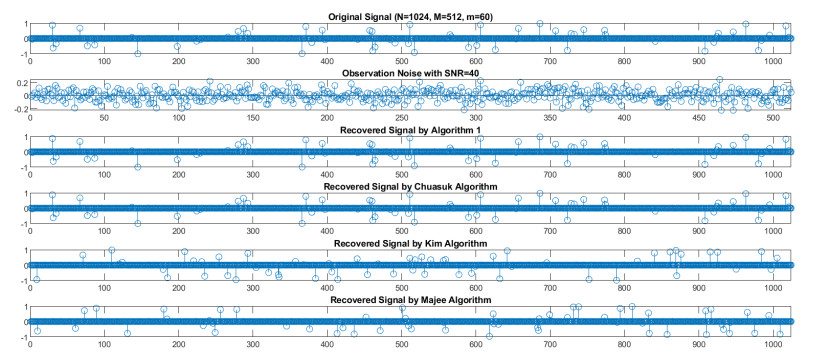
In this study, we establish a new inertial generalized viscosity approximation method and prove that the resulting sequence strongly converges to a common solution of a split generalized mixed equilibrium problem, fixed point problem for a finite family of nonexpansive mappings and hierarchical fixed point problem in real Hilbert spaces. As an application, we demonstrate the use of our main finding in compressed sensing in signal processing. Additionally, we include numerical examples to evaluate the efficiency of the suggested method and then conduct a comparative analysis of its efficiency with different methods. Our findings can be used in a variety of contexts to improve results.
Citation: Charu Batra, Renu Chugh, Mohammad Sajid, Nishu Gupta, Rajeev Kumar. Generalized viscosity approximation method for solving split generalized mixed equilibrium problem with application to compressed sensing[J]. AIMS Mathematics, 2024, 9(1): 1718-1754. doi: 10.3934/math.2024084
[1] | Yasir Arfat, Muhammad Aqeel Ahmad Khan, Poom Kumam, Wiyada Kumam, Kanokwan Sitthithakerngkiet . Iterative solutions via some variants of extragradient approximants in Hilbert spaces. AIMS Mathematics, 2022, 7(8): 13910-13926. doi: 10.3934/math.2022768 |
[2] | James Abah Ugboh, Joseph Oboyi, Hossam A. Nabwey, Christiana Friday Igiri, Francis Akutsah, Ojen Kumar Narain . Double inertial extrapolations method for solving split generalized equilibrium, fixed point and variational inequity problems. AIMS Mathematics, 2024, 9(4): 10416-10445. doi: 10.3934/math.2024509 |
[3] | Lu-Chuan Ceng, Shih-Hsin Chen, Yeong-Cheng Liou, Tzu-Chien Yin . Modified inertial subgradient extragradient algorithms for generalized equilibria systems with constraints of variational inequalities and fixed points. AIMS Mathematics, 2024, 9(6): 13819-13842. doi: 10.3934/math.2024672 |
[4] | Bancha Panyanak, Chainarong Khunpanuk, Nattawut Pholasa, Nuttapol Pakkaranang . A novel class of forward-backward explicit iterative algorithms using inertial techniques to solve variational inequality problems with quasi-monotone operators. AIMS Mathematics, 2023, 8(4): 9692-9715. doi: 10.3934/math.2023489 |
[5] | Habib ur Rehman, Poom Kumam, Kanokwan Sitthithakerngkiet . Viscosity-type method for solving pseudomonotone equilibrium problems in a real Hilbert space with applications. AIMS Mathematics, 2021, 6(2): 1538-1560. doi: 10.3934/math.2021093 |
[6] | Yali Zhao, Qixin Dong, Xiaoqing Huang . A self-adaptive viscosity-type inertial algorithm for common solutions of generalized split variational inclusion and paramonotone equilibrium problem. AIMS Mathematics, 2025, 10(2): 4504-4523. doi: 10.3934/math.2025208 |
[7] | Yajun Xie, Changfeng Ma . The hybird methods of projection-splitting for solving tensor split feasibility problem. AIMS Mathematics, 2023, 8(9): 20597-20611. doi: 10.3934/math.20231050 |
[8] | Jamilu Abubakar, Poom Kumam, Jitsupa Deepho . Multistep hybrid viscosity method for split monotone variational inclusion and fixed point problems in Hilbert spaces. AIMS Mathematics, 2020, 5(6): 5969-5992. doi: 10.3934/math.2020382 |
[9] | Junaid Ahmad, Kifayat Ullah, Reny George . Numerical algorithms for solutions of nonlinear problems in some distance spaces. AIMS Mathematics, 2023, 8(4): 8460-8477. doi: 10.3934/math.2023426 |
[10] | Mohammad Dilshad, Aysha Khan, Mohammad Akram . Splitting type viscosity methods for inclusion and fixed point problems on Hadamard manifolds. AIMS Mathematics, 2021, 6(5): 5205-5221. doi: 10.3934/math.2021309 |
In this study, we establish a new inertial generalized viscosity approximation method and prove that the resulting sequence strongly converges to a common solution of a split generalized mixed equilibrium problem, fixed point problem for a finite family of nonexpansive mappings and hierarchical fixed point problem in real Hilbert spaces. As an application, we demonstrate the use of our main finding in compressed sensing in signal processing. Additionally, we include numerical examples to evaluate the efficiency of the suggested method and then conduct a comparative analysis of its efficiency with different methods. Our findings can be used in a variety of contexts to improve results.
Consider H a Hilbert space and Q a nonempty, closed and convex subset of H. Let F:Q×Q→R be a bifunction, g:Q→H a nonlinear mapping and ψ:Q→R a function. Then, the generalized mixed equilibrium problem (GMEP) identifies ξ∈Q such that
F(ξ,z)+⟨gξ,z−ξ⟩+ψ(z)−ψ(ξ)≥0 for all z∈Q. | (1.1) |
If g=0, Problem (1.1) becomes a mixed equilibrium problem to identify ξ∈Q such that
F(ξ,z)+ψ(z)−ψ(ξ)≥0 for all z∈Q. | (1.2) |
If ψ=0, Problem (1.2) becomes a mixed equilibrium problem (MEP), which is to identify ξ∈Q such that
F(ξ,z)≥0for allz∈Q. | (1.3) |
If F(t,z)=0 for all t,z∈Q, Problem (1.1) becomes a generalized vector variational inequality problem which identifying ξ∈Q such that
⟨gξ,z−ξ⟩+ψ(z)−ψ(ξ)≥0for allz∈Q. | (1.4) |
Censor et al. [1] proposed the split feasibility problem (SFP) for modeling inverse problems for the first time in 1994. SFPs are used in various applications, including signal processing, image restoration, computer tomography, intensity-modulated radiation therapy (IMRT) and so on; see [2]. SFP involves the use of a bounded linear operator for identifying a point in a nonempty closed and convex set in the space whose image corresponds to another nonempty closed and convex set in the image space.
Suppose that H1 and H2 are Hilbert spaces and Q1 and Q2 are nonempty, closed and convex subsets of H1 and H2, respectively. Suppose that D:H1→H2 is a bounded linear operator. Let F1:Q1×Q1→R, F2:Q2×Q2→R be bifunctions, g1:Q1→H1, g2:Q2→H2 be nonlinear mappings and ψ1:Q1→R, ψ2:Q2→R be functions. Then, the split generalized mixed equilibrium problem (SGMEP), which involves finding ξ∈Q1 such that
F1(ξ,z)+⟨g1ξ,z−ξ⟩+ψ1(z)−ψ1(ξ)≥0for allz∈Q1, | (1.5) |
and w∗=Dξ∈Q2 solve
F2(w∗,w)+⟨g2w∗,w−w∗⟩+ψ2(w)−ψ2(w∗)≥0for allw∈Q2. | (1.6) |
Let the solution set of (1.5), (1.6) and SGMEP be denoted by GMEP(F1,g1,ψ1,Q1), GMEP(F2,g2,ψ2,Q2) and Θ, respectively.
Fan [3] was the first to introduce the equilibrium problem in 1972, but Blum and Oettli [4] made the most significant contributions to the issue in 1994. They studied variational principles and existence theorems for equilibrium problems, which have a significant role on the establishment of numerous domains in both pure and applied sciences; see [5,6]. These equilibrium problems serve as generalizations of various mathematical problems, including Nash equilibrium, optimization, variational inequality, minimization, saddle point problems and so on. Equilibrium problems have several applications in image reconstruction, networks, engineering, physics, game theory, economics, transportation and elasticity. As a result, the equilibrium problem has been expanded to broader issues in various ways.
GMEP was introduced by Peng and Yao [7] in 2008 and it includes the variational inequality problem (VIP), minimization problem (MP), fixed point problem (FPP) and many more as its special cases, see [8,9]. SGMEP includes split monotone variational inclusion problem (SMVIP), generalized mixed equilibrium problem (GMEP), mixed equilibrium problem (MEP), equilibrium problem (EP), variational inequality (Ⅵ), minimization problem, mixed variational inequality (MVI), split mixed equilibrium problem (SMEP), split generalized equilibrium problem (SGEP), split variational inequality (SVI), split minimization problem, split feasibility problem (SFP), split equilibrium problem (SEP) and many more as its special cases, see [10,11].
Moudafi and Mainge [12] initiated the hierarchical fixed point problem (HFPP) for a nonexpansive mapping S related to another nonexpansive mapping U on Q1, which can be defined as finding ξ∈ Fix(U), such that
⟨ξ−Sξ,ξ−w⟩≤0for allw∈Fix(U). | (1.7) |
Let Ω represent the solution set of HFPP. Using the normal cone's definition
NFix(S)={t∈H1:⟨ˉr−ˉp,t⟩,for allˉr∈Fix(S)ifˉp∈Fix(S),ϕotherwise, | (1.8) |
one can easily see that ξ∈ Fix(U) satisfies a VIP by using a criterion S, namely: Identify ξ∈ Fix(U) and
0∈(I−S)ξ+NFix(S)ξ. | (1.9) |
The HFPP (1.7) is clearly identical to the problem of identifying the fixed point of a map G=PFix(U)∘S, see [12], which includes monotone problems over equilibrium constraints, monotone variational inequality problems, and many more; see [13] and references therein.
The following mapping was described by Kangtunyakarn and Suantai [14] in 2009 as
Tn,0=ITn,1=ηn,1S1Tn,0+(1−ηn,1)ITn,2=ηn,2S2Tn,1+(1−ηn,2)Tn,1⋮ | (1.10) |
Tn,M−1=ηn,M−1SM−1Tn,M−2+(1−ηn,M−1)Tn,M−2Kn=Tn,M=ηn,MSMTn,M−1+(1−ηn,M)Tn,M−1, | (1.11) |
where Sj:Q1→Q1 represents a finite collection of nonexpansive mappings, {ηn,j}Mj=1⊂(0,1] with ηn,j→ηj and ∑+∞n=0|ηn,j−ηn−1,j|<+∞ for 1≤j≤M. The mapping Kn is the K-mapping generated by S1,S2,...,SM and ηn,1,ηn,2,...,ηn,M.
Recently, various common problems, namely the common solution of fixed point [15,16], variational inequality [17], variational inclusion [18], equilibrium [19,20], hierarchical fixed point [14] and split feasibility [21,22] problems with fixed point problems have been investigated by numerous authors. In 2009, Kangtunyakarn and Suantai [14] introduced an iterative technique and established a strong convergence theorem. In 2017, Kazmi et al. [23] proposed the following Krasnosel'skii-Mann iteration method to find common solutions of HFPP and SMEP.
{yn=(1−τn)χn+τn(φnSχn+(1−φn)Uχn),χn+1=KQ1(yn+δD∗(KQ2−I)Dyn), | (1.12) |
where KQ1=TF1rn(I−rng1), KQ2=TF2rn(I−rng2) and δ∈(0,1‖D‖2). In 2017, Majee and Nahak [24] initiated the following hybrid viscosity algorithm to find a common solution of SEP and FPP with the finite family of nonexpansive mappings.
{yn=KQ1(χn+δD∗(KQ2−I)Dyn),tn=σnχn+(1−σn)UnNUnN−1...Un2Un1yn,χn+1=ωnγh(χn)+[I−ωnμA]tn, | (1.13) |
where KQ1=TF1rn, KQ2=TF2rn, Uni=(1−κin)I+κinUi and δ∈(0,1‖D‖2). In 2018, Majee and Nahak [25] proposed the following viscosity approximation hybrid steepest-descent method to find a common solution of a SGEP and FPP for a finite collection of nonexpansive mappings.
{yn=KQ1(χn+δD∗(KQ2−I)Dyn),tn=σnχn+(1−σn)UnNUnN−1...Un2Un1yn,χn+1=ωnγh(χn)+ρnχn+[(1−ρn)I−ωnμA]tn, | (1.14) |
where KQ1=TF1rn, KQ2=TF2rn, Uni=(1−κin)I+κinUi and δ∈(0,1‖D‖2). In 2020, Kim and Majee [26] proposed the following modified Krasnosel'skii-Mann type iterative method in order to identify a common solution of SMEP and HFPP of a finite collection of k-strictly pseudocontractive operators.
{yn=KQ1(wn),ln=KQ2(Dyn),un=yn−δD∗(Dyn−ln),χn+1=(1−φn)un+φn[σnUun+(1−σn)UnNUnN−1...Un2Un1un], | (1.15) |
where KQ1=TF1rn, KQ2=TF2rn and Uni=(1−κin)I+κinPQ1(ζinI+(1−ζin)Ui). They proved its strong and weak convergence. In 2022, Yazdi and Sababe [27] proposed the following method in order to identify a common solution of a GMEP, common fixed points of a finite collection of nonexpansive mappings and a general system of variational inequalities.
{wn=τnχn+(1−τn)ln,F1(tn,z)+⟨g1wn,z−ξ⟩+ψ1(z)−ψ1(tn)+1rn⟨z−tn,tn−wn⟩≥0for allz∈Q1yn=PQ1(I−βg1)(tn),ln=PQ1(I−ρg2)yn,χn+1=ωnγh(χn)+ρnχn+[(1−ρn)I−ωnμA]UnNUnN−1...Un2Un1ln, | (1.16) |
where Uni=(1−κin)I+κinUi. They proved its strong convergence by taking some conditions on parameters.
The fixed point problem and its applications are very important in nonlinear analysis. In recent years, significant progress has also been made in research results; see [28,29,30,31,32,33]. We have applied our result for solving compressed sensing, and one can solve various nonlinear analysis problems using our algorithm. But, the applicability of our algorithm is not limited to the problems discussed above. It can be further used to solve many important problems, for instance, uncertain fractional-order differential equation with Caputo type [34,35].
In recent times, numerous researchers have explored inertial-type methods, drawing inspiration from the concept of heavy ball techniques. Polyak, in their work from 1964 [27] introduced an iterative approach aimed at enhancing the convergence rate of iterative sequences through the incorporation of an inertial extrapolation factor. Inertial approaches typically involve a two-step iterative process, where the next iteration is determined based on the previous two iterations. In 2021, Rehman et al. [19] introduced an innovative approach by combining an inertial term with a subgradient extragradient algorithm. They provided a proof of weak convergence for their proposed method. In the same year, Chuasuk and Kaewcharoen [37] introduced a Krasnosel'skii-Mann-type inertial technique designed for solving SGMEP and HFPP involving k-strictly pseudocontractive operators. They demonstrated its weak convergence properties. Recently, a variety of inertial techniques have emerged to address a wide range of equilibrium problems, as documented in the literature [10,37]. In 2023, Ugwunnadi et al. [38] introduced a Krasnosel'skii-Mann-type inertial technique for solving SMVIP and HFPP. These techniques offer valuable tools for solving mathematical problems efficiently and effectively.
In this study, influenced and inspired by aforementioned work, we give a new generalized viscosity approximation method for solving an SGMEP, fixed point problem for a finite collection of nonexpansive mappings Sj with 1≤j≤M and an HFPP for a finite collection of μi-strictly pseudocontractive mappings which involve finding a point ξ∈Q1 and
ξ∈M⋂j=1Fix(Sj)∩Ω∩GMEP(F1,g1,ψ1,Q1)andDξ∈GMEP(F2,g2,ψ2,Q2). | (1.17) |
Let the solution set of problem (1.17) be represented by Γ. We will prove strong convergence for Problem (1.17).
Remark 1.1. In this paper, our contribution can be highlighted as
1) For proving the convergence result, we have embedded an inertial term which accelerates the convergence speed of the algorithm. Majee and Nahak [24,25], Kim and Majee [26], and Yazdi and Sababe [27] do not consider the inertial approach in their method.
2) We consider KQ1=TF1rn(I−rng1), KQ2=TF2rn(I−rng2) in our algorithm, and if we take g1=g2=0, then various results are special cases of our result.
3) We consider τ-strictly pseudocontractive mappings for solving HFPP which include various mappings like pseudocontractive and nonexpansive mappings. Additionally, τ-strictly pseudocontractive mappings have more powerful applications than nonexpansive mappings in solving inverse problems.
4) Yazdi and Sababe [27] take the condition lim, whereas our main proof does not require such a condition.
5) Our result improved the results of Kazmi et al. [23] from the common solution of HFPP and SMEP, Majee and Nahak [24] from common solution of SEP and finite family of FPP, Majee and Nahak [25] from the common solution of a SGEP and finite family of FPP, Kim and Majee [26] from common solution of SMEP and HFPP to common solution of SGMEP, HFPP and finite family of FPP.
6) We provide a real-life application to compressed sensing for our problem and show that our method requires less computation time to recover the signal in comparison with other methods.
7) We compare our iterative technique to other approaches and present numerical examples to show the effectiveness of our algorithm.
8) Our result generalizes the result of Kazmi et al. [23] from weak convergence to strong convergence.
In Section 1, we introduce the background and motivation for our research, highlighting the significance of GMEP and HFPP in real-world applications. Section 2 provides a comprehensive literature review, discussing previous methods and techniques proposed for solving GMEP, HFPP, and related problems. Section 3 outlines our proposed method and Algorithm 1, and we prove our main result. Section 4 discusses the practical applicability of our approach in compressed sensing. Section 5 presents numerical experiments to validate the effectiveness of the algorithm and compare it with other existing approaches.
In this section, we consider a real Hilbert space denoted as H , equipped with an inner product denoted as \langle., .\rangle and the corresponding norm denoted as \|.\| . We assume that Q is a nonempty, closed and convex subset of this real Hilbert space H . We will use the notations \chi_n \rightharpoonup \chi and \chi_n \rightarrow \chi to signify weak and strong convergence, respectively, of the sequence \{\chi_n\} to the limit \chi . Furthermore, we denote the set of all fixed points of the mapping U as {\rm{Fix}}(U) .
