1.1278 | 1.1277 | 1.1276 |
(0.0016) | (0.0015) | (0.0015) | (0.0014) | (0.0012) | ||
Ⅱ | 1.1229 | 1.1228 | 1.1228 | 1.1227 | 1.1226 | |
(0.0019) | (0.0017) | (0.0017) | (0.0016) | (0.0014) | ||
Ⅲ | 1.1192 | 1.1191 | 1.1191 | 1.1190 | 1.1189 | |
(0.0015) | (0.0014) | (0.0014) | (0.0013) | (0.0011) | ||
(60, 15) | Ⅰ | 1.1256 | 1.1255 | 1.1255 | 1.1254 | 1.1253 |
(0.0015) | (0.0014) | (0.0014) | (0.0013) | (0.0012) | ||
Ⅱ | 1.1247 | 1.1246 | 1.1246 | 1.1245 | 1.1244 | |
(0.0016) | (0.0015) | (0.0015) | (0.0014) | (0.0012) | ||
Ⅲ | 1.1195 | 1.1194 | 1.1194 | 1.1193 | 1.1192 | |
(0.0016) | (0.0015) | (0.0015) | (0.0013) | (0.0011) | ||
(60, 20) | Ⅰ | 1.1257 | 1.1256 | 1.1256 | 1.1255 | 1.1254 |
(0.0016) | (0.0015) | (0.0015) | (0.0014) | (0.0013) | ||
Ⅱ | 1.1248 | 1.1247 | 1.1247 | 1.1246 | 1.1245 | |
(0.0017) | (0.0016) | (0.0016) | (0.0015) | (0.0013) | ||
Ⅲ | 1.1196 | 1.1195 | 1.1195 | 1.1194 | 1.1193 | |
(0.0017) | (0.0016) | (0.0016) | (0.0015) | (0.0012) |
α | β | ||||
(n, m) | CS | MLE | MCMC | MLE | MCMC |
(30, 20) | Ⅰ | 1.1444 | 0.0234 | 0.0751 | 0.0016 |
(0.9435) | (0.9446) | (0.9332) | (0.9418) | ||
Ⅱ | 1.1063 | 0.0236 | 0.0714 | 0.0015 | |
(0.9252) | (0.9367) | (0.9405) | (0.942) | ||
Ⅲ | 1.1302 | 0.0243 | 0.0688 | 0.0015 | |
(0.9572) | (0.9253) | (0.9396) | (0.9549) | ||
(40, 15) | Ⅰ | 1.2277 | 0.0268 | 0.0781 | 0.0017 |
(0.9698) | (0.9507) | (0.9377) | (0.9456) | ||
Ⅱ | 1.3317 | 0.0283 | 0.078 | 0.0016 | |
(0.9625) | (0.947) | (0.9608) | (0.9655) | ||
Ⅲ | 1.301 | 0.0281 | 0.0716 | 0.0015 | |
(0.951) | (0.9606) | (0.9712) | (0.9447) | ||
(40, 20) | Ⅰ | 1.0855 | 0.0231 | 0.0707 | 0.0015 |
(0.9615) | (0.9566) | (0.9573) | (0.9539) | ||
Ⅱ | 1.0899 | 0.0232 | 0.0698 | 0.0015 | |
(0.9256) | (0.947) | (0.9408) | (0.959) | ||
Ⅲ | 1.077 | 0.0227 | 0.0632 | 0.0013 | |
(0.9468) | (0.9568) | (0.9461) | (0.9616) | ||
(50, 15) | Ⅰ | 1.2419 | 0.0262 | 0.0754 | 0.0016 |
(0.9325) | (0.9845) | (0.9781) | (0.9863) | ||
Ⅱ | 1.3984 | 0.0293 | 0.0776 | 0.0016 | |
(0.9124) | (0.9683) | (0.9786) | (0.9452) | ||
Ⅲ | 1.2576 | 0.0274 | 0.0694 | 0.0015 | |
(0.9568) | (0.9565) | (0.9468) | (0.9478) | ||
(60, 15) | Ⅰ | 1.2813 | 0.0269 | 0.0719 | 0.0015 |
(0.9461) | (0.9616) | (0.9256) | (0.947) | ||
Ⅱ | 1.2959 | 0.0278 | 0.075 | 0.0016 | |
(0.9605) | (0.9641) | (0.9468) | (0.9568) | ||
Ⅲ | 1.2552 | 0.0275 | 0.0686 | 0.0015 | |
(0.9416) | (0.9486) | (0.9568) | (0.9665) | ||
(60, 20) | Ⅰ | 1.2832 | 0.0270 | 0.0720 | 0.0016 |
(0.9462) | (0.9617) | (0.9257) | (0.948) | ||
Ⅱ | 1.2960 | 0.0279 | 0.076 | 0.0017 | |
(0.9606) | (0.9642) | (0.94670) | (0.9569) | ||
Ⅲ | 1.2553 | 0.0276 | 0.0687 | 0.0016 | |
(0.9417) | (0.9487) | (0.9569) | (0.9666) |
S(t) | h(t) | ||||
(n, m) | CS | MLE | MCMC | MLE | MCMC |
(30, 20) | Ⅰ | 0.0755 | 0.0029 | 1.2694 | 0.0022 |
(0.9511) | (0.9489) | (0.952) | (0.9472) | ||
Ⅱ | 0.10705 | 0.003 | 1.2627 | 0.0021 | |
(0.9626) | (0.9546) | (0.9539) | (0.9278) | ||
Ⅲ | 0.0687 | 0.003 | 1.2572 | 0.0021 | |
(0.9576) | (0.9661) | (0.9361) | (0.9429) | ||
(40, 15) | Ⅰ | 0.0836 | 0.0033 | 1.2711 | 0.0024 |
(0.9421) | (0.9507) | (0.927) | (0.9296) | ||
Ⅱ | 0.081 | 0.0034 | 1.2593 | 0.0024 | |
(0.9325) | (0.9458) | (0.9736) | (0.9856) | ||
Ⅲ | 0.0748 | 0.0033 | 1.2469 | 0.0022 | |
(0.958) | (0.9603) | (0.9601) | (0.9602) | ||
(40, 20) | Ⅰ | 0.0739 | 0.0029 | 1.2693 | 0.0022 |
(0.9462) | (0.9467) | (0.9502) | (0.9506) | ||
Ⅱ | 0.07 | 0.0029 | 1.2633 | 0.0021 | |
(0.9256) | (0.9592) | (0.9704) | (0.9694) | ||
Ⅲ | 0.0645 | 0.00327 | 1.252 | 0.0019 | |
(0.9544) | (0.9581) | (0.9382) | (0.9454) | ||
(50, 15) | Ⅰ | 0.0839 | 0.0032 | 1.2671 | 0.0024 |
(0.9814) | (0.9145) | (0.9745) | (0.9635) | ||
Ⅱ | 0.0832 | 0.0035 | 1.2541 | 0.0023 | |
(0.9748) | (0.9862) | (0.9742) | (0.9681) | ||
Ⅲ | 0.0735 | 0.0032 | 1.2483 | 0.0022 | |
(0.9254) | (0.9438) | (0.9152) | (0.9634) | ||
(60, 15) | Ⅰ | 0.0835 | 0.0032 | 1.2625 | 0.0023 |
(0.9637) | (0.9504) | (0.9667) | (0.9567) | ||
Ⅱ | 0.0803 | 0.0033 | 1.2605 | 0.0024 | |
(0.9617) | (0.9325) | (0.9486) | (0.9479) | ||
Ⅲ | 0.0735 | 0.0032 | 1.2489 | 0.0021 | |
(0.9672) | (0.9488) | (0.9457) | (0.9639) | ||
(60, 20) | Ⅰ | 0.0836 | 0.0033 | 1.2626 | 0.0024 |
(0.9638) | (0.9505) | (0.9668) | (0.9568) | ||
Ⅱ | 0.0804 | 0.0034 | 1.2606 | 0.0025 | |
(0.9618) | (0.9326) | (0.9487) | (0.9480) | ||
Ⅲ | 0.0736 | 0.0033 | 1.2490 | 0.0022 | |
(0.9673) | (0.9489) | (0.9458) | (0.940) |
The following conclusions can be made based on the results:
(1) According to Tables 1–4, MSEs decrease as sample size increases, and Bayes estimates get the smallest MSEs for α, β,S(t)and h(t). As a result, in every case that has been studied, Bayes estimates outperform MLE approaches.
(2) With c=2, the Bayes estimator under LINEX provides superior results in terms of smaller MSEs.
(3) Scheme Ⅱ is better than schemes Ⅰ and Ⅲ in terms of decreased MSEs for a specified sample n and m effective size values.
(4) It is evident from Tables 5 and 6 that, for various sample sizes, observed failures, and schemes, CRIs provide more accurate results than ACIs.
In the following subsections, two real data selected from the medical field are examined. The purpose of this study is to test the suitability of the proposed RTFIW distribution with these data sets.
