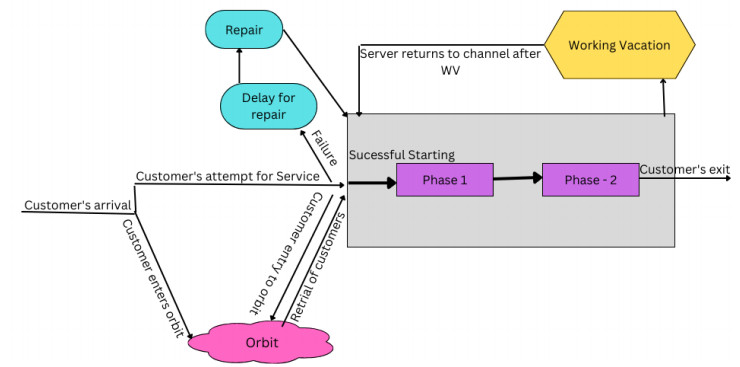
This paper investigates an unreliable M/G(P1,P2)/1 retrial queueing system with a woking vacation. An arriving customer successfully starts the first phase service with the probability α or the server fails with the probability ˉα. Once failure happens, the serving customer is taken to the orbit. The failed server is taken for repair with some delay. Once the repair is comleted, the server is ready to provide service once again. In this background, we implemented the working vacation scenario. During working vacation, the service will be provided at a slower rate, rather than entirely stopping the service. The supplementary variable method was adopted to find the orbit and system lengths. Additionally, some unique results and numerical evaluations have been presented.
Citation: Bharathy Shanmugam, Mookkaiyah Chandran Saravanarajan. Unreliable retrial queueing system with working vacation[J]. AIMS Mathematics, 2023, 8(10): 24196-24224. doi: 10.3934/math.20231234
[1] | S. Sundarapandiyan, S. Nandhini . Sensitivity analysis of a non-Markovian feedback retrial queue, reneging, delayed repair with working vacation subject to server breakdown. AIMS Mathematics, 2024, 9(8): 21025-21052. doi: 10.3934/math.20241022 |
[2] | Zhen Wang, Liwei Liu, Yuanfu Shao, Yiqiang Q. Zhao . Joining strategies under two kinds of games for a multiple vacations retrial queue with $ N $-policy and breakdowns. AIMS Mathematics, 2021, 6(8): 9075-9099. doi: 10.3934/math.2021527 |
[3] | Rani Rajendiran, Indhira Kandaiyan . Transient scrutiny of $ M^X/G(a, b)/1 $ queueing system with feedback, balking and two phase of service subject to server failure under Bernoulli vacation. AIMS Mathematics, 2023, 8(3): 5391-5412. doi: 10.3934/math.2023271 |
[4] | Yuejiao Wang, Chenguang Cai . Equilibrium strategies of customers and optimal inventory levels in a make-to-stock retrial queueing system. AIMS Mathematics, 2024, 9(5): 12211-12224. doi: 10.3934/math.2024596 |
[5] | Shaojun Lan, Yinghui Tang . An unreliable discrete-time retrial queue with probabilistic preemptive priority, balking customers and replacements of repair times. AIMS Mathematics, 2020, 5(5): 4322-4344. doi: 10.3934/math.2020276 |
[6] | YanLing Li, GenQi Xu, Hao Chen . Analysis of two components parallel repairable degenerate system with vacation. AIMS Mathematics, 2021, 6(10): 10602-10619. doi: 10.3934/math.2021616 |
[7] | K. Jeganathan, S. Selvakumar, N. Anbazhagan, S. Amutha, Porpattama Hammachukiattikul . Stochastic modeling on M/M/1/N inventory system with queue-dependent service rate and retrial facility. AIMS Mathematics, 2021, 6(7): 7386-7420. doi: 10.3934/math.2021433 |
[8] | Mengrao Ma, Linmin Hu, Yuyu Wang, Fang Luo . Discrete-time stochastic modeling and optimization for reliability systems with retrial and cold standbys. AIMS Mathematics, 2024, 9(7): 19692-19717. doi: 10.3934/math.2024961 |
[9] | Ciro D'Apice, Alexander Dudin, Sergei Dudin, Rosanna Manzo . Analysis of a multi-server retrial queue with a varying finite number of sources. AIMS Mathematics, 2024, 9(12): 33365-33385. doi: 10.3934/math.20241592 |
[10] | Linhong Li, Wei Xu, Zhen Wang, Liwei Liu . Improving efficiency of the queueing system with two types of customers by service decomposition. AIMS Mathematics, 2023, 8(11): 25382-25408. doi: 10.3934/math.20231295 |
This paper investigates an unreliable M/G(P1,P2)/1 retrial queueing system with a woking vacation. An arriving customer successfully starts the first phase service with the probability α or the server fails with the probability ˉα. Once failure happens, the serving customer is taken to the orbit. The failed server is taken for repair with some delay. Once the repair is comleted, the server is ready to provide service once again. In this background, we implemented the working vacation scenario. During working vacation, the service will be provided at a slower rate, rather than entirely stopping the service. The supplementary variable method was adopted to find the orbit and system lengths. Additionally, some unique results and numerical evaluations have been presented.
Retrial queues with unreliable servers are extensively researched in a variety of fields, such as telephone switching systems, call centers, computer communication and networks of telecommunication, automobile industries, and manufacturing systems. Here, the retrial queuing model is reflected in customer service requirements. For example, if arriving customers found the server to be unavailable, busy, under repair, and on working vacation, they would have joined a retrial group. This term is called an orbit and requests the service at a later time.
This model played in a wide range in the field of telecommunications and engineering literary works, as well as in mathematical queueing theory. Falin [1] surveyed and discussed the considerable aspects of retrial queues. Falin and Templeton [2] dealt with more information about retrial queues. Artalejo and Gomez-Corral [3], Gomez-Corral and Antonio [4], Artalejo and Jesus [5] have deeply delved into the historical background of retrial queues. Mohamed [6] investigated an unreliable retrial model and framed it with a single server, customer balking, and server undergoing breakdown and repairs. Gao and Zhang [7] focused on retrial queues linked with orbital search and applied them to call centers. Mohammadi et al. [8] considered an M/G/1 retrial model which is embedded with k consecutive heterogeneous service processes and vacations ran under the transient state. Sztrik et al. [9] looked at a unique retrial queues system that implied a two-way communication mechanism because the models suddenly gained a lot of attention.
The servicing pattern played an important role in the modelling of retry waits. There have been studies conducted on a variety of services such as including phase services, optional services, multi-optional services, and single services. The service is provided to the customer as either a single phase or more than a single phase. In a network communication scenario, the data transmission process has two phases: In the first phase, the availability of the communication channels is checked, and in the second phase, the packets are transmitted to the destination. Varalakshmi et al. [10] took a look an M/G/1 retrial model where the server provided service in two phases: single vacation, Bernoulli feedback, and starting failure. JintingWang and FangWang [11] focused on retrial queues with finite sources along with two phases of services. Alem et al. [12] made a comparison of their model M/G/1 retrial queue and their M/M/1 retrial queue with two ways of communication together with n types of outgoing calls. Boualem et al. [13] conducted a thorough analysis of the single server classical retrial queue with repeated tries and customers receive service in two phases.
When the server is idle, the visiting (new or returning) customers face the starting failure. If the server launched with a predefined probability, the server would immediately be reported to the customer. If it is not, the server would be a failure. Ke [14] established a model which covered starting failure and disruption of service. Krishnakumar et al. [15] covered a retrial queue of a single server by including feedback and startup errors. Lan and Tang [16] made a study on a discrete-time Geo/G/1 retrial queueing system for the probabilistic preemptive priority, together with server startup errors and replacements in the repair with balking. Rajadurai et al. [17] carried out a wide discussion on server retrial queueing architecture with multiple working vacations. Arivudainambi et al. and Ammar et al. [18,19] discussed starting failure together with a working breakdown, displaying how the normal busy server becomes insufficient due to disasters occurring at any time and causing the major server to fail. When a server failed, the server was taken for repair and operated at a lower speed (known as the working breakdown period) during the repair period.
