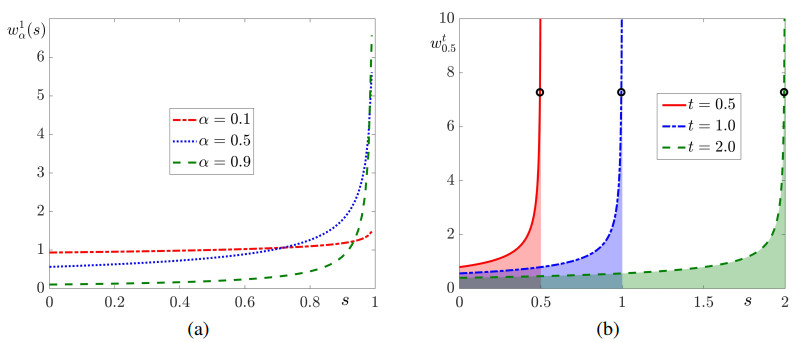
In this study, we proposed a normalized time-fractional diffusion equation and conducted a numerical investigation of the dynamics of the proposed equation. We discretized the governing equation by using a finite difference method. The proposed normalized time-fractional diffusion equation features a different time scale compared to the conventional time-fractional diffusion equation. This distinct time scale provides an intuitive understanding of the fractional time derivative, which represents a weighted average of the temporal history of the time derivative. Furthermore, the sum of the weight function is one for all values of the fractional parameter and time. The primary advantage of the proposed model over conventional time-fractional equations is the unity property of the sum of the weight function, which allows us to investigate the effects of the fractional order on the evolutionary dynamics of time-fractional equations. To highlight the differences in performance between the conventional and normalized time-fractional diffusion equations, we have conducted several numerical experiments.
Citation: Chaeyoung Lee, Yunjae Nam, Minjoon Bang, Seokjun Ham, Junseok Kim. Numerical investigation of the dynamics for a normalized time-fractional diffusion equation[J]. AIMS Mathematics, 2024, 9(10): 26671-26687. doi: 10.3934/math.20241297
[1] | Muhammad Asim Khan, Norma Alias, Umair Ali . A new fourth-order grouping iterative method for the time fractional sub-diffusion equation having a weak singularity at initial time. AIMS Mathematics, 2023, 8(6): 13725-13746. doi: 10.3934/math.2023697 |
[2] | Zihan Yue, Wei Jiang, Boying Wu, Biao Zhang . A meshless method based on the Laplace transform for multi-term time-space fractional diffusion equation. AIMS Mathematics, 2024, 9(3): 7040-7062. doi: 10.3934/math.2024343 |
[3] | Junseok Kim . A normalized Caputo–Fabrizio fractional diffusion equation. AIMS Mathematics, 2025, 10(3): 6195-6208. doi: 10.3934/math.2025282 |
[4] | Majeed A. Yousif, Juan L. G. Guirao, Pshtiwan Othman Mohammed, Nejmeddine Chorfi, Dumitru Baleanu . A computational study of time-fractional gas dynamics models by means of conformable finite difference method. AIMS Mathematics, 2024, 9(7): 19843-19858. doi: 10.3934/math.2024969 |
[5] | Mubashara Wali, Sadia Arshad, Sayed M Eldin, Imran Siddique . Numerical approximation of Atangana-Baleanu Caputo derivative for space-time fractional diffusion equations. AIMS Mathematics, 2023, 8(7): 15129-15147. doi: 10.3934/math.2023772 |
[6] | Zhiqiang Li . The finite time blow-up for Caputo-Hadamard fractional diffusion equation involving nonlinear memory. AIMS Mathematics, 2022, 7(7): 12913-12934. doi: 10.3934/math.2022715 |
[7] | Fouad Mohammad Salama, Faisal Fairag . On numerical solution of two-dimensional variable-order fractional diffusion equation arising in transport phenomena. AIMS Mathematics, 2024, 9(1): 340-370. doi: 10.3934/math.2024020 |
[8] | Fouad Mohammad Salama, Nur Nadiah Abd Hamid, Norhashidah Hj. Mohd Ali, Umair Ali . An efficient modified hybrid explicit group iterative method for the time-fractional diffusion equation in two space dimensions. AIMS Mathematics, 2022, 7(2): 2370-2392. doi: 10.3934/math.2022134 |
[9] | Ailing Zhu, Yixin Wang, Qiang Xu . A weak Galerkin finite element approximation of two-dimensional sub-diffusion equation with time-fractional derivative. AIMS Mathematics, 2020, 5(5): 4297-4310. doi: 10.3934/math.2020274 |
[10] | Krunal B. Kachhia, Jyotindra C. Prajapati . Generalized iterative method for the solution of linear and nonlinear fractional differential equations with composite fractional derivative operator. AIMS Mathematics, 2020, 5(4): 2888-2898. doi: 10.3934/math.2020186 |
