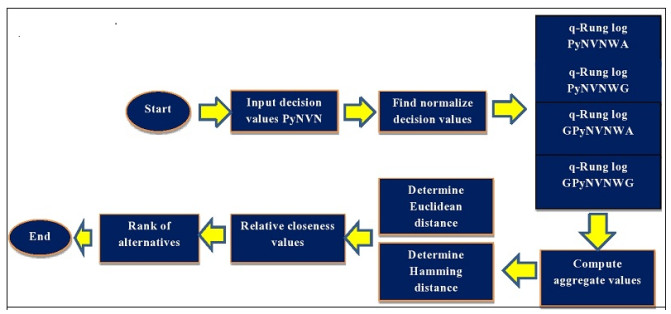
The article explores multiple attribute decision making problems through the use of the Pythagorean neutrosophic vague normal set (PyNVNS). The PyNVNS can be generalized to the Pythagorean neutrosophic interval valued normal set (PyNIVNS) and vague set. This study discusses q-rung log Pythagorean neutrosophic vague normal weighted averaging (q-rung log PyNVNWA), q-rung logarithmic Pythagorean neutrosophic vague normal weighted geometric (q-rung log PyNVNWG), q-rung log generalized Pythagorean neutrosophic vague normal weighted averaging (q-rung log GPyNVNWA), and q-rung log generalized Pythagorean neutrosophic vague normal weighted geometric (q-rung log GPyNVNWG) sets. The properties of q-rung log PyNVNSs are discussed based on algebraic operations. The field of agricultural robotics can be described as a fusion of computer science and machine tool technology. In addition to crop harvesting, other agricultural uses are weeding, aerial photography with seed planting, autonomous robot tractors and soil sterilization robots. This study entailed selecting five types of agricultural robotics at random. There are four types of criteria to consider when choosing a robotics system: robot controller features, cheap off-line programming software, safety codes and manufacturer experience and reputation. By comparing expert judgments with the criteria, this study narrows the options down to the most suitable one. Consequently, q has a significant effect on the results of the models.
Citation: Murugan Palanikumar, Chiranjibe Jana, Biswajit Sarkar, Madhumangal Pal. q-rung logarithmic Pythagorean neutrosophic vague normal aggregating operators and their applications in agricultural robotics[J]. AIMS Mathematics, 2023, 8(12): 30209-30243. doi: 10.3934/math.20231544
[1] | Sumbal Ali, Asad Ali, Ahmad Bin Azim, Ahmad ALoqaily, Nabil Mlaiki . Averaging aggregation operators under the environment of q-rung orthopair picture fuzzy soft sets and their applications in MADM problems. AIMS Mathematics, 2023, 8(4): 9027-9053. doi: 10.3934/math.2023452 |
[2] | Dilshad Alghazzwi, Arshad Ali, Ahmad Almutlg, E. A. Abo-Tabl, A. A. Azzam . A novel structure of q-rung orthopair fuzzy sets in ring theory. AIMS Mathematics, 2023, 8(4): 8365-8385. doi: 10.3934/math.2023422 |
[3] | Attaullah, Shahzaib Ashraf, Noor Rehman, Asghar Khan, Muhammad Naeem, Choonkil Park . Improved VIKOR methodology based on q-rung orthopair hesitant fuzzy rough aggregation information: application in multi expert decision making. AIMS Mathematics, 2022, 7(5): 9524-9548. doi: 10.3934/math.2022530 |
[4] | Attaullah, Shahzaib Ashraf, Noor Rehman, Asghar Khan, Choonkil Park . A decision making algorithm for wind power plant based on q-rung orthopair hesitant fuzzy rough aggregation information and TOPSIS. AIMS Mathematics, 2022, 7(4): 5241-5274. doi: 10.3934/math.2022292 |
[5] | Jian Qi . Artificial intelligence-based intelligent computing using circular q-rung orthopair fuzzy information aggregation. AIMS Mathematics, 2025, 10(2): 3062-3094. doi: 10.3934/math.2025143 |
[6] | Yasir Yasin, Muhammad Riaz, Kholood Alsager . Synergy of machine learning and the Einstein Choquet integral with LOPCOW and fuzzy measures for sustainable solid waste management. AIMS Mathematics, 2025, 10(1): 460-498. doi: 10.3934/math.2025022 |
[7] | Hafiz Muhammad Athar Farid, Muhammad Riaz, Muhammad Jabir Khan, Poom Kumam, Kanokwan Sitthithakerngkiet . Sustainable thermal power equipment supplier selection by Einstein prioritized linear Diophantine fuzzy aggregation operators. AIMS Mathematics, 2022, 7(6): 11201-11242. doi: 10.3934/math.2022627 |
[8] | Muhammad Riaz, Hafiz Muhammad Athar Farid, Hafiz Muhammad Shakeel, Muhammad Aslam, Sara Hassan Mohamed . Innovative q-rung orthopair fuzzy prioritized aggregation operators based on priority degrees with application to sustainable energy planning: A case study of Gwadar. AIMS Mathematics, 2021, 6(11): 12795-12831. doi: 10.3934/math.2021739 |
[9] | Hanan Alohali, Muhammad Bilal Khan, Jorge E. Macías-Díaz, Fahad Sikander . On (p,q)-fractional linear Diophantine fuzzy sets and their applications via MADM approach. AIMS Mathematics, 2024, 9(12): 35503-35532. doi: 10.3934/math.20241685 |
[10] | Ghous Ali, Kholood Alsager, Asad Ali . Novel linguistic q-rung orthopair fuzzy Aczel-Alsina aggregation operators for group decision-making with applications. AIMS Mathematics, 2024, 9(11): 32328-32365. doi: 10.3934/math.20241551 |
The article explores multiple attribute decision making problems through the use of the Pythagorean neutrosophic vague normal set (PyNVNS). The PyNVNS can be generalized to the Pythagorean neutrosophic interval valued normal set (PyNIVNS) and vague set. This study discusses q-rung log Pythagorean neutrosophic vague normal weighted averaging (q-rung log PyNVNWA), q-rung logarithmic Pythagorean neutrosophic vague normal weighted geometric (q-rung log PyNVNWG), q-rung log generalized Pythagorean neutrosophic vague normal weighted averaging (q-rung log GPyNVNWA), and q-rung log generalized Pythagorean neutrosophic vague normal weighted geometric (q-rung log GPyNVNWG) sets. The properties of q-rung log PyNVNSs are discussed based on algebraic operations. The field of agricultural robotics can be described as a fusion of computer science and machine tool technology. In addition to crop harvesting, other agricultural uses are weeding, aerial photography with seed planting, autonomous robot tractors and soil sterilization robots. This study entailed selecting five types of agricultural robotics at random. There are four types of criteria to consider when choosing a robotics system: robot controller features, cheap off-line programming software, safety codes and manufacturer experience and reputation. By comparing expert judgments with the criteria, this study narrows the options down to the most suitable one. Consequently, q has a significant effect on the results of the models.
The complexity of real-world systems makes it challenging for decision makers to select the most appropriate option among the various options available. Even though condensing is difficult, it is not impossible. It proves difficult for many businesses to set goals, incentive systems, and viewpoint restrictions. In decision making (DM), therefore, multiple objectives must be considered at the same time. Multiple-attribute DM (MADM) is the process of choosing the most appropriate option from many possibilities. The MADM team deals with a wide range of MADM problems on a daily basis. As a result, researchers need to improve their DM skills. DM problems are of interest to a number of researchers. Real life systems often present MADM problems due to their complexity, consequently introducing uncertainty to the evaluation information. The possibility that artificial intelligence (AI) could contribute significantly to addressing the grand social challenges of the future has been emphasized by Kaplan and Haenlein [1]. Margetts and Dorobantu [2] have explained the concept of major economies promoting AI research and development with substantial policy interventions. It has been argued that the key technical subsystems that define the current AI paradigm include machine learning, neural networks, natural language processing, smart robots, knowledge graphs and expert systems [3,4]. As well as ethical concerns, AI negatively impacts democracy and the labor market, which is a risk to society. The assessment of these risks and opportunities requires interdisciplinary technology assessment (TA). AI is perceived as a general-purpose technology according to a number of studies. Online platforms not only provide profit from deep learning technology, they also provide highly effective social governance tools. Furthermore, AI's potential to transform society continues to increase with its application in specific economic and financial areas [5,6]. To better understand the potential impacts and necessary governance of AI, this study intends to identify critical AI research topics from a TA perspective. It can be challenging for decision-makers to decide what action to take as real-world systems continue to evolve. Despite the difficulty, it is possible to reduce many goals to one. Several businesses have found it difficult to restrict people's goals, motivations, and viewpoints. Multiple goals must be considered simultaneously when people or committees make decisions. The decision-makers are unable to select the most practical course of action based on this view. The best option is therefore identified by decision makers using more practical and reliable methods. An efficient interactive DM framework for robotic applications has been discussed by Agostini et al. [7].
Many authors have contributed to this field of study by using different techniques. As a result of the uncertainties the following sets have been developed: fuzzy set (FS) [8], intuitionistic FS (IFS) [9], interval valued FS (IVFS) [10], vague set (VS) [11], Pythagorean FS (PyFS) [12], Pythagorean IVFS (PyIVFS) [13] an aggregation operator (AO), spherical FS (SFS) [14], and neutrosophic FS (NFS) [15]. Xu [16] discussed the concept of regression prediction for fuzzy time series. The membership grade (MG) in a set consists of degrees of belongingness that lie between 0 and 1. As a result, Atanassov [9] introduced the concept of an IFS and the condition that the sum of MG with a non-membership grade (NMG) is less than one. The DM approach sometimes generates a single problem when the sum of the MGs and NMGs exceeds one. The concept of the PyFS was developed by Yager [12] to generalize the IFS by ensuring that the square total of its MG and NMG does not exceed one. Expanding the scope of the PyFS, Yager [17] developed the q-rung orthopair FS (q-ROFS). In terms of their outcomes, the MG and NMG were constrained within [0,1]. By definition, the q-ROFSs are extensions of IFSs and PyFSs: if q = 1, they transform into IFSs, and if q = 2, they transform into PyFSs. The concept of q-rung orthopair IVFSs and their properties were discussed by Joshi et al. [18]. A generalized orthopair FS was introduced by Yager [19]. They proved that every PyFS is a q-ROFS, but the converse is not true. For an example, (0.8)2+(0.75)2=1.2025>1 and (0.8)3+(0.75)3=0.933875≤1. Habib et al. [20] used an improved possibilistic programming approach for supply chain networking. Sarkar et al. [21] applied an advanced approach of metaheuristic approaches for reverse logistics management. Besides other studies [22,23] have been found to not apply a fuzzy or uncertainty concept to find the optimum solutions.
According to these hypotheses, the neutral state cannot be demonstrated (neither favor nor disfavor). Cuong and Kreinovich [24] proposed the notion of a picture FS, and they used three pointers; positive, neutral and negative, with a total of not more than one grade. Moreover, it has more advantages than the IFS and PyFS for a few applications [25,26,27] in terms of supporting the use of these sets for DM. Owing to Liu et al. [28], the idea of a generalized PyFS with an AO was introduced and its applications were described. The characteristics of PyIVFSs with AOs were described by Rahman [29,30] and Yang [31]. DM challenge a one problem, where the sum of the truth MG (TMG), the interminacy MG (IMG) and falsity MG (FMG) is greater than one. Thus, Ashraf et al. [14] proposed an SFS with a square total of TMG, IMG, and FMG less than one. According to Fatmaa and Cengiza [32], SFS could be conceptualized by using the technique for order of preference by similarity to ideal solution (TOPSIS) approach. A study, conducted by Liu et al. [33], examined the topic of particular types of q-rung picture FSs with an AO for DM in 2020. The q-ROFS is a generalized orthopair FS which quantifies vague information comprehensively. Yang et al. [34] introduced the notion of q-rung orthopair normal fuzzy AOs and their application in MADM. As a result of Yager [19], the concept of q-ROFSs emerged as the most significant generalization of PyFS. A q-ROFS must contain the sum of the MG's qth power and NMG's qth power within the unit interval [0,1], and when rung q increases, the orthopair's range satisfies the boundary restriction. In this sense a q-ROFS is more powerful and useful than IFSs and PyFSs because they are special cases of the q-ROFS. The basic properties of q-ROFSs have been described by Yager and Alajlan [35]. The concept of orbits was proposed by Ali [36] for another view of q-ROFSs. A number of concepts have been proposed by Liu and Wang [37], including q-ROF weighted averaging (q-ROFWA) and q-rung orthopair fuzzy weighted geometric (q-ROFWG). Liu and Liu [38] combined Bonferroni means (BMs) with the q-ROFS to study the q-rung orthopair fuzzy BM operators and geometric BMs with their desirable properties. Jana et al. [39] initiated the q-rung orthopair fuzzy Dombi averaging and geometric AOs. Wang et al. [40] explored the combined concept of Muirhead mean (MM) operators and the q-ROFS to obtain new AOs that are q-rung orthopair fuzzy MM operators. An idea of q-rung orthopair normal FSs was presented by [41], wherein its operational laws and a score function were defined. In addition to those operators, they initiated some aggregation operations for the same concept, known as q-rung orthopair neutrosophic fuzzy weighted averaging (q-RONFWA) and q-rung orthopair neutrosophic fuzzy orhopair weighted averaging (q-RONFOWG). A further discussion of hesitant q-ROFWA and hesitant q-ROFWG operators was presented by Hussain et al. [42]. The generalized and group generalized averaging operations using the q-rung orthopair fuzzy information were proposed by Hussain et al. [43].
Recently, neutrosophic logic and sets theory were introduced. Neutrosophy refers to the knowledge of neutral thought, and that neutrality is the main difference between an FS and IFS. The concept of a neutrosophic set (NSS) was introduced by Smarandache [44]. A degree of truth, an indeterminacy degree and a falsity degree were assigned to each proposition in this logic. An NSS is a set in which every element of the universe has a degree of truth, indeterminacy and falsity, respectively, between 0 and 1. Philosophically, the NSS generalizes a classical set, an FS and an IVFS. A method based on AOs for multi-criteria DM (MCDM) under interval neutrosophic conditions was presented by Ye [45]. A discussion of interval-valued neutrosophic set in MCDM problems was presented by Zhang et al. [46]. The VS was introduced by Biswas [11]. In VS, two functions are defined, namely, a TMG tv and an FMG fv, with tv(x) denoting the TMG of x derived from the evidence for x, and fv(x) denoting the FMG of x derived from the evidence against x, as well as tv(x) and fv(x) belonging to [0,1] and the sum is not exceed one. There are several recognized applications of IVFSs and FSs, which are extensions of a VS [47,48,49]. As suggested by Zhang and Xu [50], a PyFS should be expanded to include MCDM by using TOPSIS. Hwang and Yoon [51] evaluated MADM practical problems in their discussion. Jana and Pal [52] investigated the generalization of the bipolar fuzzy soft set (BFSS) with applications. Jana [53] developed an approach to DM based on an extended bipolar FS with multi-attributive border approximation area comparison. A novel approach for robust single-valued NS AOs was developed by Jana and Pal [54] for BFSS. In a study by Jana et al. [55], the PyFS was implemented with DOMBI AOs. According to Ullah et al. [56], distance measuring in complex PyFSs can be applied to practical pattern recognition applications. Under the conditions of MADM, Jana et al. [57] introduced AOs that were derived from trapezoidal NS algorithms. According to Jana and Pal [58], MCDM can be realized by combining the NS with DOMBI power AOs. A study of MADM spherical vague normal operators was conducted by Palanikumar et al. [59], who evaluated and their applications in farmer selection. Recently, Ulucay [60,61] and Ulucay et al. [62] discussed the concept of the generalization of neutrosophic soft set and its various applications. The authors of [63,64] studied neutrosophic applications. Lu et al. [65] discussed the concept of consensus progress for group DM in social networks with incomplete probabilistic hesitant fuzzy information. Lu et al. [66] combined the concept of social network clustering and consensus-based distrust behavioral management for large scale group DM with incomplete hesitant fuzzy preference relations.
Recently, Jana et al. [67] described the MCDM technique by using single valued triangular neutrosophic Dombi AOs. Palanikumar et al. [68] presented the idea of a Pythogorean interval-valued neutrosophic normal set (PyIVNNS) with AOs. A significant role is played by the AOs in the solution of MADM problems. Under the conditions of PyFS weighted, ordered weighted and weighted power circumstances, Yager [12] presented some geometric and averaging AOs. In a subsequent study, Peng and Yuan [69] examined several fundamental features of PyFSs based on AOs. By using AOs, this study obtains q-rung log Pythagorean neutrosophic vague normal set (PyNVNS) information. The remainder of this paper is organized as follows. The Pythagorean neutrosophic set and VS information is described in Section 2. The definition and some operations of q-rung log PyNVNSs are provided in Section 3. This study discusses the relation between q-rung log PyNVNS and neutrosophic fuzzy numbers (NFNs). Therefore, H1=([logTH1,log(1−FH1)], [logIH1,logIH1],[logFH1,log(1−TH1)])= ([1,1],[1,1],[0,0]) and H2=([logTH2,log(1−FH2)],[logIH2,logIH2], [logFH2,log(1−TH2)])=([1,1],[1,1],[0,0]) which is known as the distance between q-rung log Pythagorean neutrosophic vague normal numbers (PyNVNNs), is transformed to the distance between NFNs. Section 4 discusses MADM from the perspective of the Hamming distance (HD) and Euclidean distance (ED) with q-rung log PyNVNNs. We demonstrate that the existence an interaction between MADM and AOs for q-rung log PyNVNNs in Section 5. Section 6 discusses an application of q-rung log PyNVNSs, as well as presents the insert algorithm, flowchart and numerical example. The conclusion is provided in Section 7. Accordingly, the paper has the following outcomes:
(ⅰ) A number of algebraic properties of q-rung log PyNVNSs have been established such as associativity, distributivity, and idempotency.
(ⅱ) A q-rung log PyNVN is characterized by HD and ED. The purpose of this method is to calculate the ED between two q-rung log PyNVNNs. In addition, the idea of converting q-rung log PyNVNNs into NFNs discussed.
(ⅲ) The purpose of this study was to demonstrate numerically that MADM and AOs can be applied to real world problems by using the q-rung log PyNVNSs. There is a need to develop an algorithm for q-rung log PyNVNs. Additionally, this study entailed using a q-rung log PyNVNN algorithm to determine a normalized decision matrix based on the response matrix.
(ⅳ) It is imperative that an ideal value can be determined for each of the concepts referenced in the q-rung log Pythagorean neutrosophic vague normal weighted averaging (PyNVNWA), q-rung log Pythagorean neutrosophic vague normal weighted geometric (PyNVNWG), q-rung log generalized PyNVNWA (GPyNVNWA), and q-rung log generalized PyNVNWG (GPyNVNWG).
(ⅴ) As a result, the decision maker can select the ranking results based on their preference by referring to the q-rung log PyNVNWA, q-rung log PyNVNWG, q-rung log GPyNVNWA, and q-rung log GPyNVNWG operators in a flexible manner.
(ⅵ) It is observed that some examples are examined in order to assess the validity of the proposed models.
(ⅶ) Results of DM are found based on q.
This section reviews the concepts of the PyFS and VS.
Definition 2.1. Let U be the universal set. The PyFS H={ε,⟨τTH(ε),τFH(ε)⟩|ε∈U}, where τTH:U→[0,1] and τFH:U→[0,1] denote the MG and NMG of ε∈U to H, respectively and 0⪯(τTH(ε))2+(τFH(ε))2⪯1. For convenience, H=⟨τTH,τFH⟩ is called the Pythagorean fuzzy number [12].
Definition 2.2. The q-rung FS H={ε,⟨τTH(ε),τFH(ε)⟩|ε∈U}, where τTH:U→[0,1] and τFH:U→[0,1] denote the MG and NMG of ε∈U to H, respectively and 0⪯(τTH(ε))q+(τFH(ε))q⪯1, where q⪰1. The degree of indeterminacy π(ε)=((τTH(ε))q+(τFH(ε))q−(τTH(ε))q(τFH(ε))q)1/q. For convenience, H=⟨τTH,τFH⟩ is called the q-rung fuzzy number [19].
Definition 2.3. The PyIVFS H={ε,⟨~τTH(ε),~τFH(ε)⟩|ε∈U}, where ~τTH:U→Int([0,1]) and ~τFH:U→Int([0,1]) denote the MG and NMG of ε∈U to H, respectively, and 0⪯(τT+H(ε))2+(τF+H(ε))2⪯1. For convenience, H=⟨[τT−H,τT+H],[τF−H,τF+H]⟩ is called the Pythagorean interval-valued fuzzy number [13].
Definition 2.4. The q-rung IVFS H={ε,⟨~τTH(ε),~τFH(ε)⟩|ε∈U}, where ~τTH:U→Int([0,1]) and ~τFH:U→Int([0,1]) denote the MG and NMG of ε∈U to H, respectively, and 0⪯(τT+H(ε))q+(τF+H(ε))q⪯1. For convenience, H=⟨[τT−H,τT+H],[τF−H,τF+H]⟩ is called the q-rung interval-valued fuzzy number [18].
