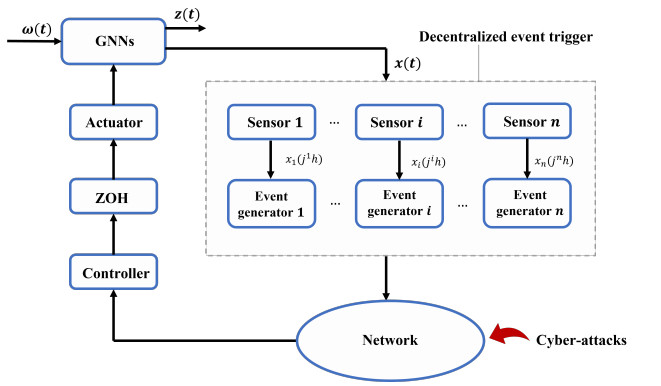
This article investigates the finite-time decentralized event-triggered feedback control problem for generalized neural networks (GNNs) with mixed interval time-varying delays and cyber-attacks. A decentralized event-triggered method reduces the network transmission load and decides whether sensor measurements should be sent out. The cyber-attacks that occur at random are described employing Bernoulli distributed variables. By the Lyapunov-Krasovskii stability theory, we apply an integral inequality with an exponential function to estimate the derivative of the Lyapunov-Krasovskii functionals (LKFs). We present new sufficient conditions in the form of linear matrix inequalities. The main objective of this research is to investigate the stochastic finite-time boundedness of GNNs with mixed interval time-varying delays and cyber-attacks by providing a decentralized event-triggered method and feedback controller. Finally, a numerical example is constructed to demonstrate the effectiveness and advantages of the provided control scheme.
Citation: Chantapish Zamart, Thongchai Botmart, Wajaree Weera, Prem Junsawang. Finite-time decentralized event-triggered feedback control for generalized neural networks with mixed interval time-varying delays and cyber-attacks[J]. AIMS Mathematics, 2023, 8(9): 22274-22300. doi: 10.3934/math.20231136
[1] | Zhaohui Chen, Jie Tan, Yong He, Zhong Cao . Decentralized observer-based event-triggered control for an interconnected fractional-order system with stochastic Cyber-attacks. AIMS Mathematics, 2024, 9(1): 1861-1876. doi: 10.3934/math.2024091 |
[2] | Sheng-Ran Jia, Wen-Juan Lin . Adaptive event-triggered reachable set control for Markov jump cyber-physical systems with time-varying delays. AIMS Mathematics, 2024, 9(9): 25127-25144. doi: 10.3934/math.20241225 |
[3] | Yidan Wang, Li Xiao, Yanfeng Guo . Finite-time stability of singular switched systems with a time-varying delay based on an event-triggered mechanism. AIMS Mathematics, 2023, 8(1): 1901-1924. doi: 10.3934/math.2023098 |
[4] | Rakkiet Srisuntorn, Wajaree Weera, Thongchai Botmart . Modified function projective synchronization of master-slave neural networks with mixed interval time-varying delays via intermittent feedback control. AIMS Mathematics, 2022, 7(10): 18632-18661. doi: 10.3934/math.20221025 |
[5] | Jiashu Gao, Jing Han, Guodong Zhang . Event-triggered fixed/preassigned time stabilization of state-dependent switching neural networks with mixed time delays. AIMS Mathematics, 2024, 9(4): 9211-9231. doi: 10.3934/math.2024449 |
[6] | Sunisa Luemsai, Thongchai Botmart, Wajaree Weera, Suphachai Charoensin . Improved results on mixed passive and $ H_{\infty} $ performance for uncertain neural networks with mixed interval time-varying delays via feedback control. AIMS Mathematics, 2021, 6(3): 2653-2679. doi: 10.3934/math.2021161 |
[7] | Yahan Deng, Zhenhai Meng, Hongqian Lu . Adaptive event-triggered state estimation for complex networks with nonlinearities against hybrid attacks. AIMS Mathematics, 2022, 7(2): 2858-2877. doi: 10.3934/math.2022158 |
[8] | Chengbo Yi, Rui Guo, Jiayi Cai, Xiaohu Yan . Pinning synchronization of dynamical neural networks with hybrid delays via event-triggered impulsive control. AIMS Mathematics, 2023, 8(10): 25060-25078. doi: 10.3934/math.20231279 |
[9] | Chao Ma, Tianbo Wang, Wenjie You . Master-slave synchronization of Lurie systems with time-delay based on event-triggered control. AIMS Mathematics, 2023, 8(3): 5998-6008. doi: 10.3934/math.2023302 |
[10] | Tiecheng Zhang, Liyan Wang, Yuan Zhang, Jiangtao Deng . Finite-time stability for fractional-order fuzzy neural network with mixed delays and inertial terms. AIMS Mathematics, 2024, 9(7): 19176-19194. doi: 10.3934/math.2024935 |
This article investigates the finite-time decentralized event-triggered feedback control problem for generalized neural networks (GNNs) with mixed interval time-varying delays and cyber-attacks. A decentralized event-triggered method reduces the network transmission load and decides whether sensor measurements should be sent out. The cyber-attacks that occur at random are described employing Bernoulli distributed variables. By the Lyapunov-Krasovskii stability theory, we apply an integral inequality with an exponential function to estimate the derivative of the Lyapunov-Krasovskii functionals (LKFs). We present new sufficient conditions in the form of linear matrix inequalities. The main objective of this research is to investigate the stochastic finite-time boundedness of GNNs with mixed interval time-varying delays and cyber-attacks by providing a decentralized event-triggered method and feedback controller. Finally, a numerical example is constructed to demonstrate the effectiveness and advantages of the provided control scheme.
Neural networks (NNs) are widely used in various fields, such as pattern recognition, combinatorial optimization, image processing, associate memory, signal processing, and fixed-point computations [1,2,3,4], due to their enormous capacity for information processing. The literatures [5,6,7,8,9,10,11,12] classifies neural networks into two categories. The first category consists of static neural networks (SNNs), which rely on the external states of neurons (neural states of neurons). The second category consists of local field neural networks (LFNNs), which depend on the internal states of neurons (local field states). In recent years, Zhang and Han [13] introduced a novel approach by combining SNNs and LFNNs to create a unified system of neural networks called generalized neural networks (GNNs). NNs may encounter a delay during execution caused by either the communication time of neurons or the finite switching speed of the neuron amplifiers. These time delays can have a detrimental effect on the performance of NNs, resulting in either instability, divergence, or oscillation. Consequently, there has been an increased focus on investigating the stability of GNNs under time delay [12,13,14,15,16,17,18]. Delayed GNNs are classified into various types: distributed delay, mixed delays, constant delay, interval time-varying delay and time-varying delay.
Event-triggered schemes are control strategies that minimize the amount of data transmitted or processed in computers and control systems. These schemes only transmit data when specific events occur, such as system state changes, errors, or specific time intervals. The event-triggered mechanism is a promising technique in networked control systems that aims to reduce communication costs and computational burden, while ensuring good control performance. This technique is necessary since the energy of sensors and network bandwidth is limited and needs to be used efficiently to accomplish other communication tasks. The event-triggered method only transmits signals when they meet the triggering threshold, conserving energy and improving communication bandwidth, as shown by the beneficial outcomes of event-triggered schemes. This success has led to its widespread usage in NNs [19,20,21,22,23,24,25,26]. For instance, Liu et al. [20] investigated a dissipativity-based synchronization method for Markovian jump NNs under the event-triggered framework. Later, Zha et al. [22] presented the problem of H∞ control for NNs with time-varying delay and cyber-attacks under the event-triggered framework.
Cyber-attacks in networked control systems are malicious and unauthorized activities that target the communication network or control algorithms. These attacks aim to either disrupt normal system operations, steal data, or cause physical damage. Such attacks can result in significant losses, making the security and resilience of networked control systems against cyber-attacks a crucial area of research and practice. Over the past few years, several researchers [22,25,27,28,29,30] have placed a considerable emphasis on the problem of cyber-attacks in networked control systems. For example, Liu et al. [30] investigated the state estimation method for T-S fuzzy neural networks under stochastic cyber-attacks and an event-triggered scheme. Recently, a technique of the networked control for neural networks vulnerable to two different types of stochastic cyber-attacks has been proposed by Feng et al. [25]. This approach utilizes a decentralized event-triggered H∞ control.
Stability analysis is a crucial concept in control theory, which usually concentrates on a system's asymptotic behavior over an infinite time interval. However, achieving faster convergence with a greater robustness is often favored in practical engineering applications. For instance, an industrial weight scale needs to attain its steady-state value within a specified threshold for a finite time. The system quickly achieves its equilibrium point using a magnetic force. The preceding situation is known as finite-time stability. The concept of finite-time stability was first introduced by Dorato in 1961 [31]. In 2001, Amato [32] presented finite-time boundedness by extending finite-time stability with external disturbance. While there has been considerable research on achieving finite-time stability for delayed NNs [9,11,12,17,26,33,34], the exploration of finite-time decentralized event-triggered feedback control for GNNs with cyber-attacks remains an unaddressed issue. This research gap serves as the primary motivation for the present study.
To address this research gap, this article introduces an innovative decentralized event-triggered method and feedback controller for GNNs with mixed interval time-varying delays and cyber-attacks. The primary contributions of this article can be summarized as follows:
(1) We propose a novel decentralized event-triggered method and feedback controller for GNNs with mixed interval time-varying delays and cyber-attacks. This approach ensures finite-time boundedness while estimating the derivative of the Lyapunov-Krasovskii functionals using an integral inequality with an exponential function. By utilizing this method, we address the challenges associated with system stability and effectiveness in the presence of time-varying delays and cyber-attacks.
(2) The event-triggered approach introduced in this work leads to a reduction in network resource utilization. This reduction alleviates the transmission burden on the network by enabling each sensor to autonomously determine the optimal time for signal transmission. By minimizing unnecessary transmissions, the proposed approach enhances the overall efficiency and scalability of the system.
(3) We describe random cyber-attacks by utilizing Bernoulli-distributed variables and represent them through a nonlinear function that satisfies a specific condition. This modeling approach allows us to capture the realistic nature of cyber-attacks and effectively incorporate them into the control strategy.
