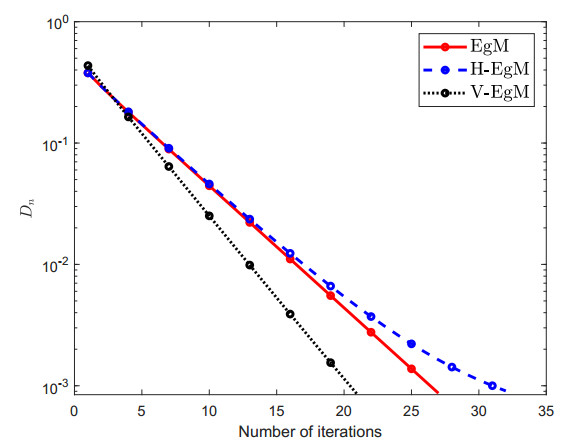
Citation: Habib ur Rehman, Poom Kumam, Kanokwan Sitthithakerngkiet. Viscosity-type method for solving pseudomonotone equilibrium problems in a real Hilbert space with applications[J]. AIMS Mathematics, 2021, 6(2): 1538-1560. doi: 10.3934/math.2021093
[1] | Lu-Chuan Ceng, Li-Jun Zhu, Tzu-Chien Yin . Modified subgradient extragradient algorithms for systems of generalized equilibria with constraints. AIMS Mathematics, 2023, 8(2): 2961-2994. doi: 10.3934/math.2023154 |
[2] | Pongsakorn Yotkaew, Nopparat Wairojjana, Nuttapol Pakkaranang . Accelerated non-monotonic explicit proximal-type method for solving equilibrium programming with convex constraints and its applications. AIMS Mathematics, 2021, 6(10): 10707-10727. doi: 10.3934/math.2021622 |
[3] | Fei Ma, Jun Yang, Min Yin . A strong convergence theorem for solving pseudo-monotone variational inequalities and fixed point problems using subgradient extragradient method in Banach spaces. AIMS Mathematics, 2022, 7(4): 5015-5028. doi: 10.3934/math.2022279 |
[4] | Habib ur Rehman, Wiyada Kumam, Poom Kumam, Meshal Shutaywi . A new weak convergence non-monotonic self-adaptive iterative scheme for solving equilibrium problems. AIMS Mathematics, 2021, 6(6): 5612-5638. doi: 10.3934/math.2021332 |
[5] | Yali Zhao, Qixin Dong, Xiaoqing Huang . A self-adaptive viscosity-type inertial algorithm for common solutions of generalized split variational inclusion and paramonotone equilibrium problem. AIMS Mathematics, 2025, 10(2): 4504-4523. doi: 10.3934/math.2025208 |
[6] | Rose Maluleka, Godwin Chidi Ugwunnadi, Maggie Aphane . Inertial subgradient extragradient with projection method for solving variational inequality and fixed point problems. AIMS Mathematics, 2023, 8(12): 30102-30119. doi: 10.3934/math.20231539 |
[7] | Bancha Panyanak, Chainarong Khunpanuk, Nattawut Pholasa, Nuttapol Pakkaranang . A novel class of forward-backward explicit iterative algorithms using inertial techniques to solve variational inequality problems with quasi-monotone operators. AIMS Mathematics, 2023, 8(4): 9692-9715. doi: 10.3934/math.2023489 |
[8] | Lu-Chuan Ceng, Shih-Hsin Chen, Yeong-Cheng Liou, Tzu-Chien Yin . Modified inertial subgradient extragradient algorithms for generalized equilibria systems with constraints of variational inequalities and fixed points. AIMS Mathematics, 2024, 9(6): 13819-13842. doi: 10.3934/math.2024672 |
[9] | Francis Akutsah, Akindele Adebayo Mebawondu, Austine Efut Ofem, Reny George, Hossam A. Nabwey, Ojen Kumar Narain . Modified mildly inertial subgradient extragradient method for solving pseudomonotone equilibrium problems and nonexpansive fixed point problems. AIMS Mathematics, 2024, 9(7): 17276-17290. doi: 10.3934/math.2024839 |
[10] | Austine Efut Ofem, Jacob Ashiwere Abuchu, Godwin Chidi Ugwunnadi, Hossam A. Nabwey, Abubakar Adamu, Ojen Kumar Narain . Double inertial steps extragadient-type methods for solving optimal control and image restoration problems. AIMS Mathematics, 2024, 9(5): 12870-12905. doi: 10.3934/math.2024629 |
Assume that C is a closed and convex subset of a real Hilbert space H with the inner product and the induced norm are denoted by ⟨⋅,⋅⟩ and ‖⋅‖, respectively. Moreover, R be a set of real numbers during whole article. Let f:H×H→R be a bifunction and satisfy f(v,v)=0 for all v∈C, the equilibrium problem (EP) [6,11] for a bifunction f on C is defined in the following way:
Findu∗∈Csuchasf(u∗,v)≥0,∀v∈C.(EP) |
Moreover, SEP(f,C) denotes the solution set of an equilibrium problem over the set C and u∗ is an arbitrary element of SEP(f,C). A metric projection PC(u) of u∈H onto a closed and convex subset C of H is defined by PC(u)=argminv∈C‖v−u‖.
In this article, the equilibrium problem is studied based on the following hypothesis:
(a1) A bifunction f:H×H→R is said to be (see [3,6]) pseudomonotone on C if
f(u1,u2)≥0⟹f(u2,u1)≤0,∀u1,u2∈C. |
(a2) A bifunction f:H×H→R is said to be Lipschitz-type continuous [21] on C if there exist two constants c1,c2>0 such that
f(u1,u3)≤f(u1,u2)+f(u2,u3)+c1‖u1−u2‖2+c2‖u2−u3‖2,∀u1,u2,u3∈C. |
(a3)lim supn→∞f(un,v)≤f(p∗,v) for all v∈C and {un}⊂C satisfies un⇀p∗;
(a4)f(u,⋅) is subdifferentiable and convex on H for every each u∈H.
The above-defined problem (EP) is a general mathematical problem in the sense that it unifies a number of mathematical problems, i.e., the fixed point problems, the vector and scalar minimization problems, the problems of variational inequalities (VIP), the complementarity problems, the saddle point problems, the Nash equilibrium problems in non-cooperative games and the inverse optimization problems [4,6,24,41]. The problem (EP) is also known as the well-known Ky Fan inequality due to his initial contribution [11]. Many authors have developed and generalized many results on the existence and nature of the solution of an equilibrium problem (see for more detail [2,4,11]). Due to the significance of the problem (EP) and its applications in both pure and applied sciences, many researchers have studied it extensively in recent years [5,10,14].
A proximal point method is used to solve the problem (EP) based on mathematical programming [13]. This method was also known as the two-step extragradient method in [34] due to initial contribution of Korpelevich [18] to solve saddle point problems. Tran et al. in [34] established an iterative sequence {un} in the following way:
{u0∈C,vn=argminv∈C{ρf(un,v)+12‖un−v‖2},un+1=argminv∈C{ρf(vn,v)+12‖un−v‖2}, |
where 0<ρ<min{12c1,12c2}. Recently, many existing methods have been extended in the case of problem (EP) in finite and infinite-dimensional spaces, such as the proximal point-like methods [13,22], the extragradient-like methods [17,19,26,27,32], the subetaadient extragradient methods [1,29,36,37], the inertial methods [35,39] and others in [9,12,16,25,30,33,38].
Inspired by the results in [8,16,23], in this paper, we introduce a viscosity-type subetaadient extragradient algorithm to solve the equilibrium problems involving pseudomonotone bifunction. A strong convergence theorem for the proposed algorithm is well-established by considering certain mild conditions on bifunction and control parameters. Some applications for our main results are studied to solve two particular classes of an equilibrium problem. In the end, the computational studies show that the new method is more efficient than the existing ones [16,34].
The remainder of this article has been organized as follows: Section 2 includes some preliminary and basic results. Section 3 contains proposed algorithm and corresponding strong convergence result. Section 4 contains applications of our main results. Section 5 involves the numerical discussion of the proposed method compared to existing ones.