Definition 2.5. The Pythagorean neutrosophic set H={ε,⟨τTH(ε),τIH(ε),τFH(ε)⟩|ε∈U}, where τTH:U→[0,1], τIH:U→[0,1] and τFH:U→[0,1] denote the TMG, IMG and FMG of ε∈U to H, respectively and 0⪯(τTH(ε))2+(τIH(ε))2+(τFH(ε))2⪯2. For convenience, H=⟨τTH,τIH,τFH⟩ is called the Pythagorean neutrosophic fuzzy number [68].
Definition 2.6. (ⅰ) A VS H in U is a pair (TH,FH), where TH:U→[0,1] and FH:U→[0,1] are mappings such that TH(ε)+FH(ε)⪯1,∀ε∈U; additionally, TH and FH denotes the truth and false membership function respectively.
(ⅱ) H(ε)=[TH(ε),1−FH(ε)] is called the vague value of ε in H [11].
Definition 2.7. (ⅰ) A VS H is contained in VS H1 and H⊆H1 if and only if H(ε)⪯H1(ε). That is, TH(ε)⪯TH1(ε) and 1−FH(ε)⪯1−FH1(ε),∀ε∈U.
(ⅱ) The union of two VSs H and H1 and X=H∪H1, TX=max{TH,TH1} and 1−FX=max{1−FH,1−FH1}=1−min{FH,FH1}.
(ⅲ) The intersection of two VSs H and H1 and X=H∩H1, TX=min{TH,TH1} and 1−FX=min{1−FH,1−FH1}=1−max{FH,FH1} [11].
Definition 2.8. Consider a VS H of a set U,∀ε∈U. Then
(ⅰ) TH(ε)=0 and FH(ε)=1 constitute a zero VS of U.
(ⅱ) TH(ε)=1 and FH(ε)=0 constitute a unit VS of U [11].
Definition 2.9. Let R be the set of real numbers. The membership function of fuzzy number M(x)=exp−(x−Γ)2δ2,(δ>0) is called the NFN M=(Γ,δ), where N is an NFN set [41].
Definition 2.10. Let L1=(κ1,δ1)∈N and L2=(κ2,δ2)∈N, (δ1,δ2>0). Then the distance between L1 and L2 is defined as D(L1,L2)=√(κ1−κ2)2+12(δ1−δ2)2, where N is an NFN set [16].
Several intriguing fundamental operations are described for the q-rung log PyNVNN.
Definition 3.1. The q-rung log Pythagorean neutrosophic VS H={ε,⟨[logTH(ε),log(1−FH(ε))], [logIH(ε),logIH(ε)],[logFH(ε),log(1−TH(ε))]⟩|ε∈U}, ~τTH:U→Int([0,1]), ~τIH:U→Int([0,1]) and ~τFH:U→Int([0,1]) denote the TMG, IMG and FMG of ε∈U to H, respectively and 0⪯(logΓi1−FH(ε))q+(logΓiIH(ε))q+(logΓi1−TH(ε))q⪯2, where Γ=∏[TH,1−FH],[IH,IH],[FH,1−TH]. For convenience, H=⟨[logTH,log(1−FH)],[logIH,logIH],[logFH,log(1−TH)]⟩ is called the q-rung log PyNVN.
Definition 3.2. Let (κ,δ)∈N and H=⟨(Γ,δ);[logTH,log(1−FH)],[logIH,logIH],[logFH,log(1−TH)]⟩ be the q-rung log PyNVNN; TMG, IMG and FMG are defined as [logΓiTH,logΓi(1−FH)]=[logΓiTH⋅exp−(x−κ)2δ2,logΓi(1−FH)⋅exp−(x−κ)2δ2], [logΓiIH,logΓiIH]=[logΓiIH⋅exp−(x−κ)2δ2,logΓiIH⋅exp−(x−κ)2δ2] and [logΓiFH,logΓi(1−TH)]=[1−(1−logΓiFH)⋅exp−(x−κ)2δ2,1−(1−logΓi(1−TH))⋅exp−(x−κ)2δ2] respectively, where x∈X is a non-empty set; also [logTH,log(1−FH)], [logIH,logIH], [logFH,log(1−TH)]∈Int([0,1]) and 0⪯(log(1−FH)(ε))q+(logIH(ε))q+(log(1−TH)(ε))q⪯2, where Γ=∏[TH,1−FH],[IH,IH],[FH,1−TH].
Definition 3.3. Let H=⟨(κ,δ);[logTH,log(1−FH)],[logIH,logIH],[logFH, log(1−TH)]⟩ be the log PyNVNN; the score function of H is defined as S(H)=S1(H)+S2(H)2, −1⪯S(H)⪯1; where
S1(H)=κ2(X2+1−Y2+1−Z2),S2(H)=δ2(X2+1−Y2+1−Z2). |
The accuracy function of H is A(H)=A1(H)+A2(H)2, where 0⪯A(H)⪯1.
A1(H)=κ2(X2+1+Y2+1+Z2),A2(H)=δ2(X2+1+Y2+1+Z2), |
where X=(logΓiTH)2+(logΓi(1−FH))2,Y=(logΓiIH)2+(logΓiIH)2, Z=(logΓiFH)2+(logΓi(1−TH))2.
Definition 3.4. Let H=⟨(κ,δ);[logTH,log(1−FH)],[logIH,logIH],[logFH,log(1−TH)]⟩, H1=⟨(κ1,δ1);[logTH1,log(1−FH1)], [logIH1,logIH1],[logFH1,log(1−TH1)]⟩ and H2=⟨(κ2,δ2);[logTH2,log(1−FH2)],[logIH2,logIH2],[logFH2,log(1−TH2)]⟩ be any three q-rung log PyNVNNs, and real number q>0 and Γ=∏[THi,1−FHi],[IHi,IHi],[FHi,1−THi]. Their corresponding operations are defined as follows:
(ⅰ) H1⊞H2=[(κ1+κ2,δ1+δ2);[2q√(logΓiTH1)2q+(logΓiTH2)2q−(logΓiTH1)2q⋅(logΓiTH2)2q,2q√(logΓi(1−FH1))2q+(logΓi(1−FH2))2q−(logΓi(1−FH1))2q⋅(logΓi(1−FH2))2q],[q√(logΓiIH1)q+(logΓiIH2)q−(logΓiIH1)q⋅(logΓiIH2)q,q√(logΓiIH1)q+(logΓiIH2)q−(logΓiIH1)q⋅(logΓiIH2)q],[logΓiFH1⋅logΓiFH2,logΓi(1−TH1)⋅logΓi(1−TH2)]],
(ⅱ) H1⊠H2=[(κ1⋅κ2,δ1⋅δ2);[logΓiTH1⋅logΓiTH2,logΓi(1−FH1)⋅logΓi(1−FH2)],[q√(logΓiIH1)q+(logΓiIH2)q−(logΓiIH1)q⋅(logΓiIH2)q,q√(logΓiIH1)q+(logΓiIH2)q−(logΓiIH1)q⋅(logΓiIH2)q],[2q√(logΓiFH1)2q+(logΓiFH2)2q−(logΓiFH1)2q⋅(logΓiFH2)2q,2q√(logΓi(1−TH1))2q+(logΓi(1−TH2))2q−(logΓi(1−TH1))2q⋅(logΓi(1−TH2))2q]],
(ⅲ) Θ⋅H=[(Θ⋅κ,Θ⋅δ);[2q√1−(1−(logΓiTH)2q)q,2q√1−(1−(logΓi(1−FH))2q)q],[(logΓiIH)q,(logΓiIH)q],[(logΓiFH)q,(logΓi(1−TH))q]],
(ⅳ) HΘ=[(κΘ,δΘ);[(logΓiTH)q,(logΓi(1−FH))q],[(logΓiIH)q,(logΓiIH)q],[2q√1−(1−(logΓiFH)2q)q,2q√1−(1−(logΓi(1−TH))2q)q]].
Measurements of the ED and HD were conducted and some mathematical characteristics of q-rung log PyNVNNs are examined here.
Definition 4.1. Let H1=⟨(κ1,δ1);[logTH1,log(1−FH1)],[logIH1,logIH1],[logFH1, log(1−TH1)]⟩ and H2=⟨(κ2,δ2);[logTH2,log(1−FH2)],[logIH2,logIH2],[logFH2, log(1−TH2)]⟩ be any two q-rung log PyNVNNs. Then, the ED between H1 and H2 is
DE(H1,H2)=12√[1+(logΓiTH1)2−(logΓiIH1)2−(logΓiFH1)2+1+(logΓi(1−FH1))2−(logΓiIH1)2−(logΓi(1−TH1))22κ1−1+(logΓiTH2)2−(logΓiIH2)2−(logΓiFH2)2+1+(logΓi(1−FH2))2−(logΓiIH2)2−(logΓi(1−TH2))22κ2]2+12[1+(logΓiTH1)2−(logΓiIH1)2−(logΓiFH1)2+1+(logΓi(1−FH1))2−(logΓiIH1)2−(logΓi(1−TH1))22δ1−1+(logΓiTH2)2−(logΓiIH2)2−(logΓiFH2)2+1+(logΓi(1−FH2))2−(logΓiIH2)2−(logΓi(1−TH2))22δ2]2 |
and the HD between H1 and H2 is defined as DH(H1,H2)= 12[|1+(logΓiTH1)2−(logΓiIH1)2−(logΓiFH1)2+1+(logΓi(1−FH1))2−(logΓiIH1)2−(logΓi(1−TH1))22κ1−1+(logΓiTH2)2−(logΓiIH2)2−(logΓiFH2)2+1+(logΓi(1−FH2))2−(logΓiIH2)2−(logΓi(1−TH2))22κ2|+12|1+(logΓiTH1)2−(logΓiIH1)2−(logΓiFH1)2+1+(logΓi(1−FH1))2−(logΓiIH1)2−(logΓi(1−TH1))22δ1−1+(logΓiTH2)2−(logΓiIH2)2−(logΓiFH2)2+1+(logΓi(1−FH2))2−(logΓiIH2)2−(logΓi(1−TH2))22δ2|].
Theorem 4.1. Let H1=⟨(κ1,δ1);[logTH1,log(1−FH1)],[logIH1,logIH1],[logFH1, log(1−TH1)]⟩,H2=⟨(κ2,δ2);[logTH2,log(1−FH2)],[logIH2,logIH2],[logFH2, log(1−TH2)]⟩ and H3=⟨(κ3,δ3);[logTH3,log(1−FH3)],[logIH3,logIH3],[logFH3, log(1−TH3)]⟩ be any three q-rung log PyNVNNs. Then, we have the following:
(ⅰ) DE(H1,H2)=0, if and only if H1=H2.
(ⅱ) DE(H1,H2) = DE(H2,H1).
(ⅲ) DE(H1,H3)⪯DE(H1,H2)+DE(H2,H3).
Proof. Conditions (ⅰ) and (ⅱ) are clear and concise. Condition (ⅲ) remains to be proven. Now, (DE(H1,H2)+DE(H2,H3))2=[12√[1+(logΓiTH1)2−(logΓiIH1)2−(logΓiFH1)2+1+(logΓi(1−FH1))2−(logΓiIH1)2−(logΓi(1−TH1))22κ1−1+(logΓiTH2)2−(logΓiIH2)2−(logΓiFH2)2+1+(logΓi(1−FH2))2−(logΓiIH2)2−(logΓi(1−TH2))22κ2]2+12[1+(logΓiTH1)2−(logΓiIH1)2−(logΓiFH1)2+1+(logΓi(1−FH1))2−(logΓiIH1)2−(logΓi(1−TH1))22δ1−1+(logΓiTH2)2−(logΓiIH2)2−(logΓiFH2)2+1+(logΓi(1−FH2))2−(logΓiIH2)2−(logΓi(1−TH2))22δ2]2+12√[1+(logΓiTH2)2−(logΓiIH2)2−(logΓiFH2)2+1+(logΓi(1−FH2))2−(logΓiIH2)2−(logΓi(1−TH2))22κ2−1+(logΓiTH3)2−(logΓiIH3)2−(logΓiFH3)2+1+(logΓi(1−FH3))2−(logΓiIH3)2−(logΓi(1−TH3))22Γ3]2+12[1+(logΓiTH2)2−(logΓiIH2)2−(logΓiFH2)2+1+(logΓi(1−FH2))2−(logΓiIH2)2−(logΓi(1−TH2))22δ2−1+(logΓiTH3)2−(logΓiIH3)2−(logΓiFH3)2+1+(logΓi(1−FH3))2−(logΓiIH3)2−(logΓi(1−TH3))22δ3]2]2
=14((ω1κ1−ω2κ2)2+12(ω1δ1−ω2δ2)2)+14((ω2κ2−ω3κ3)2+12(ω2δ2−ω3δ3)2)+12(√(ω1κ1−ω2κ2)2+12(ω1δ1−ω2δ2)2×√(ω2κ2−ω3κ3)2+12(ω2δ2−ω3δ3)2)⪰14((ω1κ1−ω2κ2)2+12(ω1δ1−ω2δ2)2)+14((ω2κ2−ω3κ3)2+12(ω2δ2−ω3δ3)2)+12((ω1κ1−ω2κ2)×(ω2κ2−ω3κ3)+12(ω1δ1−ω2δ2)×(ω2δ2−ω3δ3))=14(ω1κ1−ω2κ2+ω2κ2−ω3κ3)2+18(ω1δ1−ω2δ2+ω2δ2−ω3δ3)2=14(ω1κ1−ω3κ3)2+18(ω1δ1−ω3δ3)2=DE(H1,H3)2 |
where
ω1=1+(logΓiTH1)2−(logΓiIH1)2−(logΓiFH1)2+1+(logΓi(1−FH1))2−(logΓiIH1)2−(logΓi(1−TH1))22, |
ω2=1+(logΓiTH2)2−(logΓiIH2)2−(logΓiFH2)2+1+(logΓi(1−FH2))2−(logΓiIH2)2−(logΓi(1−TH2))22, |
ω3=1+(logΓiTH3)2−(logΓiIH3)2−(logΓiFH3)2+1+(logΓi(1−FH3))2−(logΓiIH3)2−(logΓi(1−TH3))22. |
Corollary 4.1. Let H1=⟨(κ1,δ1);[logTH1,log(1−FH1)],[logIH1,logIH1],[logFH1, log(1−TH1)]⟩,H2=⟨(κ2,δ2);[logTH2,log(1−FH2)],[logIH2,logIH2],[logFH2, log(1−TH2)]⟩ and H3=⟨(κ3,δ3);[logTH3,log(1−FH3)],[logIH3,logIH3],[logFH3, log(1−TH3)]⟩ be any three q-rung log PyNVNNs. Then, we have the following
ⅰ. DH(H1,H2)=0 if and only if H1=H2.
ⅱ. DH(H1,H2) = DH(H2,H1).
ⅲ. DH(H1,H3)⪯DH(H1,H2)+DH(H2,H3).
Proof. This proof is based on Theorem 4.1.
Remark 4.1. Note that H1=([logTH1,log(1−FH1)], [logIH1,logIH1],
[logFH1,log(1−TH1)])=([1,1],[1,1],[0,0]) and H2=([logTH2,log(1−FH2)],
[logIH2,logIH2], [logFH2,log(1−TH2)])=([1,1],[1,1],[0,0]) which is known as the distance between q-rung log PyNVNNs is transformed to the distance between NFNs.
A novel concept is presented in this section and it includes q-rung log PyNVNWA, q-rung log PyNVNWG, q-rung log GPyNVNWA, and q-rung log GPyNVNWG operators in the environment of a q-rung log PyNVNS. Based on the operational rules of q-rung log PyNVNNs, the weighed AOs for q-rung log PyNVNNs are presented.
Definition 5.1. Let Hi=⟨(κi,δi);[logTHi,log(1−FHi)],[logIHi,logIHi],[logFHi, log(1−THi)]⟩ be the family of q-rung log PyNVNNs, Ξ=(Ξ1,Ξ2,...,Ξn) be the weight of Hi where Ξi⪰0 and ⊘ni=1Ξi=1; also, Γ=∏[THi,1−FHi],[IHi,IHi],[FHi,1−THi]. Then, the q-rung log PyNVNWA operator is the q-rung log PyNVNWA operator (H1,H2,...,Hn)=⊘ni=1ΞiHi for i=1,2,...,n.
Theorem 5.1 illustrates the aggregated q-rung log PyNVNWA result based on the above definition.
Theorem 5.1. Let Hi=⟨(κi,δi);[logTHi,log(1−FHi)],[logIHi,logIHi],[logFHi, log(1−THi)]⟩ be the family of q-rung log PyNVNNs. Then, the q-rung log PyNVNWA operator (H1,H2,...,Hn)= (associativity property).
[(⊘ni=1Ξiκi,⊘ni=1Ξiδi);[2q√1−◯ni=1(1−(logΓiTHi)2q)Ξi,2q√1−◯ni=1(1−(logΓi(1−FHi))2q)Ξi],[q√1−◯ni=1(1−(logΓiIHi)q)Ξi,q√1−◯ni=1(1−(logΓiIHi)q)Ξi],[◯ni=1(logΓiFHi)Ξi,◯ni=1(logΓi(1−THi))Ξi]]. |
Proof. Using the induction method, the proof can be established.
If n=2, then the q-rung log PyNVNWA operator (H1,H2)=Ξ1H1⊞Ξ2H2, where
Ξ1H1=[(Ξ1κ1,Ξ1δ1);[2q√1−(1−(logΓiTH1)2q)Ξ1,2q√1−(1−(logΓi(1−FH1))2q)Ξ1],[q√1−(1−(logΓiIH1)q)Ξ1,q√1−(1−(logΓiIH1)q)Ξ1],[(logΓiFH1)Ξ1,(logΓi(1−TH1))Ξ1]] |
and
Ξ2H2=[(Ξ2κ2,Ξ2δ2);[2q√1−(1−(logΓiTH2)2q)Ξ2,2q√1−(1−(logΓi(1−FH2))2q)Ξ2],[q√1−(1−(logΓiIH2)q)Ξ2,q√1−(1−(logΓiIH2)q)Ξ2],[(logΓiFH2)Ξ2,(logΓi(1−TH2))Ξ2]]. |
Hence,
Ξ1H1⊞Ξ2H2=[(Ξ1κ1+Ξ2κ2,Ξ1δ1+Ξ2δ2);[2q√(1−(1−(logΓiTH1)2q)Ξ1)+(1−(1−(logΓiTH2)2q)Ξ2)−(1−(1−(logΓiTH1)2q)Ξ1)⋅(1−(1−(logΓiTH2)2q)Ξ2),2q√(1−(1−(logΓi(1−FH1))2q)Ξ1)+(1−(1−(logΓi(1−FH2))2q)Ξ2)−(1−(1−(logΓi(1−FH1))2q)Ξ1)⋅(1−(1−(logΓi(1−FH2))2q)Ξ2)],[q√(1−(1−(logΓiIH1)q)Ξ1)+(1−(1−(logΓiIH2)q)Ξ2)−(1−(1−(logΓiIH1)q)Ξ1)⋅(1−(1−(logΓiIH2)q)Ξ2),q√(1−(1−(logΓiIH1)q)Ξ1)+(1−(1−(logΓiIH2)q)Ξ2)−(1−(1−(logΓiIH1)q)Ξ1)⋅(1−(1−(logΓiIH2)q)Ξ2)],[(logΓiFH1)Ξ1⋅(logΓiFH2)Ξ2,(logΓi(1−TH1))Ξ1⋅(logΓi(1−TH2))Ξ2]] |
=[(Ξ1κ1+Ξ2κ2,Ξ1δ1+Ξ2δ2);[2q√1−(1−(logΓiTH1)2q)Ξ1⋅(1−(logΓiTH2)2q)Ξ2,2q√1−(1−(logΓi(1−FH1))2q)Ξ1⋅(1−(logΓi(1−FH2))2q)Ξ2],[q√1−(1−(logΓiIH1)q)Ξ1⋅(1−(logΓiIH2)q)Ξ2,q√1−(1−(logΓiIH1)q)Ξ1⋅(1−(logΓiIH2)q)Ξ2],[(logΓiFH1)Ξ1⋅(logΓiFH2)Ξ2,(logΓi(1−TH1))Ξ1⋅(logΓi(1−TH2))Ξ2]]. |
Thus, the q-rung log PyNVNWA operator (H1,H2)=
[(⊘2i=1Ξiκi,⊘2i=1Ξiδi);[2q√1−◯2i=1(1−(logΓiTHi)2q)Ξi,2q√1−◯2i=1(1−(logΓi(1−FHi))2q)Ξi],[q√1−◯2i=1(1−(logΓiIHi)q)Ξi,q√1−◯2i=1(1−(logΓiIHi)q)Ξi],[◯2i=1(logΓiFHi)Ξi,◯2i=1(logΓi(1−THi))Ξi]]. |
It is valid for n=l and l⪰3.
