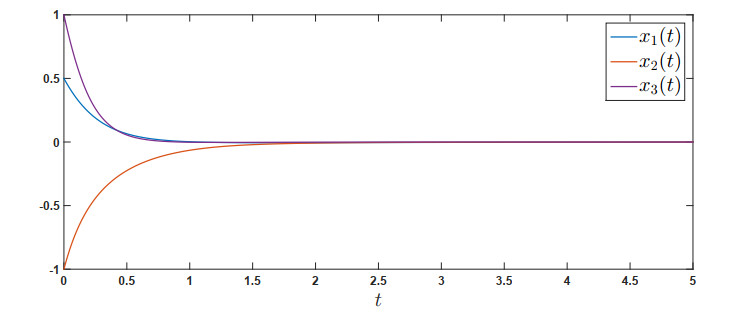
Of concern is the Hopfield neural network system comprising discrete as well as distributed delays in the form of a convolution. For a desired convergence rate of the solution to the equilibrium state, we establish sufficient conditions on the delay kernels ensuring this matter. Our result improves an existing one in the literature. The adopted approach is completely different. It relies on a judicious choice of a Lyapunov-like function and careful manipulations.
Citation: Mohammed D. Kassim. Controlling stability through the rate of decay of the delay feedback kernels[J]. AIMS Mathematics, 2023, 8(11): 26343-26356. doi: 10.3934/math.20231344
[1] | Qinghua Zhou, Li Wan, Hongbo Fu, Qunjiao Zhang . Exponential stability of stochastic Hopfield neural network with mixed multiple delays. AIMS Mathematics, 2021, 6(4): 4142-4155. doi: 10.3934/math.2021245 |
[2] | Ravi P. Agarwal, Snezhana Hristova . Stability of delay Hopfield neural networks with generalized proportional Riemann-Liouville fractional derivative. AIMS Mathematics, 2023, 8(11): 26801-26820. doi: 10.3934/math.20231372 |
[3] | Li Wan, Qinghua Zhou, Hongbo Fu, Qunjiao Zhang . Exponential stability of Hopfield neural networks of neutral type with multiple time-varying delays. AIMS Mathematics, 2021, 6(8): 8030-8043. doi: 10.3934/math.2021466 |
[4] | Jenjira Thipcha, Presarin Tangsiridamrong, Thongchai Botmart, Boonyachat Meesuptong, M. Syed Ali, Pantiwa Srisilp, Kanit Mukdasai . Robust stability and passivity analysis for discrete-time neural networks with mixed time-varying delays via a new summation inequality. AIMS Mathematics, 2023, 8(2): 4973-5006. doi: 10.3934/math.2023249 |
[5] | Jingjing Yang, Jianqiu Lu . Stabilization in distribution of hybrid stochastic differential delay equations with Lévy noise by discrete-time state feedback controls. AIMS Mathematics, 2025, 10(2): 3457-3483. doi: 10.3934/math.2025160 |
[6] | Sunisa Luemsai, Thongchai Botmart, Wajaree Weera, Suphachai Charoensin . Improved results on mixed passive and $ H_{\infty} $ performance for uncertain neural networks with mixed interval time-varying delays via feedback control. AIMS Mathematics, 2021, 6(3): 2653-2679. doi: 10.3934/math.2021161 |
[7] | Huahai Qiu, Li Wan, Zhigang Zhou, Qunjiao Zhang, Qinghua Zhou . Global exponential periodicity of nonlinear neural networks with multiple time-varying delays. AIMS Mathematics, 2023, 8(5): 12472-12485. doi: 10.3934/math.2023626 |
[8] | Mohammed D. Kassim . A fractional Halanay inequality for neutral systems and its application to Cohen-Grossberg neural networks. AIMS Mathematics, 2025, 10(2): 2466-2491. doi: 10.3934/math.2025115 |
[9] | Tian Xu, Ailong Wu . Stabilization of nonlinear hybrid stochastic time-delay neural networks with Lévy noise using discrete-time feedback control. AIMS Mathematics, 2024, 9(10): 27080-27101. doi: 10.3934/math.20241317 |
[10] | Chantapish Zamart, Thongchai Botmart, Wajaree Weera, Prem Junsawang . Finite-time decentralized event-triggered feedback control for generalized neural networks with mixed interval time-varying delays and cyber-attacks. AIMS Mathematics, 2023, 8(9): 22274-22300. doi: 10.3934/math.20231136 |
Of concern is the Hopfield neural network system comprising discrete as well as distributed delays in the form of a convolution. For a desired convergence rate of the solution to the equilibrium state, we establish sufficient conditions on the delay kernels ensuring this matter. Our result improves an existing one in the literature. The adopted approach is completely different. It relies on a judicious choice of a Lyapunov-like function and careful manipulations.