A normal cone of C at u∈C is defined by
NC(u)={w∈H:⟨w,v−u⟩≤0,∀v∈C}. |
Let φ:C→R is convex function. The subdifferential of φ at u∈C is defined by
∂φ(u)={w∈H:φ(v)−φ(u)≥⟨w,v−u⟩,∀v∈C}. |
Lemma 2.1. [15] Assume that PC:H→C is a metric projection such that
(ⅰ)
‖u1−PC(u2)‖2+‖PC(u2)−u2‖2≤‖u1−u2‖2,u1∈C,u2∈H. |
(ⅱ) u3=PC(u1) if and only if
⟨u1−u3,u2−u3⟩≤0,∀u2∈C. |
(ⅲ)
‖u1−PC(u1)‖≤‖u1−u2‖,u2∈C,u1∈H. |
Lemma 2.2. [40] Assume that {an}⊂(0,+∞) is a sequence satisfying
an+1≤(1−γn)an+γnδn,∀n∈N, |
where {γn}⊂(0,1) and {δn}⊂R satisfies the following conditions:
limn→∞γn=0,+∞∑n=1γn=+∞andlim supn→∞δn≤0. |
Then, limn→∞an=0.
Lemma 2.3. [20] Assume that a sequence {an}⊂R and there exists a subsequence {ni} of {n} such that ani<ani+1, for all i∈N. Then, there is a non decreasing sequence {mk}⊂N such that mk→+∞ as k→∞, and the following conditions are fulfilled by all (sufficiently large) numbers k∈N,
amk≤amk+1andak≤amk+1. |
In fact mk is the largest number n in the set {1,2,⋯,k} such that an≤an+1.
Lemma 2.4. [15] For each u1,u2∈H and δ∈R, then the following relationships are true.
(ⅰ)
‖δu1+(1−δ)u2‖2=δ‖u1‖2+(1−δ)‖u2‖2−δ(1−δ)‖u1−u2‖2. |
(ⅱ)
‖u1+u2‖2≤‖u1‖2+2⟨u2,u1+u2⟩. |
Lemma 2.5. (Theorem 27.4 [28]) Let φ:C→R be a proper convex, subdifferentiable and lower semi-continuous function on C. An element u∈C is a minimizer of a function φ iff
0∈∂φ(u)+NC(u), |
where ∂φ(u) stands for the sub-differential of φ at u∈C and NC(u) the normal cone of C at u.
In this section, we present an iterative scheme for solving pseudomonotone equilibrium problems that is based on Tran et al. in [34] and viscosity scheme [23]. It is important to note that the proposed method has a straightforward structure for achieving strong convergence. Suppose that g:H→H be a strict contraction function with constant ξ∈[0,1). The main algorithm has been presented as follows:
Remark 3.1. It can be easily prove that C⊂Hn. By vn and Lemma 2.5, we have
0∈∂2{ρf(un,v)+12‖un−v‖2}(vn)+NC(vn). |
Indeed, for ωn∈∂f(un,vn) there exists ¯ωn∈NC(vn) such that
ρωn+vn−un+¯ωn=0. |
Thus, we have
⟨un−vn,v−vn⟩=ρ⟨ωn,v−vn⟩+⟨¯ωn,v−vn⟩,∀v∈C. |
Due to ¯ωn∈NC(vn) means that ⟨¯ωn,v−vn⟩≤0, for all v∈C. It implies that
⟨un−vn,v−vn⟩≤ρ⟨ωn,v−vn⟩,∀v∈C, |
which imply that ⟨un−ρωn−vn,v−vn⟩≤0,∀v∈C. It proves that C⊂Hn for each n∈N.
Theorem 3.1. Assume that {un} is a sequence generated by Algorithm 1 and for some u∗∈SEP(f,C)≠∅. Then, {un} converges strongly to u∗=PSEP(f,C)∘g(u∗).
Algorithm 1 (A Viscosity Method for Pseudomonotone Equilibrium Problems) |
Step 0: Let u0∈C, 0<ρ<min{12c1,12c2} and a sequence χn⊂(0,1) satisfies the conditions, i.e.,
limn→∞χn=0and+∞∑nχn=+∞. Step 1: Compute vn=argminv∈C{ρf(un,v)+12‖un−v‖2}. If un=vn, then stop the sequence. Otherwise, go to Step 2. Step 2: Compute Hn={w∈H:⟨un−ρωn−vn,w−vn⟩≤0}, where ωn∈∂2f(un,vn) and evaluate tn=argminv∈Hn{ρf(vn,v)+12‖un−v‖2}. Step 3: Compute un+1=χng(un)+(1−χn)tn, where g is a contraction. Set n:=n+1 and go back to Step 1. |
Proof. Claim 1: The {un} sequence is bounded.
By Lemma 2.5, we have
0∈∂2(ρf(vn,v)+12‖un−v‖2)(tn)+NHn(tn). |
Thus, there exists ωn∈∂f(vn,tn) and ¯ωn∈NHn(tn) such that ρωn+tn−un+¯ωn=0. Thus,
⟨un−tn,v−tn⟩=ρ⟨ωn,v−tn⟩+⟨¯ωn,v−tn⟩,∀v∈Hn. |
Since ¯ωn∈NHn(tn) follows that ⟨¯ωn,v−tn⟩≤0, for all v∈Hn. Thus, we have
ρ⟨ωn,v−tn⟩≥⟨un−tn,v−tn⟩,∀v∈Hn. | (3.1) |
Since ωn∈∂f(vn,tn) and using the subdifferential definition, we get
f(vn,v)−f(vn,tn)≥⟨ωn,v−tn⟩,∀v∈H. | (3.2) |
Combining expressions (3.1) and (3.2), we obtain
ρf(vn,v)−ρf(vn,tn)≥⟨un−tn,v−tn⟩,∀v∈Hn. | (3.3) |
Substituting v=u∗ in expression (3.3), we obtain
ρf(vn,u∗)−ρf(vn,tn)≥⟨un−tn,u∗−tn⟩. | (3.4) |
Since u∗∈SEP(f,C), it follows that f(u∗,vn)≥0, implies that f(vn,u∗)≤0 due to the pseudomonotonicity of the bifunction f. From expression (3.4), we have
⟨un−tn,tn−u∗⟩≥ρf(vn,tn). | (3.5) |
Due to the Lipschitz-type condition on a bifunction f, we obtain
f(un,tn)≤f(un,vn)+f(vn,tn)+c1‖un−vn‖2+c2‖vn−tn‖2. | (3.6) |
Combining expressions (3.5) and (3.6), we have
⟨un−tn,tn−u∗⟩≥ρ{f(un,tn)−f(un,vn)}−c1ρ‖un−vn‖2−c2ρ‖vn−tn‖2. | (3.7) |
Since tn∈Hn and it gives that ⟨un−ρωn−vn,tn−vn⟩≤0, which implies that
⟨un−vn,tn−vn⟩≤ρ⟨ωn,tn−vn⟩. | (3.8) |
Since ωn∈∂2f(un,vn) and using the subdifferential definition, we obtain
f(un,v)−f(un,vn)≥⟨ωn,v−vn⟩,∀v∈H. |
Substituting v=tn in the above expression, we get
f(un,tn)−f(un,vn)≥⟨ωn,tn−vn⟩. | (3.9) |
It follows from inequalities (3.8) and (3.9) that
ρ{f(un,tn)−f(un,vn)}≥⟨un−vn,tn−vn⟩. | (3.10) |
From (3.7) and (3.10), we have
⟨un−tn,tn−u∗⟩≥⟨un−vn,tn−vn⟩−c1ρ‖un−vn‖2−c2ρ‖vn−tn‖2. | (3.11) |
We have the following equalities:
2⟨un−tn,tn−u∗⟩=‖un−u∗‖2−‖tn−un‖2−‖tn−u∗‖2 |
and
2⟨vn−un,vn−tn⟩=‖un−vn‖2+‖tn−vn‖2−‖un−tn‖2. |
The above facts and (3.11) implies that
‖tn−u∗‖2≤‖un−u∗‖2−(1−2c1ρ)‖un−vn‖2−(1−2c2ρ)‖tn−vn‖2. | (3.12) |
It is given that u∗∈SEP(f,C). From the definition of sequence {un+1} and due to the fact that g is a contraction with ξ∈[0,1), we have
‖un+1−u∗‖=‖χng(un)+(1−χn)tn−u∗‖=‖χn[g(un)−u∗]+(1−χn)[tn−u∗]‖=‖χn[g(un)+g(u∗)−g(u∗)−u∗]+(1−χn)[tn−u∗]‖≤χn‖g(un)−g(u∗)‖+χn‖g(u∗)−u∗‖+(1−χn)‖tn−u∗‖≤χnξ‖un−u∗‖+χn‖g(u∗)−u∗‖+(1−χn)‖tn−u∗‖. | (3.13) |
Combining expressions (3.12) and (3.13) and χn⊂(0,1), we deduce that
‖un+1−u∗‖≤χnξ‖un−u∗‖+χn‖g(u∗)−u∗‖+(1−χn)‖un−u∗‖=[1−χn+ξχn]‖un−u∗‖+χn(1−ξ)‖g(u∗)−u∗‖(1−ξ)≤max{‖un−u∗‖,‖g(u∗)−u∗‖(1−ξ)}≤max{‖u0−u∗‖,‖g(u∗)−u∗‖(1−ξ)}. | (3.14) |
Thus, we conclude that the {un} is bounded sequence. Due to the reflexivity of H and the boundedness of {un} guarantees that there exists a subsequence {unk} of {un} such that {unk}⇀u∗∈H as k→∞.