Definition 2.1. [39] A \{graph (D_n) \} converges to \{graph (D) \} in the Kuratowski-Painleve sense, if
\begin{align*} \limsup\limits_{n\to +\infty} {\rm{graph}}(D_n)\subset {\rm{graph}}(D)\subset \liminf\limits_{n\to +\infty} {\rm{graph}}(D_n), \end{align*} | (2.1) |
where D_n is a sequence of maximal monotone mappings and D is a multivalued mapping.
Definition 2.2. [40] The metric projection P_{Q}: H\rightarrow Q is defined as
\begin{equation} \quad \|u'-P_{Q}u'\| = \inf \{\|u'-z'\|; z'\in Q\} \;{for \; all}\; u' \in H. \end{equation} | (2.2) |
Definition 2.3. [41] Suppose that U:H\rightarrow H is an operator. Then U is called
1) contraction on H if there is a constant \mu \in [0, 1) and
\begin{align*} \|U\bar{u}'-U\bar{v}'\|\leq \mu\|\bar{u}'-\bar{v}'\| \;\text{for all}\; \bar{u}', \bar{v}'\in H. \end{align*} |
2) L -Lipschitz continuous on H if
\begin{align*} \|U\bar{u}'-U\bar{v}'\|\leq L\|\bar{u}'-\bar{v}'\| \;\text{for all}\; \bar{u}', \bar{v}'\in H. \end{align*} |
3) monotone on Q if
\begin{align*} \langle U\bar{u}'-U\bar{v}', \bar{u}'-\bar{v}'\rangle \geq 0 \;\text{for all}\; \bar{u}', \bar{v}'\in Q. \end{align*} |
4) \gamma -inverse strongly monotone on Q if
\begin{align*} \gamma\|U\bar{u}'-U\bar{v}'\|^2 \le \langle \bar{u}'-\bar{v}', U\bar{u}'-U\bar{v}'\rangle \;\text{for all}\; \bar{u}', \bar{v}'\in Q. \end{align*} |
5) \tau -strictly pseudocontractive mapping if there exists \tau \in [0, 1) , such that
\begin{align*} \|U\bar{u}'-U\bar{v}'\|^2\leq\|\bar{u}'-\bar{v}'\|^2+ \tau\|(I-U)\bar{u}'-(I-U)\bar{v}'\|^2 \;\text{for all}\; \bar{u}', \bar{v}'\in Q. \end{align*} |
6) nonexpansive if
\begin{align*} \|U\bar{u}'-U\bar{v}'\|\leq\|\bar{u}'-\bar{v}'\| \;\text{for all}\; \bar{u}', \bar{v}' \in H. \end{align*} |
Definition 2.4. [42] The monotone bifunction g :Q\times Q\rightarrow \mathbb{R} on Q is defined as
\begin{align*} g(\zeta, x')+g(x', \zeta)\le 0 \;{for \; all}\; \zeta, x'\in Q. \end{align*} |
Definition 2.5. [43] The normal cone of Q at z' \in Q is defined as
\begin{align*} N_{Q}(z') = \{u' \in H: \langle u', \varrho-z'\rangle \le 0 \;{for \;all} \; \varrho\in Q\}. \end{align*} |
Definition 2.6. [44] A bounded linear operator D defined on H is called strongly positive if there is a constant \gamma > 0 such that
\begin{equation} \langle Dv, v\rangle\ge\gamma\|v\|^2 \;{for \; all}\;v\in H. \end{equation} | (2.3) |
Lemma 2.7. [45] Consider a strongly positive bounded linear, self-adjoint operator denoted as D . This operator has a positive coefficient \gamma > 0 , and 0 < \rho \le \|D\|^{-1} . Then, \|I - \rho D\| \le 1 - \rho \gamma .
Lemma 2.8. [46] For u' \in H and y' \in Q , y' = P_Qu' iff \langle u'-y', y'-z' \rangle\leq 0 for all z'\in Q , where P_Q is a metric projection.
Lemma 2.9. [47] Assume that \{U_i\}_{i = 1}^N are averaged mappings with a common fixed point. Then,
\begin{align*} {\bigcap^N_{i = 1} {{Fix}}(U_i)} = Fix(U_1U_2U_3...U_N). \end{align*} | (2.4) |
Lemma 2.10. [48] Let u', v', z'\in H . Then, the following conditions hold:
1) \|\xi u'+(1-\xi)z'\|^2 = \xi \|u'\|^2+ (1-\xi) \|z'\|^2-\xi (1-\xi) \|u'-z'\|^2 for all u', z' \in H and \xi \in [0, 1].
2) \|u'+z'\|^2 \leq \|u'\|^2+2\langle z', u'+z'\rangle for all u', z'\in H.
3) (Opial's condition) Consider a sequence y_n with y_n\rightharpoonup z' , then the following conclusions hold:
\begin{align*} \liminf\limits_{n \to +\infty}\|y_n-z'\| < \liminf\limits_{n \to +\infty}\|y_n-\varrho\|\;\text{for all}\;\varrho \in H \;\text{and}\;z'\ne \varrho. \end{align*} |
Lemma 2.11. [49] Suppose that U:Q \rightarrow H is a \eta -strictly pseudocontractive mapping with Fix(U)\ne \phi . Consider a mapping S as Sv = \tau v+(1-\tau)Uv for all v\in H , where \tau\in [\eta, 1) . Then, the following conclusions hold:
1) Fix(P_{Q}U) = Fix(U).
2) S is nonexpansive and Fix(U) = Fix(S).
Lemma 2.12. [50] If \{v_n\}\subset[0, +\infty) , \{w_n\} \subset(0, 1) , \{\tau_n\}\subset(0, 1) and \{\eta_n\} are real sequences satisfying the inequality
\begin{equation} v_{n+1}\le(1-w_n)v_n+\eta_n+\tau_n \;\text{for all}\; n\ge n_0. \end{equation} | (2.5) |
Suppose \sum_{n = 0}^{+\infty}\tau_n < +\infty, then the conclusions stated below hold:
1) If \eta_n \le w_n M for some M \ge 0 , then \{v_n\} is bounded sequence.
2) If \sum_{n = 0}^{+\infty}w_n = +\infty and \lim\limits_{n\rightarrow +\infty} \frac{\eta_n}{w_n} \le0 , then \lim\limits_{n\rightarrow +\infty}v_n = 0.
We need the following assumptions on bifunction g: Q\to Q to solve the split generalized mixed equilibrium problem:
Assumption 1.
1) g is monotone.
2) g(u', u')\ge 0 for all u' \in Q .
3) For each u', w', y'\in Q , \limsup\limits_{t\rightarrow 0^+} g(t'u'+(1-t')w', y') \leq h(w', y') .
4) For each u' \in Q, y' \rightarrow h(u', y') is lower semi-continuous and convex.
Now, we mention the following lemma which will be utilized for solving the monotone split generalized mixed equilibrium problem.
Lemma 2.13. [51] Assume that g:Q \times Q \rightarrow \mathbb{R} is a bifunction satisfying Assumption 1. Consider g_1:Q \to H a nonlinear mapping, \psi: Q \to \mathbb{R} \cup \{+\infty\} a convex and proper lower semicontinuous function. Define S_r^{g}(w) as follows:
\begin{align*} S_r^g(w) = \{x \in Q: g(x, z)+\langle g_1(x), z-x \rangle+ \psi(z)-\psi(x)+\frac{1}{r}\langle z-x, x-w\rangle \geq0\text{ for all } z\in Q\}, \end{align*} |
where w\in H and r > 0 . Then, the following statements hold:
1) For every u' \in H , S_r^g(u') \ne \phi .
2) S_r^g is single-valued.
3) Fix( S_r^g ) = GMEP( g, g_1, \psi ).
4) Solution set GMEP( g, g_1, \psi ) is closed and convex.
5) S_r^g is firmly nonexpansive, i.e., for any u', y' \in H
\begin{align*} {\|S_r^g(u')-S_r^g(y')\|}^2 \leq\langle S_r^g(u')-S_r^g(y'), u'-y'\rangle. \end{align*} |
Lemma 2.14. [52] Consider C a Lipschitz maximal monotone mapping and \{D_n\} a sequence of maximal monotone mappings defined on H . The statements are as follows:
1) If D_n is graph convergent to a mapping D on H , then C+D is maximal monotone and \{C+D_n\} is also graph convergent to C+D .
2) In addition, if D is a maximal monotone mapping defined on H and D^{-1}0 \ne \phi , then \{s_n^{-1}D\} is graph convergent to N_{D^{-1}0} as s_n \to +\infty .
Lemma 2.15. [53] Suppose \{v_n\} , \{w_n\} and \{\tau_n\} are bounded sequences in a Hilbert space H such that \{\tau_n\}\subset (0, 1) with 0 < \liminf_{n \to +\infty}\tau_n\le \limsup_{n \to +\infty}\tau_n < 1 . If v_{n+1} = (1-\tau_n)w_n+\tau_nv_n for all integers n \ge 0 and \limsup_{n \to +\infty}\|w_{n+1}-w_n\|-\|v_{n+1}-v_{n}\|\le 0 , then \lim_{n\rightarrow +\infty}\|w_n-v_n\| = 0 .
Lemma 2.16. [54] Suppose that \{U_j\}:Q \to Q is a finite family of nonexpansive mappings with 1\le j\le M and {\bigcap^M_{j = 1} {{Fix}}(U_j)}\ne \phi . Assume that the sequence \{\eta_{n, j}\} converges to \{\eta_{j}\} , where \eta_{n, j} \subset [0, 1] for 1\le j\le M , \eta_j \subset (0, 1) for 1\le j\le M-1 and \eta_M \subset (0, 1] . Consider a K -mapping generated by U_1, U_2, ..., U_M and \eta_1, \eta_2, ..., \eta_n . Let K_n be the K -mapping generated by U_1, U_2, ..., U_M and \eta_{n, 1}, \eta_{n, 2}, ..., \eta_{n, M} . Then, the conclusions stated below hold:
1) Fix( K ) = {\bigcap^M_{j = 1} {\rm{Fix}}(U_j)} .
2) \lim_{n\rightarrow +\infty}\|K_nv-Kv\| = 0 for each v \in Q_1.
Lemma 2.17. [55] Suppose U: Q \to Q is a nonexpansive mapping. Let \{v_n\} be a sequence in Q converging weakly to v \in Q and \{(I-U)v_n\} converging strongly to w \in Q , then (I -U)v = w and if w = 0 , then v \in Fix(U) .
In this section, we propose a new inertial generalized viscosity approximation method and prove a strong convergence theorem for solving split generalized mixed equilibrium problem, common fixed point problem of a finite family of nonexpansive mappings and hierarchical fixed point problem. Let F_1:Q_1\times Q_1 \to \mathbb{R}, F_2 :Q_2\times Q_2 \to \mathbb{R} be bifunctions satisfying Assumption 1 and F_2 be upper semicontinuous. Suppose D:H_1\rightarrow H_2 is a bounded linear operator with adjoint D^* such that \delta \in (0, \frac{1}{L}) , where L is the spectral radius of D Let g_1:Q_1 \to H_1 , g_2:Q_2 \to H_2 be \alpha_1 , \alpha_2 -ism mappings respectively, h:Q_1 \to Q_1 be a \nu -contraction mapping, U_i:Q_1 \to Q_1 be \mu_i -strictly pseudocontractive mappings for 1\le i \le N , \psi_1: Q_1 \to \mathbb{R} \cup \{+\infty\} , \psi_2: Q_2 \to \mathbb{R} \cup \{+\infty\} be convex and proper lower semicontinuous functions, U:Q_1 \to Q_1 be nonexpansive mapping and S_j:Q_1 \to Q_1 be nonexpansive mappings for 1 \le j\le M and A is a strongly positive bounded linear self-adjoint operator on H_1 with constant \bar{\gamma} > 0 such that 0 < \gamma < \frac{\bar{\gamma}}{\nu} < \gamma+\frac{1}{\nu} .
Algorithm 3.1. Consider \lambda_n \subset [0, +\infty) with \sum_{n = 0}^{+\infty}\lambda_n < +\infty , \tau \in [0, 1) , \varphi_n, \sigma_n, \kappa_n^i, \omega_n, \rho_n \in (0, 1) , \rho = \sup\{\rho_n; n\in \mathbb{{N}}\} with \lim\limits_{n\rightarrow +\infty}|\varphi_{n+1}-\varphi_n| = 0 , \lim\limits_{n\rightarrow +\infty}|\sigma_{n+1}-\sigma_n| = 0 and \sum_{n = 0}^{+\infty}\sigma_n < +\infty . Set n = 1 . Choose x_0, x_1 \in Q_1 and \tau_n such that 0 \le \tau_n\le \bar{\tau_n}, where
\begin{align} \bar{\tau_{n}} = \begin{cases} \min\Big\{{\frac{\lambda_n}{\|\chi_n-\chi_{n-1}\|}}, \tau\Big\} & \;{\rm{if}}\; \chi_n\neq \chi_{n-1}, \\\tau & otherwise. \end{cases} \end{align} | (3.1) |
Step 1: Compute
\begin{align} { } \begin{cases} w_n = \chi_n+\tau_n(\chi_n-\chi_{n-1}), \\y_n = K_{Q_1}(w_n), l_n = K_{Q_2}(Dy_n), \\u_n = y_n-\delta D^*(Dy_n-l_n), \end{cases} \end{align} | (3.2) |
where K_{Q_1} = T_{r_n}^{F_1}(I-r_ng_1) , K_{Q_2} = T_{r_n}^{F_2}(I-r_ng_2) with r_n\subset\min\{\alpha_1, \alpha_2\} = 2\alpha , \liminf_{n \to +\infty}r_n > 0 and \lim\limits_{n\rightarrow +\infty}|r_{n+1}-r_n| = 0 .
Step 2: Compute
\begin{align} t_n = (1-\varphi_n)u_n+\varphi_n[\sigma_nUu_n+(1-\sigma_n)U_N^nU_{N-1}^n...U_{2}^nU_{1}^nu_n], \end{align} | (3.3) |
where U_{i}^n = (1- \kappa_n^i)I+ \kappa_n^iP_{Q_1}(\zeta_{n}^iI+(1-\zeta_{n}^i)U_i) with 0 \le \mu_i \le\zeta_{n}^i < 1 and \lim\limits_{n\rightarrow +\infty}|\kappa^i_{n+1}-\kappa_n^i| = 0 for i\le i\le M .
Step 3: Evaluate
\begin{align} \chi_{n+1} = \omega_n\gamma h(K_n\chi_n)+\rho_n\chi_n+[(1-\rho_n)I-\omega_nA]K_nt_n. \end{align} | (3.4) |
Step 4: If \chi_{n+1} = \chi_n , terminate the process. Otherwise, set n: = n+1 and return to Step 1.
Remark 3.2. From Eq (3.1) and \sum_{n = 0}^{+\infty}\lambda_n < +\infty , we get \sum_{n = 0}^{+\infty}\tau_n(\chi_n-\chi_{n-1}) < +\infty .
Theorem 3.3. Let \Gamma , the solution set defined in Eq (1.17) be nonempty. Suppose that Assumption 1 holds and the following conditions are satisfied:
1) \lim\limits_{n\rightarrow +\infty}\omega_n = 0 and \sum_{n = 0}^{+\infty}\omega_n = +\infty ,
2) 0 < \liminf_{n \to +\infty}\rho_n\le \limsup_{n \to +\infty}\rho_n < 1,
3) \lim\limits_{n\rightarrow +\infty}\frac{\|t_n-u_n\|}{\sigma_n\varphi_n} = 0.
Then, \{\chi_n\} generated by Algorithm 3.1 converges strongly to \varrho , where \varrho\in \Gamma and \varrho is the unique fixed point of contraction mapping P_{\Gamma}(I+\gamma h-A).
Proof. We have divided the proof in various steps. We will establish the theorem for the case when N = 2 and, subsequently, we will illustrate how the procedure can be readily applied to the general case.
Claim 1: The sequence \{\chi_n\} is bounded.