In this study, we proposed a normalized time-fractional diffusion equation and conducted a numerical investigation of the dynamics of the proposed equation. We discretized the governing equation by using a finite difference method. The proposed normalized time-fractional diffusion equation features a different time scale compared to the conventional time-fractional diffusion equation. This distinct time scale provides an intuitive understanding of the fractional time derivative, which represents a weighted average of the temporal history of the time derivative. Furthermore, the sum of the weight function is one for all values of the fractional parameter and time. The primary advantage of the proposed model over conventional time-fractional equations is the unity property of the sum of the weight function, which allows us to investigate the effects of the fractional order on the evolutionary dynamics of time-fractional equations. To highlight the differences in performance between the conventional and normalized time-fractional diffusion equations, we have conducted several numerical experiments.
In recent decades, time-fractional diffusion equations have received significant attention across various fields. Time-fractional derivatives extend classical models by incorporating memory effects, which better capture real-world processes where the rate of change is not constant [1]. They are particularly useful for modeling phenomena with irregular or non-standard diffusion behaviors, such as in heterogeneous media [2], complex fluids [3], or biological systems [4]. These time-fractional derivatives allow for a more accurate representation of systems with long-range dependencies, thus providing deeper insights and improved predictions in various scientific and engineering fields. Some specific applications of time-fractional derivatives are as follows. The time-fractional Burgers equation, applying a fractional differential method to the classical Burgers equation [5,6], was used to model a range of physical processes such as turbulence, shock waves, and traffic flow, incorporating memory effects over time. In biology, the time-fractional method was applied to the reaction-diffusion equation, specifically Fisher's equation, which models species propagation [7,8]. In the field of finance, European and double-barrier options were evaluated using the time-fractional Black–Scholes equation [9,10,11]. In particular, for double barrier options, the closer the underlying asset price is to the lower barrier, the more the Black–Scholes model tends to overestimate the option's value. Furthermore, a smaller α exacerbates this price bias. The reaction-diffusion equation, known as the Allen–Cahn (AC) equation, finds applications in many fields such as physics, materials science, and biology. By applying the time-fractional method to the AC equation, these approaches accurately model complex dynamical systems by incorporating memory effects and history dependence associated with time [12,13].
There have been many numerical methods for the time-fractional diffusion equations [14,15,16]. For simplicity of exposition, let us consider the following one-dimensional conventional time-fractional diffusion equation:
∂αu(x,t)∂tα=∂2u(x,t)∂x2 for (x,t)∈Ω×(0,∞), | (1) |
u(x,0)=u0(x),x∈Ω, | (2) |
u(0,t)=u(1,t)=0,t≥0, | (3) |
where u(x,t) is the concentration at x and t, and u0(x) is the initial condition,
∂αu(x,t)∂tα=1Γ(1−α)∫t0∂u(x,s)∂sds(t−s)α,0<α<1, | (4) |
where Γ(z)=∫∞0τz−1e−τdτ is the gamma function. It is noted that when α=1, Eq (1) reduces to the conventional diffusion equation [17]. Let us define a weight function wtα(s) as follows:
wtα(s)=1Γ(1−α)(t−s)α. | (5) |
Then, Eq (4) can be rewritten as
∂αu(x,t)∂tα=∫t0wtα(s)∂u(x,s)∂sds,0<α<1. | (6) |
For different values of α=0.1,0.5, and 0.9, the weight functions wtα(s) at t=1 are illustrated in Figure 1(a). It can be seen that the weight functions wtα(s) remain flat for small α and show a sharp transition near time t for large α. Figure 1(b) shows the weight functions for different time values of t=0.5,1, and 2 with α=0.5. As t increases, the functions simply translate to the right direction.