Claim 2: The sequence {un} is strongly convergent.
Next, we prove the strong convergence of the iterative sequence {un} generated by Algorithm 1. The Lipschitz-continuity and pseudomonotone property of the bifunction f implies that the solution set SEP(f,C) is a closed and convex set (for more details see [34]). Since the mapping is a contraction and so does PSEP(f,C)∘g. Now, we are in position to use the Banach contraction theorem for the existence of a unique fixed point u∗∈SEP(f,C) such that
u∗=PSEP(f,C)(g(u∗)). |
By using Lemma 2.1 (ⅱ), we have
⟨g(u∗)−u∗,v−u∗⟩≤0,∀v∈SEP(f,C). | (3.15) |
By Lemma 2.4 (ⅰ) and (3.12), we have
‖un+1−u∗‖2=‖χng(un)+(1−χn)tn−u∗‖2=‖χn[g(un)−u∗]+(1−χn)[tn−u∗]‖2=χn‖g(un)−u∗‖2+(1−χn)‖tn−u∗‖2−χn(1−χn)‖g(un)−tn‖2≤χn‖g(un)−u∗‖2+(1−χn)[‖un−u∗‖2−(1−2c1ρ)‖un−vn‖2−(1−2c2ρ)‖tn−vn‖2]−χn(1−χn)‖g(un)−tn‖2≤χn‖g(un)−u∗‖2+‖un−u∗‖2−(1−χn)(1−2c1ρ)‖un−vn‖2−(1−χn)(1−2c2ρ)‖tn−vn‖2. | (3.16) |
The remainder of the proof shall be split into the following two parts:
Case 1: Assume that there is a fixed number N1∈N such that
‖un+1−u∗‖≤‖un−u∗‖,∀n≥N1. | (3.17) |
Thus, above implies that limn→∞‖un−u∗‖ exists and let limn→∞‖un−u∗‖=l. From (3.16), we get
(1−χn)(1−2c1ρ)‖un−vn‖2+(1−χn)(1−2c2ρ)‖tn−vn‖2≤χn‖g(un)−u∗‖2+‖un−u∗‖2−‖un+1−u∗‖2. | (3.18) |
Due to the existence of limn→∞‖un−u∗‖=l and χn→0, we infer that
limn→∞‖un−vn‖=limn→∞‖tn−vn‖=0. | (3.19) |
It follows that
limn→∞‖un−tn‖≤limn→∞‖un−vn‖+limn→∞‖vn−tn‖=0. | (3.20) |
We can also obtain
‖un+1−un‖=‖χng(un)+(1−χn)tn−un‖=‖χn[g(un)−un]+(1−χn)[tn−un]‖≤χn‖g(un)−un‖+(1−χn)‖tn−un‖⟶0. | (3.21) |
The above expression implies that
limn→∞‖un+1−un‖=0. | (3.22) |
Thus, implies that the sequences {vn} and {tn} are bounded. Let {unk} be subsequence of {un} such that {unk} converges weakly to ˆu∈H. Next, we need to prove that ˆu∈SEP(f,C). Due to the inequality (3.3), the Lipschitz-like condition of f and (3.10), we obtain
ρf(vnk,v)≥ρf(vnk,tnk)+⟨unk−tnk,v−tnk⟩≥ρf(unk,tnk)−ρf(unk,vnk)−c1ρ‖unk−vnk‖2−c2ρ‖vnk−tnk‖2+⟨unk−tnk,v−tnk⟩≥⟨unk−vnk,tnk−vnk⟩−c1ρ‖unk−vnk‖2−c2ρ‖vnk−tnk‖2+⟨unk−tnk,v−tnk⟩, | (3.23) |
where v is an arbitrary member in Hn. From (3.19), (3.20) and the boundedness of {un} imply that right-hand side goes to zero. From ρ>0, the condition (a3) and vnk⇀ˆu, we obtain
0≤lim supk→∞f(vnk,v)≤f(ˆu,v),∀v∈Hn. | (3.24) |
It follows that f(ˆu,v)≥0, for all v∈C, and hence ˆu∈SEP(f,C). Next, we consider
lim supn→∞⟨g(u∗)−u∗,un−u∗⟩=lim supk→∞⟨g(u∗)−u∗,unk−u∗⟩=⟨g(u∗)−u∗,ˆu−u∗⟩≤0. | (3.25) |
We have limn→∞‖un+1−un‖=0. We can deduce that
lim supn→∞⟨g(u∗)−u∗,un+1−u∗⟩≤lim supn→∞⟨g(u∗)−u∗,un+1−un⟩+lim supn→∞⟨g(u∗)−u∗,un−u∗⟩≤0. | (3.26) |
From Lemma 2.4(ⅱ) and (3.12), we have
‖un+1−u∗‖2=‖χng(un)+(1−χn)tn−u∗‖2=‖χn[g(un)−u∗]+(1−χn)[tn−u∗]‖2≤(1−χn)2‖tn−u∗‖2+2χn⟨g(un)−u∗,(1−χn)[tn−u∗]+χn[g(un)−u∗]⟩=(1−χn)2‖tn−u∗‖2+2χn⟨g(un)−g(u∗)+g(u∗)−u∗,un+1−u∗⟩=(1−χn)2‖tn−u∗‖2+2χn⟨g(un)−g(u∗),un+1−u∗⟩+2χn⟨g(u∗)−u∗,un+1−u∗⟩≤(1−χn)2‖tn−u∗‖2+2χnξ‖un−u∗‖‖un+1−u∗‖+2χn⟨g(u∗)−u∗,un+1−u∗⟩≤(1+χ2n−2χn)‖un−u∗‖2+2χnξ‖un−u∗‖2+2χn⟨g(u∗)−u∗,un+1−u∗⟩=(1−2χn)‖un−u∗‖2+χ2n‖un−u∗‖2+2χnξ‖un−u∗‖2+2χn⟨g(u∗)−u∗,un+1−u∗⟩=[1−2χn(1−ξ)]‖un−u∗‖2+2χn(1−ξ)[χn‖un−u∗‖22(1−ξ)+⟨g(u∗)−u∗,un+1−u∗⟩1−ξ]. | (3.27) |
It follows from expressions (3.26) and (3.27), we obtain
lim supn→∞[χn‖un−u∗‖22(1−ξ)+⟨g(u∗)−u∗,un+1−u∗⟩1−ξ]≤0. | (3.28) |
Choose n≥N2∈N (N2≥N1) large enough such that 2χn(1−ξ)<1. By using (3.27), (3.28) and applying Lemma 2.2, we conclude that ‖un−u∗‖→0 as n→∞.
