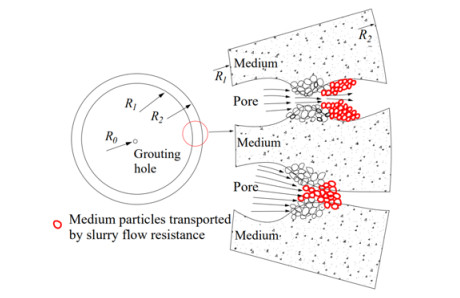
In recent years, the application of variable-order (VO) fractional differential equations for describing complex physical phenomena ranging from biology, hydrology, mechanics and viscoelasticity to fluid dynamics has become one of the most hot topics in the context of scientific modeling. An interesting aspect of VO operators is their capability to address the behavior of scientific and engineering systems with time and spatially varying properties. The VO fractional diffusion equation is a fundamental model that allows transitions among sub-diffusive, diffusive and super-diffusive behaviors without altering the underlying governing equations. In this paper, we considered the two-dimensional fractional diffusion equation with the Caputo time VO derivative, which is essential for describing anomalous diffusion in real-world complex systems. A new Crank-Nicolson (C-N) difference scheme and an efficient explicit decoupled group (EDG) method were proposed to solve the problem under consideration. The proposed EDG method is based on a skewed difference scheme in conjunction with a grouping procedure of the solution grid points. Special attention was devoted to investigating the stability and convergence of the proposed methods. Three numerical examples with known exact analytical solutions were provided to illustrate our considerations. The proposed methods were shown to be stable and convergent theoretically as well as numerically. In addition, a comparative study was done between the EDG method and the C-N difference scheme. It was found that the proposed methods are accurate in simulating the considered problem, while the EDG method is superior to the C-N difference method in terms of Central Processing Unit (CPU) timing, verifying the efficiency of the former method in solving the VO problem.
Citation: Fouad Mohammad Salama, Faisal Fairag. On numerical solution of two-dimensional variable-order fractional diffusion equation arising in transport phenomena[J]. AIMS Mathematics, 2024, 9(1): 340-370. doi: 10.3934/math.2024020
[1] | Jiwei Jia, Young-Ju Lee, Yue Feng, Zichan Wang, Zhongshu Zhao . Hybridized weak Galerkin finite element methods for Brinkman equations. Electronic Research Archive, 2021, 29(3): 2489-2516. doi: 10.3934/era.2020126 |
[2] | Linlin Tan, Bianru Cheng . Global well-posedness of 2D incompressible Navier–Stokes–Darcy flow in a type of generalized time-dependent porosity media. Electronic Research Archive, 2024, 32(10): 5649-5681. doi: 10.3934/era.2024262 |
[3] | Nisachon Kumankat, Kanognudge Wuttanachamsri . Well-posedness of generalized Stokes-Brinkman equations modeling moving solid phases. Electronic Research Archive, 2023, 31(3): 1641-1661. doi: 10.3934/era.2023085 |
[4] | Xiang Zhang, Tingting Zheng, Yantao Luo, Pengfei Liu . Analysis of a reaction-diffusion AIDS model with media coverage and population heterogeneity. Electronic Research Archive, 2025, 33(1): 513-536. doi: 10.3934/era.2025024 |
[5] | Jichun Li . Recent progress on mathematical analysis and numerical simulations for Maxwell's equations in perfectly matched layers and complex media: a review. Electronic Research Archive, 2024, 32(3): 1901-1922. doi: 10.3934/era.2024087 |
[6] | Ning Li, Yuequn Gao . Modified fractional order social media addiction modeling and sliding mode control considering a professionally operating population. Electronic Research Archive, 2024, 32(6): 4043-4073. doi: 10.3934/era.2024182 |
[7] | Xin-Guang Yang, Lu Li, Xingjie Yan, Ling Ding . The structure and stability of pullback attractors for 3D Brinkman-Forchheimer equation with delay. Electronic Research Archive, 2020, 28(4): 1395-1418. doi: 10.3934/era.2020074 |
[8] | N. Bazarra, J. R. Fernández, R. Quintanilla . A dual-phase-lag porous-thermoelastic problem with microtemperatures. Electronic Research Archive, 2022, 30(4): 1236-1262. doi: 10.3934/era.2022065 |
[9] | Wenya Qi, Padmanabhan Seshaiyer, Junping Wang . A four-field mixed finite element method for Biot's consolidation problems. Electronic Research Archive, 2021, 29(3): 2517-2532. doi: 10.3934/era.2020127 |
[10] | Quan Dai, Biao Zhao, Shuguang Wang, Dongliang Huang, Changrui Jin . Numerical simulation scheme of jointed rock masses using UAV photogrammetry and a disk-based discontinuous deformation analysis model. Electronic Research Archive, 2023, 31(6): 3381-3399. doi: 10.3934/era.2023171 |
In recent years, the application of variable-order (VO) fractional differential equations for describing complex physical phenomena ranging from biology, hydrology, mechanics and viscoelasticity to fluid dynamics has become one of the most hot topics in the context of scientific modeling. An interesting aspect of VO operators is their capability to address the behavior of scientific and engineering systems with time and spatially varying properties. The VO fractional diffusion equation is a fundamental model that allows transitions among sub-diffusive, diffusive and super-diffusive behaviors without altering the underlying governing equations. In this paper, we considered the two-dimensional fractional diffusion equation with the Caputo time VO derivative, which is essential for describing anomalous diffusion in real-world complex systems. A new Crank-Nicolson (C-N) difference scheme and an efficient explicit decoupled group (EDG) method were proposed to solve the problem under consideration. The proposed EDG method is based on a skewed difference scheme in conjunction with a grouping procedure of the solution grid points. Special attention was devoted to investigating the stability and convergence of the proposed methods. Three numerical examples with known exact analytical solutions were provided to illustrate our considerations. The proposed methods were shown to be stable and convergent theoretically as well as numerically. In addition, a comparative study was done between the EDG method and the C-N difference scheme. It was found that the proposed methods are accurate in simulating the considered problem, while the EDG method is superior to the C-N difference method in terms of Central Processing Unit (CPU) timing, verifying the efficiency of the former method in solving the VO problem.
Since the 20th century, there have been tremendous changes in the infrastructure construction in China, and underground excavation projects such as tunnels and subways have been continuously developed [1]. However, geological hazards such as large deformation of tunnel surrounding rock, water inrush, groundwater and weak strata also pose a threat to underground engineering. Reinforcement is the most effective way to solve such disasters, such as anchor reinforcement [2,3,4], grouting reinforcement. Currently, grouting is widely used in engineering projects such as plugging and reinforcement during subway and tunnel construction [5,6].