(4) We provide an illustrative example accompanied by simulations to demonstrate the feasibility and effectiveness of the proposed control strategy. The results showcase the improved effectiveness achieved in terms of system stability, finite-time boundedness, and resilience against cyber-attacks.
The remainder of this article is structured as follows. In Section 2, we introduce GNNs and provide preliminaries. Section 3 uses a state feedback controller to examine the stochastic finite-time bounded conditions for delayed GNNs with cyber-attacks. Section 4 presents a numerical example to illustrate the effectiveness of the proposed methods. In Section 5, we conclude and discuss our article.
Notations: This article utilizes the following notations: I denotes the identity matrix; ‖⋅‖ represents the Euclidean vector norm of a matrix; Rn indicates the n-dimensional Euclidean space; Prob{X} represents the probability of event X to occur; diag{⋯} refers a block-diagonal matrix; the notation PT and P−1 stand for the transpose and inverse of matrix P, respectively; the expression P<0 (or P≤0) signifies that the real symmetric matrix P is negative definite (or negative semi-definite); λmin(P) (or λmax(P)) denotes the minimum (or maximum) eigenvalue of real symmetric matrix P; the term L2[0,∞) denotes a function space consisting of quadratically integrable functions over the interval [0,∞); the notation Sym{P} represents the sum of P and its transpose, i.e., P+PT; the symbol ∗ indicates the elements below the main diagonal in a symmetric matrix.
In this article, we introduce a problem involving GNNs with mixed interval time-varying delays. The problem statement is described as follows:
{˙x(t)=ˉAx(t)+B0f(Wx(t))+B1f(Wx(t−τ(t)))+B2∫t−η1(t)t−η2(t)h(Wx(u))du+Bwω(t)+Buu(t),z(t)=x(t), | (2.1) |
where x(t)∈Rn represents the state vector at time t; n is the number of neurals; z(t)∈Rn represents the output of the system; ˉA=diag{a1,a2,...,an} indicates a diagonal matrix; W,B0,B1 and B2 refer connection weight matrices; the matrices Bw and Bu are real constant matrices with known values; ω(t) refers the external disturbance input; u(t)∈Rm denotes the control input; and f(Wx(t))=[f1(Wx1(t)),...,fn(Wxn(t))]T and h(Wx(t))=[h1(Wx1(t)),...,hn(Wxn(t))]T indicate the activation functions. The time delays in the system are represented by ηi(t)(i=1,2) and τ(t), which correspond to interval distributed time-varying delays and interval time-varying delays, respectively.
The functions τ(t) and ηi(t)(i=1,2) are continuous and satisfy the following conditions:
0≤τm≤τ(t)≤τMand0≤η1≤η1(t)≤η2(t)≤η2, t∈[0,T], |
where τm,τM,η1,η2 ∈R refer known real constants.
Additionally, we assume that the neuron activation function and communication network delays satisfy the following assumptions.
Assumption (A1). Each of the activation functions, fi(t) and hi(t), where i=1,2,...,n, is assumed to be continuous and bounded, satisfies the following conditions: there exist constants F−i, F+i, H−i, and H+i such that
F−i≤fi(Wα1)−fi(Wα2)Wα1−Wα2≤F+i,H−i≤hi(Wα1)−hi(Wα2)Wα1−Wα2≤H+i,∀α1,α2∈R,α1≠α2. |
Assumption (A2). Let τtik denote the communication network delay at the sampled instant tik in the ith sensor. It is assumed that 0<τtik<ˉτi, where ˉτ=maxi∈1,2,...,nˉτi.
The diagram of the decentralized event-triggered control for GNNs with cyber-attacks is shown in Figure 1. This structure involves multiple sensors and controllers exchanging information over a communication network, which may experience time delays and potential cyber-attacks. To minimize network transmissions, event generators are employed at each sensor. When a new signal is sampled, it is promptly sent to the corresponding event generator. Additionally, the signal includes the disturbance input vector ω(t), representing either external factors or disturbances that influence the controlled system. The controlled output z(t) represents the desired or targeted system output. Only signals that violate the event-triggered condition are transmitted over the network, thereby reducing communication bandwidth requirements. The primary objective of this decentralized event-triggered structure is to ensure the stability of the neural network while minimizing the impact of cyber-attacks. Furthermore, a three-line table summarizing the algorithm of the proposed method is provided below in Table 1.
Step | Description |
1 | Initialize network parameters, event-triggering thresholds and cyber-attack detection |
mechanisms | |
2 | Sample input signals, check event-triggering conditions and detect potential |
cyber-attacks | |
3 | Update network states, transmit relevant information among nodes and implement |
countermeasures against cyber-attacks |
The following expression gives the predefined event-triggered criterion for the ith sensor:
eTi(t)Ωiei(t)<σixTi(tikh+jih)Ωixi(tikh+jih), | (2.2) |
where a weighting matrix is denoted by Ωi>0, σi∈[0,1), i∈{1,2,..,n}, h is the sampling period of sensor, jih is the sampling instant for the ith sensor, and ei(t) is the difference between the latest transmitted signal xi(tikh) and the current sampled signal x(tikh+jih) at time t.
Remark 2.1. In the event generator of the ith sensor, the most recently triggered instant is tikh, and the current sampling instant is tikh+jih. It is important to note that the set {ti1,ti2,...,}⊆{h,2h,...,jih,...} for i∈{1,2,...,n}.
The zero holder order (ZOH) holding interval [tikh,tik+1h) can be divided into several subsets denoted by ∪jiMji=0Υji. Each subset ∪jiMji=0Υji is given by Υji=[tikh+jih+βtik+ji,tikh+jih+h+βtik+ji+1) for ji=0,1,...,jiM, jiU=tik+1−tik−1.
Following a similar approach to [35], we establish a sequence of buffers on the actuator side, each with a unique timestamp to hold the controller outputs. This enables the actuators to update the controlled inputs by selecting the corresponding controller output from the buffers. Thus, the input update time set for the actuators is defined as tk+1h=tkh+jh, where jh=argminii∈{1,2,...,n}{jih} can be obtained from (2.2).
Define β(t)=t−tkh−jh. It is evident that 0≤βtk≤β(t)≤ˉβ, where ˉβ=h+βtk+j+1. Based on (2.2), the condition for n channels can be derived as follows:
eT(t)Ωe(t)<σxT(t−β(t))Ωx(t−β(t)), | (2.3) |
where e(t)=x(tkh)−x(tk+1h+jh), Ω=diag{Ω1,...,Ωn} and σ=diag{σ1,...,σn}.
The following is a description of the proposed method for designing the controller model, which takes into account both the decentralized event-triggered scheme and cyber-attacks:
u(t)=ρ(tk)Kx(tkh)+(1−ρ(tk))Kg(x(t−d(t))), | (2.4) |
where ρ(tk)∈{0,1}, Prob{ρ(tk)=1}=ˉρ, Prob{ρ(tk)=0}=1−ˉρ, the controller gain is denoted by K, and the function of cyber-attacks is represented by g(x(t−d(t))), where g(x(t))=[g1(x1(t)),g2(x2(t)), ...,gn(xn(t))]T, d(t)∈(0,ˉd] and ˉd is a positive constant.
Remark 2.2. The dynamic event-triggered system (ETS) utilizes periodic sampling and a waiting period of h seconds to evaluate the event-triggering condition, allowing for ample decision-making time and preventing the occurrence of Zeno behavior. Zeno behavior refers to the destabilizing effect of rapid and continuous event triggering. The introduction of this temporal constraint ensures a balanced operation, averting undesirable system behavior and instability by triggering events at appropriate intervals.
Remark 2.3. This article considers the probability distribution of cyber-attacks, which is assumed to follow the Bernoulli distribution. The received sensor measurements are represented by ρ(tk), with ρ(tk)=1 indicating actual sensor measurements and ρ(tk)=0 indicating that the sensor measurements accessible through the communication network have been attacked.
Combining (2.1) and (2.4), we can derive that
˙x(t)=ˉAx(t)+B0f(Wx(t))+B1f(Wx(t−τ(t)))+B2∫t−η1(t)t−η2(t)h(Wx(u))du+Bwω(t)+ρ(tk)BuKx(tkh)+(1−ρ(tk))BuKg(x(t−d(t))),t∈[tkh+βtk,tk+1h+βtk+1]. | (2.5) |
Taking the definition of β(t) and the features of ρ(tk) into account, we can express (2.5) as the following:
˙x(t)=ˉAx(t)+ˉρBuK[x(t−β(t))+e(t)]+(1−ˉρ)BuKg(x(t−d(t)))+B0f(Wx(t))+B1f(Wx(t−τ(t)))+B2∫t−η1(t)t−η2(t)h(Wx(s))ds+Bwω(t)+(ρ(tk)−ˉρ)BuK[x(t−β(t))+e(t)−g(x(t−d(t)))],t∈[tkh+βtk,tk+1h+βtk+1]. | (2.6) |
We present the following assumptions and lemmas instrumental to deriving our main results.
Assumption (A3). For each gi(t), i=1,2,...,n represents a cyber-attack function that is bounded. There exist constants G−i and G+i such that
G−i≤gi(α1)−gi(α2)α1−α2≤G+i,∀α1,α2∈R,α1≠α2. |
Remark 2.4. Assumption (A3) allows for the analysis of the system's response to cyber-attacks, even in the absence of detailed information about the attack signals. It facilitates the design of resilient control strategies that can handle various attack scenarios and maintain system stability, despite bounded cyber-attacks.