Case 2: Assume there is a subsequence {ni} of {n} such that
‖uni−u∗‖≤‖uni+1−u∗‖,∀i∈N. |
Thus, by Lemma 2.3 there is a sequence {mk}⊂N as {mk}→∞, such that
‖umk−u∗‖≤‖umk+1−u∗‖and‖uk−u∗‖≤‖umk+1−u∗‖,for allk∈N. | (3.29) |
Similar to Case 1, the expression (3.16) provides that
(1−χmk)(1−2c1ρ)‖umk−vmk‖2+(1−χmk)(1−2c2ρ)‖tmk−vmk‖2≤χmk‖g(umk)−u∗‖2+‖umk−u∗‖2−‖umk+1−u∗‖2. | (3.30) |
Due to χmk→0, we deduce the following:
limk→∞‖umk−vmk‖=limk→∞‖tmk−vmk‖=0. | (3.31) |
Also, we can obtain
‖umk+1−umk‖=‖χmkg(umk)+(1−χmk)tmk−umk‖=‖χmk[g(umk)−umk]+(1−χmk)[tmk−umk]‖≤χmk‖g(umk)−umk‖+(1−χmk)‖tmk−umk‖⟶0. | (3.32) |
We have to use the same justification as in the Case 1, such that
lim supk→∞⟨g(u∗)−u∗,umk+1−u∗⟩≤0. | (3.33) |
By using expressions (3.27) and (3.29), we have
‖umk+1−u∗‖2≤[1−2χmk(1−ξ)]‖umk−u∗‖2+2χmk(1−ξ)[χmk‖umk−u∗‖22(1−ξ)+⟨g(u∗)−u∗,umk+1−u∗⟩1−ξ]≤[1−2χmk(1−ξ)]‖umk+1−u∗‖2+2χmk(1−ξ)[χmk‖umk−u∗‖22(1−ξ)+⟨g(u∗)−u∗,umk+1−u∗⟩1−ξ]. | (3.34) |
It follows that
‖umk+1−u∗‖2≤χmk‖umk−u∗‖22(1−ξ)+⟨g(u∗)−u∗,umk+1−u∗⟩1−ξ. | (3.35) |
Since χmk→0, and ‖umk−u∗‖ is a bounded. Thus, expressions (3.33) and (3.35) implies that
‖umk+1−u∗‖2→0,ask→∞. | (3.36) |
The above implies that
limk→∞‖uk−u∗‖2≤limk→∞‖umk+1−u∗‖2≤0. | (3.37) |
Consequently, un→u∗. This completes the proof of the theorem.
Remark 3.2. If we define g(u)=u0 in Algorithm 1, we obtain the Halpern subetaadient extragradient method in [16].
Now, we consider the application of our main results to solve the problem of classic variational inequalities [31] for an operator G:H→H is defined in the following way:
Finsu∗∈Csuch that⟨G(u∗),v−u∗⟩≥0,∀v∈C.(VIP) |
We consider the following conditions to study variational inequalities.
(b1) The solution set of the problem (VIP) denoted by VI(G,C) is nonempty.
(b2)G:H→H is said to be a pseudomonotone, i.e.,
⟨G(u),v−u⟩≥0⟹⟨G(v),u−v⟩≤0,∀u,v∈C. |
(b3) G:H→H is said to be a Lipschitz continuous if there exits a constants L>0 such that
‖G(u)−G(v)‖≤L‖u−v‖,∀u,v∈C; |
(b4)G:H→H is called to be sequentially weakly continuous, i.e., {G(un)} weakly converges to G(u) for every sequence {un} converges weakly to u.
If we define f(u,v):=⟨G(u),v−u⟩, for all u,v∈C. Then, the equilibrium problem becomes the problem of variational inequalities described above where L=2c1=2c2. From the above value of the bifunction f, we have
{vn=argminv∈C{ρf(un,v)+12‖un−v‖2}=PC(un−ρG(un)),tn=argminv∈Hn{ρf(vn,v)+12‖un−v‖2}=PHn(un−ρG(vn)). | (4.1) |
As a consequence of the results in Section 3, we have the following results:
Corollary 4.1. Let G:C→H be a mapping satisfying the conditions (b1)–(b4). Choose u0∈C, 0<ρ<1L and a sequence χn⊂(0,1) satisfying the conditions, i.e.,
limn→∞χn=0and+∞∑nχn=+∞. |
Assume that {un} is the sequence generated in the following way:
{vn=PC(un−ρG(un)),tn=PHn(un−ρG(vn)),un+1=χng(un)+(1−χn)tn, |
where
Hn={z∈H:⟨un−ρG(un)−vn,z−vn⟩≤0}. |
Then, the sequence {un} converges strongly to u∗∈VI(G,C).
Note that condition (b4) can be deleted in the case when G is monotone. Indeed, this condition is a particular case of condition (a3) is used to prove (3.24). Without condition (b4), the inequality (3.23) is obtained by imposing the monotonocity on G. In that case, we obtain
⟨G(v),v−vn⟩≥⟨G(vn),v−vn⟩,∀v∈C. | (4.2) |
By the use f(u,v)=⟨G(u),v−u⟩ in (3.23), we get
lim supk→∞⟨G(vnk),v−vnk⟩≥0,∀v∈Hn. | (4.3) |
Combining (4.2) with (4.3), we obtain
lim supk→∞⟨G(v),v−vnk⟩≥0,∀v∈C. | (4.4) |
Let vs=(1−s)ˆu+sy, for all s∈[0,1]. Due to convexity of C implies that vs∈C for any s∈(0,1). Since vnk⇀ˆu∈C and ⟨G(v),v−ˆu⟩≥0 for every v∈C. Thus, we have
0≤⟨G(vs),vs−ˆu⟩=s⟨G(vs),v−ˆu⟩. | (4.5) |
Therefore, ⟨G(vs),v−ˆu⟩≥0, for all s∈(0,1). Since vs→ˆu as s→0 and due to continuity of G, we have ⟨G(ˆu),v−ˆu⟩≥0, for all v∈C, which implies that ˆu∈VI(G,C).
Remark 4.1. From the above discussion, it can be concluded that the Corollary 4.1 still holds, even if we remove the condition (b4) in case of monotone variational inequaltiy.
Next, we consider the application of our results to solve fixed-point problems involving κ-strict pseudocontraction mapping and the fixed point problem for an operator T:H→H is defined in the following way:
Findu∗∈Csuch thatT(u∗)=u∗.(FPP) |
We assume that the following requirements have been met to study fixed point problems.
(c1)T:C→C is said to be a κ-strict pseudo-contraction [7] on C if
‖Tu−Tv‖2≤‖u−v‖2+κ‖(u−Tu)−(v−Tv)‖2,∀u,v∈C; |
(c2) weakly sequentially continuous on C if
T(un)⇀T(u∗)for any sequence inCsatisfyun⇀u∗. |
If we consider that the mapping T is a κ-strict pseudocontraction and weakly continuous then f(u,v)=⟨u−Tu,v−u⟩ satisfies the conditions (a1)–(a4) and 2c1=2c2=3−2κ1−κ. The values of vn and tn in Algorithm 1, we have
{vn=argminv∈C{ρf(un,v)+12‖un−v‖2}=PC[un−ρ(un−T(un))],tn=argminv∈Hn{ρf(vn,v)+12‖un−v‖2}=PHn[un−ρ(vn−T(vn))]. | (4.6) |
Corollary 4.2. Let C be a nonempty, convex and closed subset of a Hilbert space H and T:C→C is a κ-strict pseudocontraction and weakly continuous with solution set Fix(T)≠∅. Choose u0∈C, 0<ρ<1−κ3−2κ and sequence χn⊂(0,1) satisfies the conditions, i.e.,
limn→∞χn=0and+∞∑nχn=+∞. |
Let {un} be the sequence generated in the following way:
{vn=PC[un−ρ(un−T(un))],tn=PHn[un−ρ(vn−T(vn))],un+1=χng(un)+(1−χn)tn. |
where
Hn={w∈H:⟨(1−ρ)un+ρT(un)−vn,w−vn⟩≤0}. |
Then, sequence {un} strongly converges to u∗∈Fix(T).