Infiltration grouting has become the most widely used and extensively researched grouting method in engineering practice due to its relatively easy control during the grouting process and its non-destructive effect on surrounding strata [7]. Domestic and foreign scholars [8,9,10,11,12] have conducted a large number of laboratory tests on infiltration grouting, studying the relationship between the diffusion range of grout mixture and grouting parameters such as grouting time, grouting pressure, and water-cement ratio of the grout mixture. Compared to the widespread application of infiltration grouting in engineering, the theoretical development of infiltration grouting has been lagging behind due to the many influencing factors involved. Earlier, scholars established the Mag theory and the Lefranc-Greenwood theory based on Darcy's law for Newtonian fluids [13,14]. Later, many scholars further studied the diffusion path of cement-based grout columns [15,16], the time-dependent viscosity of the grout mixture [17,18,19], the filtration effect of the grout mixture [20,21,22], and the gravity of the grout mixture [23,24] on the diffusion range of infiltration grouting.
The above studies assume that the pores in rock and soil porous media are uniformly distributed. However, the distribution of pores in rock and soil porous media is complex, and assuming that the pores are uniformly distributed often results in an overestimation of the diffusion range of the grout mixture in infiltration grouting practice.
Pore distribution in porous media is characterized by curvature and uncertainty and can generally be classified into capillary, throat, and pipe-ball models [25,26]. When fluid flows through curved and unevenly distributed pores and throats in porous media, it inevitably acts on the particles around the porous media, causing changes in pore shape and size. The distribution and size of pores in porous media determine the permeability of porous media. Therefore, the permeability of rock and soil porous media will inevitably change when fluid flows through it. Permeability of porous media is an important factor in analyzing and studying porous media. For example, Sun [27] studied the effect of pore pressure on the permeability of mudstone sandstone, and Qu et al. [28] used machine learning to study the effect of pore stress concentration on the permeability of porous media and verified a stress-dependent permeability model influenced by stress concentration with experimental data. In the field of oil and gas engineering, the stress sensitivity of permeability is often considered [29,30,31].
Bear [32] proposes that when fluid flows through porous media, it will produce flow resistance along the velocity direction of the solid boundary. However, the influence of fluid flow resistance on the permeability of porous media in infiltration grouting has not been considered in the study of the factors affecting the diffusion range of grout mixture. As shown in Figure 1, after the grout mixture is injected into the porous media, the flow resistance it produces will act on the curved and unevenly distributed pores, causing the particles around the pores to migrate in the direction of the grout mixture velocity, resulting in a narrowing of the pores, and even blocking them, thereby changing the permeability of the porous media.
Therefore, this paper aims to investigate the impact of flow resistance of the grout mixture on the permeability of porous media during the infiltration grouting process. A model for the variation of permeability in porous media is obtained by applying the diffusion law of infiltration grouting column-hemispherical diffusion. An infiltration grouting diffusion model is then developed by combining the rheological equation and the equilibrium equation of the Newtonian fluid, which takes into account the variation of permeability in rock and soil porous media. Also the effectiveness of the proposed model is evaluated by comparing the numerical simulation results with the laboratory test data.
1) Neglecting the effects of filtration, gravity, and flow pattern changes during the grouting process 2) considering the grout mixture as an incompressible, isotropic fluid during the grouting process; 3) ignoring the effects of turbulence near the injection hole and assuming laminar flow throughout the grouting process and 4) assuming that the grout mixture diffuses according to the column-hemispherical infiltration diffusion and Darcy's law applies.
Iberall [33] derived an expression for the permeability of porous media using a flow resistance model,
K=316⋅ϕ1−ϕ⋅δ2⋅2−lnRe4−lnRe | (1) |
Re=ρvδμ | (2) |
where Re represents the Reynolds number, ρ represents the density of the grout mixture, v represents the average velocity of the grout mixture, δ represents the initial pore diameter of the porous media, μ represents the viscosity of the grout mixture, andφ represents the porosity of the porous media.
When the diffusion radius of the grout mixture is l, the grouting volume Q can be expressed as:
Q=vAt | (3) |
where v represents the average velocity of the grout mixture during diffusion, t represents the grouting time, and A represents the spherical surface area over which the grout mixture diffuses during time t.
The surface area A of the column-hemispheric diffusion zone can be expressed as [37]:
A=2πl2+2πlh | (4) |
where l is the slurry diffusion radius in the hemisphere in time t, h is the slurry diffusion height in the cylindrical area.
Combining Eq (1) to Eq (4)
Re=ρδμ⋅Q2πl(l+h)t | (5) |
The total grouting volume Q as:
Q=(23πl3+πl2h)⋅ϕ | (6) |
Bear derived an expression for the permeability of porous media using a flow resistance model that depends on the geometry of the pore system, as shown in Eq (7):
C = K0δ2=πϕ26λ(1−ϕ) | (7) |
where C is a coefficient that depends on the geometry of the pore system, K0 is the initial value of permeability, λ is the influence coefficient of soil particles in porous media, and for spherical soil particles, λ = 3π [32].
By substituting the initial permeability of the porous media into Eq (7), the value of the pore diameter d can be obtained, Harleman et al. [34] verified the correctness of this formula through experiments.
Combining Eqs (1) and (5) to Eq (7), the permeability variation model in porous media can be expressed as:
K=316⋅6K0λπϕ⋅(1−24−lnρδϕα2μt) | (8) |
where α = ((2/3l + h)l)/(l + h), δ = sqrt((6K0λ(1 - φ))/πφ2), t is the grouting time, l is the slurry diffusion radius in the hemisphere, h is the slurry diffusion height in the cylindrical region, K0 is the initial permeability of porous media, ρ is the slurry density, δ is the initial pore diameter of porous media, μ is the slurry viscosity, Φ is the porosity of porous media, λ is the influence coefficient of porous media soil particles, and for spherical soil particles, λ = 3π.
The flow diagram of Newtonian fluid in the tube is shown in Figure 2 [35].
The force analysis of the fluid unit is carried out without considering the gravity of the slurry.
pπr2−(p + Δp)πr2−2πrτdl = 0 | (9) |
where r0 is the radius of the tube, r is the radius of the fluid unit, where r < r0. p and p+Δp are the pressure at both ends of the unit body, Δp is the slurry unit body pressure increment, dl is for the length of the unit body, and τ is the surface shear stress of the unit body.
The rheological equation of Newton fluid is
τ=μγ | (10) |
where μ is the viscosity coefficient, γ is the shear rate.