The server experiences failure at any time. If it happened, the repair work could not start immediately and the arbitrary amount of time is known as the delay time. After accomplishing the repair work the server instantly resumes serving the customer. Choudhury and Ke [20] invented a batch arrival retrial queue with a combination of Bernoulli vacation and delayed repair. Liu et al. [21] exhibited a multi-server retrial system that included a delayed reboot. Kumar et al., Saggou et al. and Wang et al. [22,23,24] revealed more aspects of the delay in the retrial queueing system.
Due to some of the reasons, such as the limited lifespan of the server, outside interference, server malfunctions and startup failures, the servers malfunctioned. Therefore, it required maintenance during a slow period or busy period since so much research is carried out on problematic servers and repairs are conducted in stages from the perspectives of queueing and dependability. Rajadurai et al. [25] released the queueing publications by using the retrial queueing system with repeated working vacations and vacation interruptions, where the server tends to fail and repair. Kulkarni and Choi, Abdolllahi and Salei Rad [26,27] provided a detailed background for failures and repairs of servers. Gao et al. [28] made a provocative investigation on the retrial queue, which included two different sorts of breakdowns and delays to repair in the M/G/1 retrial queue.
In the vacation queuing system, the server fully ceased to provide service while on vacation, whereas, in the working vacation scheme, the server continued to provide service at a reduced rate rather than ceasing altogether. Markovian queueing systems with working vacations have been researched to use in the area of computer systems, communication networks and production management. Bouchentouf et al. [29] made a study on the model of the multi-station unreliable machine by combining the policy of a working vacation and patient customers. Jeyakumar and Senthilnathen [30] also discussed bulk arrival and service with a multi-working vacation model. Manoharan and Majid [31] made a detailed study on multiple working vacations for the multi-server model. Murugan and Vijayalakshmi [32] worked on multiple working vacations for a single server with bulk arrival retrial queueing model, whereas Gupta and Kumar [33] denoted a wide investigation on working vacation together with servers vacation interruption for M/M/1retrial queueing model. Bouchentouf [34] made an interesting investigation on a machine model that had multi stations along with a working vacation. Zhang [35] considered the Markovian queues which contained unreliable servers undergoing working vacation.
An examination of these papers reveals that the retrial queueing model with a single server delivering two stages of important services, commencing starting failure, delayed repair, and working vacation have been studied. Our goal is to explore the performance of such a single-server queue with simultaneous two different phases of service, both the phases are mandatory. At the start, the customer's failure in the service area was taken to the orbit. When the server failed, it was taken for repair with some delay. Additionally, service will be delivered in a slower way during vacation, in light of the growing demands for flexible service in practice. It has uses in many different industries, including wired networks, email systems, local area networks, client-server networks, web servers, inventory and production, maintenance, and quality control, among others.
We have established our analysis by the following segments. The first segment 1 is supported by an introduction with a review of recent literature and Section 2 provides a pictorial and a mathematical representation of the model with a practical justification. Section 2.1 of the paper analyses a study on the system's steady state probability. Section 2.2 engages with the solution for the framed model under steady state. Certain measures of performance are given in Section 2.3. Additional evaluations are covered in Section 2.4. In Section 3, important numerical examples, along with a 2D and 3D representation, are created from the model's mathematical results. Section 4 deals with the conclusion.
In addition to commencing failure, delayed repair, and two crucial phase services with a working vacation, the framed M/G(P1,P2)/1 retrial queueing model was also taken into consideration. The following are extensive instructions for using the framed model.
Figure 1 shows the diagram of M/G(P1,P2)/1 model.
● The arrival process: Customers enter the system in a Poisson stream with an arrival rate of ˆλ, (ˆλ>0). The arriving customer receives service right away after discovering the server to be open and then exits the system.
● The retrial process: If a customer arrives and discovers the server is with another customer or has left to provide low-cost service while on vacation, they are whisked away to a crowd of backed-up customers known as the orbit from that service region. The request for service is then repeated later. The primary customer enters the orbit by discovering the server to be busy and then tries for repeated requests of service later on at a random time, on the basis of the "First Come, First Out" discipline. It is also assumed that the waiting area is not vacant. It was assumed that the inter-retrial periods follow a general random variable ˆA along with the distribution function ˆA(t), and its related density function θ(˜x) with an equivalent Laplace-Stieltijes transform (LST) ˆA∗(Φ) govern the time between trials.
● Regular two phase services: If a new customer or a customer from an orbit who is taking a trial sees the server to be free at a typically busy period, they must engage the server to become busy. Once the service has started successfully, the customer will be served right away with the requested service with a specific probability of α; on the other hand, failure will start right away with a probability of ˉα. The customer who is in the service area is taken to orbit after the beginning failure occurs. After an arbitrary amount of time, the orbited customer accepts their request. During the period of the regular service time, both the phase of the essential service was provided by only one server having a general distribution with rate μb, μsb for the first phase P1 and second phase P2, which follows a random variable ˆLb and ˆˆRb, respectively, with probability distribution function ˆLb(t), and ˆˆRsb(t) with Laplace-Stieltjes transforms ˆL∗b(Φ) and ˆˆR∗b(Φ), provided that the first and second moments are E(ˆLb), E(ˆˆRb) and E(ˆL2b), E(ˆˆR2b), respectively.
● The process of working vacation (WV): When the server discovers that the orbit is empty, they promptly take a working holiday. The server's rate of service is low during the working vacation period, which is indicated by μv; accomplishments made on the spot during the vacation period, followed by a random variable through ˆWv with a probability distribution function ˆWv(t) and Laplace-Stieltjes transforms ˆW∗v(Φ), provided that the first and second moments are E(ˆWv), E(ˆW2v) respectively. Instead of entirely ceasing service throughout this process, a low rate of service μv is provided to the arriving customer. When the low-cost service finishes, the switch from μv to μb occurs.
● Delayed repair: The service will be stopped for a newly arriving customer at the event of a breakdown, that customer will be moved to the orbit from the service station and retrial their request after a certain period of time, while that server is not immediately scheduled for repair but is issued a delay in repair. This process is followed by a random variable ˆEf, together with the probability distribution provided by ˆEf(t) and the Laplace-Stieltjes transforms ˆE∗f(Φ), provided that the first and second moments are E(ˆEf), E(ˆE2f) respectively.
● Repair process: The service will be stopped for newly arriving customers at the moment of a breakdown, and that customer will be moved to the orbit from the service station before starting their requests after a random period of time, while that server is immediately scheduled for repair. Then the random variable ˆSf is then used and the probability distribution is provided by ˆSf(t) and the Laplace-Stieltjes transform ˆS∗f(Φ), provided that the first and second moments are E(ˆSf), E(ˆS2f), respectively.
Inter-arrival time, first and second-phase service during the typical busy season, low service rate, and delays in repair time are all regarded as independent of one another. Numerous stochastic methods are intertwined with this system.
Practical justification
Customer support ticketing system: The customer service ticketing system is one of the most significant pieces of software used by top service providers and customer support operations. Teams may manage and keep track of customer concerns with these tools, all in a very coordinated and cooperative way. The ticket system centralises all client communications via email, web forms, phone, video, social media, SMS, and chat. The banking, insurance, automobile, utility, e-commerce, software, telecommunications, and other industries all use ticketing systems. When customers submitted support tickets, their arrival can be modelled as a Poisson process. The system may experience starting failures due to technical issues or overloaded servers. The support tickets went through a two-phase service: In the first phase (P1), the tickets are assigned to a support representative, and in the second phase (P2), the representative addressed the issue and provided a resolution. If the representative is busy, the tickets are stored in a buffer(orbit) and retried later. The system may also experience delays in repairing any technical problems that arise, and a repair process is initiated to restore the system to an operational status. We have established that this work encompasses two crucial service phases, commencing with starting failure, and including a working vacation.
Equations of differential difference under steady state for the framed structure were thought through by including additional variables such as elapsed time for retrial, service, working vacation, and delayed repair. Now, the system's and orbit's customer numbers, along with the server's current condition probability generating function (PGF) are acquired.