Assumption (A4). The external disturbance ω(t) satisfies
∫T0ωT(t)ω(t)dt≤dw,dw≥0,Tis a time constant. |
Definition 2.1. [32] Given positive constants c1,c2 and T with 0<c1<c2 and X is a symmetric positive definite matrix. The GNNs (2.1) is finite-time bounded with respect to (c1,c2,X,T), if ∀t∈[0,T]
sup−τM≤s≤0{xT(s)Xx(s),˙xT(s)X˙x(s)}≤c1⟹xT(t)Xx(t)<c2. | (2.7) |
Lemma 2.2. (Jensen's inequality [36]) For a symmetric positive-definite matrix, M∈Rm×m, and any given scalars d1 and d2, the following inequality holds:
(d2−d1)∫d2d1xT(u)Mx(u)du≥(∫d2d1x(u)du)TM(∫d2d1x(u)du). | (2.8) |
Lemma 2.3. [35] Assume β(t)∈[0,ˉβ], for any matrices R∈R and L that satisfies [RLLTR]≥0, the inequality holds as follows:
−ˉβ∫tt−ˉβ˙xT(u)R˙x(u)du≤[x(t)x(t−β(t))x(t−ˉβ)]T[−R∗∗RT−LT−2R+L+LT∗LTRT−LT−R][x(t)x(t−β(t))x(t−ˉβ)]. | (2.9) |
Lemma 2.4. [37] For any positive scalars a,b>a and α, and any symmetric matrix M=MT>0 with dimension n×n, the following inequality holds:
∫baeα(t−u)˙xT(u)M˙x(u)du≥1Φ0ΣT0MΣ0+1Φ1ΣT1MΣ1, | (2.10) |
where
Σ0=x(b)−x(a),Σ1=ε1x(a)+ε2x(b)−∫bax(u)du,ε1=(b−a)e−α(t−b)e−α(t−b)−e−α(t−a)−1α,ε2=1α−(b−a)e−α(t−a)e−α(t−b)−e−α(t−a),Φ0=∫bae−α(t−u)du=1α(e−α(t−b)−e−α(t−a)),Φ1=∫bae−α(t−u)l21(u)du=e−2α(t−a)−(2+α2(b−a)2)e−α(2t−a−b)+e−2α(t−b)α3(e−α(t−b)−e−α(t−a)),l1(u)=u−(∫bae−α(t−u)du)−1(∫bae−α(t−u)udu). |
Remark 2.5. When α=0, then the specific values of Φ0, Φ1, Σ0, and Σ1 are given by Φ0=b−a, Φ1=(b−a)312, Σ0=x(b)−x(a), and Σ1=b−a2[x(a)+x(b)−2b−a∫bax(u)du]. This implies that Lemma 2.4 reduces to the well-known Wirtinger's inequality.
Lemma 2.5. [38] For a full column rank matrix L∈Rn×m, the singular decomposition is L=U1ΣUT2, where U1 and U2 are orthogonal matrices, and Σ∈Rn×m is a rectangular diagonal matrix with positive real numbers. Let M be a matrix of the form M=U1[R100R2]UT1, then there exists X∈Rm×m such that ML=LX.
Lemma 2.6. (Schur complement [39]) If matrices X, Y, and Z have appropriate dimensions and satisfy X=XT and Y=YT>0, then the inequality X+ZTY−1Z<0 holds if and only if
[XZTZ−Y]<0or[−YZZTX]<0. | (2.11) |
In this section, we introduce new sufficient conditions for delayed GNNs that build on the main theorems. To start, we define the parameters as follows: τMm=τM−τm,τMm≠0,
θ1a=1α(1−e−ατm),θ1b=e−2ατm−(2+α2τ2m)e−ατm+1α3(1−e−ατm),θ2a=1α(1−e−ατM),θ2b=e−2ατM−(2+α2τ2M)e−ατM+1α3(1−e−ατM),θ3a=1α(e−ατm−e−ατ(t)),θ3b=1α3(e−ατm−e−ατ(t))[e−2ατ(t)−(2+α2(τ(t)−τm)2)e−α(τ(t)+τm)+e−2ατm],θ4a=1α(e−ατ(t)−e−ατM),θ4b=1α3(e−ατ(t)−e−ατM)[e−2ατM−(2+α2(τM−τ(t))2)e−α(τ(t)+τM)+e−2ατ(t)],ϵ11=τm1−e−ατm−1α,ϵ12=1α−τme−ατm1−e−ατm,ϵ21=τM1−e−ατM−1α,ϵ22=1α−τMe−ατM1−e−ατM,ϵ31=(τ(t)−τm)e−ατme−ατm−e−ατ(t)−1α,ϵ32=1α−(τ(t)−τm)e−ατ(t)e−ατm−e−ατ(t),ϵ41=(τM−τ(t))e−ατ(t)e−ατ(t)−e−ατM−1α,ϵ42=1α−(τM−τ(t))e−ατMe−ατ(t)−e−ατM,Γ1=[eT1−eT2]T,Γ2=[ϵ11eT2+ϵ12eT1−τmeT13]T,Γ3=[eT1−eT4]T,Γ4=[ϵ21eT4+ϵ22eT1−τMeT14]T,Γ5=[eT2−eT3]T,Γ6=[ϵ31eT3+ϵ32eT2−τMmeT15]T,Γ7=[eT3−eT4]T,Γ8=[ϵ41eT4+ϵ42eT3−τMmeT16]T,Γ9=[eT9−eT1WTFTM]T,Γ10=[FPWe1−e9],Γ11=[eT10−eT3WTFTM]T,Γ12=[FPWe3−e10],Γ13=[eT9−eT10−eT1WTFTM+eT3WTFTM]T,Γ14=[FPWe1−FPWe3−e9+e10],Γ15=[eT11−eT7GTM]T,Γ16=[GPe7−e11],Γ17=[eT12−eT1WTHTM]T,Γ18=[HPWe1−e12],FM=diag{F−1,...,F−n},FP=diag{F+1,...,F+n},GM=diag{G−1,...,G−n},GP=diag{G+1,...,G+n},HM=diag{H−1,...,H−n},HP=diag{H+1,...,H+n},γ1=eατm−1α,γ2=eατM−1α,γ3=eαˉβ−1α,γ4=eαˉβ−1α,γ5=eατm−ατm−1α2,γ6=eατM−ατM−1α2,γ7=eατM−eατm−ατMmα2,γ8=eαˉβ−αˉβ−1α2,γ9=eαˉd−αˉd−1α2,γ10=eαη2−eαη1−αη21α2,η21=η2−η1, |
and we define vectors as follows:
ξ(t)=[xT(t),xT(t−τm),xT(t−τ(t)),xT(t−τM),xT(t−β(t)),xT(t−ˉβ),xT(t−d(t)),xT(t−ˉd),fT(Wx(t)),fT(Wx(t−τ(t))),gT(x(t−d(t))),hT(Wx(t)),1τm∫tt−τmxT(u)du,1τM∫tt−τMxT(u)du,1τ(t)−τm∫t−τmt−τ(t)xT(u)du,1τM−τ(t)∫t−τ(t)t−τMxT(u)du,∫t−η1(t)t−η2(t)hT(Wx(u))du,eT(t),ωT(t)]T, |
ei=[0n×(i−1)nIn0n×(19−i)n],i=1,2,...,19. |
First, we obtain new sufficient conditions of the finite-time decentralized event-triggered feedback control problem for GNNs with mixed interval time-varying delays and cyber-attacks as follows.
Theorem 3.1. Assume that Assumptions (A1)-(A4) are satisfied. Then, for given scalars ˉβ,ˉd,ˉρ,σ,dw,τm,τM, η1,η2,T, c1,c2 and α, the delayed GNNs with cyber-attacks (2.6) under the state feedback controller is stochastic finite-time bounded regarding (c1,c2,T,X,dw), if there exist symmetric positive definite matrices P, Qi,Rj(i=1,2,3,4,j=1,2,3,4,5), M,S and positive diagonal matrices G1,G2,G3,G4,G5, such that the following conditions hold:
[Π∗∗Γa−Λ∗Γb0−Λ]<0, | (3.1) |
eαT[Πλc1+dwλ13(1−e−αT)]<λ1c2, | (3.2) |
where