Substituting Eq (10) to Eq (9)
γ=−dvdr=τμ=−r2μdpdl | (11) |
Substitute boundary conditions
r=r0,v=0 | (12) |
Combining Eqs (11) and (12), the grout flow rate in the pipe is
vx(r)=−14μdpdl(r02−r2) | (13) |
The unit grouting volume is
q=∫r002πrvx(r)dr | (14) |
Combining Eq (13) to Eq (14),
q=−π408μdpdl | (15) |
Therefore, the average flow velocity of the slurry in the tube is
¯v=qπr02=−r028μdpdl | (16) |
Introducing permeability, defined as [36]
K=ϕr028 | (17) |
The seepage velocity of grout in the injected medium is
vp=¯vϕ=Kμ⋅(−dpdl) | (18) |
Therefore, the amount of grouting in time t is
Q=vp⋅A⋅t | (19) |
Substituting Eq (5) into Eq (19),
Q=vp⋅2πl(l+h)⋅t | (20) |
The relationship between hemispherical diffusion radius and cylindrical diffusion height is [14].
h=2l3(2a+1) | (21) |
where a is the number of grouting holes for the side wall of the grouting pipe.
Combining Eqs (18)-(21) the pressure gradient expression in the grouting process is obtained as
dpdl=−3Q2πl2(4a+5)⋅t⋅μK | (22) |
Substitute boundary conditions
l=l0,p=p0 | (23) |
where l0 is the Grouting tube radius, p0 is the grouting initial pressure.
Get
p=p0+3Qμ2πK(4a+5)⋅t⋅(l−1−l0−1) | (24) |
For column-hemispheric diffusion grout, the total grouting amount can be expressed as
Q=(23πl3+πl2h)⋅ϕ | (25) |
Combining Eq (7), the governing equation of the diffusion radius of Newtonian fluid seepage grouting considering the permeability change of porous media is obtained:
p=p0+32μ(a+1)3⋅K0λβϕ⋅(1−24−lnρδϕα2μt)(4a+5)⋅t⋅(l2−l0−1l3) | (26) |
where α = ((2/3l + h)l)/(l + h), δ = sqrt((6K0λ(1 - φ))/πφ2), t is the grouting time, l is the hemispherical region slurry diffusion radius during the time t, h is the cylindrical region slurry diffusion height, which satisfies the condition h = (2l/3(a2 + l), φ is the porosity of porous media, μ is the slurry viscosity, δ is the porous media pore diameter, ρ is the slurry density, l0 is the grouting hole radius, p0 is the grouting pressure, a is the grouting pipe side wall grouting number, λ = 3π, K0 is the initial permeability.
When the permeability change of porous media is not considered, Equation (26) can be transformed into Eq (27), which is equivalent to the control equation of Newtonian fluid seepage grouting column-hemispherical diffusion radius derived by Yang et al. [37].
p=p0+2μϕ(a+1)Kt(4a+5)⋅(l2−l0−1l3) | (27) |
where the symbolic meaning is the same as in Eq (26).
In order to study the relationship between the change of permeability of porous media and grouting pressure, this paper needs to calculate the mathematical equation between permeability of porous media and grouting pressure.
From Eq (8), the factors affecting the change of permeability are parameters such as grouting time t and diffusion distance l. Meanwhile, from Eq (15), it is known that the diffusion distance l has a function relationship with the grouting pressure p. Therefore, substituting Eq (25) into Eq (22)
dpdl=−64l(a+1)μ(4a+5)tδ2 | (28) |
Substituting Eq (22) boundary conditions into Eq (28)
l=√(p0−p)(4a+5)tδ232(a+1)μ | (29) |
where the meaning of the symbols is consistent with Eq (26).
Based on the mathematical expressions of permeability versus grouting time and grouting pressure, the relationship between permeability of porous media and grouting pressure and grouting time is investigated in this paper. Based on the relevant laboratory test study [13,14,15,17,23,37,38,40] the slurry parameters and medium parameters are selected, as shown in Table 1. When studying the influence of grouting time on permeability, the time range is 0-300 s, and three groups of control examples of grouting pressure 80, 100 and 120 KPa are set. When studying the influence of grouting pressure on permeability, the pressure range is selected as 0-600 KPa, and three groups of control examples of grouting time of 300, 600 and 900 s are set.
W/C | μ0/(Pa·s) | ρ/(g/cm3) | K0/(cm/s) | φ | r0/cm | τ0/Pa |
2.0 | 0.0067 | 1.30 | 0.0334 | 0.4 | 0.5 | 0.0971 |
Based on Eq (8), the relationship between the permeability of geotechnical porous media affected by slurry flow resistance and grouting time and grouting pressure is obtained, as shown in Figure 2.
It can be seen from Figure 3(a) that with the increase of grouting time, the permeability of geotechnical porous media decreases first and then increases. This is because the slurry flow rate is large at the moment of grouting, which has a great influence on the pore medium particles near the grouting hole, which is bound to cause a large number of medium particles to migrate, so that the permeability of porous media decreases greatly. With the increase of grouting time, the slurry pressure and flow rate gradually dissipate, and the influence on the surrounding medium particles decreases, so that the permeability of porous media gradually rises. It can be seen from Figure 3(b) that with the increase of grouting pressure, the permeability of geotechnical porous media decreases gradually. This is because the greater the grouting pressure, the greater the slurry diffusion range and the top pressure of the slurry at a certain grouting time, the greater the influence on the pore medium particles, and the more significant the influence on the permeability of the porous medium of the rock and soil, resulting in the gradual decrease of the permeability of the porous medium.
In order to verify the validity of the theory in this paper, the indoor test results in Reference 37 are compared with the theoretical results in this paper. In the test of Reference [37], three groups of ordinary Portland cement slurry with water cement ratio of 2.0, 5.0 and 10.0 were used to carry out three groups of different grouting pressure tests. The grouting test device is shown in Figure 4, which is composed of a pressure supply device, a slurry storage container and a model test box. The water of the cement slurry and the temperature of the test environment were both 20 ℃. According to Reference [38], it can be seen that the cement slurry of these three water cement ratios conforms to the properties of Newtonian fluid. The properties of the injected medium and grouting parameters are shown in Tables 2 and 3, and the rheological equation of cement slurry is shown in Table 4.
Group | Injection medium particle size distribution/mm | Porosity | Permeability coefficient/(cm/s) |
G1 | 1-3 | 0.3993 | 0.65 |
G2 | 3-5 | 0.4505 | 2.11 |
G3 | 5-10 | 0.5074 | 8.94 |
Based on Eqs (26) and (27), the diffusion radius of Newtonian fluid hemisphere considering the change of permeability of porous media and not considering the change of permeability of porous media is obtained. And the diffusion height of cylinder can also be obtained according to the diffusion radius of sphere part by Eq (20). And the theoretical diffusion radius and diffusion height, compared with the established literature indoor test values were analyzed, the results are shown in Figure 5.
