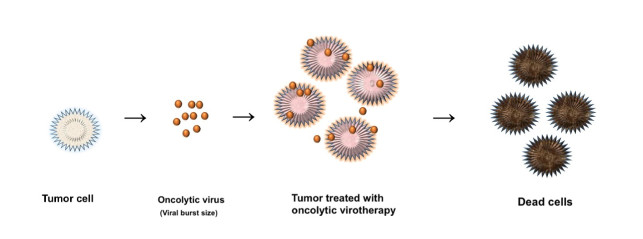
New cancer therapies, methods and protocols are needed to treat affected patients. Oncolytic viral therapy is a good suggestion for such treatment. This paper proposes a diffusive cancer model with virotherapy and an immune response. This work aims to study the aforementioned model while theoretically including positivity, boundedness and stability, as well as to find the analytical solutions. The analytical solutions are found by using the tanh-expansion method. As a result, we realized that the relative immune cell killing rate can be controlled by the viral burst size. The viral burst size is the number of viruses released from each infected cell during cell lysis. The increasing diffusion of the activated immune system leads to an increase in the uninfected cells. The presented model can be used to study the combination of immunotherapy and virotherapy.
Citation: Noufe H. Aljahdaly, Nouf A. Almushaity. A diffusive cancer model with virotherapy: Studying the immune response and its analytical simulation[J]. AIMS Mathematics, 2023, 8(5): 10905-10928. doi: 10.3934/math.2023553
[1] | Afnan Al Agha, Hakim Al Garalleh . Oncolysis by SARS-CoV-2: modeling and analysis. AIMS Mathematics, 2024, 9(3): 7212-7252. doi: 10.3934/math.2024351 |
[2] | Necati Özdemir, Esmehan Uçar . Investigating of an immune system-cancer mathematical model with Mittag-Leffler kernel. AIMS Mathematics, 2020, 5(2): 1519-1531. doi: 10.3934/math.2020104 |
[3] | Abdon Atangana, Saima Rashid . Analysis of a deterministic-stochastic oncolytic M1 model involving immune response via crossover behaviour: ergodic stationary distribution and extinction. AIMS Mathematics, 2023, 8(2): 3236-3268. doi: 10.3934/math.2023167 |
[4] | Kasbawati, Yuliana Jao, Nur Erawaty . Dynamic study of the pathogen-immune system interaction with natural delaying effects and protein therapy. AIMS Mathematics, 2022, 7(5): 7471-7488. doi: 10.3934/math.2022419 |
[5] | Irina Volinsky, Svetlana Bunimovich-Mendrazitsky . Mathematical analysis of tumor-free equilibrium in BCG treatment with effective IL-2 infusion for bladder cancer model. AIMS Mathematics, 2022, 7(9): 16388-16406. doi: 10.3934/math.2022896 |
[6] | Ru Meng, Yantao Luo, Tingting Zheng . Stability analysis for a HIV model with cell-to-cell transmission, two immune responses and induced apoptosis. AIMS Mathematics, 2024, 9(6): 14786-14806. doi: 10.3934/math.2024719 |
[7] | Muhammad Farman, Aqeel Ahmad, Ali Akgül, Muhammad Umer Saleem, Kottakkaran Sooppy Nisar, Velusamy Vijayakumar . Dynamical behavior of tumor-immune system with fractal-fractional operator. AIMS Mathematics, 2022, 7(5): 8751-8773. doi: 10.3934/math.2022489 |
[8] | A. M. Elaiw, A. S. Shflot, A. D. Hobiny . Stability analysis of SARS-CoV-2/HTLV-I coinfection dynamics model. AIMS Mathematics, 2023, 8(3): 6136-6166. doi: 10.3934/math.2023310 |
[9] | Hsiu-Chuan Wei . Mathematical modeling of ER-positive breast cancer treatment with AZD9496 and palbociclib. AIMS Mathematics, 2020, 5(4): 3446-3455. doi: 10.3934/math.2020223 |
[10] | Deokro Lee, Amit Koul, Nikhat Lubna, Sean A. McKenna, Stéphanie Portet . Mathematical modelling of OAS2 activation by dsRNA and effects of dsRNA lengths. AIMS Mathematics, 2021, 6(6): 5924-5941. doi: 10.3934/math.2021351 |
New cancer therapies, methods and protocols are needed to treat affected patients. Oncolytic viral therapy is a good suggestion for such treatment. This paper proposes a diffusive cancer model with virotherapy and an immune response. This work aims to study the aforementioned model while theoretically including positivity, boundedness and stability, as well as to find the analytical solutions. The analytical solutions are found by using the tanh-expansion method. As a result, we realized that the relative immune cell killing rate can be controlled by the viral burst size. The viral burst size is the number of viruses released from each infected cell during cell lysis. The increasing diffusion of the activated immune system leads to an increase in the uninfected cells. The presented model can be used to study the combination of immunotherapy and virotherapy.
Recently, cancer therapies have been heavily developed in order to find a therapy that is able to decay the tumor in a short time without harming the neighboring healthy tissue. In the field of genetic engineering, scientists discovered a new cancer treatment by using genetically altered viruses [1]. Oncolytic viruses are genetically altered viruses that infect cancer cells. They grow in an abnormal tumor cell and destroy it without infecting healthy cells or normal tissue. The oncolytic viruses interact with the tumor cell and generate a burst of an oncolytic virus (see Figure 1). The burst size is the number of new viruses resulting from the lysis of an infected tumor cell, and some of the new viruses may infect nearby tumor cells. The burst size is used to measure the replicability of an oncolytic virus [2].
Mathematical models are able to describe biological and medical problems, such as cancer models with a variety of therapies [3,4], epidemic models [5], HIV infection models [6,7,8] and prey-predator models [9]. A few researchers have studied mathematical models of cancer with virotherapy and described the interaction between the virus and the tumor. Wodarz introduced a basic mathematical model that studied tumor growth in the presence of virotherapy treatment [10]. The model has been modified to study the relationship between burst size and virus replicability. The results showed that cancer cells decrease if the burst size is large [11]. On the other hand, some mathematical models have demonstrated the immune system response to virotherapy. Since the immune system treats viruses as foreign bodies and thus destroys them, it shows a negative response to virotherapy that may reduce the quality of viral treatment [12]. Also, many studies describe the interactions between uninfected and infected cells and different kinds of immune responses [13,14].
