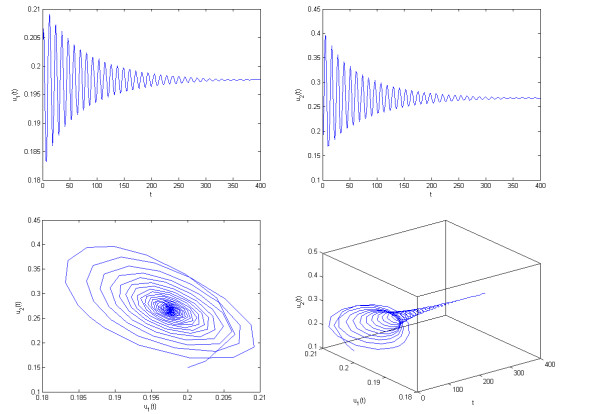
Recently, delayed dynamical model has witnessed a great interest from many scholars in biological and mathematical areas due to its potential application in describing the interaction of different biological populations. In this article, relying the previous studies, we set up two new predator-prey systems incorporating delay. By virtue of fixed point theory, inequality tactics and an appropriate function, we explore well-posedness (includes existence and uniqueness, boundedness and non-negativeness) of the solution of the two formulated delayed predator-prey systems. With the aid of bifurcation theorem and stability theory of delayed differential equations, we gain the parameter conditions on the emergence of stability and bifurcation phenomenon of the two formulated delayed predator-prey systems. By applying two controllers (hybrid controller and extended delayed feedback controller) we can efficaciously regulate the region of stability and the time of occurrence of bifurcation phenomenon for the two delayed predator-prey systems. The effect of delay on stabilizing the system and adjusting bifurcation is investigated. Computer simulation plots are provided to sustain the acquired prime outcomes. The conclusions of this article are completely new and can provide some momentous instructions in dominating and balancing the densities of predator and prey.
Citation: Wei Ou, Changjin Xu, Qingyi Cui, Yicheng Pang, Zixin Liu, Jianwei Shen, Muhammad Zafarullah Baber, Muhammad Farman, Shabir Ahmad. Hopf bifurcation exploration and control technique in a predator-prey system incorporating delay[J]. AIMS Mathematics, 2024, 9(1): 1622-1651. doi: 10.3934/math.2024080
[1] | Yingyan Zhao, Changjin Xu, Yiya Xu, Jinting Lin, Yicheng Pang, Zixin Liu, Jianwei Shen . Mathematical exploration on control of bifurcation for a 3D predator-prey model with delay. AIMS Mathematics, 2024, 9(11): 29883-29915. doi: 10.3934/math.20241445 |
[2] | Hairong Li, Yanling Tian, Ting Huang, Pinghua Yang . Hopf bifurcation and hybrid control of a delayed diffusive semi-ratio-dependent predator-prey model. AIMS Mathematics, 2024, 9(10): 29608-29632. doi: 10.3934/math.20241434 |
[3] | Chenxuan Nie, Dan Jin, Ruizhi Yang . Hopf bifurcation analysis in a delayed diffusive predator-prey system with nonlocal competition and generalist predator. AIMS Mathematics, 2022, 7(7): 13344-13360. doi: 10.3934/math.2022737 |
[4] | Xin-You Meng, Fan-Li Meng . Bifurcation analysis of a special delayed predator-prey model with herd behavior and prey harvesting. AIMS Mathematics, 2021, 6(6): 5695-5719. doi: 10.3934/math.2021336 |
[5] | Jinting Lin, Changjin Xu, Yiya Xu, Yingyan Zhao, Yicheng Pang, Zixin Liu, Jianwei Shen . Bifurcation and controller design in a 3D delayed predator-prey model. AIMS Mathematics, 2024, 9(12): 33891-33929. doi: 10.3934/math.20241617 |
[6] | San-Xing Wu, Xin-You Meng . Dynamics of a delayed predator-prey system with fear effect, herd behavior and disease in the susceptible prey. AIMS Mathematics, 2021, 6(4): 3654-3685. doi: 10.3934/math.2021218 |
[7] | Ruizhi Yang, Dan Jin, Wenlong Wang . A diffusive predator-prey model with generalist predator and time delay. AIMS Mathematics, 2022, 7(3): 4574-4591. doi: 10.3934/math.2022255 |
[8] | Qinghui Liu, Xin Zhang . Chaos detection in predator-prey dynamics with delayed interactions and Ivlev-type functional response. AIMS Mathematics, 2024, 9(9): 24555-24575. doi: 10.3934/math.20241196 |
[9] | Sahabuddin Sarwardi, Hasanur Mollah, Aeshah A. Raezah, Fahad Al Basir . Direction and stability of Hopf bifurcation in an eco-epidemic model with disease in prey and predator gestation delay using Crowley-Martin functional response. AIMS Mathematics, 2024, 9(10): 27930-27954. doi: 10.3934/math.20241356 |
[10] | Fatao Wang, Ruizhi Yang, Yining Xie, Jing Zhao . Hopf bifurcation in a delayed reaction diffusion predator-prey model with weak Allee effect on prey and fear effect on predator. AIMS Mathematics, 2023, 8(8): 17719-17743. doi: 10.3934/math.2023905 |
Recently, delayed dynamical model has witnessed a great interest from many scholars in biological and mathematical areas due to its potential application in describing the interaction of different biological populations. In this article, relying the previous studies, we set up two new predator-prey systems incorporating delay. By virtue of fixed point theory, inequality tactics and an appropriate function, we explore well-posedness (includes existence and uniqueness, boundedness and non-negativeness) of the solution of the two formulated delayed predator-prey systems. With the aid of bifurcation theorem and stability theory of delayed differential equations, we gain the parameter conditions on the emergence of stability and bifurcation phenomenon of the two formulated delayed predator-prey systems. By applying two controllers (hybrid controller and extended delayed feedback controller) we can efficaciously regulate the region of stability and the time of occurrence of bifurcation phenomenon for the two delayed predator-prey systems. The effect of delay on stabilizing the system and adjusting bifurcation is investigated. Computer simulation plots are provided to sustain the acquired prime outcomes. The conclusions of this article are completely new and can provide some momentous instructions in dominating and balancing the densities of predator and prey.
As is known to us, predator-prey models play a vital role in describing the interaction between predator population and prey population in real natural world. In order to expose the internal change process and development law of predator population and prey population, a great deal of predator-prey models have been established. Through the discussion on predator-prey models, we can find the impact of parameters on the biological population densities in some specific environment. During the past decades, a lot of work on predator-prey models has been carried out and abundant fruits have been resulted. For example, Balc [1] explored the stability, well-posedness, and bifurcation issue of a fractional prey-predator model. Pandey et al. [2] explored the rich dynamics (e.g., transcritical, saddle-node, Hopf-bifurcation, etc.) of a delayed predator-prey model. Rao and Kang [3] established the conditions for the existence of a unique ergodic stationary distribution and the extinction conditions of predator species and prey species for a stochastic predator-prey model. Sarkar and Khajanchi [4] dealt with the spatiotemporal dynamical trait of a prey-predator model involving the fear effect. For more concrete examples, one can see [5,6,7,8].
In 2020, Sen et al. [9] formulated the following predator-prey system:
{du1(t)dt=u1(t)(h1−a1u1(t))−d1u1(t)u2(t)1+bu1(t),du2(t)dt=u2(t)[h2−a2u2(t)]+dd1u1(t)u2(t)1+bu1(t), | (1.1) |
where u1(t) stands for the density of prey at time t and u2(t) stands for the density of predator at time t, h1 is the intrinsic growth rate of prey and h2 is the intrinsic growth rate of predator, a1 denotes the intra-species competition of prey and a2 denotes the intra-species competition of predator, b denotes the handling parameter, which is the product of the handling time and the searching efficiency, d>0 is the conversion efficiency and d1 represents the searching efficiency by an individual predator per unit time. All other parameters are positive real numbers. In details, one can see [9,10,11,12].
In many cases, the densities of prey and predator are affected due to the time delay of population development, then it is necessary to introduce the delay into the predator-prey models. Based on this viewpoint, we can establish more suitable delayed predator-prey models. Assume that the density of prey is affected by the self feedback time from u1 to u1, then we can modify model (1.1) as follows:
{du1(t)dt=u1(t)(h1−a1u1(t))−d1u1(t)u2(t)1+bu1(t),du2(t)dt=u2(t)[h2−a2u2(t−δ)]+dd1u1(t)u2(t)1+bu1(t), | (1.2) |
where δ>0 is a time delay. All other parameters are positive real numbers. Assume that the density of prey is affected by the self feedback time from u1 to u1 and the density of predator is affected by the self feedback time from u2 to u2, then we can modify model (1.1) as follows:
{du1(t)dt=u1(t)(h1−a1u1(t−δ))−d1u1(t)u2(t)1+bu1(t),du2(t)dt=u2(t)[h2−a2u2(t−δ)]+dd1u1(t)u2(t)1+bu1(t), | (1.3) |
where δ>0 is a time delay. All other parameters are positive real numbers.
Many studies show that delay is often a vital factor that affects the dynamical behavior of the delayed dynamical model. In many instances, delay will make the system lose its stability, produce periodic vibration, generate chaotic behavior and so on [13,14,15,16,17,18,19,20,21,22]. In particular, delay-induced Hopf bifurcation is an important dynamical peculiarity. Biologically, delay-induced Hopf bifurcation plays a vital role in describing the balanced relationship among the concentrations of numerous biological populations. In the light of this viewpoint, we argue that exploring the delay-induced Hopf bifurcation in abundant predator-prey models has very important theoretical significance. Inspired by the above idea, we are going to investigate the delay-induced Hopf bifurcation and control of bifurcation for models (1.2) and (1.3). Specifically, we are to deal with the following three core points: (1) Study the well-posedness (e.g., non-negativeness, boundedness, existence and uniqueness) of solution to models (1.2) and (1.3). (2) Explore the emergence of Hopf bifurcation and stability of models (1.2) and (1.3). (3) Construct two different controllers to control the region of stability and the time of generation of bifurcation behavior of models (1.2) and (1.3).
The key highlights of this study are stated as follows: (Ⅰ) Depending on the previous studies, a new bifurcation and stability criterion without relying on time delay for model (1.2) is built. (Ⅱ) By virtue of two different controllers, the domain of stability and the time of generation of Hopf bifurcation of models (1.2) and (1.3) are effectively under control. (Ⅲ) The impact of time delay on dominating Hopf bifurcation phenomenon and stabilizing the densities of predators and preys of models (1.2) and (1.3) is presented.