Group | Water cement ratio | Grouting pressure/m | Grouting hole radius/cm | Grouting time/s |
G1 | 2.0 | 4.08 | 0.75 | 8 |
G2 | 5.0 | 6.12 | 0.75 | 6 |
G3 | 10.0 | 8.16 | 0.75 | 4 |
Water cement ratio | Rheological equation |
2.0 | τ = 0.0372 + 0.0047γ |
5.0 | τ = 0.0880 + 0.0027γ |
10.0 | τ = 0.0454 + 0.0019γ |
It can be seen from Figure 5 that the theoretical value considering the change of permeability is about 1.14 times of the experimental value, while the theoretical value without considering the change of permeability is about 1.60 times of the experimental value, indicating that the slurry diffusion length considering the change of permeability of geotechnical porous media is closer to the actual diffusion radius of Newtonian fluid infiltration grouting, thus verifying the validity of the theory in this paper. With the increase of water-cement ratio, the theoretical value considering the change of permeability is closer to the experimental value. This is because with the increase of water-cement ratio, the larger the slurry diffusion radius is, the greater the influence of slurry on the permeability of porous media is. It is more necessary to consider the change of permeability of porous media, so the theoretical results considering the change of permeability are closer to the experimental values.
In order to further verify the adaptability and reliability of the theory in this paper, based on the numerical simulation software COMSOL, the numerical simulation of Newtonian fluid seepage grouting column-hemisphere seepage diffusion with different grouting pressure heads is carried out, and the theoretical results are compared with the simulation results and test results to verify the effectiveness of the theory in this paper.
1) Model construction
The size of the model is 1000 mm × 1000 mm × 2000 mm, the grouting hole is located in the center, the radius of the orifice and the side grouting hole is 7.5 mm, the side grouting hole is set to 4, and the vertical interval is 20 mm (Figure 6). The pressure inlet boundary is selected, and the model boundary is a non-pressure boundary. The numerical simulation is based on the COMSOL two-phase Darcy's law module, the permeability change model function (Eq 8) derived in this paper is introduced through the built-in custom function of the software, so as to realize the simulation of diffusion of the infiltration column-hemisphere of porous media considering the permeability change. The grouting parameters and the parameters of the injected medium are set according to Tables 2-4.
2) Numerical results
In the numerical calculation, the surface Darcy velocity is used to represent the slurry diffusion range, that is, when the slurry diffusion velocity is 0, the slurry is no longer diffused [39]. Figure 7 shows the G3 group of Newtonian fluid cement slurry column-hemispherical diffusion model diagram. Figure 5 shows that the lower part of the slurry model diagram conforms to the hemispherical diffusion, and the upper part of the model diagram is not the standard cylindrical diffusion, but the diffusion form of the cylinder and the top semi-ellipsoid, which is different from the standard cylindrical diffusion. The simulation results are consistent with the indoor test results obtained by Yang et al. [40].
In order to verify the validity of the theoretical results, the numerical simulation values of the diffusion radius of the three groups of G1, G2 and G3 with different grouting pressure heads and the theoretical calculation values of the diffusion radius considering the permeability change of the porous medium are selected to compare with the experimental actual values. In order to make the comparison effect obvious, the middle section of the three-dimensional simulation diagram is selected. Since the upper cylindrical diffusion result is not a standard cylindrical shape, in order to better compare and verify the theoretical cylindrical diffusion results, this paper refers to Eq (30), that is, the equal product method adopted by Yang et al. [40] to calculate the equivalent height of cylindrical diffusion. The results are shown in Figure 8 and Table 5.
hd=√Vπl | (30) |
where hd is the cylindrical diffusion equivalent height; V the diffusion volume of Newtonian fluid stone can be obtained by calculation. l is a hemispherical diffusion radius, which can be measured.
Group | Hemisphere part diffusion radius/cm | Cylindrical part diffusion height (cm) | ||||
Simulated value | theoretical value | experimental value | Simulated value | theoretical value | experimental value | |
G1 | 16.32 | 14.39 | 11.8 | 50.12 | 47.98 | 39.33 |
G2 | 20.01 | 18.27 | 15.2 | 62.21 | 60.91 | 50.67 |
G3 | 21.58 | 20.98 | 18.4 | 70.37 | 69.93 | 61.33 |
It can be seen from Figure 6 and Table 5 that the theoretical calculation results are compared with the numerical simulation results. The radius difference of the hemisphere part is 2-10 %, and the height difference of the cylinder part is 1.5-4 %. It shows that the numerical simulation results of Newtonian fluid column-hemisphere diffusion inserted into the permeability change of porous media are basically consistent with the theoretical calculation results, which further verifies the validity of the theory in this paper. Both results are greater than the indoor test results, because this paper does not consider the filtration effect of slurry, diffusion path and other reasons.
Through the above analysis, it can be seen that there is still a difference between the theoretical analytical value and the numerical simulation value of the Newtonian fluid seepage grouting column-hemisphere diffusion radius considering the permeability change and the indoor test value. This is because this paper does not take into account the time-varying viscosity of the slurry, slurry diffusion path, slurry filtration effect and other effects on the slurry diffusion range.
Ruan et al. [38] obtained the relationship curve between slurry viscosity and time through laboratory test analysis. It can be seen that the slurry viscosity gradually increases with the increase of time, so the viscosity of the slurry in the diffusion process gradually increases. In this paper, the slurry viscosity value is certain, which leads to the theoretical value of the slurry diffusion radius in this paper is larger than the actual value of the slurry diffusion. Maghous et al. [41] and Fang et al. [20] theoretically analyzed that the cement slurry will produce cement particles to block the pores during the diffusion process of the porous medium, resulting in a decrease in the permeability of the porous medium. Because this article does not take into account the filtration effect of the cement slurry, the theoretical results are greater than the actual diffusion results. Bear et al. [32] and Lu et al. [15] proposed that the path of the slurry in the diffusion process is a curve rather than a straight line, and proved this view through theoretical analysis and laboratory tests. However, this paper does not consider the tortuosity in the slurry diffusion process, resulting in a deviation between the theoretical value and the actual value. Therefore, the influence of the above factors on the diffusion range of slurry infiltration grouting can be considered in the follow-up study.
In order to obtain the relationship between the diffusion range of Newtonian fluid seepage grouting column-hemisphere and grouting parameters considering the permeability change of porous media, the sensitivity analysis of slurry diffusion radius to grouting pressure and grouting time is carried out in this paper.
Based on the relevant literature research [15,16,17,23,37,38,39,40], the grouting pressure head range is 0-8 m, and the grouting time range is 0-10 min. The porosity is set to 0.4, the radius of grouting hole and side grouting hole is set to 15 mm, the side grouting hole is set to 4, the water cement ratio of slurry is 5.0, the viscosity of slurry is 0.0027 Pa·s, and the permeability coefficient is 0.6 cm/s.