Herein, we will review the basic model of oncolytic virus replication which was introduced by Wodarz [15]. The model studies the tumor growth and the infection term and it is given by the following system of ordinary differential equations (ODEs):
dfdt=ˉrX(f,y)−ˉβyG(f,y),dydt=ˉβyG(f,y)−ˉδy, | (1) |
where f and y are the uninfected and infected cells, respectively, the function X represents the growth of f and y and the function G denotes the rate at which tumor cells become infected by the virus. The coefficients ˉβ and ˉδ are the infection rate of the virus and the death rate of virus-infected cells, respectively, and the coefficient ˉr is the logistical growth rate of uninfected cells. Then, Tian [2] proposed a following common basic model for the virotherapy of three populations:
dfdt=ˉrf(1−f+yk)−ˉβfv,dydt=ˉβfv−ˉδy,dvdt=ˉbˉδy−ˉβfv−ˉγv, | (2) |
where v is the free virus population and ˉγ is the death rate of the virus. The coefficient ˉbˉδ refers to the burst size of new viral swarms resulting from killing an infected cell y. The model (2) was also modified in [16] to study the dynamics of oncolytic virotherapy, and it includes four populations, as follows:
dfdt=ˉrf(1−f+yC)−ˉβfv−αfz−df,dydt=ˉβfv−ˉδy−ˉμyz,dvdt=ˉbˉδy−ˉβfv−ˉγv−ˉkvz,dzdt=ˉsyz−ˉpz, | (3) |
where z denotes the innate immune cell population, ˉs is the rate of stimulation of the innate immune cell and ˉp is the rate of immune clearance. Moreover, some models have demonstrated the spread of tumor cells under viral therapy and radiation therapy [17,18].
The immune response and its impact in both uninfected and infected cells were considered in the model (3) in Reference [19] as follows:
dfdt=ˉrf(1−f+yC)−ˉβfv−αfz−df,dydt=ˉβfv−ˉδy−ˉμ1yz,dvdt=ˉbˉδy−ˉβfv−ˉγv−ˉkvz,dzdt=ˉs1yz+ˉs2zf−ˉpz, | (4) |
where the infected and uninfected cells stimulate the immune response at rates s1 and s2, respectively. The novelty of this paper is its study of the mathematical model in [19] by distinguishing between the naive and activated immune system cells. In addition, we study the spread of viruses and cells by taking a diffusion term into account. Thus, we obtain a system of partial differential equations (PDEs). The immune system cells can be activated by immunotherapy or biological therapy. Therefore, it is very important to distinguish between the naive and activated immune system cells in the mathematical model. Thus, the model introduced in this paper can be useful to study the effects of combining immunotherapy with virotherapy.
The paper is organized as follows. The second section introduces the studied mathematical model, the third section is the theoretical study of the system, the fourth section shows the analytical solutions obtained via the tanh-expansion method, the fifth section discuses the results and the last section is a summary of the work.
In this section, we reformulate the mathematical model in [19] by considering the five cell populations, which are uninfected cancer cells f, infected cancer cells y, the free virus v, the naive immune cells z and the activated immune cells za, as follows:
∂f∂t=ˉrf(1−f+yC)−ˉβfv−¯αffza+d1∂2f∂x2,∂y∂t=ˉβfv−ˉδy−ˉμ1yza+d2∂2y∂x2,∂v∂t=ˉbˉδy−ˉβxv−ˉγv−ˉkvza+d3∂2z∂x2,∂z∂t=ˉλz−ˉpz−ˉs1yz−ˉs2zf+d4∂2z∂x2,∂za∂t=ˉs1yz+ˉs2zf−ˉμ2za+d5∂2z∂x2, | (5) |
where di,i=1,2,3,4,5 denotes the diffusion terms of f,y,v,z and za, respectively. The rest of the parameters are converted to dimensionless parameters in the following system of PDEs and described in Table 1.
Parameters | Description |
c | Carrying capacity of the tumor cells |
r | Tumor growth rate |
λz | Stimulation rate of the immune response |
b | Burst size of the virus |
αf | Rate of immune-mediated uninfected tumor cell death |
δ | Death rate of infected tumor cells |
β | Infection rate of the virus |
p | Clearance rate of the immune response |
κ | Rate of immune-mediated virus death |
γ | Clearance rate of the virus |
μ1 | Rate of immune-mediated infected tumor cell death |
μ2 | Clearance rate of active immune cells |
s1 | Stimulation rate of the immune response by infected cells |
s2 | Stimulation rate of the immune response by uninfected cells |
The system becomes dimensionless by setting t=τδ, F=Cf, Y=Cy, V=Cv, Z=Cz and Za=Cza and renaming the parameters as follows:
r=ˉrδ,β=Cˉβδ,αf=Cˉαδ,μ1=¯μ1δ,γ=ˉγδ,k=ˉkδ,λz=ˉλzδ,p=ˉpδ,s1=¯s1δ,s2=¯s2δμ2=¯μ2δ. |
Thus, we obtain the following diffusive PDEs:
∂F∂t−d1∂2F∂x=rF(1−(F+Y))−βFV−αfFZa,∂Y∂t−d2∂2Y∂x=βFV−Y−μ1YZa,∂V∂t−d3∂2V∂x=bY−βFV−γV−κVZa,∂Z∂t−d4∂2Z∂x=λz−pZ−s1YZ−s2FZ,∂Za∂t−d5∂2Za∂x=s1YZ+s2FZ−μ2Za. | (6) |
Then, the initial conditions become F(0)=f0C, Y(0)=y0C, V(0)=v0C, Z(0)=x0C, Za(0)=xa0C.
Theorem 1. Let (F(x,t),Y(x,t),V(x,t),Z(x,t),Za(x,t))=(ˆf(ξ),ˆy(ξ),ˆv(ξ),ˆz(ξ),ˆza(ξ))∈R5+, where ξ=kx+ct+ξ0; then, the solutions (ˆf(ξ),ˆy(ξ),ˆv(ξ),ˆz(ξ),ˆza(ξ)) are nonnegative and bounded in the following region:
Ω=(ˆf,ˆy,ˆv,ˆz,ˆza)∈R5+∣,ˆf≤1,ˆf+ˆy≤1,ˆv≤bγ,ˆz+^za≤λzζ, |
where ζ=min{p,μ2}.