This structure of this article is presented as follows: The well-posedness involving existence and uniqueness, non-negativeness and boundedness of the solution of system (1.2) is discussed in Section 2. Section 3 explores the bifurcation phenomenon and stability nature of system (1.2). Section 4 focuses on the control problem of bifurcation phenomenon for system (1.2) by virtue of a reasonable hybrid controller incorporating state feedback and parameter perturbation involving delay. Section 5 handles the control problem of bifurcation phenomenon and stability for system (1.3). Section 6 handles the control problem of bifurcation phenomenon for system (1.3) by virtue of a reasonable hybrid controller incorporating state feedback and parameter perturbation involving delay. Section 7 carries out numerical experiments to verify the rationality of the acquired key outcomes. A brief conclusion is included to finish this work in Section 8.
In this part, we are going to explore the well-posedness of solutions to model (1.2) and model (1.3) (include boundedness, existence and uniqueness, non-negativeness) via making use of fixed point theory, inequality technique and construction of a reasonable function.
Theorem 2.1. Denote Ψ={u1,u2∈R2:max{|u1|,|u2|}≤U}, where U>0 denotes a constant. For each (u10,u20)∈Ψ, system (1.2) under the initial state (u10,u20) owns a unique solution U=(u1,u2)∈Ψ.
Proof. Define the following mapping:
f(U)=(f1(U),f2(U)), | (2.1) |
where
{f1(U)=u1(t)(h1−a1u1(t))−d1u1(t)u2(t)1+bu1(t),f2(U)=u2(t)[h2−a2u2(t−δ)]+dd1u1(t)u2(t)1+bu1(t). | (2.2) |
For every U,ˉU∈Ψ, we can get
||f(U)−f(ˉU)||=|u1(h1−a1u1)−d1u1u21+bu1−[ˉu1(h1−a1ˉu1)−d1ˉu1ˉu21+bˉu1]|+|u2[h2−a2u2(t−δ)]+dd1u1u21+bu1−[ˉu2[h2−a2ˉu2(t−δ)]+dd1ˉu1ˉu21+bˉu1]|=|u1h1−a1u21−d1u1u21+bu1−ˉu1h1+a1ˉu21+d1ˉu1ˉu21+bˉu1|+|u2h2−a2u2(t)u2(t−δ)+dd1u1u21+bu1−ˉu2h2+a2ˉu2(t)ˉu2(t−δ)−dd1ˉu1ˉu21+bˉu1|=|h1(u1−ˉu1)−a1(u21−ˉu21)−d1(u1u21+bu1−ˉu1ˉu21+bˉu1)|+|h2(u2−ˉu2)−a2[u2(t)u2(t−δ)−ˉu2(t)ˉu2(t−δ)]+dd1(u1u21+bu1−ˉu1ˉu21+bˉu1)|≤h1|u1−ˉu1|+2Ua1|u1−ˉu1|+d1|u1u2(1+bˉu1)−ˉu1ˉu2(1+bu1)(1+bu1)(1+bˉu1)|+h2|u2−ˉu2|+2Ua2|u2−ˉu2|+dd1|u1u2(1+bˉu1)−ˉu1ˉu2(1+bu1)(1+bu1)(1+bˉu1)|≤(h1+2Ua1)|u1−ˉu1|+d1|u1u2(1+bˉu1)−ˉu1ˉu2(1+bu1)|+(h2+2Ua2)|u2−ˉu2|+dd1|u1u2(1+bˉu1)−ˉu1ˉu2(1+bu1)|=(h1+2Ua1)|u1−ˉu1|+(h2+2Ua2)|u2−ˉu2|+d1(1+d)|u1u2(1+bˉu1)−ˉu1u2+ˉu1u2−ˉu1ˉu2+bu1ˉu1(u2−ˉu2)|≤(h1+2Ua1)|u1−ˉu1|+(h2+2Ua2)|u2−ˉu2|+d1(1+d)|U(u1−ˉu1)|+d1(1+d)|U(u2−ˉu2)|+d1(1+d)|bU2(u2−ˉu2)|=[h1+2Ua1+d1(1+d)U]|u1−ˉu1|+[h2+2Ua2+d1(1+d)(U+bU2)]|u2−ˉu2|≤ρ||U−ˉU||, | (2.3) |
where
ρ=max{h1+2Ua1+d1(1+d)U,h2+2Ua2+d1(1+d)(U+bU2)}. | (2.4) |
Thus f(U) obeys the Lipschitz condition for U. Using fixed point theorem, one can conclude that Theorem 2.1 is right.
Theorem 2.2. Every solution of system (1.2) starting with R2+ is non-negative.
Proof. In view of the first equation of system (1.2), we can get
du1dt=u1(h1−a1u1)−d1u1u21+bu1, | (2.5) |
then
du1u1=(h1−a1u1−d1u21+bu1)dt, | (2.6) |
which leads to
∫t0du1u1=∫t0[h1−a1u1(s)−d1u2(s)1+bu1(s)]ds, | (2.7) |
and then one gets
u1(t)u1(0)=exp{∫t0[h1−a1u1(s)−d1u2(s)1+bu1(s)]ds}. | (2.8) |
Thus,
u1(t)=u1(0)exp{∫t0[h1−a1u1(s)−d1u2(s)1+bu1(s)]ds}>0. | (2.9) |
In a same way, we know
u2(t)=u2(0)exp{∫t0[h2−a2u2(s−δ)+dd1u1(s)1+bu1(s)]ds}>0. | (2.10) |
Thus, Theorem 2.2 is correct.
Theorem 2.3. The solutions of system (1.2) are uniformly bounded.
Proof. We consider two cases: d>1 and 0<d<1.
Case 1. If d>1, let W(t)=u1(t)+u2(t). Then
dWdt=du1dt+du2dt=u1(h1−a1u1)−d1u1u21+bu1+u2[h2−a2u2(t−δ)]+dd1u1u21+bu1=u1(h1−a1u1)+u2[h2−a2u2(t−δ)]−d1u1u21+bu1(1−d)≤u1(h1−a1u1)+u2(h2−a2u2)−d1u1u2bu1(1−d)=u1h1−a1u21+u2h2−a2u22−d1u2b(1−d)=−h1(u1+u2)+2u1h1−a1u21+u2[h1+h2−d1b(1−d)]−a2u22≤−h1(u1+u2)+h21a1+[h1+h2−d1b(1−d)]24a2, | (2.11) |
where
h21a1=maxt∈R+{2u1h1−a1u21},[h1+h2−d1b(1−d)]24a2=maxt∈R+{u2[h1+h2−d1b(1−d)]−a2u22}. |
Let
L=h21a1+[h1+h2−d1b(1−d)]24a2. | (2.12) |
Then,
dWdt≤−h1W+L. | (2.13) |
According to the differential inequality theorem, we get
0≤W(t)≤Lh1(1−e−h1t)+W(0)e−h1t, | (2.14) |
then
0≤W(t)≤Lh1,t→∞. | (2.15) |
Case 2. If 0<d<1, let W(t)=u1(t)+u2(t). Then,
dWdt=du1dt+du2dt=u1(h1−a1u1)−d1u1u21+bu1+u2[h2−a2u2(t−δ)]+dd1u1u21+bu1=u1(h1−a1u1)+u2[h2−a2u2(t−δ)]+d1u1u21+bu1(d−1)≤u1(h1−a1u1)+u2(h2−a2u2)=u1h1−a1u21+u2h2−a2u22=−h1(u1+u2)+2u1h1+u2(h1+h2)−a1u21−a2u22≤−h1(u1+u2)+h21a1+(h1+h2)24a2, | (2.16) |
where
h21a1=maxt∈R+{2u1h1−a1u21},(h1+h2)24a2=maxt∈R+{u2(h1+h2)−a2u22}. |
Let
L=h21a1+(h1+h2)24a2, | (2.17) |
so
dWdt≤−h1W+L. | (2.18) |
According to the differential inequality theorem, one gets
0≤W(t)≤Lh1(1−e−h1t)+W(0)e−h1t, | (2.19) |
which results in
0≤W(t)≤Lh1,t→∞. | (2.20) |
Based on these two cases, we can conclude that Theorem 2.3 is correct.
In this section, we are going to explore the bifurcation and stability issue of model (1.2). Firstly, we assume that E(u1⋆,u2⋆) is the equilibrium point of model (1.2), then u1⋆,u2⋆ obey the following condition:
{u1⋆(h1−a1u1⋆)−d1u1⋆u2⋆1+bu1⋆=0,u2⋆(h2−a2u2⋆)+dd1u1⋆u2⋆1+bu1⋆=0. | (3.1) |
Let
{ˉu1(t)=u1(t)−u1⋆,ˉu2(t)=u2(t)−u2⋆. | (3.2) |
Substitute system (3.2) into system (1.2), we gain the linear system of model (1.2) at E(u1⋆,u2⋆):
{dˉu1dt=b1ˉu1−b2ˉu2,dˉu2dt=b3ˉu1+b4ˉu2−b5ˉu2(t−δ), | (3.3) |
where
{b1=h1−2a1u1⋆−d1u2⋆1+bu1⋆+bd1u1⋆u2⋆(1+bu1⋆)2,b2=d1u1⋆1+bu1⋆,b3=d1u2⋆1+bu1⋆+bdd1u1⋆u2⋆(1+bu1⋆)2,b4=h2−a2u2⋆+dd1u1⋆1+bu1⋆,b5=a2u2⋆. | (3.4) |
The characteristic equation of system (3.3) owns the following expression:
det[λ−b1b2−b3λ−b4+b5e−λδ]=0, | (3.5) |
which leads to
λ2+(−b1−b4)λ+(b5λ−b1b5)e−λδ+b1b4+b2b3=0. | (3.6) |
If δ=0, then Eq (3.6) becomes
λ2+(b5−b1−b4)λ+b1b4+b2b3−b1b5=0. | (3.7) |
If
(A1){b5−b1−b4>0,b1b4+b2b3−b1b5>0, | (3.8) |
is fulfilled, then the two roots λ1,λ2 of Eq (3.7) have negative real parts. Thus the equilibrium point E(u1⋆,u2⋆) of system (1.2) with δ=0 is locally asymptotically stable.