Hence, the q-rung log PyNVNWA operator (H1,H2,...,Hl)=
[(⊘li=1Ξiκi,⊘li=1Ξiδi);[2q√1−◯li=1(1−(logΓiTHi)2q)Ξi,2q√1−◯li=1(1−(logΓi(1−FHi))2q)Ξi],[q√1−◯li=1(1−(logΓiIHi)q)Ξi,q√1−◯li=1(1−(logΓiIHi)q)Ξi],[◯li=1(logΓiFHi)Ξi,◯li=1(logΓi(1−THi))Ξi]]. |
If n=l+1 and we apply q-rung log PyNVNWA operator (H1,H2,...,Hl,Hl+1)
=[(⊘li=1Ξiκi+Ξl+1Γl+1,⊘li=1Ξiδi+Ξl+1δl+1);[2q√⊘li=1(1−(1−(logΓiTHi)2q)Ξi)+(1−(1−(logΓiTHl+1)2q)Ξl+1)−◯li=1(1−(1−(logΓiTHi)2q)Ξi)⋅(1−(1−(logΓiTHl+1)2q)Ξl+1),2q√⊘li=1(1−(1−(logΓi(1−FHi))2q)Ξi)+(1−(1−(logΓi(1−FHl+1))2q)Ξl+1)−◯li=1(1−(1−(logΓi(1−FHi))2q)Ξi)⋅(1−(1−(logΓi(1−FHl+1))2q)Ξl+1)],[q√⊘li=1(1−(1−(logΓiIHi)q)Ξi)+(1−(1−(logΓiIHl+1)q)Ξl+1)−◯li=1(1−(1−(logΓiIHi)q)Ξi)⋅(1−(1−(logΓiIHl+1)q)Ξl+1),q√⊘li=1(1−(1−(logΓiIHi)q)Ξi)+(1−(1−(logΓiIHl+1)q)Ξl+1)−◯li=1(1−(1−(logΓiIHi)q)Ξi)⋅(1−(1−(logΓiIHl+1)q)Ξl+1)],[◯li=1(logΓiFHi)Ξi⋅(logΓiFHl+1)Ξl+1,◯li=1(logΓi(1−THi))Ξi⋅(logΓi(1−THl+1))Ξl+1]] |
=[(⊘l+1i=1Ξiκi,⊘l+1i=1Ξiδi);[2q√1−◯l+1i=1(1−(logΓiTHi)2q)Ξi,2q√1−◯l+1i=1(1−(logΓi(1−FHi))2q)Ξi],[q√1−◯l+1i=1(1−(logΓiIHi)q)Ξi,q√1−◯l+1i=1(1−(logΓiIHi)q)Ξi],[◯l+1i=1(logΓiFHi)Ξi,◯l+1i=1(logΓi(1−THi))Ξi]]. |
Theorem 5.2. (idempotency property) If all Hi=⟨(κi,δi);[logTHi,log(1−FHi)], [logIHi,logIHi][logFHi, log(1−THi)]⟩(i=1,2,...,n) are equal and Hi=H, then the q-rung log PyNVNWA (H1,H2,...,Hn)=H.
Proof. Note that (κi,δi)=(κ,δ), [logTHi,log(1−FHi)]=[logTH,log(1−FH)], [logIHi,logIHi]=[logIH,logIH] and [logFHi,log(1−THi)]=[logFH,log(1−TH)], for i=1,2,...,n and ⊘ni=1Ξi=1.
Now, the q-rung log PyNVNWA operator (H1,H2,...,Hn)
=[(⊘ni=1Ξiκi,⊘ni=1Ξiδi);[2q√1−◯ni=1(1−(logΓiTHi)2q)Ξi,2q√1−◯ni=1(1−(logΓi(1−FHi))2q)Ξi],[q√1−◯ni=1(1−(logΓiIHi)q)Ξi,q√1−◯ni=1(1−(logΓiIHi)q)Ξi],[◯ni=1(logΓiFHi)Ξi,◯ni=1(logΓi(1−THi))Ξi]]=[(κ⊘ni=1Ξi,δ⊘ni=1Ξi);[2q√1−(1−(logΓiTH)2q)⊘ni=1Ξi,2q√1(1−(logΓi(1−FH))2q)⊘ni=1Ξi],[q√1−(1−(logΓiIH)q)⊘ni=1Ξi,q√1(1−(logΓiIH)q)⊘ni=1Ξi],[(logΓiFH)⊘ni=1Ξi,(logΓi(1−TH))⊘ni=1Ξi]]=[(κ,δ);[2q√1−(1−(logΓiTH)2q),2q√1−(1−(logΓi(1−FH))2q)],[q√1−(1−(logΓiIH)q),q√1−(1−(logΓiIH)q)],[(logΓiFH),(logΓi(1−TH))]]=H. |
Theorem 5.3. (boundedness property) Let Hi=⟨(κij,δij);[logTHij,log(1−FHij)], [logIHij,logIHij][logFHij, log(1−THij)]⟩(i=1,2,...,n).(j=1,2,...,ij) be the collection of q-rung log PyNVNWA operators, where κ_=minκij, ¯κ=maxκij, δ_=maxδij, ¯δ=minδij, logΓiTH_=minlogΓiTHij, ¯logΓiTH=maxlogΓiTHij, logΓi(1−FH)_=minlogΓi(1−FHij), ¯logΓi(1−FH)=maxlogΓi(1−FHij), logΓiIH_=minlogΓiIHij, ¯logΓiIH=maxlogΓiIHij, logΓiIH_=minlogΓiIHij, ¯logΓiIH=maxlogΓiIHij, logΓiFH_=minlogΓiFHij, ¯logΓiFH=maxlogΓiFHij, logΓi(1−TH)_=minlogΓi(1−THij), ¯logΓi(1−TH)=maxlogΓi(1−THij).
Then, ⟨(κ_,δ_);[logΓiTH_,logΓi(1−FH)_],[logΓiIH_,logΓiIH_],[¯logΓiFH,¯logΓi(1−TH)]⟩
⪯q−rung logPyNVNWA(H1,H2,...,Hn)⪯⟨(¯κ,¯δ);[¯logΓiTH,¯logΓi(1−FH)],[¯logΓiIH,¯logΓiIH],[logΓiFH_,logΓi(1−TH)_]⟩, |
where 1⪯i⪯n,j=1,2,...,ij.
Proof. Suppose that, logΓiTH_=minlogΓiTHij, ¯logΓiTH=maxlogΓiTHij logΓi(1−FH)_=minlogΓi(1−FHij), ¯logΓi(1−FH)=maxlogΓi(1−FHij), logΓiTH_⪯logΓiTHij⪯¯logΓiTH and logΓi(1−FH)_⪯logΓi(1−FH)ij⪯¯logΓi(1−FH).
Now, logΓiTH_+logΓi(1−FH)_
=2q√1−◯ni=1(1−(logΓiTH_)2q)Ξi+2q√1−◯ni=1(1−(logΓi(1−FH)_)2q)Ξi⪯2q√1−◯ni=1(1−(logΓiTHij)2q)Ξi+2q√1−◯ni=1(1−(logΓi(1−FHij))2q)Ξi⪯2q√1−◯ni=1(1−(¯logΓiTH)2q)Ξi+2q√1−◯ni=1(1−(¯logΓi(1−FH))2q)Ξi=¯logΓiTH+¯logΓi(1−FH). |
Suppose that, logΓiIH_=minlogΓiIHij, ¯logΓiIH=maxlogΓiIHij, logΓiIH_=minlogΓiIHij, ¯logΓiIH=maxlogΓiIHij, logΓiIH_⪯logΓiIHij⪯¯logΓiIH and logΓiIH_⪯logΓiIHij⪯¯logΓiIH. Now,
logΓiIH_+logΓiIH_=q√1−◯ni=1(1−(logΓiIH_)q)Ξi+q√1−◯ni=1(1−(logΓiIH_)q)Ξi⪯q√1−◯ni=1(1−(logΓiIHij)q)Ξi+q√1−◯ni=1(1−(logΓiIHij)q)Ξi⪯q√1−◯ni=1(1−(¯logΓiIH)q)Ξi+q√1−◯ni=1(1−(¯logΓiIH)q)Ξi=¯logΓiIH+¯logΓiIH. |
Suppose that, logΓiFH_=minlogΓiFHij, ¯logΓiFH=maxlogΓiFHij logΓi(1−TH)_=minlogΓi(1−THij), ¯logΓi(1−TH)=maxlogΓi(1−THij), logΓiFH_⪯logΓiFHij⪯¯logΓiFH and logΓi(1−TH)_⪯logΓi(1−THij)⪯¯logΓi(1−TH). Now,
logΓiFH_+logΓi(1−TH)_=◯ni=1(logΓiFH_)Ξi+◯ni=1(logΓi(1−TH)_)Ξi⪯◯ni=1(logΓiFHij)Ξi+◯ni=1(logΓi(1−THij))Ξi⪯◯ni=1(¯logΓiFH)Ξi+◯ni=1(¯logΓi(1−TH))Ξi=¯logΓiFH+¯logΓi(1−TH). |
Suppose that, κ_=minκij, ¯κ=maxκij, δ_=maxδij, ¯δ=minδij, κ_⪯κij⪯¯κ and ¯δ⪯δij⪯δ_.
Hence, ⊘ni=1Ξiκ_⪯⊘ni=1Ξiκij⪯⊘ni=1Ξi¯κ and ⊘ni=1Ξi¯δ⪯⊘ni=1Ξiδij⪯⊘ni=1Ξiδ_.
Therefore,
⊘ni=1Ξiκ_2×[(2q√1−◯ni=1(1−(logΓiTH_)2q)Ξi)2+(2q√1−◯ni=1(1−(logΓi(1−FH)_)2q)Ξi)22+1−(q√1−◯ni=1(1−(logΓiIH_)q)Ξi)2+(q√1−◯ni=1(1−(logΓiIH_)q)Ξi)22+1−(◯ni=1(¯logΓiFH)Ξi)2+(◯ni=1(¯logΓi(1−TH))Ξi)22]⪯⊘ni=1Ξiκij2×[(2q√1−◯ni=1(1−(logΓiTHij)2q)Ξi)2+(2q√1−◯ni=1(1−(logΓi(1−FHij))2q)Ξi)22+1−(q√1−◯ni=1(1−(logΓiIHij)q)Ξi)2+(q√1−◯ni=1(1−(logΓiIHij)q)Ξi)22+1−(◯ni=1(logΓiFHij)Ξi)2+(◯ni=1(logΓi(1−THij))Ξi)22] |
⪯⊘ni=1Ξi¯κ2×[(2q√1−◯ni=1(1−(¯logΓiTH)2q)Ξi)2+(2q√1−◯ni=1(1−(¯logΓi(1−FH))2q)Ξi)22+1−(q√1−◯ni=1(1−(¯logΓiIH)q)Ξi)2+(q√1−◯ni=1(1−(¯logΓiIH)q)Ξi)22+1−(◯ni=1(logΓiFH_)Ξi)2+(◯ni=1(logΓi(1−TH)_)Ξi)22]. |
Hence, ⟨(κ_,δ_);[logTH_,log(1−FH)_],[logIH_,logIH_],[¯logFH,¯log(1−TH)]⟩
⪯q−runglogPyNVNWA(H1,H2,...,Hn)⪯⟨(¯κ,¯δ);[¯logTH,¯log(1−FH)],[¯logIH,¯logIH],[logFH_,log(1−TH)_]⟩. |
Theorem 5.4. (monotonicity property) Let Hi=⟨(κtij,δtij);[logTHtij,log(1−FHtij)], [logIHtij,logIHtij],[logFHtij, log(1−THtij)]⟩ and Ξi=⟨(κhij,δhij); [logTHhij, log(1−FHhij)],[logIHhij,logIHhij], [logFHhij,log(1−THhij)]⟩(i=1,2,...,n),(j=1,2,...,ij) be the families of q-rung log PyNVNWA operators. For any i, Suppose that there is κtij⪯δhij, (logΓiTHtij)2+(logΓi(1−FHtij))2⪯(logΓiTHhij)2+(logΓi(1−FHhij))2 and (logΓiIHtij)2+(logΓiIHtij)2⪰(logΓiIHhij)2+(logΓiIHhij)2, (logΓiFHtij)2+(logΓi(1−TH)tij)2⪰(logΓiFHhij)2+(logΓi(1−THhij))2 or Hi⪯Wi. Then, theq-rung log PyNVNWA operator (H1,H2,...,Hn)⪯q-rung log PyNVNWA operator (W1,W2,...,Wn).
Proof. For any i, κtij⪯δhij. Therefore, ⊘ni=1κtij⪯⊘ni=1δhij.
For any i, (logΓiTHtij)2+(logΓi(1−FHtij))2⪯(logΓiTHhij)2+(logΓi(1−FHhij))2.
Therefore, 1−(logΓiTHti)2+1−(logΓi(1−FHti))2⪰1−(logΓiTHhi)2+1−(logΓi(1−FHhi))2.
Hence, ◯ni=1(1−(logΓiTHti)2)Ξi+◯ni=1(1−(logΓi(1−FHti))2)Ξi⪰ ◯ni=1(1−(logΓiTHhi)2)Ξi+◯ni=1(1−(logΓi(1−FHhi))2)Ξi and 2q√1−◯ni=1(1−(logΓiTHti)2q)Ξi+2q√1−◯ni=1(1−(logΓi(1−FHti))2q)Ξi ⪯2q√1−◯ni=1(1−(logΓiTHhi)2q)Ξi+2q√1−◯ni=1(1−(logΓi(1−FHhi))2q)Ξi.
For any i, (logΓiIHtij)q+(logΓiIHtij)q⪰(logΓiIHhij)q+(logΓiIHhij)q.
Therefore, 1−(logΓiIHti)q+1−(logΓiIHti)q⪯1−(logΓiIHhi)q+1−(logΓiIHhi)q.
Hence, ◯ni=1(1−(logΓiIHti)q)Ξi+◯ni=1(1−(logΓiIHti)q)Ξi⪯◯ni=1(1−(logΓiIHhi)q)Ξi+◯ni=1(1−(logΓiIHhi)q)Ξi implies that q√1−◯ni=1(1−(logΓiIHti)q)Ξi+q√1−◯ni=1(1−(logΓiIHti)q)Ξi⪰q√1−◯ni=1(1−(logΓiIHhi)q)Ξi+q√1−◯ni=1(1−(logΓiIHhi)q)Ξi.
Hence, 1−q√1−◯ni=1(1−(logΓiIHti)q)Ξi+q√1−◯ni=1(1−(logΓiIHti)q)Ξi⪯1−q√1−◯ni=1(1−(logΓiIHhi)q)Ξi+q√1−◯ni=1(1−(logΓiIHhi)q)Ξi.
For any i, (logΓiFHtij)2+(logΓi(1−THtij))2⪰(logΓiFHhij)2+(logΓi(1−THhij))2.
Therefore, 1−(◯ni=1logΓiFHtij)2+(◯ni=1logΓi(1−THtij))22⪯1−(◯ni=1logΓiFHhij)2+(◯ni=1logΓi(1−THhij))22.
⊘ni=1κtij2×[(2q√1−◯ni=1(1−(logΓiTHti)2q)Ξi)2+(2q√1−◯ni=1(1−(logΓi(1−FHti))2q)Ξi)22+1−(q√1−◯ni=1(1−(logΓiIHti)q)Ξi)2+(q√1−◯ni=1(1−(logΓiIHti)q)Ξi)22+1−(◯ni=1(logΓiFHtij))2+(◯ni=1(logΓi(1−THtij)))22] |
⪯⊘ni=1κhij2×[(2q√1−◯ni=1(1−(logΓiTHhi)2q)Ξi)2+(2q√1−◯ni=1(1−(logΓi(1−FHhi))2q)Ξi)22+1−(q√1−◯ni=1(1−(logΓiIHhi)q)Ξi)2+(q√1−◯ni=1(1−(logΓiIHhi)q)Ξi)22+1−(◯ni=1(logΓiFHhij))2+(◯ni=1(logΓi(1−THhij)))22]. |
Hence, the q-rung log PyNVNWA operator (H1,H2,...,Hn)⪯q-rung log PyNVNWA operator (W1,W2,...,Wn).
Definition 5.2. Let Hi=⟨(κi,δi);[logTHi,log(1−FHi)],[logIHi,logIHi],[logFHi, log(1−THi)]⟩ be the family of q-rung log PyNVNNs. Then, the q-rung log PyNVNWG operator is the q-rung log PyNVNWG operator (H1,H2,...,Hn)=◯ni=1HΞii(i=1,2,...,n).
Theorem 5.5. Let Hi=⟨(κi,δi);[logTHi,log(1−FHi)],[logFHi,log(1−THi)]⟩ be the family of q-rung log PyNVNNs. Then, the q -rung log PyNVNWG operator (H_{1}, H_{2}, ..., H_{n}) =
\begin{eqnarray*} \begin{bmatrix} \Big(\bigcirc^{n}_{i = 1} \kappa_{i}^{\Xi_{i}}, \bigcirc^{n}_{i = 1} \delta_{i}^{\Xi_{i}} \Big); \begin{bmatrix} \bigcirc^{n}_{i = 1} (\log_{\Gamma_{i}}{T_{H}}_{i})^{\Xi_{i}}, \bigcirc^{n}_{i = 1}(\log_{\Gamma_{i}}{(1-F_{H}}_{i}))^{\Xi_{i}} \end{bmatrix},\\ \begin{bmatrix} \sqrt[q]{1-\bigcirc^{n}_{i = 1}\Big( 1-(\log_{\Gamma_{i}}{I_{H}}_{i})^{q} \Big)^{\Xi_{i}}}, \sqrt[q]{1-\bigcirc^{n}_{i = 1}\Big( 1-(\log_{\Gamma_{i}}{I_{H}}_{i})^{q} \Big)^{\Xi_{i}}}\,\, \end{bmatrix},\\ \begin{bmatrix} \sqrt[2q]{1-\bigcirc^{n}_{i = 1}\Big( 1-(\log_{\Gamma_{i}}{F_{H}}_{i})^{2q} \Big)^{\Xi_{i}}}, \sqrt[2q]{1-\bigcirc^{n}_{i = 1}\Big( 1-(\log_{\Gamma_{i}}{(1-T_{H}}_{i}))^{2q} \Big)^{\Xi_{i}}}\,\, \end{bmatrix} \end{bmatrix}. \end{eqnarray*} |
Proof. This proof is based on Theorem 5.1.
Theorem 5.6. Suppose that all H_{i} = \Big\langle (\kappa_{i}, \delta_{i}); [\log{T_{H}}_{i}, \log{(1-F_{H}}_{i})], [\log{I_{H}}_{i}, \log{I_{H}}_{i}] [\log{F_{H}}_{i} , \log{(1-T_{H}}_{i})] \Big\rangle are equal and H_{i} = H , for i = 1, 2, ..., n . Then, the q -rung log PyNVNWG operator (H_{1}, H_{2}, ..., H_{n}) = H .
Proof. This proof is based on Theorem 5.2.
Corollary 5.1. The q -rung log PyNVNWG operator is used to satisfy the boundedness and monotonicity properties.
Proof. This proof is based on Theorems 5.3 and 5.4.
As generalizations of the q -rung log PyNVNWA operators, some generalized q -rung log GPyNVNWA operators are developed in Section 5.3.
Definition 5.3. Let H_{i} = \Big\langle (\kappa_{i}, \delta_{i}); [\log{T_{H}}_{i}, \log{(1-F_{H}}_{i})], [\log{I_{H}}_{i}, \log{I_{H}}_{i}], [\log{F_{H}}_{i} , \log{(1-T_{H}}_{i})] \Big\rangle be the family of q -rung log PyNVNN. Then, the q -rung log GPyNVNWA operator (H_{1}, H_{2}, ..., H_{n}) = \Big(\oslash^{n}_{i = 1} \Xi_{i}H_{i}^{\Theta} \Big)^{1/\Theta} is called the q -rung log GPyNVNWA operator.
Theorem 5.7 Illustrates the q -rung log GPyNVNWA result based on the above definition.