Let \varrho\in \bigcap^M_{j = 1} {\rm{Fix}}(S_j) \cap \Omega\cap \Theta = \Gamma . Also, with \omega_n\to 0 as n\to +\infty , we can assume that
\begin{align} \omega_n < \frac{1-\rho}{\|A\|}, \;\text{for all}\;n \end{align} | (3.5) |
and then
\begin{align*} \omega_n < \frac{1-\rho}{\bar{\gamma}}, \;\text{for all}\;n. \end{align*} |
Using Lemma 2.7, we get
\begin{align} \|I-\omega_n A\|\le 1-\omega_n\bar{\gamma}. \end{align} | (3.6) |
As we know that A is strongly positive bounded linear operator, then \langle Av, v\rangle \ge \bar{\gamma}\|v\|^2 and \|A\| = \sup\{|\langle Av, v\rangle|; \|v\| = 1, v \in H_1 \}. Now consider
\begin{align} \langle( (1-\rho_n)I-\omega_n A)v, v\rangle & = 1-\rho_n-\omega_n \langle Av, v\rangle\\&\ge 1-\rho-\omega_n\|A\|\ge0 \;\text{for all}\;v\in H_1. \end{align} | (3.7) |
Thus, using Eq (3.5), we get (1-\rho_n)I-\omega_n A is positive operator. Also,
\begin{align} 0\le\|(1-\rho_n)I-\omega_n A\|& = \sup\{|\langle (1-\rho_n)I-\omega_n Av, v\rangle|; \|v\| = 1, v \in H_1 \}\\& = \sup\{| 1-\rho_n-\omega_n \langle Av, v\rangle|; \|v\| = 1, v \in H_1 \}\\&\le1-\rho_n-\omega_n \bar{\gamma}. \end{align} | (3.8) |
Consider
\begin{align} \|w_n-\varrho\|& = \|\chi_n+\tau_n(\chi_n-\chi_{n-1})-\varrho\|\\&\le\|\chi_n-\varrho\|+\tau_n\|\chi_n-\chi_{n-1}\|. \end{align} | (3.9) |
Using Lemma 2.9, I - r_n g_1 is a nonexpansive mapping and hence K_{Q_1} is a nonexpansive mapping. From Eq (3.2), we have
\begin{align} \|y_n-\varrho\|^2& = \|K_{Q_1}(w_n)-K_{Q_1}(\varrho)\|^2\\& = \|T_{r_n}^{F_1}(I-r_ng_1)w_n-T_{r_n}^{F_1}(I-r_ng_1)\varrho\|^2\\&\le\|(w_n-\varrho)-r_n(g_1(w_n)-g_1(\varrho))\|^2\\&\le\|w_n-\varrho\|^2+r_n^2\|g_1(w_n)-g_1(\varrho)\|^2-2r_n\alpha_1\|g_1(w_n)-g_1(\varrho)\|^2\\& = \|w_n-\varrho\|^2-r_n(2\alpha_1-r_n)\|g_1(w_n)-g_1(\varrho)\|^2 \end{align} | (3.10) |
\begin{align} & \le\|w_n-\varrho\|^2. \end{align} | (3.11) |
Similarly,
\begin{align} \|l_n-D\varrho\|& = \|K_{Q_2}(Dy_n)-K_{Q_2}(D\varrho)\|\\&\le\|Dy_n-D\varrho\|. \end{align} | (3.12) |
Using Eq (3.12), we have
\begin{align} \langle y_n-\varrho, D^*(l_n-Dy_n) \rangle& = \langle Dy_n-D\varrho, l_n-Dy_n \rangle\\& = \langle Dy_n-D\varrho-(l_n-Dy_n)+(l_n-Dy_n), l_n-Dy_n \rangle\\& = \langle l_n-D\varrho, l_n-Dy_n \rangle-\|l_n-Dy_n\|^2\\& = \frac{1}{2}\bigg[\| l_n-D\varrho\|^2+\|l_n-Dy_n\|^2-\|Dy_n-D\varrho\|^2\bigg]\\&-\|l_n-Dy_n\|^2\\&\le\frac{1}{2}\bigg[\| Dy_n-D\varrho\|^2-\|Dy_n-D\varrho\|^2\bigg]-\frac{1}{2}\|l_n-Dy_n\|^2\\& = -\frac{1}{2}\|l_n-Dy_n\|^2. \end{align} | (3.13) |
From Eqs (3.2), (3.11), (3.13) and \delta \in(0, \frac{1}{L}) , we have
\begin{align} \|u_n-\varrho\|^2& = \|y_n-\delta D^*(Dy_n-l_n)-\varrho\|^2\\& = \|y_n-\varrho\|^2+\delta^2\|D^*(Dy_n-l_n)\|^2-2\delta\langle y_n-\varrho, D^*(Dy_n-l_n) \rangle\\& = \|y_n-\varrho\|^2+\delta^2\|D^*(Dy_n-l_n)\|^2+2\delta\langle y_n-\varrho, D^*(l_n-Dy_n) \rangle\\&\le \|y_n-\varrho\|^2+\delta^2L\|l_n-Dy_n\|^2+2\delta\big[-\frac{1}{2}\|l_n-Dy_n\|^2\big]\\& = \|y_n-\varrho\|^2+(\delta^2L-\delta)\|l_n-Dy_n\|^2\\& = \|y_n-\varrho\|^2-\delta(1-\delta L)\|l_n-Dy_n\|^2 \end{align} | (3.14) |
\begin{align} & \le \|w_n-\varrho\|^2. \end{align} | (3.15) |
From Eqs (3.9) and (3.15), we have
\begin{align} \|u_n-\varrho\|&\le \|w_n-\varrho\|\\& \le \|\chi_n-\varrho\|+\tau_n\|\chi_n-\chi_{n-1}\|. \end{align} | (3.16) |
Using Lemmas 2.9 and 2.11, we have U_{2}^nU_{1}^n\varrho = \varrho . From Eqs (3.3) and (3.16), we have
\begin{align} \|t_n-\varrho\|& = \|(1-\varphi_n)u_n+\varphi_n[\sigma_nUu_n+(1-\sigma_n)U_{2}^nU_{1}^nu_n]-\varrho\|\\& \le(1-\varphi_n)\|u_n-\varrho\|+\varphi_n[\sigma_n\|Uu_n-\varrho\|+(1-\sigma_n)\|U_{2}^nU_{1}^nu_n-\varrho\|]\\& \le(1-\varphi_n)\|u_n-\varrho\|+\varphi_n[\sigma_n\|Uu_n-U\varrho\|+\sigma_n\|U\varrho-\varrho\|\\&+(1-\sigma_n)\|u_n-\varrho\|]\\& \le(1-\varphi_n)\|u_n-\varrho\|+\varphi_n[\|u_n-\varrho\|+\sigma_n\|U\varrho-\varrho\|]\\& = \|u_n-\varrho\|+\varphi_n\sigma_n\|U\varrho-\varrho\| \end{align} | (3.17) |
\begin{align} & \le \|\chi_n-\varrho\|+\tau_n\|\chi_n-\chi_{n-1}\|+\varphi_n\sigma_n\|U\varrho-\varrho\|. \end{align} | (3.18) |
Using Eqs (3.4) and (3.18), we have
\begin{align} \|\chi_{n+1}-\varrho\|& = \| \omega_n\gamma h(K_n\chi_n)+\rho_n\chi_n+[(1-\rho_n)I-\omega_nA]K_nt_n-\varrho\|\\& \le\| \omega_n\gamma h(K_n\chi_n)-\omega_n\gamma h(\varrho)\|+\|\omega_n\gamma h(\varrho)-\omega_n A\varrho\|+\rho_n\|\chi_n-\varrho\|+[(1-\rho_n)-\omega_n\bar{\gamma}]\|K_nt_n-\varrho\|\\& \le\omega_n\gamma \nu \|\chi_n- \varrho\|+\omega_n\|\gamma h(\varrho)- A\varrho\|+\rho_n\|\chi_n-\varrho\|+[(1-\rho_n)-\omega_n\bar{\gamma}]\|t_n-\varrho\|\\& \le \omega_n\gamma \nu \|\chi_n- \varrho\|+\omega_n\|\gamma h(\varrho)- A\varrho\|+\rho_n\|\chi_n-\varrho\|\\&+[(1-\rho_n)-\omega_n\bar{\gamma}]\big[\|\chi_n-\varrho\|+\tau_n\|\chi_n-\chi_{n-1}\|+\varphi_n\sigma_n\|U\varrho-\varrho\|\big]\\& \le (1-\omega_n(\bar{\gamma}-\gamma \nu)) \|\chi_n- \varrho\|+\omega_n\|\gamma h(\varrho)- A\varrho\|\\&+[(1-\rho_n)-\omega_n\bar{\gamma}]\big[\tau_n\|\chi_n-\chi_{n-1}\|+\varphi_n\sigma_n\|U\varrho-\varrho\|\big]\\& \le(1-\omega_n(\bar{\gamma}-\gamma \nu)) \|\chi_n- \varrho\|+\omega_n\|\gamma h(\varrho)- A\varrho\|\\&+\tau_n\|\chi_n-\chi_{n-1}\|+\sigma_n\|U\varrho-\varrho\|\\& \le(1-\omega_n(\bar{\gamma}-\gamma \nu)) \|\chi_n- \varrho\|+\omega_n\|\gamma h(\varrho)- A\varrho\|\\&+\tau_n\|\chi_n-\chi_{n-1}\|+\sigma_n\|U\varrho-\varrho\|. \end{align} | (3.19) |
Let v_n = \|\chi_n- \varrho\| , w_n = \omega_n(\bar{\gamma}-\gamma \nu) , \eta_n\le w_n M = \frac{\omega_n(\bar{\gamma}-\gamma \nu)\|\gamma h(\varrho)- A\varrho\|}{(\bar{\gamma}-\gamma \nu)} and \tau_n^1 = \tau_n\|\chi_n-\chi_{n-1}\|+\sigma_n\|U\varrho-\varrho\| . Thus, we have
\begin{align*} v_{n+1}\le (1-w_n) v_n+\eta_n+\tau_n^1 \end{align*} |
Using Lemma 2.12, Remark 3.2, condition (i) and \sum_{n = 0}^{+\infty}\sigma_n < +\infty , we get that \{v_n\} is bounded, which implies \{\|\chi_n- \varrho\|\} is bounded. Hence, \{\chi_n\} is bounded. Consequently, \{t_n\} , \{u_n\} , \{w_n\} , \{y_n\} , \{h(K_n \chi_n)\} are also bounded.
Claim 2: \limsup_{n \to +\infty} (\|f_{n+1}-f_{n}\|-\|\chi_{n+1}-\chi_n\|)\le0.
Consider
\begin{align} \|w_n-\varrho\|^2& = \|\chi_n+\tau_n(\chi_n-\chi_{n-1})-\varrho\|^2\\&\le\|\chi_n-\varrho\|^2+\tau_n^2\|\chi_n-\chi_{n-1}\|^2+2\tau_n\|\chi_n-\chi_{n-1}\|\|\chi_n-\varrho\|\\& = \|\chi_n-\varrho\|^2+\tau_n\|\chi_n-\chi_{n-1}\|[\tau_n\|\chi_n-\chi_{n-1}\|+2\|\chi_n-\varrho\|]\\&\le\|\chi_n-\varrho\|^2+\tau_n\|\chi_n-\chi_{n-1}\|M, \end{align} | (3.20) |
where M = \sup\{\tau_n\|\chi_n-\chi_{n-1}\|+2\|\chi_n-\varrho\|; n\in \mathbb{{N}} \} .
Also,
\begin{align} \|w_{n+1}-w_n\|& = \|\chi_{n+1}+\tau_{n+1}(\chi_{n+1}-\chi_n)-(\chi_n+\tau_n(\chi_n-\chi_{n-1}))\|\\&\le\|\chi_{n+1}-\chi_n\|+\tau_{n+1}\|\chi_{n+1}-\chi_n\|+\tau_n\|\chi_n-\chi_{n-1}\|. \end{align} | (3.21) |
As y_n = T_{r_n}^{F_1}(I-r_ng_1)(w_n) and y_{n+1} = T_{r_{n+1}}^{F_1}(I-r_{n+1}g_1)(w_{n+1}) , we get
\begin{align} F_1(y_n, z)+\langle g_1(w_n), z-y_n \rangle+ \psi_1(z)-\psi_1(y_n)+\frac{1}{r_n}\langle z-y_n, y_n-w_n\rangle \geq0\text{ for all } z\in Q_1, \end{align} | (3.22) |
and
\begin{align} F_1(y_{n+1}, z)+\langle g_1(w_{n+1}), z-y_{n+1} \rangle+ \psi_1(z)-\psi_1(y_{n+1}) \;\;\;\;\;\;\\ +\frac{1}{r_{n+1}}\langle z-y_{n+1}, y_{n+1}-w_{n+1}\rangle \geq0\text{ for all } z\in Q_1. \end{align} | (3.23) |
Putting z = y_{n+1} and z = y_n in Eqs (3.22) and (3.23), respectively, we get
\begin{align} F_1(y_n, y_{n+1})+& \langle g_1(w_n), y_{n+1}-y_n \rangle\\&+ \psi_1(y_{n+1})-\psi_1(y_n)+\frac{1}{r_n}\langle y_{n+1}-y_n, y_n-w_n\rangle \geq0, \end{align} | (3.24) |
and
\begin{align} F_1(y_{n+1}, y_n)+\langle g_1(w_{n+1}), y_n-&y_{n+1} \rangle+ \psi_1(y_n)-\psi_1(y_{n+1})\\&+\frac{1}{r_{n+1}}\langle y_n-y_{n+1}, y_{n+1}-w_{n+1}\rangle \geq0. \end{align} | (3.25) |
Adding Eqs (3.24) and (3.25) and using the monotonicity of F_1 , we get
\begin{align} \langle g_1(w_{n+1})-g_1(w_n), y_n-y_{n+1} \rangle+\langle y_n-y_{n+1}, \frac{y_{n+1}-w_{n+1}}{r_{n+1}}-\frac{y_n-w_{n}}{r_{n}}\rangle \geq0. \end{align} | (3.26) |
Upon rearranging the terms in Eq (3.26), we get
\begin{align} 0&\le\langle y_n-y_{n+1}, r_n(g_1(w_{n+1})-g_1(w_n))+\frac{r_n}{r_{n+1}}(y_{n+1}-w_{n+1})-(y_n-w_{n})\rangle \\&\le\langle y_{n+1}-y_n, y_n-y_{n+1}+(1-\frac{r_n}{r_{n+1}})y_{n+1}\rangle\\&+\langle y_{n+1}-y_n, (w_{n+1}-r_ng_1(w_{n+1}))-(w_{n}-r_ng_1(w_n))-w_{n+1}+\frac{r_n}{r_{n+1}}w_{n+1} \rangle \\&\le\langle y_{n+1}-y_n, y_n-y_{n+1}+(1-\frac{r_n}{r_{n+1}})(y_{n+1}-w_{n+1})\rangle\\&+\langle y_{n+1}-y_n, (w_{n+1}-r_ng_1(w_{n+1}))-(w_{n}-r_ng_1(w_n)) \rangle. \end{align} | (3.27) |
Hence, we get
\begin{align} \|y_{n+1}-y_n\|^2\le\|y_{n+1}-y_n\|\bigg[\|w_{n+1}-w_{n}\|+\bigg|1-\frac{r_n}{r_{n+1}}\bigg| \|y_{n+1}-w_{n+1}\|\bigg]. \end{align} | (3.28) |
Subsequently, we have
\begin{align} \|y_{n+1}-y_n\|&\le\|w_{n+1}-w_{n}\|+\bigg|1-\frac{r_n}{r_{n+1}}\bigg| \|y_{n+1}-w_{n+1}\|\\&\le\|w_{n+1}-w_{n}\|+\frac{1}{r_{n+1}}\big|r_{n+1}-r_n\big|\|y_{n+1}-w_{n+1}\|. \end{align} | (3.29) |
Assume that for any n > 0 , there is a real number c_1 such that r_n > c_1 > 0 . From Eq (3.29), we get
\begin{align} \|y_{n+1}-y_n\|&\le\|w_{n+1}-w_{n}\|+\frac{1}{c_1}\big|r_{n+1}-r_n\big|M_1, \end{align} | (3.30) |
where M_1 = \sup \{\|w_{n+1}-y_{n+1}\|; n\in \mathbb{{N}}\} . In a similar way, we can deduce that
\begin{align} \|l_{n+1}-l_{n}\|&\le\|Dy_{n+1}-Dy_n\|+\frac{1}{c_2}\big|r_{n+1}-r_n\big|M_2, \end{align} | (3.31) |
where M_2 = \sup \{\|l_{n+1}-Dy_{n+1}\|; n\in \mathbb{{N}}\} , l_{n} = T_{r_{n}}^{F_2}(I-r_{n}g_2)Dy_n and l_{n+1} = T_{r_{n+1}}^{F_2}(I-r_{n+1}g_2)Dy_{n+1}. Also,