Assume that λ=iε is the root of Eq (3.6), then Eq (3.6) becomes
−ε2+(−b1−b4)iε+(b5iε−b1b5)e−iεδ+b1b4+b2b3=0. | (3.9) |
It follows from (3.9) that
{b5εsinεδ−b1b5cosεδ=ε2−b1b4−b2b3,b5εcosεδ+b1b5sinεδ=(b1+b4)ε. | (3.10) |
Then
ε4+(b21+b24−b25−2b2b3)ε2+(b1b4+b2b3)2−(b1b5)2=0. | (3.11) |
Let
Π1(ε)=ε4+(b21+b24−b25−2b2b3)ε2+(b1b4+b2b3)2−(b1b5)2. | (3.12) |
Assume that
(A2)|b1b4+b2b3|<|b1b5|. |
By virtue of (A2), we know Π1(0)=(b1b4+b2b3)2−(b1b5)2<0, since limε→∞Π1(ε)>0, then we will know Eq (3.11) has at least one positive real root. Therefore Eq (3.6) has at least one pair of purely imaginary roots. Without loss of generality, we can assume that Eq (3.11) has four positive real roots (say εj,j=1,2,3,4). Relying on (3.10), we know
δ(n)j=1εj[arcsin(ε3j+(b21−b2b3)εjb5ε2j+b21b5)+2nπ], | (3.13) |
where j=1,2,3,4;n=0,1,2,⋯. Assume δ0=min{j=1,2,3,4;n=0,1,2,⋯}{δ(n)j} and suppose that when δ=δ0, Eq (3.6) has a pair of imaginary roots ±iε0.
Next we present the following assumption:
(A3)G1RG2R+G1IG2I>0, |
where
{G1R=b5cosε0δ0−b1−b4,G1I=2ε0−b5sinε0δ0,G2R=−ε20b5cosε0δ0−b1b5ε0sinε0δ0,G2I=ε20b5cosε0δ0−b1b5ε0cosε0δ0. | (3.14) |
Lemma 3.1. Suppose that λ(θ)=ϵ1(δ)+iϵ2(δ) is the root of Eq (3.6) at δ=δ0 such that ϵ1(δ0)=0, ϵ2(δ0)=ε0, then Re(dλdδ)|δ=δ0,ε=ε0>0.
Proof. By Eq (3.6), we can get
(2λ−b1−b4)dλdδ+b5e−λδdλdδ−(δdλdδ+λ)(b5λ−b1b5)e−λδ=0. | (3.15) |
It means that
(dλdδ)−1=G1(λ)G2(λ)−δλ, | (3.16) |
where
{G1(λ)=2λ−b1−b4+b5eλδ,G2(λ)=λ(b5λ−b1b5)eλδ. | (3.17) |
Hence
Re[(dλdδ)−1]δ=δ0,ε=ε0=Re[G1(λ)G2(λ)]δ=δ0,ε=ε0=G1RG2R+G1IG2IG22R+G22I. | (3.18) |
By the assumption (A3), we get
Re[(dλdδ)−1]δ=δ0,ε=ε0>0, | (3.19) |
which ends the proof. According to the above discussion, the following outcome is easily derived.
Theorem 3.1. Suppose that (A1)–(A3) hold, then the equilibrium point E(u1⋆,u2⋆) of model (1.2) holds a locally asymptotically stable state if δ∈[0,δ0) and model (1.2) generates a cluster of Hopf bifurcations around the equilibrium point E(u1⋆,u2⋆) when δ=δ0.
In this section, we are to study the Hopf bifurcation issue of system (1.2) by using a reasonable hybrid controller consisting of parameter perturbation with delay and state feedback. By virtue of the idea in [19,20], we formulate the following controlled predator-prey model:
{du1dt=α1u1(h1−a1u1)−d1u1u21+bu1+k[u1(t−δ)−u1(t)],du2dt=u2[h2−a2u2(t−δ)]+dd1u1u21+bu1. | (4.1) |
System (4.1) owns the same equilibrium point E(u1⋆,u2⋆) as that in system (1.2). Let
{u1⋆=u1(t)−ˉu1(t),u2⋆=u2(t)−ˉu2(t). | (4.2) |
The linear system of system (4.1) near E(u1⋆,u2⋆) can be expressed as follows:
{dˉu1dt=c1ˉu1−c2ˉu2+kˉu1(t−δ),dˉu2dt=c3ˉu1+c4ˉu2−c5ˉu2(t−δ), | (4.3) |
where
{c1=α1h1−k−2a1u1∗α1−d1u2⋆1+bu1⋆+bd1u1⋆u2⋆(1+bu1⋆)2,c2=d1u1⋆1+bu1⋆,c3=d1u2⋆1+bu1⋆+bdd1u1⋆u2⋆(1+bu1⋆)2,c4=h2−a2u2⋆+dd1u1⋆1+bu1⋆,c5=a2u2⋆. | (4.4) |
The characteristic equation of system (4.3) owns the following expression:
det[λ−c1−ke−λδc2−c3λ−c4+c5e−λδ]=0, | (4.5) |
which leads to
(c5−k)λ+kc4−c1c5+[λ2+(−c1−c4)λ+c2c3+c1c4]eλδ−kc5e−λδ=0. | (4.6) |
If δ=0, then Eq (4.6) reads as:
λ2+(c5−k−c1−c4)λ+kc4+c2c3−c1c5+c1c4−kc5=0. | (4.7) |
If
(A4){c5−k−c1−c4>0,kc4+c2c3−c1c5+c1c4−kc5>0, | (4.8) |
is fulfilled, there are two roots λ1 and λ2 of Eq (4.6) that have negative real parts. Thus the equilibrium point E(u1⋆,u2⋆) of system (4.1) with δ=0 holds a locally asymptotically stable state. Suppose that λ=iε∗ is the root of Eq (4.6), then Eq (4.6) becomes:
(c5−k)iε∗+kc4−c1c5+[−ε∗2+(−c1−c4)iε∗+c2c3+c1c4]eiε∗δ−kc5e−iε∗δ=0. | (4.9) |
It follows from (4.9) that
{(−ε∗2+c2c3+c1c4−kc5)cosε∗δ−ε∗(−c1−c4)sinε∗δ=c1c5−kc4,ε∗(−c1−c4)cosε∗δ+(−ε∗2+c2c3+c1c4+kc5)sinε∗δ=(k−c5)ε∗. | (4.10) |
By (4.10), we can get
{E1cos(ε∗δ)−E2sin(ε∗δ)=E3,E2cos(ε∗δ)+E4sin(ε∗δ)=E5, | (4.11) |
where
{E1=−ε∗2+c2c3+c1c4−kc5,E2=ε∗(−c1−c4),E3=c1c5−kc4,E4=−ε∗2+c2c3+c1c4+kc5,E5=(k−c5)ε∗. | (4.12) |
So there is
{cosε∗δ=E1E2E5+E1E3E4E1(E22+E1E4),sinε∗δ=E21E5−E1E2E3E1(E22+E1E4). | (4.13) |
Because of cos2ε∗δ+sin2ε∗δ=1, we can get
[E1E2E5+E1E3E4E1(E22+E1E4)]2+[E21E5−E1E2E3E1(E22+E1E4)]2=1. | (4.14) |
So
E21E22E25+2E21E2E3E4E5+E21E23E24+E21E22E23−2E31E2E3E5+E41E25−E21E42−2E31E22E4−E41E24=0. | (4.15) |
According to Eq (4.12), one gets
{E1=−ε∗2+g1,E2=g2ε∗,E3=c1c5−kc4,E4=−ε∗2+g3,E5=g4ε∗, | (4.16) |
where
{g1=c2c3+c1c4−kc5,g2=−c1−c4,g3=c2c3+c1c4+kc5,g4=k−c5. | (4.17) |
Using (4.15) and (4.16), we know
−ε∗12+D1ε∗10+D2ε∗8+D3ε∗6+D4ε∗4+D5ε∗2+D6=0, | (4.18) |
therefore, the results can be obtained as follows:
ε∗12−D1ε∗10−D2ε∗8−D3ε∗6−D4ε∗4−D5ε∗2−D6=0, | (4.19) |
where
{D1=g21−2g22+2g3+4g1,D2=E23−2g2E3g4+g22g24−4g1g24+2g2E3g4−g42+6g1g22+2g22E3−6g21−8g1g3−g23,D3=−2g1E23−2g3E23+4g1g2E3g4+2g2g3E3g4−2g1g22g24+6g21g24−6g1g2E3g4+g22E23+2g1g42−6g21g22−6g1g22g3+4g31+12g21g3+4g1g23,D4=g21E23+4g1g3E23+g23E23−2g21g2E3g4−4g1g2g3E3g4+g21g22g24−4g31g24+6g21g2E3g4−2g1g22E23−g21g42+2g31g22+6g21g22g3−g41−8g31g3−6g21g23,D5=−2g21g3E23−2g1g23E23+2g21g2g3E3g4+g41g24−2g31g2E3g4+g21g22E23−2g31g22g3+2g41g3+4g31g23,D6=g21g23E23−g41g23. | (4.20) |
Let
Π2(ε∗)=ε∗12−D1ε∗10−D2ε∗8−D3ε∗6−D4ε∗4−D5ε∗2−D6. | (4.21) |
We can make the following assumption:
(A5)|g1g3E3|>|g21g3|. |
If (A5) holds, then Π2(0)=−D6<0. Since limε∗→∞Π2(ε∗)=+∞>0, then Eq (4.19) has at least one pair of positive real roots, and Eq (4.6) has at least one pair of pure roots. So we can assume that Eq (4.19) has 12 positive solid roots (say ε∗j,j=1,2,3,...,12). It is available according to Eq (4.11),
δ(k)j=1ε∗j[arccos(E1(ε∗j)E2(ε∗j)E5(ε∗j)+E1(ε∗j)E3E4(ε∗j)E1(ε∗j)(E22(ε∗j)+E1(ε∗j)E4(ε∗j))+2kπ)], | (4.22) |
where j=1,2,3,⋯,12; k=0,1,2,⋯. Let δ∗=min{j=1,2,3,⋯,12;k=0,1,2,⋯}{δ(k)j}, and assume that when δ=δ∗, Eq (4.6) has at least one pair of pure real roots ±iε∗0.
Next the following assumption is given:
(A6)H1RH2R+H1IH2I>0, |
where
{H1R=c5−k−2ε∗0sinε∗0δ∗−(c1+c4)cosε∗0δ∗,H1I=2ε∗0cosε∗0δ∗−(c1+c4)sinε∗0δ∗,H2R=[ε∗30+(c2c3+c1c4)ε∗0−kc5ε∗0]sinε∗0δ∗−(c1+c4)ε∗20cosε∗0δ∗,H2I=[−ε∗30−(c2c3+c1c4)ε∗0−kc5ε∗0]cosε∗0δ∗−(c1+c4)ε∗20sinε∗0δ∗. | (4.23) |
Lemma 4.1. Suppose that λ(θ)=ˉϵ1(δ)+iˉϵ2(δ) is the root of Eq (4.6) at δ=δ∗ such that ˉϵ1(δ∗)=0, ˉϵ2(δ∗)=ε∗0, then Re(dλdδ)|δ=δ∗,ε=ε∗0>0.