Numerical results are presented in this section to show the efficiency of the proposed method. The MATLAB codes was run in MATLAB version 9.5 (R2018b) on the Intel(R) Core(TM)i5-6200 CPU PC @ 2.30GHz 2.40GHz, RAM 8.00 GB.
Example 5.1. Assume that f:C×C→R is defined by
f(u,v)=⟨Pu+Qv+c,v−u⟩,∀u,v∈C, |
where c∈Rn and P, Q are matrices of order n. The matrix P is symmetric positive semi-definite and the matrix Q−P is symmetric negative semi-definite with Lipschitz-type constants c1=c2=12‖P−Q‖ (see [34] for details). The matrices P,Q and vector c are defined by
P=(3.1200023.6000003.5200023.3000003)Q=(1.6100011.6000001.5100011.5000002)c=(1−2−12−1). |
The constraint set C⊂Rn is considered as C:={u∈Rn:−5≤ui≤5}. Furthermore, control parameters conditions are taken as follows: ρ=14c1 and Dn=‖un−vn‖ for Algorithm 1 (EgM) in [34]; ρ=14c1, χn=1100(n+2) and Dn=‖un−vn‖ for Algorithm 2 (H-EgM) in [16]; ρ=14c1,χn=1100(n+2), g(u)=u2 and Dn=‖un−vn‖ for Algorithm 1 (V-EgM). The numerical and graphical results of three methods are shown in Figures 1–4 and Table 1.
Number of Iterations | Execution Time in Seconds | |||||
u0 | EgM | H-EgM | V-EgM | EgM | H-EgM | V-EgM |
(0,0,0,0,0)T | 27 | 32 | 21 | 0.217359 | 0.242564 | 0.226170 |
(2,2,2,2,2)T | 27 | 60 | 21 | 0.264663 | 0.553107 | 0.175542 |
(1,0,−1,2,1)T | 31 | 49 | 24 | 0.292574 | 0.576171 | 0.219645 |
(2,−1,3,−4,5)T | 32 | 80 | 25 | 0.303238 | 0.635728 | 0.204971 |
Example 5.2. Let f:C×C→R defined in the following way:
f(u,v)=5∑i=2(vi−ui)‖u‖,∀u,v∈R5, |
where C={(u1,⋯,u5):u1≥−1,ui≥1,i=2,⋯,5}. Thus, the bifunction f is Lipschitz-type continuous with c1=c2=2, and satisfies the conditions (a1)–(a4). The solution set of an equilibrium problem is EP(f,C)={(u1,1,1,1,1):u1>1}. Furthermore, the control conditions ρ=16c1 for Algorithm 1 (EgM) in [34]; ρ=16c1 and χn=1200(n+2) for Algorithm 2 (H-EgM) in [16]; ρ=16c1,χn=1100(n+2) and g(u)=u3 for Algorithm 1 (V-EgM). The numerical results of three methods are shown in Tables 2–4.
Iter (n) | u1 | u2 | u3 | u4 | u5 |
1 | 1.9999999902 | 8.9034597770 | 11.903459749 | 3.9034598398 | 1.9034598209 |
2 | 2.0000001112 | 7.9193730581 | 10.919372983 | 2.9193733082 | 1.0000084500 |
3 | 2.0000001050 | 7.0300765337 | 10.030076426 | 2.0300768272 | 1.0000000224 |
4 | 2.0000002139 | 6.2245554051 | 9.2245552403 | 1.2245575794 | 1.0000004995 |
5 | 2.0000002018 | 5.4892537351 | 8.4892535376 | 1.0000000391 | 1.0000000272 |
6 | 2.0000001984 | 4.8171736152 | 7.8171733928 | 1.0000000297 | 1.0000000297 |
⋮ | ⋮ | ⋮ | ⋮ | ⋮ | ⋮ |
26 | 2.0000015507 | 1.0000019717 | 1.0384612829 | 1.0000019717 | 1.0000019717 |
27 | 2.0000016692 | 1.0000021759 | 1.0000028248 | 1.0000021759 | 1.0000021759 |
28 | 2.0000017877 | 1.0000021759 | 1.0000021759 | 1.0000021759 | 1.0000021759 |
CPU time is seconds | 0.850380 |
Iter (n) | |||||
1 | 1.999999942 | 8.7515611443 | 11.751561124 | 3.7515611399 | 1.7515612532 |
2 | 1.999999938 | 7.6461082189 | 10.646108180 | 2.6461082675 | 1.0016667229 |
3 | 1.999999939 | 6.6610509620 | 9.6610509019 | 1.6610510862 | 1.0012500202 |
4 | 2.000000061 | 5.7776478420 | 8.7776477159 | 1.0020019229 | 1.0010004543 |
5 | 2.000000055 | 4.9810038039 | 7.9810036568 | 1.0016666917 | 1.0008333584 |
6 | 2.000000048 | 4.2622453450 | 7.2622451730 | 1.0014285992 | 1.0007143134 |
⋮ | ⋮ | ⋮ | ⋮ | ⋮ | ⋮ |
38 | 2.0000028210 | 1.0005787475 | 1.0007710569 | 1.0002582317 | 1.0001300255 |
39 | 2.0000029393 | 1.0005643243 | 1.0007518260 | 1.0002518214 | 1.0001268203 |
40 | 2.0000030576 | 1.0005506046 | 1.0007335331 | 1.0002457238 | 1.0001237715 |
CPU time is seconds | 1.191645 |
Iter (n) | |||||
1 | 1.9950000119 | 8.5308876762 | 11.523387660 | 3.5433877312 | 1.54838779261 |
2 | 1.9916750267 | 7.2594419489 | 10.246954421 | 2.2802545702 | 0.99924734095 |
3 | 1.9891855417 | 6.1501278861 | 9.1339059323 | 1.1771670518 | 0.99874942183 |
4 | 1.9871963569 | 5.1700590512 | 8.1508532923 | 0.9991771920 | 0.99899876985 |
5 | 1.9855403611 | 4.3036279625 | 7.2819381754 | 0.9991660040 | 0.99916585540 |
6 | 1.9841221147 | 3.5358595487 | 6.5120423683 | 0.9992851446 | 0.99928514452 |
⋮ | ⋮ | ⋮ | ⋮ | ⋮ | ⋮ |
19 | 1.9741765217 | 0.99975135569 | 1.0030932482 | 0.99975135569 | 0.99975135569 |
20 | 1.9737065994 | 0.99976333843 | 0.9997641538 | 0.99976333843 | 0.99976333843 |
21 | 1.9732581496 | 0.99977416665 | 0.99977416684 | 0.99977416665 | 0.99977416665 |
CPU time is seconds | 0.711785 |
Example 5.3. Suppose that H=L2([0,1]) is a Hilbert space with the inner product ⟨u,v⟩=∫10u(t)v(t)dt, for all u,v∈H and the induced norm is
‖u‖=√∫10|u(t)|2dt. |
Moreover, assume that C:={u∈L2([0,1]):‖u‖≤1}. Assume that G:C→H is defined by
G(u)(t)=∫10[u(t)−H(t,s)f(u(s))]ds+g(t) |
where H(t,s)=2tse(t+s)e√e2−1,f(u)=cos(u) and g(t)=2tete√e2−1. We consider the bifunction f(u,v)=⟨G(u),v−u⟩ with the Lipschitz-type continuous with c1=c2=1. Furthermore, control conditions ρ=15c1 for Algorithm 1 (EgM) in [34]; ρ=15c1 and χn=1300(n+2) for Algorithm 2 (H-EgM) in [16]; ρ=15c1,χn=1100(n+2) and g(u)=u2 for Algorithm 1 (V-EgM). The numerical results of three methods are shown in Figures 5 and 6 and Table 5.