Stability conditions: By the time of steady state, it was assumed that when ˜x=0,ˆA(0)=0,ˆA(∞)=1,ˆLb(0)=0,ˆLb(∞)=1,ˆˆRb(0)=0,ˆˆRb(∞)=1,ˆWv(0)=0,ˆWv(∞)=1,ˆEf(0)=0,ˆEf(∞)=1, ˆSf(0)=0, and ˆSf(∞)=1 are continuous, by which, the conditional rate of completion for retrial, first phase service, second phase service, working vacation, delayed repair and for repair are given by the following:
The hazard rate θ(˜x) is assigned for retrial of ˆA(˜x):θ(˜x)=dˆA(˜x)1−ˆA(˜x);
The hazard rate μb(˜x) is assigned for first phase service of ˆLb(˜x) : μb(˜x)=dˆLb(˜x)1−ˆLb(˜x);
The hazard rate μsb(˜x) is assigned for second phase service of ˆˆRb(˜x): μsb(˜x)=dˆˆRb(˜x)1−ˆˆRb(˜x);
The hazard rate μv(˜x) is assigned for lower rate service of ˆWv(˜x): μv(˜x)=dˆWv(˜x)1−ˆWv(˜x);
The hazard rate η(˜x) is assigned for delayed repair of ˆEf(˜x): η(˜x)=dˆEf(˜x)1−ˆEf(˜x); and
The hazard rate ϑ(˜x) is assigned for repair of ˆSf(˜x): ϑ(˜x)=dˆSf(˜x)1−ˆSf(˜x).
Apart from these, ˆA0(t),ˆL0b(t),ˆˆR0b(t),ˆW0v(t),ˆE0f(t),ˆS0f(t) were taken to be elapsed retrial times, elapsed first phase service, elapsed second phase service, elapsed lower rate service, elapsed delay and repairs respectively. Furthermore, introducing the random variables,
ˇΔ(t)={0 The server seems to be in idle.1The server seems to be busy with the first phase service.2 The server seems to be busy with second phase service.3The server seems to be in working vacation period and server is busy.4 The server seems to be on delay.5 The server seems to be on regular repair period. |
Moreover, we see that the system's state at time t can be characterised by the bivariate Markov process {ˇΔ(t),˙N(t);t≥0}; here, ˇΔ(t) represents the state of the server as (0,1,2,3,4,5) based on the server's idle, P1,P2, WV, delay to repair and repair at the time t, and ˙N(t) denotes the available customer's number in the orbit.
To work with a Markov process where ˇΔ(t)>0, we introduce five more variables: if ˇΔ(t)=0 and ˙N(t)>0, then ˆA0(t) represents the elapsed retrial time; if ˇΔ(t)=1 and ˙N(t)>0, then ˆL0b(t) represents the elapsed first phase service time during normal busy period at time t; if ˇΔ(t)=2 and ˙N(t)>0, then ˆˆR0b(t) represents the elapsed second phase service time during normal busy period at time t; if ˇΔ(t)=3 and ˙N(t)>0, then ˆW0v(t) represents the elapsed working vacation time at time t; if ˇΔ(t)=4 and ˙N(t)>0, then ˆE0f(t) represents the elapsed delayed time to repair during normal busy period at time t; and if ˇΔ(t)=5 and ˙N(t)>0, then ˆS0f(t) reflects the amount of time spent making repair during a typical busy time t.
Ergodicity of the designed dynamic model
Let {tn;n∈1,2,...} represent a sequence of time for either the end of a service or the completion of a vacation. The random vector's sequence Zn={ˇΔ(tn,+),˙N(tn,+)} was shown as the embedded Markov chain for the suggested retrial queueing paradigm. Additionally S={0,1,2,3,4,5}×˙N is its state space.
Theorem 1. The embedded Markov chain {Zn;n∈˙N} is ergodic if and only if ρ<ˆA∗(ˆλ), where ρ=ˉα+αˆλ(E(ˆLb)+E(ˆˆRb))+ˉαˆλ(E(ˆEf)+E(ˆSf)).
Proof. It is strong where the chain {Zn;n∈˙N} seems to be not only irreducible, but also an aperiodic Markov chain.
Here, Foster's criterion, according to which an irreducible and an aperiodic Markov chain are only ergodic when a non-negative function exists, was used to demonstrate the necessary condition for ergodicity. Let it be f(ˊu), ˊu∈˙N and ∈>0 were the mean drift, which is given to be χˊu=E[(f(˜zn+1)+f(˜zn)∣˜zn=ˊu))] appears to be a finite measure for all values of ˊu∈˙N and χˊu≤−∈; the same is true for all values of ˊu∈˙N, omitting theoretically for a certain finite number ˊu. Under this circumstance, let us deliberate the function f(ˊu)=ˊu. Then we have
χˊu={ˉα+αˆλ(E(ˆLb)+E(ˆˆRb))+ˉαˆλ(E(ˆEf)+E(ˆSf))−ˆA∗(ˆλ),ˊu=1,2,…ˉα+αˆλ(E(ˆLb)+E(ˆˆRb))+ˉαˆλ(E(ˆEf)+E(ˆSf))−1,ˊu=0 |
It is clear that the necessary condition and the sufficient condition for ergodicity were given by an inequality ˉα+αˆλ(E(ˆLb)+E(ˆˆRb))+ˉαˆλ(E(ˆEf)+E(ˆSf))<ˆA∗(ˆλ).
For proving the necessary condition, as professed in Sennott et al. [40], the Markov chain {Zn;n∈N} gratifies Kaplan's form, specifically χˊu<∞ for all the values of ˊu≥0 and for the existence ˊu0∈N such that χˊu≥0 for all the datas of ˊu>ˊu0. In this case, Kaplan's condition appears to be satisfied because of the existence of ˘a such that φ˘u,ˊu=0 for ˘u>0 and ˊu<˘u−˘a, where R=(φ˘u,ˊu) was taken as a one step transition matrix of {Zn;n≥1}. Then, it was taken that the inequality ˉα+αˆλ(E(ˆLb)+E(ˆˆRb))+ˉαˆλ(E(ˆEf)+E(ˆSf))≥ˆA∗(ˆλ) indicates the non-ergodicity of the Markov chain.
For the Markov process {Zn;n∈N}, the probability was defined by
Υ0(t)=P{ˇΔ(t)=0,X(t)=0} also the probability densities are given by
Υn(˜x,t)d˜x=P{ˇΔ(t)=0,X(t)=n,˜x≤ˆA0(t)<˜x+d˜x},t≥0,˜x≥0, and n≥1
Ψn,b(˜x,t)d˜x=P{ˇΔ(t)=1,X(t)=n,˜x≤ˆL0b(t)<˜x+d˜x},t≥0,˜x≥0 and n≥0
Πn,b(˜x,t)d˜x=P{ˇΔ(t)=2,X(t)=n,˜x≤ˆˆR0b(t)<˜x+d˜x},t≥0,˜x≥0 and n≥0
℧n,v(˜x,t)d˜x=P{ˇΔ(t)=3,X(t)=n,˜x≤ˆW0v(t)<˜x+d˜x},t≥0,˜x≥0 and n≥0
ˊDn(˜x,t)d˜x=P{ˇΔ(t)=4,X(t)=n,˜x≤ˆE0f(t)<˜x+d˜x},t≥0,˜x≥0 and n≥1
Ωn(˜x,t)d˜x=P{ˇΔ(t)=4,X(t)=n,˜x≤S0f(t)<˜x+d˜x},t≥0,˜x≥0 and n≥1
In the parts that follow, the probabilities listed were used.
Υn(˜x,t): At time t, the probability of accurately n customers present in the orbit at the time of retrial along with the ˜x amount elapsed retrial time.
Ψn,b(˜x,t): At time t, the probability of accurately n customers present in the orbit at the time of first phase service along with ˜x amount of elapsed service time for the first phase.
Πn,b(˜x,t): At time t, the probability of accurately n customers present in the orbit at the time of second phase of service along with ˜x amount of elapsed service time for the second phase.
℧n,v(˜x,t): At time t, the probability of accurately n customer present in the orbit at the time of working vacation along with ˜x amount of elapsed low rate service time.
ˊDn(˜x,t): At time t, the probability of accurately n customer present in the orbit at the time of delay for repair along with ˜x amount of elapsed delayed time.