Π=6∑i=1Πi,Π1=2eT1PˉAe1+2eT1ˉρPBuK[e5+e18]+2(1−ˉρ)eT1PBuKe11+2eT1PB0e9+2eT1PB1e10+2eT1PB2e17+2eT1PBwe19−αeT1Pe1,Π2=eT1(Q1+Q2+Q3+Q4)e1−eατmeT2Q1e2−eατMeT4Q2e4−eαˉβeT6Q3e6−eαˉdeT8Q4e8,Π3=−τmθ1aΓT1R1Γ1−τmθ1bΓT2R1Γ2−τMθ2aΓT3R2Γ3−τMθ2bΓT4R2Γ4−τMmθ3aΓT5R3Γ5−τMmθ3bΓT6R3Γ6−τMmθ4aΓT7R3Γ7−τMmθ4bΓT8R3Γ8+eαˉβ[−eT1R4e1+2eT1(R4−L1)e5+2eT1L1e6+eT5(−2R4+L1+LT1)e5+2eT5(R4−L1)e6+eT6R4e6]+eαˉd[−eT1R5e1+2eT1(R5−L2)e7+2eT1L2e8+eT7(−2R5+L2+LT2)e7+2eT7(R5−L2)e8+eT8R5e8],Π4=η221eT12Me12−eαη2eT17Me17,Π5=2ΓT9G1Γ10+2ΓT11G2Γ12+2ΓT13G3Γ14+2ΓT15G4Γ16+2ΓT17G5Γ18,Π6=−αeT19Se19−eT18Ωe18+σeT5Ωe5,Πλ=λ2+γ1λ3+γ2λ4+γ3λ5+γ4λ6+τmγ5λ7+τMγ6λ8+τMmγ7λ9+ˉβγ8λ10+ˉdγ9λ11+η21γ10λ12,λ1=λmin(¯P),λ2=λmax(¯P),λ3=λmax(¯Q1),λ4=λmax(¯Q2),λ5=λmax(¯Q3),λ6=λmax(¯Q4),λ7=λmax(¯R1),λ8=λmax(¯R2),λ9=λmax(¯R3),λ10=λmax(¯R4),λ11=λmax(¯R5),λ12=λmax(¯M),λ13=λmax(¯S),Λ=diag{PR−11P,PR−12P,PR−13P,PR−14P,PR−15P},Γa=[τmΓTa1τMΓTa1τMmΓTa1ˉβΓTa1ˉdΓTa1]T,Γb=[τmΓTb1τMΓTb1τMmΓTb1ˉβΓTb1ˉdΓTb1]T,Γa1=[Γa11Γa12],Γa11=[PA01×3ˉρPBuK01×3PB0PB1],Γa12=[(1−ˉρ)PBuK01×5PB2ˉρPBuKPBw],Γb1=[01×4δPBuK01×5δPBuK01×6δPBuK0],δ=√ˉρ(1−ˉρ). |
Proof. We formulate the LKFs as follows:
V(xt,t)=3∑i=1Vi(xt,t), | (3.3) |
where
V1(xt,t)=x(t)TPx(t),V2(xt,t)=∫tt−τmeα(t−u)xT(u)Q1x(u)du+∫tt−τMeα(t−u)xT(u)Q2x(u)du+∫tt−ˉβeα(t−u)xT(u)Q3x(u)du+∫tt−ˉdeα(t−u)xT(u)Q4x(u)du,V3(xt,t)=τm∫tt−τm∫tueα(t−s)˙xT(s)R1˙x(s)dsdu+τM∫tt−τM∫tueα(t−s)˙xT(s)R2˙x(s)dsdu+τMm∫t−τmt−τM∫tueα(t−s)˙xT(s)R3˙x(s)dsdu+ˉβ∫tt−ˉβ∫tueα(t−s)˙xT(s)R4˙x(s)dsdu+ˉd∫tt−ˉd∫tueα(t−s)˙xT(s)R5˙x(s)dsdu+(η2−η1)∫t−η1t−η2∫tueα(t−s)hT(Wx(s))Mh(Wx(s))dsdu. |
Taking the mathematical expectation of the derivative of (3.3) over the trajectory of the GNNs (2.6), we obtain
E{˙V1(xt,t)}=2xT(t)P˙x(t)−αxT(t)Px(t)+αV1(xt,t)=ξT(t)Π1ξ(t)+αV1(xt,t), | (3.4) |
E{˙V2(xt,t)}=xT(t){Q1+Q2+Q3+Q4}x(t)−eατmxT(t−τm)Q1x(t−τm)−eατMxT(t−τM)Q2x(t−τM)−eαˉβxT(t−ˉβ)Q3x(t−ˉβ)−eαˉdxT(t−ˉd)Q4x(t−ˉd)+αV2(xt,t)=ξT(t)Π2ξ(t)+αV2(xt,t), | (3.5) |
E{˙V3(xt,t)}=E{˙xT(t)ˆR˙x(t)}−τm∫tt−τmeα(t−u)˙xT(u)R1˙x(u)du−τM∫tt−τMeα(t−u)˙xT(u)R2˙x(u)du−τMm∫t−τmt−τMeα(t−u)˙xT(u)R3˙x(u)du−ˉβ∫tt−ˉβeα(t−u)˙xT(u)R4˙x(u)du−ˉd∫tt−ˉdeα(t−u)˙xT(u)R4˙x(u)du+αV3(xt,t), | (3.6) |
where ˆR=τ2mR1+τ2MR2+τ2MmR3+ˉβ2R4+ˉd2R5,
E{˙V4(xt,t)}=(η2−η1)2hT(Wx(t))Mh(Wx(t))−(η2−η1)∫t−η1t−η2eα(t−u)hT(Wx(u))Mh(Wx(u))du+αV4(xt,t)≤(η2−η1)2hT(Wx(t))Mh(Wx(t))−(η2(t)−η1(t))eαη2∫t−η1(t)t−η2(t)hT(Wx(u))Mh(Wx(u))du+αV4(xt,t). | (3.7) |
Applying Lemma 2.4 to the integral, we obtain
−τm∫tt−τmeα(t−u)˙xT(u)R1˙x(u)du≤ξT(t){−τmθ1a(e1−e2)TR1(e1−e2)−τmθ1b(ϵ11e2+ϵ12e1−τme13)T×R1(ϵ11e2+ϵ12e1−τme13)}ξ(t)=ξT(t){−τmθ1aΓT1R1Γ1−τmθ1bΓT2R1Γ2}ξ(t), | (3.8) |
−τM∫tt−τMeα(t−u)˙xT(u)R2˙x(u)du≤ξT(t){−τMθ2a(e1−e4)TR2(e1−e4)−τMθ2b(ϵ21e4+ϵ22e1−τMe14)T×R2(ϵ21e4+ϵ22e1−τMe14)}ξ(t)=ξT(t){−τMθ2aΓT3R2Γ3−τMθ2bΓT4R2Γ4}ξ(t), | (3.9) |
−τMm∫t−τmt−τMeα(t−u)˙xT(u)R3˙x(u)du=−τMm∫t−τmt−τ(t)eα(t−u)˙xT(u)R3˙x(u)du−τMm∫t−τ(t)t−τMeα(t−u)˙xT(u)R3˙x(u)du≤ξT(t){−τMmθ3a(e2−e3)TR3(e2−e3)−τMmθ3b(ϵ31e3+ϵ32e2−τMme15)T×R3(ϵ31e3+ϵ32e2−τMme15)−τMmθ4a(e3−e4)TR3(e3−e4)−τMmθ4b(ϵ41e4+ϵ42e3−τMme16)T×R3(ϵ41e4+ϵ42e3−τMme16)}ξ(t)=ξT(t){−τMmθ3aΓT5R3Γ5−τMmθ3bΓT6R3Γ6−τMmθ4aΓT7R3Γ7−τMmθ4bΓT8R3Γ8}ξ(t). | (3.10) |
Applying Lemma 2.2, we obtain
−(η2(t)−η1(t))eαη2∫t−η1(t)t−η2(t)hT(Wx(u))Mh(Wx(u))du≤−eαη2(∫t−η1(t)t−η2(t)hT(Wx(u))du)T×M(∫t−η1(t)t−η2(t)hT(Wx(u))du)=ξT(t){−eT17(eαη2M)e17}ξ(t). | (3.11) |
Applying Lemma 2.3, we obtain
−ˉβ∫tt−ˉβeα(t−u)˙xT(u)R4˙x(u)du≤eαˉβ[x(t)x(t−β(t))x(t−ˉβ)]T[−R4∗∗RT4−LT1−2R4+L1+LT1∗LT1RT4−LT1−R4][x(t)x(t−β(t))x(t−ˉβ)]=eαˉβξT(t){−eT1R4e1+2eT1(R4−L1)e5+2eT1L1e6+eT5(−2R4+L1+LT1)e5+2eT5(R4−L1)e6+eT6R4e6}ξ(t), | (3.12) |
−ˉd∫tt−ˉdeα(t−u)˙xT(u)R5˙x(u)du≤eαˉd[x(t)x(t−d(t))x(t−ˉd)]T[−R5∗∗RT5−LT2−2R5+L2+LT2∗LT2RT5−LT2−R5][x(t)x(t−d(t))x(t−ˉd)]=eαˉdξT(t){−eT1R5e1+2eT1(R5−L2)e7+2eT1L2e8+eT7(−2R5+L2+LT2)e7+2eT7(R5−L2)e8+eT8R5e8}ξ(t). | (3.13) |
Noting that E{˙xT(t)ˆR˙x(t)}=ATˆRA+ˉρ(1−ˉρ)BTˆRB, where A=ˉAx(t)+ˉρBuK[x(t−β(t))+e(t)]+(1−ˉρ)BuKg(x(t−d(t)))+B0f(Wx(t))+B1f(Wx(t−τ(t)))+B2∫t−η1(t)t−η2(t)h(Wx(s))ds+Bwω(t),B=BuK[x(t−β(t))+e(t)−g(x(t−d(t)))].
One can deduce from Assumption (A1) that if β1i,β2i,β3i,β4i,β5i>0 for i=1,2,...,n, then
2[fi(Wix(t))−F−iWix(t)]β1i[F+iWix(t)−fi(Wix(t))]≥0, | (3.14) |
2[fi(Wix(t−τ(t)))−F−iWix(t−τ(t))]β2i[F+iWix(t−τ(t))−fi(Wix(t−τ(t)))]≥0, | (3.15) |
2[fi(Wix(t))−fi(Wix(t−τ(t)))−F−i(Wix(t)−Wix(t−τ(t)))]β3i[F+i(Wix(t)−Wix(t−τ(t)))−fi(Wix(t))+fi(Wix(t−τ(t)))]≥0, | (3.16) |
2[gi(Wix(t−d(t)))−G−iWix(t−d(t))]β4i[G+iWix(t−d(t))−gi(Wix(t−d(t)))]≥0, | (3.17) |
2[hi(Wix(t))−H−iWix(t)]β5i[H+iWix(t)−hi(Wix(t))]≥0, | (3.18) |
which implies that
2ξT(t)ΓT9G1Γ10ξ(t)≥0,2ξT(t)ΓT11G2Γ12ξ(t)≥0,2ξT(t)ΓT13G3Γ14ξ(t)≥0,2ξT(t)ΓT15G4Γ16ξ(t)≥0,2ξT(t)ΓT17G5Γ18ξ(t)≥0. |
Thus, we have
0≤ξT(t){2ΓT9G1Γ10+2ΓT11G2Γ12+2ΓT13G3Γ14+2ΓT15G4Γ16+2ΓT17G5Γ18}ξ(t)=ξT(t)Π5ξ(t), | (3.19) |
where G1=diag{β11,...,β1n}, G2=diag{β21,...,β2n}, G3=diag{β31,...,β3n}, G4=diag{β41,...,β4n} and G5=diag{β51,...,β5n}.