The problem of concern here is the following Hopfield neural network (HNN) system with two types of delays: discrete and distributed
{ϰ′i(t)=−ciϰi(t)+m∑j=1aijfj(ϰj(t))+m∑j=1bijfj(ϰj(t−τ))+m∑j=1dij∫∞0kj(s)fj(ϰj(t−s))ds+Ii,t>0,ϰi(t)=φi(t),t≤0, | (1.1) |
for i=1,2,…,m, where m is the number of existing units, ϰi are the state of the neuron number i at the instant t, ci>0 are the rates of the passive delay, aij,bij,dij denote the connection weight matrices, Ii stand for the external inputs assumed constants, fj are the activation functions, kj are the delay feedback kernels, τ>0 is the discrete delay and φi describe the history of the states.
The activation functions in the discrete and distributed delays are in general different but we are considering them here equal just for simplicity.
The continuous deterministic HNN is a recurrent artificial neural network that is used in many applications to model the dynamics of systems with a large number of inputs and unknown parameters. The first model introduced in [8] had the form
ϰ′i(t)=−ciϰi(t)+m∑j=1aijfj(ϰj(t)),t>0,i=1,2,…,m. |
Hopfield [8] introduced this continuous deterministic model to describe the time evolution of the state of electronic devices with a large number of amplifiers in conjunction with feedback circuits made up of wires, resistors and capacitors. Such circuits have integrative time delays due to capacitance. Since then, HNN has been used to describe various systems that occur in engineering, biology and economy [1,2,3,7,9,10,11,12,15,18,20,21,23].
Many complex processes with delays can be modeled as Hopfield neural network (HNN) systems with discrete and/or continuously distributed delays. Time retardation in electronic neural networks occur on account of the finite switching speed of amplifiers and can lead to instabilities in the form of oscillations [4,5,6,13,14,17,19,22,24,25,26,27,28].
Guo [6] analyzed the global asymptotic stability for (1.1) with piecewise continuous kernels. The global and local stability of the equilibrium states of (1.1) has been investigated under various conditions on the different coefficients, activation functions and delays [4,5,6,13,14,17,19,22,24,25,26,27,28]. In addition, there is an interest in determining the speed of convergence to the equilibrium states. For this purpose, various exponential stability results have been established, see for example [19]. In all these papers, the main condition for exponential asymptotic stability is ∫∞0eβsK(s)ds<∞ for some β>0 in addition to the standard condition of the dominance of the damping on the other coefficients [13,16,17,19,22,24,28].
Yin and Fu [25] studied the μ-stability issue for a class of NNs (1.1) subject to impulses with a diagonal K and unbounded time-varying lags. They used a Lyapunov-Krasovskii functional to derive some conditions in the form of linear matrix inequalities. The μ-stability, roughly means that the states converge asymptotically to equilibrium at the rate 1/μ(t) in a certain norm. Cui et al. [4] extended (1.1) to a reaction-diffusion cellular NN. The delays there were unbounded and time-varying and the distributed delays were bounded. In both papers, the function μ(t) must satisfy the conditions
μ′(t)μ(t)≤β1,μ(t−τ)μ(t)≥β2,∫∞0kj(s)μ(t+s)dsμ(t)≤β3,t>0, |
where β1,β2 and β3 are nonnegative scalars.
Zhang and Jin [26] established conditions for existence, uniqueness and global asymptotic stability of the stationary state of HNN with fixed or distributed time delays. The results apply in case the interconnection matrices are symmetric and nonsymmetric. The activation functions are continuous and non-monotonic functions.
The most important issue in this field is the stability of the equilibrium. The first results have been shown for simple HNNs with some specific activation functions like the Sigmoid function f(u) = 11+e−u/T, Hyperbolic tangent function f(u)=tanh(u/T), Inverse tangent function f(u)=2πtan−1(u/T), Threshold function f(u)={−1,u<0,1,u>0, Gaussian radial basis function f(u)=exp{−‖u−m‖2/σ2} and the Linear function f(u)=au+b. Because of the need in applications, these activation functions have been extended to bounded, monotone and differentiable functions. In turn, these conditions have been weakened to a mere Lipschitz continuity condition. These conditions on the monotonicity, boundedness and differentiability of the activation functions have been improved thereafter to simply a global (or local) Lipschitz continuity condition. There are also a fairly large number of papers dealing with different conditions on the different coefficients involved in the system. Indeed, for the parameters, the LMI method, M-Matrix and other techniques are very efficient. They have been used and improved in an impressive number of references that cannot fit in this limited size paper. Unfortunately, in spite of the many appearing cases in the applications (as mentioned in the book of Kosko), this issue has not received much attention. In this work, we want to fill this gap by establishing reasonable conditions on the kernels ensuring exponential stability and other types of stability.