At the same time, based on COMSOL numerical simulation software, numerical simulation tests with grouting pressure head of 0-8 m and grouting time of 0-10 min were carried out respectively. The numerical simulation results were compared with the theoretical calculation results to obtain the relationship between the diffusion radius of Newtonian fluid seepage grouting column-hemisphere and grouting time and grouting pressure. The parameter setting is the same as the above theoretical calculation parameters.
From Section 2.1, it can be seen that the change trend of diffusion radius and diffusion height of Newtonian fluid seepage grouting column-hemisphere is completely consistent, so this section only studies the sensitivity analysis of hemisphere diffusion radius. Based on the Eq (26), the relationship between the diffusion radius of Newtonian fluid cement slurry and the grouting pressure head and grouting time considering the permeability change of porous media is obtained, and the results are compared with the numerical simulation results. The comparison results are shown in Figures 9 and 10.
Figure 9 shows that with the increase of grouting pressure head, the slurry diffusion range gradually increases. At low grouting pressure, the slurry diffusion radius increases rapidly, from 0 to 1 m is the most obvious; with the further increase of grouting pressure, the rate gradually decreases. This is because the greater the grouting pressure, the greater the diffusion radius of the slurry, the greater the influence range on the permeability of the porous medium, resulting in a decrease in the rate of increase in the diffusion radius.
It can be seen from Figure 10 that with the increase of grouting time, the slurry diffusion radius increases rapidly at first and then increases slowly. This is because the slurry increases rapidly at the initial stage of grouting, but with the increase of grouting time, the slurry pressure gradually dissipates, so the diffusion rate gradually decreases.
In this paper, the influence of slurry flow resistance on the permeability of geotechnical porous media is introduced. Combined with the Newtonian fluid seepage grouting column-hemispherical diffusion law, the permeability change model of geotechnical porous media is obtained. Combined with the rheological equation and equilibrium equation of Newtonian fluid, a seepage grouting diffusion model considering the influence of slurry flow resistance on the permeability of porous media is established. The theoretical results are compared with the existing literature results and numerical simulation results to verify the effectiveness of the theoretical mechanism. At the same time, the parameter analysis of the diffusion radius of Newtonian fluid seepage grouting considering the permeability change of porous media is carried out. The following conclusions are obtained:
1) The influence of cement slurry flow resistance on the permeability of porous media in rock and soil during grouting is introduced. The analytical expression of the permeability of porous media with grouting construction parameters, slurry diffusion radius and pore characteristics of porous media is established.
2) The comparison between the theoretical results of this paper and the experimental results of the existing literature shows that the theoretical results considering the change of permeability are about 1.14 times of the experimental results, and the theoretical results without considering the change of permeability are about 1.6 times of the experimental results. At the same time, based on COMSOL numerical simulation software, the permeability change function of porous media is introduced, and the numerical simulation results are basically consistent with the theoretical results, which verifies the effectiveness of the theoretical mechanism in this paper.
3) The parametric analysis can be obtained that with the increase of grouting pressure and grouting time, the diffusion radius of Newtonian fluid infiltration grouting considering the change of permeability of the porous medium increases rapidly at first, and then the rate of increase becomes smaller gradually.
The authors declare they have not used Artificial Intelligence (AI) tools in the creation of this article.
This work was supported by the Scientific and Technological Research and Development Project of China Railway Guangzhou Bureau Co. Ltd. (No.2020K033-G), the Scientific and Technological Progress and Innovation Project of Hunan Provincial Department of Transportation (Nos. 202017; 202115), and the Fundamental Research Funds for the Central Universities of Central South University (No. 2023ZZTS0341). All financial supports are greatly appreciated.
The authors declare there is no conflict of interest.
[1] |
O. Nave, Analysis of the two-dimensional polydisperse liquid sprays in a laminar boundary layer flow using the similarity transformation method, Adv. Model. and Simul. in Eng. Sci., 2 (2015), 20. https://doi.org/10.1186/s40323-015-0042-8 doi: 10.1186/s40323-015-0042-8
![]() |
[2] |
A. Lozynskyy, A. Chaban, T. Perzynski, A. Szafraniec, L. Kasha, Application of fractional-order calculus to improve the mathematical model of a two-mass system with a long shaft, Energies, 14 (2021), 1854. https://doi.org/10.3390/en14071854 doi: 10.3390/en14071854
![]() |
[3] |
M. I. Asjad, R. Ali, A. Iqbal, T. Muhammad, Y. M. Chu, Application of water based drilling clay-nanoparticles in heat transfer of fractional maxwell fluid over an infinite flat surface, Sci. Rep., 11 (2021), 18833. https://doi.org/10.1038/s41598-021-98066-w doi: 10.1038/s41598-021-98066-w
![]() |
[4] |
D. Baleanu, A. Fernandez, A. Akgl, On a fractional operator combining proportional and classical differintegrals, Mathematics, 8 (2020), 360. https://doi.org/10.3390/math8030360 doi: 10.3390/math8030360