We note that ∫t0wtα(s)ds approaches infinity as t increases, for any values of 0<α<1. That is,
Wα(t)=∫t0wtα(s)ds=∫t01Γ(1−α)(t−s)αds=t1−αΓ(2−α), | (7) |
which approaches infinity as t increases, for any values of 0<α<1. From Eq (7), we can see there are scaling differences associated with values of α when comparing the effects of α on the dynamics of the time-fractional diffusion equations because Wα(t) depends on both α and time t. This is physically sound and can be inferred intuitively from previous studies [18,19] related to wave propagation and diffusion problems.
To resolve these scaling differences associated with α values, we propose a normalized time-fractional diffusion equation and conduct numerical investigations of the dynamics of the proposed equation. In this study, we propose the following normalized time-fractional diffusion equation:
∂βu(x,t)∂tβ=∂2u(x,t)∂x2 for (x,t)∈Ω×(0,∞), | (8) |
u(x,0)=u0(x),x∈Ω, | (9) |
u(0,t)=u(1,t)=0,t≥0, | (10) |
where
∂βu(x,t)∂tβ=1−βt1−β∫t0∂u(x,s)∂sds(t−s)β,0<β<1, | (11) |
where (1−β)/t1−β is the normalizing factor, which makes the right-hand side term in Eq (11) ∂u/∂x when ∂u/∂x is constant. That is
1−βt1−β∫t0ds(t−s)β=1,0<β<1. | (12) |
Let us define a weight function wtβ(s) as follows:
wtβ(s)=1−βt1−β(t−s)β. | (13) |
Then, from Eq (7), we have
Wβ(t)=∫t0wtβ(s)ds=1, | (14) |
which is independent of the fractional order β and time t, unlike that of the conventional time-fractional derivative, Wα(t)=t1−α/Γ(2−α). To the authors' knowledge, this is the first time that the normalized time-fractional diffusion equation is proposed, where the total integration of the weight function is always one for all time-fractional orders and times. For β values of 0.1, 0.5, and 0.9, the weight functions wtβ(s) at t=1 are as shown in Figure 2(a). We can observe that the weight functions wtβ(s) are flat when β is small and exhibit a sharp transition near time t when β is large. Figure 2(b) shows the weight functions for different times t=0.5,1, and 2 with β=0.5.
Figure 3 shows the temporal evolutions of Wα(t)=∫t0wtα(s)ds=t1−α/Γ(2−α) for α=0.1,0.5, and 0.9. Wα(t) is an increasing function with respect to time t for a fixed fractional order α. At early times, Wα(t) increases with respect to the fractional order α, whereas at later times, Wα(t) decreases with respect to the fractional order α for a fixed time t. However, Wβ(t) is independent of the fractional order β and time t.
The contents of this paper are as follows. In Section 2, numerical solution algorithms for the conventional and normalized time-fractional diffusion equations are presented. In Section 3, numerical experiments are provided. Finally, Section 4 presents conclusion and potential progress for future study.
Let Ω=(Lx,Rx) be the computational domain, which is discretized as follows: Ωh={xi|xi=Lx+(i−1)h,i=1,…,Nx}, where h=(Rx−Lx)/(Nx−1) for some positive integer Nx, see Figure 4.