\begin{align} \|u_{n+1}-u_{n}\|^2& = \|y_{n+1}-\delta D^*(Dy_{n+1}-l_{n+1})-(y_n-\delta D^*(Dy_n-l_{n}))\|^2\\&\le\|y_{n+1}-y_n\|^2+\delta^2\|D^*\|^2\|D(y_{n+1})-D(y_n)-(l_{n+1}-l_n)\|^2\\&+2\delta\langle Dy_{n+1}-Dy_n+(l_{n+1}-Dy_{n+1}), (l_{n+1}-Dy_{n+1})-(l_{n}-Dy_n)\rangle\\&-\langle(l_{n}-Dy_n), (l_{n+1}-Dy_{n+1})-(l_{n}-Dy_n)\rangle\\&-2\delta\langle (l_{n+1}-Dy_{n+1})-(l_{n}-Dy_n), (l_{n+1}-Dy_{n+1})-(l_{n}-Dy_n)\rangle \\&\le\|y_{n+1}-y_n\|^2+\delta^2\|D^*\|^2\|D(y_{n+1})-D(y_n)-(l_{n+1}-l_n)\|^2\\&+2\delta\bigg[\frac{1}{2}\|l_{n+1}-l_n\|^2+\frac{1}{2}\|D(y_{n+1})-D(y_n)-(l_{n+1}-l_n)\|^2\\&-\frac{1}{2}\|Dy_{n+1}-Dy_n\|^2\bigg]-2\delta\| (l_{n+1}-Dy_{n+1})-(l_{n}-Dy_n)\|^2 \\& = \|y_{n+1}-y_n\|^2-\delta(1-\delta\|D\|^2)\|D(y_{n+1})-D(y_n)-(l_{n+1}-l_n)\|^2\\&+\delta(\|l_{n+1}-l_n\|^2-\|D(y_{n+1})-D(y_n)\|^2)\\&\le\|y_{n+1}-y_n\|^2-\delta(1-\delta L)\|D(y_{n+1})-D(y_n)-(l_{n+1}-l_n)\|^2\\&+\frac{M_2}{c_2}\delta|r_{n+1}-r_{n}|(\|l_{n+1}-l_n\|+\|D(y_{n+1})-D(y_n)\|)\\&\le\|y_{n+1}-y_n\|^2+\frac{M_2}{c_2}\delta|r_{n+1}-r_{n}|(\|l_{n+1}-l_n\|+\|D(y_{n+1})-D(y_n)\|). \end{align} | (3.32) |
Using the inequality \sqrt{|c'|+|d'|}\le \sqrt{|c'|}+\sqrt{|d'|} , we get
\begin{align} \|u_{n+1}-u_{n}\|\le\|y_{n+1}-y_n\|+\sqrt{\frac{M_2}{c_2}\delta|r_{n+1}-r_{n}|(\|l_{n+1}-l_n\|+\|D(y_{n+1})-D(y_n)\|)}. \end{align} | (3.33) |
Using Eq (3.30), we get
\begin{align} \|u_{n+1}-u_{n}\|&\le\|w_{n+1}-w_n\|+\sqrt{\frac{M_2}{c_2}\delta|r_{n+1}-r_{n}|(\|l_{n+1}-l_n\|+\|D(y_{n+1})-D(y_n)\|)} \\&+\frac{1}{c_1}\big|r_{n+1}-r_n\big|M_1. \end{align} | (3.34) |
Choose constant M_3 such that
\begin{align} \sqrt{\frac{M_2}{c_2}\delta(\|l_{n+1}-l_n\|+\|D(y_{n+1})-D(y_n)\|)} \le M_3. \end{align} | (3.35) |
From Eqs (3.34) and (3.35), we have
\begin{align} \|u_{n+1}-u_{n}\|&\le\|w_{n+1}-w_{n}\|+M_3\sqrt{|r_{n+1}-r_n|}+\frac{1}{c_1}\big|r_{n+1}-r_n\big|M_1\\&\le\|\chi_{n+1}-\chi_n\|+\tau_{n+1}\|\chi_{n+1}-\chi_n\|+\tau_n\|\chi_n-\chi_{n-1}\|\\&+M_3\sqrt{|r_{n+1}-r_n|}+\frac{1}{c_1}\big|r_{n+1}-r_n\big|M_1. \end{align} | (3.36) |
Let s_n = \sigma_nUu_n+(1-\sigma_n)U_{2}^nU_{1}^nu_n , then t_n = (1-\varphi_n)u_n+\varphi_ns_n , and we estimate
\begin{align} \|s_{n+1}-s_n\|& = \|\sigma_{n+1}Uu_{n+1}+(1-\sigma_{n+1})U_{2}^{n+1}U_{1}^{n+1}u_{n+1}-(\sigma_nUu_n\\&+(1-\sigma_n)U_{2}^nU_{1}^nu_n)\|\\& = \|\sigma_{n+1}Uu_{n+1}+\sigma_{n+1}Uu_{n}-\sigma_{n+1}Uu_{n}+(1-\sigma_{n+1})U_{2}^{n+1}U_{1}^{n+1}u_{n+1}\\&+(1-\sigma_{n+1})U_{2}^{n}U_{1}^{n}u_{n}-(1-\sigma_{n+1})U_{2}^{n}U_{1}^{n}u_{n}-(\sigma_nUu_n\\&+(1-\sigma_n)U_{2}^nU_{1}^nu_n)\|\\&\le\sigma_{n+1}\|Uu_{n+1}-Uu_n\|+|\sigma_{n+1}-\sigma_{n}|\|U_{2}^nU_{1}^nu_{n}-Uu_n\|\\&+(1-\sigma_{n+1})\|U_{2}^{n+1}U_{1}^{n+1}u_{n+1}-U_{2}^nU_{1}^nu_{n}\|. \end{align} | (3.37) |
In a similar way,
\begin{align} \| t_{n+1}-t_n\|& = \|(1-\varphi_{n+1})u_{n+1}+\varphi_{n+1}s_{n+1}-((1-\varphi_n)u_n+\varphi_ns_{n})\|\\&\le(1-\varphi_{n+1})\|u_{n+1}-u_n\|+(\varphi_{n+1}-\varphi_n)\|u_{n}-s_n\|+\varphi_{n+1}\|s_{n+1}-s_n\|. \end{align} | (3.38) |
Using Eqs (3.37) and (3.38), we get
\begin{align} \| t_{n+1}-t_n\|&\le(1-\varphi_{n+1})\|u_{n+1}-u_n\|+(\varphi_{n+1}-\varphi_n)\|u_{n}-s_n\|\\&+\varphi_{n+1}\big[\sigma_{n+1}\|Uu_{n+1}-Uu_n\|+|\sigma_{n+1}-\sigma_{n}|\|U_{2}^nU_{1}^nu_{n}-Uu_n\|\\&+(1-\sigma_{n+1})\|U_{2}^{n+1}U_{1}^{n+1}u_{n+1}-U_{2}^nU_{1}^nu_{n}\|\big]. \end{align} | (3.39) |
Now, consider
\begin{align} \|U_{2}^{n+1}U_{1}^{n+1}u_{n+1}-U_{2}^nU_{1}^nu_{n}\|&\le\|U_{2}^{n+1}U_{1}^{n+1}u_{n+1}-U_{2}^nU_{1}^nu_{n+1}\|\\&+\|U_{2}^{n}U_{1}^{n}u_{n+1}-U_{2}^nU_{1}^nu_{n}\|\\&\le\|U_{2}^{n+1}U_{1}^{n+1}u_{n+1}-U_{2}^{n+1}U_{1}^nu_{n+1}\|\\&+\|U_{2}^{n+1}U_{1}^{n}u_{n+1}-U_{2}^nU_{1}^nu_{n+1}\|+\|u_{n+1}-u_n\|\\&\le\|U_{1}^{n+1}u_{n+1}-U_{1}^nu_{n+1}\|\\&+\|U_{2}^{n+1}U_{1}^{n}u_{n+1}-U_{2}^nU_{1}^nu_{n+1}\|+\|u_{n+1}-u_n\|. \end{align} | (3.40) |
Using the definition of U_i^n , we estimate that
\begin{align} \|U_1^{n+1}u_{n+1}-U_1^nu_{n+1}\|& = \|(1- \kappa_{n+1}^1)I+ \kappa_{n+1}^1P_{Q_1}(\zeta_{n+1}^1I+(1-\zeta_{n+1}^1)U_1)u_{n+1}\\&-((1- \kappa_n^1)I+ \kappa_n^1P_{Q_1}(\zeta_{n}^1I+(1-\zeta_{n}^1)U_1)u_{n+1})\|\\&\le\|(1- \kappa_{n+1}^1)I+ \kappa_{n+1}^1P_{Q_1}(\zeta_{n}^1I+(1-\zeta_{n}^1)U_1)u_{n+1}\\&+\kappa_{n+1}^1P_{Q_1}(\zeta_{n}^1I+(1-\zeta_{n}^1)U_1)u_{n+1}-\kappa_{n+1}^1P_{Q_1}(\zeta_{n+1}^1I+(1\\&-\zeta_{n+1}^1)U_1)u_{n+1}-((1- \kappa_n^1)I+ \kappa_n^1P_{Q_1}(\zeta_{n}^1I+(1-\zeta_{n}^1)U_1)u_{n+1})\|\\&\le|\kappa_{n+1}^1-\kappa_{n}^1|[\|u_{n+1}\|+\|P_{Q_1}(\zeta_{n}^1I+(1-\zeta_{n}^1)U_1)u_{n+1}\|]\\&\le|\kappa_{n+1}^1-\kappa_{n}^1|J_1, \end{align} | (3.41) |
where J_1 = \|P_{Q_1}(\zeta_{n}^1I+(1-\zeta_{n}^1)U_1)u_{n+1}\|+\|u_{n+1}\| . As \lim\limits_{n\rightarrow +\infty}|\kappa_{n+1}^1-\kappa_{n}^1| = 0 , \{u_n\} and \{P_{Q_1}(\zeta_{n}^1I+(1-\zeta_{n}^1)U_1)u_{n+1}\} are bounded, we have
\begin{align} \lim\limits_{n\rightarrow +\infty} \|U_1^{n+1}u_{n+1}-U_1^nu_{n+1}\| = 0. \end{align} | (3.42) |
Similarly,
\begin{align} \|U_2^{n+1}U_1^nu_{n+1}-U_2^nU_1^nu_{n+1}\|&\le|\kappa_{n+1}^2-\kappa_{n}^2|[\|U_1^nu_{n+1}\|\\&+\|P_{Q_1}(\zeta_{n}^2I+(1-\zeta_{n}^2)U_2)U_1^nu_{n+1}\|]\\&\le|\kappa_{n+1}^2-\kappa_{n}^2|J_2, \end{align} | (3.43) |
where J_2 = \|U_1^nu_{n+1}\|+\|P_{Q_1}(\zeta_{n}^2I+(1-\zeta_{n}^2)U_1)u_{n+1}\| . Using Eqs (3.36), (3.39), (3.40), (3.41) and (3.43), we get
\begin{align} \| t_{n+1}-t_n\|&\le(1-\varphi_{n+1})\|u_{n+1}-u_n\|+(\varphi_{n+1}-\varphi_n)\|u_{n}-s_n\|+\varphi_{n+1}\big[\|u_{n+1}-u_n\|\\&+(1-\sigma_{n+1})(|\kappa_{n+1}^1-\kappa_{n}^1|J_1+|\kappa_{n+1}^2-\kappa_{n}^2|J_2)\\&+|\sigma_{n+1}-\sigma_{n}|\|U_{2}^nU_{1}^nu_{n}-Uu_n\|\big]\\&\le(1-\varphi_{n+1})\|u_{n+1}-u_n\|+(\varphi_{n+1}-\varphi_n)\|u_{n}-s_n\|+\varphi_{n+1}\big[\|u_{n+1}-u_n\|\\&+|\kappa_{n+1}^1-\kappa_{n}^1|J_1+|\kappa_{n+1}^2-\kappa_{n}^2|J_2+|\sigma_{n+1}-\sigma_{n}|\|U_{2}^nU_{1}^nu_{n}-Uu_n\|\big]\\&\le\|u_{n+1}-u_n\|+(\varphi_{n+1}-\varphi_n)\|u_{n}-s_n\|+|\sigma_{n+1}-\sigma_{n}|\|U_{2}^nU_{1}^nu_{n}-Uu_n\|\\&+|\kappa_{n+1}^1-\kappa_{n}^1|J_1+|\kappa_{n+1}^2-\kappa_{n}^2|J_2\\&\le\|u_{n+1}-u_n\|+(\varphi_{n+1}-\varphi_n)\|u_{n}-s_n\|+|\sigma_{n+1}-\sigma_{n}|\|U_{2}^nU_{1}^nu_{n}-Uu_n\|\\&+|\kappa_{n+1}^1-\kappa_{n}^1|J_1+|\kappa_{n+1}^2-\kappa_{n}^2|J_2\\&\le\|\chi_{n+1}-\chi_n\|+\tau_{n+1}\|\chi_{n+1}-\chi_n\|+\tau_n\|\chi_n-\chi_{n-1}\|\\&+M_3\sqrt{|r_{n+1}-r_n|}+\frac{1}{c_1}\big|r_{n+1}-r_n\big|M_1\\&+(\varphi_{n+1}-\varphi_n)\|u_{n}-s_n\|+|\sigma_{n+1}-\sigma_{n}|\|U_{2}^nU_{1}^nu_{n}-Uu_n\|\\&+|\kappa_{n+1}^1-\kappa_{n}^1|J_1+|\kappa_{n+1}^2-\kappa_{n}^2|J_2. \end{align} | (3.44) |
Let \chi_{n+1} = (1-\rho_n)f_n+\rho_n\chi_n . Then, f_n = \frac{\chi_{n+1}-\rho_n\chi_n}{1-\rho_n} and
\begin{align} \|f_{n+1}-f_{n}\|&\le\bigg\| \frac{\omega_{n+1}\gamma h(K_{n+1}\chi_{n+1})+[(1-\rho_{n+1})I-\omega_{n+1}A]K_{n+1}t_{n+1}}{1-\rho_{n+1}}\\&-\frac{\omega_n\gamma h(K_n\chi_n)+[(1-\rho_n)I-\omega_nA]K_nt_n}{1-\rho_n}\bigg\|\\&\le\frac{\omega_{n+1}}{1-\rho_{n+1}}[\|\gamma h(K_{n+1}\chi_{n+1})\|+\|AK_{n+1}t_{n+1}\|]\\&+\frac{\omega_n}{1-\rho_{n}}[\|\gamma h(K_{n}\chi_n)\|+\|AK_{n}t_{n}\|]\\&+\|K_{n+1}t_{n+1}-K_{n+1}t_{n}\| +\|K_{n+1}t_{n}-K_{n}t_{n}\|\\&\le\frac{\omega_{n+1}}{1-\rho_{n+1}}[\|\gamma h(K_{n+1}\chi_{n+1})\|+\|AK_{n+1}t_{n+1}\|]\\&+\frac{\omega_n}{1-\rho_{n}}[\|\gamma h(K_{n}\chi_n)\|+\|AK_{n}t_{n}\|]\\&+\|t_{n+1}-t_{n}\| +\|K_{n+1}t_{n}-K_{n}t_{n}\|. \end{align} | (3.45) |
Now, calculating \|K_{n+1}t_{n}-K_{n}t_{n}\| for each j \in\{2, 3, ..., M-2\} , we get
\begin{align} \|T_{n+1, M-j}t_n-T_{n, M-j}t_n\|& = \|\eta_{n+1, M-j}S_{ M-j}T_{n+1, M-j-1}t_n+(1-\eta_{n+1, M-j})T_{n+1, M-j-1}t_n\\&-\eta_{n, M-j}S_{ M-j}T_{n, M-j-1}t_n-(1-\eta_{n, M-j})T_{n, M-j-1}t_n\|\\&\le \|\eta_{n+1, M-j}S_{ M-j}T_{n+1, M-j-1}t_n+\eta_{n+1, M-j}S_{ M-j}T_{n, M-j-1}t_n\\&-\eta_{n+1, M-j}S_{ M-j}T_{n, M-j-1}t_n+(1-\eta_{n+1, M-j})T_{n+1, M-j-1}t_n\\&-\eta_{n, M-j}S_{ M-j}T_{n, M-j-1}t_n+(1-\eta_{n+1, M-j})T_{n, M-j-1}t_n\\&-(1-\eta_{n+1, M-j})T_{n, M-j-1}t_n-(1-\eta_{n, M-j})T_{n, M-j-1}t_n\|\\&\le\eta_{n+1, M-j}\|S_{ M-j}T_{n+1, M-j-1}t_n-S_{ M-j}T_{n, M-j-1}t_n\|\\&+(1-\eta_{n+1, M-j})\|T_{n+1, M-j-1}t_n-T_{n, M-j-1}t_n\|\\&+|\eta_{n+1, M-j}-\eta_{n, M-j}|[\|T_{n, M-j-1}t_n\|+\|S_{ M-j}T_{n, M-j-1}t_n\|]\\&\le\|T_{n+1, M-j-1}t_n-T_{n, M-j-1}t_n\|+|\eta_{n+1, M-j}-\eta_{n, M-j}|M_4, \end{align} | (3.46) |
where M_4 = \sup\{\sum_{j = 2}^{M}\|S_jT_{n, j-1}\|+\|T_{n, j-1}t_n\|+\|S_1t_n\|+\|t_n\|\} .
Consider
\begin{align} \|T_{n+1, 1}t_n-T_{n, 1}t_n\|& = \|\eta_{n+1, 1}S_{1}t_n+(1-\eta_{n+1, 1})t_n-\eta_{n, 1}S_{1}t_n-(1-\eta_{n, 1})t_n\|\\&\le|\eta_{n+1, 1}-\eta_{n, 1}|[\|S_1t_n\|+\|t_n\|]\\&\le|\eta_{n+1, 1}-\eta_{n, 1}|M_4. \end{align} | (3.47) |
Also,
\begin{align} \|K_{n+1}t_n-K_{n}t_n\|& = \|T_{n+1, M}t_n-T_{n, M}t_n\|\\&\le\|T_{n+1, M-1}t_n-T_{n, M-1}t_n\|+M_4|\eta_{n+1, M}-\eta_{n, M}|\\&\le\|T_{n+1, M-2}t_n-T_{n, M-2}t_n\|\\&+M_4|\eta_{n+1, M}-\eta_{n, M}|+M_4|\eta_{n+1, M-1}-\eta_{n, M-1}|\\&\vdots\\&\le \|T_{n+1, 1}t_n-T_{n, 1}t_n\|+M_4\sum\limits_{j = 2}^{M}|\eta_{n+1, j}-\eta_{n, j}|\\&\le M_4\sum\limits_{j = 1}^{M}|\eta_{n+1, j}-\eta_{n, j}|. \end{align} | (3.48) |
From Eqs (3.44), (3.45) and (3.48), we get
\begin{align} \|f_{n+1}-f_{n}\|&\le\frac{\omega_{n+1}}{1-\rho_{n+1}}[\|\gamma h(K_{n+1}\chi_{n+1})\|+\|AK_{n+1}t_{n+1}\|]\\&+\frac{\omega_n}{1-\rho_{n}}[\|\gamma h(K_{n}\chi_n)\|+\|AK_{n}t_{n}\|]+\|\chi_{n+1}-\chi_n\|+\tau_{n+1}\|\chi_{n+1}-\chi_n\|\\&+\tau_n\|\chi_n-\chi_{n-1}\|+M_3\sqrt{|r_{n+1}-r_n|}+\frac{1}{c_1}\big|r_{n+1}-r_n\big|M_1\\&+(\varphi_{n+1}-\varphi_n)\|u_{n}-s_n\|+|\sigma_{n+1}-\sigma_{n}|\|U_{2}^nU_{1}^nu_{n}-Uu_n\|\\&+|\kappa_{n+1}^1-\kappa_{n}^1|J_1+|\kappa_{n+1}^2-\kappa_{n}^2|J_2+ M_4\sum\limits_{j = 1}^{M}|\eta_{n+1, j}-\eta_{n, j}|. \end{align} | (3.49) |
Using Remark 3.2, \lim\limits_{n\rightarrow +\infty}|\kappa_{n+1}^i-\kappa_{n}^i| = 0 , for i = 1, 2 , \lim\limits_{n\rightarrow +\infty}\omega_n = 0 , \lim\limits_{n\rightarrow +\infty}|r_{n+1}-r_n| = 0 , \lim\limits_{n\rightarrow +\infty}|\sigma_{n+1}-\sigma_{n}| = 0 , \lim\limits_{n\rightarrow +\infty}|\varphi_{n+1}-\varphi_n| = 0 and taking lim sup in Eq (3.49), we have
\begin{align*} \limsup\limits_{n \to +\infty} (\|f_{n+1}-f_{n}\|-\|\chi_{n+1}-\chi_n\|)\le0. \end{align*} |
Claim 3: \lim_{n\rightarrow +\infty}\|\chi_n-\chi_{n-1}\| = \lim\limits_{n\rightarrow +\infty}\|f_n-\chi_n\| = 0 and \lim_{n\rightarrow +\infty}\|t_n-u_{n}\| = \lim\limits_{n\rightarrow +\infty} \|w_n-\chi_n\| = \lim\limits_{n\rightarrow +\infty}\|\chi_n-K_nt_n\| = 0.