Theorem 5.7. Let H_{i} = \Big\langle (\kappa_{i}, \delta_{i}); [\log{T_{H}}_{i}, \log{(1-F_{H}}_{i})], [\log{I_{H}}_{i}, \log{I_{H}}_{i}], [\log{F_{H}}_{i}, \log{(1-T_{H}}_{i})] \Big\rangle be the family of q -rung log PyNVNNs. Then q -rung log GPyNVNWA (H_{1}, H_{2}, ..., H_{n}) = \\
\begin{bmatrix} \Bigg( \Big(\oslash^{n}_{i = 1} \Xi_{i}\kappa_{i}^{\Theta}\Big)^{1/\Theta}, \Big(\oslash^{n}_{i = 1} \Xi_{i}\delta_{i}^{\Theta}\Big)^{1/\Theta}\Bigg);\\ \begin{bmatrix} \Bigg(\sqrt[2q]{ \begin{aligned} 1-\bigcirc^{n}_{i = 1} \Bigg( 1-\Big((\log_{\Gamma_{i}}{T_{H}}_{i})^{q}\Big)^{2q}\Bigg)^{\Xi_{i}}\\ \end{aligned}}\,\,\Bigg)^{1/q}, \Bigg(\sqrt[2q]{ \begin{aligned} 1-\bigcirc^{n}_{i = 1} \Bigg( 1-\Big((\log_{\Gamma_{i}}{(1-F_{H}}_{i}))^{q}\Big)^{2q}\Bigg)^{\Xi_{i}}\\ \end{aligned}}\,\,\Bigg)^{1/q} \end{bmatrix},\\ \begin{bmatrix} \Bigg(\sqrt[q]{ \begin{aligned} 1-\bigcirc^{n}_{i = 1} \Bigg( 1-\Big((\log_{\Gamma_{i}}{I_{H}}_{i})^{q}\Big)^{q}\Bigg)^{\Xi_{i}}\\ \end{aligned}}\,\,\Bigg)^{1/q}, \Bigg(\sqrt[q]{ \begin{aligned} 1-\bigcirc^{n}_{i = 1} \Bigg( 1-\Big((\log_{\Gamma_{i}}{I_{H}}_{i})^{q}\Big)^{q}\Bigg)^{\Xi_{i}}\\ \end{aligned}}\,\,\Bigg)^{1/q} \end{bmatrix},\\ \begin{bmatrix} \sqrt[2q]{1-\Bigg( \begin{aligned} 1-\Bigg(\bigcirc^{n}_{i = 1} \Bigg( \sqrt[2q]{1-\Big(1-(\log_{\Gamma_{i}}{F_{H}}_{i})^{2q}\Big)^{q}}\Bigg)^{\Xi_{i}}\\ \end{aligned}\,\,\Bigg)^{2q}\Bigg)^{1/q}},\\ \sqrt[2q]{1-\Bigg( \begin{aligned} 1-\Bigg(\bigcirc^{n}_{i = 1} \Bigg( \sqrt[2q]{1-\Big(1-(\log_{\Gamma_{i}}{(1-T_{H}}_{i}))^{2q}\Big)^{q}}\Bigg)^{\Xi_{i}}\\ \end{aligned}\,\,\Bigg)^{2q}\Bigg)^{1/q}} \end{bmatrix}\\ \end{bmatrix}. |
Proof. \oslash^{n}_{i = 1} \Xi_{i} H_{i}^{\Theta} = \\ \begin{bmatrix} \Bigg(\Big(\oslash^{n}_{i = 1} \Xi_{i}\kappa_{i}^{\Theta}\Big), \Big(\oslash^{n}_{i = 1} \Xi_{i}\delta_{i}^{\Theta}\Big)\Bigg); \\ \begin{bmatrix} \sqrt[2q]{ \begin{aligned} 1-\bigcirc^{n}_{i = 1} \Bigg(1-\Big((\log_{\Gamma_{i}}{T_{H}}_{i})^{q}\Big)^{2q}\Bigg)^{\Xi_{i}}\\ \end{aligned}}\, \, , \sqrt[2q]{ \begin{aligned} 1-\bigcirc^{n}_{i = 1} \Bigg(1-\Big((\log_{\Gamma_{i}}{(1-F_{H}}_{i}))^{q}\Big)^{2q}\Bigg)^{\Xi_{i}}\\ \end{aligned}} \, \, \end{bmatrix}, \\ \begin{bmatrix} \sqrt[q]{ \begin{aligned} 1-\bigcirc^{n}_{i = 1} \Bigg(1-\Big((\log_{\Gamma_{i}}{I_{H}}_{i})^{q}\Big)^{q}\Bigg)^{\Xi_{i}}\\ \end{aligned}}\, \, , \sqrt[q]{ \begin{aligned} 1-\bigcirc^{n}_{i = 1} \Bigg(1-\Big((\log_{\Gamma_{i}}{I_{H}}_{i})^{q}\Big)^{q}\Bigg)^{\Xi_{i}}\\ \end{aligned}} \, \, \end{bmatrix}, \\ \begin{bmatrix} \begin{aligned} \bigcirc^{n}_{i = 1} \Bigg(\sqrt[2q]{1-\Big(1-(\log_{\Gamma_{i}}{F_{H}}_{i})^{2q}\Big)^{q}}\Bigg)^{\Xi_{i}}\\ \end{aligned}, \begin{aligned} \bigcirc^{n}_{i = 1} \Bigg(\sqrt[2q]{1-\Big(1-(\log_{\Gamma_{i}}{(1-T_{H}}_{i}))^{2q}\Big)^{q}}\Bigg)^{\Xi_{i}}\\ \end{aligned} \end{bmatrix} \end{bmatrix}.
This proof is based on the induction method.
If n = 2 , then
\Xi_{1}H_{1}\boxplus \Xi_{2}H_{2} =
\begin{eqnarray*} &&\begin{bmatrix} \Big(\Xi_{1}\kappa_{1}^{\Theta}+ \Xi_{2}\kappa_{2}^{\Theta}, \Xi_{1}\delta_{1}^{\Theta} + \Xi_{2}\delta_{2}^{\Theta}\Big);\\ \begin{bmatrix} \sqrt[2q]{ \begin{aligned} \Bigg(\sqrt[2q]{ \begin{aligned} 1-\bigg( 1-\Big((\log_{\Gamma_{i}}{T_{H}}_{1})^{q}\Big)^{2q}\bigg)^{\Xi_{1}}\\ \end{aligned}}\,\, \Bigg)^{2q} + \Bigg(\sqrt[2q]{ \begin{aligned} 1-\bigg( 1-\Big((\log_{\Gamma_{i}}{T_{H}}_{2})^{q}\Big)^{2q}\bigg)^{\Xi_{1}}\\ \end{aligned}}\,\, \Bigg)^{2q}, \\ - \Bigg(\sqrt[2q]{ \begin{aligned} 1-\bigg( 1-\Big((\log_{\Gamma_{i}}{T_{H}}_{1})^{q}\Big)^{2q}\bigg)^{\Xi_{1}}\\ \end{aligned}}\,\, \Bigg)^{2q} \cdot \Bigg(\sqrt[2q]{ \begin{aligned} 1-\bigg( 1-\Big((\log_{\Gamma_{i}}{T_{H}}_{2})^{q}\Big)^{2q}\bigg)^{\Xi_{1}}\\ \end{aligned}}\,\, \Bigg)^{2q} \end{aligned}}\\ \sqrt[2q]{ \begin{aligned} \Bigg(\sqrt[2q]{ \begin{aligned} 1-\bigg( 1-\Big((\log_{\Gamma_{i}}{(1-F_{H}}_{1}))^{q}\Big)^{2q}\bigg)^{\Xi_{1}}\\ \end{aligned}}\,\, \Bigg)^{2q} + \Bigg(\sqrt[2q]{ \begin{aligned} 1-\bigg( 1-\Big((\log_{\Gamma_{i}}{(1-F_{H}}_{2}))^{q}\Big)^{2q}\bigg)^{\Xi_{1}}\\ \end{aligned}}\,\, \Bigg)^{2q} \\ - \Bigg(\sqrt[2q]{ \begin{aligned} 1-\bigg( 1-\Big((\log_{\Gamma_{i}}{(1-F_{H}}_{1}))^{q}\Big)^{2q}\bigg)^{\Xi_{1}}\\ \end{aligned}}\,\, \Bigg)^{2q} \cdot \Bigg(\sqrt[2q]{ \begin{aligned} 1-\bigg( 1-\Big((\log_{\Gamma_{i}}{(1-F_{H}}_{2}))^{q}\Big)^{2q}\bigg)^{\Xi_{1}}\\ \end{aligned}}\,\, \Bigg)^{2q} \end{aligned}}\\ \,\,\,\,\,\end{bmatrix},\\ \begin{bmatrix} \sqrt[q]{ \begin{aligned} \Bigg(\sqrt[q]{ \begin{aligned} 1-\bigg( 1-\Big((\log_{\Gamma_{i}}{I_{H}}_{1})^{q}\Big)^{q}\bigg)^{\Xi_{1}}\\ \end{aligned}}\,\, \Bigg)^{q} + \Bigg(\sqrt[q]{ \begin{aligned} 1-\bigg( 1-\Big((\log_{\Gamma_{i}}{I_{H}}_{2})^{q}\Big)^{q}\bigg)^{\Xi_{1}}\\ \end{aligned}}\,\, \Bigg)^{q}, \\ - \Bigg(\sqrt[q]{ \begin{aligned} 1-\bigg( 1-\Big((\log_{\Gamma_{i}}{I_{H}}_{1})^{q}\Big)^{q}\bigg)^{\Xi_{1}}\\ \end{aligned}}\,\, \Bigg)^{q} \cdot \Bigg(\sqrt[q]{ \begin{aligned} 1-\bigg( 1-\Big((\log_{\Gamma_{i}}{I_{H}}_{2})^{q}\Big)^{q}\bigg)^{\Xi_{1}}\\ \end{aligned}}\,\, \Bigg)^{q} \end{aligned}}\\\\ \sqrt[q]{ \begin{aligned} \Bigg(\sqrt[q]{ \begin{aligned} 1-\bigg( 1-\Big((\log_{\Gamma_{i}}{I_{H}}_{1})^{q}\Big)^{q}\bigg)^{\Xi_{1}}\\ \end{aligned}}\,\, \Bigg)^{q} + \Bigg(\sqrt[q]{ \begin{aligned} 1-\bigg( 1-\Big((\log_{\Gamma_{i}}{I_{H}}_{2})^{q}\Big)^{q}\bigg)^{\Xi_{1}}\\ \end{aligned}}\,\, \Bigg)^{q} \\ - \Bigg(\sqrt[q]{ \begin{aligned} 1-\bigg( 1-\Big((\log_{\Gamma_{i}}{I_{H}}_{1})^{q}\Big)^{q}\bigg)^{\Xi_{1}}\\ \end{aligned}}\,\, \Bigg)^{q} \cdot \Bigg(\sqrt[q]{ \begin{aligned} 1-\bigg( 1-\Big((\log_{\Gamma_{i}}{I_{H}}_{2})^{q}\Big)^{q}\bigg)^{\Xi_{1}}\\ \end{aligned}}\,\, \Bigg)^{q} \end{aligned}}\\ \,\,\,\,\,\end{bmatrix},\\ \begin{bmatrix} \begin{aligned} \Bigg( \sqrt[2q]{1-\Big(1-(\log_{\Gamma_{i}}{F_{H}}_{1})^{2q}\Big)^{q}}\Bigg)^{\Xi_{1}} \cdot \Bigg( \sqrt[2q]{1-\Big(1-(\log_{\Gamma_{i}}{F_{H}}_{2})^{2q}\Big)^{q}}\Bigg)^{\Xi_{1}} \\ \end{aligned},\\ \begin{aligned} \Bigg( \sqrt[2q]{1-\Big(1-(\log_{\Gamma_{i}}{(1-T_{H}}_{1}))^{2q}\Big)^{q}}\Bigg)^{\Xi_{1}}\cdot \Bigg( \sqrt[2q]{1-\Big(1-(\log_{\Gamma_{i}}{(1-T_{H}}_{2}))^{2q}\Big)^{q}}\Bigg)^{\Xi_{1}}\\ \end{aligned} \end{bmatrix} \end{bmatrix} \end{eqnarray*} |
\begin{eqnarray*} & = &\begin{bmatrix} \Big(\oslash^{2}_{i = 1} \Xi_{i}\kappa_{i}^{\Theta}, \oslash^{2}_{i = 1} \Xi_{i}\delta_{i}^{\Theta}\Big);\\ \begin{bmatrix} \sqrt[2q]{ 1- \bigcirc^{2}_{i = 1} \bigg(1-\Big((\log_{\Gamma_{i}}{T_{H}}_{1})^{q}\Big)^{2q}\bigg)^{\Xi_{i}}}, \sqrt[2q]{ 1- \bigcirc^{2}_{i = 1} \bigg(1-\Big((\log_{\Gamma_{i}}{(1-F_{H}}_{1}))^{q}\Big)^{2q}\bigg)^{\Xi_{i}}} \,\,\end{bmatrix},\\ \begin{bmatrix} \sqrt[q]{ 1- \bigcirc^{2}_{i = 1} \bigg(1-\Big((\log_{\Gamma_{i}}{I_{H}}_{1})^{q}\Big)^{q}\bigg)^{\Xi_{i}}}, \sqrt[q]{ 1- \bigcirc^{2}_{i = 1} \bigg(1-\Big((\log_{\Gamma_{i}}{I_{H}}_{1})^{q}\Big)^{q}\bigg)^{\Xi_{i}}} \,\,\end{bmatrix},\\ \begin{bmatrix} \bigcirc^{2}_{i = 1}\Bigg( \sqrt[2q]{1-\Big(1-(\log_{\Gamma_{i}}{F_{H}}_{i})^{2q}\Big)^{q}}\Bigg)^{\Xi_{i}}, \bigcirc^{2}_{i = 1} \Bigg( \sqrt[2q]{1-\Big(1-(\log_{\Gamma_{i}}{(1-T_{H}}_{i}))^{2q}\Big)^{q}}\Bigg)^{\Xi_{i}} \end{bmatrix} \end{bmatrix}. \end{eqnarray*} |
It is valid for n = l and l\succeq 3 .
Hence, \oslash^{l}_{i = 1} \Xi_{i} H_{i}^{\Theta} = \begin{bmatrix} \Big(\oslash^{l}_{i = 1} \Xi_{i}\kappa_{i}^{\Theta}, \oslash^{l}_{i = 1} \Xi_{i}\delta_{i}^{\Theta}\Big); \\ \begin{bmatrix} \sqrt[2q]{ 1- \bigcirc^{l}_{i = 1} \bigg(1-\Big((\log_{\Gamma_{i}}{T_{H}}_{1})^{q}\Big)^{2q}\bigg)^{\Xi_{i}}}, \sqrt[2q]{ 1- \bigcirc^{l}_{i = 1} \bigg(1-\Big((\log_{\Gamma_{i}}{(1-F_{H}}_{1}))^{q}\Big)^{2q}\bigg)^{\Xi_{i}}} \, \, \end{bmatrix}, \\ \begin{bmatrix} \sqrt[q]{ 1- \bigcirc^{l}_{i = 1} \bigg(1-\Big((\log_{\Gamma_{i}}{I_{H}}_{1})^{q}\Big)^{q}\bigg)^{\Xi_{i}}}, \sqrt[q]{ 1- \bigcirc^{l}_{i = 1} \bigg(1-\Big((\log_{\Gamma_{i}}{I_{H}}_{1})^{q}\Big)^{q}\bigg)^{\Xi_{i}}} \, \, \end{bmatrix}, \\ \begin{bmatrix} \bigcirc^{l}_{i = 1}\Bigg(\sqrt[2q]{1-\Big(1-(\log_{\Gamma_{i}}{F_{H}}_{i})^{2q}\Big)^{q}}\Bigg)^{\Xi_{i}}, \bigcirc^{l}_{i = 1} \Bigg(\sqrt[2q]{1-\Big(1-(\log_{\Gamma_{i}}{F_{H}}_{i})^{2q}\Big)^{q}}\Bigg)^{\Xi_{i}} \end{bmatrix} \end{bmatrix} .
If n = l+1 , then \oslash^{l}_{i = 1} \Xi_{i}H_{i}^{\Theta} +\Xi_{l+1} H_{l+1}^{\Theta} = \oslash^{l+1}_{i = 1} \Xi_{i}H_{i}^{\Theta} .
Now, \oslash^{l}_{i = 1} \Xi_{i}H_{i}^{\Theta} +\Xi_{l+1} H_{l+1}^{\Theta} = \Xi_{1}H_{1}^{\Theta} \boxplus \Xi_{2}H_{2}^{\Theta} \boxplus... \boxplus w_{l}H_{l}^{\Theta} \boxplus \Xi_{l+1}H_{l+1}^{\Theta} =
\begin{eqnarray*} &&\begin{bmatrix} \Big(\oslash^{l}_{i = 1} \Xi_{i}\kappa_{i}^{\Theta}+ \Xi_{l+1}\Gamma_{l+1}^{\Theta}, \oslash^{l}_{i = 1} \Xi_{i}\delta_{i}^{\Theta}+ \Xi_{l+1}\delta_{l+1}^{\Theta}\Big);\\ \begin{bmatrix} \sqrt[2q]{ \begin{aligned} \Bigg(\sqrt[2q]{ \begin{aligned} 1-\bigcirc^{l}_{i = 1}\bigg( 1-\Big((\log_{\Gamma_{i}}{T_{H}}_{i})^{q}\Big)^{2q}\bigg)^{\Xi_{i}}\\ \end{aligned}}\,\, \Bigg)^{2q} + \Bigg(\sqrt[2q]{ \begin{aligned} 1-\bigg( 1-\Big((\log_{\Gamma_{i}}{T_{H}}_{l+1})^{q}\Big)^{2q}\bigg)^{\Xi_{1}}\\ \end{aligned}}\,\, \Bigg)^{2q}, \\ - \Bigg(\sqrt[2q]{ \begin{aligned} 1-\bigcirc^{l}_{i = 1}\bigg( 1-\Big((\log_{\Gamma_{i}}{T_{H}}_{i})^{q}\Big)^{2q}\bigg)^{\Xi_{i}}\\ \end{aligned}}\,\, \Bigg)^{2q} \cdot \Bigg(\sqrt[2q]{ \begin{aligned} 1-\bigg( 1-\Big((\log_{\Gamma_{i}}{T_{H}}_{l+1})^{q}\Big)^{2q}\bigg)^{\Xi_{1}}\\ \end{aligned}}\,\, \Bigg)^{2q} \end{aligned}}\\ \sqrt[2q]{ \begin{aligned} \Bigg(\sqrt[2q]{ \begin{aligned} 1-\bigcirc^{l}_{i = 1}\bigg( 1-\Big((\log_{\Gamma_{i}}{(1-F_{H}}_{i}))^{q}\Big)^{2q}\bigg)^{\Xi_{i}}\\ \end{aligned}}\,\, \Bigg)^{2q} + \Bigg(\sqrt[2q]{ \begin{aligned} 1-\bigg( 1-\Big((\log_{\Gamma_{i}}{(1-F_{H}}_{l+1}))^{q}\Big)^{2q}\bigg)^{\Xi_{1}}\\ \end{aligned}}\,\, \Bigg)^{2q} \\ - \Bigg(\sqrt[2q]{ \begin{aligned} 1-\bigcirc^{l}_{i = 1}\bigg( 1-\Big((\log_{\Gamma_{i}}{(1-F_{H}}_{i}))^{q}\Big)^{2q}\bigg)^{\Xi_{i}}\\ \end{aligned}}\,\, \Bigg)^{2q} \cdot \Bigg(\sqrt[2q]{ \begin{aligned} 1-\bigg( 1-\Big((\log_{\Gamma_{i}}{(1-F_{H}}_{l+1}))^{q}\Big)^{2q}\bigg)^{\Xi_{1}}\\ \end{aligned}}\,\, \Bigg)^{2q} \end{aligned}}\\ \,\,\,\,\,\end{bmatrix},\\ \begin{bmatrix} \sqrt[q]{ \begin{aligned} \Bigg(\sqrt[q]{ \begin{aligned} 1-\bigcirc^{l}_{i = 1}\bigg( 1-\Big((\log_{\Gamma_{i}}{I_{H}}_{i})^{q}\Big)^{q}\bigg)^{\Xi_{i}}\\ \end{aligned}}\,\, \Bigg)^{q} + \Bigg(\sqrt[q]{ \begin{aligned} 1-\bigg( 1-\Big((\log_{\Gamma_{i}}{I_{H}}_{l+1})^{q}\Big)^{q}\bigg)^{\Xi_{1}}\\ \end{aligned}}\,\, \Bigg)^{q}, \\ - \Bigg(\sqrt[q]{ \begin{aligned} 1-\bigcirc^{l}_{i = 1}\bigg( 1-\Big((\log_{\Gamma_{i}}{I_{H}}_{i})^{q}\Big)^{q}\bigg)^{\Xi_{i}}\\ \end{aligned}}\,\, \Bigg)^{q} \cdot \Bigg(\sqrt[q]{ \begin{aligned} 1-\bigg( 1-\Big((\log_{\Gamma_{i}}{I_{H}}_{l+1})^{q}\Big)^{q}\bigg)^{\Xi_{1}}\\ \end{aligned}}\,\, \Bigg)^{q} \end{aligned}}\\ \sqrt[q]{ \begin{aligned} \Bigg(\sqrt[q]{ \begin{aligned} 1-\bigcirc^{l}_{i = 1}\bigg( 1-\Big((\log_{\Gamma_{i}}{I_{H}}_{i})^{q}\Big)^{q}\bigg)^{\Xi_{i}}\\ \end{aligned}}\,\, \Bigg)^{q} + \Bigg(\sqrt[q]{ \begin{aligned} 1-\bigg( 1-\Big((\log_{\Gamma_{i}}{I_{H}}_{l+1})^{q}\Big)^{q}\bigg)^{\Xi_{1}}\\ \end{aligned}}\,\, \Bigg)^{q} \\ - \Bigg(\sqrt[q]{ \begin{aligned} 1-\bigcirc^{l}_{i = 1}\bigg( 1-\Big((\log_{\Gamma_{i}}{I_{H}}_{i})^{q}\Big)^{q}\bigg)^{\Xi_{i}}\\ \end{aligned}}\,\, \Bigg)^{q} \cdot \Bigg(\sqrt[q]{ \begin{aligned} 1-\bigg( 1-\Big((\log_{\Gamma_{i}}{I_{H}}_{l+1})^{q}\Big)^{2q}\bigg)^{\Xi_{1}}\\ \end{aligned}}\,\, \Bigg)^{q} \end{aligned}}\\ \,\,\end{bmatrix},\\ \begin{bmatrix} \begin{aligned} \bigcirc^{l}_{i = 1}\Bigg( \sqrt[2q]{1-\Big(1-(\log_{\Gamma_{i}}{F_{H}}_{i})^{2q}\Big)^{q}}\Bigg)^{\Xi_{i}} \cdot \Bigg( \sqrt[2q]{1-\Big(1-(\log_{\Gamma_{i}}{F_{H}}_{l+1})^{2q}\Big)^{q}}\Bigg)^{\Xi_{1}} \\ \end{aligned},\\ \begin{aligned} \bigcirc^{l}_{i = 1}\Bigg( \sqrt[2q]{1-\Big(1-(\log_{\Gamma_{i}}{(1-T_{H}}_{i}))^{2q}\Big)^{q}}\Bigg)^{\Xi_{i}} \cdot \Bigg( \sqrt[2q]{1-\Big(1-(\log_{\Gamma_{i}}{(1-T_{H}}_{l+1}))^{2q}\Big)^{q}}\Bigg)^{\Xi_{1}}\\ \end{aligned} \end{bmatrix} \end{bmatrix}. \end{eqnarray*} |
Thus,
\oslash^{l+1}_{i = 1} \Xi_{i}H_{i}^{\Theta} = \begin{bmatrix} \Big(\oslash^{l+1}_{i = 1} \Xi_{i}\kappa_{i}^{\Theta}, \oslash^{l+1}_{i = 1} \Xi_{i}\delta_{i}^{\Theta}\Big);\\ \begin{bmatrix} \sqrt[2q]{ 1- \bigcirc^{l+1}_{i = 1} \bigg(1-\Big((\log_{\Gamma_{i}}{T_{H}}_{1})^{q}\Big)^{2q}\bigg)^{\Xi_{i}}}, \sqrt[2q]{ 1- \bigcirc^{l+1}_{i = 1} \bigg(1-\Big((\log_{\Gamma_{i}}{(1-F_{H}}_{1}))^{q}\Big)^{2q}\bigg)^{\Xi_{i}}} \,\,\end{bmatrix},\\ \begin{bmatrix} \sqrt[q]{ 1- \bigcirc^{l+1}_{i = 1} \bigg(1-\Big((\log_{\Gamma_{i}}{I_{H}}_{1})^{q}\Big)^{q}\bigg)^{\Xi_{i}}}, \sqrt[q]{ 1- \bigcirc^{l+1}_{i = 1} \bigg(1-\Big((\log_{\Gamma_{i}}{I_{H}}_{1})^{q}\Big)^{q}\bigg)^{\Xi_{i}}} \,\,\end{bmatrix},\\ \begin{bmatrix} \bigcirc^{l+1}_{i = 1}\Bigg( \sqrt[2q]{1-\Big(1-(\log_{\Gamma_{i}}{F_{H}}_{i})^{2q}\Big)^{q}}\Bigg)^{\Xi_{i}}, \bigcirc^{l+1}_{i = 1} \Bigg( \sqrt[2q]{1-\Big(1-(\log_{\Gamma_{i}}{F_{H}}_{i})^{2q}\Big)^{q}}\Bigg)^{\Xi_{i}} \end{bmatrix} \end{bmatrix}. |
Hence, \left(\oslash^{l+1}_{i = 1} \Xi_{i}H_{i}^{\Theta}\right)^{1/\Theta} \begin{bmatrix} \Bigg(\Big(\oslash^{l+1}_{i = 1} \Xi_{i}\kappa_{i}^{\Theta}\Big)^{1/\Theta}, \Big(\oslash^{l+1}_{i = 1} \Xi_{i}\delta_{i}^{\Theta}\Big)^{1/\Theta}\Bigg); \\ \begin{bmatrix} \Bigg(\sqrt[2q]{ \begin{aligned} 1-\bigcirc^{l+1}_{i = 1} \Bigg(1-\Big((\log_{\Gamma_{i}}{T_{H}}_{i})^{q}\Big)^{2q}\Bigg)^{\Xi_{i}}\\ \end{aligned}}\, \, \Bigg)^{1/q}, \Bigg(\sqrt[2q]{ \begin{aligned} 1-\bigcirc^{l+1}_{i = 1} \Bigg(1-\Big((\log_{\Gamma_{i}}{(1-F_{H}}_{i}))^{q}\Big)^{2q}\Bigg)^{\Xi_{i}}\\ \end{aligned}}\, \, \Bigg)^{1/q} \, \, \, \, \end{bmatrix}, \\ \begin{bmatrix} \Bigg(\sqrt[q]{ \begin{aligned} 1-\bigcirc^{l+1}_{i = 1} \Bigg(1-\Big((\log_{\Gamma_{i}}{I_{H}}_{i})^{q}\Big)^{q}\Bigg)^{\Xi_{i}}\\ \end{aligned}}\, \, \Bigg)^{1/q}, \Bigg(\sqrt[q]{ \begin{aligned} 1-\bigcirc^{l+1}_{i = 1} \Bigg(1-\Big((\log_{\Gamma_{i}}{I_{H}}_{i})^{q}\Big)^{q}\Bigg)^{\Xi_{i}}\\ \end{aligned}}\, \, \Bigg)^{1/q} \, \, \, \, \end{bmatrix}, \\ \begin{bmatrix} \sqrt[2q]{1-\Bigg(\begin{aligned} 1-\Bigg(\bigcirc^{l+1}_{i = 1} \Bigg(\sqrt[2q]{1-\Big(1-(\log_{\Gamma_{i}}{F_{H}}_{i})^{2q}\Big)^{q}}\Bigg)^{\Xi_{i}}\\ \end{aligned}\, \, \Bigg)^{2}\Bigg)^{1/q}}, \\ \sqrt[2q]{1-\Bigg(\begin{aligned} 1-\Bigg(\bigcirc^{l+1}_{i = 1} \Bigg(\sqrt[2q]{1-\Big(1-(\log_{\Gamma_{i}}{(1-T_{H}}_{i}))^{2q}\Big)^{q}}\Bigg)^{\Xi_{i}}\\ \end{aligned}\, \, \Bigg)^{2}\Bigg)^{1/q}} \end{bmatrix}\\ \end{bmatrix} .