Combining (3.4)–(3.19) with (2.3), we obtain
E{˙V(xt,t)−αV(xt,t)}−αωT(t)Sω(t)≤ξT(t){Π1+Π2+Π3+Π4+Π5}ξ(t)−αωT(t)Sω(t)−eT(t)Ωe(t)+σxT(t−β(t))Ωx(t−β(t))+ATˆRA+ˉρ(1−ˉρ)BTˆRB=ξT(t)¯Πξ(t)+ATˆRA+ˉρ(1−ˉρ)BTˆRB. | (3.20) |
Applying the Schur complement [39], we can derive that (3.1) is equal to:
E{˙V(xt,t)−αV(xt,t)}−αωT(t)Sω(t)<0. | (3.21) |
Then, multiplying (3.21) by e−αt, we can be written as
E{ddt(e−αtV(xt,t))}<αe−αtωT(t)Sω(t). | (3.22) |
Assumption (A2) and integrating (3.22) from 0 to t with t∈[0,T], we obtain
E{V(xt,t)}<eαT[V(x0,0)+α∫T0e−αuωT(u)Sω(u)du]<eαT[V(x0,0)+dwλ13(1−e−αT)]. | (3.23) |
Considering V(x0,0), we can derive that
E{V(x0,0)}=E{xT(0)Px(0)+∫0−τme−αuxT(u)Q1x(u)du+∫0−τMe−αuxT(u)Q2x(u)du+∫0−ˉβe−αuxT(u)Q3x(u)du+∫0−ˉde−αuxT(u)Q4x(u)du+τm∫0−τm∫0ue−αs˙x(s)R1˙x(s)dsdu+τM∫0−τM∫0ue−αs˙x(s)R2˙x(s)dsdu+τMm∫−τm−τM∫0ue−αs˙x(s)R3˙x(s)dsdu+ˉβ∫0−ˉβ∫0ue−αs˙x(s)R4˙x(s)dsdu+ˉd∫0−ˉd∫0ue−αs˙x(s)R5˙x(s)dsdu+η21∫−η1−η2∫0ue−αshT(Wx(t))Mh(Wx(t))dsdu}≤E{xT(0)Px(0)+∫0−τme−αuxT(u)Q1x(u)du+∫0−τMe−αuxT(u)Q2x(u)du+∫0−ˉβe−αuxT(u)Q3x(u)du+∫0−ˉde−αuxT(u)Q4x(u)du+τm∫0−τm∫0ue−αs˙x(s)R1˙x(s)dsdu+τM∫0−τM∫0ue−αs˙x(s)R2˙x(s)dsdu+τMm∫−τm−τM∫0ue−αs˙x(s)R3˙x(s)dsdu+ˉβ∫0−ˉβ∫0ue−αs˙x(s)R4˙x(s)dsdu+ˉd∫0−ˉd∫0ue−αs˙x(s)R5˙x(s)dsdu+η21∫−η1−η2∫0ue−αsxT(s)FwMFwx(s)dsdu}, |
where Fw=diag{F+1,F+2,...,F+n}W.
Setting ¯P=X−12PX−12, ¯Qi=X−12QiX−12, ¯Rj=X−12RjX−12, ¯M=X−12FwMFwX−12, i=1,2,3,4,j=1,2,3,4,5, we have
E{V(x0,0)}≤E{xT(0)X12¯PX12x(0)+∫0−τme−αuxT(u)X12¯Q1X12x(u)du+∫0−τMe−αuxT(u)X12¯Q2X12x(u)du+∫0−ˉβe−αuxT(u)X12¯Q3X12x(u)du+∫0−ˉde−αuxT(u)X12¯Q4X12x(u)du+τm∫0−τm∫0ue−αs˙x(s)X12¯R1X12˙x(s)dsdu+τM∫0−τM∫0ue−αs˙x(s)X12¯R2X12˙x(s)dsdu+τMm∫−τm−τM∫0ue−αs˙x(s)X12¯R3X12˙x(s)dsdu+ˉβ∫0−ˉβ∫0ue−αs˙x(s)X12¯R4X12˙x(s)dsdu+ˉd∫0−ˉd∫0ue−αs˙x(s)X12¯R5X12˙x(s)dsdu+η21∫−η1−η2∫0ue−αsxT(s)X12¯MX12x(s)dsdu}≤{λ2+γ1λ3+γ2λ4+γ3λ5+γ4λ6+τmγ5λ7+τMγ6λ8+τMmγ7λ9+ˉβγ8λ10+ˉdγ9λ11+η21γ10λ12}c1=Πλc1. |
Furthermore, by referring Eq (3.3), we obtain the following:
E{V(xt,t)}≥E{xT(t)Px(t)}≥λmin(¯P)E{xT(t)Xx(t)}=λ1E{xT(t)Xx(t)}. | (3.24) |
Subsequently, by utilizing Eqs (3.23) to (3.24), we obtain the following:
E{xT(t)Xx(t)}≤eαTλ1[Πλc1+dwλ13(1−e−αT)]. |
Thus, if the relation in (3.2) holds true, then it implies that E{xT(t)Xx(t)}<c2,∀t∈[0,T]. Consequently, it can be concluded that the delayed GNNs (2.6) with cyber-attacks are stochastically finite-time bounded with respect to (c1,c2,T,X,dw). This completes the proof.
Remark 3.1. The activation functions play a crucial role in determining the existence and uniqueness of solutions in GNNs. Specifically, the activation functions in GNNs need to satisfy the Lipschitz condition, which ensures that the functions possess certain properties that guarantee the existence and uniqueness of solutions. The Lipschitz condition requires the functions to have a bounded derivative, thereby controlling the rate of change of the functions. In this article, the activation function is assumed to satisfy Assumptions (A1) and (A3). In this case, it is important to note that the activation function does not necessarily need to be non-monotonic and differentiable. The constants F−i, F+i, H−i, H+i, G−i and G+i, where i=1,2,…,n, can take either positive, zero, or negative values. Assumptions (A1) and (A3), as considered in Eqs (3.14)–(3.18) of this article, ensuring not only F−i≤fi(Wx(t))Wx(t)≤F+i, F−i≤fi(Wx(t−τ(t)))Wx(t−τ(t))≤F+i, H−i≤hi(Wx(t))Wx(t)≤H+i, H−i≤hi(Wx(t−τ(t)))Wx(t−τ(t))≤H+i, G−i≤gi(x(t))x(t)≤G+i and G−i≤gi(x(t−τ(t)))x(t−τ(t))≤G+i, but also F−i≤fi(Wx1)−fi(Wx2)Wx1−Wx2≤F+i, H−i≤hi(Wx1)−hi(Wx2)Wx1−Wx2≤H+i and G−i≤gi(x1)−gi(x2)x1−x2≤G+i. Therefore, this assumption is weaker and more general than the usual Lipschitz condition. In conclusion, the activation functions in GNNs play a critical role in ensuring the existence and uniqueness of solutions. While the assumption considered in this article relaxes the requirement for non-monotonicity and differentiability, it provides a more general condition that still guarantees the desired properties.
Based on the results of Theorem 3.1, we can now propose a controller design approach for the delayed GNNs (2.6) as follows.
Assumption (A5) To handle the nonlinear terms in Theorem 3.1, we adopt a similar assumption to [38], B is assumed to be full column rank, and the singular decomposition for B as B=L1[Bu00]L2, where Bu0 is the first u0 columns of B, L1 and L2 are appropriate matrices with compatible dimensions.
Theorem 3.2. Assume that Assumptions (A1)–(A5) are satisfied. Then, for the given scalars ˉβ,ˉd,ˉρ,σ,dw,τm,τM, η1,η2,T, c1,c2 and α, the delayed GNNs with cyber attacks (2.6) under the state feedback controller is stochastic finite-time bounded regarding (c1,c2,T,X,dw), if there exist symmetric positive definite matrices P, Qi,Rj(i=1,2,3,4,j=1,2,3,4,5), M,S, and positive diagonal matrices G1,G2,G3,G4,G5, such that the conditions hold as follows:
[Πu∗∗¯Γau−Λu∗¯Γbu0−Λu]<0, | (3.25) |
eαT[Πλc1+dwλ13(1−e−αT)]<λ1c2, | (3.26) |
where
Πu=¯Π1+6∑i=2Πi,¯Π1=2eT1PˉAe1+2eT1ˉρBuY[e5+e18]+2(1−ˉρ)eT1BuYe11+2eT1PB0e9+2eT1PB1e10+2eT1PB2e17+2eT1PBwe19−αeT1Pe1,Λu=diag{−2κ1P+κ21R1,−2κ2P+κ22R2,−2κ3P+κ23R3,−2κ4P+κ24R4,−2κ5P+κ25R5},¯Γau=[τm¯ΓTa1τM¯ΓTa1τMm¯ΓTa1ˉβ¯ΓTa1ˉd¯ΓTa1]T,¯Γbu=[τm¯ΓTb1τM¯ΓTb1τMm¯ΓTb1ˉβ¯ΓTb1ˉd¯ΓTb1]T,¯Γa1=[¯Γa11¯Γa12],¯Γa11=[PA01×3ˉρBuY01×3PB0PB1],¯Γa12=[(1−ˉρ)BuY01×5PB2ˉρBuYPBw],¯Γb1=[01×4δBuY01×5δBuY01×6BuY0],δ=√ˉρ(1−ˉρ), |
where P=L1diag{P1,P2}LT1, P1∈Rm×m,P2∈R(n−m)×(n−m). The other matrices are given in Theorem 3.1. The gain of the controller can be determined as follows:
K=P−1kY,Pk=(Bu0L2)−1P1Bu0L2, | (3.27) |
in which Bu0 and L2 are defined in Assumption (A5).
Proof. Let P and Bu be defined as follows based on Assumption (A5):
P=L1[P100P2]LT1andBu=L1[Bu00]L2. |
By applying Lemma 2.5, we can find a new variable Pk that satisfies PBu=BuPk, from
L1[P100P2]LT1L1[Bu00]L2=L1[Bu00]L2Pk, |
we get
Pk=(Bu0L2)−1P1Bu0L2. |
From PBu=BuPk, then we substitute PBuK=BuPkK and define Y=PkK, it follows from (3.1) that
[Πu∗∗¯Γau−Λ∗¯Γbu0−Λ]<0. | (3.28) |
In terms of the following inequality:
(Rj−κ−1jP)R−1j(Rj−κ−1jP)≥0,(j=1,2,3,4,5). |
One has that
−PR−1jP≤−2κjP+κ2jRj. |
By replacing −PR−1jP with −2κjP+κ2jRj in (3.28), it becomes evident that (3.25) is obtained. Thus, the proof is complete.