Ωn(˜x,t): At time t, the probability of accurately n customer present in the orbit at the time of repair along with ˜x amount of elapsed repair time.
We can establish t≥0, ˜x≥0 and n≥1 because of the assumption of the satisfaction of the stability condition. Additionally, we present the random variable as follows:
Υ0=lim |
The system's governing equations
Depending on the assumptions made above, the differential difference equations for the model was designed with the help of the following supplementary variable technique:
\begin{align} \hat{\lambda} \varUpsilon_{0} = &\int_{0}^{\infty}\mho_{0,v}(\tilde{x})\mu_{v}(\tilde{x})d\tilde{x} \end{align} | (2.1) |
\begin{align} \frac{d}{d\tilde{x}}\varUpsilon_{n}(\tilde{x})+(\hat{\lambda} +\theta(\tilde{x}))\varUpsilon_{n}(\tilde{x}) = &0, n\geq1 \end{align} | (2.2) |
\begin{align} \frac{d}{d\tilde{x}}\varPsi_{0,b}(\tilde{x})+(\hat{\lambda}+\mu_{b}(\tilde{x}))\varPsi_{0,b}(\tilde{x}) = &0, n = 0 \end{align} | (2.3) |
\begin{align} \frac{d}{d\tilde{x}}\varPsi_{n,b}(\tilde{x})+(\hat{\lambda} +\mu_{b})(\tilde{x})\varPsi_{n,b}(\tilde{x}) = &\hat{\lambda} \varPsi_{n-1,b}(\tilde{x}), n\geq1 \end{align} | (2.4) |
\begin{align} \frac{d}{d\tilde{x}}\Pi_{0,b}(\tilde{x})+(\hat{\lambda} +\mu_{sb}(\tilde{x}))\Pi_{0,b}(\tilde{x}) = &0, n = 0 \end{align} | (2.5) |
\begin{align} \frac{d}{d\tilde{x}}\Pi_{n,b}(\tilde{x})+(\hat{\lambda} +\mu_{sb}(\tilde{x}))\Pi_{n,b}(\tilde{x}) = &\hat{\lambda} \Pi_{n-1,b}(\tilde{x}),n\geq1 \end{align} | (2.6) |
\begin{align} \frac{d}{d\tilde{x}}\mho_{0,v}(\tilde{x})+(\hat{\lambda} +\mu_{v}(\tilde{x}))\mho_{0,v}(\tilde{x}) = &0, n = 0 \end{align} | (2.7) |
\begin{align} \frac{d}{d\tilde{x}}\mho_{n,v}(\tilde{x})+(\hat{\lambda} +\mu_{v}(\tilde{x}))\mho_{n,v}(\tilde{x}) = &\hat{\lambda} \mho_{n-1,v}(\tilde{x}), n\geq1 \end{align} | (2.8) |
\begin{align} \frac{d}{d\tilde{x}}\acute{D}_{n}(\tilde{x})+(\hat{\lambda} +\acute{d}(\tilde{x}))\mho_{n}(\tilde{x}) = &\hat{\lambda} \acute{D}_{n-1}(\tilde{x}),n\geq1 \end{align} | (2.9) |
\begin{align} \frac{d}{d\tilde{x}}\varOmega_{0}(\tilde{x}) = &0,n = 0 \end{align} | (2.10) |
\begin{align} \frac{d}{d\tilde{x}}\varOmega_{n}(\tilde{x})+(\hat{\lambda} +v(\tilde{x}))\varOmega_{n}(\tilde{x}) = &\hat{\lambda} \varOmega_{n-1}(\tilde{x}),n\geq1. \end{align} | (2.11) |
The stationary probability solutions for this model occur as non negative iff \bar{\alpha}+\alpha \hat{\lambda} (E(\hat{L}_{b})+E(\hat{\hat{R}}_{b}))+\bar{\alpha}\hat{\lambda}(E(E_{f})+\hat{\lambda} E(\hat{S}_{f})) < A^{*}(\hat{\lambda}) , [possible under the ergodicity condition only].
The system's steady state boundary conditions when \tilde{x} = 0 ,
\begin{align} \varUpsilon_{n}(0)& = \int_{0}^{\infty}\mho_{n,v}(\tilde{x})\mu_{v}(\tilde{x})d\tilde{x}+ \int_{0}^{\infty}\Pi_{n,b}(\tilde{x})\mu_{sb}(\tilde{x})d\tilde{x}+ \int_{0}^{\infty}\varOmega_{n}(\tilde{x})v(\tilde{x})d\tilde{x}, n\geq1 \end{align} | (2.12) |
\begin{align} \varPsi_{0,b}(0)& = \alpha \hat{\lambda} \varUpsilon_{0}+\alpha\int_{0}^{\infty}\varUpsilon_{1}(\tilde{x})\theta(\tilde{x})d\tilde{x},\quad n = 0 \end{align} | (2.13) |
\begin{align} \varPsi_{n,b}(0)& = \alpha\hat{\lambda}\int_{0}^{\infty}\varUpsilon_{n}(\tilde{x})d\tilde{x}+\alpha\int_{0}^{\infty}\varUpsilon_{n+1}(\tilde{x})\theta(\tilde{x})d\tilde{x}, \quad n\geq1 \end{align} | (2.14) |
\begin{align} \Pi_{0,b}(0)& = \int_{0}^{\infty}\varPsi_{0,b}(\tilde{x})\mu_{b}(\tilde{x})d\tilde{x},\quad n = 0 \end{align} | (2.15) |
\begin{align} \Pi_{n,b}(0)& = \int_{0}^{\infty}\varPsi_{n,b}(\tilde{x})\mu_{b}(\tilde{x})d\tilde{x},\quad n\geq1 \end{align} | (2.16) |
\begin{align} \mho_{0,v}(0)& = \int_{0}^{\infty}\Pi_{0,b}(\tilde{x})\mu_{sb}(\tilde{x})d\tilde{x},\quad n = 0 \end{align} | (2.17) |
\begin{align} \mho_{0,n}(0)& = 0,\quad n = 0 \end{align} | (2.18) |
\begin{align} \acute{D}_{1}(0)& = \bar{\alpha}\int_{0}^{\infty}\varUpsilon_{1}(\tilde{x})\theta(\tilde{x})d\tilde{x}+ \bar{\alpha}\hat{\lambda}\varUpsilon_{0} , \quad n\geq1 \end{align} | (2.19) |
\begin{align} \acute{D}_{n}(0)& = \bar{\alpha}\int_{0}^{\infty}\varUpsilon_{n}(\tilde{x})\theta(\tilde{x})d\tilde{x}+ \bar{\alpha}\hat{\lambda}\int_{0}^{\infty}\varUpsilon_{n-1}(\tilde{x})d\tilde{x}, \quad n\geq2 \end{align} | (2.20) |
\begin{align} \varOmega_{n}(0)& = \int_{0}^{\infty}\acute{D}_{n}(\tilde{x})\eta(\tilde{x})d\tilde{x}, \quad n\geq1 . \end{align} | (2.21) |
The normalising condition is given by
\begin{align*} \label{22} \varUpsilon_{0}+ \sum\limits_{n = 0}^{\infty}\int_{0}^{\infty}\varUpsilon_{n}(\tilde{x})d\tilde{x}+ \sum\limits_{n = 0}^{\infty}\int_{0}^{\infty}\varPsi_{n,b}(\tilde{x})d\tilde{x}+ \sum\limits_{n = 0}^{\infty}\int_{0}^{\infty}\Pi_{n,b}(\tilde{x})d\tilde{x}+ \sum\limits_{n = 0}^{\infty}\int_{0}^{\infty} \mho_{n,v}(\tilde{x})d\tilde{x} \end{align*} |
\begin{align} + \sum\limits_{n = 0}^{\infty}\int_{0}^{\infty}\varOmega_{n}(\tilde{x})d\tilde{x} = 0. \end{align} | (2.22) |
Here, the technique used for the retrial queueing model to reach its steady state solution is probability generating function (PGF). The generating functions used for solving the overhead equations are defined as follows: \lvert\tilde{z}\lvert\leq1 ,