The first data set of failure numbers for 20 patients' analgesic relief times (in minutes) is used for the suggested estimation methods. This lifetime data set was introduced by Gross and Clark [26] and is associated with relief times (in minutes) of patients taking an analgesic. These data are provided in Table 7. By comparing the new model RTFIW with the modified Fréchet-exponential distribution (MFED) and exponentiated exponential (EE) under the data set where the value of b is the maximum value of the data. Table 8 shows the MLEs for the distributions. To assess the fitted models, the following measures were used: Bayesian information criterion (BIC), consistent Akaike information criterion (CAIC), Akaike information criterion (AIC), Hannan-Quinn information criterion (HQIC), Kolmogorov-Smirnov (K-S) and the negative log-likelihood (-Log), to seek out the model with the lowest information criterion and good fit parameters. The numerical results in Table 9 show that the RTFIW model fits the data better than other models. The considered data is fitted as shown in Figure 7.
1.1 | 1.4 | 1.3 | 1.7 | 1.9 | 1.8 | 1.6 | 2.2 | 1.7 | 2.7 |
1.8 | 1.5 | 1.2 | 1.4 | 3 | 1.7 | 2.3 | 1.6 | 2 | 4.1 |
Model | α | β | θ | λ |
MFED | 32.433 | - | 2.0038 | - |
EE | 36.68 | - | 2.235 | |
RTFIW | 6.7199 | 3.7354 | - | - |
Model | K–S | p-Value | -logL | AIC | BIC | HQIC |
MFED | 0.120612 | 0.899975 | 15.9512 | 35.9024 | 37.8938 | 36.2911 |
EE | 0.1343 | 0.817352 | 16.2606 | 36.5212 | 38.5125 | 36.91 |
RTFIW | 0.100217 | 0.955062 | 14.9239 | 33.8478 | 35.8392 | 35.6581 |
The empirical failure data distribution's Kolmogorov-Smirnov (K-S) distance from the RTFIW distribution's CDF is 0.100217, with a P-value of 0.955062. As a result, the RTFIW distribution fits the provided data. From n=20 failure data, a progressive type-Ⅱ censored a sample of size m=10 with R=(6,4,0,0,0,0,0,0,0,0) based on the information that these generated. The MLE are computed to be ˆα,ˆβ,ˆS(t) and ˆh(t) such that t=0.4 depend on the progressive type-Ⅱ data reported in Table 7 are shown in Table 10. The Bayes estimator in relation to SEL and LINEX functions are calculated and are also shown in Table 10. From Table 11, the 95% ACIs and CRIs are computed for parameters. Additionally, the 95% ACIs and CRIs for S(t) and h(t) are derived using the delta approach, with the results displayed in Table 11.
Baysian | |||||
Parameters | MLE | SLE | LINEX | ||
c = - 2 | c = 0.0001 | c = 2 | |||
α | 1.16548 | 1.15804 | 1.15869 | 1.15804 | 1.15739 |
β | 2.85092 | 2.78356 | 2.796 | 2.78356 | 2.76981 |
S | 0.999772 | 0.99954 | 0.999541 | 0.99954 | 0.99954 |
h | 0.0109181 | 0.0184367 | 0.0185916 | 0.0184367 | 0.0182849 |
Parameter | MLE | MCMC |
α | (0.337871, 1.99309) | (1.11849, 1.2089) |
β | (0.8467, 4.85513) | (2.55267, 2.95076) |
S(0.4) | (0.996234, 1.00331) | (0.998601, 0.999898) |
h(0.4) | (−0.131477, 0.153313) | (0.00553594, 0.0472466) |
Substituting the MLEs of the unknown parameters α and β for the data set into the inverse of the observed information matrix, we get an estimation of the variance-covariance matrix as follows:
I−1=(3.562041.097231.097230.607173).
The two sided 95% confidence interval ranges are [1.9207,13.7014] and [2.5453,4.92549], for the parameters α and β respectively.
The second data set given by Abouammoh et al. [27] represents 40 patients suffering from leukemia in one of the Ministry of Health Hospitals in Saudi Arabia. The survival times of those patients are recorded as follows:
11518125541844146151673974392498310241062106311651191122212517898078651277129013571357136914081369140814551455147812221251127712901455147812221549157815781599160316051852169617351815 |
We aim to compare the new model RTFIW distribution with exponentiated generalized inverse Weibull (EGIW) distribution and generalized inverse Weibull (GIW) distribution under the data set where the value of b is the maximum value of the data.
Table 12 shows the MLEs for the distributions. To assess the fitted models, the following measures were used: Bayesian information criterion (BIC), Consistent Akaike information criterion (CAIC), Akaike information criterion (AIC), Hannan-Quinn information criterion (HQIC), Kolmogorov-Smirnov (K-S) and the negative log-likelihood (-Log), to seek out the model with the lowest information criterion and good fit parameters. The numerical results in Table 13 show that the RTFIW model fits the data better than other models. The considered data is fitted as shown in Figure 8.
Model | α | β | θ | λ |
GIW | 0.792 | 0.298 | 3.261 | |
EGIW | .933 | 1.09 | 0.453 | 1.102 |
RTFIWD | 1.10716 | 1.37948 |
Model | K−S | P−Value | -2logL | AIC | BIC | CAIC |
GIW | 0.737 | 0.7584 | 795.582 | 801.582 | 806.648 | 802.248 |
EGIW | 0.831 | .82464 | 709.471 | 717.471 | 724.226 | 718.614 |
RTFIWD | 1.001 | 0.9744 | 594.72585 | 598.7258 | 602.1036 | 598.325 |
From n=40 failure data, a progressive type-Ⅱ censored a sample of size m= 20 with R=(10,5,5,0,0,0,0,0,0,0,0,0,0,0,0,0,0,0,0,0) based on the information that these generated. The MLEs computed for ˆα,ˆβ,ˆS(t) and ˆh(t) such that t=0.4 and b=1815 based on the progressive type-Ⅱ data are shown in Table 14. The Bayes estimates of SEL and LINEX functions are calculated and are also shown in Table 14.
Parameters | MLE | Baysian | |||
SEL | LINEX | ||||
c=−2 | c=0.0001 | c=2 | |||
α | 1.10716 | 1.11602 | 1.11604 | 1.11601 | 1.11602 |
β | 1.37948 | 1.3736 | 1.37364 | 1.37357 | 1.3736 |
S | 0.94189 | 0.942492 | 0.942492 | 0.942491 | 0.942492 |
h | 0.490308 | 0.484726 | 0.484731 | 0.48472 | 0.484726 |
From Table 15, the 95% ACIs and CRIs are computed for parameters. Furthermore, the delta method is employed to derive the 95% ACIs and CRIs for S(t) and h(t), hence, the results are shown in Table 15.
Parameter | MLE | MCMC |
α | (0.545939,1.66837) | (1.1084,1.12323) |
β | (0.701971,2.057) | (1.36354,1.38614) |
S(0.4) | (0.821262,1.06252) | (0.941573,0.94353) |
h(0.4) | (−0.0904752,1.07109) | (0.478703,0.489044) |
This study introduces a new truncated distribution called the RTFIW distribution. The new distribution's statistical properties are derived. Using progressive type-Ⅱ censoring, this study aims to provide a number of methods for generating confidence intervals for RTFIW distribution parameters and derivations. The time-relief periods of patients receiving analgesic treatment were studied in addition to Leukemia patient recovery numbers by using various estimation techniques proposed in this study. Different confidence intervals are provided when the MLEs of the unknown parameters are calculated. On the other hand, a simulation study was carried out to assess the accuracy of the suggested estimations. As a result, it is demonstrated that Bayesian approaches perform well in all cases. Due to the pattern of RTFIW's good fit to the applied data, Scheme Ⅱ also performs better than Schemes Ⅰ and Ⅲ in terms of smaller MSEs. In future works, estimation of the RTFIW distribution's parameters using adaptive progressive type-Ⅱ censored data can be obtained, and also can be compared with other types of censored algorithms.
The authors declare they have not used Artificial Intelligence (AI) tools in the creation of this article.
This work was supported by the Deanship of Scientific Research, Vice Presidency for Graduate Studies and Scientific Research, King Faisal University, Saudi Arabia [GRANT No. 5023].
The authors declare no conflict of interest.