Proof. By Eq (4.6), one gets
(c5−k)dλdδ+(2λ−c1−c4)eλδdλdδ+[λ2+(−c1−c4)λ+c2c3+c1c4]×eλδ(λ+δdλdδ)+kc5e−λδ(λ+δdλdδ)=0, | (4.24) |
which implies
(dλdδ)−1=H1(λ)H2(λ)−δλ, | (4.25) |
where
{H1(λ)=c5−k+(2λ−c1−c4)eλδ,H2(λ)=[−λ2+(−c1−c4)λ+c2c3+c1c4]λeλδ−kc5λe−λδ. | (4.26) |
Hence
Re[(dλdδ)−1]δ=δ∗,ε=ε∗0=Re[H1(λ)H2(λ)]δ=δ∗,ε=ε∗0=H1RH2R+H1IH2IH22R+H22I. | (4.27) |
According to (A6), one gets
Re[(dλdδ)−1]δ=δ∗,ε=ε∗0>0, | (4.28) |
which completes the proof.
Depending on the analysis above, the following conclusion is acquired:
Theorem 4.1. Suppose that (A4)–(A6) hold, then the equilibrium point E(u1⋆,u2⋆) of model (4.1) is locally asymptotically stable if δ∈[0,δ∗) and model (4.1) generates a cluster of Hopf bifurcations near the equilibrium point E(u1⋆,u2⋆) when δ=δ∗.
In this section, we are going to explore the Hopf bifurcation phenomenon of system (1.3). System (1.3) owns the same equilibrium point E(u1⋆,u2⋆) as that in system (1.2). Let
{u1⋆=u1(t)−ˉu1(t),u2⋆=u2(t)−ˉu2(t). | (5.1) |
The linear system of system (1.3) near E(u1⋆,u2⋆) can be expressed as follows:
{du1dt=e1ˉu1−e2ˉu2−e3ˉu1(t−δ),du2dt=e4ˉu1+e5ˉu2−e6ˉu2(t−δ), | (5.2) |
where
{e1=h1−a1u1⋆−d1u2⋆1+bu1⋆+bd1u1⋆u2⋆(1+bu1⋆)2,e2=d1u1⋆1+bu1⋆,e3=a1u1⋆,e4=dd1u2⋆1+bu1⋆−bdd1u1⋆u2⋆(1+bu1⋆)2,e5=h2−a2u2⋆+dd1u1⋆1+bu1⋆,e6=a2u2⋆. | (5.3) |
The characteristic equation of system (5.2) owns the following expression:
det[λ−e1+e3e−λδe2−e4λ−e5+e6e−λδ]=0, | (5.4) |
which leads to:
λ2+(−e1−e5)λ+(e3λ+e6λ+e1e6−e3e5)e−λδ+e3e6e−2λδ+e1e5+e2e4=0, | (5.5) |
that is,
(e3+e6)λ+e1e6−e3e5+[λ2+(−e1−e5)λ+e1e5+e2e4]eλδ+e3e6e−λδ=0. | (5.6) |
If δ=0, then Eq (5.6) reads as:
λ2+(e3+e6−e1−e5)λ+e1e6−e3e5+e1e5+e2e4+e3e6=0. | (5.7) |
If
(A7){e3+e6−e1−e5>0,e1e6−e3e5+e1e5+e2e4+e3e6>0, | (5.8) |
is fulfilled, there are two roots λ1,λ2 of Eq (5.7) that have negative real parts. Thus the equilibrium point E(u1⋆,u2⋆) of system (1.3) with δ=0 is locally asymptotically stable.
Suppose that λ=iετ is the root of Eq (5.6), then Eq (5.6) becomes:
(e3+e6)iετ+e1e6−e3e5+[−ετ2+(−e1−e5)iετ+e1e5+e2e4]eiετδ+e3e6e−iετδ=0. | (5.9) |
By (5.9), we have
{(−ετ2+e1e5+e2e4+e3e6)cosετδ+ετ(e1+e5)sinετδ=e3e5−e1e6,−ετ(e1+e5)cosετδ+(−ετ2+e1e5+e2e4−e3e6)sinετδ=−(e3+e6)ετ, | (5.10) |
which means
{Y1cosετδ+Y2sinετδ=Y3,−Y2cosετδ+Y4sinετδ=Y5, | (5.11) |
where
{Y1=−ετ2+e1e5+e2e4+e3e6,Y2=ετ(e1+e5),Y3=e3e5−e1e6,Y4=−ετ2+e1e5+e2e4−e3e6,Y5=−(e3+e6)ετ. | (5.12) |
So, we can get
{cosετδ=Y1Y3Y4−Y1Y2Y5Y1(Y22+Y1Y4),sinετδ=Y21Y5+Y1Y2Y3Y1(Y22+Y1Y4). | (5.13) |
Because of cos2ετδ+sin2ετδ=1, then
[Y1Y3Y4−Y1Y2Y5Y1(Y22+Y1Y4)]2+[Y21Y5+Y1Y2Y3Y1(Y22+Y1Y4)]2=1. | (5.14) |
It follows from (5.14) that
Y21Y22Y25−2Y21Y2Y3Y4Y5+Y21Y23Y24+Y21Y22Y23+2Y31Y2Y3Y5+Y41Y25−Y21Y42−2Y31Y22Y4−Y41Y24=0. | (5.15) |
By (5.12), one gets
{Y1=−ετ2+y1,Y2=y2ετ,Y4=−ετ2+y3,Y5=y4ετ, | (5.16) |
where
{y1=e1e5+e2e4+e3e6,y2=e1+e5,y3=e1e5+e2e4−e3e6,y4=−(e3+e6). | (5.17) |
Using (5.15) and (5.16), we know
−ετ12+N1ετ10+N2ετ8+N3ετ6+N4ετ4+N5ετ2+N6=0. | (5.18) |
Therefore, the results can be obtained as follows
ετ12−N1ετ10−N2ετ8−N3ετ6−N4ετ4−N5ετ2−N6=0, | (5.19) |
where
{N1=2y3+4y1−2y22+4y24,N2=Y23+2y2Y3y4+y22y24−4y1y24−2y2Y3y4−y42+6y1y22+2y22Y3−6y21−8y1y3−y23,N3=−2y1Y23−2y3Y23−4y1y2Y3y4−2y2y3Y3y4+2y1y22y24+6y21y24+6y1y2Y3y4+y22Y23+2y1y42−6y21y22−6y1y22y3+4y31+12y21y3+4y1y23,N4=y21Y23+4y1y3Y23+y23Y23+2y21y2Y3y4+4y1y2y3Y3y4+y21y22y24−4y31y24−6y21y2Y3y4−2y1y22Y23−y21y42+2y31y22+6y21y22y3−y41−8y31y3−6y21y23,N5=−2y21y3Y23−2y1y23Y23−2y21y2y3Y3y4+y41y24+2y31y2Y3y4+y21y22Y23−2y31y22y3+2y41y3+4y31y23,N6=y21y23Y23−y41y23. | (5.20) |
Let
Π3(ετ)=ετ12−N1ετ10−N2ετ8−N3ετ6−N4ετ4−N5ετ2−N6. | (5.21) |
We can make the following assumptions:
(A8)|y1y3Y3|>|y21y3|. |
If (A8) holds, then Π3(0)=−N6<0, since limετ→∞Π3(ετ)=+∞>0, then Eq (5.19) has at least one pair of positive real roots, and Eq (5.6) has at least one pair of pure roots. So we can assume that Eq (5.19) has 12 positive real roots (say ετj,j=1,2,3,...,12).
By Eq (5.11), one gets
δ(m)j=1ετj[arccos(Y1(εj)Y3Y4(ετj)−Y1(ετj)Y2(ετj)Y5(ετj)Y1(ετj)(Y22(ετj)+Y1(ετj)Y4(ετj))+2mπ)], | (5.22) |
where j=1,2,3,⋯,12;m=0,1,2,⋯.
Let δ⋆=min{j=1,2,3,⋯,12;m=0,1,2,⋯.}{δ(m)j}, and assume that when δ=δ⋆, Eq (5.6) has at least one pair of pure of real roots ±iετ0.
Next the following assumption is needed:
(A9)F1RF2R+F1IF2I>0, |
where
{F1R=e3+e6−2ετ0sinετ0δ⋆−(e1+e5)cosετ0δ⋆,F1I=2ετ0cosετ0δ⋆−(e1+e5)sinετ0δ⋆,F2R=[ετ30+(e1e5+e2e4+e3e6)ετ0]sinετ0δ⋆−(e1+e5)ετ0cosετ0δ⋆,F2I=[−ετ30+(e3e6−e1e5−e2e4)ετ0]cosετ0δ⋆−(e1+e5)ετ0sinετ0δ⋆. | (5.23) |
Lemma 5.1. Suppose that λ(θ)=ξ1(θ)+iξ2(θ) is the root of Eq (5.6) at δ=δ⋆, such that ξ1(δ⋆)=0, ξ2(δ⋆)=ετ0, then Re(dλdδ)|δ=δ⋆,ε=ετ0>0.
Proof. By Eq (5.6), one gets
(e3+e6)dλdδ+(2λ−e1−e5)eλδdλdδ+[λ2+(−e1−e5)λ+e2e4+e1e5]×eλδ(λ+δdλdδ)−e3e6e−λδ(λ+δdλdδ)=0, | (5.24) |
which implies
(dλdδ)−1=F1(λ)F2(λ)−δλ, | (5.25) |
where
{F1(λ)=e3+e6+(2λ−e1−e5)eλδ,F2(λ)=−[λ2+(−e1−e5)λ+e2e4+e1e5]λeλδ+e3e6λe−λδ. | (5.26) |
Hence
Re[(dλdδ)−1]δ=δ⋆,ε=ετ0=Re[F1(λ)F2(λ)]δ=δ⋆,ε=ετ0=F1RF2R+F1IF2IF22R+F22I. | (5.27) |
By (A9), we have
Re[(dλdδ)−1]δ=δ⋆,ε=ετ0>0, | (5.28) |
which ends the proof.
Depending on the discussion above, the following results is obtained:
Theorem 5.1. Suppose that (A7)–(A9) are fulfilled, then the equilibrium point E(u1⋆,u2⋆) of model (5.1) is locally asymptotically stable if δ∈[0,δ⋆) and model (5.1) generates a cluster of Hopf bifurcations near the equilibrium point E(u1⋆,u2⋆) when δ=δ⋆.