It is valid for l \succeq 1 .
Remark 5.1. If q = 1 , then the q -rung log GPyNVNWA operator is modified to the q -rung log PyNVNWA operator.
Theorem 5.8. If all H_{i} = \Big\langle (\kappa_{i}, \delta_{i}); [\log{T_{H}}_{i}, \log{(1-F_{H}}_{i})], [\log{I_{H}}_{i}, \log{I_{H}}_{i}] [\log{F_{H}}_{i} , \log{(1-T_{H}}_{i})] \Big\rangle \, \, \, (i = 1, 2, ..., n) are equal and H_{i} = H , then the q -rung log GPyNVNWA operator (H_{1}, H_{2}, ..., H_{n}) = H .
Proof. This proof is based on Theorem 5.2.
Remark 5.2. To satisfy the boundedness and monotonicity conditions, we use the q -rung log GPyNVNWA operator.
Proof. This proof is based on Theorems 5.3 and 5.4.
As generalizations of the q -rung log PyNVNWG operators, some q -rung log GPyNVNWG operators are developed in Section 5.4.
Definition 5.4. Let H_{i} = \Big\langle (\kappa_{i}, \delta_{i}); [\log{T_{H}}_{i}, \log{(1-F_{H}}_{i})], [\log{I_{H}}_{i}, \log{I_{H}}_{i}], [\log{F_{H}}_{i}, \log{(1-T_{H}}_{i})] \Big\rangle be the family of q -rung log PyNVNNs, then the q -rung log GPyNVNWG operator (H_{1}, H_{2}, ..., H_{n}) = \frac{1}{\Theta}\Big(\bigcirc^{n}_{i = 1} (\Theta H_{i})^{\Xi_{i}} \Big) \, \, \, (i = 1, 2, ..., n) is called the q -rung log GPyNVNWG operator.
Theorem 5.9. Let H_{i} = \Big\langle (\kappa_{i}, \delta_{i}); [\log{T_{H}}_{i}, \log{(1-F_{H}}_{i})], [\log{I_{H}}_{i}, \log{I_{H}}_{i}], [\log{F_{H}}_{i} , \log{(1-T_{H}}_{i})] \Big\rangle be the family of q -rung log PyNVNNs. Then, the q -rung log GPyNVNWG operator (H_{1}, H_{2}, ..., H_{n}) = \\
\begin{bmatrix} \Bigg( \frac{1}{\Theta} \bigcirc^{n}_{i = 1} (\Theta \kappa_{i})^{\Xi_{i}}, \frac{1}{\Theta} \bigcirc^{n}_{i = 1} (\Theta \delta_{i})^{\Xi_{i}}\Bigg);\\ \begin{bmatrix} \sqrt[2q]{1-\Bigg( \begin{aligned} 1-\Bigg(\bigcirc^{n}_{i = 1} \Bigg( \sqrt[2q]{1-\Big(1-(\log_{\Gamma_{i}}{T_{H}}_{i})^{2q}\Big)^{q}}\Bigg)^{\Xi_{i}}\\ \end{aligned}\,\,\Bigg)^{2q}\Bigg)^{1/q}},\\ \sqrt[2q]{1-\Bigg( \begin{aligned} 1-\Bigg(\bigcirc^{n}_{i = 1} \Bigg( \sqrt[2q]{1-\Big(1-(\log_{\Gamma_{i}}{(1-F_{H}}_{i}))^{2q}\Big)^{q}}\Bigg)^{\Xi_{i}}\\ \end{aligned}\,\,\Bigg)^{2q}\Bigg)^{1/q}} \end{bmatrix},\\ \begin{bmatrix} \Bigg(\sqrt[q]{ \begin{aligned} 1-\bigcirc^{n}_{i = 1} \Bigg( 1-\Big((\log_{\Gamma_{i}}{I_{H}}_{i})^{q}\Big)^{q}\Bigg)^{\Xi_{i}}\\ \end{aligned}}\,\,\Bigg)^{1/q}, \Bigg(\sqrt[q]{ \begin{aligned} 1-\bigcirc^{n}_{i = 1} \Bigg( 1-\Big((\log_{\Gamma_{i}}{I_{H}}_{i})^{q}\Big)^{q}\Bigg)^{\Xi_{i}}\\ \end{aligned}}\,\,\Bigg)^{1/q} \,\, \end{bmatrix},\\ \begin{bmatrix} \Bigg(\sqrt[2q]{ \begin{aligned} 1-\bigcirc^{n}_{i = 1} \Bigg( 1-\Big((\log_{\Gamma_{i}}{F_{H}}_{i})^{q}\Big)^{2q}\Bigg)^{\Xi_{i}}\\ \end{aligned}}\,\,\Bigg)^{1/q}, \Bigg(\sqrt[2q]{ \begin{aligned} 1-\bigcirc^{n}_{i = 1} \Bigg( 1-\Big((\log_{\Gamma_{i}}{(1-T_{H}}_{i}))^{q}\Big)^{2q}\Bigg)^{\Xi_{i}}\\ \end{aligned}}\,\,\Bigg)^{1/q} \,\, \end{bmatrix} \end{bmatrix}. |
Proof. This proof is based on the Theorem 5.7.
Remark 5.3. If q = 1 , then the q -rung log GPyNVNWG operator is converted to the q -rung log PyNVNWG operator.
Remark 5.4. Boundedness and monotonicity properties can be met by using the q -rung log GPyNVNWG operator.
Proof. This proof is based on Theorems 5.3 and 5.4.
Corollary 5.2. If all H_{i} = \Big\langle (\kappa_{i}, \delta_{i}); [\log{T_{H}}_{i}, \log{(1-F_{H}}_{i})], [\log{I_{H}}_{i}, \log{I_{H}}_{i}] [\log{F_{H}}_{i} , \log{(1-T_{H}}_{i})] \Big\rangle are equal and H_{i} = H , for i = 1, 2, ..., n , then the q -rung log GPyNVNWG operator (H_{1}, H_{2}, ..., H_{n}) = H .
Proof. This proof is based on Theorem 5.2.
The complexity of real-world systems increases daily, making it difficult for decision-makers to choose the right option. It is difficult to summarize the objective of achieving a single goal, but it is not impossible. Motivating employees, setting goals, and addressing opinions and complications have been challenges for many organizations. The DM process, whether by an individual or by a committee, must be transparent and consider multiple objectives at once. According to this reflection, each decision- maker is prevented from achieving an ideal solution under each of the criteria involved in practical problems. Therefore, decision-makers can develop more effective and reliable methods to identify the most appropriate option. DM problems involving ambiguity and uncertainty do not always respond well to classical or crisp methods. This paper presents an MADM approach based on q -rung log PyNVNNs with AOs, which involves an algorithm for selecting the most suitable option from a set of options in the MADM problem, using q -rung log PyNVNNs with AOs. The purpose of this paper is to propose a multi-attribute neutrosophic approach for DM problems with weights.
Let H = \{H_{1}, H_{2}, ..., H_{n}\} be a set of n -alternatives, \mathscr{Z} = \{\mathscr{Z}_{1}, \mathscr{Z}_{2}, ..., \mathscr{Z}_{m}\} be a set of m -attributes and the weights \Xi = \{\Xi_{1}, \Xi_{2}, ..., \Xi_{m}\} , where \Xi_{i} \in [0, 1] and \sum^{m}_{i} \Xi_{i} = 1 .
Let H_{ij} = \Big\langle (\kappa_{ij}, \delta_{ij}); [\log_{\Gamma_{i}}{T_{H}}_{ij}, \log_{\Gamma_{i}}{(1-F_{H}}_{ij})], [\log_{\Gamma_{i}}{I_{H}}_{ij}, \log_{\Gamma_{i}}{I_{H}}_{ij}], [\log_{\Gamma_{i}}{F_{H}}_{ij}, \log_{\Gamma_{i}}{(1-T_{H}}_{ij})]\Big\rangle denote the q -rung log PyNVNNs of alternative H_{i} in attribute \mathscr{Z}_{j} , i = 1, 2, ..., n and j = 1, 2, ..., m .
Suppose that \Big[\log_{\Gamma_{i}}{T_{H}}_{ij}, \log_{\Gamma_{i}}{(1-F_{H}}_{ij})\Big], \Big[\log_{\Gamma_{i}}{I_{H}}_{ij}, \log_{\Gamma_{i}}{I_{H}}_{ij}\Big], \Big[\log_{\Gamma_{i}}{F_{H}}_{ij}, \log_{\Gamma_{i}}{(1-T_{H}}_{ij})\Big]\in Int([0, 1]) and 0 \preceq (\log_{\Gamma_{i}}{1-F_{H}}_{ij}(\varepsilon))^{q}+(\log_{\Gamma_{i}}{I_{H}}_{ij}(\varepsilon))^{q} +(\log_{\Gamma_{i}}{1-T_{H}}_{ij}(\varepsilon))^{q}\preceq 2 . The MADM process is represented by the following flowchart based on the q -rung log PyNVNN.
Using the proposed method, one can summarize the process depicted in Figure 1 as follows:
Step (a). Decision values for the q -rung log PyNVNNs.
Step (b). Compute the normalized decision values. The decision matrix \mathscr{D} = (\widetilde{\mathscr{C}}_{ij})_{n \times m}
is normalized into \overrightarrow{\mathscr{D}} = (\breve{\mathscr{C}_{ij}})_{n \times m} ,
where \breve{\mathscr{C}_{ij}} = \Big\langle (\overrightarrow{\kappa_{ij}}, \overrightarrow{\delta_{ij}}); [\overrightarrow{\log_{\Gamma_{i}}{T_{H}}_{ij}}, \overrightarrow{\log_{\Gamma_{i}}{(1-F_{H}}_{ij})}], [\overrightarrow{\log_{\Gamma_{i}}{I_{H}}_{ij}}, \overrightarrow{\log_{\Gamma_{i}}{I_{H}}_{ij}}],
[\overrightarrow{\log_{\Gamma_{i}}{F_{H}}_{ij}}, \overrightarrow{\log_{\Gamma_{i}}{(1-T_{H}}_{ij})}] \Big\rangle ; \overrightarrow{\kappa_{ij}} = \frac{\Gamma_{ij}} {\max_{i}(\kappa_{ij})}, \overrightarrow{\delta_{ij}} = \frac{\delta_{ij}}{\max_{i}(\delta_{ij})} \cdot \frac{\delta_{ij}}{\kappa_{ij}}, \overrightarrow{\log_{\Gamma_{i}}{T_{H}}_{ij}} = \log_{\Gamma_{i}}{T_{H}}_{ij},
\overrightarrow{\log_{\Gamma_{i}}{(1-F_{H}}_{ij})} = \log_{\Gamma_{i}}{(1-F_{H}}_{ij}) , where \Gamma_{i} = \prod [T_{H_i}, 1-F_{H_i}], [I_{H_i}, I_{H_i}], [F_{H_i}, 1-T_{H_i}] .
Step (c). Find the aggregate values for every alternative. On the basis of q -rung log PyNVNN
AOs, attribute \mathscr{Z}_{j} in \widetilde{\mathscr{C}}_{i} , \breve{\mathscr{C}_{ij}} = \Big\langle (\overrightarrow{\kappa_{ij}}, \overrightarrow{\delta_{ij}}); [\overrightarrow{\log_{\Gamma_{i}}{T_{H}}_{ij}}, \overrightarrow{\log_{\Gamma_{i}}{(1-F_{H}}_{ij})}],
[\overrightarrow{\log_{\Gamma_{i}}{I_{H}}_{ij}}, \overrightarrow{\log_{\Gamma_{i}}{I_{H}}_{ij}}], [\overrightarrow{\log_{\Gamma_{i}}{F_{H}}_{ij}}, \overrightarrow{\log_{\Gamma_{i}}{(1-T_{H}}_{ij})}] \Big\rangle is aggregated into
\breve{\mathscr{C}_{i}} = \Big\langle (\overrightarrow{\kappa_{i}}, \overrightarrow{\delta_{i}}); [\overrightarrow{\log_{\Gamma_{i}}{T_{H}}_{i}}, \overrightarrow{\log_{\Gamma_{i}}{(1-F_{H}}_{i})}] , [\overrightarrow{\log_{\Gamma_{i}}{I_{H}}_{i}}, \overrightarrow{\log_{\Gamma_{i}}{I_{H}}_{i}}], [\overrightarrow{\log_{\Gamma_{i}}{F_{H}}_{i}}, \overrightarrow{\log_{\Gamma_{i}}{(1-T_{H}}_{i})}] \Big\rangle .
Step (d). The ideal values for each option can be calculated as follows:
{\breve{\mathscr{C}}}^{+}= \begin{bmatrix} \Big(\max_{1\preceq i\preceq n}(\overrightarrow{\kappa_{ij}}), \min_{1\preceq i\preceq n}(\overrightarrow{\delta_{ij}})\Big);\\ [1,1],[1,1],[0,0] \end{bmatrix} and {\breve{\mathscr{C}}}^{-}= \begin{bmatrix} \Big(\min_{1\preceq i\preceq n}(\overrightarrow{\kappa_{ij}}), \max_{1\preceq i\preceq n}(\overrightarrow{\delta_{ij}})\Big);\\ [0,0],[0,0],[1,1] \end{bmatrix}.
Step (e). The EDs between each alternative with various ideal values are as follows:
\mathscr{D}_{i}^{+} = \mathscr{D}_{E}\Big(\breve{\mathscr{C}_i}, {\breve{\mathscr{C}}}^{+}\Big); \, \, \mathscr{D}_{i}^{-} = \mathscr{D}_{E}\Big(\breve{\mathscr{C}_i}, {\breve{\mathscr{C}}}^{-}\Big).
Step (f). The relative closeness values are calculated as follows: \mathscr{D}^{\ast}_{i} = \frac{\mathscr{D}_{i}^{-}}{\mathscr{D}_{i}^{+} + \mathscr{D}_{i}^{-}}.
Step (g). The greatest value is \max \mathscr{D}^{\ast}_{i} .
The MADM process has the potential to improve and evaluate multiple conflicting criteria in all areas of data mining. A business needs to respond more accurately and more effectively to changing customer needs in this competitive environment. As a result, MADM is capable of handling the evaluation process for multiple contradictory criteria successfully. In order to make an intelligent decision, experts analyze every characteristic of an alternative and then make their decision based on that analysis. Furthermore, this study presents the model for MADM and the basic steps to construct it by using the q-ROFS AOs. MADM is demonstrated here by using the AOs of q -rung log PyNVNNs as a practical example. A significant transformation has taken place in the agriculture sector in India over the past few years, from conventional farming to smart farming; we have come a long way. In rural agriculture, technology has risen to the occasion and yielded cutting-edge strategies to boost productivity. Ultimately, the goal is to increase farmer productivity and to provide high yields of crops to feed an expanding population. This sustainable growth is achieved by combining AI with technical tools, such as drones, moisture sensors, etc. One example is the use of robots in agriculture.
A description and classification of agricultural robotics can be found here:
(1) Crop-Harvesting robots ( \mathscr{B}_1 ):
There are many physical and mental demands associated with crop harvesting. A sensitive touch is required, as well as a certain amount of knowledge. Several robotic components are used in crop harvesting robots to enable them to operate in hot and unfavorable conditions. To handle delicate crops and avoid unripe or diseased goods, these robots use machine learning algorithms and computer vision. Abundant Robotics, Harvest Automation, and Harvest Croo are the top three companies for manufacturing crop harvesting robots in the world.