Let uni=u(xi,tn) and tn=(n−1)Δt, where Δt is the time step. Equation (4) can be approximated by the following numerical quadrature formula:
∂αu(xi,tn+1)∂tα=1Γ(1−α)n∑p=1∫tp+1tp∂u(xi,s)∂sds(tn+1−s)α≈n∑p=11Γ(1−α)∫tp+1tpds(tn+1−s)αup+1i−upiΔt | (15) |
=n∑p=1(n+1−p)1−α−(n−p)1−α(Δt)α−1Γ(2−α)up+1i−upiΔt, | (16) |
where we have used the identity (1−α)Γ(1−α)=Γ(2−α) and approximated ∂u(xi,s)/∂s over the interval [tp,tp+1] using the finite difference (up+1i−upi)/Δt in Eq (15). Therefore, we have the following finite difference discretization of Eq (1) using Eq (16):
n∑p=1wnpup+1i−upiΔt=un+1i−1−2un+1i+un+1i+1h2, | (17) |
where
wnp=(n+1−p)1−α−(n−p)1−α(Δt)α−1Γ(2−α). | (18) |
Here, we use the zero Dirichlet boundary condition: un+10=0 and un+1Nx=0. Then, Eq (17) can be rewritten as follows:
wnnun+1i−uniΔt+n−1∑p=1wnpup+1i−upiΔt=un+1i−1−2un+1i+un+1i+1h2, | (19) |
which can be rearranged as follows:
−1h2un+1i−1+(wnnΔt+2h2)un+1i−1h2un+1i+1=wnnΔtuni−n−1∑p=1wnpup+1i−upiΔt. | (20) |
Note that an implicit temporal discretization is used for stability. Although fully explicit schemes are generally sufficient in terms of stability and efficiency for second-order partial differential equations, as indicated in [20,21], an implicit scheme is necessary due to the presence of the source term, −∑n−1p=1wnp(up+1i−upi)/Δt, in Eq (20).
Equation (20) is a tridiagonal system with a zero Dirichlet boundary condition and we can use the Thomas algorithm [22] to efficiently solve this system. Thus, the solution vector un+1 can be found by solving the tridiagonal system using the Thomas algorithm:
Aun+1=f, |
where A is a tridiagonal matrix with zero Dirichlet at i=1 and i=Nx. The detailed procedure is provided below.
A=(wnnΔt+2h2−1h20⋯000−1h2wnnΔt+2h2−1h2⋯0000−1h2wnnΔt+2h2⋯000⋮⋮⋮⋱⋮⋮⋮000⋯−1h2wnnΔt+2h2−1h2000⋯0−1h2wnnΔt+2h2),un+1=(un+12un+13⋮un+1Nx−1) and f=(wnnun2/Δt−Fwnnun3/Δt−F⋮wnnunNx−1/Δt−F), |
where F=∑n−1p=1wnp(up+1i−upi)/Δt.
Equation (11) can be approximated by the following numerical quadrature formula:
∂βu(xi,tn+1)∂tβ=1−βt1−βn+1n∑p=1∫tp+1tp∂u(xi,s)∂sds(tn+1−s)β≈n∑p=11−βt1−βn+1∫tp+1tpds(tn+1−s)βup+1i−upiΔt=n∑p=1(n+1−p)1−β−(n−p)1−βn1−βup+1i−upiΔt. | (21) |
Therefore, we have the following finite difference discretization of Eq (8) using Eq (21):
n∑p=1wnpup+1i−upiΔt=un+1i−1−2un+1i+un+1i+1h2, | (22) |
where
wnp=(n+1−p)1−β−(n−p)1−βn1−β. | (23) |
We note that the weight parameter wnp satisfies the following condition for any value of n:
n∑p=1wnp=1. | (24) |
Then, Eq (22) can be rewritten as follows:
−1h2un+1i−1+(wnnΔt+2h2)un+1i−1h2un+1i+1=wnnΔtuni−n−1∑p=1wnpup+1i−upiΔt. | (25) |
Equation (25) is a tridiagonal system with a zero Dirichlet boundary condition and we can use the Thomas algorithm to efficiently solve this system. Thus, the solution vector un+1 can be found by solving the tridiagonal system using the Thomas algorithm:
Aun+1=f, |
where A is a tridiagonal matrix with zero Dirichlet at i=1 and i=Nx.