![]() |
[5] |
G. Liu, S. Li, J. Wang, New green-ampt model based on fractional derivative and its application in 3d slope stability analysis, J. Hydrol., 603 (2021), 127084. https://doi.org/10.1016/j.jhydrol.2021.127084 doi: 10.1016/j.jhydrol.2021.127084
![]() |
[6] |
P. Kumar, V. S. Erturk, R. Banerjee, M. Yavuz, V. Govindaraj, Fractional modeling of plankton-oxygen dynamics under climate change by the application of a recent numerical algorithm, Phys. Scr., 96 (2021), 124044. https://doi.org/10.1088/1402-4896/ac2da7 doi: 10.1088/1402-4896/ac2da7
![]() |
[7] |
M. Inc, B. Acay, H. W. Berhe, A. Yusuf, A. Khan, S. W. Yao, Analysis of novel fractional COVID-19 model with real-life data application, Results Phys., 23 (2021), 103968. https://doi.org/10.1016/j.rinp.2021.103968 doi: 10.1016/j.rinp.2021.103968
![]() |
[8] |
W. Y. Shen, Y. M. Chu, M. ur Rahman, I. Mahariq, A. Zeb, Mathematical analysis of hbv and hcv co-infection model under nonsingular fractional order derivative, Results Phys., 28 (2021), 104582. https://doi.org/10.1016/j.rinp.2021.104582 doi: 10.1016/j.rinp.2021.104582
![]() |
[9] | I. Podlubny, Fractional differential equations: an introduction to fractional derivatives, fractional differential equations, to methods of their solution and some of their applications, New York: Academic Press, 1999. |
[10] | A. A. Kilbas, H. M. Srivastava, J. J. Trujillo, Theory and applications of fractional differential equations, Amsterdam: Elsevier Science, 2006. |
[11] | C. Li, F. Zeng, Numerical methods for fractional calculus, Boca Raton: CRC Press, 2015. |
[12] |
G. S. Teodoro, J. A. T. Machado, E. C. de Oliveira, A review of definitions of fractional derivatives and other operators, J. Comput. Phys., 388 (2019), 195–208. https://doi.org/10.1016/j.jcp.2019.03.008 doi: 10.1016/j.jcp.2019.03.008
![]() |
[13] |
M. D. Ortigueira, J. A. T. Machado, What is a fractional derivative, J. Comput. Phys., 293 (2015), 4–13. https://doi.org/10.1016/j.jcp.2014.07.019 doi: 10.1016/j.jcp.2014.07.019
![]() |
[14] |
V. E. Tarasov, No nonlocality. no fractional derivative, Commun. Nonlinear Sci., 62 (2018), 157–163. https://doi.org/10.1016/j.cnsns.2018.02.019 doi: 10.1016/j.cnsns.2018.02.019
![]() |
[15] |
H. G. Sun, W. Chen, H. Wei, Y. Q. Chen, A comparative study of constant-order and variable-order fractional models in characterizing memory property of systems, Eur. Phys. J. Spec. Top., 193 (2011), 185–192. https://doi.org/10.1140/epjst/e2011-01390-6 doi: 10.1140/epjst/e2011-01390-6
![]() |
[16] |
S. G. Samko, B. Ross, Integration and differentiation to a variable fractional order, Integr. Transf. Spec. F., 1 (1993), 277–300. https://doi.org/10.1080/10652469308819027 doi: 10.1080/10652469308819027
![]() |
[17] |
C. F. Lorenzo, T. T. Hartley, Initialization, conceptualization, and application in the generalized (fractional) calculus, Crit. Rev. Biomed. Eng., 35 (2007), 447–553. https://doi.org/10.1615/CritRevBiomedEng.v35.i6.10 doi: 10.1615/CritRevBiomedEng.v35.i6.10
![]() |
[18] |
C. F. Lorenzo, T. T. Hartley, Variable order and distributed order fractional operators, Nonlinear Dynamics, 29 (2002), 57–98. https://doi.org/10.1023/A:1016586905654 doi: 10.1023/A:1016586905654
![]() |
[19] |
C. F. Coimbra, Mechanics with variable-order differential operators, Ann. Phys., 515 (2003), 692–703. https://doi.org/10.1002/andp.200351511-1203 doi: 10.1002/andp.200351511-1203
![]() |
[20] |
X. Zheng, H. Wang, Optimal-order error estimates of finite element approximations to variable-order time-fractional diffusion equations without regularity assumptions of the true solution, IMA J. Numer. Anal., 41 (2021), 1522–1545. https://doi.org/10.1093/imanum/draa013 doi: 10.1093/imanum/draa013
![]() |
[21] |
P. Pandey, J. F. Gomez-Aguilar, On solution of a class of nonlinear variable order fractional reaction-diffusion equation with mittag-effler kernel, Numer. Meth. Part. D. E., 37 (2021), 998–1011. https://doi.org/10.1002/num.22563 doi: 10.1002/num.22563
![]() |
[22] |
M. Hosseininia, M. H. Heydari, F. M. M. Ghaini, A numerical method for variable-order fractional version of the coupled 2d burgers equations by the 2D chelyshkov polynomials, Math. Method. Appl. Sci., 44 (2021), 6482–6499. https://doi.org/10.1002/mma.7199 doi: 10.1002/mma.7199
![]() |
[23] |
H. Hassani, Z. Avazzadeh, J. A. T. Machado, Numerical approach for solving variable-order space-time fractional telegraph equation using transcendental Bernstein series, Engineering with Computers, 36 (2020), 867–878. https://doi.org/10.1007/s00366-019-00736-x doi: 10.1007/s00366-019-00736-x
![]() |
[24] |
K. Sadri, H. Aminikhah, An efficient numerical method for solving a class of variable-order fractional mobile-immobile advection-dispersion equations and its convergence analysis, Chaos Soliton. Fract., 146 (2021), 110896. https://doi.org/10.1016/j.chaos.2021.110896 doi: 10.1016/j.chaos.2021.110896
![]() |
[25] |
M. Hosseininia, M. H. Heydari, C. Cattani, A wavelet method for non-linear variable-order time fractional 2D Schrodinger equation, Discrete Cont. Dyn.-S, 14 (2021), 2273–2295. https://doi.org/10.3934/dcdss.2020295 doi: 10.3934/dcdss.2020295
![]() |
[26] |
M. D. Ortigueira, D. Valerio, J. T. Machado, Variable order fractional systems, Commun. Nonlinear Sci., 71 (2019), 231–243. https://doi.org/10.1016/j.cnsns.2018.12.003 doi: 10.1016/j.cnsns.2018.12.003
![]() |
[27] |
H. Wang, X. Zheng, Wellposedness and regularity of the variable-order time-fractional diffusion equations, J. Math. Anal. Appl., 475 (2019), 1778–1802. https://doi.org/10.1016/j.jmaa.2019.03.052 doi: 10.1016/j.jmaa.2019.03.052
![]() |
[28] | R. Almeida, D. Tavares, D. F. M. Torres, The variable-order fractional calculus of variations, Heidelberg: Springer, 2019. https://doi.org/10.1007/978-3-319-94006-9 |
[29] |