(2) Weeding robots ( \mathscr{B}_2 ):
Weed control is an extremely critical and challenging aspect of agriculture. Farmers continue to use herbicides even when crop rotation is in place. Chemically modified food has become increasingly disgusting to people and herbicide use is no longer a remedy. Robotic weed management makes sense in these circumstances. AI is used by these robots to identify crops from weeds. The use of traditional blades and finger weeders along the base of the plant reduces the use of herbicides. Among the world's leading manufacturers of weeding robots are the French companies Nexus robotics and Naio technologies.
(3) Aerial imagery drones and seed-planting drones ( \mathscr{B}_3 ):
Planting seeds and agricultural imagery seem to be in the air these days. Since aerial imaging gives farmers a bird's eye view of their crops, farmers save a lot of time. This technique can be used by farmers to quickly assess the state of their plants, pest problems, and weed growth. Additionally, they can use it to calculate how much seeds and fertilizer to apply. Precision farming is becoming increasingly dependent on drones. Computer vision and data analytics are applied to gather and analyze agricultural stress data through these high-technology, self-charging devices. Additionally, they assist farmers in identifying growth opportunities.
(4) Autonomous robotic tractor ( \mathscr{B}_4 ):
The world's most advanced self-steering tractor has been developed in Belgium by researchers from Katholieke Universiteit Leuven and Flanders Mechatronics Technology Center. Engineers hope to create a robotic tractor that is precise, versatile, and capable of operating anywhere on earth. As the tractor travels along the unpredictable and uneven terrain, keep an eye out for obstacles that could change the direction of the tractor. There are three components to an autonomous system: a steering system, an acceleration system, and a location detection system, including GPS. Tractors cannot steer themselves on the right path even with strong sensors and computers. With the software program being developed by the development team, any terrain can be customized.
(5) Soil sterilization robot ( \mathscr{B}_5 ):
A robot that has been developed by the Japanese Mitsubishi Research Institute administers sterilizing medication to soil. Laser triangulation guidance allows the machine to calculate and modify its position in constrained spaces. There is an addition of chemicals to the soil after cultivation to combat weeds, bacteria, fungi, and viruses.
Suppose that five robots (alternatives) are represented by \mathscr{B} = \{\mathscr{B}_1, \mathscr{B}_2, \mathscr{B}_3, \mathscr{B}_4, \mathscr{B}_5 \} . Four attributes are considered; robot controller features (\mathscr{Z}_{1}) , affordable off line programming software (\mathscr{Z}_{2}) , safety codes (\mathscr{Z}_{3}) , experience and reputation of the robot manufacturer (\mathscr{Z}_{4}) . In this example, the weights of \mathscr{Z}_{1}, \mathscr{Z}_{2}, \mathscr{Z}_{3}, \mathscr{Z}_{4} are 0.4, 0.3, 0.2 and 0.1, respectively. Next, this study employs the methodology developed in order to arrive at the most optimal solution. One must consider different alternatives.
Step (ⅰ). DM information is given as follows:
\mathscr{Z}_{1} | \mathscr{Z}_{2} | \mathscr{Z}_{3} | \mathscr{Z}_{4} | |
\mathscr{B}_{1} | \begin{bmatrix} (0.7,0.75)\\ [0.6,0.63]\\ [0.8,0.85]\\ [0.37,0.4]\\\end{bmatrix} | \begin{bmatrix} (0.6,0.45)\\ [0.5,0.55]\\ [0.6,0.65]\\ [0.45,0.5]\\\end{bmatrix} | \begin{bmatrix} (0.7,0.4)\\ [0.6,0.65]\\ [0.5,0.55]\\ [0.35,0.4]\\\end{bmatrix} | \begin{bmatrix} (0.7,0.3)\\ [0.4,0.45]\\ [0.3,0.35]\\ [0.55,0.6]\\\end{bmatrix} |
\mathscr{B}_{2} | \begin{bmatrix} (0.65,0.45)\\ [0.3,0.35]\\ [0.4,0.45]\\ [0.65,0.7]\\\end{bmatrix} | \begin{bmatrix} (0.7,0.55)\\ [0.45,0.5]\\ [0.5,0.55]\\ [0.5,0.55]\\\end{bmatrix} | \begin{bmatrix} (0.75,0.45)\\ [0.4,0.45]\\ [0.5,0.6]\\ [0.55,0.6]\\\end{bmatrix} | \begin{bmatrix} (0.6,0.4)\\ [0.6,0.65]\\ [0.5,0.55]\\ [0.35,0.4]\\\end{bmatrix} |
\mathscr{B}_{3} | \begin{bmatrix} (0.75,0.7)\\ [0.35,0.4]\\ [0.15,0.2]\\ [0.6,0.65]\\\end{bmatrix} | \begin{bmatrix} (0.8,0.6)\\ [0.6,0.7]\\ [0.3,0.5]\\ [0.3,0.4]\\\end{bmatrix} | \begin{bmatrix} (0.9,0.3)\\ [0.45,0.5]\\ [0.6,0.65]\\ [0.5,0.55]\\\end{bmatrix} | \begin{bmatrix} (0.85,0.65)\\ [0.35,0.4]\\ [0.4,0.5]\\ [0.6,0.65]\\\end{bmatrix} |
\mathscr{B}_{4} | \begin{bmatrix} (0.6,0.55)\\ [0.45,0.5]\\ [0.3,0.4]\\ [0.5,0.55]\\\end{bmatrix} | \begin{bmatrix} (0.75,0.5)\\ [0.5,0.6]\\ [0.4,0.45]\\ [0.4,0.5]\\\end{bmatrix} | \begin{bmatrix} (0.85,0.2)\\ [0.5,0.55]\\ [0.45,0.5]\\ [0.45,0.5]\\\end{bmatrix} | \begin{bmatrix} (0.8,0.5)\\ [0.45,0.5]\\ [0.45,0.55]\\ [0.5,0.55]\\\end{bmatrix} |
\mathscr{B}_{5} | \begin{bmatrix} (0.75,0.65)\\ [0.25,0.3]\\ [0.35,0.45]\\ [0.7,0.75]\\\end{bmatrix} | \begin{bmatrix} (0.55,0.45)\\ [0.55,0.65]\\ [0.35,0.4]\\ [0.35,0.45]\\\end{bmatrix} | \begin{bmatrix} (0.7,0.4)\\ [0.55,0.6]\\ [0.4,0.55]\\ [0.4,0.45]\\\end{bmatrix} | \begin{bmatrix} (0.75,0.4)\\ [0.4,0.45]\\ [0.55,0.6]\\ [0.55,0.6]\\\end{bmatrix} |
Step (ⅱ). We obtain the normalized decision matrix is as follows:
\mathscr{Z}_{1} | \mathscr{Z}_{2} | \mathscr{Z}_{3} | \mathscr{Z}_{4} | |
\mathscr{B}_{1} | \begin{bmatrix} (0.9412, 0.9375)\\ [0.6, 0.63]\\ [0.8, 0.85]\\ [0.37, 0.4]\\\end{bmatrix} | \begin{bmatrix} (0.8824, 0.6205)\\ [0.5, 0.55]\\ [0.6, 0.65]\\ [0.45, 0.5]\\\end{bmatrix} | \begin{bmatrix} (1, 0.7686)\\ [0.6, 0.65]\\ [0.5, 0.55]\\ [0.35, 0.4]\\\end{bmatrix} | \begin{bmatrix} (0.9375, 0.8711)\\ [0.4, 0.45]\\ [0.3, 0.35]\\ [0.55, 0.6]\\\end{bmatrix} |
\mathscr{B}_{2} | \begin{bmatrix} (0.7059, 0.6722)\\ [0.3, 0.35]\\ [0.4, 0.45]\\ [0.65, 0.7]\\\end{bmatrix} | \begin{bmatrix} (0.9412, 0.6923)\\ [0.45, 0.5]\\ [0.5, 0.55]\\ [0.5, 0.55]\\\end{bmatrix} | \begin{bmatrix} (0.6471, 0.6061)\\ [0.4, 0.45]\\ [0.5, 0.6]\\ [0.55, 0.6]\\\end{bmatrix} | \begin{bmatrix} (0.8750, 0.8048)\\ [0.6, 0.65]\\ [0.5, 0.55]\\ [0.35, 0.4]\\\end{bmatrix} |
\mathscr{B}_{3} | \begin{bmatrix} (1, 0.5647)\\ [0.35, 0.4]\\ [0.15, 0.2]\\ [0.6, 0.65]\\\end{bmatrix} | \begin{bmatrix} (1, 0.6516)\\ [0.6, 0.7]\\ [0.3, 0.5]\\ [0.3, 0.4]\\\end{bmatrix} | \begin{bmatrix} (0.9412, 0.9375)\\ [0.45, 0.5]\\ [0.6, 0.65]\\ [0.5, 0.55]\\\end{bmatrix} | \begin{bmatrix} (0.8125, 0.7385)\\ [0.35, 0.4]\\ [0.4, 0.5]\\ [0.6, 0.65]\\\end{bmatrix} |
\mathscr{B}_{4} | \begin{bmatrix} (0.7647, 0.5128)\\ [0.45, 0.5]\\ [0.3, 0.4]\\ [0.5, 0.55]\\\end{bmatrix} | \begin{bmatrix} (0.8235, 0.6648)\\ [0.5, 0.6]\\ [0.4, 0.45]\\ [0.4, 0.5]\\\end{bmatrix} | \begin{bmatrix} (0.9412, 0.7042)\\ [0.5, 0.55]\\ [0.45, 0.5]\\ [0.45, 0.5]\\\end{bmatrix} | \begin{bmatrix} (1, 0.9375)\\ [0.45, 0.5]\\ [0.45, 0.55]\\ [0.5, 0.55]\\\end{bmatrix} |
\mathscr{B}_{5} | \begin{bmatrix} (0.8235, 0.8048)\\ [0.25, 0.3]\\ [0.35, 0.45] \\ [0.7, 0.75]\\ \end{bmatrix} | \begin{bmatrix} (0.8824, 0.8667)\\ [0.55, 0.65]\\ [0.35, 0.4]\\ [0.35, 0.45]\\\end{bmatrix} | \begin{bmatrix} (0.7647, 0.7385)\\ [0.55, 0.6]\\ [0.4, 0.55]\\ [0.4, 0.45]\\\end{bmatrix} | \begin{bmatrix} (0.9375, 0.8711)\\ [0.4, 0.45]\\ [0.55, 0.6]\\ [0.55, 0.6]\\\end{bmatrix} |
Step (ⅲ). Based on the q -rung log PyNVNWA operator, aggregated information regarding alternatives (q = 1) are can be expressed as follows:
\breve{\mathscr{B}_{1}} | \breve{\mathscr{B}_{2}} | \breve{\mathscr{B}_{3}} | \breve{\mathscr{B}_{4}} | \breve{\mathscr{B}_{5}} |
\left[ \begin{matrix} (0.9349,0.8020); \\ [0.2991,0.3566], \\ [0.2380,0.2464], \\ [0.2009,0.2048] \\ \end{matrix} \right] | \left[ \begin{matrix} (0.7816,0.6783); \\ [0.3060,0.3490], \\ [0.2023,0.2137], \\ [0.1736,0.1805] \\ \end{matrix} \right] | \left[ \begin{matrix} (0.9695,0.6827); \\ [0.2583,0.2648], \\ [0.2612,0.3697], \\ [0.2450,0.2465] \\ \end{matrix} \right] | \left[ \begin{matrix} (0.8412,0.6392); \\ [0.3209,0.3591], \\ [0.2588,0.2590], \\ [0.2009,0.2040] \\ \end{matrix} \right] | \left[ \begin{matrix} (0.8408,0.8167); \\ [0.4358,0.4774], \\ [0.2568,0.3106], \\ [0.1649,0.1718] \\ \end{matrix} \right] |
Step (ⅳ). Among the various ideal values, the values of every alternative are given by {\breve{\mathscr{B}}}^{+} = \begin{bmatrix} (0.9695, 0.6392); [1, 1], [1, 1], [0, 0] \end{bmatrix} and {\breve{\mathscr{B}}}^{-} = \begin{bmatrix} (0.7816, 0.8167);[0, 0], [0, 0], [1, 1] \end{bmatrix}.
Step (ⅴ). The EDs between every alternative with various ideal values are as follows: \mathscr{D}^{+}_{1} = 0.0540, \mathscr{D}^{+}_{2} = 0.0911, \mathscr{D}^{+}_{3} = 0.0248, \mathscr{D}^{+}_{4} = 0.0770, \mathscr{D}^{+}_{5} = 0.0923, and \mathscr{D}^{-}_{1} = 0.2204, \mathscr{D}^{-}_{2} = 0.1798, \mathscr{D}^{-}_{3} = 0.2435, \mathscr{D}^{-}_{4} = 0.1915, \mathscr{D}^{-}_{5} = 0.1826.
Step (ⅵ). The relative closeness values are \mathscr{D}^{*}_{1} = 0.8033, \mathscr{D}^{*}_{2} = 0.6636, \mathscr{D}^{*}_{3} = 0.9076, \mathscr{D}^{*}_{4} = 0.7132, \mathscr{D}^{*}_{5} = 0.6642.
Step (ⅶ). The ranking of alternatives is \mathscr{B}_{3} > \mathscr{B}_{1} > \mathscr{B}_{4} > \mathscr{B}_{5} > \mathscr{B}_{2}.
Thus the alternative aerial imagery drones and seed planting drones \mathscr{B}_3 is the most desirable alternative based on the q -rung log PyNVNWG operator.
The ED and HD are extremely important for the MADM process. In contrast, few studies have been conducted on MADM using ED and HD as criterion values for alternatives, which include NSSs, interval NSSs and vague NSSs. Here, this study will compare ED and HD methods to validate the feasibility of our proposed DM method. An overview of interval-valued Pythagorean normal fuzzy information AOs was presented by Yang and Chang [31]. MADM-based Pythagorean neutrosophic normal interval-valued AOs were discussed in Palanikumar et al. [68]. Its usefulness and benefits can be seen in this example. q -rung log PyNVNWG, q -rung log GPyNVNWA, and q -rung log GPyNVNWG approaches were used by focusing on ED and HD, respectively. Here, existing and proposed methods of the comparative study are given in Table 1.
q=1 | q-log PyNVNWA | q-log PyNVNWG | q-log GPyNVNWA | q-log GPyNVNWG |
TOPSIS-\, ED | \mathscr{B}_{3}> \mathscr{B}_{1}> \mathscr{B}_{4} | \mathscr{B}_{3}> \mathscr{B}_{1}> \mathscr{B}_{5} | \mathscr{B}_{3}> \mathscr{B}_{1}> \mathscr{B}_{4} | \mathscr{B}_{3}> \mathscr{B}_{1}> \mathscr{B}_{5} |
({\bf Proposed}) | \mathscr{B}_{5}> \mathscr{B}_{2} | \mathscr{B}_{4}> \mathscr{B}_{2} | \mathscr{B}_{5}> \mathscr{B}_{2} | \mathscr{B}_{4}> \mathscr{B}_{2} |
TOPSIS-\, HD | \mathscr{B}_{3}> \mathscr{B}_{1}> \mathscr{B}_{4} | \mathscr{B}_{3}> \mathscr{B}_{1}> \mathscr{B}_{5} | \mathscr{B}_{3}> \mathscr{B}_{1}> \mathscr{B}_{4} | \mathscr{B}_{3}> \mathscr{B}_{1}> \mathscr{B}_{5} |
({\bf Proposed}) | \mathscr{B}_{5}> \mathscr{B}_{2} | \mathscr{B}_{4}> \mathscr{B}_{2} | \mathscr{B}_{5}> \mathscr{B}_{2} | \mathscr{B}_{4}> \mathscr{B}_{2} |
TOPSIS-\, ED | \mathscr{B}_{3}> \mathscr{B}_{1}> \mathscr{B}_{4} | \mathscr{B}_{3}> \mathscr{B}_{4}> \mathscr{B}_{1} | \mathscr{B}_{3}> \mathscr{B}_{1}> \mathscr{B}_{4} | \mathscr{B}_{3}> \mathscr{B}_{4}> \mathscr{B}_{1} |
[68] | \mathscr{B}_{5}> \mathscr{B}_{2} | \mathscr{B}_{5}> \mathscr{B}_{2} | \mathscr{B}_{5}> \mathscr{B}_{2} | \mathscr{B}_{5}> \mathscr{B}_{2} |
TOPSIS-\, HD | \mathscr{B}_{3}> \mathscr{B}_{1}> \mathscr{B}_{4} | \mathscr{B}_{3}> \mathscr{B}_{4}> \mathscr{B}_{1} | \mathscr{B}_{3}> \mathscr{B}_{1}> \mathscr{B}_{4} | \mathscr{B}_{3}> \mathscr{B}_{4}> \mathscr{B}_{1} |
[68] | \mathscr{B}_{5}> \mathscr{B}_{2} | \mathscr{B}_{5}> \mathscr{B}_{2} | \mathscr{B}_{5}> \mathscr{B}_{2} | \mathscr{B}_{5}> \mathscr{B}_{2} |
ED [31] | \mathscr{B}_{3}> \mathscr{B}_{1}> \mathscr{B}_{5} | \mathscr{B}_{3}> \mathscr{B}_{1}> \mathscr{B}_{4} | \mathscr{B}_{3}> \mathscr{B}_{1}> \mathscr{B}_{5} | \mathscr{B}_{3}> \mathscr{B}_{1}> \mathscr{B}_{4} |
\mathscr{B}_{4}> \mathscr{B}_{2} | \mathscr{B}_{5}> \mathscr{B}_{2} | \mathscr{B}_{4}> \mathscr{B}_{2} | \mathscr{B}_{5}> \mathscr{B}_{2} | |
HD [31] | \mathscr{B}_{3}> \mathscr{B}_{1}> \mathscr{B}_{5} | \mathscr{B}_{3}> \mathscr{B}_{1}> \mathscr{B}_{4} | \mathscr{B}_{3}> \mathscr{B}_{1}> \mathscr{B}_{5} | \mathscr{B}_{3}> \mathscr{B}_{1}> \mathscr{B}_{4} |
\mathscr{B}_{4}> \mathscr{B}_{2} | \mathscr{B}_{5}> \mathscr{B}_{2} | \mathscr{B}_{4}> \mathscr{B}_{2} | \mathscr{B}_{5}> \mathscr{B}_{2} | |
ED [59] | \mathscr{B}_{3}> \mathscr{B}_{1}> \mathscr{B}_{4} | \mathscr{B}_{3}> \mathscr{B}_{4}> \mathscr{B}_{1} | \mathscr{B}_{3}> \mathscr{B}_{1}> \mathscr{B}_{4} | \mathscr{B}_{3}> \mathscr{B}_{4}> \mathscr{B}_{1} |
\mathscr{B}_{5}> \mathscr{B}_{2} | \mathscr{B}_{5}> \mathscr{B}_{2} | \mathscr{B}_{5}> \mathscr{B}_{2} | \mathscr{B}_{5}> \mathscr{B}_{2} | |
HD [59] | \mathscr{B}_{3}> \mathscr{B}_{1}> \mathscr{B}_{4} | \mathscr{B}_{3}> \mathscr{B}_{4}> \mathscr{B}_{1} | \mathscr{B}_{3}> \mathscr{B}_{1}> \mathscr{B}_{4} | \mathscr{B}_{3}> \mathscr{B}_{4}> \mathscr{B}_{1} |
\mathscr{B}_{5}> \mathscr{B}_{2} | \mathscr{B}_{5}> \mathscr{B}_{2} | \mathscr{B}_{5}> \mathscr{B}_{2} | \mathscr{B}_{5}> \mathscr{B}_{2} | |
Score [59] | \mathscr{B}_{3}> \mathscr{B}_{1}> \mathscr{B}_{5} | \mathscr{B}_{3}> \mathscr{B}_{1}> \mathscr{B}_{4} | \mathscr{B}_{3}> \mathscr{B}_{1}> \mathscr{B}_{5} | \mathscr{B}_{3}> \mathscr{B}_{1}> \mathscr{B}_{4} |
\mathscr{B}_{4}> \mathscr{B}_{2} | \mathscr{B}_{5}> \mathscr{B}_{2} | \mathscr{B}_{4}> \mathscr{B}_{2} | \mathscr{B}_{5}> \mathscr{B}_{2} |
Consequently, the alternative third is the most suitable option among the other possibilities, so it should be selected. In Figures 2 and 3, the distances between each alternative are ranked by using EDs and HDs respectively.
We Analyzed the relationship between the MADM approach and the alternatives based on the reliability of the circumstances. The closeness values and rankings are as follows. As a result, this analysis shows that the developed methods are superior and more capable than existing ones. It has been estimated by using the q-rung log PyNVNWA algorithm.
Below presents the aggregate information with the q-rung log PyNVNWA operator for alternatives (q = 2) .