Using Lemma 2.15, we have
\begin{align} \lim\limits_{n\rightarrow +\infty}\|f_n-\chi_n\| = 0. \end{align} | (3.50) |
Also, \chi_{n+1} = (1-\rho_n)f_n+\rho_n\chi_n , which implies \|\chi_{n+1}-\chi_n\| = \|(1-\rho_n)(f_n-\chi_n)\| . Now using Eq (3.50), we have
\begin{align} \lim\limits_{n\rightarrow +\infty}\|\chi_{n+1}-\chi_n\| = 0. \end{align} | (3.51) |
From Eq (3.2), we have \|w_n-\chi_n\| = \|\tau_n(\chi_n-\chi_{n-1})\| . Taking the limit n \to +\infty , we get
\begin{align} \lim\limits_{n\rightarrow +\infty} \|w_n-\chi_n\| = 0. \end{align} | (3.52) |
Also,
\begin{align*} \|\chi_n-K_nt_n\|& = \|\chi_n-\chi_{n+1}+\chi_{n+1}-K_nt_n\|\nonumber\\&\le\|\chi_n-\chi_{n+1}\|+\|\omega_n\gamma h(K_n\chi_n)+\rho_n\chi_n\nonumber\\&+[(1-\rho_n)I-\omega_nA]K_nt_n-K_nt_n\|\nonumber\\&\le\|\chi_n-\chi_{n+1}\|+\omega_n\|\gamma h(K_n\chi_n)-AK_nt_n\|+\rho_n\|\chi_n-K_nt_n\|, \end{align*} |
which implies
\begin{align} (1-\rho_n) \|\chi_n-K_nt_n\|&\le\|\chi_n-\chi_{n+1}\|+\omega_n\|\gamma h(K_n\chi_n)-AK_nt_n\|. \end{align} | (3.53) |
Taking the limit n\to +\infty and using \lim\limits_{n\rightarrow +\infty}\omega_n = 0 , we have
\begin{align} \lim\limits_{n\rightarrow +\infty}\|\chi_n-K_nt_n\| = 0. \end{align} | (3.54) |
Consider
\begin{align} \|\chi_{n+1}-\varrho\|^2& = \| \omega_n\gamma h(K_n\chi_n)+\rho_n\chi_n+[(1-\rho_n)I-\omega_nA]K_nt_n-\varrho\|^2\\& \le\|(1-\omega_nA)(K_nt_n-\varrho)+\rho_n(\chi_n-K_nt_n)\|^2\\&+2\omega_n \langle \gamma h(K_n\chi_n)-A\varrho, \chi_{n+1}-\varrho\rangle\\&\le(1-\omega_n\bar{\gamma})^2\|t_n-\varrho\|^2+\rho_n^2\|\chi_n-K_nt_n\|^2\\&+2\rho_n(1-\omega_n\bar{\gamma})\|t_n-\varrho\|\|\chi_n-K_nt_n\|+2\omega_n\langle \gamma h(K_n\chi_n)-A\varrho, \chi_{n+1}-\varrho\rangle. \end{align} | (3.55) |
Also,
\begin{align} \|y_n-\varrho\|^2& = \|T_{r_n}^{F_1}(I-r_ng_1)w_n-T_{r_n}^{F_1}(I-r_ng_1)\varrho\|^2\\&\le \langle y_n-\varrho, (I-r_ng_1)w_n-(I-r_ng_1)\varrho \rangle\\& = \frac{1}{2}\bigg[\|y_n-\varrho\|^2+\|(I-r_ng_1)w_n-(I-r_ng_1)\varrho\|^2\\&-\|y_n-\varrho-((I-r_ng_1)w_n-(I-r_ng_1)\varrho)\|^2\bigg]\\&\le \|(I-r_ng_1)w_n-(I-r_ng_1)\varrho\|^2-\|(y_n-w_n)-r_n(g_1(w_n)-g_1(\varrho))\|^2, \end{align} | (3.56) |
which implies
\begin{align} \|y_n-\varrho\|^2\le\|w_n-\varrho\|^2-\{\|w_n-y_n\|^2-2r_n\|w_n-y_n\|\|g_1w_n-g_1\varrho\|\}. \end{align} | (3.57) |
Using Eq (3.14), we have
\begin{align} \|u_n-\varrho\|^2& = \|y_n+\delta D^*(l_n-Dy_n)-\varrho\|^2\\&\le \langle u_n-\varrho, y_n-\delta D^*(Dy_n-l_n)-\varrho \rangle\\& = \frac{1}{2}\bigg[\|u_n-\varrho\|^2+\|y_n-\delta D^*(Dy_n-l_n)-\varrho\|^2-\|u_n-\varrho-(y_n-\delta D^*(Dy_n-l_n)-\varrho)\|^2\bigg]\\& \le \frac{1}{2}\bigg[\|u_n-\varrho\|^2+\|y_n-\varrho\|^2-\|u_n-\varrho-(y_n-\delta D^*(Dy_n-l_n)-\varrho)\|^2\bigg]\\& = \frac{1}{2}\bigg[\|u_n-\varrho\|^2+\|y_n-\varrho\|^2-\{\|u_n-y_n\|^2+\delta^2\| D^*(l_n-Dy_n)\|^2-2\delta\langle u_n-y_n, D^*(Dy_n-l_n)\rangle \}\bigg], \end{align} | (3.58) |
which implies
\begin{align} \|u_n-\varrho\|^2\le\|y_n-\varrho\|^2-\|u_n-y_n\|^2+2\delta\|Dy_n-l_n\|\|u_n-y_n\|\| D^*\|. \end{align} | (3.59) |
Using Eqs (3.11), (3.14), (3.17) and (3.55), we get
\begin{align} \|\chi_{n+1}-\varrho\|^2& = (1-\omega_n\bar{\gamma})^2(\|u_n-\varrho\|+\varphi_n\sigma_n\|U\varrho-\varrho\|)^2+\rho_n^2\|\chi_n-K_nt_n\|^2\\&+2\rho_n(1-\omega_n\bar{\gamma})\|t_n-\varrho\|\|\chi_n-K_nt_n\|\\&+2\omega_n\langle h(K_n\chi_n)-A\varrho, \chi_{n+1}-\varrho\rangle\\&\le(1-\omega_n\bar{\gamma})^2(\|u_n-\varrho\|^2+\varphi_n^2\sigma_n^2\|U\varrho-\varrho\|^2\\&+2\varphi_n\sigma_n\|U\varrho-\varrho\|\|u_n-\varrho\|)+\rho_n^2\|\chi_n-K_nt_n\|^2\\&+2\rho_n(1-\omega_n\bar{\gamma})\|t_n-\varrho\|\|\chi_n-K_nt_n\|+2\omega_n\langle h(K_n\chi_n)-A\varrho, \chi_{n+1}-\varrho\rangle \end{align} | (3.60) |
\begin{align} & \le(1-\omega_n\bar{\gamma})^2(\|w_n-\varrho\|^2-\delta(1-\delta L)\|l_n-Dy_n\|^2)\\&+\varphi_n^2\sigma_n^2\|U\varrho-\varrho\|^2+2\varphi_n\sigma_n\|U\varrho-\varrho\|\|u_n-\varrho\|\\&+\rho_n^2\|\chi_n-K_nt_n\|^2+2\rho_n(1-\omega_n\bar{\gamma})\|t_n-\varrho\|\|\chi_n-K_nt_n\|\\&+2\omega_n\langle h(K_n\chi_n)-A\varrho, \chi_{n+1}-\varrho\rangle. \end{align} | (3.61) |
Using Eqs (3.20) and (3.60), we get
\begin{align} (1-\omega_n\bar{\gamma})^2\delta(1-\delta L)\|l_n-Dy_n\|^2&\le\|\chi_n-\varrho\|^2-\|\chi_{n+1}-\varrho\|^2+(\omega_n\bar{\gamma})^2\|\chi_n-\varrho\|^2\\&+(1-\omega_n\bar{\gamma})^2 (\tau_n\|\chi_n-\chi_{n-1}\|M\\&+\varphi_n^2\sigma_n^2\|U\varrho-\varrho\|^2+2\varphi_n\sigma_n\|U\varrho-\varrho\|\|u_n-\varrho\|)\\&+\rho_n^2\|\chi_n-K_nt_n\|^2\\&+2\rho_n(1-\omega_n\bar{\gamma})\|t_n-\varrho\|\|\chi_n-K_nt_n\|\\&+2\omega_n\langle h(K_n\chi_n)-A\varrho, \chi_{n+1}-\varrho\rangle\\&\le(\|\chi_n-\varrho\|+\|\chi_{n+1}-\varrho\|)\|\chi_n-\chi_{n+1}\|\\&+(\omega_n\bar{\gamma})^2\|\chi_n-\varrho\|^2+(1-\omega_n\bar{\gamma})^2 (\tau_n\|\chi_n-\chi_{n-1}\|M\\&+\varphi_n^2\sigma_n^2\|U\varrho-\varrho\|^2+2\varphi_n\sigma_n\|U\varrho-\varrho\|\|u_n-\varrho\|)\\&+\rho_n^2\|\chi_n-K_nt_n\|^2\\&+2\rho_n(1-\omega_n\bar{\gamma})\|t_n-\varrho\|\|\chi_n-K_nt_n\|\\&+2\omega_n\langle h(K_n\chi_n)-A\varrho, \chi_{n+1}-\varrho\rangle \end{align} | (3.62) |
and using Eqs (3.20), (3.59) and (3.60), we estimate
\begin{align} (1-\omega_n\bar{\gamma})^2\|u_n-y_n\|^2&\le \|\chi_n-\varrho\|^2- \|\chi_{n+1}-\varrho\|^2+\rho_n^2\|\chi_n-K_nt_n\|^2\\&+ (1-\omega_n\bar{\gamma})^2 (2\delta\|Dy_n-l_n\|\|u_n-y_n\|\| D^*\|+\varphi_n^2\sigma_n^2\|U\varrho-\varrho\|^2\\&+2\varphi_n\sigma_n\|U\varrho-\varrho\|\|u_n-\varrho\|)+2\rho_n(1-\omega_n\bar{\gamma})\|t_n-\varrho\|\|\chi_n-K_nt_n\|\\&+2\omega_n\langle h(K_n\chi_n)-A\varrho, \chi_{n+1}-\varrho\rangle\\&\le (\|\chi_n-\varrho\|+\|\chi_{n+1}-\varrho\|)\|\chi_n-\chi_{n+1}\|+\rho_n^2\|\chi_n-K_nt_n\|^2\\&+ (1-\omega_n\bar{\gamma})^2(2 \delta\|Dy_n-l_n\|\|u_n-y_n\|\| D^*\|+\varphi_n^2\sigma_n^2\|U\varrho-\varrho\|^2\\&+2\varphi_n\sigma_n\|U\varrho-\varrho\|\|u_n-\varrho\|)+2\rho_n(1-\omega_n\bar{\gamma})\|t_n-\varrho\|\|\chi_n-K_nt_n\|\\&+2\omega_n\langle h(K_n\chi_n)-A\varrho, \chi_{n+1}-\varrho\rangle. \end{align} | (3.63) |
Using Eqs (3.14), (3.20) and (3.57), we have
\begin{align} (1-\omega_n\bar{\gamma})^2r_n(2\alpha-r_n)\|g_1(w_n)-g_1(\varrho)\|^2&\le \|\chi_n-\varrho\|^2- \|\chi_{n+1}-\varrho\|^2+(\omega_n\bar{\gamma})^2\|\chi_n-\varrho\|^2\\&+(1-\omega_n\bar{\gamma})^2( \tau_n\|\chi_n-\chi_{n-1}\|M+\varphi_n^2\sigma_n^2\|U\varrho-\varrho\|^2\\&+2\varphi_n\sigma_n\|U\varrho-\varrho\|\|u_n-\varrho\|)+\rho_n^2\|\chi_n-K_nt_n\|^2\\&+2\rho_n(1-\omega_n\bar{\gamma})\|t_n-\varrho\|\|\chi_n-K_nt_n\|\\&+2\omega_n\langle h(K_n\chi_n)-A\varrho, \chi_{n+1}-\varrho\rangle\\&\le (\|\chi_n-\varrho\|+\|\chi_{n+1}-\varrho\|)\|\chi_n-\chi_{n+1}\|\\&+(\omega_n\bar{\gamma})^2\|\chi_n-\varrho\|^2\\&+(1-\omega_n\bar{\gamma})^2 (\tau_n\|\chi_n-\chi_{n-1}\|M\\&+\varphi_n^2\sigma_n^2\|U\varrho-\varrho\|^2\\&+2\varphi_n\sigma_n\|U\varrho-\varrho\|\|u_n-\varrho\|)\\&+\rho_n^2\|\chi_n-K_nt_n\|^2\\&+2\rho_n(1-\omega_n\bar{\gamma})\|t_n-\varrho\|\|\chi_n-K_nt_n\|\\&+2\omega_n\langle h(K_n\chi_n)-A\varrho, \chi_{n+1}-\varrho\rangle. \end{align} | (3.64) |
Using Eqs (3.20), (3.60) and (3.57), we have
\begin{align} (1-\omega_n\bar{\gamma})^2\|w_n-y_n\|^2&\le \|\chi_n-\varrho\|^2- \|\chi_{n+1}-\varrho\|^2\\&+\rho_n^2\|\chi_n-K_nt_n\|^2+(\omega_n\bar{\gamma})^2\|\chi_n-\varrho\|^2\\&+(1-\omega_n\bar{\gamma})^2 [\tau_n\|\chi_n-\chi_{n-1}\|M+2r_n\|w_n-y_n\|\|g_1(w_n)-g_1(\varrho)\|\\&+\varphi_n^2\sigma_n^2\|U\varrho-\varrho\|^2+2\varphi_n\sigma_n\|U\varrho-\varrho\|\|u_n-\varrho\|]\\&+\rho_n^2\|\chi_n-K_nt_n\|^2+2\rho_n(1-\omega_n\bar{\gamma})\|t_n-\varrho\|\|\chi_n-K_nt_n\|\\&+2\omega_n\langle h(K_n\chi_n)-A\varrho, \chi_{n+1}-\varrho\rangle\\&\le (\|\chi_n-\varrho\|+\|\chi_{n+1}-\varrho\|)\|\chi_n-\chi_{n+1}\|+\rho_n^2\|\chi_n-K_nt_n\|^2\\&+\varphi_n^2\sigma_n\|U\varrho-\varrho\|^2\\&+2\varphi_n^2\sigma_n\|U\varrho-\varrho\|+\rho_n^2\|\chi_n-K_nt_n\|^2+(\omega_n\bar{\gamma})^2\|\chi_n-\varrho\|^2\\&+2(1-\omega_n\bar{\gamma})^2r_n\|w_n-y_n\|\|g_1(w_n)-g_1(\varrho)\|\\&+(1-\omega_n\bar{\gamma})^2( \tau_n\|\chi_n-\chi_{n-1}\|M+\varphi_n^2\sigma_n^2\|U\varrho-\varrho\|^2\\&+2\varphi_n\sigma_n\|U\varrho-\varrho\|\|u_n-\varrho\|)\\&+\rho_n^2\|\chi_n-K_nt_n\|^2+2\rho_n(1-\omega_n\bar{\gamma})\|t_n-\varrho\|\|\chi_n-K_nt_n\|\\&+2\omega_n\langle h(K_n\chi_n)-A\varrho, \chi_{n+1}-\varrho\rangle. \end{align} | (3.65) |
From Eqs (3.54), (3.62) and (3.64) and using \lim_{n\rightarrow +\infty}\sigma_n < +\infty , \lim_{n\rightarrow +\infty}\|\chi_n-\chi_{n-1}\| = 0 , \lim_{n\rightarrow +\infty}\omega_n = 0 and Remark 3.2, we get
\begin{align} \lim\limits_{n\rightarrow +\infty} \|l_n-Dy_n\| = 0 \;\text{and}\; \lim\limits_{n\rightarrow +\infty}\|g_1(w_n)-g_1(\varrho)\| = 0. \end{align} | (3.66) |
Using Eqs (3.54), (3.62) and (3.66) and using \lim_{n\rightarrow +\infty}\sigma_n < +\infty , \lim_{n\rightarrow +\infty}\|\chi_n-\chi_{n-1}\| = 0 , \lim_{n\rightarrow +\infty}\omega_n = 0 and Remark 3.2, we get
\begin{align} \lim\limits_{n\rightarrow +\infty} \|u_n-y_n\| = 0\;\text{and}\; \lim\limits_{n\rightarrow +\infty}\|w_n-y_n\| = 0. \end{align} | (3.67) |
Using the triangle inequality and Eqs (3.52) and (3.67), we get
\begin{align} \lim\limits_{n\rightarrow +\infty} \|u_n-\chi_n\| = 0. \end{align} | (3.68) |
As we know that U and U_{2}^nU_{1}^n are nonexpansive mappings and \{u_n\} is bounded, one may suppose that there is a nonnegative real number k such that \|Uu_n-U_{2}^nU_{1}^nu_n\|\le k for all n\ge0 . Now, consider
\begin{align} \|t_n-U_{2}^nU_{1}^nu_n\|& = \|(1-\varphi_n)u_n+\varphi_n[\sigma_nUu_n+(1-\sigma_n)U_{2}^nU_{1}^nu_n]-U_{2}^nU_{1}^nu_n\|\\&\le(1-\varphi_n)\|u_n-U_{2}^nU_{1}^nu_n\|+\varphi_n\sigma_n\|Uu_n-U_{2}^nU_{1}^nu_n\|\\&\le(1-\varphi_n)\|u_n-t_n\|+(1-\varphi_n)\|t_n-U_{2}^nU_{1}^nu_n\|+\varphi_n\sigma_n\|Uu_n-U_{2}^nU_{1}^nu_n\|. \end{align} | (3.69) |
Subsequently, we have
\begin{align} \|t_n-U_{2}^nU_{1}^nu_n\|\le\frac{(1-\varphi_n)}{\varphi_n}\|u_n-t_n\|+\sigma_nk. \end{align} | (3.70) |
Using condition (iii) and \lim_{n\rightarrow +\infty}\sigma_n < +\infty , we get
\begin{align} \lim\limits_{n\rightarrow +\infty}\|t_n-U_{2}^nU_{1}^nu_n\| = 0. \end{align} | (3.71) |
Also,
\begin{align} \|t_n-u_n\|& = \|(1-\varphi_n)u_n+\varphi_n[\sigma_nUu_n+(1-\sigma_n)U_{2}^nU_{1}^nu_n]-u_n\|\\&\le\varphi_n\sigma_n\|Uu_n-u_n\|+(1-\sigma_n)\varphi_n\|U_{2}^nU_{1}^nu_n-u_n\|\\&\le\varphi_n\sigma_n[\|Uu_n-t_n\|+\|t_n-u_n\|]+(1-\sigma_n)\varphi_n[\|U_{2}^nU_{1}^nu_n-t_n\|+\|t_n-u_n\|]. \end{align} | (3.72) |
Hence, we have
\begin{align} (1-\varphi_n)\|t_n-u_n\|&\le \sigma_n\|Uu_n-t_n\|+(1-\sigma_n)\|U_{2}^nU_{1}^nu_n-t_n\|. \end{align} | (3.73) |
From Eq (3.71) and \lim_{n\rightarrow +\infty}\sigma_n = 0 , we have
\begin{align} \lim\limits_{n\rightarrow +\infty}\|t_n-u_n\| = 0. \end{align} | (3.74) |
Claim 4: u' \in GMEP(F_1, g_1, \psi_1, Q_1) .