[1] |
S. Abdurrahman, Karakteristin fuzzy subsemiring, J. Fourier, 9 (2020), 19–23. https://doi.org/10.14421/fourier.2020.91.19-23 doi: 10.14421/fourier.2020.91.19-23
![]() |
[2] |
S. Abou-Zaid, On generalized characteristic fuzzy subgroups of a finite group,
J. Fuzzy sets Syst., 43 (1991), 235–241. https://doi.org/10.1016/0165-0114(91)90080-A doi: 10.1016/0165-0114(91)90080-A
![]() |
[3] |
D. Afkhami Taba, A. Hasankhani, M. Bolourian, Soft nexuses, Comput. Math. Appl., 64 (2012), 1812–1821. https://doi.org/10.1016/j.camwa.2012.02.048 doi: 10.1016/j.camwa.2012.02.048
![]() |
[4] |
D. Alghazzwi, A. Ali, A. Almutlg, E. A. Abo-Tabl, A. A. Azzam, A novel structure of q-rung orthopair fuzzy sets in ring theory, AIMS Mathematics, 8 (2023), 8365–8385. https://doi.org/10.3934/math.2023422 doi: 10.3934/math.2023422
![]() |
[5] | M. Bolourian, Theory of plenices, PhD thesis, University of Surrey, 2009. |
[6] |
M. Bolourian, R, Kamrani, A. Hasankhani, The Structure of Moduloid on a Nexus, Mathematics, 7 (2019), 82. https://doi.org/10.3390/math7010082 doi: 10.3390/math7010082
![]() |
[7] | A. A. Estaji, T. Haghdadi, J. Farokhi, Fuzzy nexus over an ordinal, J. Algebr. Syst., 3 (2015), 65–82. |
[8] | J. S. Golan, Semirings and their applications, Dordrecht: Kluwer Academic Publishers, 1999. |
[9] | Y. Q. Gno, F. Pastijn, Semirings which are unions of rings, Sci. China (Ser. A), 45 (2002), 172–195. |
[10] | M. Haristchain, Formex and plenix structural analysis, PhD thesis, University of Surrey, 1980. |
[11] | U. Hebisch, H. J. Weinert, Semirings: Algebraic theory and applications in computer science, Singapore: World Scientific, 1998. |
[12] | H. Hedayati, A. Asadi, Normal, maximal and product fuzzy subnexuses of nexuses, J. Intell. Fuzzy Syst., 26 (2014), 1341–1348. |
[13] | I. Hee, Plenix structural analysis, PhD thesis, University of Surrey, 1985. |
[14] | R. Kamrani, A. Hasankhani, M. Bolourian, Finitely Generated Submoduloids and Prime Submoduloids on a nexus, Mathematics, 32 (2020), 88–109. |
[15] | R. Kamrani, A. Hasankhani, M. Bolourian, On Submoduloids of a Moduloid on a nexus, Appl. Appl. Math., 15 (2020), 1407–1435. |
[16] | W. J. Liu, Fuzzy invariant subgroups and fuzzy ideals, J. Fuzzy Sets Syst., 8 (1982), 133–139. |
[17] |
P. Nasehpour, Some remarks on semiring and their ideals, Asian-Eur. J. Math., 12 (2019), 2050002. https://doi.org/10.1142/S1793557120500023 % doi: 10.1142/S1793557120500023
![]() |
[18] |
H. Nooshin, Algebraic representation and processing of structural configurations, Comput. Struct., 5 (1975), 119–130. https://doi.org/10.1016/0045-7949(75)90002-4 doi: 10.1016/0045-7949(75)90002-4
![]() |
[19] | H. Nooshin, Formex configuration processing in structural engineering, London: Elsevier Applied Science, 1984. |
[20] | M. Norouzi, A. Asadi, Y. B. Jun, A generalization of subnexuses based on N-structures, Thai J. Math., 18 (2020), 563–575. |
[21] |
A. Razaq, G. Alhamzi, On Pythagorean fuzzy ideals of a classical ring, AIMS Mathematics, 8 (2023), 4280–4303. https://doi.org/10.3934/math.2023213 doi: 10.3934/math.2023213
![]() |
[22] |
A. Razaq, I. M. Ahmad, M. A. Yousaf, S. Masood, A novel finite rings based algebraic scheme of evolving secure S-boxes for images encryption, Multimed. Tools Appl., 80 (2021), 20191–20215. https://doi.org/10.1007/s11042-021-10587-8 doi: 10.1007/s11042-021-10587-8
![]() |
[23] |
A. Razzaque, A. Razaq, G. Alhamzi, H. Garg, M. I. Faraz, A Detailed Study of Mathematical Rings in q-Rung Orthopair Fuzzy Framework, Symmetry, 15 (2023), 697. https://doi.org/10.3390/sym15030697 doi: 10.3390/sym15030697
![]() |
[24] | A. Rosenfeld, Fuzzy groups, J. Math. Anal. Appl., 35 (1971), 512–517. |
[25] | A. Saeidi Rashkolia, A. Hasankhani, Fuzzy subnexuses, Ital. J. Pure Appl. Math., 28 (2011), 229–242. |
[26] | M. K. Sen, S. K. Maity, K. P. Shum, Some aspects of semirings, J. Math., 45 (2021), 919–930. |
[27] | N. Sulochana, M. Amala, T. Vasanthi, A study on the classes of semiring and ordered semiring, Adv. Algebr., 9 (2016), 11–15. |
[28] | U. M. Swamy, K. L. N. Swamy, Fuzzy prime ideals of rings, J. Math. Anal. Appl., 134 (1988), 94–103. |
[29] | L. A. Zadeh, Fuzzy sets, Inf. Control, 8 (1965), 338–353. |
1. | Jamilu Abubakar Jiddah, Mohammed Shehu Shagari, Graphical approach to the study of fixed point results involving hybrid contractions, 2024, 14, 26667207, 100394, 10.1016/j.rico.2024.100394 | |
2. | Mohammed Shehu Shagari, Rosemary O. Ogbumba, Maha Noorwali, Trad Alotaibi, Afis Saliu, Fixed Point Results of FF‐Contractive–Type Operators With Applications, 2025, 0170-4214, 10.1002/mma.10735 |
LINEX | ||||||
(n, m) | CS | MLE | SEL | c = - 2 | c = 0.0001 | c = 2 |
(30, 20) | Ⅰ | 0.3853 | 0.3843 | 0.3843 | 0.3842 | 0.3843 |
(0.0727) | (0.0739) | (0.0739) | (0.0738) | (0.0737) | ||
Ⅱ | 0.4046 | 0.4036 | 0.4037 | 0.4036 | 0.4036 | |
(0.063) | (0.0626) | (0.0626) | (0.0625) | (0.0624) | ||
Ⅲ | 0.4238 | 0.4237 | 0.4236 | 0.4237 | 0.4236 | |
(0.641) | (0.064) | (0.064) | (0.063) | (0.0639) | ||
(40, 15) | Ⅰ | 0.3794 | 0.3793 | 0.3794 | 0.3793 | 0.3792 |
(0.0783) | (0.0789) | (0.079) | (0.0785) | (0.0784) | ||
Ⅱ | 0.4256 | 0.4264 | 0.4265 | 0.4264 | 0.4263 | |
(0.0768) | (0.767) | (0.0767) | (0.0767) | (0.766) | ||
Ⅲ | 0.4564 | 0.4567 | 0.4569 | 0.4567 | 0.4565 | |