\breve{\mathscr{B}_{1}} | \breve{\mathscr{B}_{2}} | \breve{\mathscr{B}_{3}} | \breve{\mathscr{B}_{4}} | \breve{\mathscr{B}_{5}} |
\begin{bmatrix} (0.9349, 0.8020);\\ [0.3269, 0.3932],\\ [0.2420, 0.2495],\\ [0.2009, 0.2048]\\ \end{bmatrix} | \begin{bmatrix} (0.7816, 0.6783);\\ [0.3153, 0.3755],\\ [0.2243, 0.2323],\\ [0.1736, 0.1805]\\ \end{bmatrix} | \begin{bmatrix} (0.9695, 0.6827);\\ [0.2654, 0.2751],\\ [0.2633, 0.4298],\\ [0.2450, 0.2465]\\ \end{bmatrix} | \begin{bmatrix} (0.8412, 0.6392);\\ [0.3408, 0.3984],\\ [0.2633, 0.2938],\\ [0.2009, 0.2040]\\ \end{bmatrix} | \begin{bmatrix} (0.8408, 0.8167);\\ [0.5045, 0.5489],\\ [0.2760, 0.3799],\\ [0.1649, 0.1718]\\ \end{bmatrix} |
Among the various ideal values of every alternative, there are the following values: {\breve{\mathscr{B}}}^{+} = \begin{bmatrix} (0.9695, 0.6392); [1, 1], [1, 1], [0, 0] \end{bmatrix} and {\breve{\mathscr{B}}}^{-} = \begin{bmatrix} (0.7816, 0.8167);[0, 0], [0, 0], [1, 1] \end{bmatrix}. There is an ED between every alternative with various values \mathscr{D}^{+}_{1} = 0.0587, \mathscr{D}^{+}_{2} = 0.0924, \mathscr{D}^{+}_{3} = 0.0248, \mathscr{D}^{+}_{4} = 0.0806, \mathscr{D}^{+}_{5} = 0.1009 and \mathscr{D}^{-}_{1} = 0.2148, \mathscr{D}^{-}_{2} = 0.1785, \mathscr{D}^{-}_{3} = 0.2435, \mathscr{D}^{-}_{4} = 0.1879, \mathscr{D}^{-}_{5} = 0.1730. The relative closeness values are \mathscr{D}^{*}_{1}0.7852, \mathscr{D}^{*}_{2} = 0.6590, \mathscr{D}^{*}_{3} = 0.9074, \mathscr{D}^{*}_{4} = 0.6998, \mathscr{D}^{*}_{5} = 0.6315. The ranking of every alternative is \mathscr{B}_{3} > \mathscr{B}_{1} > \mathscr{B}_{4} > \mathscr{B}_{2} > \mathscr{B}_{5} .
A q -rung log PyNVNN is converted to an NFN. The q -rung log PyNVNWA, q -rung log PyNVNWG, q -rung log GPyNVNWA and q -rung log GPyNVNWG operators satisfy the properties of associativity, boundedness, and monotonicity. When q = 1 , this study converts the q -rung log GPyNVNWA operator to the q -rung log PyNVNWA operator. Regarding the for q = 1 output depicted in Figure 4, the q -rung log GPyNVNWG operator is converted into the q -rung log PyNVNWG operator. Using the q -rung log PyNVNWA method, if q = 1 , then the ranking of alternatives is \mathscr{B}_{3} > \mathscr{B}_{1} > \mathscr{B}_{4} > \mathscr{B}_{5} > \mathscr{B}_{2} . If q = 2 , then the ranking of alternatives in a new order is \mathscr{B}_{3} > \mathscr{B}_{1} > \mathscr{B}_{4} > \mathscr{B}_{2} > \mathscr{B}_{5} . Thus, the robotic \mathscr{B}_{5} becomes the robotic \mathscr{B}_{2} as the best alternative. As well, one can apply the q -rung log PyNVNWG, the q -rung log GPyNVNWA, and the q -rung log GPyNVNWG operators.
As a result of the analysis presented above, the recommended approach has the following advantages. This paper introduces the notion of q -rung log PyNVNN by combining the ideas of a PyNVNS. As the square sum of its TMG, IMG, and FMG is less than one, the q -rung log PyNVNN explains ambiguous information. Humans, natural phenomena, and ambiguous information can be interpreted by using a q -rung log PyNVNN. As a result, these methods are more general. Observe that all of the NSSs, interval-valued NSSs, vague NSSs, Pythagorean neutrosophic interval-valued normal sets and PyNVNSs in this study were derived from the q-rung log PyNVNN, which is a special case. In real life, it analyzes human behavior and natural events that follow a normal distribution, as well as analyzes the human behavior itself. Depending on q and the decision-maker's preferences, the outcome can be chosen. It is possible to achieve a variety of ranking outcomes of alternatives by using operators like the q -rung log PyNVNWA, q -rung log PyNVNWG, q -rung log GPyNVNWA, and q -rung log GPyNVNWA operators. Furthermore, our method is more flexible, allowing decision-makers to choose a different value of parameter q based on their risk attitude.
MADM has the potential and discipline to improve and evaluate multiple conflicting criteria in all areas of data mining. When making an intelligent decision, experts analyze each and every characteristic of an alternative. To arrive at a well-informed and intelligent decision, experts must carefully prepare and analyze each and every aspect of an alternative. If they have all of the data and information that they need, they can make a good decision. Classical set theory has been generalized to cope with uncertain information through the use of FSs, IFSs, PyFSs, NSSs, interval-valued NSSs and VSs. This research focuses on q -rung log PyNVNS problems, which arise in many DM domains. In the discussion of some AOs for q -rung log PyNVNSs, this study has resulted in a number of conclusions that are applicable to their q -rung log PyNVNSs. This study has yielded AO rules for q -rung log PyNVNWA, q -rung log PyNVNWG, q -rung log GPyNVNWA, and q -rung log GPyNVNWG operations. Using the q -rung log PyNVN-based MADM method can assist people in selecting the most appropriate course of action in uncertain and inconsistent information contexts. This study entailed applying the operators for q -rung log PyNVNWA, q -rung log PyNVNWG, q -rung log GPyNVNWA, and q -rung log GPyNVNWG to the MADM problem based on q . It is possible to compute distinct rankings by using q -rung log PyNVNWA, q -rung log PyNVNWG, q -rung log GPyNVNWA, and q -rung log GPyNVNWG operators based on q . Thus, one can examine the q with the greatest impact on alternative rankings. According to the examples, the proposed neutrosophic DM method is more appropriate for real scientific and engineering applications. The reason for this is that it can handle not only incomplete information, but also indeterminate information and inconsistent data that are present in real-life situations. It is hoped that this paper will improve existing DM methods and provide decision makers with an improved method for DM. Based on the real-life scenario, decision makers can set the values of q to determine the optimal ranking. Therefore, the decision-maker may decide on the outcome based on the actual value of q . The proposed approach facilitates MCDM and stepwise DM by utilizing the proposed approaches. Finally, the developed method has been illustrated numerically and compared with some existing methods to show that the proposed models are more effective than existing methods. One can focus on the few other advanced and intelligent techniques for DM that have been proposed by several researches [70]. Few studies proposed advanced technologies for environmental improvement [71,72,73] and technology usage [74,75], which can be combined with the present study for further development. The following topics will be discussed further in the future:
(1) An investigation of the q -rung logPythagorean neutrosophic vague normal types of soft sets and expert sets.
(2) Investigating Pythagorean cubic FSs and spherical cubic FSs of generalized q -rungs.
(3) MADM problems by using other DM methodologies based on square root Fermatean cubic FSs.
(4) An investigation of complex PyNVNSs with q -rungs.
The authors declare that they have not used Artificial Intelligence tools in the creation of this article.
The authors also declare that there is no conflict of interests regarding the publication of the paper.
[1] |
A. Kaplan, M. Haenlein, Rulers of the world, unite! The challenges and opportunities of artificial intelligence, Bus. Horizons, 63 (2020), 37–50. http://doi.org/10.1016/j.bushor.2019.09.003 doi: 10.1016/j.bushor.2019.09.003
![]() |
[2] |
H. Margetts, C. Dorobantu, Rethink government with AI, Nature, 568 (2019), 163–165. http://doi.org/10.1038/d41586-019-01099-5 doi: 10.1038/d41586-019-01099-5
![]() |
[3] |
K. Cresswell, M. Callaghan, S. Khan, Z. Sheikh, H. Mozaffar, A. Sheikh, Investigating the use of data-driven artificial intelligence in computerised decision support systems for health and social care: A systematic review, Health Inf. J., 26 (2020), 2138–2147. https://doi.org/10.1177/1460458219900452 doi: 10.1177/1460458219900452
![]() |
[4] | S. A. Yablonsky, Multidimensional data-driven artificial intelligence innovation, Technol. Innov. Magag. Rev., 9 (2019), 16–28. |
[5] |
J. Klinger, J. Mateos Garcia, K. Stathoulopoulos, Deep learning, deep change? Mapping the development of the artificial intelligence general purpose technology, Scientometrics, 126 (2021), 5589–5621. http://doi.org/10.1007/s11192-021-03936-9 doi: 10.1007/s11192-021-03936-9
![]() |
[6] | V. Rasskazov, Financial and economic consequences of distribution of artificial intelligence as a general-purpose technology, Financ.: Theory Pract., 24 (2020), 120–132. |
[7] |
A. Agostini, C. Torras, F. Worgotter, Efficient interactive decision-making framework for robotic applications, Artif. Intell., 247 (2017), 187–212. http://doi.org/10.1016/j.artint.2015.04.004 doi: 10.1016/j.artint.2015.04.004
![]() |
[8] |
L. A. Zadeh, Fuzzy sets, Inform. Control, 8 (1965), 338–353. http://doi.org/10.1016/S0019-9958(65)90241-X doi: 10.1016/S0019-9958(65)90241-X
![]() |
[9] |
K. Atanassov, Intuitionistic fuzzy sets, Fuzzy Sets Syst., 20 (1986), 87–96. http://doi.org/10.1016/S0165-0114(86)80034-3 doi: 10.1016/S0165-0114(86)80034-3
![]() |
[10] |
M. Gorzalczany, A method of inference in approximate reasoning based on interval valued fuzzy sets, Fuzzy Sets Syst., 21 (1987), 1–17. http://doi.org/10.1016/0165-0114(87)90148-5 doi: 10.1016/0165-0114(87)90148-5
![]() |
[11] | R. Biswas, Vague Groups, Int. J. Comput. Cogn., 4 (2006), 20–23. |
[12] |
R. R. Yager, Pythagorean membership grades in multi criteria decision-making, IEEE T. Fuzzy Sets Syst., 22 (2014), 958–965. http://doi.org/10.1109/TFUZZ.2013.2278989 doi: 10.1109/TFUZZ.2013.2278989
![]() |
[13] | X. Peng, Y. Yang, Fundamental properties of interval valued Pythagorean fuzzy aggregation operators, Int. J. Int. Syst., 31 (2016), 444–487. |
[14] |
S. Ashraf, S. Abdullah, T. Mahmood, F. Ghani, T. Mahmood, Spherical fuzzy sets and their applications in multi-attribute decision making problems, J. Intell. Fuzzy Syst., 36 (2019), 2829–2844. http://doi.org/10.3233/JIFS-172009 doi: 10.3233/JIFS-172009
![]() |
[15] |
A. Nicolescu, M. Teodorescu, A unifying field in logics - Book review, Int. Lett. Soc. Humanistic Sci., 43 (2015), 48–59. http://doi.org/10.18052/www.scipress.com/ILSHS.43.48 doi: 10.18052/www.scipress.com/ILSHS.43.48
![]() |
[16] | R. N. Xu, Regression prediction for fuzzy time series, Appl. Math. J. Chin. Univ. Ser. B, 16 (2001), 124376140. |
[17] |
R. R. Yager, Generalized orthopair fuzzy sets, IEEE T. Fuzzy Syst., 25 (2016), 1222–1230. http://doi.org/10.1109/TFUZZ.2016.2604005 doi: 10.1109/TFUZZ.2016.2604005
![]() |
[18] |
B. P. Joshi, A. Singh, P. K. Bhatt, K. S. Vaisla, Interval valued q-rung orthopair fuzzy sets and their properties, J. Intell. Fuzzy Syst., 35 (2018), 5225–5230. http://doi.org/10.3233/JIFS-169806 doi: 10.3233/JIFS-169806
![]() |
[19] |
R. R. Yager, Generalized orthopair fuzzy sets, IEEE T. Fuzzy Syst., 25 (2017), 1222–1230. http://doi.org/10.1109/TFUZZ.2016.2604005 doi: 10.1109/TFUZZ.2016.2604005
![]() |
[20] |
M. S. Habib, O. Asghar, A. Hussain, M. Imran, M. P. Mughal, B. Sarkar, A robust possibilistic programming approach toward animal fat-based biodiesel supply chain network design under uncertain environment, J. Clean. Prod., 278 (2021), 122403. http://doi.org/10.1016/j.jclepro.2020.122403 doi: 10.1016/j.jclepro.2020.122403
![]() |
[21] |
B. Sarkar, M. Tayyab, N. Kim, M. S. Habib, Optimal production delivery policies for supplier and manufacturer in a constrained closed-loop supply chain for returnable transport packaging through metaheuristic approach, Comput. Ind. Eng., 135 (2019), 987–1003. http://doi.org/10.1016/j.cie.2019.05.035 doi: 10.1016/j.cie.2019.05.035
![]() |
[22] |
D. Yadav, R. Kumari, N. Kumar, B. Sarkar, Reduction of waste and carbon emission through the selection of items with crossprice elasticity of demand to form a sustainable supply chain with preservation technology, J. Clean. Prod., 297 (2019), 126298. http://doi.org/10.1016/j.jclepro.2021.126298 doi: 10.1016/j.jclepro.2021.126298
![]() |
[23] |
B. K. Dey, S. Bhuniya, B. Sarkar, Involvement of controllable lead time and variable demand for a smart manufacturing system under a supply chain management, Expert Syst. Appl., 184 (2021), 115464. http://doi.org/10.1016/j.eswa.2021.115464 doi: 10.1016/j.eswa.2021.115464
![]() |
[24] | B. C. Cuong, V. Kreinovich, Picture fuzzy sets-A new concept for computational intelligence problems, 2013 Third World Congress on Information and Communication Technologies, 2013. http://doi.org/10.1109/WICT.2013.7113099 |
[25] |
M. Akram, W. A. Dudek, F. Ilyas, Group decision making based on Pythagorean fuzzy TOPSIS method, Int. J. Intell. Syst., 34 (2019), 1455–1475. http://doi.org/10.1002/int.22103 doi: 10.1002/int.22103
![]() |
[26] |
M. Akram, W. A. Dudek, J. M. Dar, Pythagorean Dombi Fuzzy Aggregation Operators with Application in Multi-criteria Decision-making, Int. J. Intell. Syst., 34 (2019), 3000–3019. http://doi.org/10.1002/int.22183 doi: 10.1002/int.22183
![]() |
[27] | Z. Xu, Intuitionistic fuzzy aggregation operators, IEEE Transactions on Fuzzy Systems, (2007), 1179–1187. |
[28] |
W. F. Liu, J. Chang, X. He, Generalized Pythagorean fuzzy aggregation operators and applications in decision making, Control Decision, 31 (2016), 2280–2286. http://doi.org/10.13195/j.kzyjc.2015.1537 doi: 10.13195/j.kzyjc.2015.1537
![]() |
[29] |
K. Rahman, S. Abdullah, M. Shakeel, M. S. A. Khan, M. Ullah, Interval valued Pythagorean fuzzy geometric aggregation operators and their application to group decision-making problem, Cogent Math., 4 (2017), 1338638. http://doi.org/10.1080/23311835.2017.1338638 doi: 10.1080/23311835.2017.1338638
![]() |
[30] |
K. Rahman, A. Ali, S. Abdullah, F. Amin, Approaches to multi-attribute group decision-making based on induced interval valued Pythagorean fuzzy Einstein aggregation operator, New Math. Natural Comput., 14 (2018), 343–361. https://doi.org/10.1142/S1793005718500217 doi: 10.1142/S1793005718500217
![]() |
[31] |
Z. Yang, J. Chang, Interval-valued Pythagorean normal fuzzy information aggregation operators for multiple attribute decision making approach, IEEE Access, 8 (2020), 51295–51314. http://doi.org/10.1109/ACCESS.2020.2978976 doi: 10.1109/ACCESS.2020.2978976
![]() |
[32] |
K. G. Fatmaa, K. Cengiza, Spherical fuzzy sets and spherical fuzzy TOPSIS method, J. Intell. Fuzzy Syst., 36 (2019), 337–352. http://doi.org/10.3233/JIFS-181401 doi: 10.3233/JIFS-181401
![]() |
[33] |
P. Liu, G. Shahzadi, M. Akram, Specific types of q-rung picture fuzzy Yager aggregation operators for decision-making, Int. J. Comput. Intell. Syst., 13 (2020), 1072–1091. http://doi.org/10.2991/ijcis.d.200717.001 doi: 10.2991/ijcis.d.200717.001
![]() |
[34] |
Z. Yang, X. Li, Z. Cao, J. Li, q-rung orthopair normal fuzzy aggregation operators and their application in multi attribute decision-making, Mathematics, 7 (2019), 1142. http://doi.org/10.3390/math7121142 doi: 10.3390/math7121142
![]() |
[35] |
R. R. Yager, N. Alajlan, Approximate reasoning with generalized orthopair fuzzy sets, Inform. Fusion., 38 (2017), 65–73. http://doi.org/10.1016/j.inffus.2017.02.005 doi: 10.1016/j.inffus.2017.02.005
![]() |
[36] |
M. I. Ali, Another view on q-rung orthopair fuzzy sets, Int. J. Intell. Syst., 33 (2019), 2139–2153. http://doi.org/10.1002/int.22007 doi: 10.1002/int.22007
![]() |
[37] |
P. Liu, P. Wang, Some q-rung orthopair fuzzy aggregation operators and their applications to multiple-attribute decision making, Int. J. Intell. Syst., 33 (2018), 259–280. http://doi.org/10.1002/int.21927 doi: 10.1002/int.21927
![]() |
[38] |
P. Liu, J. Liu, Some q-rung orthopai fuzzy Bonferroni mean operators and their application to multi-attribute group decision making, Int. J. Intell. Syst., 33 (2018), 315–347. http://doi.org/10.1002/int.21933 doi: 10.1002/int.21933
![]() |
[39] |
C. Jana, G. Muhiuddin, M. Pal, Some Dombi aggregation of q-rung orthopair fuzzy numbers in multiple-attribute decision making, Int. J. Intell. Syst., 34 (2019), 3220–3240. http://doi.org/10.1002/int.22191 doi: 10.1002/int.22191
![]() |
[40] |