As we know that \{u_n\} is bounded, there exists a subsequence \{u_{n_i}\} of \{u_n\} that converges weakly to some u'\in Q_1 . Also, from Eq (3.54), we have K_nt_{n_i}\rightharpoonup u' . Now, we show u' \in GMEP(F_1, g_1, \psi_1, Q_1) . Using Lemma 2.13, we have
\begin{align*} F_1(u_n, z)+\langle g_1(y_n), z-u_n \rangle+ \psi_1(z)-\psi_1(u_n)+\frac{1}{r_n}\langle z-u_n, u_n-y_n\rangle \geq0\text{ for all } z\in Q_1. \end{align*} |
Using the monotonicity of F_1 , we have
\begin{align*} \langle g_1(y_n), z-u_n \rangle+ \psi_1(z)-\psi_1(u_n)+\frac{1}{r_n}\langle z-u_n, u_n-y_n\rangle \geq F_1(z, u_n)\text{ for all } z\in Q_1. \end{align*} |
Replacing n by n_k , we have
\begin{align*} \langle g_1(z_{n_k}), z-u_{n_k} \rangle+ \psi_1(z)-\psi_1(u_{n_k})+\frac{1}{r_{n_k}}\langle z-u_{n_k}, u_{n_k}-z_{n_k}\rangle \geq F_1(z, u_{n_k})\text{ for all } z\in Q_1. \end{align*} |
Let m with 0 < m \le 1 and u \in Q_1 satisfying u_m = mu+(1-m)u' , then u_m \in Q_1 and, from the above inequality, we have
\begin{align} \langle u_m-u_{n_k}, g_1(u_m) \rangle&\geq \langle u_m-u_{n_k}, g_1(u_m) \rangle+ \psi_1(u_{n_k})-\psi_1(u_m)- \langle g_1(z_{n_k}), z-u_{n_k} \rangle\\&+\langle u_m-u_{n_k}, \frac{1}{r_{n_k}}(u_{n_k}-z_{n_k})\rangle + F_1(u_m, u_{n_k}) \\& = \langle u_m-u_{n_k}, g_1(u_m)-g_1(u_{n_k}) \rangle+\langle u_m-u_{n_k}, g_1(u_{n_k})-g_1(z_{n_k}) \rangle\\&+\psi_1(u_{n_k})-\psi_1(u_m)+\langle u_m-u_{n_k}, \frac{1}{r_{n_k}}(u_{n_k}-z_{n_k})\rangle + F_1(u_m, u_{n_k}). \end{align} | (3.75) |
Using the Lipschitz continuity of g_1 and Eq (3.67), we have \|g_1u_{n_k}-g_1z_{n_k}\| = 0 as k\to +\infty . Further, as F_1 is monotone and \phi_1 is convex and lower semicontinuous, the above equation implies
\begin{align} \langle u_m-u', g_1(u_m) \rangle&\geq F_1(u_m, u')+\psi_1(u')-\psi_1(u_m). \end{align} | (3.76) |
Consider for m > 0
\begin{align} 0& = F_1(u_m, u_m)\le m F_1(u_m, u)+(1-m) F_1(u_m, u')\\&\le mF_1(u_m, u)+(1-m)(\langle u_m-u', g_1(u_m) \rangle-\psi_1(u')+\psi_1(u_m))\\& \le mF_1(u_m, u)+(1-m)m(\langle u-u', g_1(u_m) \rangle-\psi_1(u')+\psi_1(u)). \end{align} | (3.77) |
Taking m \to 0_+ , we get
\begin{align} F_1(u', u)+\langle u-u', g_1(u') \rangle-\psi_1(u')+\psi_1(u) \ge 0 \;\text{for all}\;u\in Q_1. \end{align} | (3.78) |
Hence, u' \in GMEP(F_1, g_1, \psi_1, Q_1) .
Claim 5: Now we will prove Du' \in GMEP(F_2, g_2, \psi_2, Q_2) .
As D is a bounded linear operator, and using Eqs (3.66) and (3.67), this implies Dz_{n_k}\rightharpoonup Du' . Taking l'_{n_k} = Dz_k-T_{r_{n_k}}^{F_2}(I-r_{n_k}g_2)Dz_k and using Eq (3.66) we have \lim_{n\rightarrow +\infty}l'_{n_k} = 0 and T_{r_{n_k}}^{F_2}(I-r_{n_k}g_2)Dz_k = Dz_k-l'_{n_k} . Now, using Lemma 2.13, we get
\begin{align} F_2(Dz_{n_k}-l'_{n_k}, s)+\langle s-Dz_{n_k}+l'_{n_k}, g_2(z_{n_k})\rangle-\psi_2(Dz_{n_k}-l'_{n_k})+\psi_2(s)&\\+\frac{1}{r_{n_k}}\langle s-(Dz_{n_k}-l'_{n_k}), Dz_{n_k}-l'_{n_k}-Dz_{n_k}\rangle& \ge 0, \;\text{for all}\;s\in Q_2. \end{align} | (3.79) |
As F_2 is upper semicontinuous, we use \limsup in the above equation as k\to +\infty . Also, with \liminf_{n \to +\infty} r_n > 0 , we have
\begin{align} F_2(Du', s)+\langle s-Du', g_2(u') \rangle-\psi_2(Du')+\psi_2(s) \ge 0 \;\text{for all}\;s\in Q_2. \end{align} | (3.80) |
Hence, Du' \in GMEP(F_2, g_2, \psi_2, Q_2) .
Claim 6: Now we will prove u' \in Fix (K) .
Assume that K is the K -mappings generated by S_1, S_2, ..., S_M and \eta_1, \eta_2, ..., \eta_M . Now, using Lemma 2.16, we have
\begin{align*} K_{n_j}x\to Kx \;\text{and}\;Fix(K) = {\bigcap^M_{j = 1} {\rm{Fix}}(S_j)}. \end{align*} | (3.81) |
We have to show u'\in Fix( K ). We will do it by contradiction. Assume that u'\notin Fix( K ), which implies Ku'\neq u' . Now using opial conditions, we get
\begin{align} \liminf\limits_{j \to +\infty}\|t_{n_j}-u'\|& < \liminf\limits_{j \to +\infty}\|t_{n_j}-Ku'\|\\&\le\liminf\limits_{j \to +\infty}\|t_{n_j}-K_{n_j}t_{n_j}\|+\|K_{n_j} t_{n_j}-K_{n_j}u'\|+\|K_{n_j}u'-Ku'\|\\&\le\liminf\limits_{j \to +\infty}\|K_{n_j}t_{n_j}-K_{n_j}u'\|\\&\le\liminf\limits_{j \to +\infty}\|t_{n_j}-u'\|, \end{align} | (3.82) |
which is a contradiction. Thus, u'\in Fix( K ) = {\bigcap^M_{j = 1} {\rm{Fix}}(S_j)} .
Claim 7: We claim that u' \in {\rm{Fix}}(U_1)\cap {\rm{Fix}}(U_2) . As the sequence \{\chi_n\} is bounded, then there is a subsequence \{x_{n_k}\} of \{\chi_n\} such that \{x_{n_k}\}\rightharpoonup u' as k\to +\infty. Also, \kappa_{n}^i is bounded, which implies \kappa_{n_k}^i\to \kappa_{+\infty}^i for i = 1, 2 and k\to +\infty , where 0 < \kappa_{+\infty}^i < 1 . Consider U_{i}^{+\infty} = (1- \kappa_{+\infty}^i)I+ \kappa_{+\infty}^iP_{Q_1}(\zeta_{+\infty}^iI+(1-\zeta_{+\infty}^i)U_i) for i = 1, 2 . Using Lemma 2.11, we conclude that {\rm{Fix}}(P_{Q_1}(\zeta_{+\infty}^iI+(1-\zeta_{+\infty}^i)U_i)) = {\rm{Fix}}(U_i). As P_{Q_1}(\zeta_{+\infty}^iI+(1-\zeta_{+\infty}^i)U_i) is a nonexpansive mapping, {\rm{Fix}}(U_i^{+\infty}) = {\rm{Fix}}(U_i) and U_i^{+\infty} is averaged. Further,
\begin{align} {\rm{Fix}}(U_1^{+\infty})\cap {\rm{Fix}}(U_2^{+\infty}) = {\rm{Fix}}(U_1)\cap {\rm{Fix}}(U_2) = {\rm{Fix}}(U)\ne \phi. \end{align} | (3.83) |
Using Lemma 2.9, we get
\begin{align} {\rm{Fix}}(U_1^{+\infty}U_2^{+\infty}) = {\rm{Fix}}(U_1^{+\infty})\cap {\rm{Fix}}(U_2^{+\infty}) = {\rm{Fix}}(U)\ne \phi. \end{align} | (3.84) |
Additionally,
\begin{align} \|U_i^{n_k}s-U_i^{+\infty}s\|\le |\kappa_{n_k}^i-\kappa_{+\infty}^i|(\|s\|+\|P_{Q_1}(\zeta_{n}^is+(1-\zeta_{n}^iU_i(s))\|). \end{align} | (3.85) |
Subsequently, we get
\begin{align} \lim\limits_{j \to +\infty}\sup\limits_{s\in K} \|U_i^{n_k}s-U_i^{+\infty}s\| = 0, \end{align} | (3.86) |
where K is any bounded subset of H_1 . Note that
\begin{align} \|x_{n_k}-U_2^{+\infty}U_1^{+\infty}x_{n_k}\|&\le \|x_{n_k}-U_2^{n_k}U_1^{n_k}x_{n_k}\|+\|U_2^{n_k}U_1^{n_k}x_{n_k}-U_2^{+\infty}U_1^{n_k}x_{n_k}\|\\&+\|U_2^{+\infty}U_1^{n_k}x_{n_k}-U_2^{+\infty}U_1^{+\infty}x_{n_k}\|\\&\le\|x_{n_k}-U_2^{n_k}U_1^{n_k}x_{n_k}\|+\|U_2^{n_k}U_1^{n_k}x_{n_k}-U_2^{+\infty}U_1^{n_k}x_{n_k}\|\\&+\|U_2^{+\infty}U_1^{n_k}x_{n_k}-U_2^{+\infty}U_1^{+\infty}x_{n_k}\|\\&\le\|x_{n_k}-U_2^{n_k}U_1^{n_k}x_{n_k}\|+\sup\limits_{s\in K_1}\|U_2^{n_k}s-U_2^{+\infty}s\|\\&+\sup\limits_{s\in K_2}\|U_1^{n_k}s-U_1^{+\infty}s\|, \end{align} | (3.87) |
where K_1 and K_2 are bounded subsets including \{U_1^{n_k}x_{n_k}\} and \{x_{n_k}\} respectively. From Eqs (3.71), (3.86) and (3.87), we conclude that
\begin{align} \lim\limits_{k \to +\infty}\|x_{n_k}-U_2^{+\infty}U_1^{+\infty}x_{n_k}\| = 0. \end{align} | (3.88) |
Subsequently, using Lemma 2.17, we get u'\in {\rm{Fix}}(U_1^{+\infty}U_2^{+\infty}) = {\rm{Fix}}(U_1)\cap {\rm{Fix}}(U_2) .
Claim 8: Next, we will show u' \in\Omega . From Eq (3.3), we get
\begin{align} t_n-u_n = \varphi_n[\sigma_n(U-I)u_n+(1-\sigma_n)(U_{2}^nU_{1}^nu_n-u_n)] \end{align} | (3.89) |
and hence
\begin{align} \frac {1}{\varphi_n\sigma_n}(u_n-t_n) = (I-U)u_n+(1-\sigma_n)(I-U_{2}^nU_{1}^n)u_n. \end{align} | (3.90) |
Using Lemma 2.14 ( i ), the sequence \bigg\{\frac{(1-\sigma_n)}{\sigma_n}(I-U_{2}^nU_{1}^n)\bigg\} is graph convergent to N_{ {\rm{Fix}}(U_1)\cap {\rm{Fix}}(U_2)} , and using Lemma 2.14 ( ii ), one can conclude that the sequence (I-U)+\bigg\{\frac{(1-\sigma_n)}{\sigma_n}(I-U_{2}^nU_{1}^n)\bigg\} is graph convergent to (I-U)+N_{ {\rm{Fix}}(U_1)\cap {\rm{Fix}}(U_2)} . Replacing n by n_j and taking the limit j \to +\infty in Eq (3.90) and using condition ( iii ), we have
By substituting n_j for n and taking the limit as j tends to infinity in Eq (3.90) while utilizing condition ( iii ), we obtain:
\begin{align} 0\in (I-U)u'+N_{ {\rm{Fix}}(U_1)\cap {\rm{Fix}}(U_2)}u', \end{align} | (3.91) |
which implies u' \in\Omega . From Claims 5–8, we have u' \in\Gamma .
Claim 9: Now we show \limsup_{n \to +\infty}\langle (\gamma h-A)u', \chi_n-v \rangle \le 0 , where u' = P_{\Gamma}(I+\gamma h-A)u' . As the sequence \{t_n\} weakly converges to u' and using Lemma 2.8, we have
\begin{align} \limsup\limits_{n \to +\infty}\langle (\gamma h-A)\varrho, \chi_n-\varrho \rangle& = \limsup\limits_{n \to +\infty}\langle (\gamma h-A)\varrho, K_nt_n-\varrho \rangle\\&\le\limsup\limits_{n \to +\infty}\langle (\gamma h-A)\varrho, t_n-\varrho \rangle\\& = 0. \end{align} | (3.92) |
As h is a contraction mapping, one can easily prove P_{\Gamma}(I+\gamma h-A) is also a contraction mapping from H_1 to itself. Using the Banach contraction principle, there exists a u'\in H_1 such that u' = P_{\Gamma}(I+\gamma h-A)u' .
Claim 10: Next we show \chi_n \to \varrho .
Consider
\begin{align} \|\chi_{n+1}-\varrho\|^2& = \langle \omega_n(\gamma h(K_n\chi_n)-A\varrho)+\rho_n(\chi_n-\varrho), \chi_{n+1}-\varrho \rangle\\&+\langle[(1-\rho_n)I-\omega_nA](K_nt_n-\varrho) , \chi_{n+1}-\varrho \rangle\\&\le \omega_n\langle\gamma h(K_n\chi_n)-A\varrho, \chi_{n+1}-\varrho \rangle+ \rho_n\langle \chi_n-\varrho, \chi_{n+1}-\varrho \rangle\\&+[(1-\rho_n)I-\omega_nA]\langle K_nt_n-\varrho , \chi_{n+1}-\varrho \rangle\\&\le \omega_n\langle\gamma h(K_n\chi_n)-A\varrho, \chi_{n+1}-\varrho \rangle\\&+ \rho_n \| \chi_n-\varrho\|\|\chi_{n+1}-\varrho\| +[(1-\rho_n)I-\omega_n\bar{\gamma}]\| K_nt_n-\varrho\| \|\chi_{n+1}-\varrho\|\\&\le \omega_n\langle\gamma h(K_n\chi_n)-\gamma h\varrho, \chi_{n+1}-\varrho \rangle+\omega_n\langle\gamma h\varrho-A\varrho, \chi_{n+1}-\varrho \rangle\\&+ \rho_n \| \chi_n-\varrho\|\|\chi_{n+1}-\varrho\| +[(1-\rho_n)I-\omega_n\bar{\gamma}]\| t_n-\varrho\| \|\chi_{n+1}-\varrho\|\\&\le \omega_n\gamma\nu \|\chi_n-\varrho\|\| \chi_{n+1}-\varrho\| +\omega_n\langle\gamma h\varrho-A\varrho, \chi_{n+1}-\varrho \rangle\\&+ \rho_n \| \chi_n-\varrho\|\|\chi_{n+1}-\varrho\| +[(1-\rho_n)I\\&-\omega_n\bar{\gamma}]\|\chi_{n+1}-\varrho\|(\|\chi_n-\varrho\|+\tau_n\|\chi_n-\chi_{n-1}\|+\varphi_n\sigma_n\|U\varrho-\varrho\|)\\&\le (1-\omega_n(\bar{\gamma}-\gamma\nu)) \|\chi_n-\varrho\|\| \chi_{n+1}-\varrho\| +\omega_n\langle\gamma h\varrho-A\varrho, \chi_{n+1}-\varrho \rangle\\& +[(1-\rho_n)I-\omega_n\bar{\gamma}]\|\chi_{n+1}-\varrho\|(\tau_n\|\chi_n-\chi_{n-1}\|+\varphi_n\sigma_n\|U\varrho-\varrho\|)\\&\le (1-\omega_n(\bar{\gamma}-\gamma\nu))\frac{1}{2} [\|\chi_n-\varrho\|^2+\| \chi_{n+1}-\varrho\|^2] \\&+\omega_n\langle\gamma h\varrho-A\varrho, \chi_{n+1}-\varrho \rangle\\& +[1-\rho_n-\omega_n\bar{\gamma}]\|\chi_{n+1}-\varrho\|(\tau_n\|\chi_n-\chi_{n-1}\|+\varphi_n\sigma_n\|U\varrho-\varrho\|), \end{align} | (3.93) |
which implies
\begin{align} \|\chi_{n+1}-\varrho\|^2&\le (1-\omega_n(\bar{\gamma}-\gamma\nu))\|\chi_n-\varrho\|^2\\&+\frac{1}{(\bar{\gamma}-\gamma\nu)}(\bar{\gamma}-\gamma\nu)\omega_n\langle\gamma h\varrho-A\varrho, \chi_{n+1}-\varrho \rangle\\& +M_5(\tau_n\|\chi_n-\chi_{n-1}\|+\sigma_n\|U\varrho-\varrho\|), \end{align} | (3.94) |
where M_5 = \sup\{\|\chi_n-\varrho\|: n\in \mathbb{N}\} . Hence, we get
\begin{align} a_{n+1}\le(1-b_n)a_n+d_n+c_n, \end{align} | (3.95) |
where a_n = \|\chi_n-\varrho\|^2 , b_n = \omega_n(\bar{\gamma}-\gamma\nu) , d_n = \frac{1}{(\bar{\gamma}-\gamma\nu)}(\bar{\gamma}-\gamma\nu)\omega_n\langle\gamma h\varrho-A\varrho, \chi_{n+1}-\varrho \rangle and c_n = M_5(\tau_n\|\chi_n-\chi_{n-1}\|+\sigma_n\|U\varrho-\varrho\|) . From Remark (3.2) and \sum_{n = 0}^{+\infty}\sigma_n < +\infty , we have \sum_{n = 0}^{+\infty}c_n < +\infty . From Eq (3.92), we get \limsup_{n \to +\infty}\frac{d_n}{b_n} \le 0 . Also, \sum_{n = 0}^+\infty b_n = +\infty and from Lemma 2.12 ( ii ), we obtain
\begin{align} \lim\limits_{n\rightarrow +\infty} a_n = \lim\limits_{n\rightarrow +\infty}\|\chi_n-\varrho\|^2 = 0. \end{align} | (3.96) |
Therefore, \chi_n\to \varrho.