The existence and uniqueness of the equilibrium has been discussed under different conditions and using different methods, such as fixed point theorems. Therefore, the well-posedness is guaranteed in our case as we are assuming standard conditions for the (dominance conditions of the) parameters and also on the activation functions (Lipschitz continuity), we obtain easily the existence and uniqueness of a solution as our kernels remain of (dissipative) fading memory type.
Here, the focus will be on the kernels kj(t) in problem (1.1). We extend the class of kernels satisfying
∫∞0kj(s)eβsds<∞,j=1,2,...,nfor some β>0, |
to a much wider class for which we have exponential stability as well as stability with general decay. Our result is proved under rather standard conditions on the other parameters and functions in the system but is ready to be adopted for more general situations. Indeed, in the existing papers, it is either kernels of exponential type or of subexponential type which are considered. In the present work, we do not use these assumptions. Instead, we assume the following condition: let ηj(t) be nonnegative continuous functions satisfying
limt→∞η(t):=limt→∞min1≤j≤nηj(t)=ˉη |
and
kj(t−s)≥ηj(t)∫∞tkj(σ−s)dσ,j=1,2,...,n,0≤s≤t. |
This new class of kernels is much wider than the existing one in the market. It contains the proper exponentially decaying functions. Moreover, it contains polynomially decaying functions and many more functions. Therefore, this improves earlier results and allows the treatment of more problems by allowing a larger class of admissible kernels. As consequence, the rates of stability are general and not necessarily exponential.
It is our objective here to derive sufficient conditions for stability with general rate, including as a special case, the exponential stability. Our results are obtained using new suitably selected functionals of Lyapunov-type in this theory and improve the existing results using completely different arguments. In view of the previous results, we shall assume the existence of continuously differentiable solutions.
In this part of the paper, we shall present our assumptions, definitions and useful lemmas.
We start with the presumptions:
(B1) The delay kernel functions kj are piecewise continuous nonnegative functions such that κj:=∫∞0kj(s)ds<∞.
(B2) The functions fi are Lipschitz continuous on R with Li, i=1,2,…,m as Lipschitz constants, that is
|fi(ϰ)−fi(y)|≤Li|ϰ−y|,∀ϰ,y∈R,i=1,2,…,m. |
(B3) The initial data φi(t), t≤0 are continuous functions.
Definition 1. The point ϰ∗=(ϰ∗1,…,ϰ∗m)T is called an equilibrium point of problem (1.1) if for i = 1,2,…,m,
ciϰ∗i=m∑j=1aijfj(ϰ∗j)+m∑j=1bijfj(ϰ∗j)+m∑j=1dij∫∞0kj(s)fj(ϰ∗j)ds+Ii=m∑j=1[aij+bij+dij∫∞0kj(s)ds]fj(ϰ∗j)+Ii,t>0. |
Definition 2. The equilibrium point ϰ∗ is said to be globally μ-stable if there exists a constant A>0 and a positive function μ(t) such that limt→∞μ(t)=∞ and
‖ϰ(t)−ϰ∗‖≤Aμ(t),t>0, |
where ‖⋅‖ denotes any norm in Rm.
The existence of a unique equilibrium for this kind of problems has been shown for instance in [26,27] when the functions fj are Lipschitz continuous. It has been proved also for 'Non-Lipschitz' continuous functions (see [5]).
These results apply for our case here. In fact, one can consult any result in Hopfield neural network theory even without (discrete and distributed) delays, as delays do not affect the proofs. As a matter of fact, they do not appear in the system satisfied by the equilibrium. However, there will be conditions on their coefficients.
This part is devoted to the study the stability of the equilibrium state ϰ∗ for (1.1). If we let
ϖ(t)=ϰ(t)−ϰ∗, |
then it is clear that the stability of ϰ∗ is equivalent to the stability of the zero state for the problem
{ϖ′i(t)=−ciϖi(t)+m∑j=1aijhj(ϖj(t))+m∑j=1bijhj(ϖj(t−τ)) +m∑j=1dij∫∞0kj(s)hj(ϖj(t−s))ds,t>0,i=1,2,…,m,ϖi(t)=ψi(t):=φi(t)−ϰ∗i,t≤0,i=1,2,…,m, | (3.1) |
where
hj(ϖj(t))=fj(ϖj(t)+ϰ∗j)−fj(ϰ∗j),t≥0. | (3.2) |
(B4) The initial data φi(t) are such that ψi∈L2(−∞,0), i=1,2,…,m.