\begin{align*} \varUpsilon(\tilde{x},\tilde{z}) = \sum\limits_{n = 1}^{\infty}\varUpsilon_{n}(\tilde{x})\tilde{z}^{n}; \quad \varUpsilon(0,\tilde{z}) = \sum\limits_{n = 1}^{\infty}\varUpsilon_{n}(0)\tilde{z}^{n}; \quad \varPsi_{b}(\tilde{x},\tilde{z}) = \sum\limits_{n = 0}^{\infty}\varPsi_{n,b}(\tilde{x})\tilde{z}^{n}; \quad\\ \varPsi_{b}(0,\tilde{z}) = \sum\limits_{n = 0}^{\infty}\varPsi_{n,b}(0)\tilde{z}^{n}; \quad \Pi_{b}(\tilde{x},\tilde{z}) = \sum\limits_{n = 0}^{\infty}\Pi_{n,b}(\tilde{x})\tilde{z}^{n};\quad\Pi_{b}(0,\tilde{z}) = \sum\limits_{n = 0}^{\infty}\Pi_{n,b}(0)\tilde{z}^{n};\\ \mho_{v}(\tilde{x},\tilde{z}) = \sum\limits_{n = 0}^{\infty}\mho_{n,v}(\tilde{x})\tilde{z}^{n};\quad\mho_{v}(0,\tilde{z}) = \sum\limits_{n = 0}^{\infty}G_{n,v}(0)\tilde{z}^{n}; \quad \acute{D}(\tilde{x},\tilde{z}) = \sum\limits_{n = 1}^{\infty}\acute{D}_{n}(\tilde{x})\tilde{z}^{n};\\ \acute{D}(0,\tilde{z}) = \sum\limits_{n = 1}^{\infty}\acute{D}_{n}(0)\tilde{z}^{n};\quad \varOmega(\tilde{x},\tilde{z}) = \sum\limits_{n = 1}^{\infty}U_{n}(\tilde{x})\tilde{z}^{n};\quad \varOmega(0,\tilde{z}) = \sum\limits_{n = 1}^{\infty}U_{n}(0)\tilde{z}^{n}. \end{align*} |
Theorem 2. For the number of customers present in an orbit during the state of server, 0, 1, 2, 3, 4 and 5 denotes the server to be idle, engaged in normal first phase and second phase service, working vacation, delay and repair, respectively. The generating functions are given by \varUpsilon(\tilde{z}), \varPsi_{b} (\tilde{z}), \Pi_{b}(\tilde{z}), \mho_{v} (\tilde{z}) , \acute{D}(\tilde{z}), \varOmega(\tilde{z}) , where \varUpsilon_{0} was determined by the normalizing condition
\begin{align*} \varUpsilon_{0}+\varUpsilon(1)+\varPsi_{b}(1)+\Pi_{b}(1)+\mho_{v}(1)+\acute{D}(1)+\varOmega(1) = 1 \\ \varUpsilon_{0} = \frac{\widehat{W}_{v}^{*}(\hat{\lambda})\left\lbrace \hat{A}^{*}(\hat{\lambda})-(\bar{\alpha}+\alpha \hat{\lambda}(E(\hat{L}_{b})+E(\hat{\hat{R}}_{b}))+\bar{\alpha}\hat{\lambda}(E(\hat{E}_{f})+\hat{\lambda} E(\hat{S}_{f}))) \right\rbrace }{\alpha A^{*}(\hat{\lambda}) \widehat{W}_{v}^{*}(\hat{\lambda})+\alpha\hat{\lambda} E(\widehat{W}_{v})}. \end{align*} |
Proof. Multiply the Eqs (2.2)–(2.11) with the appropriate powers for \tilde{z} ; then, by summing over for n , the succeeding set of partially differential equations were obtained:
\begin{align} \frac{\partial \varUpsilon_{n}(\tilde{x},\tilde{z})}{\partial \tilde{x}}+(\hat{\lambda}+\theta(\tilde{x}))\varUpsilon(\tilde{x},\tilde{z}) = &0 \end{align} | (2.23) |
\begin{align} \frac{\partial \varPsi_{b}(\tilde{x},\tilde{z})}{\partial \tilde{x}}+(\hat{\lambda}-\hat{\lambda} \tilde{z}+\mu_{b}(\tilde{x}))\varPsi_{b}(\tilde{x},\tilde{z}) = &0 \end{align} | (2.24) |
\begin{align} \frac{\partial \Pi_{b}(\tilde{x},\tilde{z})}{\partial \tilde{x}}+(\hat{\lambda}-\hat{\lambda} \tilde{z}+\mu_{sb}(\tilde{x}))\Pi_{b}(\tilde{x},\tilde{z}) = &0 \end{align} | (2.25) |
\begin{align} \frac{\partial \mho_{v}(\tilde{x},\tilde{z})}{\partial \tilde{x}}+(\hat{\lambda}-\hat{\lambda} \tilde{z}+\mu_{v}(\tilde{x}))\mho_{v}(\tilde{x},\tilde{z}) = &0 \end{align} | (2.26) |
\begin{align} \frac{\partial \acute{D}(\tilde{x},\tilde{z})}{\partial \tilde{x}}+(\hat{\lambda}-\hat{\lambda} \tilde{z}+\eta(\tilde{x}))\acute{D}(\tilde{x},\tilde{z}) = &0 \end{align} | (2.27) |
\begin{align} \frac{\partial \varOmega(\tilde{x},\tilde{z})}{\partial \tilde{x}}+(\hat{\lambda}-\hat{\lambda} \tilde{z}+\vartheta(\tilde{x}))\varOmega(\tilde{x},\tilde{z}) = &0. \end{align} | (2.28) |
Above partial differential Eqs (2.23)–(2.28) were solved as follows
\begin{align} \varUpsilon(\tilde{x},\tilde{z})& = \varUpsilon(0,\tilde{z})e^{-\hat{\lambda} \tilde{x}}(1-\hat{A}(\tilde{x})) \end{align} | (2.29) |
\begin{align} \varPsi_{b}(\tilde{x},\tilde{z})& = \varPsi_{b}(0,\tilde{z})e^{-\hat{\lambda}(1-\tilde{z}) \tilde{x}}(1-\hat{L}_{b}(\tilde{x})) \end{align} | (2.30) |
\begin{align} \Pi_{b}(\tilde{x},\tilde{z})& = \Pi_{b}(0,\tilde{z})e^{-\hat{\lambda}(1-\tilde{z}) \tilde{x}}(1-\hat{\hat{R}}_{b}(\tilde{x})) \end{align} | (2.31) |
\begin{align} \mho_{v}(\tilde{x},\tilde{z})& = \mho_{v}(0,\tilde{z})e^{-\hat{\lambda}(1-\tilde{z}) \tilde{x}}(1-\widehat{W}_{v}(\tilde{x})) \end{align} | (2.32) |
\begin{align} \acute{D}(\tilde{x},\tilde{z})& = \acute{D}(0,\tilde{z})e^{-\hat{\lambda}(1-\tilde{z}) \tilde{x}}(1-\hat{E}_{f}(\tilde{x})) \end{align} | (2.33) |
\begin{align} \varOmega(\tilde{x},\tilde{z})& = \varOmega(0,\tilde{z})e^{-\hat{\lambda}(1-\tilde{z}) \tilde{x}}(1-\hat{S}_{f}(\tilde{x})). \end{align} | (2.34) |
Similarly solving for (2.12)–(2.21), we obtain the following:
\begin{align} \varUpsilon(0,\tilde{z})& = \int_{0}^{\infty}\mho_{v}(\tilde{x},\tilde{z})\mu_{v}(\tilde{x})d\tilde{x}+ \int_{0}^{\infty}\Pi_{b}(\tilde{x},\tilde{z})\mu_{sb}(\tilde{x})d\tilde{x}+ \int_{0}^{\infty}\varOmega(\tilde{x},\tilde{z})v(\tilde{x})d\tilde{x} \end{align} | (2.35) |
\begin{align} &-\hat{\lambda} \varUpsilon_{0}-\mho_{0,v}(0) \\ \varPsi_{b}(0,\tilde{z})& = \frac{\alpha}{\tilde{z}}\int_{0}^{\infty}\varUpsilon(\tilde{x},\tilde{z})\theta(\tilde{x})d\tilde{x}+\alpha \hat{\lambda} \int_{0}^{\infty}\varUpsilon(\tilde{x},\tilde{z})d\tilde{x} +\alpha \hat{\lambda} \varUpsilon_{0} \end{align} | (2.36) |