The delayed GNNs (2.6) take on the following forms under the decentralized event-triggered scheme without cyber-attacks:
˙x(t)=ˉAx(t)+BuK[x(t−β(t))+e(t)]+B0f(Wx(t))+B1f(Wx(t−τ(t)))+B2∫t−η1(t)t−η2(t)h(Wx(s))ds+Bwω(t),∀t∈[tkh+βtk,tk+1h+βtk+1]. | (3.29) |
Following the same approach as the proof for Theorem 3.2, we can derive the following corollary. Furthermore, we also define the vectors as follows:
ξ(t)=[xT(t),xT(t−τm),xT(t−τ(t)),xT(t−τM),xT(t−β(t)),xT(t−ˉβ),fT(Wx(t)),fT(Wx(t−τ(t))),hT(Wx(t)),1τm∫tt−τmxT(u)du,1τM∫tt−τMxT(u)du,1τ(t)−τm∫t−τmt−τ(t)xT(u)du,1τM−τ(t)∫t−τ(t)t−τMxT(u)du,∫t−η1(t)t−η2(t)hT(Wx(u))du,eT(t),ωT(t)]T, |
ei=[0n×(i−1)nIn0n×(16−i)n],i=1,2,...,16. |
Corollary 3.3. Assume that Assumptions (A1), (A2), (A4) and (A5) are satisfied. Then, for the given scalars ˉβ,ˉρ,σ,dw, τm,τM,η1,η2,T,c1,c2 and α, the delayed GNNs without cyber attacks (3.29) under the state feedback controller are stochastic finite-time bounded regarding (c1,c2,T,X,dw), if there exist symmetric positive definite matrices P, Qi,Rj(i=1,2,3,j=1,2,3,4), M,S, and positive diagonal matrices G1,G2,G3,G5, such that the conditions hold as follows:
[ˆΠu∗ˆΓau−ˆΛu]<0, | (3.30) |
eαT[ˆΠλc1+dwλ13(1−e−αT)]<λ1c2, | (3.31) |
where
ˆΠu=6∑i=1ˆΠui,ˆΠu1=2eT1PˉAe1+2eT1BuY[e5+e15]+2eT1PB0e7+2eT1PB1e8+2eT1PB2e14+2eT1PBwe16−αeT1Pe1,ˆΠu2=eT1(Q1+Q2+Q3)e1−eατmeT2Q1e2−eατMeT4Q2e4−eαˉβeT6Q3e6,ˆΠu3=−τmθ1aΓT1R1Γ1−τmθ1bˆΓT2R1ˆΓ2−τMθ2aΓT3R2Γ3−τMθ2bˆΓT4R2ˆΓ4−τMmθ3aΓT5R3Γ5−τMmθ3bˆΓT6R3ˆΓ6−τMmθ4aΓT7R3Γ7−τMmθ4bˆΓT8R3ˆΓ8+eαˉβ[−eT1R4e1+2eT1(R4−L1)e5+2eT1L1e6+eT5(−2R4+L1+LT1)e5+2eT5(R4−L1)e6+eT6R4e6],ˆΠu4=η221eT9Me9−eαη2eT14Me14,ˆΠu5=2ˆΓT9G1ˆΓ10+ˆΓT11G2ˆΓ12+ˆΓT13G3ˆΓ14+ˆΓT17G5ˆΓ18,ˆΠu6=−αeT16Se16−eT15Ωe15+σeT5Ωe5,ˆΠλ=λ2+γ1λ3+γ2λ4+γ3λ5+τmγ5λ7+τMγ6λ8+τMmγ7λ9+ˉβγ8λ10+η21γ10λ12,ˆΛu=diag{−2κ1P+κ21R1,−2κ2P+κ22R2,−2κ3P+κ23R3,−2κ4P+κ24R4},ˆΓau=[τmˆΓTa1τMˆΓTa1τMmˆΓTa1ˉβˆΓTa1]T,ˆΓa1=[ˆΓa11ˆΓa12],ˆΓa11=[PA01×3BuY01×3PB0PB1],ˆΓa12=[01×3PB2BuYPBw],ˆΓ2=[ϵ11eT2+ϵ12eT1−τmeT10]T,ˆΓ4=[ϵ21eT4+ϵ22eT1−τMeT11]T,ˆΓ6=[ϵ31eT3+ϵ32eT2−τMmeT12]T,ˆΓ8=[ϵ41eT4+ϵ42eT3−τMmeT13]T,ˆΓ9=[eT7−eT1WTFTM]T,ˆΓ10=[FPWe1−e7],ˆΓ11=[eT8−eT3WTFTM]T,ˆΓ12=[FPWe3−e8],ˆΓ13=[eT7−eT8−eT1WTFTM+eT3WTFTM]T,ˆΓ14=[FPWe1−FPWe3−e7+e8],ˆΓ17=[eT9−eT1WTHTM]T,ˆΓ18=[HPWe1−e9], |
where P=L1diag{P1,P2}LT1, P1∈Rm×m,P2∈R(n−m)×(n−m). The other matrices are given in Theorem 3.1. The gain of the controller can be determined as follows:
K=P−1kY,Pk=(Bu0L2)−1P1Bu0L2, | (3.32) |
in which Bu0 and L2 are defined in Assumption (A5).
Remark 3.2. The problem of NNs with a time-varying delay and cyber-attacks under the event-triggered framework using H∞ control was investigated by Zha et al. in [22]. In [30], Liu et al. presented a state estimation method for T-S fuzzy neural networks under stochastic cyber-attacks and an event-triggered scheme. However, previous works mainly focused on the asymptotic stability of NNs and did not address finite-time stability for GNNs. Asymptotic stability refers to the long-term behavior of a system, whereas finite-time stability is concerned with short-term behavior. Thus, this article aims to tackle the finite-time bounded problem of GNNs with decentralized event-triggered communication and cyber-attacks. The proposed decentralized event-triggered scheme enhances system effectiveness and minimizes network transmissions, resulting in a more efficient and secure system.
A simulation example is presented in this section to showcase the effectiveness and superiority of the theoretical method on the finite-time decentralized event-triggered feedback control problem. The parameters of the system (2.1) are given as follows:
ˉA=[−0.5000−0.1000−0.1],B0=[0.20.20.3−0.20.30.1−0.3−0.15−0.2],B1=[0.10.20−0.10.20.1−0.20−0.3],B2=[0.150.30.15−0.150.250.15−0.30.1−0.2],Bw=[0.50000.50000.5],Bu=[0.10000.20000.1],W=diag{1,1,1},Fm=diag{0,0,0},FP=diag{0.03,0.06,0.03},Gm=Fm,Gp=diag{0.03,0.06,0.03},Hm=Fm,Hp=diag{0.04,0.04,0.04},f(x)=[tanh(0.03x1(t))tanh(0.06x2(t))tanh(0.03x3(t))] and h(x)=[tanh(0.04x1(t))tanh(0.04x2(t))tanh(0.04x3(t))]. |
The function of cyber-attacks is given by g(x)=[tanh(0.03x1(t))tanh(0.06x2(t))tanh(0.03x3(t))]. Furthermore, the selected initial condition for generating the simulation results is given by x(0)=[−1.1−0.50.8]T.
Two cases will be analyzed for delayed GNNs. The first case considers both the event-triggered scheme and the cyber-attacks in delayed GNNs (2.6). The second case, on the other hand, looks at the event-triggered scheme used in delayed GNNs without cyber-attacks (3.29).
Case Ⅰ: In this case, we investigate the effects of both the event-triggered method and cyber-attacks on delayed GNNs (2.6). Assuming that the following parameter values are used: c1=2.1,T=50,α=0.3,dw=0.5,ˉβ=0.5,ˉd=0.5,ˉρ=0.1,σ=0.1,κi=1 (for i=1,2,3,...,5), τm=0.25, τM=0.9, τ(t)=0.7|sin(t)|+0.25, η1=0.1, η2=0.7, η1(t)=0.4+0.3sin(t), η2(t)=0.5+0.2sin(t), and an external disturbance given by 0.5e−0.5tsin(t). We solve the LMIs in Theorem 3.2 to obtain a feasible solution that guarantees finite-time boundedness with respect to (c1,c2,T,X,dw).
Applying (3.25), (3.26) and (3.27), we derive the corresponding minimum allowable lower bounds (MALBs) of c2=5.1788 and control gain as follows:
K=[−0.0235−0.01160.0236−0.0092−0.9716−0.00480.0142−0.0007−0.2283]. |
We show the effectiveness of our results in Case Ⅰ. Figure 2 illustrates the state responses of x(t) for the delayed GNNs (2.6) without u(t). The responses of the state variable x(t) and the time history of xT(t)Xx(t) in Case Ⅰ of the delayed GNNs with cyber-attacks (2.6) are depicted in Figures 3 and 4, respectively. Furthermore, the computed MALBs of c2 for different c1 are presented in Table 2. From the table, we can see that the MALBs of c2 from our results increase as c1 increases. Based on the simulation results presented above, it has been determined that the event-triggered state feedback control scheme for delayed GNNs (2.6) is stochastically finite-time bounded within a specified time interval. This conclusion remains valid even in the presence of cyber-attacks and gains fluctuations.
c1 | 1.2 | 1.3 | 1.4 | 1.5 | 1.6 | 1.7 | 1.8 | 1.9 | 2.0 | 2.1 |
c2 | 3.1058 | 3.3361 | 3.5674 | 3.7970 | 4.0288 | 4.2617 | 4.4885 | 4.7190 | 4.9508 | 5.1788 |
Case Ⅱ: This case focuses on delayed GNNs (3.29) under the event-triggered scheme without considering cyber-attacks. Assuming that the following parameter values are used: c1=2.1,T=50,α=0.3,dw=0.5,ˉβ=0.5,ˉd=0.5,ˉρ=0.1,σ=0.1,κi=1 (for i=1,2,3,...,5), τm=0.25, τM=0.9, τ(t)=0.7|sin(t)|+0.25, η1=0.1, η2=0.7, η1(t)=0.4+0.3sin(t), η2(t)=0.5+0.2sin(t), and an external disturbance given by 0.5e−0.5tsin(t). We solve the LMIs in Corollary 3.3 to obtain a feasible solution that guarantees finite-time boundedness with respect to (c1,c2,T,X,dw).