Corollary 3.4. Let x_0, x_1 \in Q_1 and \tau_n such that 0 \le \tau_n\le \bar{\tau_n}. Define a sequence \left\lbrace \chi_n \right\rbrace as:
\begin{align} \begin{cases} w_n = \chi_n+\tau_n(\chi_n-\chi_{n-1}), \\u_n = K_{Q_1}(w_n), \\ t_n = (1-\varphi_n)u_n+\varphi_n[\sigma_nUu_n+(1-\sigma_n)U_N^nU_{N-1}^n...U_{2}^nU_{1}^nu_n], \\ \chi_{n+1} = \omega_n\gamma h(K_n\chi_n)+\rho_n\chi_n+[(1-\rho_n)I-\omega_nA]K_nt_n. \end{cases} \end{align} | (3.97) |
\begin{align} \bar{\tau_{n}} = \begin{cases} \min\Big\{{\frac{\lambda_n}{\|\chi_n-\chi_{n-1}\|}}, \tau\Big\} & \;{\rm{if}}\; \chi_n\neq \chi_{n-1}, \\\tau & \;{\rm{if}}\; otherwise, \end{cases} \end{align} | (3.98) |
where K_{Q_1} = T_{r_n}^{F_1}(I-r_ng_1) , \liminf_{n \to +\infty}r_n > 0 and \lim\limits_{n\rightarrow +\infty}|r_{n+1}-r_n| = 0 , U_{i}^n = (1- \kappa_n^i)I+ \kappa_n^iP_{Q_1}(\zeta_{n}^iI+(1-\zeta_{n}^i)U_i) with 0 \le \mu_i \le\zeta_{n}^i < 1 and \lim\limits_{n\rightarrow +\infty}|\kappa^i_{n+1}-\kappa_n^i| = 0 for i\le i\le M . Also \lambda_n \subset [0, +\infty) with \sum_{n = 0}^{+\infty}\lambda_n < +\infty , \tau \in [0, 1) , \varphi_n, \sigma_n, \kappa_n^i, \omega_n, \rho_n \in (0, 1) , \rho = \sup\{\rho_n; n\in \mathbb{{N}}\} with \lim\limits_{n\rightarrow +\infty}|\varphi_{n+1}-\varphi_n| = 0 , \eta_{n, j}\to \eta_j , \sum_{n = 0}^{+\infty}|\eta_{n, j}-\eta_{n-1, j}| < +\infty , \lim\limits_{n\rightarrow +\infty}|\sigma_{n+1}-\sigma_n| = 0 and \sum_{n = 0}^{+\infty}\sigma_n < +\infty . Under the assumptions that conditions (i) – (iii) of Theorem 3.3 are satisfied, we can conclude that the sequence {\chi_n} generated by Eq (3.97) strongly converges to the element \xi\in \Delta . This element \xi represents the unique solution to the fixed-point problem associated with the contraction mapping P_{\Delta}(I+\gamma h-A) . In other words, \xi is the solution to the variational inequality stated below:
\begin{align*} \langle(A-\gamma h)\xi, y-\xi\rangle\ge 0, \;\text{for all}\;y\in \Delta. \end{align*} |
Proof. By taking D = O , H_1 = H_2 , Q_1 = Q_2 , F_1 = F_2 , g_1 = g_2 and \psi_1 = \psi_2 in Theorem 3.3, we get the required conclusion.
Corollary 3.5. Let x_0, x_1 \in Q_1 and \tau_n such that 0 \le \tau_n\le \bar{\tau_n}. Define a sequence \left\lbrace \chi_n \right\rbrace as:
\begin{align} \begin{cases} w_n = \chi_n+\tau_n(\chi_n-\chi_{n-1}), \\y_n = K_{Q_1}(w_n), l_n = K_{Q_1}(y_n), \\u_n = y_n-\delta(y_n-l_n), \\ t_n = (1-\varphi_n)u_n+\varphi_n[\sigma_nUu_n+(1-\sigma_n)U_N^nU_{N-1}^n...U_{2}^nU_{1}^nu_n], \\ \chi_{n+1} = \omega_n\gamma h(K_n\chi_n)+\rho_n\chi_n+[(1-\rho_n)I-\omega_nA]K_nt_n. \end{cases} \end{align} | (3.99) |
\begin{align} \bar{\tau_{n}} = \begin{cases} \min\Big\{{\frac{\lambda_n}{\|\chi_n-\chi_{n-1}\|}}, \tau\Big\} & \;{\rm{if}}\; \chi_n\neq \chi_{n-1}, \\\tau & \;{\rm{if}}\; otherwise, \end{cases} \end{align} | (3.100) |
where K_{Q_1} = T_{r_n}^{F_1}(I-r_ng_1) , \liminf_{n \to +\infty}r_n > 0 and \lim\limits_{n\rightarrow +\infty}|r_{n+1}-r_n| = 0 , U_{i}^n = (1- \kappa_n^i)I+ \kappa_n^iP_{Q_1}(\zeta_{n}^iI+(1-\zeta_{n}^i)U_i) with 0 \le \mu_i \le\zeta_{n}^i < 1 and \lim\limits_{n\rightarrow +\infty}|\kappa^i_{n+1}-\kappa_n^i| = 0 for i\le i\le M . Also \lambda_n \subset [0, +\infty) with \sum_{n = 0}^{+\infty}\lambda_n < +\infty , \tau \in [0, 1) , \varphi_n, \sigma_n, \kappa_n^i, \omega_n, \rho_n \in (0, 1) , \rho = \sup\{\rho_n; n\in \mathbb{{N}}\} with \lim\limits_{n\rightarrow +\infty}|\varphi_{n+1}-\varphi_n| = 0 , \eta_{n, j}\to \eta_j , \sum_{n = 0}^{+\infty}|\eta_{n, j}-\eta_{n-1, j}| < +\infty , \lim\limits_{n\rightarrow +\infty}|\sigma_{n+1}-\sigma_n| = 0 and \sum_{n = 0}^{+\infty}\sigma_n < +\infty . Under the assumptions that conditions (i) – (iii) of Theorem 3.3 are satisfied, we can conclude that the sequence {\chi_n} generated by Eq (3.97) strongly converges to the element \xi\in \Delta . This element \xi represents the unique solution to the fixed-point problem associated with the contraction mapping P_{\Delta}(I+\gamma h-A) . In other words, \xi is the solution to the variational inequality stated below:
\begin{align*} \langle(A-\gamma h)\xi, y-\xi\rangle\ge 0, \;\text{for all}\;y\in \Delta. \end{align*} |
Proof. By taking D = I , H_1 = H_2 , Q_1 = Q_2 , F_1 = F_2 , g_1 = g_2 and \psi_1 = \psi_2 in Theorem 3.3, we get the required conclusion.
Remark 3.6.
1) Theorem 3.3 generalizes and enhances the findings of Rizvi [56] from a nonexpansive mapping to a finite family of nonexpansive mappings. Furthermore, our findings extend the outcomes of Rizvi [56] from a common solution of SMEP and HFPP to a common solution of HFPP, SGMEP and FPP for a finite collection of nonexpansive mappings.
2) Theorem 3.3 generalizes the Husain and Singh [57] result from a common solution of SMEP and HFPP to a common solution of HFPP, SGMEP and FPP for a finite family of nonexpansive operators. In addition, we consider HFPP for a finite collection of strictly pseudocontractive operators, which is more general than the nonexpansive mappings taken in Husain and Singh [57] result.
3) Theorem 3.3 generalizes and enhances the findings of Kim and Majee [26] (Theorem 3.6) from a common solution of SEP and HFPP to a common solution of HFPP, SGMEP and FPP for a finite collection of nonexpansive operators.
4) Theorem 3.3 generalizes and enhances the result of Majee and Nahak [24] from a common solution of SEP and HFPP to a common solution of HFPP, SGMEP and FPP for a finite collection of nonexpansive operators.
Compressed sensing in signal processing [58] can be represented by the following linear equation:
\begin{align} y = Dx + \epsilon. \end{align} | (4.1) |
Here, \epsilon is the noise, D is an M \times N matrix with M < N, x \in \mathbb{R}^N is a recovered vector with m non-zero components and y \in \mathbb{R}^M is the observed data. The problem described in Eq (4.1) can be considered as a LASSO problem:
\begin{align} \min\limits_{x \in \mathbb{R}^N} & \frac{1}{2}\|y - Dx\|_2^2 \text{ subject to } \|x\|_1 \leq u. \end{align} | (4.2) |
Here, u > 0 is constant.
In this case, a uniform distribution in the interval [-1, 1] is used to construct the sparse vector x \in \mathbb{R}^N, which has m non-zero members. A normal distribution with a zero mean and a unit variance is used to produce the matrix D. As \delta\in(0, 1/L) , it is randomly generated in MATLAB. By applying white Gaussian noise with a signal-to-noise ratio (SNR) of 40, the observation y is produced. The process starts with an initial point x_1 = ones_{N \times 1} and u = m. Specifically, the LASSO problem can be seen as an SFP (Split Feasibility Problem) if Q_1 = \{x \in \mathbb{R}^N : \|x\|_1 \leq u\} and Q_2 = \{y\}. In this connection, we can solve Eq (4.2) using the CQ technique. The stopping criterion is given by the mean squared error (MSE):
E_n = \frac{1}{N} \left\|\chi_n - \varrho\right\|_2^2 < \Lambda, |
where \Lambda is a tolerance and \chi_n is the estimated signal of x . Note that if in Problem (1.5)–(1.6) we set g_1 = g_2 = \psi_1 = \psi_2 = 0 , we obtain the split equilibrium problems (SEQ) and if, in addition, F_1(v, w) = I_{Q_1}(v)-I_{Q_1}(w) and F_2(v', w') = I_{Q_2}(v')-I_{Q_2}(w') , where I_{Q_1} and I_{Q_2} are identity operators on Q_1 and Q_2 respectively, then SEQ becomes SFP. Hence, we can apply our algorithm to the SFP with the resolvent operator T_r^{F_1} and T_r^{F_2} being the projection onto Q_1 and Q_2 , respectively. In order to implement our algorithm, we choose the following parameters: \lambda_n = \frac{1}{n^2} , \tau = 0.5 , U = U_i = S_j = I for all i, j so that K_n = I (Identity mapping), A = I (Identity operator), \rho_n = \omega_n = \frac{1}{n+1} and h(x) = x/2 so that \gamma = 1 and \Lambda = 10^{-10}.
Figure 1 represents the original signal, observed value and recovered signals by Algorithm 3.1, the Chuasuk Algorithm [37], the Kim Algorithm [26] and the Majee Algorithm [24]. Table 1 and Figure 2 give the mean square error of Algorithm 3.1, the Chuasuk Algorithm [37], the Kim Algorithm [26] and the Majee Algorithm [24]. The experiment shows that all three methods are effective in recovering the signal, however, the time taken by the Chuasuk Algorithm [37] (Average time = 7.8654s), the Majee Algorithm [24] (Average time = 10.9854s) and the Kim Algorithm [26] (Average time = 13.5864s) is more than the time taken by the proposed algorithm (Average time = 5.8754s).
Number of Iterations | CPU Time (Seconds) | |
Algorithm 3.1 | 11 | 0.2965 |
Chuasuk Algorithm | 27 | 0.7008 |
Majee Algorithm | 17 | 0.8665 |
Kim Algorithm | 41 | 0.9545 |
In this section, we first conduct a comparison of the convergence rates between our algorithm and those presented in the Chuasuk Algorithm [37], the Kim Algorithm [26] (Theorem 3.6), and the Majee Algorithm [24]. We implemented the proposed algorithm using MATLAB 9.10.0 ( R2021a ) on a laptop equipped with an Intel Core i5 CPU running at 1.60 GHz , 256 GB SSD and 1 TB hard-disk capacity. The operating system used is Microsoft Windows 11 , version 21 H2 . Secondly, we present numerical experiments related to compressed sensing.
Example 5.1. Assume that H_1 = H_2 = \mathbb{R}^5 , and
\begin{align*} Q_1 = Q_2 = \{x\in \mathbb{R}^5:\sum\limits_{i = 1}^{5}x_i\geq -1, -6\leq x_i\leq 6, 1\le i\le5 \}. \end{align*} |
Let g_1: Q_1 \to \mathbb{R} , g_2: Q_2 \to \mathbb{R} be inverse strongly monotone mappings defined by g_1(x) = 3x and g_2(x) = 3x . Suppose F_1:Q_1\times Q_1 \to \mathbb{R}, F_2:Q_2\times Q_2 \to \mathbb{R} are the bifunctions defined by F_1(x, y) = F_2(x, y) = \langle Px+Qy+q, y-x\rangle , arising from Nash Cournot Oligopolistic market equilibrium model [17] where q\in \mathbb{R}^5 and P, Q \in \mathbb{R}^{5\times 5} are two matrices of order 5 with Q being symmetric, positive semidefinite and Q-P being negative semidefinite. Obviously, bifunction g satisfies Assumption 1 and A: \mathbb{R}\to \mathbb{R} is defined by A(x) = x with constant \bar{\gamma} = 1 . Let \psi_1: Q_1 \to \mathbb{R} \cup \{+\infty\} , \psi_2: Q_2 \to \mathbb{R} \cup \{+\infty\} be defined by \psi_1(x) = \psi_2(x) = 0 , D: \mathbb{R}\to \mathbb{R} be defined by D(x) = x , D^*(x) = x , then T_r^{F_1}(x) = T_r^{F_2}(x) = ((P+Q+3I)r+I)^{-1}x . Let h:Q_1 \to Q_1 be \frac{1}{2} -contraction defined by h(x) = \frac{x}{2} and S_j:Q_1 \to Q_1 be pseudocontractive mappings defined by S_j(x) = \frac{x}{6(j+1)} , for j = 1, 2 . Assume that U:Q_1 \to Q_1 and U_i:Q_1 \to Q_1 are nonexpansive mappings defined by U(x) = \frac{x}{4} and U_i(x) = \frac{x}{10i} , for i, = 1, 2 , x = (x_1, x_2, x_3, x_4, x_5)^{T} . Choose \delta = \frac{1}{16} , r_n = 1 , \lambda_n = \frac{1}{n^2} , \tau = 0.5 , \kappa_n^i = \frac{n+i}{n+5+i} , \zeta_{n}^i = \frac{1}{20} , \eta_n^j = \frac{1}{20n+5j} for i, j = 1, 2 , \sigma_n = \frac{1}{(n+1)^2} , \varphi_n = \frac{5}{6} , \rho_n = \frac{n+1}{2(n+50)} and \omega_n = \frac{1}{n+200} . One can easily see that Fix (\Gamma) = \{0\}\ne \phi. We can obtain K_{Q_1}(x) = K_{Q_2}(x) = -2(P+Q+4I)^{-1}x . Take P = I_5 , Q = 0_{5\times 5} , x_0 = (0.5, 0.5, 0.5, 0.5, 0.5)^{T}, x_1 = (0.8, 0.8, 0.8, 0.8, 0.8)^{T} and q = [0, 0, 0, 0, 0]^\mathit{T} . We take a stopping criterion of E_n = \|\chi_n-\chi_{n+1}\| < 10^{-4} and plot the graphs between number of iterations n and errors E_n . We do comparative analysis of the numerical result of Algorithm 3.1 with the Chuasuk Algorithm [37], the Kim Algorithm [26] (Theorem (3.6)) and the Majee Algorithm [24]. Table 2 and Figure 3 represent the comparative analysis.
Number of Iterations | CPU Time (Seconds) | |
Algorithm 3.1 | 38 | 0.2755 |
Chuasuk Algorithm | 172 | 0.9870 |
Majee Algorithm | 93 | 0.8106 |
Kim Algorithm | 307 | 1.0956 |
Example 5.2. Assume that H_1 = H_2 = l_2 are real Hilbert spaces with square-summable infinite sequences of real numbers as its elements and Q_1 = Q_2 = \{v\in l_2:\|v\| \le 3 \} . Let g_1: [-5, 5] \to \mathbb{R} , g_2: [-5, 5] \to \mathbb{R} be ism mappings defined by g_1(x) = 10x and g_2(x) = 2x . Suppose F_1:Q_1\times Q_1 \to \mathbb{R}, F_2:Q_2\times Q_2 \to \mathbb{R} are the bifunctions defined by F_1(x, y) = -5x^2+xy+4y^2 , F_2(x, y) = -3x^2+xy+2y^2 for all x = (x_1, x_2, x_3, ..., x_n, ...) and y = (y_1, y_2, y_3, ..., y_n, ...) with \|.\|:l_2 \rightarrow \mathbb{R} and \langle., .\rangle:l_2\times l_2 \rightarrow \mathbb{R} given by \|x\| = (\sum_{k = 1}^{+\infty}|x_k|^2)^\frac{1}{2} and \langle x, y\rangle = \sum_{k = 1}^{+\infty}x_ky_k , where x = \{x_k\}_{k = 1}^{+\infty} , y = \{y_k\}_{k = 1}^{+\infty} . Suppose that A: \mathbb{R}\to \mathbb{R} is defined by A(x) = x for all x = (x_1, x_2, x_3, ..., x_n, ...) with constant \bar{\gamma} = 1 . Let \psi_1: Q_1 \to \mathbb{R} \cup \{+\infty\} , \psi_2: Q_2 \to \mathbb{R} \cup \{+\infty\} be given by \psi_1(x) = x^2 , \psi_2(x) = 2x^2 , D: \mathbb{R}\to \mathbb{R} be defined by D(x) = -5x , D^* = -5x , then T_r^{F_1}(x) = \frac{x}{21r+1} and T_r^{F_2}(x) = \frac{x}{11r+1} . Let h:Q_1 \to Q_1 be \frac{1}{2} -contraction defined by h(x) = \frac{x}{2} and S_j:Q_1 \to Q_1 be pseudocontractive mappings defined by S_j(x) = \frac{x}{2(j+1)} , for j = 1, 2 . Assume that U:Q_1 \to Q_1 and U_i:Q_1 \to Q_1 are nonexpansive mappings defined by U(x) = x and U_i(x) = \frac{x}{100i} , for i, = 1, 2 . Choose \delta = \frac{1}{16} , r_n = 1 , \lambda_n = \frac{1}{n^2} , \tau = 0.9 , \kappa_n^i = \frac{n+i}{n+5+i} , \zeta_{n}^i = \frac{7}{8i} , \eta_n^j = \frac{1}{20n+5j} for i, j = 1, 2 , \sigma_n = \frac{1}{n^2} , \varphi_n = \frac{5}{6} , \rho_n = \frac{n+1}{2000(n+5)} and \omega_n = \frac{1}{700n+4} . One can easily see that Fix (\Gamma) = \{0\}\ne \phi. We can obtain K_{Q_1}(x) = \frac{-9x}{21} and K_{Q_2}(x) = \frac{-x}{12} . We take a stopping criterion of E_n = \|\chi_n-\chi_{n+1}\| < 10^{-4} and plot the graphs between errors E_n and the number of iterations n . Take initial values x_0 = (0.5, 0.5, 0.5, 0.5, ..., 0.5, ...) and x_1 = (0.8, 0.8, 0.8, 0.8, ..., 0.8, ...) . We do comparative analysis of the numerical result obtain from Algorithm 3.1 with the Chuasuk [37], the Kim [26] (Theorem (3.6)) and the Majee [24] algorithms. Table 3 and Figure 4 show the numerical results
Number of Iterations | CPU Time (Seconds) | |
Algorithm 3.2 | 12 | 0.03876 |
Chuasuk Algorithm | 38 | 1.756 |
Kim Algorithm | 25 | 0.1638 |
Majee Algorithm | 21 | 0.1548 |
This paper discussed a new inertial generalized viscosity approximation method for solving split generalized mixed equilibrium problem, fixed point problem for a finite family of nonexpansive mappings and hierarchical fixed point problem in real Hilbert spaces. Under certain appropriate conditions, we have established the result of strong convergence. We have demonstrated the use of our main finding with compressed sensing in signal processing. We have explained the numerical effectiveness of our approach in comparison to another method. The results discussed in this paper enhance and summarize previously published findings in the literature.