Remark 5.1. In model (1.3), there is only one delay. If there are two different delays in model (1.3), we can deal with the effect of two delays on the stability and bifurcation. We leave it for future work.
In this section, we are to study the Hopf bifurcation issue of system (1.3) by using a reasonable extended delayed feedback controller consisting of parameter perturbation with delay. By virtue of the idea in [23,24,25], we formulate the following controlled predator-prey model:
{du1dt=u1[h1−a1u1(t−δ)]−d1u1u21+bu1+k1[u1(t−δ)−u1(t)],du2dt=u2[h2−a2u2(t−δ)]+dd1u1u21+bu1+k2[u2(t−δ)−u2(t)]. | (6.1) |
System (6.1) owns the same equilibrium point E(u1⋆,u2⋆) as that of system (1.3). Let
{u1⋆=u1(t)−ˉu1(t),u2⋆=u2(t)−ˉu2(t). | (6.2) |
The linear system of system (6.1) around E(u1⋆,u2⋆) takes the following expression:
{dˉu1dt=τ1ˉu1−τ2ˉu2+τ3ˉu1(t−δ),dˉu2dt=τ4ˉu1+τ5ˉu2+τ6ˉu2(t−δ), | (6.3) |
where
{τ1=h1−k1−a1u1⋆−d1u2⋆1+bu1⋆+bd1u1⋆u2⋆(1+bu1⋆)2,τ2=d1u1⋆1+bu1⋆,τ3=k1−a1u1⋆,τ4=dd1u2⋆1+bu1⋆−bdd1u1⋆u2⋆(1+bu1⋆)2,τ5=h2−a2u2⋆+dd1u1⋆1+bu1⋆−k2,τ6=k2−a2u2⋆. | (6.4) |
The characteristic equation of system (6.3) owns the following expression:
det[λ−τ1+τ3e−λδτ2−τ4λ−τ5−τ6e−λδ]=0, | (6.5) |
which leads to:
λ2+(−τ1−τ5)λ+(−τ3λ−τ6λ+τ1τ6+τ3τ5)e−λδ+τ3τ6e−2λδ+τ1τ5+τ2τ4=0, | (6.6) |
that is
(τ3+τ6)λ−(τ1τ6+τ3τ5)+[λ2+(−τ1−τ5)λ+τ1τ5+τ2τ4]eλδ−τ3τ6e−λδ=0. | (6.7) |
If δ=0, then Eq (6.7) reads as:
λ2−(τ1+τ3+τ5+τ6)λ+τ1τ6+τ3τ5+τ1τ5+τ2τ4+τ3τ6=0. | (6.8) |
If
(A10){−(τ1+τ3+τ5+τ6)>0,τ1τ6+τ3τ5+τ1τ5+τ2τ4+τ3τ6>0, | (6.9) |
is fulfilled, then the two roots λ1,λ2 of Eq (6.7) have negative real parts. Thus the equilibrium point E(u1⋆,u2⋆) of system (6.1) with δ=0 is locally asymptotically stable.
Suppose that λ=iεμ is the root of Eq (6.7), then Eq (6.7) becomes:
(τ3+τ6)iεμ−(τ1τ6+τ3τ5)−[εμ2+(−τ1−τ5)iεμ+τ1τ5+τ2τ4]eiεμδ−τ3τ6e−iεμδ=0. | (6.10) |
By (6.10), we have
{(εμ2−τ1τ5−τ2τ4−τ3τ6)cosεμδ−εμ(τ1+τ5)sinεμδ=τ1τ6+τ3τ5,εμ(τ1+τ5)cosεμδ+(εμ2−τ1τ5−τ2τ4+τ3τ6)sinεμδ=−(τ3+τ6)εβ, | (6.11) |
which means
{T1cosεμδ−T2sinεμδ=T3,T2cosεμδ+T4sinεμδ=T5, | (6.12) |
where
{T1=−εμ2−τ1τ5−τ2τ4−τ3τ6,T2=(τ1+τ5)εμ,T3=τ3τ5+τ1τ6,T4=−εμ2−τ1τ5−τ2τ4+τ3τ6,T5=−(τ3+τ6)εμ. | (6.13) |
So, we can get
{cosεμδ=T1T3T4+T1T2T5T1(T22+T1T4),sinεμδ=T21T5−T1T2T3T1(T22+T1T4). | (6.14) |
Because of cos2εμδ+sin2εμδ=1,
[T1T3T4+T1T2T5T1(T22+T1T4)]2+[T21T5−T1T2T3T1(T22+T1T4)]2=1. | (6.15) |
It follows from (6.15) that
T21T22T25+2T21T2T3T4T5+T21T23T24+T21T22T23−2T31T2T3T5+T41T25−T21T42−2T31T22T4−T41T24=0. | (6.16) |
By (6.13), one gets
{T1=−εμ2+x1,T2=x2εμ,T4=−εμ2+x3,T5=x4εμ, | (6.17) |
where
{x1=−τ1τ5−τ2τ4−τ3τ6,x2=τ1+τ5,x3=−τ1τ5−τ2τ4+τ3τ6,x4=−(τ3+τ6). | (6.18) |
Using (6.16) and (6.17), we know
−εμ12+X1εμ10+X2εμ8+X3εμ6+X4εμ4+X5εμ2+X6=0, | (6.19) |
therefore, the results can be obtained as follows:
εμ12−X1εμ10−X2εμ8−X3εμ6−X4εμ4−X5εμ2−X6=0, | (6.20) |
where
{X1=x24−2x22−2x3+4x1,X2=T23+2x2T3x4+x22x24+4x1x24−2x2T3x4−x42−6x1x22−2x22T3−6x21−8x1x3−x23,X3=2x1T23+2x3T23+4x1x2T3x4+2x2x3T3x4+2x1x22x24+6x21x24−6x1x2T3x4+x22T23−2x1x42−6x21x22−6x1x22x3−4x31−12x21x3−4x1x23,X4=x21T23+4x1x3T23+x23T23+2x21x2T3x4+4x1x2x3T3x4+x21x22x24+4x31x24−6x21x2T3x4+2x1x22T23−x21x42−2x31x22−6x21x22x3−x41−8x31x3−6x21x23,X5=x21x3T23+2x1x23T23+2x21x2x3T3x4+x41x24−2x31x2T3x4+x21x22T23−2x31x22x3−2x41x3−4x31x23,X6=x21x23T23−x41x23. | (6.21) |
Let
Π4(εμ)=εμ12−X1εμ10−X2εμ8−X3εμ6−X4εμ4−X5εμ2−X6. | (6.22) |
We can make the following assumption:
(A11)|x1x3T3|>|x21x3|. |
If (A11) holds, then Π4(0)=−X6<0. Notice that limεμ→∞Π4(εμ)=+∞>0, then Eq (6.20) has at least one pair of positive real roots, and Eq (6.7) has at least one pair of purely real roots. So we can assume that Eq (6.20) has 12 positive real roots (say εμj,j=1,2,3,⋯,12).
By Eq (6.14), one gets
δ(θ)j=1εμj[arccos(X1(εμj)X3X4(εμj)−X1(εμj)X2(εμj)X5(εμj)X1(εμj)(X22(εμj)+X1(εμj)X4(εμj))+2θπ)], | (6.23) |
where j=1,2,3,⋯,12;θ=0,1,2,⋯.
Let δ0∗=min{j=1,2,3,⋯,12;θ=0,1,2,⋯}{δ(θ)j}, and assume that when δ=δ0∗, Eq (6.7) has at least one pair of pure real roots ±εμ0.
Next the following assumption is needed:
(A12)M1RM2R+M1IM2I>0, |
where
{M1R=τ3+τ6+2εμ0sinεμ0δ0∗+(τ1+τ5)cosεμ0δ0∗,M1I=−2εμ0cosεμ0δ0∗+(τ1+τ5)sinεμ0δ0∗,M2R=[εμ30−(τ1τ5+τ2τ4+τ3τ6)εμ0]sinεμ0δ0∗+(τ1+τ5)εμ0cosεμ0δ0∗,M2I=[−εμ30+(τ1τ5+τ2τ4−τ3τ6)εμ0]cosεμ0δ0∗+(τ1+τ5)εμ0sinεμ0δ0∗. | (6.24) |
Lemma 6.1. Suppose that λ(θ)=ˉξ1(θ)+iˉξ2(θ) is the root of Eq (6.7) at δ=δ0∗ such that ˉξ1(δ0∗)=0, ˉξ2(δ0∗)=εμ0, then Re(dλdδ)|δ=δ0∗,ε=εμ0>0.
Proof. By Eq (6.7), one gets
(τ3+τ6)dλdδ−(2λ−τ1−τ5)eλδdλdδ−[λ2+(−τ1−τ5)λ+τ2τ4+τ1τ5]×eλδ(λ+δdλdδ)−eτ3τ6e−λδ(λ+δdλdδ)=0, | (6.25) |
which implies
(dλdδ)−1=M1(λ)M2(λ)−δλ, | (6.26) |
where
{M1(λ)=τ3+τ6−(2λ−τ1−τ5)eλδ,M2(λ)=[λ2+(−τ1−τ5)λ+τ2τ4+τ1τ5]λeλδ−τ3τ6λe−λδ. | (6.27) |
Hence
Re[(dλdδ)−1]δ=δ0∗,ε=εμ0=Re[F1(λ)F2(λ)]δ=δ0∗,ε=εμ0=M1RM2R+M1IM2IM22R+M22I. | (6.28) |
By (A12), we have
Re[(dλdδ)−1]δ=δ0∗,ε=εμ0>0, | (6.29) |
which completes the proof.
Depending on the study above, the following conclusion is acquired:
Theorem 6.1. Suppose that (A10)–(A12) hold, then the equilibrium point E(u1⋆,u2⋆) of model (6.1) is locally asymptotically stable if δ∈[0,δ0∗) and model (6.1) generates a cluster of Hopf bifurcations at the equilibrium point E(u1⋆,u2⋆) when δ=δ0∗.
Remark 6.1. In this paper, some mathematical formulas and assumptions are very complicated (for example, (A6),(A12), etc.), but we can check their correctness using computerized calculations.
Remark 6.2. The control methods in this paper can be applied to control the bifurcation or chaos of fractional-order dynamical system.
In this section, to verify the obtained key outcomes of this paper, we give some computer simulations.