To investigate the stability of the system (1.1), we employ the 'energy' functional
E(t):=m∑i=1ϖ2i(t),t≥0. | (3.3) |
The first lemma is a straightforward consequence of (B2) and (3.2).
Lemma 1. Let assumption (B2) hold. Then
2|ϖi(t)hj(ϖj(t))|≤ϖ2i(t)+L2jϖ2j(t),t>0,i,j=1,2,…,m |
and
2|ϖi(t)hj(ϖj(t−τ))|≤ϖ2i(t)+L2jϖ2j(t−τ),t>0,i,j=1,2,…,m. |
Lemma 2. Let presumptions (B1)–(B3) hold. Then for t≥0
E′(t)≤m∑j=1{−2ci+m∑j=1[aij+L2iaji+bij+dij]}ϖ2i(t)+m∑j=1λ1jϖ2j(t−τ)+m∑j=1λ2j∫∞0kj(s)ϖ2j(t−s)ds, |
where
λ1j=(m∑i=1bij)L2j,λ2j=(m∑i=1dij)L2jκj,j=1,2,…,m. | (3.4) |
Proof. The differentiation of E(t) in (3.3), along solutions of (3.1), yields for t≥0
E′(t)=2m∑i=1[−ciϖ2i(t)+m∑j=1aijϖi(t)hj(ϖj(t))+m∑j=1bijϖi(t)hj(ϖj(t−τ))+m∑j=1dijϖi(t)∫∞0kj(s)hj(ϖj(t−s))ds]. |
By Lemma 2 we can write for t≥0
E′(t)≤−2m∑i=1ciϖ2i(t)+m∑i,j=1aij[ϖ2i(t)+L2jϖ2j(t)]+m∑i,j=1bij[ϖ2i(t)+L2jϖ2j(t−τ)]+m∑i,j=1dij[ϖ2i(t)+(∫∞0kj(s)Lj|ϖj(t−s)|ds)2]. |
From Cauchy-Schwartz inequality we have the bound
(∫∞0kj(s)Ljϖj(t−s)ds)2≤∫∞0kj(s)ds∫∞0kj(s)L2jϖ2j(t−s)ds≤L2jκj∫∞0kj(s)ϖ2j(t−s)ds,t≥0. |
Consequently, for t≥0
E′(t)≤m∑i=1[−2ci+m∑j=1aij+L2im∑j=1aji+m∑j=1bij+m∑j=1dij]ϖ2i(t)+m∑i,j=1bijL2jϖ2j(t−τ)+m∑i,j=1dijL2jκj∫∞0kj(s)ϖ2j(t−s)ds=m∑i=1[−2ci+m∑j=1aij+L2im∑j=1aji+m∑j=1bij+m∑j=1dij]ϖ2i(t)+m∑j=1(m∑i=1bij)L2jϖ2j(t−τ)+m∑j=1(m∑i=1dij)L2jκj∫∞0kj(s)ϖ2j(t−s)ds=m∑i=1[−2ci+m∑j=1aij+L2im∑j=1aji+m∑j=1bij+m∑j=1dij]ϖ2i(t)+m∑j=1λ1jϖ2j(t−τ)+m∑j=1λ2j∫∞0kj(s)ϖ2j(t−s)ds. |
Theorem 1. Let assumptions (B1)–(B4) hold. If
m∑j=1[aij+bij+dij+L2i(aji+bji+κ2idji)]<2ci,i=1,2,…,m, |
then E(t) is uniformly bounded.