Number of Iterations | Execution Time in Seconds | |||||
u0 | EgM | H-EgM | V-EgM | EgM | H-EgM | V-EgM |
t | 49 | 106 | 41 | 0.058515 | 0.107587 | 0.052661 |
3t2 | 52 | 54 | 40 | 0.057655 | 0.058550 | 0.042137 |
sin(t) | 52 | 76 | 41 | 0.056489 | 0.083268 | 0.057777 |
exp(t) | 55 | 97 | 52 | 0.130014 | 0.106885 | 0.061795 |
Example 5.4. Assume that f:H×H→R is defined by
f(u,v)=(5−‖u‖)⟨u,v−u⟩,∀u,v∈H, |
where H=l2 is a real Hilbert space having the elements are square-summable sequences and C={u∈H:‖u‖≤3}. The bifunction f is Lipschitz-type continuous and value of Lipschitz-constants are c1=c2=112. Furthermore, control conditions ρ=14c1 for Algorithm 1 (EgM) in [34]; ρ=14c1 and χn=1200(n+2) for Algorithm 2 (H-EgM) in [16]; ρ=14c1,χn=1100(n+2) and g(u)=u2 for Algorithm 1 (V-EgM). The numerical results of three methods are shown in Figures 7 and 8 and Table 6. The projection onto C is evaluated in the following way:
PC(u)={uif‖u‖≤3,3u‖u‖,else. |
Number of Iterations | Execution Time in Seconds | ||||||
u0 | EgM | H-EgM | V-EgM | EgM | H-EgM | V-EgM | |
(1,1,⋯,1500,0,0,⋯) | 39 | 59 | 33 | 1.233697 | 1.849991 | 1.8499918 | |
(1,2,⋯,1000,0,0,⋯) | 55 | 68 | 49 | 1.773837 | 2.065840 | 1.5172537 |
We have studied a viscosity type extragradient-like method for determining the solution of pseudomonotone equilibrium problem in real Hilbert spaces and also prove that the generated sequence converges strongly to the solution. Numerical conclusions were drawn to explain the numerical efficiency of our algorithms in comparison to other methods. These numerical studies showed that viscosity influences improve the efficiency of the iterative sequence in this context.
This research work was financially supported by King Mongkut's University of Technology Thonburi through the "KMUTT 55th Anniversary Commemorative Fund". In particular, Habib ur Rehman was financed by the Petchra Pra Jom Doctoral Scholarship Academic for Ph.D. Program at KMUTT [grant number 39/2560]. Moreover, this project was supported by Center of Excellence in Theoretical and Computational Science (TaCS-CoE), KMUTT. Also, this project was financially supported by King Mongkut’s University of Technology North Bangkok, Contract no. KMUTNB-BasicR-64-22.
The first author would like to thank the "Petchra Pra Jom Klao Ph.D. Research Scholarship from King Mongkut’s University of Technology Thonburi". We are very grateful to the editor and the anonymous referees for their valuable and useful comments, which helps in improving the quality of this work.
No potential conflict of interest was reported by the author(s).
[1] | P. N. Anh, L. T. H. An, The subgradient extragradient method extended to equilibrium problems, Optimization, 64 (2012), 225-248. |
[2] | A. Antipin, Equilibrium programming: proximal methods, Comput. Math. Math. Phys., 37 (1997), 1285-1296. |
[3] | M. Bianchi, S. Schaible, Generalized monotone bifunctions and equilibrium problems, J. Optimiz. Theory App., 9 (1996), 31-43. |
[4] |
G. Bigi, M. Castellani, M. Pappalardo, M. Passacantando, Existence and solution methods for equilibria, Eur. J. Oper. Res., 227 (2013), 1-11. doi: 10.1016/j.ejor.2012.11.037
![]() |
[5] | G. Bigi, M. Castellani, M. Pappalardo, M. Passacantando, Nonlinear programming techniques for equilibria, Springer International Publishing, 2019. |
[6] | E. Blum, From optimization and variational inequalities to equilibrium problems, Mathematics Students, 63 (1994), 123-145. |
[7] |
F. Browder, W. Petryshyn, Construction of fixed points of nonlinear mappings in hilbert space, J. Math. Anal. Appl., 20 (1967), 197-228. doi: 10.1016/0022-247X(67)90085-6
![]() |
[8] | Y. Censor, A. Gibali, S. Reich, The subgradient extragradient method for solving variational inequalities in hilbert space, J. Optimiz. Theory App., 148 (2010), 318-335. |
[9] |
C. E. Chidume, A. Adamu, L. C. Okereke, A krasnoselskii-type algorithm for approximating solutions of variational inequality problems and convex feasibility problems, J. Nonlinear Var. Anal., 2 (2018), 203-218. doi: 10.23952/jnva.2.2018.2.07
![]() |
[10] |
S. Dafermos, Traffic equilibrium and variational inequalities, Transport. Sci., 14 (1980), 42-54. doi: 10.1287/trsc.14.1.42
![]() |
[11] | K. Fan, A minimax inequality and applications, Inequalities Ⅲ, New York: Academic Press, 1972. |
[12] | M. Farid, The subgradient extragradient method for solving mixed equilibrium problems and fixed point problems in hilbert spaces, J. Appl. Numer. Optim., 1 (2019), 335-345. |
[13] |
S. D. Flåm, A. S. Antipin, Equilibrium programming using proximal-like algorithms, Math. Program., 78 (1996), 29-41. doi: 10.1007/BF02614504
![]() |
[14] | F. Giannessi, A. Maugeri, P. M. Pardalos, Equilibrium problems: Nonsmooth optimization and variational inequality models, Springer Science & Business Media, 2006. |
[15] | H. H. Bauschke, P. L. Combettes, Convex analysis and monotone operator theory in Hilbert spaces, New York: Springer, 2017. |
[16] | D. V. Hieu, Halpern subgradient extragradient method extended to equilibrium problems, RACSAM, 111 (2016), 823-840. |
[17] |
D. V. Hieu, P. K. Quy, L. V. Vy, Explicit iterative algorithms for solving equilibrium problems, Calcolo, 56 (2019), 11. doi: 10.1007/s10092-019-0308-5
![]() |
[18] | G. Korpelevich, The extragradient method for finding saddle points and other problems, Matecon, 12 (1976), 747-756. |
[19] | S. I. Lyashko, V. V. Semenov, A new two-step proximal algorithm of solving the problem of equilibrium programming, In: Optimization and its applications in control and data sciences, Springer International Publishing, 2016,315-325. |
[20] |
P. E. Maingé, Strong convergence of projected subgradient methods for nonsmooth and nonstrictly convex minimization, Set-Valued Anal., 16 (2008), 899-912. doi: 10.1007/s11228-008-0102-z
![]() |
[21] | G. Mastroeni, On auxiliary principle for equilibrium problems, In: Nonconvex optimization and its applications, Springer, 2003,289-298. |