\begin{align} \Pi_{b}(0,\tilde{z})& = \int_{0}^{\infty}\varPsi_{b}(\tilde{x},\tilde{z})\mu_{b}(\tilde{x})d\tilde{x} \end{align} | (2.37) |
\begin{align} \mho_{v}(0,\tilde{z})& = \mho_{0,v}(0) \end{align} | (2.38) |
\begin{align} \acute{D}(0,\tilde{z})& = \bar{\alpha}\int_{0}^{\infty}\varUpsilon(\tilde{x},\tilde{z})\theta(\tilde{x})d\tilde{x}+\bar{\alpha}\hat{\lambda} \tilde{z} \int_{0}^{\infty}\varUpsilon(\tilde{x},\tilde{z})d\tilde{x} +\tilde{z}\bar{\alpha}\hat{\lambda} \varUpsilon_{0} \end{align} | (2.39) |
\begin{align} \mho_{0,v}(0)& = \frac{\hat{\lambda} \varUpsilon_{0}}{\widehat{W}_{v}^{*}(\hat{\lambda})} \end{align} | (2.40) |
\begin{align} \mho_{v}(0,\tilde{z})& = \frac{\hat{\lambda} \varUpsilon_{0}}{\widehat{W}_{v}^{*}(\hat{\lambda})} \end{align} | (2.41) |
\begin{align} \Pi_{b} (0,\tilde{z})& = \hat{L}_{b}^{*}(\hat{\lambda}(1-\tilde{z})) \left[ \frac{\alpha}{\tilde{z}} (\tilde{z}+(1-\tilde{z})A^{*}(\hat{\lambda}))\varUpsilon(0,\tilde{z})+\alpha\hat{\lambda} \varUpsilon_{0} \right] \end{align} | (2.42) |
\begin{align} \mho_{v} (0,\tilde{z})& = \Pi_{b} (0,\tilde{z})\hat{L}_{b}^{*}(\hat{\lambda}(1-\tilde{z})) \end{align} | (2.43) |
\begin{align} \acute{D}(0,\tilde{z})& = \bar{\alpha}\hat{\lambda} \tilde{z} \varUpsilon_{0}+\bar{\alpha}(\tilde{z}+(1-\tilde{z})\hat{A}^{*}(\hat{\lambda}))\varUpsilon(0,\tilde{z}) \end{align} | (2.44) |
\begin{align} \varOmega _{v} (0,\tilde{z})& = \acute{D}(0,\tilde{z})E_{f}^{*}(\hat{\lambda}(1-\tilde{z})). \end{align} | (2.45) |
Obtaining Eqs (2.31), (2.32), and (2.34) to (2.35) and then (2.41), (2.42) and (2.45) we get the following equation:
\begin{align} \varUpsilon(0,\tilde{z}) = \frac{\hat{\lambda} \tilde{z} \varUpsilon_{0}}{\widehat{W}_{v}^{*}(\hat{\lambda})} \left\lbrace \frac{ {(1-\widehat{W}_{v}^{*}(\hat{\lambda} (1-\tilde{z})))+\widehat{W}_{v}^{*}(\hat{\lambda})(1-\alpha \hat{L}_{b}^{*}(\hat{\lambda}(1-\tilde{z}))\hat{\hat{R}}_{b}^{*}(\hat{\lambda}(1-\tilde{z})))}\\{-\bar{\alpha} \tilde{z} \hat{E}_{f}^{*}(\hat{\lambda}(1-\tilde{z})) \hat{S}_{f}^{*}(\hat{\lambda}(1-\tilde{z}))}}{{(\alpha \hat{L}_{b}^{*}(\hat{\lambda}(1-\tilde{z}))\hat{\hat{R}}_{b}^{*}(\hat{\lambda}(1-\tilde{z})))+\bar{\alpha} \tilde{z}\hat{E}_{f}^{*}(\hat{\lambda}(1-\tilde{z})) \hat{S}_{f}^{*}(\hat{\lambda}(1-\tilde{z}))}\\{(\tilde{z}+(1-\tilde{z})\hat{A}^{*}(\hat{\lambda}))-\tilde{z}}}\right\rbrace. \end{align} | (2.46) |
Similarly, substitute Eq (2.29) and then (2.46) in (2.36), we get the following equation:
\begin{align} \varPsi_{b}(0,\tilde{z})& = \frac{\hat{\lambda} \alpha \varUpsilon_{0} }{\widehat{W}_{v}^{*}(\hat{\lambda})} \left\lbrace \frac{(1-\widehat{W}_{v}^{*}(\hat{\lambda} (1-\tilde{z})))(\tilde{z}+(1-\tilde{z})\hat{A}^{*}(\hat{\lambda}))+\hat{A}^{*}(\hat{\lambda}) \widehat{W}_{v}^{*}(\hat{\lambda})(1-\tilde{z})}{{(\alpha \hat{L}_{b}^{*}(\hat{\lambda}(1-\tilde{z}))\hat{\hat{R}}_{b}^{*}(\hat{\lambda}(1-\tilde{z})))+\bar{\alpha} \tilde{z}\hat{E}_{f}^{*}(\hat{\lambda}(1-\tilde{z})) \hat{S}_{f}^{*}(\hat{\lambda}(1-\tilde{z}))}\\{(\tilde{z}+(1-\tilde{z})\hat{A}^{*}(\hat{\lambda}))-\tilde{z}}}\right\rbrace . \end{align} | (2.47) |
Similarly, substitute Eq (2.46) in (2.42), we get the following equation:
\begin{align} \Pi_{b}(0,\tilde{z})& = \frac{\hat{\lambda} \alpha \varUpsilon_{0}\hat{L}_{b}^{*}(\hat{\lambda} (1-\tilde{z}))}{\widehat{W}_{v}^{*}(\hat{\lambda})} \left\lbrace \frac{(1-\widehat{W}_{v}^{*}(\hat{\lambda} (1-\tilde{z})))(\tilde{z}+(1-\tilde{z})\hat{A}^{*}(\hat{\lambda}))+\hat{A}^{*}(\hat{\lambda}) \widehat{W}_{v}^{*}(\hat{\lambda})(1-\tilde{z})}{{(\alpha \hat{L}_{b}^{*}(\hat{\lambda}(1-\tilde{z}))\hat{\hat{R}}_{b}^{*}(\hat{\lambda}(1-\tilde{z})))+\bar{\alpha} \tilde{z}\hat{E}_{f}^{*}(\hat{\lambda}(1-\tilde{z})) \hat{S}_{f}^{*}(\hat{\lambda}(1-\tilde{z}))}\\{(\tilde{z}+(1-\tilde{z})\hat{A}^{*}(\hat{\lambda}))-\tilde{z}} }\right\rbrace. \end{align} | (2.48) |
Taking Eq (2.46) to the Eq (2.44), we move to the following equation:
\begin{align} \acute{D}(0,\tilde{z})& = \frac{\hat{\lambda} \bar{\alpha}\tilde{z}\varUpsilon_{0}}{\widehat{W}_{v}^{*}(\hat{\lambda})} \left\lbrace \frac{(1-\widehat{W}_{v}^{*}(\hat{\lambda} (1-\tilde{z})))(\tilde{z}+(1-\tilde{z})\hat{A}^{*}(\hat{\lambda}))+\hat{A}^{*}(\hat{\lambda}) \widehat{W}_{v}^{*}(\hat{\lambda})(1-\tilde{z})}{(\alpha \hat{L}_{b}^{*}(\hat{\lambda}(1-\tilde{z}))\hat{\hat{R}}_{b}^{*}(\hat{\lambda}(1-\tilde{z})))+\bar{\alpha} \tilde{z} \hat{S}_{f}^{*}(\hat{\lambda}(1-\tilde{z})))(\tilde{z}+(1-\tilde{z})\hat{A}^{*}(\hat{\lambda}))-\tilde{z}}\right\rbrace . \end{align} | (2.49) |
Similarly, substitute Eq (2.49) in (2.45), we get the following equation:
\begin{align} \varOmega(0,\tilde{z})& = \frac{\hat{\lambda} \bar{\alpha}\tilde{z}\varUpsilon_{0}\hat{E}_{f}^{*}(\hat{\lambda}(1-\tilde{z}))}{\widehat{W}_{v}^{*}(\hat{\lambda})} \left\lbrace \frac{(1-\widehat{W}_{v}^{*}(\hat{\lambda} (1-\tilde{z})))(\tilde{z}+(1-\tilde{z})\hat{A}^{*}(\hat{\lambda}))+\hat{A}^{*}(\hat{\lambda}) \widehat{W}_{v}^{*}(\hat{\lambda})(1-\tilde{z}\tilde{z})}{{(\alpha \hat{L}_{b}^{*}(\hat{\lambda}(1-\tilde{z}))\hat{\hat{R}}_{b}^{*}(\hat{\lambda}(1-\tilde{z})))+\bar{\alpha} \tilde{z}\hat{E}_{f}^{*}(\hat{\lambda}(1-\tilde{z})) \hat{S}_{f}^{*}(\hat{\lambda}(1-\tilde{z}))}\\{(\tilde{z}+(1-\tilde{z})\hat{A}^{*}(\hat{\lambda}))-\tilde{z}}}\right\rbrace. \end{align} | (2.50) |