Example 7.1. Consider the following predator-prey system incorporating delay:
{du1(t)dt=u1(t)(h1−a1u1(t))−d1u1(t)u2(t)1+bu1(t),du2(t)dt=u2(t)[h2−a2u2(t−δ)]+dd1u1(t)u2(t)1+bu1(t), | (7.1) |
where h1=0.5,h2=0.5,a1=2,a2=2,d1=0.4,b=0.1,d=0.45. Clearly, model (7.1) admits a unique positive equilibrium point E(0.1975,0.2674). One can easily derive that the conditions (A1)–(A3) of Theorem 3.1 hold. Making use of computer software, one can obtain that δ0≈2.9. To verify the correctness of the gained outcomes of Theorem 3.1, we choose two nonidentical values of delay. One is δ=2.8 and the other is δ=2.97. If δ=2.8<δ0≈2.9, we gain computer simulation diagrams that are given in Figure 1. From Figure 1, we can easily understand that u1→0.1975,u2→0.2674 when t→+∞. Namely, unique positive equilibrium point E(0.1975,0.2674) of model (7.1) maintains locally asymptotically stable status. If δ=2.97>δ0≈2.9, we gain computer simulation diagrams that are given in Figure 2. From Figure 2, we are able to see that u1 is to keep a periodic quavering level around the value 0.1975, u2 is to keep a periodic quavering level around the value 0.2674. In other words, a cluster of periodic solutions (namely, Hopf bifurcations) arise near the positive equilibrium point E(0.1975,0.2674).
Example 7.2. Consider the following controlled predator-prey system incorporating delay:
{du1(t)dt=α1u1(t)(h1−a1u1(t))−d1u1(t)u2(t)1+bu1(t)+k[u1(t−δ)−u1(t)],du2(t)dt=u2(t)[h2−a2u2(t−δ)]+dd1u1(t)u2(t)1+bu1(t), | (7.2) |
where h1=0.5,h2=0.5,a1=2,a2=2,d1=0.4,b=0.1,d=0.45. Let α1=0.6,k=0.5. Clearly, model (7.2) admits a unique positive equilibrium point E(0.1975,0.2674). One can easily derive that the conditions (A5)–(A7) of Theorem 4.1 hold. Making use of computer software, one can obtain that δ⋆≈2.85. To verify the correctness of the gained outcomes of Theorem 4.1, we choose two nonidentical values of delay. One is δ=2.83 and the other is δ=3.0. If δ=2.83<δ∗≈2.85, we gain computer simulation diagrams that are given in Figure 3. From Figure 3, we can easily understand that u1→0.1975,u2→0.2674 when t→+∞. Namely, unique positive equilibrium point E(0.1975,0.2674) of model (7.2) maintains locally asymptotically stable status. If δ=3.0>δ∗≈2.85, we gain computer simulation diagrams that are given in Figure 4. From Figure 4, we are able to see that u1 is to keep a periodic quavering level around the value 0.1975, u2 is to keep a periodic quavering level around the value 0.2674. In other words, a cluster of periodic solutions (namely, Hopf bifurcations) arise near the positive equilibrium point E(0.1975,0.2674).
Example 7.3. Consider the following predator-prey system incorporating delay:
{du1(t)dt=u1(t)(h1−a1u1(t−θ))−d1u1(t)u2(t)1+bu1(t),du2(t)dt=u2(t)[h2−a2u2(t−θ)]+dd1u1(t)u2(t)1+bu1(t), | (7.3) |
where h1=0.5,h2=0.5,a1=2,a2=2,d1=0.4,b=0.1,d=0.45. Clearly, model (7.3) admits a unique positive equilibrium point E(0.1975,0.2674). One can easily derive that the conditions (A8)–(A10) of Theorem 5.1 hold. Making use of computer software, one can obtain that δ⋆≈2.8. To verify the correctness of the gained outcomes of Theorem 5.1, we choose two nonidentical values of delay. One is δ=2.7 and the other is δ=2.88. If δ=2.7<δ⋆≈2.8, we gain computer simulation diagrams that are given in Figure 5. From Figure 5, we can easily understand that u1→0.1975,u2→0.2674 when t→+∞. Namely, unique positive equilibrium point E(0.1975,0.2674) of model (7.3) maintains locally asymptotically stable status. If δ=2.88>δ⋆≈2.8, we gain computer simulation diagrams that are given in Figure 6. From Figure 6, we are able to see that u1 is to keep a periodic quavering level around the value 0.1975, u2 is to keep a periodic quavering level around the value 0.2674. In other words, a cluster of periodic solutions (namely, Hopf bifurcations) arise near the positive equilibrium point E(0.1975,0.2674).
Example 7.4. Consider the following controlled predator-prey system incorporating delay:
{du1(t)dt=u1(t)(h1−a1u1(t−δ))−d1u1(t)u2(t)1+bu1(t)+k1[u1(t−δ)−u1(t)],du2(t)dt=u2(t)[h2−a2u2(t−δ)]+dd1u1(t)u2(t)1+bu1(t)+k2[u2(t−δ)−u2(t)], | (7.4) |
where h1=0.5,h2=0.5,a1=2,a2=2,d1=0.4,b=0.1,d=0.45. Let k1=0.3,k2=0.1. Clearly, model (7.4) admits a unique positive equilibrium point E(0.1975,0.2674). One can easily derive that the conditions (A8)–(A10) of Theorem 6.1 hold. Making use of computer software, one can obtain that δ0∗≈4.1. To verify the correctness of the gained outcomes of Theorem 6.1, we choose two nonidentical values of delay. One is δ=3.8 and the other is δ=4.4. If δ=3.8<δ0∗≈4.1, we gain computer simulation diagrams that are given in Figure 7. From Figure 7, we can easily understand that u1→0.1975,u2→0.2674 when t→+∞. Namely, unique positive equilibrium point E(0.1975,0.2674) of model (7.4) maintains locally asymptotically stable status. If δ=4.4>δ0∗≈4.1, we gain computer simulation diagrams that are given in Figure 8. From Figure 8, we are able to see that u1 is to keep a periodic quavering level around the value 0.1975, u2 is to keep a periodic quavering level around the value 0.2674. In other words, a cluster of periodic solutions (namely, Hopf bifurcations) arise near the positive equilibrium point E(0.1975,0.2674).
Remark 7.1. Based on the computer simulation figures in Examples 7.1 and 7.2, one can easily know that the bifurcation values of model (7.1) and model (7.2) are δ0≈2.9 and δ∗≈2.85, which implies that we can reduce the domain of stability and shorten the time of emergence of bifurcation of model (7.1) via the designed hybrid controller. Based on the computer simulation figures in Examples 7.3 and 7.4, one can easily know that the bifurcation values of model (7.3) and model (7.3) are δ⋆≈2.8 and δ0∗≈4.1, which implies that we can enlarge the domain of stability and delay the time of emergence of bifurcation of model (7.3) via the designed extended delayed feedback controller.
Nowadays, the investigation of predator-prey models has attracted much interest from mathematical and biological circles. From a mathematical point of view, revealing the effect of time delay on the many dynamical peculiarities of predator-prey models is a very significant topic. In this article, two new delayed predator-prey models are formulated. The non-negativeness, existence and uniqueness, and boundedness of solution of the established delayed predator-prey models are detailedly analyzed. By regarding the delay as the parameter of bifurcation, we gain two delay-independent criteria to guarantee the emergence of bifurcation and stability of the established two delayed predator-prey models. Making use of two different controllers, we have availably adjusted the region of stability and the time of onset of the bifurcation phenomenon of the two delayed predator-prey models. The fruits of this article have immense theoretical significance in taking control of the balance of the concentrations of predator and prey. Furthermore, the exploration idea can be applied to explore the control problem of bifurcation in many other differential models. In the near future, we will adopt other controllers to deal with the bifurcation control of these two delayed predator-prey models. Recently, there have many studies on Hopf bifurcation of fractional-order dynamical models [26,27,28,29,30,31]. We will also focus on Hopf bifurcation of fractional-order predator-prey models in the near future.
The authors declare they have not used Artificial Intelligence (AI) tools in the creation of this article.
This work is supported by National Natural Science Foundation of China (No.12261015, No. 62062018), Project of High-level Innovative Talents of Guizhou Province ([2016]5651), University Science and Technology Top Talents Project of Guizhou Province (KY[2018]047), Foundation of Science and Technology of Guizhou Province ([2019]1051), Guizhou University of Finance and Economics (2018XZD01). The authors would like to thank the referees and the editor for helpful suggestions incorporated into this paper.
The authors declare that they have no conflict of interest.