(0.071) | (0.0719) | (0.72) | (0.0716) | (0.714) | ||
(40, 20) | Ⅰ | 0.3784 | 0.3781 | 0.3781 | 0.3780 | 0.3779 |
(0.0689) | (0.0686) | (0.0689) | (0.0685) | (0.0684) | ||
Ⅱ | 0.3988 | 0.3989 | 0.399 | 0.43988 | 0.3987 | |
(0.0636) | (0.0639) | (0.0639) | (0.0631) | (0.0630) | ||
Ⅲ | 0.4364 | 0.4363 | 0.4364 | 0.4363 | 0.4362 | |
(0.0608) | (0.0613) | (0.0613) | (0.0611) | (0.0610) | ||
(50, 15) | Ⅰ | 0.3817 | 0.3815 | 0.3814 | 0.3810 | 0.3809 |
(0.0759) | (0.0748) | (0.0759) | (0.0749) | (0.0741) | ||
Ⅱ | 0.4338 | 0.4344 | 0.4346 | 0.4344 | 0.4342 | |
(0.081) | (0.0813) | (0.0814) | (0.0810) | (0.0807) | ||
Ⅲ | 0.4499 | 0.4496 | 0.4497 | 0.4496 | 0.4495 | |
(0.0683) | (0.0682) | (0.0683) | (0.0680) | (0.0677) | ||
(60, 15) | Ⅰ | 0.406 | 0.4058 | 0.406 | 0.4058 | 0.4057 |
(0.0883) | (0.0889) | (0.0889) | (0.0884) | (0.0880) | ||
Ⅱ | 0.4198 | 0.4197 | 0.4197 | 0.4196 | 0.4195 | |
(0.0783) | (0.0785) | (0.0786) | (0.0784) | (0.0779) | ||
Ⅲ | 0.4474 | 0.448 | 0.4482 | 0.4481 | 0.4477 | |
(0.0673) | (0.0684) | (0.0686) | (0.0683) | (0.0680) | ||
(60, 20) | Ⅰ | 0.407 | 0.4059 | 0.407 | 0.4059 | 0.4058 |
(0.0884) | (0.0890) | (0.0890) | (0.0885) | (0.0881) | ||
Ⅱ | 0.4199 | 0.4198 | 0.4198 | 0.4197 | 0.4196 | |
(0.0784) | (0.0784) | (0.0787) | (0.0785) | (0.0780) | ||
Ⅲ | 0.4475 | 0.449 | 0.4483 | 0.4482 | 0.4478 | |
(0.0675) | (0.0686) | (0.0687) | (0.0684) | (0.0683) |
LINEX | ||||||
(n, m) | CS | MLE | SEL | c = - 2 | c = 0.0001 | c = 2 |
(30, 20) | Ⅰ | 0.0633 | 0.0632 | 0.0632 | 0.0631 | 0.0630 |
(0.0008) | (0.0006) | (0.0008) | (0.0005) | (0.0004) | ||
Ⅱ | 0.0614 | 0.0613 | 0.0614 | 0.0612 | 0.0611 | |
(0.0006) | (0.0005) | (0.0005) | (0.0004) | (0.0003) | ||
Ⅲ | 0.06 | 0.06 | 0.063 | 0.062 | 0.060 | |
(0.0005) | (0.0004) | (0.0004) | (0.0003) | (0.0002) | ||
(40, 15) | Ⅰ | 0.0634 | 0.0633 | 0.0634 | 0.0633 | 0.0631 |
(0.0007) | (0.0006) | (0.0006) | (0.0005) | (0.0004) | ||
Ⅱ | 0.0608 | 0.0607 | 0.0607 | 0.0606 | 0.0605 | |
(0.0006) | (0.0005) | (0.0005) | (0.0004) | (0.0003) | ||
Ⅲ | 0.0582 | 0.0581 | 0.0581 | 0.0580 | 0.0579 | |
(0.0005) | (0.0004) | (0.0004) | (0.0003) | (0.0002) | ||
(40, 20) | Ⅰ | 0.0632 | 0.0628 | 0.0628 | 0.0627 | 0.0625 |
(0.0006) | (0.0005) | (0.0005) | (0.0004) | (0.0003) | ||
Ⅱ | 0.0617 | 0.0614 | 0.0614 | 0.0613 | 0.0612 | |
(0.0005) | (0.0004) | (0.0004) | (0.0003) | (0.0002) | ||
Ⅲ | 0.0586 | 0.0584 | 0.0584 | 0.0583 | 0.0582 | |
(0.0004) | (0.0003) | (0.0003) | (0.0002) | (0.0001) | ||
(50, 15) | Ⅰ | 0.063 | 0.062 | 0.062 | 0.061 | 0.060 |
(0.0006) | (0.0005) | (0.0005) | (0.0004) | (0.0003) | ||
Ⅱ | 0.0598 | 0.0597 | 0.0597 | 0.40596 | 0.0595 | |
(0.0006) | (0.0005) | (0.0005) | (0.0004) | (0.0003) | ||
Ⅲ | 0.0577 | 0.0577 | 0.0577 | 0.0576 | 0.0574 | |
(0.0004) | (0.0003) | (0.0003) | (0.0002) | (0.0001) | ||
(60, 15) | Ⅰ | 0.0608 | 0.0607 | 0.0607 | 0.0606 | 0.0675 |
(0.0004) | (0.0003) | (0.0003) | (0.0002) | (0.0001) | ||
Ⅱ | 0.0608 | 0.0607 | 0.0607 | 0.0606 | 0.40605 | |
(0.0005) | (0.0004) | (0.0004) | (0.0003) | (0.0002) | ||
Ⅲ | 0.0578 | 0.40579 | 0.0579 | 0.0578 | 0.0577 | |
(0.0005) | (0.0004) | (0.0004) | (0.0003) | (0.0002) | ||
(60, 20) | Ⅰ | 0.0609 | 0.0608 | 0.0608 | 0.0607 | 0.06076 |
(0.0005) | (0.0004) | (0.0004) | (0.0003) | (0.0002) | ||
Ⅱ | 0.0609 | 0.40608 | 0.40608 | 0.0607 | 0.0606 | |
(0.0006) | (0.0005) | (0.0005) | (0.0004) | (0.0003) | ||
Ⅲ | 0.0579 | 0.0580 | 0.0580 | 0.0579 | 0.0578 | |
(0.0006) | (0.0005) | (0.0005) | (0.0004) | (0.0003) |
LINEX | ||||||
(n, m) | CS | MLE | SEL | c = - 2 | c = 0.0001 | c = 2 |
(30, 20) | Ⅰ | 0.0457 | 0.0455 | 0.0455 | 0.0454 | 0.0453 |
(0.0003) | (0.0004) | (0.0004) | (0.0003) | (0.0002) | ||
Ⅱ | 0.0475 | 0.0474 | 0.0474 | 0.0472 | 0.0471 | |
(0.0004) | (0.0003) | (0.0003) | (0.0002) | (0.0001) | ||
Ⅲ | 0.0489 | 0.0488 | 0.0488 | 0.0487 | 0.0486 | |
(0.0004) | (0.0003) | (0.0003) | (0.0002) | (0.0001) | ||
(40, 15) | Ⅰ | 0.0449 | 0.0448 | 0.0448 | 0.0447 | 0.0446 |
(0.005) | (0.004) | (0.004) | (0.003) | (0.002) | ||
Ⅱ | 0.0479 | 0.0478 | 0.0478 | 0.0477 | 0.0476 | |
(0.0005) | (0.0004) | (0.0004) | (0.0003) | (0.0001) | ||
Ⅲ | 0.0505 | 0.0504 | 0.0504 | 0.0503 | 0.0502 | |
(0.0005) | (0.0004) | (0.0004) | (0.0003) | (0.0001) | ||
(40, 20) | Ⅰ | 0.0455 | 0.0454 | 0.0454 | 0.0453 | 0.0452 |
(0.0004) | (0.0003) | (0.0003) | (0.0002) | (0.0001) | ||
Ⅱ | 0.048 | 0.047 | 0.047 | 0.046 | 0.045 | |
(0.0004) | (0.0003) | (0.0003) | (0.0002) | (0.0001) | ||
Ⅲ | 0.0495 | 0.0494 | 0.0494 | 0.0493 | 0.0492 | |
(0.0004) | (0.0003) | (0.0003) | (0.0002) | (0.0001) | ||
(50, 15) | Ⅰ | 0.0446 | 0.0445 | 0.0445 | 0.0444 | 0.0443 |
(0.0004) | (0.0004) | (0.0004) | (0.0004) | (0.0004) | ||
Ⅱ | 0.048 | 0.0479 | 0.0479 | 0.0478 | 0.0477 | |
(0.0006) | (0.0005) | (0.0005) | (0.0004) | (0.0002) | ||
Ⅲ | 0.0499 | 0.0498 | 0.0498 | 0.0497 | 0.0495 | |
(0.0006) | (0.0004) | (0.0004) | (0.0002) | (0.0001) | ||
(60, 15) | Ⅰ | 0.0457 | 0.0456 | 0.0456 | 0.0455 | 0.0453 |
(0.0006) | (0.0006) | (0.0004) | (0.0003) | (0.0002) | ||
Ⅱ | 0.0473 | 0.0472 | 0.0472 | 0.0471 | 0.0470 | |
(0.0007) | (0.0006) | (0.0006) | (0.0004) | (0.0003) | ||
Ⅲ | 0.0497 | 0.0496 | 0.0496 | 0.0495 | 0.0494 | |
(0.0004) | (0.0003) | (0.0003) | (0.0002) | (0.0001) | ||
(60, 20) | Ⅰ | 0.0458 | 0.0457 | 0.0457 | 0.0456 | 0.0454 |
(0.0007) | (0.0006) | (0.0006) | (0.0004) | (0.0002) | ||