In this section, we present several numerical experiments in a finite domain Ω×(0,T), where Ω=(0,1) and T is a final time, to investigate the effects of α and β on the evolution dynamics of the conventional and normalized time-fractional diffusion equations. As the first numerical test, we consider the following low-frequency initial condition:
u(x,0)=sin(2πx), for x∈Ω. | (26) |
Figure 5(a) and (b) show u(x,T) for different values of α=0.1,0.5, and 0.9; and β=0.1,0.5, and 0.9, respectively. Here, T=0.001 is used. As shown in Figure 5(a), for the conventional time-fractional diffusion equation, we observe that the temporal evolution is faster when the value of α is smaller. This phenomenon is attributed to the different weight function associated with different α values, as shown in Figure 1(a). When α is smaller, the total sum of the weight function at early times is smaller as shown in Figure 3, which results in an effectively larger diffusion process and faster temporal evolution. This can be understood as follows: if we divide both sides of Eq (1) by the smaller weight value, we obtain an effectively larger diffusion coefficient, which leads to an increased diffusion process. However, in the case of the normalized time-fractional diffusion equation, there is little variation with respect to different values of β compared to the conventional time-fractional diffusion equation, as seen in Figure 5(b).
In the second numerical experiment, let us consider the following high-frequency initial condition:
u(x,0)=sin(10πx), for x∈Ω. | (27) |
Figures 6(a) and (b) show u(x,T) for different values of α=0.1,0.5, and 0.9; and β=0.1,0.5, and 0.9, respectively. We observe that the temporal evolutions are faster than those of the low-frequency initial condition for both the conventional and normalized time-fractional diffusion equations. As illustrated in Figure 6(a), the temporal evolution for the conventional time-fractional diffusion equation accelerates more than that of the low-frequency initial condition as the value of α decreases. A smaller α results in a smaller total sum of the weight function, which leads to an effectively larger diffusion process and consequently faster temporal evolution. However, for the normalized time-fractional diffusion equation, there is a little variation concerning different β values, unlike the conventional time-fractional diffusion equation, as shown in Figure 6(b).
For the third numerical test, let us consider the following random initial condition:
u(x,0)=rand(x), for x∈Ω, | (28) |
u(0,t)=u(1,t)=0, | (29) |
where rand(x) is a random number between −1 and 1. Figures 7(a) and (b) show u(x,T) for different values of α=0.1,0.5, and 0.9; and β=0.1,0.5, and 0.9, respectively. The random initial condition can be considered as a combination of multiple frequency modes. As expected from the previous computational results, we observe large variation with respect to α values and small variation with respect to β values.
In the fourth numerical test, we consider the following initial condition:
u(x,0)=sin(7√πx), for x∈(0,π). | (30) |
This initial condition contains multiple modes with gradually changing profiles. Figure 8(a) shows the numerical solution u(x,T) for a relatively short time, T=0.0005, and Figure 8(b) and 8(c) display u(x,T) for a relatively long time, T=0.05. Figures 8(a) and 8(b) present results for different values of α=0.1,0.5, and 0.9, and Fig. 8(c) shows results for different values of β=0.1,0.5, and 0.9. Because the initial condition is a combination of multiple frequency modes, we observe significant evolutions at high frequencies and minor changes at low frequencies.
In the final numerical test, we examine the evolution process of the conventional time-fractional method and the normalized time-fractional method for different fractional orders α=0.1,0.9 and β=0.1,0.9. We consider the following initial condition:
u(x,0)=sin(0.2πx), for x∈(0,5). | (31) |
In this test, we used a final time of T=5. Figures 9(a) and (b) represent the evolution of the conventional time-fractional diffusion equation for the different α=0.1 and 0.9, respectively. When α is small, the temporal evolution is fast at early times but suddenly slows down at later times. This phenomenon, as shown in Figure 3, can be attributed to the small weight function values at early times and the large weight function values at later times. When α is large, the opposite behavior can be observed. Next, Figures 9(c) and (d) illustrate the evolution of the normalized time-fractional diffusion equation for the different values of β=0.1 and 0.9, respectively. In this case, the temporal evolution depends on the β values but it is relatively independent of time.