Proof. First, we specify the initial value problems for System (6) as follows:
∂Fdt−d1∂2F∂x=0,∂Ydt−d2∂2Y∂x=βFV,≥0,∂Vdt−d3∂2V∂x=bY,≥0,∂Zdt−d4∂2Z∂x=λz≥0,∂Zadt−d5∂2Za∂x=s1YZ+s2FZ,≥0. |
As a result, the solutions are non-decreasing. In order to study the boundedness for the aforementioned system of PDEs, we will transfer the system to a system of ODEs using the traveling wave transformation. Let us define ξ=kx+ct+ξ0; then, we get
dˆfdξ=rcˆf(1−(ˆf+ˆy))−βcˆfˆv−αfcˆf^za+d1k2cˆfξξ,dˆydξ=βcˆfˆv−1cˆy−μ1cˆy^za+d2k2cˆyξξ,dˆvdξ=bcˆy−βcˆfˆv−γcˆv−κcˆv^za+d3k2cˆvξξ,dˆzdξ=λzc−pcˆz−s1cˆyˆz−s2cˆfˆz+d4k2cˆzξξ,d^zadξ=s1cˆyˆz+s2cˆfˆz−μ2c^za+d5k2c^zaξξ. | (7) |
From the first equation in System (7), we have
dˆfdξ=rcˆf(1−(ˆf+ˆy))−βcˆfˆv−αcˆf^za≤rcˆf(1−ˆf). |
Let us assume the differential equationdF1dt=r1F1(1−F1) with the initial condition F1(0)=F0, which satisfies
F1(t)=F0F0+(1−F0)exp−r1t; |
hence,
limt→∞supF1=1. |
Note that dˆfdt≤dF1dt, which implies that limt→∞supˆf≤limt→∞supF1. Therefore, we have
limt→∞supˆf≤1. |
From the first and second equations in System (7), we have
dˆfdξ+dˆydξ≤rcˆf(1−(ˆf+ˆy)),≤rc(1−(ˆf+ˆy)), |
which satisfy
limt→∞supˆf+ˆy≤1. |
Again, from the third equation in System (7), we have
dˆvdξ≤bcˆy−γcˆv,≤bc−γcˆv, |
which yields
limt→∞supˆv≤bγ. |
Also, from the fourth and fifth equations in System (7), we note that
dˆzdξ+d^zadξ≤λzc−pcˆz−μ2c^za,≤λzc−ζc(ˆz+ˆza), |
where ζ=min{p,μ2}. Thus, we obtain
limt→∞supˆz+ˆza≤λzζ |
and
ˆz+ˆza≤λzζ. |
Model (6) has equilibrium points of the form (F,Y,V,Z,Za) such that F≥0,Y≥0,V≥0,Z≥0 and Za≥0. The equilibrium points of the system are the steady-state solutions, which are obtained by setting the following:
∂Fdt−d1∂2F∂x=0,∂Ydt−d2∂2Y∂x=0,∂Vdt−d3∂2V∂x=0,∂Zdt−d4∂2Z∂x=0,∂Zadt−d5∂2Za∂x=0. |
However, with help of Mathematica, we obtained five equilibrium points:
E0=(0,0,0,0,0),E1=(1,0,0,0,0),E2=(γ(b−1)β,γ(b−1)β(βr(b−1)−γr(b−1)β+γr),r((b−1)β−γ)β((b−1)β+γr),0,0),E3=((λz−p)s2,0,0,μ2r(−λz+p+s2)αfs2(λz−p),r(−λz+p+s2)αfs2),E4=(F4,Y4,V4,Z4,Za4), |
where
r(1−(F4+Y4))−βV4−αfZa4=0,Y4=βF5V4μ1Za4+1,V4=bY4βF4+κZa4+γ,Z4=λzs1Y5+s2F5+p,Za4=s1Y4+s2F4μ2Z4. |
E2 is positive if b>1+(γ/β), and E3 is positive if p<λz<p+s2.
The basic reproduction number is the number of cases that are generated by a signal virus in the population [20]. Let P=(Y,F,V,Z,Za), model (7) is rewritten as
P′=υ1(P)−υ2(P), |
where
υ1(P)=(βFV000),υ2(P)=(μ1YZa−rF(1−(F+Y))+βFV+αfFZa−bY+βFV+κVZa+γV−λz+pZ+s1YZ+s2FZ−s1YZ−s2FZ+μ2Za). |
Next, the Jacobian matrix for υ1(P) and υ2(P) at E1 are computed as follows:
Υ1=D(υ1(E1))=(00β00000000000000000), |
Υ2=D(υ2(E1))=(10000rrβ0αF−b0β+γ00000p+s20000−s2μ2), |
(Υ1Υ−12)(bββ+γ0ββ+γ000000), |
R0=ρ(Υ1Υ−12)=bββ+γ, | (8) |
where ρ is the spectral radius. Thus, if R0<1, the cancer will decline; if R0=1, the cancer will stay alive and stable; if R0>1, the cancer will experience growth and outbreak. Therefore, E0 and E1 always exist, E2 exists if R0>1, E3 exists if p+s2>λz>p and E4 exists if R0>1.
We investigate the local stability of the equilibrium points by evaluating the Jacobian matrix of the nonlinear system [21].
Theorem 2. The trivial equilibrium E0 of the system is locally unstable.
Proof. The Jacobian matrix of System (6) at E0 is
J(E0)=(r00000−10000b−γ00000−p00000−μ2). | (9) |
It is obvious that the eigenvalues for J(E0) are as follows:
λ1=r,λ2=−1,λ3=−γ,λ4=−p,λ5=−μ2. |
All eigenvalues are negative if r<0. Hence, E0 is locally unstable if r>0.
Theorem 3. The equilibrium point E1 is locally asymptotically stable if only if R0<1.
Proof. By the calculation of the Jacobian matrix at E1, we obtain
J(E1)=(−r−r−β0−α0−1β000b−β−γ00000−p−s20000s2−μ2). | (10) |
The characteristic polynomial is
det(J(E1)−λI)=(−μ2−λ)(−(p+s2)−λ)(−λ−r)((−bβ+β+γ)+λ(β+γ+1)+λ2). |
The eigenvalues of J(E1), which are λ1=−μ2, λ2=−r and λ3=−(p+s2), are negative. By the Routh-Hurwitz criteria, since (β+γ+1) is positive, λ4,5 has a real negative part if b≤1+γβ, which implies that R0<1. Biologically, when the viral burst size b is smaller than the critical value which is 1+γβ, the new produced viruses will be enough to infect tumor cells.
Theorem 4. The equilibrium point E2 is locally asymptotically stable if b>1 and β<r.
Proof. The jacobian matrix J(E2) is
(γrβ−bβγrβ−bβ−γb−10αγβ−bβr((b−1)β−γ)(b−1)β+γr−1γb−10−γμ1r((b−1)β−γ)(b−1)β((b−1)β+γr)r(−bβ+β+γ)(b−1)β+γrbbγ1−b0kr(−bβ+β+γ)β((b−1)β+γr)000γ(rs1(−bβ+β+γ)+s2(−bβ+β−γr))(b−1)β((b−1)β+γr)0000γ(rs1((b−1)β−γ)+s2((b−1)β+γr))(b−1)β((b−1)β+γr)−μ2), | (11) |
det(J(E2)−λI)=(−μ2−λ)(−βs1F3V3−s2F3−p−λ)(λ3+A1λ2+A2λ+A3), |
where
A1=bβγ+β(b−1)+βγr(b−1)β,A2=(rβ−1)(γr(−β+bβ−γ))(b−1)(−β+bβ+γr)+r(bγ2+(b−1)γ)(b−1)2β,A3=γr(β−bβ+γ)((b−1)β+γr)(b−1)β(β−bβ−γr). |
The eigenvalues are λ1=−μ2 and λ2=−βs1F3V3−s2F3−p, which are both negative, while λ3,4,5 denotes the zeros of the polynomial λ3+A1λ2+A2λ+A3. It is obvious that A1>0 if b>1, A2>0 if b>1, β<r and R0>1, A3>0 if b>1 and R0>1, and
A1A2−A3=β(b−1)(bβ−β+rγ)bβγ−β+bβ+γr−(b+γr−1)(bβ−β+γr)(b−1)β(bβ−β+γ)=β(b−1)(β(b−1)+rγ)β(bγ−1)+bβ+γr−(b+γr−1)(β(b−1)+γr)(b−1)β(β(b−1)+γ). |
Therefore, by the Routh-Hurwitz criterion, λ3,4,5 have negative real parts if A1A2−A3>0, which occurs if b>1 and β<r. Thus, E2 is locally asymptotically stable.