Ⅱ | 0.0474 | 0.0473 | 0.0473 | 0.0472 | 0.0471 | |
(0.0008) | (0.0007) | (0.0007) | (0.0005) | (0.0004) | ||
Ⅲ | 0.0498 | 0.0497 | 0.0497 | 0.0496 | 0.0495 | |
(0.0005) | (0.0004) | (0.0004) | (0.0003) | (0.0002) |
LINEX | ||||||
(n, m) | CS | MLE | SEL | c = - 2 | c = 0.0001 | c = 2 |
(30, 20) | Ⅰ | 1.1289 | 1.1288 | 1.1288 | 1.1287 | 1.1286 |
(0.0019) | (0.0016) | (0.0016) | (0.0014) | (0.0012) | ||
Ⅱ | 1.1253 | 1.1252 | 1.1252 | 1.1251 | 1.1250 | |
(0.0015) | (0.0013) | (0.0013) | (0.0012) | (0.0011) | ||
Ⅲ | 1.1229 | 1.1229 | 1.1229 | 1.1227 | 1.1226 | |
(0.0013) | (0.0012) | (0.0012) | (0.0011) | (0.0010) | ||
(40, 15) | Ⅰ | 1.1295 | 1.1294 | 1.1294 | 1.1293 | 1.1292 |
(0.0017) | (0.0016) | (0.0016) | (0.0014) | (0.0013) | ||
Ⅱ | 1.1242 | 1.1241 | 1.1241 | 1.1240 | 1.1239 | |
(0.0015) | (0.0013) | (0.0013) | (0.0011) | (0.0010) | ||
Ⅲ | 1.1193 | 1.1192 | 1.1192 | 1.1191 | 1.1190 | |
(0.0011) | (0.0009) | (0.0009) | (0.0008) | (0.0007) | ||
(40, 20) | Ⅰ | 1.1283 | 1.1282 | 1.1282 | 1.1281 | 1.1280 |
(0.0015) | (0.0014) | (0.0014) | (0.0013) | (0.0011) | ||
Ⅱ | 1.1256 | 1.1255 | 1.1255 | 1.1254 | 1.1252 | |
(0.0013) | (0.0012) | (0.0012) | (0.0011) | (0.0010) | ||
Ⅲ | 1.1203 | 1.1202 | 1.1202 | 1.1201 | 1.1200 | |
(0.004) | (0.003) | (0.003) | (0.002) | (0.001) | ||
(50, 15) | Ⅰ | 1.1279 | 1.1278 | 1.1278 | 1.1277 | 1.1276 |
(0.0016) | (0.0015) | (0.0015) | (0.0014) | (0.0012) | ||
Ⅱ | 1.1229 | 1.1228 | 1.1228 | 1.1227 | 1.1226 | |
(0.0019) | (0.0017) | (0.0017) | (0.0016) | (0.0014) | ||
Ⅲ | 1.1192 | 1.1191 | 1.1191 | 1.1190 | 1.1189 | |
(0.0015) | (0.0014) | (0.0014) | (0.0013) | (0.0011) | ||
(60, 15) | Ⅰ | 1.1256 | 1.1255 | 1.1255 | 1.1254 | 1.1253 |
(0.0015) | (0.0014) | (0.0014) | (0.0013) | (0.0012) | ||
Ⅱ | 1.1247 | 1.1246 | 1.1246 | 1.1245 | 1.1244 | |
(0.0016) | (0.0015) | (0.0015) | (0.0014) | (0.0012) | ||
Ⅲ | 1.1195 | 1.1194 | 1.1194 | 1.1193 | 1.1192 | |
(0.0016) | (0.0015) | (0.0015) | (0.0013) | (0.0011) | ||
(60, 20) | Ⅰ | 1.1257 | 1.1256 | 1.1256 | 1.1255 | 1.1254 |
(0.0016) | (0.0015) | (0.0015) | (0.0014) | (0.0013) | ||
Ⅱ | 1.1248 | 1.1247 | 1.1247 | 1.1246 | 1.1245 | |
(0.0017) | (0.0016) | (0.0016) | (0.0015) | (0.0013) | ||
Ⅲ | 1.1196 | 1.1195 | 1.1195 | 1.1194 | 1.1193 | |
(0.0017) | (0.0016) | (0.0016) | (0.0015) | (0.0012) |
α | β | ||||
(n, m) | CS | MLE | MCMC | MLE | MCMC |
(30, 20) | Ⅰ | 1.1444 | 0.0234 | 0.0751 | 0.0016 |
(0.9435) | (0.9446) | (0.9332) | (0.9418) | ||
Ⅱ | 1.1063 | 0.0236 | 0.0714 | 0.0015 | |
(0.9252) | (0.9367) | (0.9405) | (0.942) | ||
Ⅲ | 1.1302 | 0.0243 | 0.0688 | 0.0015 | |
(0.9572) | (0.9253) | (0.9396) | (0.9549) | ||
(40, 15) | Ⅰ | 1.2277 | 0.0268 | 0.0781 | 0.0017 |
(0.9698) | (0.9507) | (0.9377) | (0.9456) | ||
Ⅱ | 1.3317 | 0.0283 | 0.078 | 0.0016 | |
(0.9625) | (0.947) | (0.9608) | (0.9655) | ||
Ⅲ | 1.301 | 0.0281 | 0.0716 | 0.0015 | |
(0.951) | (0.9606) | (0.9712) | (0.9447) | ||
(40, 20) | Ⅰ | 1.0855 | 0.0231 | 0.0707 | 0.0015 |
(0.9615) | (0.9566) | (0.9573) | (0.9539) | ||
Ⅱ | 1.0899 | 0.0232 | 0.0698 | 0.0015 | |
(0.9256) | (0.947) | (0.9408) | (0.959) | ||
Ⅲ | 1.077 | 0.0227 | 0.0632 | 0.0013 | |
(0.9468) | (0.9568) | (0.9461) | (0.9616) | ||
(50, 15) | Ⅰ | 1.2419 | 0.0262 | 0.0754 | 0.0016 |
(0.9325) | (0.9845) | (0.9781) | (0.9863) | ||
Ⅱ | 1.3984 | 0.0293 | 0.0776 | 0.0016 | |
(0.9124) | (0.9683) | (0.9786) | (0.9452) | ||
Ⅲ | 1.2576 | 0.0274 | 0.0694 | 0.0015 | |
(0.9568) | (0.9565) | (0.9468) | (0.9478) | ||
(60, 15) | Ⅰ | 1.2813 | 0.0269 | 0.0719 | 0.0015 |
(0.9461) | (0.9616) | (0.9256) | (0.947) | ||
Ⅱ | 1.2959 | 0.0278 | 0.075 | 0.0016 | |
(0.9605) | (0.9641) | (0.9468) | (0.9568) | ||
Ⅲ | 1.2552 | 0.0275 | 0.0686 | 0.0015 | |
(0.9416) | (0.9486) | (0.9568) | (0.9665) | ||
(60, 20) | Ⅰ | 1.2832 | 0.0270 | 0.0720 | 0.0016 |
(0.9462) | (0.9617) | (0.9257) | (0.948) | ||
Ⅱ | 1.2960 | 0.0279 | 0.076 | 0.0017 | |
(0.9606) | (0.9642) | (0.94670) | (0.9569) | ||
Ⅲ | 1.2553 | 0.0276 | 0.0687 | 0.0016 | |
(0.9417) | (0.9487) | (0.9569) | (0.9666) |
S(t) | h(t) | ||||
(n, m) | CS | MLE | MCMC | MLE | MCMC |
(30, 20) | Ⅰ | 0.0755 | 0.0029 | 1.2694 | 0.0022 |
(0.9511) | (0.9489) | (0.952) | (0.9472) | ||
Ⅱ | 0.10705 | 0.003 | 1.2627 | 0.0021 | |
(0.9626) | (0.9546) | (0.9539) | (0.9278) | ||
Ⅲ | 0.0687 | 0.003 | 1.2572 | 0.0021 | |
(0.9576) | (0.9661) | (0.9361) | (0.9429) | ||
(40, 15) | Ⅰ | 0.0836 | 0.0033 | 1.2711 | 0.0024 |
(0.9421) | (0.9507) | (0.927) | (0.9296) | ||
Ⅱ | 0.081 | 0.0034 | 1.2593 | 0.0024 | |
(0.9325) | (0.9458) | (0.9736) | (0.9856) | ||
Ⅲ | 0.0748 | 0.0033 | 1.2469 | 0.0022 | |
(0.958) | (0.9603) | (0.9601) | (0.9602) | ||
(40, 20) | Ⅰ | 0.0739 | 0.0029 | 1.2693 | 0.0022 |
(0.9462) | (0.9467) | (0.9502) | (0.9506) | ||
Ⅱ | 0.07 | 0.0029 | 1.2633 | 0.0021 | |
(0.9256) | (0.9592) | (0.9704) | (0.9694) | ||
Ⅲ | 0.0645 | 0.00327 | 1.252 | 0.0019 | |
(0.9544) | (0.9581) | (0.9382) | (0.9454) | ||
(50, 15) | Ⅰ | 0.0839 | 0.0032 | 1.2671 | 0.0024 |
(0.9814) | (0.9145) | (0.9745) | (0.9635) | ||
Ⅱ | 0.0832 | 0.0035 | 1.2541 | 0.0023 | |
(0.9748) | (0.9862) | (0.9742) | (0.9681) | ||
Ⅲ | 0.0735 | 0.0032 | 1.2483 | 0.0022 | |
(0.9254) | (0.9438) | (0.9152) | (0.9634) | ||
(60, 15) | Ⅰ | 0.0835 | 0.0032 | 1.2625 | 0.0023 |
(0.9637) | (0.9504) | (0.9667) | (0.9567) | ||
Ⅱ | 0.0803 | 0.0033 | 1.2605 | 0.0024 | |