Proof. Consider the functionals
V1(t):=m∑j=1λ1j∫tt−τϖ2j(s)ds,t≥0 | (3.5) |
and
V2(t):=m∑j=1λ2j∫t−∞(∫∞tkj(σ−s)dσ)ϖ2j(s)ds=m∑j=1λ2j∫∞0kj(s)∫tt−sϖ2j(σ)dσds,t≥0. | (3.6) |
Note that
V1(0)=m∑j=1λ1j∫0−τϖ2j(s)ds=m∑j=1λ1j∫0−τψ2j(s)ds<∞ |
and
V2(0)=∞∑j=1λ2j∫∞0kj(s)∫0−sψ2j(σ)dσds<∞. |
Moreover,
V′1(t)=m∑j=1λ1j[ϖ2j(t)−ϖ2j(t−τ)],t≥0 | (3.7) |
and
V′2(t)=m∑j=1λ2j(∫∞tkj(σ−t)dσ)ϖ2j(t)−m∑j=1λ2j∫t−∞kj(t−s)ϖ2j(s)ds=m∑j=1λ2j(∫∞0kj(s)ds)ϖ2j(t)−m∑j=1λ2j∫∞0kj(s)ϖ2j(t−s)ds=m∑j=1λ2jκjϖ2j(t)−m∑j=1λ2j∫∞0kj(s)ϖ2j(t−s)ds,t≥0. | (3.8) |
Let
E(t)=E(t)+V1(t)+V2(t),t≥0. | (3.9) |
Then, E(0)<∞ and
E′(t)=E′(t)+V′1(t)+V′2(t)≤m∑i=1[−2ci+m∑j=1aij+L2im∑j=1aji+m∑j=1bij+m∑j=1dij]ϖ2i(t)+m∑j=1λ1jϖ2j(t−τ)+m∑j=1λ2j∫∞0kj(s)ϖ2j(t−s)ds+m∑j=1λ1j[ϖ2j(t)−ϖ2j(t−τ)]+m∑j=1λ2jκjϖ2j(t)−m∑j=1λ2j∫∞0kj(s)ϖ2j(t−s)ds |
or for t≥0,
E′(t)≤m∑i=1[−2ci+m∑j=1(aij+L2iaji+bij+dij)]ϖ2i(t)+m∑i=1λ1iϖ2i(t)+m∑i=1λ2iκiϖ2i(t). |
This may be rewritten simply as
E′(t)≤m∑i=1{−2ci+m∑j=1[aij+L2iaji+bij+dij+L2ibji+L2iκ2idji]}ϖ2i(t)=m∑i=1{−2ci+m∑j=1[aij+bij+dij+L2i(aji+bji+κ2idji)]}ϖ2i(t),t≥0. | (3.10) |
From the condition stated in the theorem and (3.10) we see that E′(t)≤0, t≥0. Therefore,
E(t)≤E(t)≤E(0),t≥0. |
The proof is complete.
We now specify our main condition on the kernels
(B5) There are nonnegative continuous functions ηj(t) such that
limt→∞η(t):=limt→∞min1≤j≤mηj(t)=ˉη |
and
kj(t−s)≥ηj(t)∫∞tkj(σ−s)dσ,j=1,2,…,m,0≤s≤t. |
Theorem 2. Let assumptions (B1)–(B5) hold and
2ci>m∑j=1{aij+bij+dij+L2i[aji+(1+ε)bji+2κ2idji]},i=1,2,…,m, |
for some ε>0. Then, if limt→∞η(t)=ˉη=0 we have
E(t)≤C1e−C2∫t0η(s)ds,t≥0 |
and
E(t)≤C3e−C4t,t≥0 |
in case 0<ˉη≤∞, for some positive constants Ci, i=1,2,3,4.
Remark 1. If η(t)=μ′(t)μ(t) for some differentiable function μ(t), then we obtain
E(t)≤A|μ(t)|σ,t≥0, |
for some positive constants A and σ.
Proof Theorem 2. For 0<δ<1/2, consider the functional
˜E(t):=E(t)+V3(t)+11−δV2(t),t≥0, | (3.11) |
where
V3(t):=e−βtm∑j=1λ1j∫tt−τeβ(s+τ)ϖ2j(s)ds,t≥0,β>0, |
λ1j as in (3.4), and V2 as in (3.6). Here β is selected so small that eβτ≤1+ε (ε is in the statement of the theorem).