Biologically, if the viruses are powerful, which means that the burst size is greater than the critical value, then the system has the equilibrium E2 with the balance of tumor cells, infected tumor cells and viruses.
Theorem 5. The equilibrium point E3 is locally asymptotically stable if b<1, bβ<R0 and R0<1. If λz=0, the point E3 is locally asymptotically stable under the conditions that αβ<κ and bβ<r.
To find the eigenvalue, solve the Jacobian J(E3):
(r(s2F3−2F3+1)−rF3−βF30−αfF30−μ1Z3a−1βF3000b−γ−βF3−κZ3a00s2Z3s1Z30−s2F3−p0−s2Z3−s1Z30−s2F3−μ2). |
The characteristic polynomial of this matrix is evaluated as follows:
P(λ)=λ5+b4λ4+b3λ3+b2λ2+b1λ1+b0=0, |
where b0,b1,b2,b3 and b4 are defined in the Appendix. All coefficients are positive if b<1. By using Descartes' rule, the number of sign changes is zero; thus, the point E3 is locally asymptotically stable if b<1.
Theorem 6. The equilibrium point E4 is locally stable if b<1, bβ<r, s1>s2, λz>Z4a and Z4>Z4a.
Proof. To find the Jacobian at the equilibrium point E4, we evaluate |J(E4)−λI|=0, where J(E4) is given by
(E1,1−βF40−αF4βV4−μ1Z4a−1βF40−μ1Y4−βV4b−γ−βF4−κZ4a0−κV4−s2Z4−s1Z40−F4s2−s1Y4−p0s2Z4s1Z40F4s2+s1Y4−μ2), |
where E1,1=r(1−Y4−2F4)−αZ4a−βV4−rF4. The characteristic polynomial is
a0λ5+a1λ4+a2λ3+a3λ2+a4λ+a5=0, |
where a0,a1,a2,a3,a4 and a5 are defined in the Appendix. All coefficients are positive if b<1, bβ<r, λz>Z4a, s1>s2 and Z4>Z4a. By using Descartes' rule, the number of sign changes is zero; thus, the point E4 is locally asymptotically stable if b<1, bβ<r, λz>Z4a, s1>s2 and Z4>Z4a.
In this section, the global stability of the equilibrium points will be investigated by using Lyapunov functions [22], which must be positive definite.
Theorem 7. E1 is globally asymptotically stable if 1+b≤r≤2,b≤1.
Proof. The Lyapunov function is defined at point E1 as follows:
L1(F,Y,V,Z,Za)=F−F1−lnF+Y+1−rbV+Z+Za. |
The derivative of L1 is
L′1=F′−F′F1+(1−r)V′b+Y′+Z′+Z′a,=(1−r)V′b+Y′+Z′+Z′a,=AYY+AVV+AZZ+AZaZa+AFVFV+AYZaYZa+AVZaVZa, |
where
AY=−2+r=−(2−r),AV=γb−rγb=γb(1−r),AZ=−p,AZa=−μ2,AFV=β+βb−rβb=1+1b(1−r),AYZa=−μ1,AVZa=κb−κrb=1b(1−r). |
The equilibrium equations at E1 are
rF1(1−F1)=rF1−rF21=0,λz=0. |
We found that L′1≤0 if 1+b≤r≤2,b≤1. Hence, E1 is globally asymptotically stable if 1+b≤r≤2,b≤1.
Theorem 8. E2 is globally asymptotically stable if 2<b,r>β R0<1 and 2<Y2<γrbβ.
Proof. The Lyapunov function is defined at E2 as follows:
L2(F,Y,V,Z,Za)=A1(F−F2−F2lnFF2)+A2(Y−Y2−Y2lnYY2)+A3(V−V2−V2lnVV2)+A4Z2+A5Z2a. |
Thus, we have L′2 as follows:
L′2=A1(1−F2F)F′+A2(1−Y2Y)Y′+A3(1−V2V)V′+A4Z′+A5Z′a. |
The equilibrium equations are
rF2(1−(F2+Y2))−βF2V2=0,r−rF2−rY2=βV2,βF2V2=Y2,βF2V2+γV2=bY2,γ=1V2(b−1)Y2,λz=0. | (12) |
Using the equilibrium equations in L′2 gives
L′2=CFF+CYY+CVV+CZZ+CZaZa+CFZaFZa+CvzaVZa+CYZaYZa+CF2F2+CFVFV+CFYFY+CFZFZ+CYVVY+CYZYZ+CFYVFVY+C∗, |
where
CF=A1F2r+A1r+A3βV2,CY=A3b+A1F2r−A2,CV=A1βF2−A3γ,CZ=−A4p,CZa=αA1F2+A3κV2+A2μ1Y2−A5μ2,CF2=−A1r,CFY=−A1r,CFV=−A3β−A1β+A2β,CFZ=−A4s2,CYZ=−A4s1,CFZa=−A1αf,CVZa=−A3κ,CYZa=−A2μ1,CYV=−A3bV2,CFYV=−A2βY2,C∗=−A1F2r+A3γV2+A2Y2+A4λz. |
Note that
CV=A1βF2−A3γ=A1Y2V2−A31V2(b−1)Y2=Y2V2(A1−A3(b−1)), |
and CV=0 if A3=A1b−1. Let A2=A3=A1b−1; then, CFV=−A1β and CF=A1(r(2−Y2)−βV2+βb−1V2). We have that CF<0 if Y2>2. Thus, we obtain CY as
CY=A1b−1(b−1)+A1F2r=A1(1+r−βV2−rβF2V2),=A1(1+r−βV2−rγb−1V2),=A1(1+r−(b−1)βγY2−rY2). |
Since Y2>2, CY<0. Thus, CZa becomes
CZa=A1(αfF2+κb−1V2+μ1b−1Y2)−A5μ2,=A1b−1(αfγβ+κV2+μ1Y2)−A5μ2=0. |
If A5=A1μ2(b−1)(αfγβ+κV2+μ1Y2) and we let A4=A1, we obtain C∗ as follows:
C∗=−A1F2r+A3γV2+A2Y2,=−A1F2r+A1b−1((b−1)Y2+Y2),=A1(−F2r+bb−1Y2). |
We get that L′2≤0 if 2<Y2<γrbβ. Hence, E2 is globally asymptotically stable.