(0.9617) | (0.9325) | (0.9486) | (0.9479) | ||
Ⅲ | 0.0735 | 0.0032 | 1.2489 | 0.0021 | |
(0.9672) | (0.9488) | (0.9457) | (0.9639) | ||
(60, 20) | Ⅰ | 0.0836 | 0.0033 | 1.2626 | 0.0024 |
(0.9638) | (0.9505) | (0.9668) | (0.9568) | ||
Ⅱ | 0.0804 | 0.0034 | 1.2606 | 0.0025 | |
(0.9618) | (0.9326) | (0.9487) | (0.9480) | ||
Ⅲ | 0.0736 | 0.0033 | 1.2490 | 0.0022 | |
(0.9673) | (0.9489) | (0.9458) | (0.940) |
1.1 | 1.4 | 1.3 | 1.7 | 1.9 | 1.8 | 1.6 | 2.2 | 1.7 | 2.7 |
1.8 | 1.5 | 1.2 | 1.4 | 3 | 1.7 | 2.3 | 1.6 | 2 | 4.1 |
Model | α | β | θ | λ |
MFED | 32.433 | - | 2.0038 | - |
EE | 36.68 | - | 2.235 | |
RTFIW | 6.7199 | 3.7354 | - | - |
Model | K–S | p-Value | -logL | AIC | BIC | HQIC |
MFED | 0.120612 | 0.899975 | 15.9512 | 35.9024 | 37.8938 | 36.2911 |
EE | 0.1343 | 0.817352 | 16.2606 | 36.5212 | 38.5125 | 36.91 |
RTFIW | 0.100217 | 0.955062 | 14.9239 | 33.8478 | 35.8392 | 35.6581 |
Baysian | |||||
Parameters | MLE | SLE | LINEX | ||
c = - 2 | c = 0.0001 | c = 2 | |||
α | 1.16548 | 1.15804 | 1.15869 | 1.15804 | 1.15739 |
β | 2.85092 | 2.78356 | 2.796 | 2.78356 | 2.76981 |
S | 0.999772 | 0.99954 | 0.999541 | 0.99954 | 0.99954 |
h | 0.0109181 | 0.0184367 | 0.0185916 | 0.0184367 | 0.0182849 |
Parameter | MLE | MCMC |
α | (0.337871, 1.99309) | (1.11849, 1.2089) |
β | (0.8467, 4.85513) | (2.55267, 2.95076) |
S(0.4) | (0.996234, 1.00331) | (0.998601, 0.999898) |
h(0.4) | (−0.131477, 0.153313) | (0.00553594, 0.0472466) |
Model | α | β | θ | λ |
GIW | 0.792 | 0.298 | 3.261 | |
EGIW | .933 | 1.09 | 0.453 | 1.102 |
RTFIWD | 1.10716 | 1.37948 |
Model | K−S | P−Value | -2logL | AIC | BIC | CAIC |
GIW | 0.737 | 0.7584 | 795.582 | 801.582 | 806.648 | 802.248 |
EGIW | 0.831 | .82464 | 709.471 | 717.471 | 724.226 | 718.614 |
RTFIWD | 1.001 | 0.9744 | 594.72585 | 598.7258 | 602.1036 | 598.325 |
Parameters | MLE | Baysian | |||
SEL | LINEX | ||||
c=−2 | c=0.0001 | c=2 | |||
α | 1.10716 | 1.11602 | 1.11604 | 1.11601 | 1.11602 |
β | 1.37948 | 1.3736 | 1.37364 | 1.37357 | 1.3736 |
S | 0.94189 | 0.942492 | 0.942492 | 0.942491 | 0.942492 |
h | 0.490308 | 0.484726 | 0.484731 | 0.48472 | 0.484726 |
Parameter | MLE | MCMC |
α | (0.545939,1.66837) | (1.1084,1.12323) |
β | (0.701971,2.057) | (1.36354,1.38614) |
S(0.4) | (0.821262,1.06252) | (0.941573,0.94353) |
h(0.4) | (−0.0904752,1.07109) | (0.478703,0.489044) |
LINEX | ||||||
(n, m) | CS | MLE | SEL | c = - 2 | c = 0.0001 | c = 2 |
(30, 20) | Ⅰ | 0.3853 | 0.3843 | 0.3843 | 0.3842 | 0.3843 |
(0.0727) | (0.0739) | (0.0739) | (0.0738) | (0.0737) | ||
Ⅱ | 0.4046 | 0.4036 | 0.4037 | 0.4036 | 0.4036 | |
(0.063) | (0.0626) | (0.0626) | (0.0625) | (0.0624) | ||
Ⅲ | 0.4238 | 0.4237 | 0.4236 | 0.4237 | 0.4236 | |
(0.641) | (0.064) | (0.064) | (0.063) | (0.0639) | ||
(40, 15) | Ⅰ | 0.3794 | 0.3793 | 0.3794 | 0.3793 | 0.3792 |
(0.0783) | (0.0789) | (0.079) | (0.0785) | (0.0784) | ||
Ⅱ | 0.4256 | 0.4264 | 0.4265 | 0.4264 | 0.4263 | |
(0.0768) | (0.767) | (0.0767) | (0.0767) | (0.766) | ||
Ⅲ | 0.4564 | 0.4567 | 0.4569 | 0.4567 | 0.4565 | |
(0.071) | (0.0719) | (0.72) | (0.0716) | (0.714) | ||
(40, 20) | Ⅰ | 0.3784 | 0.3781 | 0.3781 | 0.3780 | 0.3779 |
(0.0689) | (0.0686) | (0.0689) | (0.0685) | (0.0684) | ||
Ⅱ | 0.3988 | 0.3989 | 0.399 | 0.43988 | 0.3987 | |
(0.0636) | (0.0639) | (0.0639) | (0.0631) | (0.0630) | ||
Ⅲ | 0.4364 | 0.4363 | 0.4364 | 0.4363 | 0.4362 | |
(0.0608) | (0.0613) | (0.0613) | (0.0611) | (0.0610) | ||
(50, 15) | Ⅰ | 0.3817 | 0.3815 | 0.3814 | 0.3810 | 0.3809 |
(0.0759) | (0.0748) | (0.0759) | (0.0749) | (0.0741) | ||
Ⅱ | 0.4338 | 0.4344 | 0.4346 | 0.4344 | 0.4342 | |
(0.081) | (0.0813) | (0.0814) | (0.0810) | (0.0807) | ||
Ⅲ | 0.4499 | 0.4496 | 0.4497 | 0.4496 | 0.4495 | |
(0.0683) | (0.0682) | (0.0683) | (0.0680) | (0.0677) | ||
(60, 15) | Ⅰ | 0.406 | 0.4058 | 0.406 | 0.4058 | 0.4057 |
(0.0883) | (0.0889) | (0.0889) | (0.0884) | (0.0880) | ||
Ⅱ | 0.4198 | 0.4197 | 0.4197 | 0.4196 | 0.4195 | |
(0.0783) | (0.0785) | (0.0786) | (0.0784) | (0.0779) | ||
Ⅲ | 0.4474 | 0.448 | 0.4482 | 0.4481 | 0.4477 | |
(0.0673) | (0.0684) | (0.0686) | (0.0683) | (0.0680) | ||
(60, 20) | Ⅰ | 0.407 | 0.4059 | 0.407 | 0.4059 | 0.4058 |
(0.0884) | (0.0890) | (0.0890) | (0.0885) | (0.0881) | ||
Ⅱ | 0.4199 | 0.4198 | 0.4198 | 0.4197 | 0.4196 | |
(0.0784) | (0.0784) | (0.0787) | (0.0785) | (0.0780) | ||
Ⅲ | 0.4475 | 0.449 | 0.4483 | 0.4482 | 0.4478 | |
(0.0675) | (0.0686) | (0.0687) | (0.0684) | (0.0683) |
LINEX | ||||||
(n, m) | CS | MLE | SEL | c = - 2 | c = 0.0001 | c = 2 |
(30, 20) | Ⅰ | 0.0633 | 0.0632 | 0.0632 | 0.0631 | 0.0630 |
(0.0008) | (0.0006) | (0.0008) | (0.0005) | (0.0004) | ||
Ⅱ | 0.0614 | 0.0613 | 0.0614 | 0.0612 | 0.0611 | |
(0.0006) | (0.0005) | (0.0005) | (0.0004) | (0.0003) | ||
Ⅲ | 0.06 | 0.06 | 0.063 | 0.062 | 0.060 | |
(0.0005) | (0.0004) | (0.0004) | (0.0003) | (0.0002) | ||
(40, 15) | Ⅰ | 0.0634 | 0.0633 | 0.0634 | 0.0633 | 0.0631 |
(0.0007) | (0.0006) | (0.0006) | (0.0005) | (0.0004) | ||
Ⅱ | 0.0608 | 0.0607 | 0.0607 | 0.0606 | 0.0605 | |
(0.0006) | (0.0005) | (0.0005) | (0.0004) | (0.0003) | ||
Ⅲ | 0.0582 | 0.0581 | 0.0581 | 0.0580 | 0.0579 | |
(0.0005) | (0.0004) | (0.0004) | (0.0003) | (0.0002) | ||
(40, 20) | Ⅰ | 0.0632 | 0.0628 | 0.0628 | 0.0627 | 0.0625 |
(0.0006) | (0.0005) | (0.0005) | (0.0004) | (0.0003) | ||
Ⅱ | 0.0617 | 0.0614 | 0.0614 | 0.0613 | 0.0612 | |
(0.0005) | (0.0004) | (0.0004) | (0.0003) | (0.0002) | ||