The limiting probability generating functions are established by utilizing the aforementioned equations from (2.47) to (2.49) in (2.29) to (2.34):
\begin{align} \varUpsilon(\tilde{x},\tilde{z})& = \frac{\hat{\lambda} \tilde{z} \varUpsilon_{0}}{\widehat{W}_{v}^{*}(\hat{\lambda})} \left\lbrace \frac{ {(1-\widehat{W}_{v}^{*}(\hat{\lambda} (1-\tilde{z})))+\widehat{W}_{v}^{*}(\hat{\lambda})(1-\alpha \hat{L}_{b}^{*}(\hat{\lambda}(1-\tilde{z}))}\\{\hat{\hat{R}}_{b}^{*}(\hat{\lambda}(1-\tilde{z})))-\bar{\alpha} \tilde{z} \hat{E}_{f}^{*}(\hat{\lambda}(1-\tilde{z})) \hat{S}_{f}^{*}(\hat{\lambda}(1-\tilde{z}))}} {{(\alpha \hat{L}_{b}^{*}(\hat{\lambda}(1-\tilde{z}))\hat{\hat{R}}_{b}^{*}(\hat{\lambda}(1-\tilde{z}))+\bar{\alpha} \tilde{z} \hat{E}_{f}^{*}(\hat{\lambda}(1-\tilde{z})) }\\{\hat{S}_{f}^{*}(\hat{\lambda}(1-\tilde{z})))(\tilde{z}+(1-\tilde{z})\hat{A}^{*}(\hat{\lambda}))-\tilde{z}}}\right\rbrace e^{-\hat{\lambda} \tilde{x}}(1-\hat{A}(\tilde{x})) \end{align} | (2.51) |
\begin{align} \varPsi_{b}(\tilde{x},\tilde{z})& = \frac{\hat{\lambda} \alpha P_{0}}{\widehat{W}_{v}^{*}(\hat{\lambda})} \left\lbrace \frac{{(1-\widehat{W}_{v}^{*}(\hat{\lambda} (1-\tilde{z})))(\tilde{z}+(1-\tilde{z})\hat{A}^{*}(\hat{\lambda}))+}\\{\hat{A}^{*}(\hat{\lambda})\widehat{W}_{v}^{*}(\hat{\lambda})(1-\tilde{z})}} {{(\alpha \hat{L}_{b}^{*}(\hat{\lambda}(1-\tilde{z}))\hat{\hat{R}}_{b}^{*}(\hat{\lambda}(1-\tilde{z})))+\bar{\alpha} \tilde{z} \hat{E}_{f}^{*}(\hat{\lambda}(1-\tilde{z})) }\\{\hat{S}_{f}^{*}(\hat{\lambda}(1-\tilde{z}))(\tilde{z}+(1-\tilde{z})\hat{A}^{*}(\hat{\lambda}))-\tilde{z}}}\right\rbrace e^{-\hat{\lambda} (1-\tilde{z}) \tilde{x}}(1-\Pi_{b}(\tilde{x})) \end{align} | (2.52) |
\begin{align} \Pi_{b}(\tilde{x},\tilde{z}) = &\frac{\hat{\lambda} \alpha \varUpsilon_{0}\hat{L}_{b}^{*}(\hat{\lambda} (1-\tilde{z}))}{\widehat{W}_{v}^{*}(\hat{\lambda})} \left\lbrace \frac{{(1-\widehat{W}_{v}^{*}(\hat{\lambda} (1-\tilde{z})))(\tilde{z}+(1-\tilde{z})\hat{A}^{*}(\hat{\lambda}))+}\\{\hat{A}^{*}(\hat{\lambda})\widehat{W}_{v}^{*}(\hat{\lambda})(1-\tilde{z})}}{{((\alpha \hat{L}_{b}^{*}(\hat{\lambda}(1-\tilde{z}))\hat{\hat{R}}_{b}^{*}(\hat{\lambda}(1-\tilde{z})))+\bar{\alpha} \tilde{z}\hat{E}_{f}^{*}(\hat{\lambda}(1-\tilde{z}))}\\{ \hat{S}_{f}^{*}(\hat{\lambda}(1-\tilde{z})))(\tilde{z}+(1-\tilde{z})\hat{A}^{*}(\hat{\lambda}))-\tilde{z}}}\right\rbrace e^{-\hat{\lambda} (1-\tilde{z}) \tilde{x}}(1-\hat{\hat{R}}_{b}(\tilde{x})) \end{align} | (2.53) |
\begin{align} \mho_{v}(\tilde{x},\tilde{z}) = &\frac{\hat{\lambda} \varUpsilon_{0}}{\widehat{W}_{v}^{*}(\hat{\lambda})}e^{-\hat{\lambda} (1-\tilde{z}) \tilde{x}}(1-\widehat{W}_{v}(\tilde{x})) \end{align} | (2.54) |
\begin{align} \acute{D}(\tilde{x},\tilde{z})& = \frac{\bar{\alpha} \tilde{z} \hat{\lambda} \varUpsilon_{0}}{\widehat{W}_{v}^{*}(\hat{\lambda})} \left\lbrace \frac{{(1-\widehat{W}_{v}^{*}(\hat{\lambda} (1-\tilde{z})))(\tilde{z}+(1-\tilde{z})\hat{A}^{*}(\hat{\lambda}))+}\\{\hat{A}^{*}(\hat{\lambda})\widehat{W}_{v}^{*}(\hat{\lambda})(1-\tilde{z})}}{{((\alpha \hat{L}_{b}^{*}(\hat{\lambda}(1-\tilde{z}))\hat{\hat{R}}_{b}^{*}(\hat{\lambda}(1-\tilde{z})))+\bar{\alpha} \tilde{z}\hat{E}_{f}^{*}(\hat{\lambda}(1-\tilde{z}))}\\{ \hat{S}_{f}^{*}(\hat{\lambda}(1-\tilde{z})))(\tilde{z}+(1-\tilde{z})\hat{A}^{*}(\hat{\lambda}))-\tilde{z}}}\right\rbrace e^{-\hat{\lambda} (1-\tilde{z}) \tilde{x}}(1-\hat{E}_{f}(\tilde{x})) \end{align} | (2.55) |
\begin{align} \varOmega(\tilde{x},\tilde{z})& = \frac{\hat{\lambda} \bar{\alpha}\tilde{z}\varUpsilon_{0}\hat{E}_{f}^{*}(\hat{\lambda}(1-\tilde{z}))}{\widehat{W}_{v}^{*}(\hat{\lambda})} \left\lbrace \frac{{(1-\widehat{W}_{v}^{*}(\hat{\lambda} (1-\tilde{z})))(\tilde{z}+(1-\tilde{z})\hat{A}^{*}(\hat{\lambda}))+}\\{\hat{A}^{*}(\hat{\lambda})\widehat{W}_{v}^{*}(\hat{\lambda})(1-\tilde{z})}}{{(\alpha \hat{L}_{b}^{*}(\hat{\lambda}(1-\tilde{z}))\hat{\hat{R}}_{b}^{*}(\hat{\lambda}(1-\tilde{z})))+\bar{\alpha} \tilde{z} \hat{E}_{f}^{*}(\hat{\lambda}(1-\tilde{z}))}\\{\hat{S}_{f}^{*}(\hat{\lambda}(1-\tilde{z}))(\tilde{z}+(1-\tilde{z})\hat{A}^{*}(\hat{\lambda}))-\tilde{z}}}\right\rbrace e^{-\hat{\lambda} (1-\tilde{z}) \tilde{x}}(1-\hat{S}_{f}(\tilde{x})). \end{align} | (2.56) |
Focusing on the server states and orbit size marginal generating functions, the required probability generating functions are turned with the help of the following:
\varUpsilon(\tilde{z}) = \int_{0}^{\infty}\varUpsilon(\tilde{x}, \tilde{z})d\tilde{x}; \quad \varPsi_{b} (\tilde{z}) = \int_{0}^{\infty}\varPsi_{b} (\tilde{x}, \tilde{z})d\tilde{x}; \quad \Pi_{b} (\tilde{z}) = \int_{0}^{\infty}\Pi_{b} (\tilde{x}, \tilde{z})d\tilde{x};
\mho_{b} (\tilde{z}) = \int_{0}^{\infty}\mho_{b} (\tilde{x}, \tilde{z})d\tilde{x}; \quad \acute{D}(\tilde{z}) = \int_{0}^{\infty}\acute{D}(\tilde{x}, \tilde{z})d\tilde{x}; \quad \varOmega(\tilde{z}) = \int_{0}^{\infty}\varOmega(\tilde{x}, \tilde{z})d\tilde{x}.