N. H. Sweilam, S. M. Al-Mekhlafi, A. O. Albalawi, J. A. T. Machado, Optimal control of variable-order fractional model for delay cancer treatments, Appl. Math. Model., 89 (2021), 1557–1574. https://doi.org/10.1016/j.apm.2020.08.012 doi: 10.1016/j.apm.2020.08.012
![]() |
[30] |
G. Xiang, D. Yin, R. Meng, C. Cao, Predictive model for stress relaxation behavior of glassy polymers based on variable-order fractional calculus, Polym. Advan. Technol., 32 (2021), 703–713. https://doi.org/10.1002/pat.5123 doi: 10.1002/pat.5123
![]() |
[31] |
X. Liu, D. Li, C. Han, Y. Shao, A Caputo variable-order fractional damage creep model for sandstone considering effect of relaxation time, Acta Geotech., 17 (2022), 153–167. https://doi.org/10.1007/s11440-021-01230-9 doi: 10.1007/s11440-021-01230-9
![]() |
[32] |
W. Fei, L. Jie, Z. Quanle, L. Cunbao, C. Jie, G. Renbo, A triaxial creep model for salt rocks based on variable-order fractional derivative, Mech. Time-Depend. Mater., 25 (2021), 101–118. https://doi.org/10.1007/s11043-020-09470-0 doi: 10.1007/s11043-020-09470-0
![]() |
[33] |
H. Jahanshahi, S. S. Sajjadi, S. Bekiros, A. A. Aly, On the development of variable-order fractional hyperchaotic economic system with a nonlinear model predictive controller, Chaos Soliton. Fract., 144 (2021), 110698. https://doi.org/10.1016/j.chaos.2021.110698 doi: 10.1016/j.chaos.2021.110698
![]() |
[34] | J. Fan, T. Gu, P. Wang, W. Cai, X. Fan, G. Zhang, Constitutive modeling of sintered nano-silver particles: A variable-order fractional model versus an anand model, 2021 22nd International Conference on Thermal, Mechanical and Multi-Physics Simulation and Experiments in Microelectronics and Microsystems (EuroSimE), St. Julian, Malta, 2021, 1–4 https://doi.org/10.1109/EuroSimE52062.2021.9410807 |
[35] |
S. Patnaik, J. P. Hollkamp, F. Semperlotti, Applications of variable-order fractional operators: a review, P. Royal Soc. A-Math. Phy., 476 (2020), 20190498. https://doi.org/10.1098/rspa.2019.0498 doi: 10.1098/rspa.2019.0498
![]() |
[36] |
H. Sun, A. Chang, Y. Zhang, W. Chen, A review on variable-order fractional differential equations: mathematical foundations, physical models, numerical methods and applications, Fract. Calc. Appl. Anal., 22 (2019), 27–59. https://doi.org/10.1515/fca-2019-0003 doi: 10.1515/fca-2019-0003
![]() |
[37] |
M. R. S. Ammi, I. Jamiai, D. F. Torres, Finite element approximation for a class of Caputo time-fractional diffusion equations, Comput. Math. Appl., 78 (2019), 1334–1344. https://doi.org/10.1016/j.camwa.2019.05.031 doi: 10.1016/j.camwa.2019.05.031
![]() |
[38] |
B. Li, H. Luo, X. Xie, Analysis of a time-stepping scheme for time fractional diffusion problems with nonsmooth data, SIAM J. Numer. Anal., 57 (2019), 779–798. https://doi.org/10.1137/18M118414X doi: 10.1137/18M118414X
![]() |
[39] |
U. Ali, M. Sohail, M. Usman, F. A. Abdullah, I. Khan, K. S. Nisar, Fourth-order difference approximation for time-fractional modified sub-diffusion equation, Symmetry, 12 (2020), 691. https://doi.org/10.3390/sym12050691 doi: 10.3390/sym12050691
![]() |
[40] |
N. H. Tuan, Y. E. Aghdam, H. Jafari, H. Mesgarani, A novel numerical manner for two‐dimensional space fractional diffusion equation arising in transport phenomena, Numer. Meth. Part. D. E., 37 (2021), 1397–1406. https://doi.org/10.1002/num.22586 doi: 10.1002/num.22586
![]() |
[41] |
F. M. Salama, N. H. M. Ali, Computationally efficient hybrid method for the numerical solution of the 2D time fractional advection-diffusion equation, Int. J. Math. Eng. Manag., 5 (2020), 432–446. https://doi.org/10.33889/IJMEMS.2020.5.3.036 doi: 10.33889/IJMEMS.2020.5.3.036
![]() |
[42] |
N. Dhiman, M. Huntul, M. Tamsir, A modified trigonometric cubic b-spline collocation technique for solving the time-fractional diffusion equation, Eng. Computation., 38 (2021), 2921–2936. https://doi.org/10.1108/EC-06-2020-0327 doi: 10.1108/EC-06-2020-0327
![]() |
[43] |
F. M. Salama, N. H. M. Ali, N. N. A. Hamid, Fast O(N) hybrid Laplace transform-finite difference method in solving 2D time fractional diffusion equation, J. Math. Comput. Sci., 23 (2021), 110–123. https://doi.org/10.22436/jmcs.023.02.04 doi: 10.22436/jmcs.023.02.04
![]() |
[44] |
F. M. Salama, N. N. A. Hamid, N. H. M. Ali, U. Ali, An efficient modified hybrid explicit group iterative method for the time-fractional diffusion equation in two space dimension, AIMS Mathematics, 7 (2022), 2370–2392. https://doi.org/10.3934/math.2022134 doi: 10.3934/math.2022134
![]() |
[45] |
F. M. Salama, N. N. A. Hamid, U. Ali, N. H. M. Ali, Fast hybrid explicit group methods for solving 2d fractional advection-diffusion equation, AIMS Mathematics, 7 (2022), 15854–15880. https://doi.org/10.3934/math.2022868 doi: 10.3934/math.2022868
![]() |
[46] |
M. A. Khan, N. Alias, U. Ali, A new fourth-order grouping iterative method for the time fractional sub-diffusion equation having a weak singularity at initial time, AIMS Mathematics, 8 (2023), 3725–13746. https://doi.org/10.3934/math.2023697 doi: 10.3934/math.2023697
![]() |
[47] |
U. Ali, M. Sohail, F. A. Abdullah, An efficient numerical scheme for variable-order fractional sub-diffusion equation, Symmetry, 12 (2020), 1437. https://doi.org/10.3390/sym12091437 doi: 10.3390/sym12091437
![]() |
[48] |
F. R. Lin, Q. Y. Wang, X. Q. Jin, Crank-Nicolson-Weighted-Shifted-Grunwald-difference schemes for space riesz variable-order fractional diffusion equations, Numer. Algor., 87 (2021), 601–631. https://doi.org/10.1007/s11075-020-00980-z doi: 10.1007/s11075-020-00980-z
![]() |
[49] |
U. Ali, M. Naeem, F. A. Abdullah, M. K. Wang, F. M. Salama, Analysis and implementation of numerical scheme for the variable-order fractional modified sub-diffusion equation, Fractals, 30 (2022), 2240253. https://doi.org/10.1142/S0218348X22402538 doi: 10.1142/S0218348X22402538
![]() |
[50] |