In summary, the evolution dynamics of the conventional and normalized time-fractional diffusion equations are different. The total integration of the weight function for the conventional time-fractional diffusion equation is not normalized, continues to increase to infinity as time progresses; it depends on the values of α and time t. Due to these dependencies, it is difficult to study the effects of α on the evolutionary dynamics. As an alternative, we proposed a normalized time-fractional diffusion equation that ensures that the total integration of the weight function remains one, regardless of the values of β and time t. The proposed weight functions provide an intuitive understanding of the fractional time derivative, which represents a weighted average of the temporal history of the time derivative. Numerical experiments indicate that the normalized time-fractional diffusion equation shows slower dynamics for simple initial conditions as the fractional order parameter β decreases. The diffusion equation is a fundamental model for various phenomena such as heat conduction, fluid flow, chemical dispersion, cellular diffusion processes, and the evolution of option prices and market risks in finance. As future work, we will apply the proposed model to the Black–Scholes equation [23,24], the AC equation [25,26,27], and the nonlocal Cahn–Hilliard equation [28], and investigate the development of a fast algorithm to reduce memory requirements [29].
Chaeyoung Lee: Investigation, Writing–original draft, Validation, Writing–Review & Editing, Software; Yunjae Nam: Formal analysis, Visualization, Writing–original draft, Writing–Review & Editing; Minjoon Bang: Writing–original draft, Writing–Review & Editing, Software, Visualization; Seokjun Ham: Validation, Writing–original draft, Writing–Review & Editing, Visualization; Junseok Kim: Conceptualization, Investigation, Formal analysis, Supervision, Writing–original draft, Writing–Review & Editing. All authors have read and agreed to the published version of the manuscript.
The first author (C. Lee) was supported by the National Research Foundation of Korea (NRF) grant funded by the Korea government (MSIT) (No. 2022R1C1C2003896). The corresponding author (J.S. Kim) was supported by the Brain Korea 21 FOUR from the Ministry of Education of Korea. The authors are grateful to the reviewers for their insightful and constructive feedback, which has significantly enhanced the quality of this paper.
The authors declare that there is no conflict of interest.
The following listings 1 and 2 are Python codes for the conventional and normalized time-fractional diffusion equations, respectively.
Listing 1. Python code for a conventional time-fractional heat equation
![]() |
Listing 2. Python code for a normalized time-fractional heat equation
![]() |
[1] |
J. J. Liu, M. Yamamoto, A backward problem for the time-fractional diffusion equation, Appl. Anal., 89 (2010), 1769–1788. https://doi.org/10.1080/00036810903479731 doi: 10.1080/00036810903479731
![]() |
[2] |
L. Feng, I. Turner, P. Perré, K. Burrage, The use of a time-fractional transport model for performing computational homogenisation of 2D heterogeneous media exhibiting memory effects, J. Comput. Phys., 480 (2023), 112020. https://doi.org/10.1016/j.jcp.2023.112020 doi: 10.1016/j.jcp.2023.112020
![]() |
[3] |
M. Biglari, A. R. Soheili, Efficient simulation of two-dimensional time-fractional Navier–Stokes equations using RBF-FD approach, Eng. Anal. Bound. Elem., 160 (2024), 134–159. https://doi.org/10.1016/j.enganabound.2023.12.021 doi: 10.1016/j.enganabound.2023.12.021
![]() |
[4] |