The authors declare they have not used Artificial Intelligence (AI) tools in the creation of this article.
Researchers would like to thank the Deanship of Scientific Research, Qassim University for funding publication of this project.
The authors declare that they have no competing interests.
[1] |
Y. Censor, T. Bortfeld, B. Martin, A. Trofimov, A unified approach for inversion problems in intensity-modulated radiation therapy, Phys. Med. Biol., 51 (2006), 2353–2365. https://doi.org/10.1088/0031-9155/51/10/001 doi: 10.1088/0031-9155/51/10/001
![]() |
[2] |
Y. Censor, A. Segal, The split common fixed point problem for directed operators, J. Convex Anal., 26 (2010), 55007. https://doi.org/10.1088/0266-5611/26/5/055007 doi: 10.1088/0266-5611/26/5/055007
![]() |
[3] | K. Fan, A minimax inequality and applications, In: Inequality III, New York: Academic Press, 1972,103–113. |
[4] | E. Blum, W. Oettli, From optimization and variational inequalities to equilibrium problems, Mathematics Student, 63 (1994), 123–145. |
[5] |
S. Suantai, S. Kesornprom, P. Cholamjiak, A new hybrid CQ algorithm for the split feasibility problem in Hilbert spaces and its applications to compressed sensing, Mathematics, 7 (2019), 789. https://doi.org/10.3390/math7090789 doi: 10.3390/math7090789
![]() |
[6] |
R. Chugh, R. Kumar, C. Batra, A novel inertial Tseng's method for solving generalized variational inequality problem, J. Appl. Math. Comput., 2023 (2023), 1–27. https://doi.org/10.1007/s12190-023-01942-z doi: 10.1007/s12190-023-01942-z
![]() |
[7] |
J. W. Peng, J. C. Yao, A new hybrid-extragradient method for generalized mixed equilibrium problems, fixed point problems and variational inequality problems, Taiwanese J. Math., 12 (2008), 1401–1432. https://doi.org/10.11650/twjm/1500405033 doi: 10.11650/twjm/1500405033
![]() |
[8] | S. Chaiyasil, S. Suantai, Aproximation method for generalized mixed equilibrium problems and fixed point problems for a countable family of nonexpansive mappings, Journal of Nonlinear Analysis and Optimization: Theory and Applications, 2 (2011), 337–353. |
[9] |
I. Inchan, Extragradient method for generalized mixed equilibrium problems and fixed point problems of finite family of nonexpansive mapping, Appl. Math. Comput., 219 (2012), 2949–2959. https://doi.org/10.1016/j.amc.2012.09.020 doi: 10.1016/j.amc.2012.09.020
![]() |
[10] |
F. U. Ogbuisi, O. T. Mewomo, On split generalised mixed equilibrium problems and fixed-point problems with no prior knowledge of operator norm, J. Fixed Point Theory Appl., 19 (2017), 2109–2128. https://doi.org/10.1007/s11784-016-0397-6 doi: 10.1007/s11784-016-0397-6
![]() |
[11] | O. K. Oyewole, O. T. Mewomo, A new inertial-projection method for solving split generalized mixed equilibrium and hierarchical fixed point problems, Kragujev. J. Math., 48 (2024), 199–223. |
[12] |
A. Moudafi, P. E. Mainge, Towards viscosity approximations of hierarchical fixed point problems, Fixed Point Theory Appl., 2006 (2006), 95453. https://doi.org/10.1155/FPTA/2006/95453 doi: 10.1155/FPTA/2006/95453
![]() |
[13] |
Y. Yao, Y. C. Liou, J. C. Yao, Iterative algorithms for the split variational inequality and fixed point problems under nonlinear transformations, J. Nonlinear Sci. Appl., 10 (2017), 843–854. https://doi.org/10.22436/jnsa.010.02.43 doi: 10.22436/jnsa.010.02.43
![]() |
[14] |
A. Kangtunyakarn, S. Suantai, A new mapping for finding common solutions of equilibrium problems and fixed point problems of finite family of nonexpansive mappings, Nonlinear Anal. Theor., 71 (2009), 4448–4460. https://doi.org/10.1016/j.na.2009.03.003 doi: 10.1016/j.na.2009.03.003
![]() |
[15] |
Anjali, R. Chugh, C. Batra, Fixed point theorems of enriched Ciric's type and enriched Hardy-Rogers contractions, Numer. Algebr. Control, 2023 (2023), 022. https://doi.org/10.3934/naco.2023022 doi: 10.3934/naco.2023022
![]() |
[16] |
A. Gangwar, A. Tomar, M. Sajid, R. C. Dimri, Common fixed points and convergence results for \alpha-Krasnosel'skii mappings, AIMS Mathematics, 8 (2023), 9911–9923. https://doi.org/10.3934/math.2023501 doi: 10.3934/math.2023501
![]() |
[17] |
C. Batra, N. Gupta, R. Chugh, R. Kumar, Generalized viscosity extragradient algorithm for pseudomonotone equilibrium and fixed point problems for finite family of demicontractive operators, J. Appl. Math. Comput., 68 (2022), 4195–4222. https://doi.org/10.1007/s12190-022-01699-x doi: 10.1007/s12190-022-01699-x
![]() |
[18] |
R. Chugh, N. Gupta, Strong convergence of new split general system of monotone variational inclusion problem, Appl. Anal., 2023 (2023), 2178423. https://doi.org/10.1080/00036811.2023.2178423 doi: 10.1080/00036811.2023.2178423
![]() |
[19] |
H. ur Rehman, W. Kumam, K. Sombut, Inertial modification using self-adaptive subgradient extragradient techniques for equilibrium programming applied to variational inequalities and fixed-point problems, Mathematics, 10 (2022), 1751. https://doi.org/10.3390/math10101751 doi: 10.3390/math10101751
![]() |
[20] | C. Batra, R. Chugh, R. Kumar, Variational inequality problem with application to convex minimization problem, Mathematics in Engineering, Science and Aerospace, 14 (2023), 71–82. |
[21] |
N. Gupta, L. O. Jolaoso, A. Nandal, R. Chugh, Convergence analysis of multiple-sets split equality common fixed point problem with applications, Numer. Algebr. Control, 2023 (2023), 012. https://doi.org/10.3934/naco.2023012 doi: 10.3934/naco.2023012
![]() |
[22] |
N. Gupta, M. Postolache, A. Nandal, R. Chugh, A cyclic iterative algorithm for multiple-sets split common fixed point problem of demicontractive mappings without prior knowledge of operator norm, Mathematics, 9 (2021), 372. https://doi.org/10.3390/math9040372 doi: 10.3390/math9040372
![]() |
[23] |
K. R. Kazmi, R. Ali, M. Furkan, Krasnosel'skii-Mann type iterative method for hierarchical fixed point problem and split mixed equilibrium problem, Numer. Algor., 77 (2018), 289–308. https://doi.org/10.1007/s11075-017-0316-y doi: 10.1007/s11075-017-0316-y
![]() |
[24] |
P. Majee, C. Nahak, A hybrid viscosity iterative method with averaged mappings for split equilibrium problems and fixed point problems, Numer. Algor., 74 (2017), 609–635. https://doi.org/10.1007/s11075-016-0164-1 doi: 10.1007/s11075-016-0164-1
![]() |
[25] |
P. Majee, C. Nahak, A modified iterative method for capturing a common solution of split generalized equilibrium problem and fixed point problem, Racsam. Rev. R. Acad. A., 112 (2018), 1327–1348. https://doi.org/10.1007/s13398-017-0428-1 doi: 10.1007/s13398-017-0428-1
![]() |
[26] |
J. K. Kim, P. Majee, Modified Krasnosel'skii-Mann iterative method for hierarchical fixed point problem and split mixed equilibrium problem, J. Inequal. Appl., 2020 (2020), 227. https://doi.org/10.1186/s13661–250-020-02493-8 doi: 10.1186/s13661–250-020-02493-8
![]() |
[27] |
M. Yazdi, S. H. Sababe, A viscosity approximation method for solving general system of variational inequalities, generalized mixed equilibrium problems and fixed point problems, Symmetry, 14 (2022), 1507. https://doi.org/10.3390/sym14081507 doi: 10.3390/sym14081507
![]() |
[28] |
K. Zhao, Solvability, approximation and stability of periodic boundary value problem for a nonlinear Hadamard fractional differential equation with p-Laplacian, Axioms, 12 (2023), 733. https://doi.org/10.3390/axioms12080733 doi: 10.3390/axioms12080733
![]() |
[29] |
K. Zhao, Generalized UH-stability of a nonlinear fractional coupling (p_1, p_2)-Laplacian system concerned with nonsingular Atangana-Baleanu fractional calculus, J. Inequal. Appl., 2023 (2023), 96. https://doi.org/10.1186/s13660-023-03010-3 doi: 10.1186/s13660-023-03010-3
![]() |
[30] |
K. Zhao, Stability of a nonlinear Langevin system of ML-type fractional derivative affected by time-varying delays and differential feedback control, Fractal Fract., 6 (2022), 725. https://doi.org/10.3390/fractalfract6120725 doi: 10.3390/fractalfract6120725
![]() |
[31] |
K. Zhao, Stability of a nonlinear fractional Langevin system with nonsingular exponential kernel and delay control, Discrete Dyn. Nat. Soc., 2022 (2022), 9169185. https://doi.org/10.1155/2022/9169185 doi: 10.1155/2022/9169185
![]() |
[32] | K. Zhao, Global asymptotic stability for a classical controlled nonlinear periodic commensalism AG-ecosystem with distributed lags on time scales, Filomat, 37 (2023), 9899–9911. |
[33] | R. Chugh, C. Batra, G. Biban, A. Ahuja, New four step iteration process for approximating fixed point of contraction mappings, Mathematics in Engineering, Science and Aerospace, 13 (2022), 1071–1087. |
[34] |
T. Jin, X. Yang, Monotonicity theorem for the uncertain fractional differential equation and application to uncertain financial market, Math. Comput. Simulat., 190 (2021), 203–221. https://doi.org/10.1016/j.matcom.2021.05.018 doi: 10.1016/j.matcom.2021.05.018
![]() |
[35] |
T. Jin, H. Xia, Lookback option pricing models based on the uncertain fractional-order differential equation with Caputo type, J. Ambient Intell. Human. Comput., 14 (2021), 6435–6448. https://doi.org/10.1007/s12652-021-03516-y doi: 10.1007/s12652-021-03516-y
![]() |
[36] |
B. T. Polyak, Some methods of speeding up the convergence of iterative methods, Comp. Math. Math. Phys. 4 (1964), 1–17. https://doi.org/10.1016/0041-5553(64)90137-5 doi: 10.1016/0041-5553(64)90137-5
![]() |
[37] |
P. Chuasuk, A. Kaewcharoen, Krasnosel'skii-Mann-type inertial method for solving split generalized mixed equilibrium and hierarchical fixed point problems, J. Inequal. Appl., 2021 (2021), 94. https://doi.org/10.1186/s13660-021-02632-9 doi: 10.1186/s13660-021-02632-9
![]() |
[38] |
G. C. Ugwunnadi, L. Y. Haruna, M. H. Harbau, Accelerated Krasnoselski-Mann type algorithm for hierarchical fixed point and split monotone variational inclusion problems in Hilbert spaces, Carpathian Math. Publ., 15 (2023), 158–179. https://doi.org/10.15330/cmp.15.1.158-179 doi: 10.15330/cmp.15.1.158-179
![]() |
[39] |
A. Moudafi, P. E. Mainge, Towards viscosity approximations of hierarchical fixed point problems, Fixed Point Theory Appl., 2006 (2006), 95453. https://doi.org/10.1155/FPTA/2006/95453 doi: 10.1155/FPTA/2006/95453
![]() |
[40] | K. Goebel, S. Reich, Uniform convexity, hyperbolic geometry, and nonexpansive mappings, New York: Dekker, 1983. |
[41] |
H. H. Bauschke, J. M. Borwein, On projection algorithms for solving convex feasibility problems, SIAM Rev., 38 (1996), 367–426. https://doi.org/10.1137/S0036144593251710 doi: 10.1137/S0036144593251710
![]() |
[42] |
M. Bianchi, S. Schaible, Generalized monotone bifunctions and equilibrium problems, J. Optimiz. Theory App., 90 (1996), 31–43. https://doi.org/10.1007/BF02192244 doi: 10.1007/BF02192244
![]() |
[43] |
H. U. Rehman, P. Kumam, Q. L. Dong, Y. Peng, W. Deebani, A new Popov' subgradient extragradient method for two classes of equilibrium programming in a real Hilbert space, Optimization, 70 (2021), 2675–2710. https://doi.org/10.1080/02331934.2020.1797026 doi: 10.1080/02331934.2020.1797026
![]() |
[44] |
H. A. Abass, C. Izuchukwu, O. T. Mewomo, Viscosity approximation method for modified split generalized equilibrium and fixed point problems, Rev. Union Mat. Argent., 61 (2020), 389–411. https://doi.org/10.33044/revuma.v61n2a13 doi: 10.33044/revuma.v61n2a13
![]() |
[45] |
G. Marino, H. K. Xu, A general iterative method for nonexpansive mapping in Hilbert spaces, J. Math. Anal. Appl., 318 (2006), 43–52. https://doi.org/10.1016/j.jmaa.2005.05.028 doi: 10.1016/j.jmaa.2005.05.028
![]() |
[46] |
B. V. Dinh, D. S. Kim, Projection algorithms for solving nonmonotone equilibrium problems in Hilbert space, J. Comput. Appl. Math., 302 (2016), 106–117. https://doi.org/10.1016/j.cam.2016.01.054 doi: 10.1016/j.cam.2016.01.054
![]() |
[47] |
A. Moudafi, Split monotone variational inclusions, J. Optim. Theory Appl., 150 (2011), 275–283. https://doi.org/10.1007/s10957-011-9814-6 doi: 10.1007/s10957-011-9814-6
![]() |
[48] | H. H. Bauschke, P. L. Combettes, Convex analysis and monotone operator theory in Hilbert space, Cham: Springer, 2017. https://doi.org/10.1007/978-3-319-48311-5 |
[49] |
H. Zhou, Convergence theorems of fixed points for k-strict pseudo-contractions in Hilbert spaces, Nonlinear Anal. Theor., 69 (2008), 456–462. https://doi.org/10.1016/j.na.2007.05.032 doi: 10.1016/j.na.2007.05.032
![]() |
[50] |
P. E. Maingè, Strong convergence of projected subgradient methods for nonsmooth and nonstrictly convex minimization, Set-Valued Analysis, 16 (2008), 899–912. https://doi.org/10.1007/s11228-008-0102-z doi: 10.1007/s11228-008-0102-z
![]() |
[51] |
S. Li, L. Li L. Cao, X. He, X. Yue, Hybrid extragradient method for generalized mixed equilibrium problem and fixed point problems in Hilbert space, Fixed Point Theory Appl., 2013 (2013), 240. https://doi.org/10.1186/1687-1812-2013-240 doi: 10.1186/1687-1812-2013-240
![]() |
[52] | P. L. Combettes, S. A. Hirstoaga, Equilibrium programming in Hilbert spaces, J. Nonlinear Convex A., 6 (2005), 117–136. |
[53] |
T. Suzuki, Strong convergence of Krasnosel'skiii and Mann's type sequences for one parameter nonexpansive semigroups without Bochner integrals, J. Math. Anal. Appl., 305 (2005), 227–239. https://doi.org/10.1016/j.jmaa.2004.11.017 doi: 10.1016/j.jmaa.2004.11.017
![]() |
[54] |
A. Kangtunyakar, S. Suantai, A new mapping for finding common solutions of equilibrium problems and fixed point problems of finite family of nonexpansive mappings, Nonlinear Anal. Theor., 71 (2009), 4448–4460. https://doi.org/10.1016/j.na.2009.03.003 doi: 10.1016/j.na.2009.03.003
![]() |
[55] | K. Goebel, W. A. Kirk, Topics in metric fixed point theory, Cambridge: Cambridge University Press, 1990. https://doi.org/10.1017/CBO9780511526152 |
[56] |
S. H. Rizvi, A strong convergence theorem for split mixed equilibrium and fixed point problems for nonexpansive mappings, J. Fixed Point Theory Appl., 20 (2018), 8. https://doi.org/10.1007/s11784-018-0487-8 doi: 10.1007/s11784-018-0487-8
![]() |
[57] |
S. Husain, N. Singh, A hybrid iterative algorithm for a split mixed equilibrium problem and a hierarchical fixed point problem, Appl. Set-Valued Anal. Optim., 1 (2019), 149–169. https://doi.org/10.23952/asvao.1.2019.2.05 doi: 10.23952/asvao.1.2019.2.05
![]() |
[58] |
R. Tibshirani, Regression shrinkage and selection via the Lasso, J. R. Stat. Soc. B, 58 (1996), 267–288. https://doi.org/10.1111/j.2517-6161.1996.tb02080.x doi: 10.1111/j.2517-6161.1996.tb02080.x
![]() |
1. | Meenakshi Gugnani, Nishu Gupta, Tseng type iterative algorithm for generalized variational inequality and variational inclusion problems with applications, 2024, 0, 2155-3289, 0, 10.3934/naco.2024044 | |
2. | Meenakshi Gugnani, Charu Batra, Strong convergence theorem for new four-step iterative method, 2024, 0, 2155-3289, 0, 10.3934/naco.2024042 |
Number of Iterations | CPU Time (Seconds) | |
Algorithm 3.1 | 11 | 0.2965 |
Chuasuk Algorithm | 27 | 0.7008 |
Majee Algorithm | 17 | 0.8665 |
Kim Algorithm | 41 | 0.9545 |
Number of Iterations | CPU Time (Seconds) | |
Algorithm 3.1 | 11 | 0.2965 |
Chuasuk Algorithm | 27 | 0.7008 |
Majee Algorithm | 17 | 0.8665 |
Kim Algorithm | 41 | 0.9545 |
Number of Iterations | CPU Time (Seconds) | |
Algorithm 3.1 | 38 | 0.2755 |
Chuasuk Algorithm | 172 | 0.9870 |
Majee Algorithm | 93 | 0.8106 |
Kim Algorithm | 307 | 1.0956 |
Number of Iterations | CPU Time (Seconds) | |
Algorithm 3.2 | 12 | 0.03876 |
Chuasuk Algorithm | 38 | 1.756 |
Kim Algorithm | 25 | 0.1638 |
Majee Algorithm | 21 | 0.1548 |