J. Jia, H. Wang, X. Zheng, A preconditioned fast finite element approximation to variable-order time-fractional diffusion equations in multiple space dimension, Appl. Numer. Math., 163 (2021), 15–29. https://doi.org/10.1016/j.apnum.2021.01.001 doi: 10.1016/j.apnum.2021.01.001
![]() |
[51] |
M. H. Heydari, Z. Avazzadeh, M. F. Haromi, A wavelet approach for solving multi-term variable-order time fractional diffusion-wave equation, Appl. Math. Comput., 341 (2019), 215–228. https://doi.org/10.1016/j.amc.2018.08.034 doi: 10.1016/j.amc.2018.08.034
![]() |
[52] |
S. Wei, W. Chen, Y. Zhang, H. Wei, R. M. Garrard, A local radial basis function collocation method to solve the variable-order time fractional diffusion equation in a two-dimensional irregular domain, Numer. Meth. Part. D. E., 34 (2018), 1209–1223. https://doi.org/10.1002/num.22253 doi: 10.1002/num.22253
![]() |
[53] |
J. Jia, H. Wang, X. Zheng, A fast collocation approximation to a two-sided variable-order space-fractional diffusion equation and its analysis, J. Comput. Appl. Math., 388 (2021), 13234. https://doi.org/10.1016/j.cam.2020.113234 doi: 10.1016/j.cam.2020.113234
![]() |
[54] |
X. Y. Li, B. Y. Wu, Iterative reproducing kernel method for nonlinear variable-order space fractional diffusion equations, Int. J. Comput. Math., 95 (2018), 1210–1221. https://doi.org/10.1080/00207160.2017.1398325 doi: 10.1080/00207160.2017.1398325
![]() |
[55] |
F. M. Salama, N. H. M. Ali, N. N. A. Hamid, Efficient hybrid group iterative methods in the solution of two-dimensional time fractional cable equation, Adv. Differ. Equ., 2020 (2020), 257. https://doi.org/10.1186/s13662-020-02717-7 doi: 10.1186/s13662-020-02717-7
![]() |
[56] |
M. A. Khan, N. H. M. Ali, N. N. A. Hamid, A new fourth-order explicit group method in the solution of two-dimensional fractional rayleigh-stokes problem for a heated generalized second-grade fluid, Adv. Differ. Equ., 2020 (2020), 598. https://doi.org/10.1186/s13662-020-03061-6 doi: 10.1186/s13662-020-03061-6
![]() |
[57] |
A. Ali, T. Abdeljawad, A. Iqbal, T. Akram, M. Abbas, On unconditionally stable new modified fractional group iterative scheme for the solution of 2D time-fractional telegraph model, Symmetry, 13 (2021), 2078. https://doi.org/10.3390/sym13112078 doi: 10.3390/sym13112078
![]() |
[58] |
F. M. Salama, A. T. Balasim, U. Ali, M. A. Khan, Efficient numerical simulations based on an explicit group approach for the time fractional advection-diffusion reaction equation, Comput. Appl. Math., 42 (2023), 157. https://doi.org/10.1007/s40314-023-02278-x doi: 10.1007/s40314-023-02278-x
![]() |
[59] |
N. Abdi, H. Aminikhah, A. H. R. Sheikhani, On rotated grid point iterative method for solving 2d fractional reaction-subdiffusion equation with Caputo-Fabrizio operator, J. Differ. Equ. Appl., 27 (2021), 1134–1160. https://doi.org/10.1080/10236198.2021.1965592 doi: 10.1080/10236198.2021.1965592
![]() |
[60] |
F. M. Salama, U. Ali, A. Ali, Numerical solution of two-dimensional time fractional mobile/immobile equation using explicit group methods, Int. J. Appl. Comput. Math., 8 (2022), 188. https://doi.org/10.1007/s40819-022-01408-z doi: 10.1007/s40819-022-01408-z
![]() |
[61] |
Z. Liu, X. Li, A Crank-Nicolson difference scheme for the time variable fractional mobile-immobile advection-dispersion equation, J. Appl. Math. Comput., 56 (2018), 391–410. https://doi.org/10.1007/s12190-016-1079-7 doi: 10.1007/s12190-016-1079-7
![]() |
[62] |
A. R. Abdullah, The four point explicit decoupled group (EDG) method: A fast Poisson solver, Int. J. Comput. Math., 38 (1991), 61–70. https://doi.org/10.1080/00207169108803958 doi: 10.1080/00207169108803958
![]() |
[63] |
X. M. Gu, H. W. Sun, Y. L. Zhao, X. Zheng, An implicit difference scheme for time-fractional diffusion equations with a time-invariant type variable order, Appl. Math. Lett., 120 (2021), 107270. https://doi.org/10.1016/j.aml.2021.107270 doi: 10.1016/j.aml.2021.107270
![]() |
W/C | μ0/(Pa·s) | ρ/(g/cm3) | K0/(cm/s) | φ | r0/cm | τ0/Pa |
2.0 | 0.0067 | 1.30 | 0.0334 | 0.4 | 0.5 | 0.0971 |
Group | Injection medium particle size distribution/mm | Porosity | Permeability coefficient/(cm/s) |
G1 | 1-3 | 0.3993 | 0.65 |
G2 | 3-5 | 0.4505 | 2.11 |
G3 | 5-10 | 0.5074 | 8.94 |
Group | Water cement ratio | Grouting pressure/m | Grouting hole radius/cm | Grouting time/s |
G1 | 2.0 | 4.08 | 0.75 | 8 |
G2 | 5.0 | 6.12 | 0.75 | 6 |
G3 | 10.0 | 8.16 | 0.75 | 4 |
Water cement ratio | Rheological equation |
2.0 | τ = 0.0372 + 0.0047γ |
5.0 | τ = 0.0880 + 0.0027γ |
10.0 | τ = 0.0454 + 0.0019γ |
Group | Hemisphere part diffusion radius/cm | Cylindrical part diffusion height (cm) | ||||
Simulated value | theoretical value | experimental value | Simulated value | theoretical value | experimental value | |
G1 | 16.32 | 14.39 | 11.8 | 50.12 | 47.98 | 39.33 |
G2 | 20.01 | 18.27 | 15.2 | 62.21 | 60.91 | 50.67 |
G3 | 21.58 | 20.98 | 18.4 | 70.37 | 69.93 | 61.33 |
W/C | μ0/(Pa·s) | ρ/(g/cm3) | K0/(cm/s) | φ | r0/cm | τ0/Pa |
2.0 | 0.0067 | 1.30 | 0.0334 | 0.4 | 0.5 | 0.0971 |
Group | Injection medium particle size distribution/mm | Porosity | Permeability coefficient/(cm/s) |
G1 | 1-3 | 0.3993 | 0.65 |
G2 | 3-5 | 0.4505 | 2.11 |
G3 | 5-10 | 0.5074 | 8.94 |
Group | Water cement ratio | Grouting pressure/m | Grouting hole radius/cm | Grouting time/s |
G1 | 2.0 | 4.08 | 0.75 | 8 |
G2 | 5.0 | 6.12 | 0.75 | 6 |
G3 | 10.0 | 8.16 | 0.75 | 4 |
Water cement ratio | Rheological equation |
2.0 | τ = 0.0372 + 0.0047γ |
5.0 | τ = 0.0880 + 0.0027γ |
10.0 | τ = 0.0454 + 0.0019γ |
Group | Hemisphere part diffusion radius/cm | Cylindrical part diffusion height (cm) | ||||
Simulated value | theoretical value | experimental value | Simulated value | theoretical value | experimental value | |
G1 | 16.32 | 14.39 | 11.8 | 50.12 | 47.98 | 39.33 |
G2 | 20.01 | 18.27 | 15.2 | 62.21 | 60.91 | 50.67 |
G3 | 21.58 | 20.98 | 18.4 | 70.37 | 69.93 | 61.33 |