F. A. Rihan, Q. M. Al-Mdallal, H. J. AlSakaji, A. Hashish, A fractional-order epidemic model with time-delay and nonlinear incidence rate, Chaos Soliton. Fract., 126 (2019), 97–105. https://doi.org/10.1016/j.chaos.2019.05.039 doi: 10.1016/j.chaos.2019.05.039
![]() |
[5] |
M. Inc, The approximate and exact solutions of the space-and time-fractional Burgers equations with initial conditions by variational iteration method, J. Math. Anal. Appl., 345 (2008), 476–484. https://doi.org/10.1016/j.jmaa.2008.04.007 doi: 10.1016/j.jmaa.2008.04.007
![]() |
[6] |
J. G. Liu, J. Zhang, A new approximate method to the time fractional damped Burger equation, AIMS Math., 8 (2023), 13317–13324. https://doi.org/10.3934/math.2023674 doi: 10.3934/math.2023674
![]() |
[7] |
A. M. Zidan, A. Khan, R. Shah, M. K. Alaoui, W. Weera, Evaluation of time-fractional Fisher's equations with the help of analytical methods, AIMS Math., 7 (2022), 18746–66. https://doi.org/10.3934/math.20221031 doi: 10.3934/math.20221031
![]() |
[8] |
X. Qin, X. Yang, P. Lyu, A class of explicit implicit alternating difference schemes for generalized time fractional Fisher equation, AIMS Math., 6 (2021), 11449–11466. https://doi.org/10.3934/math.2021663 doi: 10.3934/math.2021663
![]() |
[9] |
W. Chen, X. Xu, S. P. Zhu, Analytically pricing double barrier options based on a time-fractional Black–Scholes equation, Comput. Math. Appl., 69 (2015), 1407–1419. https://doi.org/10.1016/j.camwa.2015.03.025 doi: 10.1016/j.camwa.2015.03.025
![]() |
[10] |
A. Golbabai, O. Nikan, T. Nikazad, Numerical analysis of time fractional Black–Scholes European option pricing model arising in financial market, Comput. Appl. Math., 38 (2019), 1–24. https://doi.org/10.1007/s40314-019-0957-7 doi: 10.1007/s40314-019-0957-7
![]() |
[11] |
H. Zhang, F. Liu, I. Turner, Q. Yang, Numerical solution of the time fractional Black–Scholes model governing European options, Comput. Math. Appl., 71 (2016), 1772–1783. https://doi.org/10.1016/j.camwa.2016.02.007 doi: 10.1016/j.camwa.2016.02.007
![]() |
[12] |
Q. Du, J. Yang, Z. Zhou, Time-fractional Allen–Cahn equations: analysis and numerical methods, J. Sci. Comput., 85 (2020), 42. https://doi.org/10.1007/s10915-020-01351-5 doi: 10.1007/s10915-020-01351-5
![]() |
[13] |
H. Liu, A. Cheng, H. Wang, J. Zhao, Time-fractional Allen–Cahn and Cahn–Hilliard phase-field models and their numerical investigation, Comput. Math. Appl., 76 (2018), 1876–1892. https://doi.org/10.1016/j.jocs.2023.102114 doi: 10.1016/j.jocs.2023.102114
![]() |
[14] |
B. Derbissaly, M. Sadybekov, Inverse source problem for multi-term time-fractional diffusion equation with nonlocal boundary conditions, AIMS Math., 9 (2024), 9969–9988. https://doi.org/10.3934/math.2024488 doi: 10.3934/math.2024488
![]() |
[15] |
W. M. Abd-Elhameed, H. M. Ahmed, Spectral solutions for the time-fractional heat differential equation through a novel unified sequence of Chebyshev polynomials, AIMS Math., 9 (2024), 2137–2166. https://doi.org/10.3934/math.2024107 doi: 10.3934/math.2024107
![]() |
[16] |
Y. E. Aghdam, H. Mesgarani, Z. Asadi, V. T. Nguyen, Investigation and analysis of the numerical approach to solve the multi-term time-fractional advection-diffusion model, AIMS Math., 8 (2023), 29474. https://doi.org/10.3934/math.20231509 doi: 10.3934/math.20231509
![]() |
[17] |
J. Kim, S. Kwak, H. G. Lee, Y. Hwang, S. Ham, A maximum principle of the Fourier spectral method for diffusion equations, Electron. Res. Arch., 31 (2023), 5396–5405. https://doi.org/10.3934/era.2023273 doi: 10.3934/era.2023273
![]() |
[18] |