Theorem 9. E3 is globally asymptotically stable if b<1; then, p<λ<p(μ2r+αfs2Z3)μ2r+αfs2, γ(r+αfs1Z3μ2)1−b<β, and r>2λzαfμ2.
Proof. The Lyapunov function at E3 is defined as follows:
L3(F,Y,V,Z,Za)=A1(F−F3−F3lnFF3)+A2Y+A3V+A4(Z−Z3−Z3lnZZ3)+A5(Za−Za3−Za3lnZaZa3), |
and its derivative is
L′3=A1(1−F3F)F′+A2Y′+A3V′+A4(1−Z3Z)Z′+A5(1−Za3Za)Z′a. |
After substituting the points F3,Z3 and Za3 in L′3, we get
L′3=BFF+BYY+BVV+BZZ+BZaZa+BFZaFZa+BvzaVZa+BYZaYZa+BF2F2+BFVFV+BFYFY+BFZFZ+BYZYZ+BFZZaFZZa+BYZZaYZZa+B∗, |
where
BF=A1r+A1F3r+A4s2Z3,BY=A4s1Z3+A1rF3+A3b−A2,BV=−A3γ+A1βF3,BZ=−A4λzZ3−A4p,BZa=−A5μ2+A1F3αf,BF2=−A1r,BFY=−A1r,BFV=−A3β+A2β−A1β,BFZ=A5s2−A4s2,BYZ=A5s1−A4s1,BFZa=−A1αf,BVZa=−A3κ,BYZa=−A2μ1,BFZZa=−A5s2Z3a,BYZZa=−A5s1Z3a,B∗=−A1F3r+A5μ2Z3a+A4λz+A4pZ3. |
The equilibrium equations at (F3,0,0,Z3,Za3) are
0=r(1−F3)−αfZ3a,0=λz−pZ3−s2F3Z3,0=s2F3Z3−μ2Z3a. | (13) |
If A4=A5, then BFZ=0 and BYZ=0. If A3=A2, then BFV<0, A3=A1βF3γ and BV=0. If A5=A1F3αfμ2, then BZa=0. Thus,
BY=A4s1Z3+A1rF3+A3b−A2,=A1(F3αfs1Z3μ2+rF3+βF3(b−1)γ); |
and since b<1, we have that γ(r+αfs1Z3μ2)1−b<β and
BF=A1r+A1F3r+A4s2Z3,=A1(r+F3r+αfF3s2Z3μ2),=A1(2r−αfZ3a+αfZ3a), |
and
B∗=−A1F3r+A5μ2Z3a+A4λz+A4pZ3,=−A1F3r+A1αfF3μ2(μ2Z3a+λz+pZ3),=−A1F3r+A1F3αfμ2(2λz),=A1F3(−r+2λzαfμ2); |
L′2≤0 if r>2λzαfμ2. Hence, E3 is globally asymptotically stable.
Finding the solutions of the system helps to understand the dynamics of the solutions. Some researchers have found numerical solutions for biological systems by using Galerkin meshless method [23] or traveling wave solutions [24]. Since there are no initial conditions available, the best way is to apply an analytical method which does not require initial or boundary conditions. We shall the use tanh-expansion method to find the solutions [25]. The following are the steps to construct the solution:
(1) Transfer the system of PDEs given by (6) into the system ODEs given by (7) using a traveling wave transformation, which is defined by ξ=kx+ct+ξ0.
(2) Assume that
ˆf=η1u(ξ),ˆy=η2u(ξ),ˆv=η3u(ξ),ˆz=η4u(ξ),^za=η5u(ξ), | (14) |
and substituting (14) into the equation of System (7) gives a polynomial of u and its derivatives:
P(u,u′,u′′,...)=0. | (15) |
(3) Assume that
u(ξ)=S(Φ)=M∑i=0aiΦi, | (16) |
where M is a positive integer and
Φ=tanh(μξ), | (17) |
where μ and ai are constants such that
dudξ=dS(Φ)dΦ=μ(1−Φ2)M∑i=0aidΦidΦ,d2udξ2=d2S(Φ)dΦ2=μ2(1−Φ2)(−2ΦM∑i=0aidΦidΦ+(1−Φ2)M∑i=0aid2ΦidΦ2). |
(4) Apply the homogeneous balance theorem to find the value of M, i.e., balance the linear terms of highest order in the previous equation with the highest-order nonlinear terms.
(5) Substitute Eq (16) into the equations of System (15) to obtain an equation of Φi.
(6) Equate the confections of Φi to zero to obtain the ai's and η's. By following Steps (1) and (2), we obtain the following system of equations:
η1ddξu=η1rcu(1−(η1u+η2u))−η1η3βcu2−η1η5αfcu2+η1d1k2cuξξ,η2ddξu=η1η3βcu2−η21cu−η2η5μ1cu2+η2d2k2cuξξ,η3ddξu=η2bcu−η1η3βcu2−η3γcu−η3η5κcu2+η3d3k2cuξξ,η4ddξu=λzc−η4pcu−η2η4s1cu2−η1η4s2cu2+η4d4k2cuξξ,η5ddξu=η2η3s1cu2+η1η3s2cu2−η5μ2cu+η5d5k2cuξξ. | (18) |
Then, we sum all of the equations to obtain a single equation, as follows:
A1u+A2u2+A3u′−k2A4u′′−A5=0, | (19) |
where
A1=η21c−η2bc+η3γc+η4pc+η5μ2c−η1rc,A2=η21rc+η1η2rc+η1η5αfc+η2η5μ1c+η1η3βc+η3η5κc,A3=η1+η2+η3+η4+η5,A4=d1η1+d2η2+d3η3+d4η4+d5η5,A5=−λzc. |
Next, we follow Steps (3) and (4) and balance between the nonlinear term u2 and the highest order of the derivative u′′; we get 2M=4+M−2, which satisfies M=2. Thus,
u(ξ)=S(Φ)=a0+a1Φ+a2Φ2. | (20) |
Substituting Eq (20) into ODE (19) gives
A1S(Φ)+A2S2(Φ)+A3μ(1−Φ2)dS(Φ)dΦ−A4μ2(1−Φ2)(−2ΦdS(Φ)dΦ+(1−Φ2)d2S(Φ)dΦ2)−A5=0. | (21) |
Note that
S=a0+a1Φ+a2Φ2,dSdΦ=a1+2a2Φ,d2SdΦ2=2a2. | (22) |
Substituting Eq (22) into Eq (21) and collecting all of the terms with the same power of Φ together implies the following:
A1(a0+a1Φ+a2Φ2)+A2+(a0+a1Φ+a2Φ2)2+A3(a1+2a2Φ)−A4(2a2)−A5=0; |
this can be rewritten as
b0+b1Φ+b2Φ2+b3Φ3+b4Φ4=0. |
Then, we equate the constant bi's to zero to obtain the algebraic system of equations and find the ai's and η's.