Applying (3.30), (3.31) and (3.32), we derive the corresponding MALBs of c2=3.2378 and control gain as follows
K=[−0.2941−0.1437−0.00080.1203−1.4131−0.26610.1263−0.0001−1.2606]. |
We present the effectiveness of our findings in Case Ⅱ. The response of the state variable x(t) and the time history of xT(t)Xx(t) in Case Ⅱ of the delayed GNNs without cyber-attacks (3.29) are illustrated in Figures 5 and 6, respectively. Additionally, we provide the computed MALBs of c2 for different c1 in Table 3. The table reveals that as c1 increases, the MALB of c2 also increases according to our findings. The simulation results above confirm that the event-triggered state feedback control scheme for delayed GNNs without cyber-attacks (3.29) is stochastically finite-time bounded within a specified time interval.
c1 | 1.2 | 1.3 | 1.4 | 1.5 | 1.6 | 1.7 | 1.8 | 1.9 | 2.0 | 2.1 |
c2 | 1.9536 | 2.0963 | 2.2390 | 2.3817 | 2.5344 | 2.6670 | 2.8097 | 2.9524 | 3.0951 | 3.2378 |
Remark 4.1. Figure 5 illustrates the state responses of the GNNs, represented by the variable x(t), in the absence of cyber-attacks. The graph displays three distinct curves, each corresponding to a specific experimental condition. The first curve represents the state response of the GNNs under normal operating conditions. The second curve represents the state response of the GNNs when subjected to external disturbances. The third curve demonstrates the state response of the GNNs when variations are introduced into the system. By examining these three curves, we can gain a comprehensive understanding of the GNNs' behavior under different experimental conditions without the presence of cyber-attacks.
This article proposes the decentralized event-triggered method and feedback controller to ensure the finite-time boundedness of GNNs with mixed interval time-varying delays and stochastic cyber-attacks. By the Lyapunov-Krasovskii stability theory, we apply the integral inequality with the exponential function to estimate the derivative of the LKFs. We also present new sufficient conditions in the form of linear matrix inequalities. The event-triggered approach reduces the network's resource utilization and transmission burden, while the random cyber-attacks are described applying Bernoulli distributed variables. A numerical example is provided to demonstrate the effectiveness and advantages of the proposed control scheme. Additionally, this research can be expanded in the future to include various dynamic systems, such as uncertain NNs [40], complex networks [41], neutral high-order Hopfield NNs [42], neutral-type NNs [43], quaternion-valued neural networks [44], spiking NNs [45], memristive NNs [46], stochastic memristive NNs [47] and synchronization of Lur'e Systems [48]. By focusing on these research directions, the proposed method can be further enhanced to achieve improved performance such as passivity [8], dissipativity [9], H∞ [5,22,25,26], and extended dissipative performances [12,15,16], even in the presence of cyber-attacks. These advancements will greatly contribute to the development of more robust and high-performing control systems specifically designed for networked applications.
The authors declare they have not used Artificial Intelligence (AI) tools in the creation of this article.
This research was supported by the Fundamental Fund of Khon Kaen University. The research on finite-time decentralized event-triggered feedback control for generalized neural networks with mixed interval time-varying delays and cyber-attacks by Khon Kaen University has received funding support from the National Science, Research and Innovation Fund or NSRF.
The authors declare that there is no conflict of interests regarding the publication of this paper.
[1] |
L. O. Chua, L. Yang, Cellular neural networks: Applications, IEEE Trans. Circuits Syst., 35 (1988), 1273–1290. https://doi.org/10.1109/31.7601 doi: 10.1109/31.7601
![]() |
[2] | A. Cochocki, R. Unbehauen, Neural networks for optimization and signal processing, Chichester: Wiley, 1993. https://doi.org/10.1002/acs.4480080309 |
[3] |
G. Joya, M. A. Atencia, F. Sandoval, Hopfield neural networks for optimization: Study of the different dynamics, Neurocomputing, 43 (2002), 219–237. https://doi.org/10.1016/S0925-2312(01)00337-X doi: 10.1016/S0925-2312(01)00337-X
![]() |
[4] |
W. J. Li, T. Lee, Hopfield neural networks for affine invariant matching, IEEE Trans. Neural Netw., 12 (2001), 1400–1410. https://doi.org/10.1109/72.963776 doi: 10.1109/72.963776
![]() |
[5] |
M. S. Ali, S. Saravanan, Q. Zhu, Non-fragile finite-time H∞ state estimation of neural networks with distributed time-varying delay, J. Franklin. Inst., 354 (2017), 7566–7584. https://doi.org/10.1016/j.jfranklin.2017.09.002 doi: 10.1016/j.jfranklin.2017.09.002
![]() |
[6] |
O. M. Kwon, M. J. Park, J. H. Park, S. M. Lee, E. J. Cha, New and improved results on stability of static neural networks with interval time-varying delays, Appl. Math. Comput., 239 (2014), 346–357. https://doi.org/10.1016/j.amc.2014.04.089 doi: 10.1016/j.amc.2014.04.089
![]() |
[7] |
U. K. Raja, R. Raja, R. Samidurai, A. Leelamani, Exponential stability for stochastic delayed recurrent neural networks with mixed time-varying delays and impulses: The continuous-time case, Phys. Scr., 87 (2013), 055802. https://doi.org/10.1088/0031-8949/87/05/055802 doi: 10.1088/0031-8949/87/05/055802
![]() |
[8] |
S. Rajavel, R. Samidurai, J. Cao, A. Alsaedi, B. Ahmad, Finite-time non-fragile passivity control for neural networks with time-varying delay, Appl. Math. Comput., 297 (2017), 145–158. https://doi.org/10.1016/j.amc.2016.10.038 doi: 10.1016/j.amc.2016.10.038
![]() |
[9] |
S. Saravanan, M. S. Ali, R. Saravanakumar, Finite-time non-fragile dissipative stabilization of delayed neural networks, Neural Process. Lett., 49 (2019), 573–591. https://doi.org/10.1007/s11063-018-9844-2 doi: 10.1007/s11063-018-9844-2
![]() |
[10] |
S. Senthilraj, R. Raja, Q. Zhu, R. Semidurai, New delay-interval-dependent stability criteria for static neural networks with time-varying delays, Neurocomputing, 186 (2016), 1–7. https://doi.org/10.1016/j.neucom.2015.12.063 doi: 10.1016/j.neucom.2015.12.063
![]() |
[11] |
R. Vadivel, P. Hammachukiattikul, G. Rajchakit, M. S. Ali, B. Unyong, Finite-time event-triggered approach for recurrent neural networks with leakage term and its application, Math. Comput. Simul., 182 (2021), 765–790. https://doi.org/10.1016/j.matcom.2020.12.001 doi: 10.1016/j.matcom.2020.12.001
![]() |
[12] |
C. Zamart, T. Botmart, W. Weera, S. Charoensin, New delay-dependent conditions for finite-time extended dissipativity based non-fragile feedback control for neural networks with mixed interval time-varying delays, Math. Comput. Simul., 201 (2022), 684–713. https://doi.org/10.1016/j.matcom.2021.07.007 doi: 10.1016/j.matcom.2021.07.007
![]() |
[13] |
X. M. Zhang, Q. L. Han, Global asymptotic stability for a class of generalized neural networks with interval time-varying delays, IEEE Trans. Neural Netw., 22 (2011), 1180–1192. https://doi.org/10.1109/TNN.2011.2147331 doi: 10.1109/TNN.2011.2147331
![]() |
[14] |
Z. Feng, H. Shao, L. Shao, Further improved stability results for generalized neural networks with time-varying delays, Neurocomputing, 367 (2019), 308–318. https://doi.org/10.1016/j.neucom.2019.07.019 doi: 10.1016/j.neucom.2019.07.019
![]() |
[15] |
R. Manivannan, R. Samidurai, J. Cao, A. Alsaedi, F. E. Alsaadi, Design of extended dissipativity state estimation for generalized neural networks with mixed time-varying delay signals, Inf. Sci., 424 (2018), 175–203. https://doi.org/10.1016/j.ins.2017.10.007 doi: 10.1016/j.ins.2017.10.007
![]() |
[16] |
R. Manivannan, R. Samidurai, J. Cao, A. Alsaedi, F. E. Alsaadi, Non-fragile extended dissipativity control design for generalized neural networks with interval time-delay signals, Asian J. Control., 21 (2019), 559–580. https://doi.org/10.1002/asjc.1752 doi: 10.1002/asjc.1752
![]() |
[17] |
P. Prasertsang, T. Botmart, Improvement of finite-time stability for delayed neural networks via a new Lyapunov-Krasovskii functional, AIMS Math., 6 (2020), 998–1023. https://doi.org/10.3934/math.2021060 doi: 10.3934/math.2021060
![]() |
[18] |
L. Sun, Y. Tang, W. Wang, S. Shen, Stability analysis of time-varying delay neural networks based on new integral inequalities, J. Franklin. Inst., 357 (2020), 10828–10843. https://doi.org/10.1016/j.jfranklin.2020.08.017 doi: 10.1016/j.jfranklin.2020.08.017
![]() |
[19] |
D. Yue, E. Tian, Q. L. Han, A delay system method for designing event-triggered controllers of networked control systems, IEEE Trans. Autom. Contr., 58 (2012), 475–481. https://doi.org/10.1109/TAC.2012.2206694 doi: 10.1109/TAC.2012.2206694
![]() |
[20] |
Y. Liu, J. H. Park, B. Z. Guo, F. Fang, F. Zhou, Event‐triggered dissipative synchronization for Markovian jump neural networks with general transition probabilities, Int. J. Robust Nonlinear Control, 28 (2018), 3893–3908. https://doi.org/10.1002/rnc.4110 doi: 10.1002/rnc.4110