J. M. Carcione, Theory and modeling of constant-Q P-and S-waves using fractional time derivatives, Geophysics, 74 (2009), T1–T11. https://doi.org/10.1190/1.3008548 doi: 10.1190/1.3008548
![]() |
[19] |
J. M. Carcione, F. Cavallini, F. Mainardi, A. Hanyga, Time-domain modeling of constant-Q seismic waves using fractional derivatives, Pure Appl. Geophys., 159 (2002), 1719–1736. https://doi.org/10.1007/s00024-002-8705-z doi: 10.1007/s00024-002-8705-z
![]() |
[20] |
S. Ham, J. Kim, Stability analysis for a maximum principle preserving explicit scheme of the Allen–Cahn equation, Math. Comput. Simul., 207 (2023), 453–465. https://doi.org/10.1016/j.matcom.2023.01.016 doi: 10.1016/j.matcom.2023.01.016
![]() |
[21] |
J. Wang, Z. Han, W. Jiang, J. Kim, A fast, efficient, and explicit phase-field model for 3D mesh denoising, Appl. Math. Comput., 458 (2023), 128239. https://doi.org/10.1016/j.amc.2023.128239 doi: 10.1016/j.amc.2023.128239
![]() |
[22] | J. W. Thomas, Numerical partial differential equations: finite difference methods in Springer Science & Business Media (2013). |
[23] |
M. Sarboland, A. Aminataei, On the numerical solution of time fractional Black-Scholes equation, Int. J. Comput. Math., 99 (2022), 1736–1753. https://doi.org/10.1080/00207160.2021.2011248 doi: 10.1080/00207160.2021.2011248
![]() |
[24] |
J. Huang, Z. Cen, J. Zhao, An adaptive moving mesh method for a time-fractional Black–-Scholes equation, Adv. Differ. Equ., 2019 (2019), 1–14. https://doi.org/10.1186/s13662-019-2453-1 doi: 10.1186/s13662-019-2453-1
![]() |
[25] |
B. Xia, R. Yu, X. Song, X. Zhang, J. Kim, An efficient data assimilation algorithm using the Allen–Cahn equation, Eng. Anal. Bound. Elem., 155 (2023), 511–517. https://doi.org/10.1016/j.enganabound.2023.06.029 doi: 10.1016/j.enganabound.2023.06.029
![]() |
[26] |
Y. Hwang, I. Kim, S. Kwak, S. Ham, S. Kim, J. Kim, Unconditionally stable monte carlo simulation for solving the multi-dimensional Allen–Cahn equation, Electron. Res. Arch., 31 (2023), 5104–5123. https://doi.org/10.3934/era.2023261 doi: 10.3934/era.2023261
![]() |
[27] |
Y. Hwang, S. Ham, C. Lee, G. Lee, S. Kang, J. Kim, A simple and efficient numerical method for the Allen–Cahn equation on effective symmetric triangular meshes, Electron. Res. Arch., 31 (2023), 4557–4578. https://doi.org/10.3934/era.2023233 doi: 10.3934/era.2023233
![]() |
[28] |
C. Lee, S. Kim, S. Kwak, Y. Hwang, S. Ham, S. Kang, J. Kim, Semi-automatic fingerprint image restoration algorithm using a partial differential equation, AIMS Math., 8 (2023), 27528-27541. https://doi.org/10.3934/math.20231408 doi: 10.3934/math.20231408
![]() |
[29] |
Z. W. Fang, H. W. Sun, H. Wang, A fast method for variable-order Caputo fractional derivative with applications to time-fractional diffusion equations, Comput. Math. Appl., 80 (2020), 1443–1458. https://doi.org/10.1016/j.camwa.2020.07.009 doi: 10.1016/j.camwa.2020.07.009
![]() |
1. | Junseok Kim, Influence of Fractional Order on the Behavior of a Normalized Time-Fractional SIR Model, 2024, 12, 2227-7390, 3081, 10.3390/math12193081 | |
2. | Junseok Kim, Nonlinear dynamic evolution of a novel normalized time-fractional Burgers equation, 2025, 13, 26668181, 101096, 10.1016/j.padiff.2025.101096 | |
3. | Soobin Kwak, Yunjae Nam, Seungyoon Kang, Junseok Kim, Computational analysis of a normalized time-fractional Fisher equation, 2025, 166, 08939659, 109542, 10.1016/j.aml.2025.109542 | |
4. | Jian Wang, Keyong Chen, Junseok Kim, Computational analysis of a normalized time-fractional Fokker–Planck equation, 2025, 665, 03784371, 130500, 10.1016/j.physa.2025.130500 | |
5. | Junseok Kim, A normalized Caputo–Fabrizio fractional diffusion equation, 2025, 10, 2473-6988, 6195, 10.3934/math.2025282 |