By direct differentiation we have
V′3(t)=−βV3(t)+eβτm∑j=1λ1jϖ2j(t)−m∑j=1λ1jϖ2j(t−τ),t≥0. | (3.12) |
Next, we estimate V′2(t) in light of our new assumption (B5) on the kernels. Clearly, for t≥0,
V′2(t)=m∑j=1λ2jκjϖ2j(t)−m∑j=1λ2j∫t−∞kj(t−s)ϖ2j(s)ds=m∑j=1λ2jκjϖ2j(t)−δm∑j=1λ2j∫t−∞kj(t−s)ϖ2j(s)ds−(1−δ)m∑j=1λ2j∫t−∞kj(t−s)ϖ2j(s)ds≤m∑j=1λ2jκjϖ2j(t)−δm∑j=1λ2jηj(t)∫t−∞(∫∞tkj(σ−s)dσ)ϖ2j(s)ds−(1−δ)m∑j=1λ2j∫t−∞kj(t−s)ϖ2j(s)ds≤m∑j=1λ2jκjϖ2j(t)−δη(t)V3(t)−(1−δ)m∑j=1λ2j∫t−∞kj(t−s)ϖ2j(s)ds. | (3.13) |
Taking into account (3.11)–(3.13), the differentiation along solutions of (3.1) yields for t≥0
˜E′(t)≤m∑i=1[−2ci+m∑j=1(aij+L2iaji+bij+dij)]ϖ2i(t)+m∑j=1λ1jϖ2j(t−τ)+m∑j=1λ2j∫∞0kj(s)ϖ2j(t−s)ds+eβτm∑j=1λ1jϖ2j(t)−βV3(t)−m∑j=1λ1jϖ2j(t−τ)+11−δ{m∑j=1λ2jκjϖ2j(t)−δη(t)V2(t)}−m∑j=1λ2j∫t−∞kj(t−s)ϖ2j(s)ds, |
or
˜E′(t)≤m∑i=1[−2ci+m∑j=1(aij+L2iaji+bij+dij)]ϖ2i(t)+m∑j=1[eβτλ1j+λ2jκj1−δ]ϖ2j(t)−βV3(t)−δ1−δη(t)V2(t). |
In view of (3.4), we find for t≥0
˜E′(t)≤m∑i=1[−2ci+m∑j=1(aij+L2iaji+bij+dij)]ϖ2i(t)+m∑j=1[eβτ(m∑i=1bij)L2j+κj1−δ(m∑i=1dij)L2jκj]ϖ2j(t)−βV3(t)−δ1−δη(t)V2(t), |
or
˜E′(t)≤m∑i=1{−2ci+m∑j=1[aij+bij+dij+L2i(aji+eβτbji+κ2i1−δdji)]}ϖ2i(t)−βV3(t)−δ1−δη(t)V2(t)≤−αE(t)−βV3(t)−δ1−δη(t)V2(t),t≥0, | (3.14) |
where
α=min1≤i≤m{2ci−m∑j=1[aij+bij+dij+L2i(aji+eβτbji+κ2i1−δdji)]}. |
From the hypotheses we have α>0.
We discuss two cases:
Case 1. limt→∞η(t)=0
Let t∗>0 be large enough so that
η(t)≤1δmin{α,β},t≥t∗. | (3.15) |
Therefore
˜E′(t)≤−αE(t)−βV3(t)−δ1−δη(t)V2(t)≤−δη(t)E(t)−δη(t)V3(t)−δ1−δη(t)V2(t)≤−δη(t)˜E(t),t≥t∗. |
This implies that
˜E(t)≤˜E(t∗)e−δ∫tt∗η(s)ds,t≥t∗. |
By continuity and Theorem 1, we may derive a similar estimate on [0,t∗].
Case 2. 0<ˉη≤∞
In this case
∃t∗>0s.t.η(t)≥ˉη2,∀t≥t∗. | (3.16) |
In case ˉη=+∞, we consider any positive constant ξ, η(t)≥ξ.
In view of (3.14) and (3.16), we see that
˜E′(t)≤−αE(t)−βV3(t)−δ1−δˉη2V2(t)≤−γ˜E(t),t≥t∗, |
where
γ=min{α,β,δˉη2}>0. |
Therefore,
˜E(t)≤˜E(t∗)e−γ(t−t∗),t≥t∗. |
A continuity argument and Theorem 1 gives a similar estimates on [0,t∗]. The proof is complete.
In this section, we shall present numerical examples validating the efficiency of the above theoretical results.
Example 1. Consider the following Hopfield neural network system, composed of three neurons
ϰ′i(t)=−ciϰi(t)+3∑j=1aijfj(ϰj(t))+3∑j=1bijfj(ϰj(t−τ))+3∑j=1dij∫∞0kj(s)fj(ϰj(t−s))ds+Ii,t>0,i=1,2,3, | (4.1) |
where the associated functions and parameters are selected as follows:
f1(x)=18(|x+1|−|x−1|),f2(x)=14tanh(x),f3(x)=14tanh(0.5x), ci=2,ki(t)=116e−√1+t,Ii=0,i=1,2,3,ϕ1(x)=0.5,ϕ2(x)=−1,ϕ3(x)=1,x∈[−1,0],a11=0.15,a12=0.12,a13=0.17,a21=0.16,a22=0.18,a23=0.2,a31=0.14,a32=0.16,a33=0.12,b11=0.17,b12=0.15,b13=0.13,b21=0.18,b22=0.12,b23=0.11,b31=0.13,b32=0.19,b33=0.16,d11=0.14,d12=0.2,d13=0.18,d21=0.16,d22=0.17,d23=0.14,d31=0.15,d32=0.14,d33=0.2,τ=1. |
Through some simple calculations, we get L1=L2=L3=14,κi=14e, and we choose ηi(t) = 14(1+√1+t),i=1,2,3.
Hence, the assumptions (B1)–(B5) are met. By virtue of Theorem 2, then the solutions of the system (4.1) decay to the stationary states. These can be depicted in Figure 1.