[22] | A. Moudafi, Proximal point algorithm extended to equilibrium problems, J. Nat. Geom., 15 (1999), 91-100. |
[23] |
A. Moudafi, Viscosity approximation methods for fixed-points problems, J. Math. Anal. Appl., 241 (2000), 46-55. doi: 10.1006/jmaa.1999.6615
![]() |
[24] |
L. Muu, W. Oettli, Convergence of an adaptive penalty scheme for finding constrained equilibria, Nonlinear Anal. Theor., 18 (1992), 1159-1166. doi: 10.1016/0362-546X(92)90159-C
![]() |
[25] | F. U. Ogbuisi, Y. Shehu, A projected subgradient-proximal method for split equality equilibrium problems of pseudomonotone bifunctions in banach spaces, J. Nonlinear Var. Anal., 3 (2019), 205-224. |
[26] | T. D. Quoc, P. N. Anh, L. D. Muu, Dual extragradient algorithms extended to equilibrium problems, J. Global Optim., 52 (2011), 139-159. |
[27] |
T. D. Quoc, M. N. V. H. Le Dung, Extragradient algorithms extended to equilibrium problems, Optimization, 57 (2008), 749-776. doi: 10.1080/02331930601122876
![]() |
[28] | R. T. Rockafellar, Convex analysis, Princeton University Press, 1970. |
[29] | P. Santos, S. Scheimberg, An inexact subgradient algorithm for equilibrium problems, Comput. Appl. Math., 30 (2011), 91-107. |
[30] | T. M. M. Sow, An iterative algorithm for solving equilibrium problems, variational inequalities and fixed point problems of multivalued quasi-nonexpansive mappings, Appl. Set-Valued Anal. Optim., 1 (2019), 171-185. |
[31] | G. Stampacchia, Formes bilinéaires coercitives sur les ensembles convexes, Comptes Rendus Hebdomadaires Des Seances De L Academie Des Sciences, 258 (1964), 4413. |
[32] |
S. Takahashi, W. Takahashi, Viscosity approximation methods for equilibrium problems and fixed point problems in hilbert spaces, J. Math. Anal. Appl., 331 (2007), 506-515. doi: 10.1016/j.jmaa.2006.08.036
![]() |
[33] |
L. Q. Thuy, C. F. Wen, J. C. Yao, T. N. Hai, An extragradient-like parallel method for pseudomonotone equilibrium problems and semigroup of nonexpansive mappings, Miskolc Math. Notes, 19 (2018), 1185. doi: 10.18514/MMN.2018.2114
![]() |
[34] |
D. Q. Tran, M. L. Dung, V. H. Nguyen, Extragradient algorithms extended to equilibrium problems, Optimization, 57 (2008), 749-776. doi: 10.1080/02331930601122876
![]() |
[35] | H. ur Rehman, P. Kumam, A. B. Abubakar, Y. J. Cho, The extragradient algorithm with inertial effects extended to equilibrium problems, Comp. Appl. Math., 39 (2010), 100. |
[36] |
H. ur Rehman, P. Kumam, Y. J. Cho, P. Yordsorn, Weak convergence of explicit extragradient algorithms for solving equilibirum problems. J. Inequal. Appl., 2019 (2019), 282. doi: 10.1186/s13660-019-2233-1
![]() |
[37] | H. ur Rehman, P. Kumam, Y. Je Cho, Y.I. Suleiman, W. Kumam, Modified popov's explicit iterative algorithms for solving pseudomonotone equilibrium problems. Optimization Methods and Software, 2020, 1-32. |
[38] |
H. ur Rehman, P. Kumam, W. Kumam, M. Shutaywi, W. Jirakitpuwapat, The inertial sub-gradient extra-gradient method for a class of pseudo-monotone equilibrium problems, Symmetry, 12 (2020), 463. doi: 10.3390/sym12030463
![]() |
[39] |
N. T. Vinh, L. D. Muu, Inertial extragradient algorithms for solving equilibrium problems, Acta Mathematica Vietnamica, 44 (2019), 639-663. doi: 10.1007/s40306-019-00338-1
![]() |
[40] |
H. K. Xu, Another control condition in an iterative method for nonexpansive mappings, B. Aust. Math. Soc., 65 (2002), 109-113. doi: 10.1017/S0004972700020116
![]() |
[41] |
J. C. Yao, Variational inequalities with generalized monotone operators, Math. Oper. Res., 19 (1994), 691-705. doi: 10.1287/moor.19.3.691
![]() |
1. | Habib ur Rehman, Wiyada Kumam, Poom Kumam, Meshal Shutaywi, A new weak convergence non-monotonic self-adaptive iterative scheme for solving equilibrium problems, 2021, 6, 2473-6988, 5612, 10.3934/math.2021332 | |
2. | Chainarong Khanpanuk, Nuttapol Pakkaranang, Nopparat Wairojjana, Nattawut Pholasa, Approximations of an Equilibrium Problem without Prior Knowledge of Lipschitz Constants in Hilbert Spaces with Applications, 2021, 10, 2075-1680, 76, 10.3390/axioms10020076 | |
3. | Charu Batra, Nishu Gupta, Renu Chugh, Rajeev Kumar, Generalized viscosity extragradient algorithm for pseudomonotone equilibrium and fixed point problems for finite family of demicontractive operators, 2022, 68, 1598-5865, 4195, 10.1007/s12190-022-01699-x | |
4. | Pongsakorn Yotkaew, Nopparat Wairojjana, Nuttapol Pakkaranang, Accelerated non-monotonic explicit proximal-type method for solving equilibrium programming with convex constraints and its applications, 2021, 6, 2473-6988, 10707, 10.3934/math.2021622 | |
5. | Olawale Kazeem Oyewole, Simeon Reich, An Inertial Subgradient Extragradient Method for Approximating Solutions to Equilibrium Problems in Hadamard Manifolds, 2023, 12, 2075-1680, 256, 10.3390/axioms12030256 | |
6. | Nopparat Wairojjana, Chainarong Khunpanuk, Nuttapol Pakkaranang, Strong convergence analysis of modified Mann-type forward–backward scheme for solving quasimonotone variational inequalities, 2023, 16, 1793-5571, 10.1142/S1793557123500912 |
Number of Iterations | Execution Time in Seconds | |||||
u0 | EgM | H-EgM | V-EgM | EgM | H-EgM | V-EgM |
(0,0,0,0,0)T | 27 | 32 | 21 | 0.217359 | 0.242564 | 0.226170 |
(2,2,2,2,2)T | 27 | 60 | 21 | 0.264663 | 0.553107 | 0.175542 |
(1,0,−1,2,1)T | 31 | 49 | 24 | 0.292574 | 0.576171 | 0.219645 |
(2,−1,3,−4,5)T | 32 | 80 | 25 | 0.303238 | 0.635728 | 0.204971 |
Iter (n) | u1 | u2 | u3 | u4 | u5 |
1 | 1.9999999902 | 8.9034597770 | 11.903459749 | 3.9034598398 | 1.9034598209 |
2 | 2.0000001112 | 7.9193730581 | 10.919372983 | 2.9193733082 | 1.0000084500 |
3 | 2.0000001050 | 7.0300765337 | 10.030076426 | 2.0300768272 | 1.0000000224 |
4 | 2.0000002139 | 6.2245554051 | 9.2245552403 | 1.2245575794 | 1.0000004995 |
5 | 2.0000002018 | 5.4892537351 | 8.4892535376 | 1.0000000391 | 1.0000000272 |