Thus, the solutions are in the following form:
F=η1u(x,t),Y=η2u(x,t),V=η3u(x,ti),Z=η4u(x,t),Za=η5u(x,t), | (23) |
where
u(x,t)=a0+a1tanh(μ(ct+kx)), | (24) |
and
d5=1η25(η5(6.58537d3−36.5854d4)a1cμ+η25(1.6d1−1.17143d2−6.85366d3+7.42509d4)−66.9643d2−423.018d3+489.983d4),η1=−1.6,η2=1.17143η5+66.9643η5,η3=−6.58537a1cμ+6.85366η5+423.018η5,η4=36.5854a1cμ−7.42509η5−489.983η5. |
The presented mathematical model aims to elucidate the effects of combining viral therapy with the immune response and its spread. First, we ignored the diffusion terms and solved the problem by using the Runge-Kutta 4th-order method using the values of the non-dimensionless parameters according to Reference [16], as follows:
r=0.36,β=0.1,αf=0.36,μ1=0.48,b=2,γ=0.2,κ=0.16,,s1=0.6,s2=0.29,p=0.036,λz=0.2,μ2=0.036. |
We considered two cases: (a) μ1=μ2=0.2,s1=0.2,s2=0.6 and (b) μ1=μ2=0.7s1=0.2,s2=0.6, where the initial conditions are F=0.9,Y=0.5,V=0.5,Z=0.1 and Za=0.2.
The results in Figure 2 show that, in Case (a), the unaffected cells F decreases during the treatment when the concentration of activated immune system cells is low. On the other hand, the high concentration of Za helps to maintain the level of healthy cells in the body. Therefore, we predict that the combination of biological therapy and virotherapy reduces the side effects of the virotherapy, and the patient's body may become less weak during the treatment.
The immune response against cancer cells has been investigated for decades. It has been determined that the immune system actively patrols the body [26]. From this standpoint, we investigate the diffusion coefficient of the immune system d5. The solutions of Model (6) are found by employing the tanh-expansion method to study the effect of the treatment on cancer growth. The solution is presented in Eq (24), which indicates that d5 is dependent on d1,d2,d3 and d4. The diffusion of activated immune system cells (d5) increases when the diffusion of the unaffected cells (d1) or naive immune system cells (d4) increases, while it decreases by increasing the diffusion of the virus (d2) or infected cells (d3).
Note that η5 is another parameter that affects the solution, as we can see in Figures 3–5. The parameter η5 is a coefficient that is associated with Za. When η5 increases, the concentrations of uninfected cells F and activated immune system cells Za increase, while the concentrations of infected cells y, virus v and naive immune system cells Z decrease. We observed that the immune cells Z have the highest concentrations compared to other components in the system, and this indicates the response of the immune system for viral treatment. {However, the immunotherapy has been widely used in different protocols to treat cancers [27]. We predict good results of the combination between immune therapy and virotherapy. The results are aligned with the clinical trial in Reference [28]. This clinical trial confirms the possibility of combining the immunotherapy and virotherapy.
In this work, we modified a mathematical model of cancer and virotherapy to study the dynamics of virotherapy with tumor cells and the effects of the immune response. In addition, this modification distinguishes between two types of immune system cells, which are the activated cells and naive cells. The results predict that the high concentration of activated immune cells leads to enhanced results of virotherapy. The activated immune cells can be generated in the patient's body through biological therapy. Moreover, the model was analyzed by using the stability theory of nonlinear systems. We studied the stability of five equilibrium points and determined the conditions of the existence and local and global stability of the equilibrium points. As a result, the success of viral treatment depends on the size of the burst b, the viral infection rate β and the clearance rate of viruses γ, which depend on the type of the virus. The treatment by virotherapy can be more effective by stimulating the activated immune cells. Furthermore, we found the analytical solutions for the studied model by using the tanh-expansion method because of a lack of initial and boundary conditions. We found that, if the concentration of activated immune cells Za increases, the unaffected cells f increase, which indicates the improvement of the treatment results.
However, for future work, the studied model can be solved numerically by finding the associated initial and boundary conditions based on real data, and by using the Galerkin method [23]. Also, diffusion terms can be added in many biological models to study the spread of the model components and deal with a system of PDEs.
The authors declare that there is no conflict of interest regarding the publication of this paper.
The definitions of b0,b1,b2,b3 and b4 that are related to the characteristic polynomial for E2 are as follows:
b0=(1−b)βF3+γ+μ1Z3a(γ+βF3+κZ3a)(F3μ2pr+F3s2(F3μ2r+αfpZ3))+κZ3a,b1=F33((1−b)βrs2+βμ2rs2+βμ1rs2Z3a)+F24((1−b)βpr+(1−b)βμ2r+(1−b)βμ2s2+αf(1−b)βs2Z3+kμ1rs2Z23a+κμ2rs2Z3a+κrs2Z3a+βpr+αfβps2Z3+γμ2rs2+γrs2+μ2rs2+γμ1rs2Z3a+μ1μ2rs2Z3a+2βμ1μ2rZ3a+αfβμ2s2Z3a+βμ1μ2s2Z3a+αfβμ1s2Z3aZ3)+F3((1−b)βμ2p+κμ2prZ3a+αfκps2Z3aZ3+2kμ1μ2rZ23a+κμ2rZ3a+kμ1μ2s2Z23a+αfκ+μ1s2Z23aZ3a+kμ2s2Z3a(αfZ3a+1)+αfκs2Z3aZ3+γμ2pr+γpr+μ2pr+μ1μ2prZ3a+αμ1ps2Z3aZ3+αfγps2Z3+αfps2Z3+βμ1μ2pZ3a+γμ2r+2γμ1μ2rZ3a+γμ2s2+αγμ2s2Z3aZ3a+γμ1μ2s2Z3a+αfγμ1s2Z3aZ3+αγs2Z3)+κμ1μ2pZ23a+γμ2p+αfμ2pZ3a+γμ1μ2pZ3a,b2=F23((1−b)βr+(1−b)βs2+κrs2Z3a+μ1pr2Z3a+βμ1prZ3a+pr+μ1μ2r2Z3a+βμ2r+γrs2+μ2rs2+μ1rs2Z3a+rs2+βμ1rZ3a+βμ1μ2rZ3a+βμ2s2+βμ1s2Z3a+αfβs2Z4)+F3((1−b)βμ2+(1−b)βp+bβr+κμ1prZ23a+κprZ3a+kμ1rZ23a+κμ1μ2rZ23a+κμ2rZ3a+κμ1s2Z23a+κμ2s2Z3a+αfκs2Z3aZ3+κs2Z3a+βμ2p+γpr+μ2pr+γμ1prZ3a+μ1μ2prZ3a+pr+αfps2Z3+γμ2r+γr+μ2r+γμ1rZ3a+γμ1μ2rZ3a+γμ2s2+γs2+μ2s2+γμ1s2Z3a+μ1μ2s2Z3a+αfμ1s2Z3aZ3+αfγs2Z3+αfs2Z3)+γμ2+αf(1−b)βZ3Z3a+βF33rs2+κμ2pZ3a+κpZ3a+κμ2Z3a+γμ2p+γp+μ2p,b3=F3((1−b)β+βμ2+κrZ3a+κs2Z3a+βp+pr+γr+μ2r+μ1rZ3a+r+γs2+μ2s2+μ1s2Z3a+αfs2Z3+s2+βμ1Z3a)+γμ2+γ+F23(βr+rs2+βs2)+Z3a(γμ1+κμ2+κp+κ+μ1μ2+μ1p)+κμ1Z23a+μ2+γp+μ2p+p,b4=γ+βF3+2F3r+F3s2+κZ3a+μ2+p+αfZ3a+μ1Z3a+1. |
The definitions of a0,a1,a2,a3,a4 and a5 that are related to the characteristic polynomial for E4 are as follows:
a1=μ1Z4a+bY4V4+F4r+μ2+λzZ4+1,a2=(1−b)βF4+Z4a(bμ2Y4V4+γμ1+βF4μ1+F4κr+F4μ2r+F4μ1r+κμ2+κp+κ+μ22+μ1μ2+μ2+μ1p+βμ1V4+αfμ1μ2Z4)+γμ2+γ+βF24r+βF4μ2+F4pr+γF4r+F4μ2r+F4r+μ2+γp+μ2p+p+μ1μ2Z24a,a3=1V4Z4F4(V4(μ1rZ4aλz+μ1μ2rZ4Z4a+αfs2Z4(μ1Z4Z4a−μ2Z4a+λz+Z4)+rY4Z4(bβ+μ1Z4(s1−s2)−bβ(λz+μ2Z4)+μ2rλz+rλz+μ2rZ4)+βV24(Z4(−βμ1Z4a−(1−b)β+μ2(r−β))+(r−β)λz+Z24(s1αf+κ(s1−s2))+bY4(rZ4(μ1Z4a+μ2+1)+rλz+αfs2Z24))+Y4(λz(b(μ1Z4a+μ2+1)+μ1s1V4Z4)+μ2Z4(−μ1Z4a(s1V4−b)+b))+μ2V4λz(μ1Z4a+1)−β(F24rV4Z4(−V4−b)+bμ1s1Y24Z24,a4=1V4Z4−β2(κF4s1Z24V34+αfF24s1Z24V24−bF4λzV24)−β2F4V24(λz+Z4aλzμ1+Z4μ2(1−b)+λzμ2+Z4Z4aμ1μ2)−βF4s1Z4(−((αf+κ)λz)+βY4Z4μ1+(αf+κ)Z4aμ2)V24−βκF4s2Z4(λz+Z4(Z4aμ1+1)−Zaμ2)V24−bαfβF24s2Z24V4+bαfβF4s1Y4Z24V4+αfF4s2Z4λzV4−bβF4s2Y4Z24μ1V4+αfF4s2Z4Z4aλzμ1V4−bβF4λzμ2V4−αfF4s2Z4Z4a(Z4aμ1+1)μ2V4+bαF4s2Y4Z24+bαfF4s2Y4Z4λz+bαfF4s2Y4Z24Z4aμ1−bαfF4s2Y4Z4Z4aμ2+bY4(λz(Z4aμ1+1)μ2+s1Y4Z4μ1(λz−Z4aμ2))+r(βκs1V24Z24F24−βκs2V24Z24F24−βV4(b+βV4)(λz+Z4μ2)F24+bY4λzF4+bβV4Y4λzF4+bs1Y24Z24μ1F4−bs2Y24Z24μ1F4−s2V4Y4Z4λzμ1F4+bY4Z4aλzμ1F4+bY4Z4μ2F4+bβV4Y4Z4μ2F4+βV24λzμ2F4+V4λzμ2F4+bY4λzμ2F4+bY4Z4Z4aμ1μ2F4+s2V4Y4Z4Z4aμ1μ2F4+V4Zaλzμ1μ2F4+s1V4Y4Z4μ1(λz−Z4aμ2)F4),a5=F4V4Z4F4r(−bμ1s2Y24Z4(−μ2Z4a+λz)+bμ1μ2s1Y4Z4a(Y4λz−s1Y4Z4)+βF4κs1V24Z4(λz−μ2Za)−βF4κs2V24Z4(λz−μ2Z4a)−βF4μ2V4λz(b+βV4)+bμ1s1Y24Z4λz+bβμ2V4Y4λz+bμ2Y4λz+F4(−αfbβF4s2V4Z4(λz−μ2Z4a)−bβμ1s2V4Y4Z4(−μ2Z4a+λz)−αfbβμ2s1V4Y4Z4Z4a+αfbs2Y4Z4(μ1Z4a+1)(−μ2Za+λz)−αfβ2F4s1V24Z4(λz−μ2Z4a)+β2μ1μ2s1V24Y4Z4Z4a−βκs2V24Z4(μ1Z4a+1)(−μ2Z4a+λz)−β2(−κμ2s1V34Z4Za+μ1μ2V24Zaλz)+αfbβs1V4Y4Z4λz−β2(μ2V24λz(1−b)+μ1s1Y4Z4+κs1V34Z4λz). |
[1] |
H. Fukuhara, Y. Ino, T. Todo, Oncolytic virus therapy: A new era of cancer treatment at dawn, Cancer Sci., 107 (2016), 1373–1379. https://doi.org/10.1111/cas.13027 doi: 10.1111/cas.13027
![]() |
[2] |
J. P. Tian, The replicability of oncolytic virus: defining conditions in tumor virotherapy, Math. Biosci. Eng., 8 (2011), 841. https://doi.org/10.13005/bbra/947 doi: 10.13005/bbra/947
![]() |
[3] |
S. Kumar, A. Kumar, B. Samet, J. F. Gómez-Aguilar, M. S. Osman, A chaos study of tumor and effector cells in fractional tumor-immune model for cancer treatment, Chaos Soliton. Fract., 141 (2020), 110321. https://doi.org/10.1016/j.chaos.2020.110321 doi: 10.1016/j.chaos.2020.110321
![]() |
[4] |
J. F. Gómez-Aguilar, M. G. López-López, V. M. Alvarado-Martínez, D. Baleanu, H. Khan, Chaos in a cancer model via fractional derivatives with exponential decay and mittag-leffler law, Entropy, 19 (2017), 681. https://doi.org/10.3390/e19120681 doi: 10.3390/e19120681
![]() |
[5] |
Z. Z. Zhang, G. Rahman, J. F. Gómez-Aguilar, J. Torres-Jiménez, Dynamical aspects of a delayed epidemic model with subdivision of susceptible population and control strategies, Chaos Soliton. Fract., 160 (2022), 112194. https://doi.org/10.1016/j.chaos.2022.112194 doi: 10.1016/j.chaos.2022.112194
![]() |
[6] | M. Umar, Z. Sabir, M. A. Z. Raja, J. F. Gómez-Aguilar, F. Amin, M. Shoaib, Neuro-swarm intelligent computing paradigm for nonlinear hiv infection model with CD4+ T-cells, Math. Comput. Simul., 188 (2021), 241–253. |
[7] |
R. A. Alharbey, N. H. Aljahdaly, On fractional numerical simulation of hiv infection for CD8+ T-cells and its treatment, Plos One, 17 (2022), e0265627. https://doi.org/10.1371/journal.pone.0265627 doi: 10.1371/journal.pone.0265627
![]() |
[8] |
N. H. Aljahdaly R. A. Alharbey, Fractional numerical simulation of mathematical model of HIV-1 infection with stem cell therapy, AIMS Math., 6 (2021), 6715–6726. https://doi.org/10.3934/math.2021395 doi: 10.3934/math.2021395
![]() |
[9] | N. H. Aljahdaly, H. A. Ashi, Exponential time differencing method for studying prey-predator dynamic during mating period, Comput. Math. Method. M., 2021 (2021). |
[10] |