Example 2. Consider the system (4.1) in which the related functions and parameters are chosen as
fi(x)=116tanh(x),ki(t)=1(1+x)2, ci=5, Ii=0,i=1,2,3,ϕ1(x)=0.25,ϕ2(x)=0.75,ϕ3(x)=−0.5,x∈[−2,0],a11=1,a12=0.25,a13=0.75,a21=0.5,a22=1,a23=0.4,a31=0.6,a32=0.3,a33=1,b11=0.8,b12=0.25,b13=1,b21=0.75,b22=0.5,b23=0.3,b31=0.6,b32=1,b33=0.25,d11=0.75,d12=1,d13=0.45,d21=0.5,d22=0.8,d23=1,d31=0.5,d32=0.75,d33=0.25,τ=2. |
Via a simple calculation, we obtain L1=L2=L3=116,κi=1,ηi(t)=12(1+t),i=1,2,3.
Therefore, as the hypotheses (B1)–(B5) of Theorem 2 are fulfilled, the solutions of the system (4.1) decay to steady points. We can see these in Figure 2.
The authors declare they have not used Artificial Intelligence (AI) tools in the creation of this article.
The authors declare no conflict of interest.
[1] |
A. Bouzerdoum, T. Pattison, Neural network for quadratic optimization with bound constraints, IEEE Trans. Neural Networ., 4 (1993), 293–304. http://dx.doi.org/10.1109/72.207617 doi: 10.1109/72.207617
![]() |
[2] |
L. Chua, T. Roska, Stability of a class of nonreciprocal cellular neural networks, IEEE Trans. Circuits-I, 37 (1990), 1520–1527. http://dx.doi.org/10.1109/31.101272 doi: 10.1109/31.101272
![]() |
[3] |
B. Crespi, Storage capacity of non-monotonic neurons, Neural Networks, 12 (1999), 1377–1389. http://dx.doi.org/10.1016/S0893-6080(99)00074-X doi: 10.1016/S0893-6080(99)00074-X
![]() |
[4] |
H. Cui, J. Guo, J. Feng, T. Wang, Global μ-stability of impulsive reaction-diffusion neural networks with unbounded time-varying delays and bounded continuously distributed delays, Neurocomputing, 157 (2015), 1–10. http://dx.doi.org/10.1016/j.neucom.2015.01.044 doi: 10.1016/j.neucom.2015.01.044
![]() |
[5] |
C. Feng, R. Plamondon, On the stability analysis of delayed neural network systems, Neural Networks, 14 (2001), 1181–1188. http://dx.doi.org/10.1016/S0893-6080(01)00088-0 doi: 10.1016/S0893-6080(01)00088-0
![]() |
[6] |
Y. Guo, Global asymptotic stability analysis for integro-differential systems modeling neural networks with delays, Z. Angew. Math. Phys., 61 (2010), 971–978. http://dx.doi.org/10.1007/s00033-009-0057-4 doi: 10.1007/s00033-009-0057-4
![]() |
[7] |
J. Hopfield, Neural networks and physical systems with emergent collective computational abilities, PNAS, 79 (1982), 2554–2558. http://dx.doi.org/10.1073/pnas.79.8.2554 doi: 10.1073/pnas.79.8.2554
![]() |
[8] |
J. Hopfield, Neurons with graded response have collective computational properties like those of two-state neurons, PNAS, 81 (1984), 3088–3092. http://dx.doi.org/10.1073/pnas.81.10.3088 doi: 10.1073/pnas.81.10.3088
![]() |
[9] |
J. Hopfield, D. Tank, Computing with neural circuits: a model, Science, 233 (1986), 625–633. http://dx.doi.org/10.1126/science.3755256 doi: 10.1126/science.3755256
![]() |
[10] |
J. Inoue, Retrieval phase diagrams of non-monotonic Hopfield networks, J. Phys. A: Math. Gen., 29 (1996), 4815–4826. http://dx.doi.org/10.1088/0305-4470/29/16/008 doi: 10.1088/0305-4470/29/16/008
![]() |
[11] |
M. Kennedy, L. Chua, Neural networks for non-linear programming, IEEE Trans. Circuits-I, 35 (1998), 554–562. http://dx.doi.org/10.1109/31.1783 doi: 10.1109/31.1783
![]() |
[12] | B. Kosko, Neural networks and fuzzy systems: a dynamical systems approach to machine intelligence, New Jersey: Prentice-Hall, 1991. |
[13] |
B. Liu, W. Lu, T. Chen, New criterion of asymptotic stability for delay systems with time-varying structures and delays, Neural Networks, 54 (2014), 103–111. http://dx.doi.org/10.1016/j.neunet.2014.03.003 doi: 10.1016/j.neunet.2014.03.003