![]() |
[21] |
L. Zha, J. A. Fang, J. Liu, E. Tian, Event-triggered non-fragile state estimation for delayed neural networks with randomly occurring sensor nonlinearity, Neurocomputing, 273 (2018), 1–8. https://doi.org/10.1016/j.neucom.2017.08.011 doi: 10.1016/j.neucom.2017.08.011
![]() |
[22] |
L. Zha, E. Tian, X. Xie, Z. Gu, J. Cao, Decentralized event-triggered H∞ control for neural networks subject to cyber-attacks, Inf. Sci., 457 (2021), 141–155. https://doi.org/10.1016/j.ins.2018.04.018 doi: 10.1016/j.ins.2018.04.018
![]() |
[23] |
M. S. Ali, R. Vadivel, O. M. Kwon, K. Murugan, Event triggered finite time H∞ boundedness of uncertain Markov jump neural networks with distributed time varying delays, Neural Process. Lett., 49 (2019), 1649–1680. https://doi.org/10.1007/s11063-018-9895-4 doi: 10.1007/s11063-018-9895-4
![]() |
[24] |
J. Qiu, K. Sun, T. Wang, H. Gao, Observer-based fuzzy adaptive event-triggered control for pure-feedback nonlinear systems with prescribed performance, IEEE Trans. Fuzzy Syst., 27 (2019), 2152–2162. https://doi.org/10.1109/TFUZZ.2019.2895560 doi: 10.1109/TFUZZ.2019.2895560
![]() |
[25] |
Z. Feng, H. Shao, L. Shao, Further results on event-triggered H∞ networked control for neural networks with stochastic cyber-attacks, Appl. Math. Comput., 386 (2020), 125431. https://doi.org/10.1016/j.amc.2020.125431 doi: 10.1016/j.amc.2020.125431
![]() |
[26] |
J. Wu, C. Peng, J. Zhang, B. L. Zhang, Event-triggered finite-time H∞ filtering for networked systems under deception attacks, J. Franklin. Inst., 357 (2020), 3792–3808. https://doi.org/10.1016/j.jfranklin.2019.09.002 doi: 10.1016/j.jfranklin.2019.09.002
![]() |
[27] |
A. Farraj, E. Hammad, D. Kundur, On the impact of cyber attacks on data integrity in storage-based transient stability control, IEEE Trans. Industr. Inform., 13 (2017), 3322–3333. https://doi.org/10.1109/TII.2017.2720679 doi: 10.1109/TII.2017.2720679
![]() |
[28] |
C. Kwon, I. Hwang, Reachability analysis for safety assurance of cyber-physical systems against cyber attacks, IEEE Trans. Automat., 63 (2017), 2272–2279. https://doi.org/10.1109/TAC.2017.2761762 doi: 10.1109/TAC.2017.2761762
![]() |
[29] |
A. Y. Lu, G. H. Yang, Event-triggered secure observer-based control for cyber-physical systems under adversarial attacks, Inf. Sci., 420 (2017), 96–109. https://doi.org/10.1016/j.ins.2017.08.057 doi: 10.1016/j.ins.2017.08.057
![]() |
[30] |
J. Liu, T. Yin, X. Xie, E. Tian, S. Fei, Event-triggered state estimation for T-S fuzzy neural networks with stochastic cyber-attacks, Int. J. Fuzzy Syst., 21 (2019), 532–544. https://doi.org/10.1007/s40815-018-0590-4 doi: 10.1007/s40815-018-0590-4
![]() |
[31] | P. Dorato, Short-time stability linear time-varying systems, Polytechnic Institute of Brooklyn, 1961. |
[32] |
F. Amato, M. Ariola, P. Dorato, Finite-time control of linear systems subject to parametric uncertainties and disturbances, Automatica, 37 (2001), 1459–1463. https://doi.org/10.1016/S0005-1098(01)00087-5 doi: 10.1016/S0005-1098(01)00087-5
![]() |
[33] |
A. Pratap, R. Raja, J. Alzabut, J. Dianavinnarasi, J. Cao, G. Rajchakit, Finite-time Mittag-Leffler stability of fractional-order quaternion-valued memristive neural networks with impulses, Neural Process. Lett., 51 (2020), 1485–1526. https://doi.org/10.1007/s11063-019-10154-1 doi: 10.1007/s11063-019-10154-1
![]() |
[34] |
S. Kanakalakshmi, R. Sakthivel, S. A. Karthick, A. Leelamani, A. Parivallal, Finite-time decentralized event-triggering non-fragile control for fuzzy neural networks with cyber-attack and energy constraints, Eur. J. Control, 57 (2021), 135–146. https://doi.org/10.1016/j.ejcon.2020.05.001 doi: 10.1016/j.ejcon.2020.05.001
![]() |
[35] |
C. Peng, E. Tian, J. Zhang, D. Du, Decentralized event-triggering communication scheme for large-scale systems under network environments, Inf. Sci., 380 (2017), 132–144. https://doi.org/10.1016/j.ins.2015.06.036 doi: 10.1016/j.ins.2015.06.036
![]() |
[36] | K. Gu, J. Chen, V. L. Kharitonov, Stability of time-delay systems, Boston: Birkhauser, 2003. https://doi.org/10.1007/978-1-4612-0039-0 |
[37] |
C. Zamart, T. Botmart, Further improvement of finite-time boundedness based nonfragile state feedback control for generalized neural networks with mixed interval time-varying delays via a new integral inequality, J. Inequal. Appl., 61 (2023), 61. https://doi.org/10.1186/s13660-023-02973-7 doi: 10.1186/s13660-023-02973-7
![]() |
[38] |
Q. L. Han, Y. Liu, F. Yang, Optimal communication network-based H∞ quantized control with packet dropouts for a class of discrete-time neural networks with distributed time delay, IEEE Trans. Neural Netw. Learn. Syst., 27 (2015), 426–434. https://doi.org/10.1109/TNNLS.2015.2411290 doi: 10.1109/TNNLS.2015.2411290
![]() |
[39] | S. Boyd, L. E. Ghaoui, E. Feron, V. Balakrishnan, Linear matrix inequalities in system and control theory, SIAM, 1994. https://doi.org/10.1137/1.9781611970777 |
[40] |
N. Yotha, T. Botmart, K. Mukdasai, W. Weera, Improved delay-dependent approach to passivity analysis for uncertain neural networks with discrete interval and distributed time-varying delays, Vietnam J. Math., 45 (2017), 721–736. https://doi.org/10.1007/s10013-017-0243-1 doi: 10.1007/s10013-017-0243-1
![]() |
[41] |
Y. Li, J. Zhang, J. Lu, J. Lou, Finite-time synchronization of complex networks with partial communication channels failure, Inf. Sci., 634 (2023), 539–549. https://doi.org/10.1016/j.ins.2023.03.077 doi: 10.1016/j.ins.2023.03.077
![]() |
[42] |
C. Aouiti, P. Coirault, F. Miaadi, E. Moulay, Finite time boundedness of neutral high-order Hopfield neural networks with time delay in the leakage term and mixed time delays, Neurocomputing, 260 (2017), 378–392. https://doi.org/10.1016/j.neucom.2017.04.048 doi: 10.1016/j.neucom.2017.04.048
![]() |
[43] |
R. Manivannan, R. Samidurai, J. Cao, A. Alsaedi, F. E. Alsaadi, Delay-dependent stability criteria for neutral-type neural networks with interval time-varying delay signals under the effects of leakage delay, Adv. Differ. Equ., 2018 (2018), 53. https://doi.org/10.1186/s13662-018-1509-y doi: 10.1186/s13662-018-1509-y
![]() |
[44] |
T. Peng, J. Zhong, Z. Tu, J. Lu, J. Lou, Finite-time synchronization of quaternion-valued neural networks with delays: A switching control method without decomposition, Neural Netw., 148 (2022), 37–47. https://doi.org/10.1016/j.neunet.2021.12.012 doi: 10.1016/j.neunet.2021.12.012
![]() |
[45] |
Z. Deng, C. Wang, H. Lin, Y. Sun, A Memristive spiking neural network circuit with selective supervised attention algorithm, IEEE Trans. Comput. Aided Des. Integr. Circuits Syst., 2022 (2022), 1–14. https://doi.org/10.1109/TCAD.2022.3228896 doi: 10.1109/TCAD.2022.3228896
![]() |
[46] |
C. Zhou, C. Wang, Y. Sun, W. Yao, H. Lin, Cluster output synchronization for memristive neural networks, Inf. Sci., 589 (2022), 459–477. https://doi.org/10.1016/j.ins.2021.12.084 doi: 10.1016/j.ins.2021.12.084
![]() |
[47] |
Z. Chao, C. Wang, W. Yao, Quasi-synchronization of stochastic memristive neural networks subject to deception attacks, Nonlinear Dyn., 111 (2023), 2443–2462. https://doi.org/10.1007/s11071-022-07925-2 doi: 10.1007/s11071-022-07925-2
![]() |
[48] |
Y. Ni, Z. Wang, Y. Fan, J. Lu, H. Shen, A switching memory-based event-trigger scheme for synchronization of Lur'e systems with actuator saturation: A hybrid Lyapunov method, IEEE Trans. Neural Netw. Learn. Syst., 2023 (2023), 1–12. https://doi.org/10.1109/TNNLS.2023.3273917 doi: 10.1109/TNNLS.2023.3273917
![]() |
Step | Description |
1 | Initialize network parameters, event-triggering thresholds and cyber-attack detection |
mechanisms | |
2 | Sample input signals, check event-triggering conditions and detect potential |
cyber-attacks | |
3 | Update network states, transmit relevant information among nodes and implement |
countermeasures against cyber-attacks |
c1 | 1.2 | 1.3 | 1.4 | 1.5 | 1.6 | 1.7 | 1.8 | 1.9 | 2.0 | 2.1 |
c2 | 3.1058 | 3.3361 | 3.5674 | 3.7970 | 4.0288 | 4.2617 | 4.4885 | 4.7190 | 4.9508 | 5.1788 |
c1 | 1.2 | 1.3 | 1.4 | 1.5 | 1.6 | 1.7 | 1.8 | 1.9 | 2.0 | 2.1 |
c2 | 1.9536 | 2.0963 | 2.2390 | 2.3817 | 2.5344 | 2.6670 | 2.8097 | 2.9524 | 3.0951 | 3.2378 |
Step | Description |
1 | Initialize network parameters, event-triggering thresholds and cyber-attack detection |
mechanisms | |
2 | Sample input signals, check event-triggering conditions and detect potential |
cyber-attacks | |
3 | Update network states, transmit relevant information among nodes and implement |
countermeasures against cyber-attacks |
c1 | 1.2 | 1.3 | 1.4 | 1.5 | 1.6 | 1.7 | 1.8 | 1.9 | 2.0 | 2.1 |
c2 | 3.1058 | 3.3361 | 3.5674 | 3.7970 | 4.0288 | 4.2617 | 4.4885 | 4.7190 | 4.9508 | 5.1788 |
c1 | 1.2 | 1.3 | 1.4 | 1.5 | 1.6 | 1.7 | 1.8 | 1.9 | 2.0 | 2.1 |
c2 | 1.9536 | 2.0963 | 2.2390 | 2.3817 | 2.5344 | 2.6670 | 2.8097 | 2.9524 | 3.0951 | 3.2378 |