[1] |
E. Balc, Predation fear and its carry-over effect in a fractional order prey-predator model with prey refuge, Chaos Soliton. Fract., 175 (2023), 114016. https://doi.org/10.1016/j.chaos.2023.114016 doi: 10.1016/j.chaos.2023.114016
![]() |
[2] |
S. Pandey, U. Ghosh, D. Das, S. Chakraborty, A. Sarkar, Rich dynamics of a delay-induced stage-structure prey-predator model with cooperative behaviour in both species and the impact of prey refuge, Math. Comput. Simulat., 216 (2024), 49–76. https://doi.org/10.1016/j.matcom.2023.09.002 doi: 10.1016/j.matcom.2023.09.002
![]() |
[3] |
F. Rao, Y. Kang, Dynamics of a stochastic prey-predator system with prey refuge, predation fear and its carry-over effects, Chaos Soliton. Fract., 175 (2023), 113935. https://doi.org/10.1016/j.chaos.2023.113935 doi: 10.1016/j.chaos.2023.113935
![]() |
[4] |
K. Sarkar, S. Khajanchi, Spatiotemporal dynamics of a predator-prey system with fear effect, J. Franklin Inst., 360 (2023), 7380–7414. https://doi.org/10.1016/j.jfranklin.2023.05.034 doi: 10.1016/j.jfranklin.2023.05.034
![]() |
[5] |
J. L. Xiao, Y. H. Xia, Spatiotemporal dynamics in a diffusive predator-prey model with multiple Allee effect and herd behavior, J. Math. Anal. Appl., 529 (2024), 127569. https://doi.org/10.1016/j.jmaa.2023.127569 doi: 10.1016/j.jmaa.2023.127569
![]() |
[6] |
P. Mishra, D. Wrzosek, Pursuit-evasion dynamics for Bazykin-type predator-prey model with indirect predator taxis, J. Diff. Equat., 361 (2023), 391–416. https://doi.org/10.1016/j.jde.2023.02.063 doi: 10.1016/j.jde.2023.02.063
![]() |
[7] |
W. Choi, K. Kim, I. Ahn, Predator-prey models with prey-dependent diffusion on predators in spatially heterogeneous habitat, J. Math. Anal. Appl., 525 (2023), 127130. https://doi.org/10.1016/j.jmaa.2023.127130 doi: 10.1016/j.jmaa.2023.127130
![]() |
[8] |
Q. Li, Y. Y Zhang, Y. N. Xiao, Canard phenomena for a slow-fast predator-prey system with group defense of the prey, J. Math. Anal. Appl., 527 (2023), 127418. https://doi.org/10.1016/j.jmaa.2023.127418 doi: 10.1016/j.jmaa.2023.127418
![]() |
[9] |
D. Sen, S. Petrovskii, S. Ghorai, M. Banerjee, Rich bifurcation structure of prey-predator model induced by the Allee effect in the growth of generalist predator, Int. J. Bifurcat. Chaos, 30 (2020), 2050084. https://doi.org/10.1142/S0218127420500844 doi: 10.1142/S0218127420500844
![]() |
[10] |
S. Dey, M. Banerjee, S. Ghorai, Analytical detection of stationary turing pattern in a predator-prey system with generalist predator, Math. Model. Nat. Phenom., 17 (2022), 33. https://doi.org/10.1051/mmnp/2022032 doi: 10.1051/mmnp/2022032
![]() |
[11] |
J. Roy, M. Banerjee, Global stability of a predator-prey model with generalist predator, Appl. Math. Lett., 142 (2023), 108659. https://doi.org/10.1016/j.aml.2023.108659 doi: 10.1016/j.aml.2023.108659
![]() |
[12] |
R. Xu. Global stability and Hopf bifurcation of a predator-prey model with stage structure and delayed predator response, Nonlinear Dynam., 67 (2012), 1683–1693. https://doi.org/10.1007/s11071-011-0096-1 doi: 10.1007/s11071-011-0096-1
![]() |
[13] |
C. J. Xu, D. Mu, Z. X. Liu, Y. C. Pang, C. Aouiti, O. Tunc, et al., Bifurcation dynamics and control mechanism of a fractional-order delayed Brusselator chemical reaction model, MATCH-Commun. Math. Co., 89 (2023), 73–106. https://doi.org/10.46793/match.89-1.073X doi: 10.46793/match.89-1.073X
![]() |
[14] |
C. J. Xu, C. Aouiti, Z. X. Liu, P. L. Li, L. Y. Yao, Bifurcation caused by delay in a fractional-order coupled Oregonator model in chemistry, MATCH-Commun. Math. Co., 88 (2022), 371–396. https://doi.org/10.46793/match.88-2.371X doi: 10.46793/match.88-2.371X
![]() |
[15] |
C. J. Xu, W. Zhang, C. Aouiti, Z. X. Liu, P. L. Li, L. Y. Yao, Bifurcation dynamics in a fractional-order Oregonator model including time delay, MATCH-Commun. Math. Co., 87 (2022), 397–414. https://doi.org/10.46793/match.87-2.397X doi: 10.46793/match.87-2.397X
![]() |
[16] |
Q. Y. Cui, C. J. Xu, W. Ou, Y. C. Pang, Z. X. Liu, P. L. Li, et al., Bifurcation behavior and hybrid controller design of a 2D Lotka-Volterra commensal symbiosis system accompanying delay, Mathematics, 11 (2023), 4808. https://doi.org/10.3390/math11234808 doi: 10.3390/math11234808
![]() |
[17] |
C. J. Xu, X. H. Cui, P. L. Li, J. L. Yan, L. Y. Yao, Exploration on dynamics in a discrete predator-prey competitive model involving feedback controls, J. Biol. Dynam., 17 (2023), 2220349. https://doi.org/10.1080/17513758.2023.2220349 doi: 10.1080/17513758.2023.2220349
![]() |
[18] |
D. Mu, C. J. Xu, Z. X. Liu, Y. C. Pang, Further insight into bifurcation and hybrid control tactics of a chlorine dioxide-iodine-malonic acid chemical reaction model incorporating delays, MATCH Commun. Math. Comput. Chem., 89 (2023), 529–566. https://doi.org/10.46793/match.89-3.529M doi: 10.46793/match.89-3.529M
![]() |
[19] |
P. L. Li, X. Q. Peng, C. J. Xu, L. Q. Han, S. R. Shi, Novel extended mixed controller design for bifurcation control of fractional-order Myc/E2F/miR-17-92 network model concerning delay, Math. Method. Appl. Sci., 46 (2023), 18878–18898. https://doi.org/10.1002/mma.9597 doi: 10.1002/mma.9597
![]() |
[20] |
P. L. Li, R. Gao, C. J. Xu, J. W. Shen, S. Ahmad, Y. Li, Exploring the impact of delay on Hopf bifurcation of a type of BAM neural network models concerning three nonidentical delays, Neural Process Lett., 55 (2023), 11595–11635. https://doi.org/10.1007/s11063-023-11392-0 doi: 10.1007/s11063-023-11392-0
![]() |
[21] |
S. Li, C. D. Huang, X. Y. Song, Detection of Hopf bifurcations induced by pregnancy and maturation delays in a spatial predator-prey model via crossing curves method, Chaos Soliton. Fract., 175 (2023), 114012. https://doi.org/10.1016/j.chaos.2023.114012 doi: 10.1016/j.chaos.2023.114012
![]() |
[22] |
X. Z. Feng, X. Liu, C. Sun, Y. L. Jiang, Stability and Hopf bifurcation of a modified Leslie-Gower predator-prey model with Smith growth rate and B-D functional response, Chaos Soliton. Fract., 174 (2023), 113794. https://doi.org/10.1016/j.chaos.2023.113794 doi: 10.1016/j.chaos.2023.113794
![]() |
[23] | Z. Z. Zhang, H. Z. Yang, Hybrid control of Hopf bifurcation in a two prey one predator system with time delay, In: Proceeding of the 33rd Chinese Control Conference, IEEE, Nanjing, China, 2014, 6895–6900. https://doi.org/10.1109/chicc.2014.6896136 |
[24] |
L. P. Zhang, H. N. Wang, M. Xu, Hybrid control of bifurcation in a predator-prey system with three delays, Acta Phys. Sin., 60 (2011), 010506. https://doi.org/10.7498/aps.60.010506 doi: 10.7498/aps.60.010506
![]() |
[25] |
Z. Liu, K. W. Chuang, Hybrid control of bifurcation in continuous nonlinear dynamical systems, Int. J. Bifurcat. Chaos, 15 (2005), 1895–3903. https://doi.org/10.1142/S0218127405014374 doi: 10.1142/S0218127405014374
![]() |
[26] |
J. Alidousti, Stability and bifurcation analysis for a fractional prey-predator scavenger model, Appl. Math. Model., 81 (2020), 342–355. https://doi.org/10.1016/j.apm.2019.11.025 doi: 10.1016/j.apm.2019.11.025
![]() |
[27] |
W. G. Zhou, C. D. Huang, M. Xiao, J. D. Cao, Hybrid tactics for bifurcation control in a fractional-order delayed predator-prey model, Physica A, 515 (2019), 183–191. https://doi.org/10.1016/j.physa.2018.09.185 doi: 10.1016/j.physa.2018.09.185
![]() |
[28] |
Y. Q. Zhang, P. L. Li, C. J. Xu, X. Q. Peng, R. Qiao, Investigating the effects of a fractional operator on the evolution of the ENSO model: Bifurcations, stability and numerical analysis, Fractal Fract., 7 (2023), 602. https://doi.org/10.3390/fractalfract7080602 doi: 10.3390/fractalfract7080602
![]() |
[29] |
P. L. Li, Y. J. Lu, C. J. Xu, J. Ren, Insight into Hopf bifurcation and control methods in fractional order BAM neural networks incorporating symmetric structure and delay, Cogn. Comput., 15 (2023), 1825–1867. https://doi.org/10.1007/s12559-023-10155-2 doi: 10.1007/s12559-023-10155-2
![]() |
[30] |
C. J. Xu, M. Farman, Dynamical transmission and mathematical analysis of Ebola virus using a constant proportional operator with a power law kernel, Fractals Fract., 7 (2023), 706. https://doi.org/10.3390/fractalfract7100706 doi: 10.3390/fractalfract7100706
![]() |
[31] |
C. J. Xu, Y. Y. Zhao, J. T. Lin, Y. C. Pang, Z. X. Liu, J. W. Shen, et al., Mathematical exploration on control of bifurcation for a plankton-oxygen dynamical model owning delay, J. Math. Chem., 2023, 1–31. https://doi.org/10.1007/s10910-023-01543-y doi: 10.1007/s10910-023-01543-y
![]() |
1. | Maciej Leszczynski, Przemyslaw Perlikowski, Piotr Brzeski, A Unified Approach for the Calculation of Different Sample-Based Measures with the Single Sampling Method, 2024, 12, 2227-7390, 987, 10.3390/math12070987 | |
2. | Chunyan Zhao, Mati Ur Rahman, Hadi Rezazadeh, Mohammad Ali Hosseinzadeh, Novel multi breather like, periodic, hybrid periodic and singular periodic waves of the Schrödinger–Hirota equation having the parabolic-law nonlinearity, 2024, 56, 1572-817X, 10.1007/s11082-024-07047-6 | |
3. | Ziyad A. Alhussain, Exploring multi-soliton patterns, bifurcation analysis, and chaos in (2+1) dimensions: A study on nonlinear dynamics, 2024, 15, 20904479, 102917, 10.1016/j.asej.2024.102917 | |