![]() |
[14] |
T. Loan, D. Tuan, Global exponential stability of a class of neural networks with unbounded delays, Ukr. Math. J., 60 (2008), 1633–1649. http://dx.doi.org/10.1007/s11253-009-0155-7 doi: 10.1007/s11253-009-0155-7
![]() |
[15] |
S. Mohamad, Exponential stability in Hopfield-type neural networks with impulses, Chaos Soliton. Fract., 32 (2007), 456–467. http://dx.doi.org/10.1016/j.chaos.2006.06.035 doi: 10.1016/j.chaos.2006.06.035
![]() |
[16] |
S. Mohamed, K. Gopalsamy, Continuous and discrete Halanay-type inequalities, Bull. Aust. Math. Soc., 61 (2000), 371–385. http://dx.doi.org/10.1017/S0004972700022413 doi: 10.1017/S0004972700022413
![]() |
[17] |
S. Mohamad, K. Gopalsamy, H. Akca, Exponential stability of artificial neural networks with distributed delays and large impulses, Nonlinear Anal.-Real, 9 (2008), 872–888. http://dx.doi.org/10.1016/j.nonrwa.2007.01.011 doi: 10.1016/j.nonrwa.2007.01.011
![]() |
[18] |
H. Qiao, J. Peng, Z. Xu, Nonlinear measures: a new approach to exponential stability analysis for Hopfield-type neural networks, IEEE Trans. Neural Networ., 12 (2001), 360–370. http://dx.doi.org/10.1109/72.914530 doi: 10.1109/72.914530
![]() |
[19] |
Q. Song, Z. Zhao, Global dissipativity of neural networks with both variable and unbounded delays, Chaos Soliton. Fract., 25 (2005), 393–401. http://dx.doi.org/10.1016/j.chaos.2004.11.035 doi: 10.1016/j.chaos.2004.11.035
![]() |
[20] |
S. Sudharsanan, M. Sundareshan, Exponential stability and a systematic synthesis of a neural network for quadratic minimization, Neural Networks, 4 (1991), 599–613. http://dx.doi.org/10.1016/0893-6080(91)90014-V doi: 10.1016/0893-6080(91)90014-V
![]() |
[21] |
P. van den Driessche, X. Zou, Global attractivity in delayed Hopfield neural network models, SIAM J. Appl. Math., 58 (1998), 1878–1890. http://dx.doi.org/10.1137/S0036139997321219 doi: 10.1137/S0036139997321219
![]() |
[22] |
Y. Wang, W. Xiong, Q. Zhou, B. Xiao, Y. Yu, Global exponential stability of cellular neural networks with continuously distributed delays and impulses, Phys. Lett. A, 350 (2006), 89–95. http://dx.doi.org/10.1016/j.physleta.2005.10.084 doi: 10.1016/j.physleta.2005.10.084
![]() |
[23] |
H. Yanai, S. Ammari, Auto-associative memory with two stage dynamics of non-monotonic neurons, IEEE Trans. Neural Networ., 7 (1996), 803–815. http://dx.doi.org/10.1109/72.508925 doi: 10.1109/72.508925
![]() |
[24] | L. Yin, Y. Chen, Y. Zhao, Global exponential stability for a class of neural networks with continuously distributed delays, Advances in Dynamical Systems and Applications, 4 (2009), 221–229. |
[25] | L. Yin, X. Fu, μ-stability of impulsive neural networks with unbounded time-varying delays and continuously distributed delays, Adv. Differ. Equ., 2011 (2011), 437842. http://dx.doi.org/0.1155/2011/437842 |
[26] |
J. Zhang, X. Jin, Global stability analysis in delayed hopfield neural network models, Neural Networks, 13 (2000), 745–753. http://dx.doi.org/10.1016/S0893-6080(00)00050-2 doi: 10.1016/S0893-6080(00)00050-2
![]() |
[27] |
J. Zhang, Y. Suda, T. Iwasa, Absolutely exponential stability of a class of neural networks with unbounded delay, Neural Networks, 17 (2004), 391–397. http://dx.doi.org/10.1016/j.neunet.2003.09.005 doi: 10.1016/j.neunet.2003.09.005
![]() |
[28] |
J. Zhou, S. Li, Z. Yang, Global exponential stability of Hopfield neural networks with distributed delays, Appl. Math. Model., 33 (2009), 1513–1520. http://dx.doi.org/10.1016/j.apm.2008.02.006 doi: 10.1016/j.apm.2008.02.006
![]() |