J. Wang, R. Zhang, X. Zhu, Z. Zhou, X. Shang, W. Li, Some q-rung orthopair fuzzy Muirhead means with their application to multi-attribute group decision making, J. Intell. Fuzzy Syst., 36 (2019), 1599–1614. http://doi.org/10.3233/JIFS-18607 doi: 10.3233/JIFS-18607
![]() |
[41] |
M. S. Yang, C. H. Ko, On a class of fuzzy c-numbers clustering procedures for fuzzy data, Fuzzy Sets. Syst., 84 (1996), 49–60. http://doi.org/10.1016/0165-0114(95)00308-8 doi: 10.1016/0165-0114(95)00308-8
![]() |
[42] |
A. Hussain, M. I. Ali, T. Mahmood, Hesitant q-rung orthopair fuzzy aggregation operators with their applications in multi-criteria decision making, Iran. J. Fuzzy Syst., 17 (2020), 117–134. http://doi.org/10.22111/IJFS.2020.5353 doi: 10.22111/IJFS.2020.5353
![]() |
[43] |
A. Hussain, M. I. Ali, T. Mahmood, M. Munir, Group based generalized q-rung orthopair average aggregation operators and their applications in multi-criteria decision making, Complex Intell. Syst., 7 (2021), 123–144. http://doi.org/10.1007/s40747-020-00176-x doi: 10.1007/s40747-020-00176-x
![]() |
[44] | F. Smarandache, A unifying field in logics, Neutrosophy neutrosophic probability, set and logic, 2 Eds., Rehoboth: American Research Press, 1999. |
[45] |
J. Ye, Similarity measures between interval neutrosophic sets and their applications in Multi-criteria decision-making, J. Intell. Fuzzy Syst., 26 (2014), 165–172. http://doi.org/10.3233/IFS-120724 doi: 10.3233/IFS-120724
![]() |
[46] |
H. Zhang, J. Wang, X. Chen, Interval neutrosophic sets and its application in multi-criteria decision making problems, Sci. World J., 2014 (2014), 645953. http://doi.org/10.1155/2014/645953 doi: 10.1155/2014/645953
![]() |
[47] |
H. Bustince, P. Burillo, Vague sets are intuitionistic fuzzy sets, Fuzzy Sets Syst., 79 (1996), 403–405. http://doi.org/10.1016/0165-0114(95)00154-9 doi: 10.1016/0165-0114(95)00154-9
![]() |
[48] |
A. Kumar, S. P. Yadav, S. Kumar, Fuzzy system reliability analysis using T based arithmetic operations on LR type interval valued vague sets, Int. J. Qual. Reliab. Manag., 24 (2007), 846–860. http://doi.org/10.1108/02656710710817126 doi: 10.1108/02656710710817126
![]() |
[49] |
J. Wang, S. Y. Liu, J. Zhang, S. Y. Wang, On the parameterized OWA operators for fuzzy MCDM based on vague set theory, Fuzzy Optim. Decis. Making, 5 (2006), 5–20. http://doi.org/10.1007/s10700-005-4912-2 doi: 10.1007/s10700-005-4912-2
![]() |
[50] |
X. Zhang, Z. Xu, Extension of TOPSIS to multiple criteria decision-making with Pythagorean fuzzy sets, Int. J. Intell. Syst., 29 (2014), 1061–1078. http://doi.org/10.1002/int.21676 doi: 10.1002/int.21676
![]() |
[51] | C. L. Hwang, K. Yoon, Multiple Attribute Decision Making-Methods and Applications, A State-of-the-Art Survey, Berlin, Heidelberg: Springer, 1981. http://doi.org/10.1007/978-3-642-48318-9 |
[52] |
C. Jana, M. Pal, Application of bipolar intuitionistic fuzzy soft sets in decision-making problem, Int. J. Fuzzy Syst. Appl., 7 (2018), 32–55. http://doi.org/10.4018/IJFSA.2018070103 doi: 10.4018/IJFSA.2018070103
![]() |
[53] |
C. Jana, Multiple attribute group decision-making method based on extended bipolar fuzzy MABAC approach, Comput. Appl. Math., 40 (2021), 227. http://doi.org/10.1007/s40314-021-01606-3 doi: 10.1007/s40314-021-01606-3
![]() |
[54] |
C. Jana, M. Pal, A Robust single valued neutrosophic soft aggregation operators in multi criteria decision-making, Symmetry, 11 (2019), 110. http://doi.org/10.3390/sym11010110 doi: 10.3390/sym11010110
![]() |
[55] |
C. Jana, T. Senapati, M. Pal, Pythagorean fuzzy Dombi aggregation operators and its applications in multiple attribute decision-making, Int. J. Intell. Syst., 34 (2019), 2019–2038. http://doi.org/10.1002/int.22125 doi: 10.1002/int.22125
![]() |
[56] |
K. Ullah, T. Mahmood, Z. Ali, N. Jan, On some distance measures of complex Pythagorean fuzzy sets and their applications in pattern recognition, Comput. Intell. Syst., 6 (2020), 15–27. http://doi.org/10.1007/s40747-019-0103-6 doi: 10.1007/s40747-019-0103-6
![]() |
[57] |
C. Jana, M. Pal, F. Karaaslan, J. Q. Wang, Trapezoidal neutrosophic aggregation operators and their application to the multi-attribute decision-making process, Sci. Iran., 27 (2020), 1655–1673. http://doi.org/10.24200/SCI.2018.51136.2024 doi: 10.24200/SCI.2018.51136.2024
![]() |
[58] |
C. Jana, M. Pal, Multi criteria decision-making process based on some single valued neutrosophic dombi power aggregation operators, Soft Comput., 25 (2021), 5055–5072. http://doi.org/10.1007/s00500-020-05509-z doi: 10.1007/s00500-020-05509-z
![]() |
[59] |
M. Palanikumar, K. Arulmozhi, C. Jana, M. Pal, Multiple‐attribute decision-making spherical vague normal operators and their applications for the selection of farmers, Expert Syst., 40 (2023), e13188. http://doi.org/10.1111/exsy.13188 doi: 10.1111/exsy.13188
![]() |
[60] |
V. Uluçay, Q-neutrosophic soft graphs in operations management and communication network, Soft Comput., 25 (2021), 8441–8459. http://doi.org/10.1007/s00500-021-05772-8 doi: 10.1007/s00500-021-05772-8
![]() |
[61] |
V. Uluçay, Some concepts on interval-valued refined neutrosophic sets and their applications, J. Ambient Intell. Human. Comput., 12 (2021), 7857–7872. http://doi.org/10.1007/s12652-020-02512-y doi: 10.1007/s12652-020-02512-y
![]() |
[62] |
V. Uluçay, I. Deli, M. Şahin, Similarity measures of bipolar neutrosophic sets and their application to multiple criteria decision making, Neural Comput. Appl., 29 (2021), 739–748. http://doi.org/10.1007/s00521-016-2479-1 doi: 10.1007/s00521-016-2479-1
![]() |
[63] |
M. Sahin, N. Olgun, V. Uluçay, H. Acioglu, Some weighted arithmetic operators and geometric operators with SVNSs and their application to multi-criteria decision making problems, New Trends Neutrosophic Theory Appl., 2 (2018), 85–104. http://doi.org/10.5281/zenodo.1237953 doi: 10.5281/zenodo.1237953
![]() |
[64] | M. Sahin, N. Olgun, V. Uluçay, A. Kargin, F. Smarandache, A new similarity measure based on falsity value between single valued neutrosophic sets based on the centroid points of transformed single valued neutrosophic numbers with applications to pattern recognition, Neutrosophic Sets Syst., 15 (2017), 31–48. |
[65] |
Y. Lu, Y. Xu, E. H. Viedma, Consensus progress for large-scale group decision making in social networks with incomplete probabilistic hesitant fuzzy information, Appl. Soft Comput., 126 (2022), 109249. http://doi.org/10.1016/j.asoc.2022.109249 doi: 10.1016/j.asoc.2022.109249
![]() |
[66] |
Y. Lu, Y. Xu, J. Huang, J. Wei, E. H. Viedma, Social network clustering and consensus-based distrust behaviors management for large scale group decision-making with incomplete hesitant fuzzy preference relations, Appl. Soft Comput., 117 (2022), 108373. http://doi.org/10.1016/j.asoc.2021.108373 doi: 10.1016/j.asoc.2021.108373
![]() |
[67] |
C. Jana, G. Muhiuddin, M. Pal, Multi-criteria decision-making approach based on SVTrN Dombi aggregation functions. Artif. Intell. Rev., 54 (2021), 3685–3723. http://doi.org/10.1007/s10462-020-09936-0 doi: 10.1007/s10462-020-09936-0
![]() |
[68] |
M. Palanikumar, K. Arulmozhi, C. Jana, Multiple attribute decision-making approach for Pythagorean neutrosophic normal interval-valued aggregation operators, Comput. Appl. Math., 41 (2022), 90. http://doi.org/10.1007/s40314-022-01791-9 doi: 10.1007/s40314-022-01791-9
![]() |
[69] |
X. Peng, H. Yuan, Fundamental properties of Pythagorean fuzzy aggregation operators, Int. J. Intell. Syst. 31 (2016), 444–487. http://doi.org/10.1002/int.21790 doi: 10.1002/int.21790
![]() |
[70] |
B. Sarkar, M. Omair, N. Kim, A cooperative advertising collaboration policy in supply chain management under uncertain conditions, Appl. Soft Comput., 88 (2020), 105948. http://doi.org/10.1016/j.asoc.2019.105948 doi: 10.1016/j.asoc.2019.105948
![]() |
[71] |
U. Mishra, J. Z. Wu, B. Sarkar, Optimum sustainable inventory management with backorder and deterioration under controllable carbon emissions, J. Clean. Prod., 279 (2021), 123699. http://doi.org/10.1016/j.jclepro.2020.123699 doi: 10.1016/j.jclepro.2020.123699
![]() |
[72] |
B. Sarkar, M. Sarkar, B. Ganguly, L. E. Cárdenas-Barrón, Combined effects of carbon emission and production quality improvement for fixed lifetime products in a sustainable supply chain management, Int. J. Prod. Econ., 231 (2021), 107867. http://doi.org/10.1016/j.ijpe.2020.107867 doi: 10.1016/j.ijpe.2020.107867
![]() |
[73] |
U. Mishra, J. Z. Wu, B. Sarkar, A sustainable production-inventory model for a controllable carbon emissions rate under shortages, J. Clean. Prod., 256 (2020), 120268. http://doi.org/10.1016/j.jclepro.2020.120268 doi: 10.1016/j.jclepro.2020.120268
![]() |
[74] |
M. Ullah, B. Sarkar, Recovery-channel selection in a hybrid manufacturing-remanufacturing production model with RFID and product quality, Int. J. Prod. Econ., 219 (2020), 360–374. http://doi.org/10.1016/j.ijpe.2019.07.017 doi: 10.1016/j.ijpe.2019.07.017
![]() |
[75] |
M. Ullah, I. Asghar, M. Zahid, M. Omair, A. AlArjani, B. Sarkar, Ramification of remanufacturing in a sustainable three-echelon closed-loop supply chain management for returnable products, J. Clean. Prod., 290 (2021), 125609. http://doi.org/10.1016/j.jclepro.2020.125609 doi: 10.1016/j.jclepro.2020.125609
![]() |
1. | Madhumangal Pal, 2024, Chapter 10, 978-3-031-56935-7, 381, 10.1007/978-3-031-56936-4_10 | |
2. | Madhumangal Pal, 2024, Chapter 9, 978-3-031-56935-7, 357, 10.1007/978-3-031-56936-4_9 |
q=1 | q-log PyNVNWA | q-log PyNVNWG | q-log GPyNVNWA | q-log GPyNVNWG |
TOPSIS-\, ED | \mathscr{B}_{3}> \mathscr{B}_{1}> \mathscr{B}_{4} | \mathscr{B}_{3}> \mathscr{B}_{1}> \mathscr{B}_{5} | \mathscr{B}_{3}> \mathscr{B}_{1}> \mathscr{B}_{4} | \mathscr{B}_{3}> \mathscr{B}_{1}> \mathscr{B}_{5} |
({\bf Proposed}) | \mathscr{B}_{5}> \mathscr{B}_{2} | \mathscr{B}_{4}> \mathscr{B}_{2} | \mathscr{B}_{5}> \mathscr{B}_{2} | \mathscr{B}_{4}> \mathscr{B}_{2} |
TOPSIS-\, HD | \mathscr{B}_{3}> \mathscr{B}_{1}> \mathscr{B}_{4} | \mathscr{B}_{3}> \mathscr{B}_{1}> \mathscr{B}_{5} | \mathscr{B}_{3}> \mathscr{B}_{1}> \mathscr{B}_{4} | \mathscr{B}_{3}> \mathscr{B}_{1}> \mathscr{B}_{5} |
({\bf Proposed}) | \mathscr{B}_{5}> \mathscr{B}_{2} | \mathscr{B}_{4}> \mathscr{B}_{2} | \mathscr{B}_{5}> \mathscr{B}_{2} | \mathscr{B}_{4}> \mathscr{B}_{2} |
TOPSIS-\, ED | \mathscr{B}_{3}> \mathscr{B}_{1}> \mathscr{B}_{4} | \mathscr{B}_{3}> \mathscr{B}_{4}> \mathscr{B}_{1} | \mathscr{B}_{3}> \mathscr{B}_{1}> \mathscr{B}_{4} | \mathscr{B}_{3}> \mathscr{B}_{4}> \mathscr{B}_{1} |
[68] | \mathscr{B}_{5}> \mathscr{B}_{2} | \mathscr{B}_{5}> \mathscr{B}_{2} | \mathscr{B}_{5}> \mathscr{B}_{2} | \mathscr{B}_{5}> \mathscr{B}_{2} |
TOPSIS-\, HD | \mathscr{B}_{3}> \mathscr{B}_{1}> \mathscr{B}_{4} | \mathscr{B}_{3}> \mathscr{B}_{4}> \mathscr{B}_{1} | \mathscr{B}_{3}> \mathscr{B}_{1}> \mathscr{B}_{4} | \mathscr{B}_{3}> \mathscr{B}_{4}> \mathscr{B}_{1} |
[68] | \mathscr{B}_{5}> \mathscr{B}_{2} | \mathscr{B}_{5}> \mathscr{B}_{2} | \mathscr{B}_{5}> \mathscr{B}_{2} | \mathscr{B}_{5}> \mathscr{B}_{2} |
ED [31] | \mathscr{B}_{3}> \mathscr{B}_{1}> \mathscr{B}_{5} | \mathscr{B}_{3}> \mathscr{B}_{1}> \mathscr{B}_{4} | \mathscr{B}_{3}> \mathscr{B}_{1}> \mathscr{B}_{5} | \mathscr{B}_{3}> \mathscr{B}_{1}> \mathscr{B}_{4} |
\mathscr{B}_{4}> \mathscr{B}_{2} | \mathscr{B}_{5}> \mathscr{B}_{2} | \mathscr{B}_{4}> \mathscr{B}_{2} | \mathscr{B}_{5}> \mathscr{B}_{2} | |
HD [31] | \mathscr{B}_{3}> \mathscr{B}_{1}> \mathscr{B}_{5} | \mathscr{B}_{3}> \mathscr{B}_{1}> \mathscr{B}_{4} | \mathscr{B}_{3}> \mathscr{B}_{1}> \mathscr{B}_{5} | \mathscr{B}_{3}> \mathscr{B}_{1}> \mathscr{B}_{4} |
\mathscr{B}_{4}> \mathscr{B}_{2} | \mathscr{B}_{5}> \mathscr{B}_{2} | \mathscr{B}_{4}> \mathscr{B}_{2} | \mathscr{B}_{5}> \mathscr{B}_{2} | |
ED [59] | \mathscr{B}_{3}> \mathscr{B}_{1}> \mathscr{B}_{4} | \mathscr{B}_{3}> \mathscr{B}_{4}> \mathscr{B}_{1} | \mathscr{B}_{3}> \mathscr{B}_{1}> \mathscr{B}_{4} | \mathscr{B}_{3}> \mathscr{B}_{4}> \mathscr{B}_{1} |
\mathscr{B}_{5}> \mathscr{B}_{2} | \mathscr{B}_{5}> \mathscr{B}_{2} | \mathscr{B}_{5}> \mathscr{B}_{2} | \mathscr{B}_{5}> \mathscr{B}_{2} | |
HD [59] | \mathscr{B}_{3}> \mathscr{B}_{1}> \mathscr{B}_{4} | \mathscr{B}_{3}> \mathscr{B}_{4}> \mathscr{B}_{1} | \mathscr{B}_{3}> \mathscr{B}_{1}> \mathscr{B}_{4} | \mathscr{B}_{3}> \mathscr{B}_{4}> \mathscr{B}_{1} |
\mathscr{B}_{5}> \mathscr{B}_{2} | \mathscr{B}_{5}> \mathscr{B}_{2} | \mathscr{B}_{5}> \mathscr{B}_{2} | \mathscr{B}_{5}> \mathscr{B}_{2} | |
Score [59] | \mathscr{B}_{3}> \mathscr{B}_{1}> \mathscr{B}_{5} | \mathscr{B}_{3}> \mathscr{B}_{1}> \mathscr{B}_{4} | \mathscr{B}_{3}> \mathscr{B}_{1}> \mathscr{B}_{5} | \mathscr{B}_{3}> \mathscr{B}_{1}> \mathscr{B}_{4} |
\mathscr{B}_{4}> \mathscr{B}_{2} | \mathscr{B}_{5}> \mathscr{B}_{2} | \mathscr{B}_{4}> \mathscr{B}_{2} | \mathscr{B}_{5}> \mathscr{B}_{2} |
q=1 | q-log PyNVNWA | q-log PyNVNWG | q-log GPyNVNWA | q-log GPyNVNWG |
TOPSIS-\, ED | \mathscr{B}_{3}> \mathscr{B}_{1}> \mathscr{B}_{4} | \mathscr{B}_{3}> \mathscr{B}_{1}> \mathscr{B}_{5} | \mathscr{B}_{3}> \mathscr{B}_{1}> \mathscr{B}_{4} | \mathscr{B}_{3}> \mathscr{B}_{1}> \mathscr{B}_{5} |
({\bf Proposed}) | \mathscr{B}_{5}> \mathscr{B}_{2} | \mathscr{B}_{4}> \mathscr{B}_{2} | \mathscr{B}_{5}> \mathscr{B}_{2} | \mathscr{B}_{4}> \mathscr{B}_{2} |
TOPSIS-\, HD | \mathscr{B}_{3}> \mathscr{B}_{1}> \mathscr{B}_{4} | \mathscr{B}_{3}> \mathscr{B}_{1}> \mathscr{B}_{5} | \mathscr{B}_{3}> \mathscr{B}_{1}> \mathscr{B}_{4} | \mathscr{B}_{3}> \mathscr{B}_{1}> \mathscr{B}_{5} |
({\bf Proposed}) | \mathscr{B}_{5}> \mathscr{B}_{2} | \mathscr{B}_{4}> \mathscr{B}_{2} | \mathscr{B}_{5}> \mathscr{B}_{2} | \mathscr{B}_{4}> \mathscr{B}_{2} |
TOPSIS-\, ED | \mathscr{B}_{3}> \mathscr{B}_{1}> \mathscr{B}_{4} | \mathscr{B}_{3}> \mathscr{B}_{4}> \mathscr{B}_{1} | \mathscr{B}_{3}> \mathscr{B}_{1}> \mathscr{B}_{4} | \mathscr{B}_{3}> \mathscr{B}_{4}> \mathscr{B}_{1} |
[68] | \mathscr{B}_{5}> \mathscr{B}_{2} | \mathscr{B}_{5}> \mathscr{B}_{2} | \mathscr{B}_{5}> \mathscr{B}_{2} | \mathscr{B}_{5}> \mathscr{B}_{2} |
TOPSIS-\, HD | \mathscr{B}_{3}> \mathscr{B}_{1}> \mathscr{B}_{4} | \mathscr{B}_{3}> \mathscr{B}_{4}> \mathscr{B}_{1} | \mathscr{B}_{3}> \mathscr{B}_{1}> \mathscr{B}_{4} | \mathscr{B}_{3}> \mathscr{B}_{4}> \mathscr{B}_{1} |
[68] | \mathscr{B}_{5}> \mathscr{B}_{2} | \mathscr{B}_{5}> \mathscr{B}_{2} | \mathscr{B}_{5}> \mathscr{B}_{2} | \mathscr{B}_{5}> \mathscr{B}_{2} |
ED [31] | \mathscr{B}_{3}> \mathscr{B}_{1}> \mathscr{B}_{5} | \mathscr{B}_{3}> \mathscr{B}_{1}> \mathscr{B}_{4} | \mathscr{B}_{3}> \mathscr{B}_{1}> \mathscr{B}_{5} | \mathscr{B}_{3}> \mathscr{B}_{1}> \mathscr{B}_{4} |
\mathscr{B}_{4}> \mathscr{B}_{2} | \mathscr{B}_{5}> \mathscr{B}_{2} | \mathscr{B}_{4}> \mathscr{B}_{2} | \mathscr{B}_{5}> \mathscr{B}_{2} | |
HD [31] | \mathscr{B}_{3}> \mathscr{B}_{1}> \mathscr{B}_{5} | \mathscr{B}_{3}> \mathscr{B}_{1}> \mathscr{B}_{4} | \mathscr{B}_{3}> \mathscr{B}_{1}> \mathscr{B}_{5} | \mathscr{B}_{3}> \mathscr{B}_{1}> \mathscr{B}_{4} |
\mathscr{B}_{4}> \mathscr{B}_{2} | \mathscr{B}_{5}> \mathscr{B}_{2} | \mathscr{B}_{4}> \mathscr{B}_{2} | \mathscr{B}_{5}> \mathscr{B}_{2} | |
ED [59] | \mathscr{B}_{3}> \mathscr{B}_{1}> \mathscr{B}_{4} | \mathscr{B}_{3}> \mathscr{B}_{4}> \mathscr{B}_{1} | \mathscr{B}_{3}> \mathscr{B}_{1}> \mathscr{B}_{4} | \mathscr{B}_{3}> \mathscr{B}_{4}> \mathscr{B}_{1} |
\mathscr{B}_{5}> \mathscr{B}_{2} | \mathscr{B}_{5}> \mathscr{B}_{2} | \mathscr{B}_{5}> \mathscr{B}_{2} | \mathscr{B}_{5}> \mathscr{B}_{2} | |
HD [59] | \mathscr{B}_{3}> \mathscr{B}_{1}> \mathscr{B}_{4} | \mathscr{B}_{3}> \mathscr{B}_{4}> \mathscr{B}_{1} | \mathscr{B}_{3}> \mathscr{B}_{1}> \mathscr{B}_{4} | \mathscr{B}_{3}> \mathscr{B}_{4}> \mathscr{B}_{1} |
\mathscr{B}_{5}> \mathscr{B}_{2} | \mathscr{B}_{5}> \mathscr{B}_{2} | \mathscr{B}_{5}> \mathscr{B}_{2} | \mathscr{B}_{5}> \mathscr{B}_{2} | |
Score [59] | \mathscr{B}_{3}> \mathscr{B}_{1}> \mathscr{B}_{5} | \mathscr{B}_{3}> \mathscr{B}_{1}> \mathscr{B}_{4} | \mathscr{B}_{3}> \mathscr{B}_{1}> \mathscr{B}_{5} | \mathscr{B}_{3}> \mathscr{B}_{1}> \mathscr{B}_{4} |
\mathscr{B}_{4}> \mathscr{B}_{2} | \mathscr{B}_{5}> \mathscr{B}_{2} | \mathscr{B}_{4}> \mathscr{B}_{2} | \mathscr{B}_{5}> \mathscr{B}_{2} |