Ⅲ | 0.0586 | 0.0584 | 0.0584 | 0.0583 | 0.0582 | |
(0.0004) | (0.0003) | (0.0003) | (0.0002) | (0.0001) | ||
(50, 15) | Ⅰ | 0.063 | 0.062 | 0.062 | 0.061 | 0.060 |
(0.0006) | (0.0005) | (0.0005) | (0.0004) | (0.0003) | ||
Ⅱ | 0.0598 | 0.0597 | 0.0597 | 0.40596 | 0.0595 | |
(0.0006) | (0.0005) | (0.0005) | (0.0004) | (0.0003) | ||
Ⅲ | 0.0577 | 0.0577 | 0.0577 | 0.0576 | 0.0574 | |
(0.0004) | (0.0003) | (0.0003) | (0.0002) | (0.0001) | ||
(60, 15) | Ⅰ | 0.0608 | 0.0607 | 0.0607 | 0.0606 | 0.0675 |
(0.0004) | (0.0003) | (0.0003) | (0.0002) | (0.0001) | ||
Ⅱ | 0.0608 | 0.0607 | 0.0607 | 0.0606 | 0.40605 | |
(0.0005) | (0.0004) | (0.0004) | (0.0003) | (0.0002) | ||
Ⅲ | 0.0578 | 0.40579 | 0.0579 | 0.0578 | 0.0577 | |
(0.0005) | (0.0004) | (0.0004) | (0.0003) | (0.0002) | ||
(60, 20) | Ⅰ | 0.0609 | 0.0608 | 0.0608 | 0.0607 | 0.06076 |
(0.0005) | (0.0004) | (0.0004) | (0.0003) | (0.0002) | ||
Ⅱ | 0.0609 | 0.40608 | 0.40608 | 0.0607 | 0.0606 | |
(0.0006) | (0.0005) | (0.0005) | (0.0004) | (0.0003) | ||
Ⅲ | 0.0579 | 0.0580 | 0.0580 | 0.0579 | 0.0578 | |
(0.0006) | (0.0005) | (0.0005) | (0.0004) | (0.0003) |
LINEX | ||||||
(n, m) | CS | MLE | SEL | c = - 2 | c = 0.0001 | c = 2 |
(30, 20) | Ⅰ | 0.0457 | 0.0455 | 0.0455 | 0.0454 | 0.0453 |
(0.0003) | (0.0004) | (0.0004) | (0.0003) | (0.0002) | ||
Ⅱ | 0.0475 | 0.0474 | 0.0474 | 0.0472 | 0.0471 | |
(0.0004) | (0.0003) | (0.0003) | (0.0002) | (0.0001) | ||
Ⅲ | 0.0489 | 0.0488 | 0.0488 | 0.0487 | 0.0486 | |
(0.0004) | (0.0003) | (0.0003) | (0.0002) | (0.0001) | ||
(40, 15) | Ⅰ | 0.0449 | 0.0448 | 0.0448 | 0.0447 | 0.0446 |
(0.005) | (0.004) | (0.004) | (0.003) | (0.002) | ||
Ⅱ | 0.0479 | 0.0478 | 0.0478 | 0.0477 | 0.0476 | |
(0.0005) | (0.0004) | (0.0004) | (0.0003) | (0.0001) | ||
Ⅲ | 0.0505 | 0.0504 | 0.0504 | 0.0503 | 0.0502 | |
(0.0005) | (0.0004) | (0.0004) | (0.0003) | (0.0001) | ||
(40, 20) | Ⅰ | 0.0455 | 0.0454 | 0.0454 | 0.0453 | 0.0452 |
(0.0004) | (0.0003) | (0.0003) | (0.0002) | (0.0001) | ||
Ⅱ | 0.048 | 0.047 | 0.047 | 0.046 | 0.045 | |
(0.0004) | (0.0003) | (0.0003) | (0.0002) | (0.0001) | ||
Ⅲ | 0.0495 | 0.0494 | 0.0494 | 0.0493 | 0.0492 | |
(0.0004) | (0.0003) | (0.0003) | (0.0002) | (0.0001) | ||
(50, 15) | Ⅰ | 0.0446 | 0.0445 | 0.0445 | 0.0444 | 0.0443 |
(0.0004) | (0.0004) | (0.0004) | (0.0004) | (0.0004) | ||
Ⅱ | 0.048 | 0.0479 | 0.0479 | 0.0478 | 0.0477 | |
(0.0006) | (0.0005) | (0.0005) | (0.0004) | (0.0002) | ||
Ⅲ | 0.0499 | 0.0498 | 0.0498 | 0.0497 | 0.0495 | |
(0.0006) | (0.0004) | (0.0004) | (0.0002) | (0.0001) | ||
(60, 15) | Ⅰ | 0.0457 | 0.0456 | 0.0456 | 0.0455 | 0.0453 |
(0.0006) | (0.0006) | (0.0004) | (0.0003) | (0.0002) | ||
Ⅱ | 0.0473 | 0.0472 | 0.0472 | 0.0471 | 0.0470 | |
(0.0007) | (0.0006) | (0.0006) | (0.0004) | (0.0003) | ||
Ⅲ | 0.0497 | 0.0496 | 0.0496 | 0.0495 | 0.0494 | |
(0.0004) | (0.0003) | (0.0003) | (0.0002) | (0.0001) | ||
(60, 20) | Ⅰ | 0.0458 | 0.0457 | 0.0457 | 0.0456 | 0.0454 |
(0.0007) | (0.0006) | (0.0006) | (0.0004) | (0.0002) | ||
Ⅱ | 0.0474 | 0.0473 | 0.0473 | 0.0472 | 0.0471 | |
(0.0008) | (0.0007) | (0.0007) | (0.0005) | (0.0004) | ||
Ⅲ | 0.0498 | 0.0497 | 0.0497 | 0.0496 | 0.0495 | |
(0.0005) | (0.0004) | (0.0004) | (0.0003) | (0.0002) |
LINEX | ||||||
(n, m) | CS | MLE | SEL | c = - 2 | c = 0.0001 | c = 2 |
(30, 20) | Ⅰ | 1.1289 | 1.1288 | 1.1288 | 1.1287 | 1.1286 |
(0.0019) | (0.0016) | (0.0016) | (0.0014) | (0.0012) | ||
Ⅱ | 1.1253 | 1.1252 | 1.1252 | 1.1251 | 1.1250 | |
(0.0015) | (0.0013) | (0.0013) | (0.0012) | (0.0011) | ||
Ⅲ | 1.1229 | 1.1229 | 1.1229 | 1.1227 | 1.1226 | |
(0.0013) | (0.0012) | (0.0012) | (0.0011) | (0.0010) | ||
(40, 15) | Ⅰ | 1.1295 | 1.1294 | 1.1294 | 1.1293 | 1.1292 |
(0.0017) | (0.0016) | (0.0016) | (0.0014) | (0.0013) | ||
Ⅱ | 1.1242 | 1.1241 | 1.1241 | 1.1240 | 1.1239 | |
(0.0015) | (0.0013) | (0.0013) | (0.0011) | (0.0010) | ||
Ⅲ | 1.1193 | 1.1192 | 1.1192 | 1.1191 | 1.1190 | |
(0.0011) | (0.0009) | (0.0009) | (0.0008) | (0.0007) | ||
(40, 20) | Ⅰ | 1.1283 | 1.1282 | 1.1282 | 1.1281 | 1.1280 |
(0.0015) | (0.0014) | (0.0014) | (0.0013) | (0.0011) | ||
Ⅱ | 1.1256 | 1.1255 | 1.1255 | 1.1254 | 1.1252 | |
(0.0013) | (0.0012) | (0.0012) | (0.0011) | (0.0010) | ||
Ⅲ | 1.1203 | 1.1202 | 1.1202 | 1.1201 | 1.1200 | |
(0.004) | (0.003) | (0.003) | (0.002) | (0.001) | ||
(50, 15) | Ⅰ | 1.1279 | 1.1278 | 1.1278 | 1.1277 | 1.1276 |
(0.0016) | (0.0015) | (0.0015) | (0.0014) | (0.0012) | ||
Ⅱ | 1.1229 | 1.1228 | 1.1228 | 1.1227 | 1.1226 | |
(0.0019) | (0.0017) | (0.0017) | (0.0016) | (0.0014) | ||
Ⅲ | 1.1192 | 1.1191 | 1.1191 | 1.1190 | 1.1189 | |
(0.0015) | (0.0014) | (0.0014) | (0.0013) | (0.0011) | ||
(60, 15) | Ⅰ | 1.1256 | 1.1255 | 1.1255 | 1.1254 | 1.1253 |
(0.0015) | (0.0014) | (0.0014) | (0.0013) | (0.0012) | ||
Ⅱ | 1.1247 | 1.1246 | 1.1246 | 1.1245 | 1.1244 | |
(0.0016) | (0.0015) | (0.0015) | (0.0014) | (0.0012) | ||
Ⅲ | 1.1195 | 1.1194 | 1.1194 | 1.1193 | 1.1192 | |
(0.0016) | (0.0015) | (0.0015) | (0.0013) | (0.0011) | ||
(60, 20) | Ⅰ | 1.1257 | 1.1256 | 1.1256 | 1.1255 | 1.1254 |
(0.0016) | (0.0015) | (0.0015) | (0.0014) | (0.0013) | ||
Ⅱ | 1.1248 | 1.1247 | 1.1247 | 1.1246 | 1.1245 | |
(0.0017) | (0.0016) | (0.0016) | (0.0015) | (0.0013) | ||
Ⅲ | 1.1196 | 1.1195 | 1.1195 | 1.1194 | 1.1193 | |
(0.0017) | (0.0016) | (0.0016) | (0.0015) | (0.0012) |
α | β | ||||