D. Wodarz, Gene therapy for killing p53-negative cancer cells: Use of replicating versus nonreplicating agents, Hum. Gene Ther., 14 (2003), 153–159. https://doi.org/10.1089/104303403321070847 doi: 10.1089/104303403321070847
![]() |
[11] | Ž. Bajzer, T. Carr, K. Josić, S. J. Russell, D. Dingli, Modeling of cancer virotherapy with recombinant measles viruses, J. Theor. Biol., 252 (2008), 109–122. |
[12] | G. Marelli, A. Howells, N. R. Lemoine, Y. H. Wang, Oncolytic viral therapy and the immune system: A double-edged sword against cancer, Front. Immunol., 9 (2018), 866. |
[13] | N. L. Komarova, D. Wodarz, Targeted cancer treatment in silico, Model. Simul. Sci. Eng. Technol., Springer, 2014. |
[14] | D. Wodarz, Viruses as antitumor weapons: Defining conditions for tumor remission, Cancer Res., 61 (2001), 3501–3507. |
[15] |
D. Wodarz, N. Komarova, Towards predictive computational models of oncolytic virus therapy: Basis for experimental validation and model selection, Plos One, 4 (2009), e4271. https://doi.org/10.1371/journal.pone.0004271 doi: 10.1371/journal.pone.0004271
![]() |
[16] | T. A. Phan, J. P. Tian, The role of the innate immune system in oncolytic virotherapy, Comput. Math. Method. M., 2017 (2017). |
[17] | N. Al-Johani, E. Simbawa, S. Al-Tuwairqi, Modeling the spatiotemporal dynamics of virotherapy and immune response as a treatment for cancer, Commun. Math. Biol. Neurosci., 2019 (2019). |
[18] | E. Simbawa, N. Al-Johani, S. Al-Tuwairqi, Modeling the spatiotemporal dynamics of oncolytic viruses and radiotherapy as a treatment for cancer, Comput. Math. Method. M., 2020 (2020). |
[19] | S. M. Al-Tuwairqi, N. O. Al-Johani, E. A. Simbawa, Modeling dynamics of cancer virotherapy with immune response, Adv. Differ. Equ., 2020 (2020), 1–26. |
[20] | P. M. Ngina, R. W. Mbogo, L. S. Luboobi, et al., Mathematical modelling of in-vivo dynamics of HIV subject to the influence of the CD8+ T-cells, Appl. Math., 8 (2017), 1153. |
[21] | L. Edelstein-Keshet, Mathematical models in biology, SIAM, 2005. |
[22] | M. Martcheva, Analysis of complex ode epidemic models: Global stability, In An Introduction to Mathematical Epidemiology, Springer, 2015,149–181. |
[23] | H. Jahanshahi, K. Shanazari, M. Mesrizadeh, S. Soradi-Zeid, J. F. Gómez-Aguilar, Numerical analysis of galerkin meshless method for parabolic equations of tumor angiogenesis problem, Eur. Phys. J. Plus, 135 (2020), 1–23. |
[24] |
R. A. M. Attia, J. Tian, D. Lu, J. F. Gómez-Aguilar, M. M. A. Khater, Unstable novel and accurate soliton wave solutions of the nonlinear biological population model, Arab J. Basic Appl. Sci., 29 (2022), 19–25. https://doi.org/10.1080/25765299.2021.2024652 doi: 10.1080/25765299.2021.2024652
![]() |
[25] |
A. M. Wazwaz, The tanh method for traveling wave solutions of nonlinear equations, Appl. Math. Comput., 154 (2004), 713–723. https://doi.org/10.1155/S1073792804132157 doi: 10.1155/S1073792804132157
![]() |
[26] | I. H. Sahin, G. Askan, Z. I. Hu, E. M. O. Reilly, Immunotherapy in pancreatic ductal adenocarcinoma: An emerging entity? Ann. Oncol., 28 (2017), 2950–2961. https://doi.org/10.1093/annonc/mdx503 |
[27] |
O. Nave, M. Sigron, A mathematical model for the treatment of melanoma with the BRAF/MEK inhibitor and Anti-PD-1, Appl. Sci., 12 (2022), 12474. https://doi.org/10.3390/app122312474 doi: 10.3390/app122312474
![]() |
[28] | E. Oh, J. E. Oh, J. W. Hong, Y. H. Chung, Y. Lee, K. D. Park, et al., Optimized biodegradable polymeric reservoir-mediated local and sustained co-delivery of dendritic cells and oncolytic adenovirus co-expressing IL-12 and GM-CSF for cancer immunotherapy, J. Control. Release, 259 (2017), 115–127. |
Parameters | Description |
c | Carrying capacity of the tumor cells |
r | Tumor growth rate |
λz | Stimulation rate of the immune response |
b | Burst size of the virus |
αf | Rate of immune-mediated uninfected tumor cell death |
δ | Death rate of infected tumor cells |
β | Infection rate of the virus |
p | Clearance rate of the immune response |
κ | Rate of immune-mediated virus death |
γ | Clearance rate of the virus |
μ1 | Rate of immune-mediated infected tumor cell death |
μ2 | Clearance rate of active immune cells |
s1 | Stimulation rate of the immune response by infected cells |
s2 | Stimulation rate of the immune response by uninfected cells |
Parameters | Description |
c | Carrying capacity of the tumor cells |
r | Tumor growth rate |
λz | Stimulation rate of the immune response |
b | Burst size of the virus |
αf | Rate of immune-mediated uninfected tumor cell death |
δ | Death rate of infected tumor cells |
β | Infection rate of the virus |
p | Clearance rate of the immune response |
κ | Rate of immune-mediated virus death |
γ | Clearance rate of the virus |
μ1 | Rate of immune-mediated infected tumor cell death |
μ2 | Clearance rate of active immune cells |
s1 | Stimulation rate of the immune response by infected cells |
s2 | Stimulation rate of the immune response by uninfected cells |