6 | 2.0000001984 | 4.8171736152 | 7.8171733928 | 1.0000000297 | 1.0000000297 |
⋮ | ⋮ | ⋮ | ⋮ | ⋮ | ⋮ |
26 | 2.0000015507 | 1.0000019717 | 1.0384612829 | 1.0000019717 | 1.0000019717 |
27 | 2.0000016692 | 1.0000021759 | 1.0000028248 | 1.0000021759 | 1.0000021759 |
28 | 2.0000017877 | 1.0000021759 | 1.0000021759 | 1.0000021759 | 1.0000021759 |
CPU time is seconds | 0.850380 |
Iter (n) | |||||
1 | 1.999999942 | 8.7515611443 | 11.751561124 | 3.7515611399 | 1.7515612532 |
2 | 1.999999938 | 7.6461082189 | 10.646108180 | 2.6461082675 | 1.0016667229 |
3 | 1.999999939 | 6.6610509620 | 9.6610509019 | 1.6610510862 | 1.0012500202 |
4 | 2.000000061 | 5.7776478420 | 8.7776477159 | 1.0020019229 | 1.0010004543 |
5 | 2.000000055 | 4.9810038039 | 7.9810036568 | 1.0016666917 | 1.0008333584 |
6 | 2.000000048 | 4.2622453450 | 7.2622451730 | 1.0014285992 | 1.0007143134 |
⋮ | ⋮ | ⋮ | ⋮ | ⋮ | ⋮ |
38 | 2.0000028210 | 1.0005787475 | 1.0007710569 | 1.0002582317 | 1.0001300255 |
39 | 2.0000029393 | 1.0005643243 | 1.0007518260 | 1.0002518214 | 1.0001268203 |
40 | 2.0000030576 | 1.0005506046 | 1.0007335331 | 1.0002457238 | 1.0001237715 |
CPU time is seconds | 1.191645 |
Iter (n) | |||||
1 | 1.9950000119 | 8.5308876762 | 11.523387660 | 3.5433877312 | 1.54838779261 |
2 | 1.9916750267 | 7.2594419489 | 10.246954421 | 2.2802545702 | 0.99924734095 |
3 | 1.9891855417 | 6.1501278861 | 9.1339059323 | 1.1771670518 | 0.99874942183 |
4 | 1.9871963569 | 5.1700590512 | 8.1508532923 | 0.9991771920 | 0.99899876985 |
5 | 1.9855403611 | 4.3036279625 | 7.2819381754 | 0.9991660040 | 0.99916585540 |
6 | 1.9841221147 | 3.5358595487 | 6.5120423683 | 0.9992851446 | 0.99928514452 |
⋮ | ⋮ | ⋮ | ⋮ | ⋮ | ⋮ |
19 | 1.9741765217 | 0.99975135569 | 1.0030932482 | 0.99975135569 | 0.99975135569 |
20 | 1.9737065994 | 0.99976333843 | 0.9997641538 | 0.99976333843 | 0.99976333843 |
21 | 1.9732581496 | 0.99977416665 | 0.99977416684 | 0.99977416665 | 0.99977416665 |
CPU time is seconds | 0.711785 |
Number of Iterations | Execution Time in Seconds | |||||
u0 | EgM | H-EgM | V-EgM | EgM | H-EgM | V-EgM |
t | 49 | 106 | 41 | 0.058515 | 0.107587 | 0.052661 |
3t2 | 52 | 54 | 40 | 0.057655 | 0.058550 | 0.042137 |
sin(t) | 52 | 76 | 41 | 0.056489 | 0.083268 | 0.057777 |
exp(t) | 55 | 97 | 52 | 0.130014 | 0.106885 | 0.061795 |
Number of Iterations | Execution Time in Seconds | ||||||
u0 | EgM | H-EgM | V-EgM | EgM | H-EgM | V-EgM | |
(1,1,⋯,1500,0,0,⋯) | 39 | 59 | 33 | 1.233697 | 1.849991 | 1.8499918 | |
(1,2,⋯,1000,0,0,⋯) | 55 | 68 | 49 | 1.773837 | 2.065840 | 1.5172537 |
Number of Iterations | Execution Time in Seconds | |||||
u0 | EgM | H-EgM | V-EgM | EgM | H-EgM | V-EgM |
(0,0,0,0,0)T | 27 | 32 | 21 | 0.217359 | 0.242564 | 0.226170 |
(2,2,2,2,2)T | 27 | 60 | 21 | 0.264663 | 0.553107 | 0.175542 |
(1,0,−1,2,1)T | 31 | 49 | 24 | 0.292574 | 0.576171 | 0.219645 |
(2,−1,3,−4,5)T | 32 | 80 | 25 | 0.303238 | 0.635728 | 0.204971 |
Iter (n) | u1 | u2 | u3 | u4 | u5 |
1 | 1.9999999902 | 8.9034597770 | 11.903459749 | 3.9034598398 | 1.9034598209 |
2 | 2.0000001112 | 7.9193730581 | 10.919372983 | 2.9193733082 | 1.0000084500 |
3 | 2.0000001050 | 7.0300765337 | 10.030076426 | 2.0300768272 | 1.0000000224 |
4 | 2.0000002139 | 6.2245554051 | 9.2245552403 | 1.2245575794 | 1.0000004995 |
5 | 2.0000002018 | 5.4892537351 | 8.4892535376 | 1.0000000391 | 1.0000000272 |
6 | 2.0000001984 | 4.8171736152 | 7.8171733928 | 1.0000000297 | 1.0000000297 |
⋮ | ⋮ | ⋮ | ⋮ | ⋮ | ⋮ |
26 | 2.0000015507 | 1.0000019717 | 1.0384612829 | 1.0000019717 | 1.0000019717 |
27 | 2.0000016692 | 1.0000021759 | 1.0000028248 | 1.0000021759 | 1.0000021759 |
28 | 2.0000017877 | 1.0000021759 | 1.0000021759 | 1.0000021759 | 1.0000021759 |
CPU time is seconds | 0.850380 |
Iter (n) | |||||
1 | 1.999999942 | 8.7515611443 | 11.751561124 | 3.7515611399 | 1.7515612532 |
2 | 1.999999938 | 7.6461082189 | 10.646108180 | 2.6461082675 | 1.0016667229 |
3 | 1.999999939 | 6.6610509620 | 9.6610509019 | 1.6610510862 | 1.0012500202 |
4 | 2.000000061 | 5.7776478420 | 8.7776477159 | 1.0020019229 | 1.0010004543 |
5 | 2.000000055 | 4.9810038039 | 7.9810036568 | 1.0016666917 | 1.0008333584 |
6 | 2.000000048 | 4.2622453450 | 7.2622451730 | 1.0014285992 | 1.0007143134 |
⋮ | ⋮ | ⋮ | ⋮ | ⋮ | ⋮ |
38 | 2.0000028210 | 1.0005787475 | 1.0007710569 | 1.0002582317 | 1.0001300255 |
39 | 2.0000029393 | 1.0005643243 | 1.0007518260 | 1.0002518214 | 1.0001268203 |
40 | 2.0000030576 | 1.0005506046 | 1.0007335331 | 1.0002457238 | 1.0001237715 |
CPU time is seconds | 1.191645 |
Iter (n) | |||||
1 | 1.9950000119 | 8.5308876762 | 11.523387660 | 3.5433877312 | 1.54838779261 |
2 | 1.9916750267 | 7.2594419489 | 10.246954421 | 2.2802545702 | 0.99924734095 |
3 | 1.9891855417 | 6.1501278861 | 9.1339059323 | 1.1771670518 | 0.99874942183 |
4 | 1.9871963569 | 5.1700590512 | 8.1508532923 | 0.9991771920 | 0.99899876985 |
5 | 1.9855403611 | 4.3036279625 | 7.2819381754 | 0.9991660040 | 0.99916585540 |
6 | 1.9841221147 | 3.5358595487 | 6.5120423683 | 0.9992851446 | 0.99928514452 |
⋮ | ⋮ | ⋮ | ⋮ | ⋮ | ⋮ |
19 | 1.9741765217 | 0.99975135569 | 1.0030932482 | 0.99975135569 | 0.99975135569 |
20 | 1.9737065994 | 0.99976333843 | 0.9997641538 | 0.99976333843 | 0.99976333843 |
21 | 1.9732581496 | 0.99977416665 | 0.99977416684 | 0.99977416665 | 0.99977416665 |
CPU time is seconds | 0.711785 |
Number of Iterations | Execution Time in Seconds | |||||
u0 | EgM | H-EgM | V-EgM | EgM | H-EgM | V-EgM |
t | 49 | 106 | 41 | 0.058515 | 0.107587 | 0.052661 |
3t2 | 52 | 54 | 40 | 0.057655 | 0.058550 | 0.042137 |
sin(t) | 52 | 76 | 41 | 0.056489 | 0.083268 | 0.057777 |
exp(t) | 55 | 97 | 52 | 0.130014 | 0.106885 | 0.061795 |
Number of Iterations | Execution Time in Seconds | ||||||
u0 | EgM | H-EgM | V-EgM | EgM | H-EgM | V-EgM | |
(1,1,⋯,1500,0,0,⋯) | 39 | 59 | 33 | 1.233697 | 1.849991 | 1.8499918 | |
(1,2,⋯,1000,0,0,⋯) | 55 | 68 | 49 | 1.773837 | 2.065840 | 1.5172537 |