(n, m) | CS | MLE | MCMC | MLE | MCMC |
(30, 20) | Ⅰ | 1.1444 | 0.0234 | 0.0751 | 0.0016 |
(0.9435) | (0.9446) | (0.9332) | (0.9418) | ||
Ⅱ | 1.1063 | 0.0236 | 0.0714 | 0.0015 | |
(0.9252) | (0.9367) | (0.9405) | (0.942) | ||
Ⅲ | 1.1302 | 0.0243 | 0.0688 | 0.0015 | |
(0.9572) | (0.9253) | (0.9396) | (0.9549) | ||
(40, 15) | Ⅰ | 1.2277 | 0.0268 | 0.0781 | 0.0017 |
(0.9698) | (0.9507) | (0.9377) | (0.9456) | ||
Ⅱ | 1.3317 | 0.0283 | 0.078 | 0.0016 | |
(0.9625) | (0.947) | (0.9608) | (0.9655) | ||
Ⅲ | 1.301 | 0.0281 | 0.0716 | 0.0015 | |
(0.951) | (0.9606) | (0.9712) | (0.9447) | ||
(40, 20) | Ⅰ | 1.0855 | 0.0231 | 0.0707 | 0.0015 |
(0.9615) | (0.9566) | (0.9573) | (0.9539) | ||
Ⅱ | 1.0899 | 0.0232 | 0.0698 | 0.0015 | |
(0.9256) | (0.947) | (0.9408) | (0.959) | ||
Ⅲ | 1.077 | 0.0227 | 0.0632 | 0.0013 | |
(0.9468) | (0.9568) | (0.9461) | (0.9616) | ||
(50, 15) | Ⅰ | 1.2419 | 0.0262 | 0.0754 | 0.0016 |
(0.9325) | (0.9845) | (0.9781) | (0.9863) | ||
Ⅱ | 1.3984 | 0.0293 | 0.0776 | 0.0016 | |
(0.9124) | (0.9683) | (0.9786) | (0.9452) | ||
Ⅲ | 1.2576 | 0.0274 | 0.0694 | 0.0015 | |
(0.9568) | (0.9565) | (0.9468) | (0.9478) | ||
(60, 15) | Ⅰ | 1.2813 | 0.0269 | 0.0719 | 0.0015 |
(0.9461) | (0.9616) | (0.9256) | (0.947) | ||
Ⅱ | 1.2959 | 0.0278 | 0.075 | 0.0016 | |
(0.9605) | (0.9641) | (0.9468) | (0.9568) | ||
Ⅲ | 1.2552 | 0.0275 | 0.0686 | 0.0015 | |
(0.9416) | (0.9486) | (0.9568) | (0.9665) | ||
(60, 20) | Ⅰ | 1.2832 | 0.0270 | 0.0720 | 0.0016 |
(0.9462) | (0.9617) | (0.9257) | (0.948) | ||
Ⅱ | 1.2960 | 0.0279 | 0.076 | 0.0017 | |
(0.9606) | (0.9642) | (0.94670) | (0.9569) | ||
Ⅲ | 1.2553 | 0.0276 | 0.0687 | 0.0016 | |
(0.9417) | (0.9487) | (0.9569) | (0.9666) |
S(t) | h(t) | ||||
(n, m) | CS | MLE | MCMC | MLE | MCMC |
(30, 20) | Ⅰ | 0.0755 | 0.0029 | 1.2694 | 0.0022 |
(0.9511) | (0.9489) | (0.952) | (0.9472) | ||
Ⅱ | 0.10705 | 0.003 | 1.2627 | 0.0021 | |
(0.9626) | (0.9546) | (0.9539) | (0.9278) | ||
Ⅲ | 0.0687 | 0.003 | 1.2572 | 0.0021 | |
(0.9576) | (0.9661) | (0.9361) | (0.9429) | ||
(40, 15) | Ⅰ | 0.0836 | 0.0033 | 1.2711 | 0.0024 |
(0.9421) | (0.9507) | (0.927) | (0.9296) | ||
Ⅱ | 0.081 | 0.0034 | 1.2593 | 0.0024 | |
(0.9325) | (0.9458) | (0.9736) | (0.9856) | ||
Ⅲ | 0.0748 | 0.0033 | 1.2469 | 0.0022 | |
(0.958) | (0.9603) | (0.9601) | (0.9602) | ||
(40, 20) | Ⅰ | 0.0739 | 0.0029 | 1.2693 | 0.0022 |
(0.9462) | (0.9467) | (0.9502) | (0.9506) | ||
Ⅱ | 0.07 | 0.0029 | 1.2633 | 0.0021 | |
(0.9256) | (0.9592) | (0.9704) | (0.9694) | ||
Ⅲ | 0.0645 | 0.00327 | 1.252 | 0.0019 | |
(0.9544) | (0.9581) | (0.9382) | (0.9454) | ||
(50, 15) | Ⅰ | 0.0839 | 0.0032 | 1.2671 | 0.0024 |
(0.9814) | (0.9145) | (0.9745) | (0.9635) | ||
Ⅱ | 0.0832 | 0.0035 | 1.2541 | 0.0023 | |
(0.9748) | (0.9862) | (0.9742) | (0.9681) | ||
Ⅲ | 0.0735 | 0.0032 | 1.2483 | 0.0022 | |
(0.9254) | (0.9438) | (0.9152) | (0.9634) | ||
(60, 15) | Ⅰ | 0.0835 | 0.0032 | 1.2625 | 0.0023 |
(0.9637) | (0.9504) | (0.9667) | (0.9567) | ||
Ⅱ | 0.0803 | 0.0033 | 1.2605 | 0.0024 | |
(0.9617) | (0.9325) | (0.9486) | (0.9479) | ||
Ⅲ | 0.0735 | 0.0032 | 1.2489 | 0.0021 | |
(0.9672) | (0.9488) | (0.9457) | (0.9639) | ||
(60, 20) | Ⅰ | 0.0836 | 0.0033 | 1.2626 | 0.0024 |
(0.9638) | (0.9505) | (0.9668) | (0.9568) | ||
Ⅱ | 0.0804 | 0.0034 | 1.2606 | 0.0025 | |
(0.9618) | (0.9326) | (0.9487) | (0.9480) | ||
Ⅲ | 0.0736 | 0.0033 | 1.2490 | 0.0022 | |
(0.9673) | (0.9489) | (0.9458) | (0.940) |
1.1 | 1.4 | 1.3 | 1.7 | 1.9 | 1.8 | 1.6 | 2.2 | 1.7 | 2.7 |
1.8 | 1.5 | 1.2 | 1.4 | 3 | 1.7 | 2.3 | 1.6 | 2 | 4.1 |
Model | α | β | θ | λ |
MFED | 32.433 | - | 2.0038 | - |
EE | 36.68 | - | 2.235 | |
RTFIW | 6.7199 | 3.7354 | - | - |
Model | K–S | p-Value | -logL | AIC | BIC | HQIC |
MFED | 0.120612 | 0.899975 | 15.9512 | 35.9024 | 37.8938 | 36.2911 |
EE | 0.1343 | 0.817352 | 16.2606 | 36.5212 | 38.5125 | 36.91 |
RTFIW | 0.100217 | 0.955062 | 14.9239 | 33.8478 | 35.8392 | 35.6581 |
Baysian | |||||
Parameters | MLE | SLE | LINEX | ||
c = - 2 | c = 0.0001 | c = 2 | |||
α | 1.16548 | 1.15804 | 1.15869 | 1.15804 | 1.15739 |
β | 2.85092 | 2.78356 | 2.796 | 2.78356 | 2.76981 |
S | 0.999772 | 0.99954 | 0.999541 | 0.99954 | 0.99954 |
h | 0.0109181 | 0.0184367 | 0.0185916 | 0.0184367 | 0.0182849 |
Parameter | MLE | MCMC |
α | (0.337871, 1.99309) | (1.11849, 1.2089) |
β | (0.8467, 4.85513) | (2.55267, 2.95076) |
S(0.4) | (0.996234, 1.00331) | (0.998601, 0.999898) |
h(0.4) | (−0.131477, 0.153313) | (0.00553594, 0.0472466) |
Model | α | β | θ | λ |
GIW | 0.792 | 0.298 | 3.261 | |
EGIW | .933 | 1.09 | 0.453 | 1.102 |
RTFIWD | 1.10716 | 1.37948 |
Model | K−S | P−Value | -2logL | AIC | BIC | CAIC |
GIW | 0.737 | 0.7584 | 795.582 | 801.582 | 806.648 | 802.248 |
EGIW | 0.831 | .82464 | 709.471 | 717.471 | 724.226 | 718.614 |
RTFIWD | 1.001 | 0.9744 | 594.72585 | 598.7258 | 602.1036 | 598.325 |
Parameters | MLE | Baysian | |||
SEL | LINEX | ||||
c=−2 | c=0.0001 | c=2 | |||
α | 1.10716 | 1.11602 | 1.11604 | 1.11601 | 1.11602 |
β | 1.37948 | 1.3736 | 1.37364 | 1.37357 | 1.3736 |
S | 0.94189 | 0.942492 | 0.942492 | 0.942491 | 0.942492 |
h | 0.490308 | 0.484726 | 0.484731 | 0.48472 | 0.484726 |
Parameter | MLE | MCMC |
α | (0.545939,1.66837) | (1.1084,1.12323) |
β | (0.701971,2.057) | (1.36354,1.38614) |
S(0.4) | (0.821262,1.06252) | (0.941573,0.94353) |
h(0.4) | (−0.0904752,1.07109) | (0.478703,0.489044) |