4. | Anil Chavada, Nimisha Pathak, Sagar R. Khirsariya, A fractional mathematical model for assessing cancer risk due to smoking habits, 2024, 4, 2767-8946, 246, 10.3934/mmc.2024020 | |
5. | Li Jia, Hongwu Tan, Hui Cao, Hopf bifurcation of the recurrent infectious disease model with disease age and two delays, 2024, 185, 09600779, 115120, 10.1016/j.chaos.2024.115120 | |
6. | Deepa Gupta, Sachin Bhalekar, Fractional order sunflower equation: stability, bifurcation and chaos, 2024, 1951-6355, 10.1140/epjs/s11734-024-01356-3 | |
7. | Sajid Ali, Aman Ullah, Saud Fahad Aldosary, Shafiq Ahmad, Shabir Ahmad, Construction of optical solitary wave solutions and their propagation for Kuralay system using tanh-coth and energy balance method, 2024, 59, 22113797, 107556, 10.1016/j.rinp.2024.107556 | |
8. | Youhua Qian, Meirong Ren, Haolan Wang, Dynamic behavior of a class of predator–prey model with two time delays, 2024, 0001-5970, 10.1007/s00707-024-04111-w | |
9. | Huayong Zhang, Fenglu Guo, Hengchao Zou, Lei Zhao, Zhongyu Wang, Xiaotong Yuan, Zhao Liu, Refuge-driven spatiotemporal chaos in a discrete predator-prey system, 2024, 182, 09600779, 114613, 10.1016/j.chaos.2024.114613 | |
10. | Mati ur Rahman, Yeliz Karaca, Mei Sun, Dumitru Baleanu, Wafa F. Alfwzan, Complex behaviors and various soliton profiles of (2+1)-dimensional complex modified Korteweg-de-Vries Equation, 2024, 56, 1572-817X, 10.1007/s11082-024-06514-4 | |
11. | Mati ur Rahman, Shafiq Ahmad, Meraj Ali Khan, Mei Sun, Wafa F. Alfwzan, A diverse array of optical solitons in the damped (2 + 1)-dimensional nonlinear Schrödinger equation via the modified exponential rational function method and other distinct strategies, 2024, 56, 1572-817X, 10.1007/s11082-024-06705-z | |
12. | Benito Chen-Charpentier, A Model of Hepatitis B Viral Dynamics with Delays, 2024, 4, 2673-9909, 182, 10.3390/appliedmath4010009 | |
13. | Amr M. S. Mahdy, Mohamed A. Abdou, Doaa Sh. Mohamed, Numerical solution, convergence and stability of error to solve quadratic mixed integral equation, 2024, 1598-5865, 10.1007/s12190-024-02194-1 | |
14. | Qingyi Cui, Changjin Xu, Yiya Xu, Wei Ou, Yicheng Pang, Zixin Liu, Jianwei Shen, Muhammad Zafarullah Baber, Chinnamuniyandi Maharajan, Uttam Ghosh, Bifurcation and Controller Design of 5D BAM Neural Networks With Time Delay, 2024, 37, 0894-3370, 10.1002/jnm.3316 | |
15. | Sajjad Ali, Meraj Ali Khan, Aman Ullah, Saud Fahad Aldosary, Mati ur Rahman, Shabir Ahmad, Optical solitary waves solutions of the eight-order dispersive Schrödinger wave equation, 2024, 56, 1572-817X, 10.1007/s11082-024-07093-0 | |
16. | R. N. Premakumari, Chandrali Baishya, Shahram Rezapour, Manisha Krishna Naik, Zaher Mundher Yaseem, Sina Etemad, Qualitative Properties and Optimal Control Strategy on a Novel Fractional Three-Species Food Chain Model, 2024, 23, 1575-5460, 10.1007/s12346-024-01110-z | |
17. | Laila A. Al-Essa, Mati ur Rahman, A survey on fractal fractional nonlinear Kawahara equation theoretical and computational analysis, 2024, 14, 2045-2322, 10.1038/s41598-024-57389-0 | |
18. | Doaa Filali, Faizan Ahmad Khan, Relation-Preserving Functional Contractions Involving a Triplet of Auxiliary Functions with an Application to Integral Equations, 2024, 16, 2073-8994, 691, 10.3390/sym16060691 | |
19. | Yunzhang Zhang, Changjin Xu, Bifurcation Exploration and Controller Design in a Fractional Oxygen–Plankton Model with Delay, 2024, 8, 2504-3110, 190, 10.3390/fractalfract8040190 | |
20. | Sadia Yasin, Meraj Ali Khan, Shabir Ahmad, Saud Fahad Aldosary, Abundant new optical solitary waves of paraxial wave dynamical model with kerr media via new extended direct algebraic method, 2024, 56, 1572-817X, 10.1007/s11082-024-06845-2 | |
21. | Shafiq Ahmad, Saud Fahad Aldosary, Meraj Ali Khan, Mati ur Rahman, Faisal Alsharif, Shabir Ahmad, Analyzing optical solitons in the generalized unstable NLSE in dispersive media, 2024, 307, 00304026, 171830, 10.1016/j.ijleo.2024.171830 | |
22. | Konstantin E. Starkov, Alexander P. Krishchenko, On the Dynamics of Immune-Tumor Conjugates in a Four-Dimensional Tumor Model, 2024, 12, 2227-7390, 843, 10.3390/math12060843 | |
23. | Kamal Shah, Muhammad Sarwar, Thabet Abdeljawad, A comprehensive mathematical analysis of fractal–fractional order nonlinear re-infection model, 2024, 103, 11100168, 353, 10.1016/j.aej.2024.06.022 | |
24. | Bing Zhao, Shuting Lyu, Qimin Zhang, Dynamics and density function for a stochastic anthrax epidemic model, 2024, 32, 2688-1594, 1574, 10.3934/era.2024072 | |
25. | Mei Wang, Baogua Jia, Finite-time stability and uniqueness theorem of solutions of nabla fractional $ (q, h) $-difference equations with non-Lipschitz and nonlinear conditions, 2024, 9, 2473-6988, 15132, 10.3934/math.2024734 | |
26. | Laila A. Al-Essa, Mati ur Rahman, Novel stochastic multi breather type, a-periodic, hybrid periodic and other type of waves of the Shrödinger–Hirota model with Wiener process, 2024, 56, 1572-817X, 10.1007/s11082-024-07042-x | |
27. | Yunzhang Zhang, Changjin Xu, Novel Hopf Bifurcation Exploration and Control Strategies in the Fractional-Order FitzHugh–Nagumo Neural Model Incorporating Delay, 2024, 8, 2504-3110, 229, 10.3390/fractalfract8040229 | |
28. | Dumitru Baleanu, Sania Qureshi, Abdullahi Yusuf, Amanullah Soomro, M.S. Osman, Bi-modal COVID-19 transmission with Caputo fractional derivative using statistical epidemic cases, 2024, 10, 26668181, 100732, 10.1016/j.padiff.2024.100732 | |
29. | Changjin Xu, Yingyan Zhao, Jinting Lin, Yicheng Pang, Zixin Liu, Jianwei Shen, Maoxin Liao, Peiluan Li, Youxiang Qin, Bifurcation investigation and control scheme of fractional neural networks owning multiple delays, 2024, 43, 2238-3603, 10.1007/s40314-024-02718-2 | |
30. | Jie Liu, Jian-Ping Sun, Pinning clustering component synchronization of nonlinearly coupled complex dynamical networks, 2024, 9, 2473-6988, 9311, 10.3934/math.2024453 | |
31. | Ke He, Jianping Shi, Hui Fang, Bifurcation and chaos analysis of a fractional-order delay financial risk system using dynamic system approach and persistent homology, 2024, 223, 03784754, 253, 10.1016/j.matcom.2024.04.013 | |
32. | Shabir Ahmad, Saud Fahad Aldosary, Meraj Ali Khan, Stochastic solitons of a short-wave intermediate dispersive variable (SIdV) equation, 2024, 9, 2473-6988, 10717, 10.3934/math.2024523 | |
33. | Ibtehal Alazman, Badr Saad T. Alkahtani, Mati ur Rahman, Manvendra Narayan Mishra, Nonlinear complex dynamical analysis and solitary waves for the (3+1)-D nonlinear extended Quantum Zakharov–Kuznetsov equation, 2024, 58, 22113797, 107432, 10.1016/j.rinp.2024.107432 | |
34. | Bikash Modak, Muthu P, Stochastic approach to a delayed in-host model of DENV transmission, 2024, 99, 0031-8949, 075006, 10.1088/1402-4896/ad4ea6 | |
35. | Changjin Xu, Wei Ou, Qingyi Cui, Yicheng Pang, Maoxin Liao, Jianwei Shen, Muhammad Zafarullah Baber, Chinnamuniyandi Maharajan, Uttam Ghosh, Theoretical exploration and controller design of bifurcation in a plankton population dynamical system accompanying delay, 2024, 0, 1937-1632, 0, 10.3934/dcdss.2024036 | |
36. | Xiao-Long Gao, Hao-Lu Zhang, Xiao-Yu Li, Research on pattern dynamics of a class of predator-prey model with interval biological coefficients for capture, 2024, 9, 2473-6988, 18506, 10.3934/math.2024901 | |
37. | Qingyi Cui, Changjin Xu, Wei Ou, Yicheng Pang, Zixin Liu, Jianwei Shen, Muhammad Farman, Shabir Ahmad, Further study on Hopf bifurcation and hybrid control strategy in BAM neural networks concerning time delay, 2024, 9, 2473-6988, 13265, 10.3934/math.2024647 | |
38. | Parvaiz Ahmad Naik, Muhammad Amer, Rizwan Ahmed, Sania Qureshi, Zhengxin Huang, Stability and bifurcation analysis of a discrete predator-prey system of Ricker type with refuge effect, 2024, 21, 1551-0018, 4554, 10.3934/mbe.2024201 | |
39. | Changjin Xu, Jinting Lin, Yingyan Zhao, Qingyi Cui, Wei Ou, Yicheng Pang, Zixin Liu, Maoxin Liao, Peiluan Li, New results on bifurcation for fractional-order octonion-valued neural networks involving delays*, 2024, 0954-898X, 1, 10.1080/0954898X.2024.2332662 | |
40. | Ilse Domínguez-Alemán, Itzel Domínguez-Alemán, Juan Carlos Hernández-Gómez, Francisco J. Ariza-Hernández, A predator-prey fractional model with disease in the prey species, 2024, 21, 1551-0018, 3713, 10.3934/mbe.2024164 | |
41. | Sayed Saifullah, Shabir Ahmad, Meraj Ali Khan, Mati ur Rahman, Multiple solitons with fission and multi waves interaction solutions of a (3+1)-dimensional combined pKP-BKP integrable equation, 2024, 99, 0031-8949, 065242, 10.1088/1402-4896/ad482b | |
42. | Qiaofeng Li, Jianli Li, pth Moment Exponential Stability of Impulsive Stochastic Functional Differential Equations, 2025, 24, 1575-5460, 10.1007/s12346-024-01170-1 | |
43. | Changjin Xu, Muhammad Farman, Aamir Shehzad, Kottakkaran Sooppy Nisar, Modeling and Ulam–Hyers stability analysis of oleic acid epoxidation by using a fractional‐order kinetic model, 2025, 48, 0170-4214, 3726, 10.1002/mma.10510 | |
44. | Zahra Sadat Aghayan, Alireza Alfi, Yashar Mousavi, Afef Fekih, Robust Delay-Dependent Output-Feedback PD Controller Design for Variable Fractional-Order Uncertain Neutral Systems With Time-Varying Delays, 2025, 55, 2168-2216, 1986, 10.1109/TSMC.2024.3508573 |