Now, for the above Eqs (2.51) to (2.56) on integrating w.r.t \tilde{x} and limiting with 0 – \infty , the essential results are (2.57) to (2.62).
\begin{align} \varUpsilon(\tilde{z})& = \frac{\tilde{z} \varUpsilon_{0}(1-\hat{A}^{*}(\hat{\lambda}))}{\widehat{W}_{v}^{*}(\hat{\lambda})} \left\lbrace \frac{ {(1-\widehat{W}_{v}^{*}(\hat{\lambda} (1-\tilde{z})))+\widehat{W}_{v}^{*}(\hat{\lambda})(1-\alpha \hat{L}_{b}^{*}(\hat{\lambda}(1-\tilde{z}))\hat{\hat{R}}_{b}^{*}(\hat{\lambda}(1-\tilde{z})))}\\{-\bar{\alpha} \tilde{z} \hat{E}_{f}^{*}(\hat{\lambda}(1-\tilde{z}))\hat{S}_{f}^{*}(\hat{\lambda}(1-\tilde{z}))}} {{(\alpha \hat{L}_{b}^{*}(\hat{\lambda}(1-\tilde{z}))\hat{\hat{R}}_{b}^{*}(\hat{\lambda}(1-\tilde{z})))+\bar{\alpha} \tilde{z} \hat{E}_{f}^{*}(\hat{\lambda}(1-\tilde{z}))\hat{S}_{f}^{*}(\hat{\lambda}(1-\tilde{z})))}\\{(\tilde{z}+(1-\tilde{z})\hat{A}^{*}(\hat{\lambda}))-\tilde{z}}}\right\rbrace \end{align} | (2.57) |
\begin{align} \varPsi_{b}(\tilde{z})& = \frac{ \alpha \varUpsilon_{0}(1-\hat{L}_{b}^{*}(\hat{\lambda} (1-\tilde{z})))}{\widehat{W}_{v}^{*}(\hat{\lambda})(1-\tilde{z})} \left\lbrace \frac{(1-\widehat{W}_{v}^{*}(\hat{\lambda} (1-\tilde{z})))(\tilde{z}+(1-\tilde{z})\hat{A}^{*}(\hat{\lambda}))+\hat{A}^{*}(\hat{\lambda})\widehat{W}_{v}^{*}(\hat{\lambda})(1-\tilde{z})}{{(\alpha \hat{L}_{b}^{*}(\hat{\lambda}(1-\tilde{z}))\hat{\hat{R}}_{b}^{*}(\hat{\lambda}(1-\tilde{z})))+\bar{\alpha} \tilde{z} \hat{E}_{f}^{*}(\hat{\lambda}(1-\tilde{z}))\hat{S}_{f}^{*}(\hat{\lambda}(1-\tilde{z})))}\\{(\tilde{z}+(1-\tilde{z})\hat{A}^{*}(\hat{\lambda}))-\tilde{z}}}\right\rbrace \end{align} | (2.58) |
\begin{align} \Pi_{b}(\tilde{z})& = \frac{{\alpha \varUpsilon_{0}\hat{L}_{b}^{*}(\hat{\lambda} (1-\tilde{z}))}\\{(1-\hat{\hat{R}}_{b}^{*}(\hat{\lambda} (1-\tilde{z})))}}{\widehat{W}_{v}^{*}(\hat{\lambda})(1-\tilde{z})} \left\lbrace \frac{(1-\widehat{W}_{v}^{*}(\hat{\lambda} (1-\tilde{z})))(\tilde{z}+(1-\tilde{z})\hat{A}^{*}(\hat{\lambda}))+\hat{A}^{*}(\hat{\lambda})\widehat{W}_{v}^{*}(\hat{\lambda})(1-\tilde{z})}{{(\alpha \hat{L}_{b}^{*}(\hat{\lambda}(1-\tilde{z}))\hat{\hat{R}}_{b}^{*}(\hat{\lambda}(1-\tilde{z})))+\bar{\alpha} \tilde{z} \hat{E}_{f}^{*}(\hat{\lambda}(1-\tilde{z}))\hat{S}_{f}^{*}(\hat{\lambda}(1-\tilde{z})))}\\{(\tilde{z}+(1-\tilde{z})\hat{A}^{*}(\hat{\lambda}))-\tilde{z}}}\right\rbrace \end{align} | (2.59) |
\begin{align} \mho_{v}(\tilde{z})& = \frac{\varUpsilon_{0}(1-\widehat{W}_{v}^{*}(\hat{\lambda}(1-\tilde{z})))}{\widehat{W}_{v}^{*}(\hat{\lambda}) (1-\tilde{z})} \end{align} | (2.60) |
\begin{align} \acute{D}(\tilde{z})& = \frac{\bar{\alpha}\tilde{z} \varUpsilon_{0}(1-\hat{E}_{b}^{*}(\hat{\lambda} (1-\tilde{z})))}{\widehat{W}_{v}^{*}(\hat{\lambda})(1-\tilde{z})} \left\lbrace \frac{(1-\widehat{W}_{v}^{*}(\hat{\lambda} (1-\tilde{z})))(\tilde{z}+(1-\tilde{z})\hat{A}^{*}(\hat{\lambda}))+\hat{A}^{*}(\hat{\lambda})\widehat{W}_{v}^{*}(\hat{\lambda})(1-\tilde{z})}{{(\alpha \hat{L}_{b}^{*}(\hat{\lambda}(1-\tilde{z}))\hat{\hat{R}}_{b}^{*}(\hat{\lambda}(1-\tilde{z})))+\bar{\alpha} \tilde{z} \hat{E}_{f}^{*}(\hat{\lambda}(1-\tilde{z}))\hat{S}_{f}^{*}(\hat{\lambda}(1-\tilde{z})))}\\{(\tilde{z}+(1-\tilde{z})\hat{A}^{*}(\hat{\lambda}))-\tilde{z}}}\right\rbrace \end{align} | (2.61) |
\begin{align} \varOmega(\tilde{z})& = \frac{{\bar{\alpha}\tilde{z}\varUpsilon_{0}\hat{E}_{f}^{*}(\hat{\lambda}(1-\tilde{z}))}\\{(1-\hat{S}_{f}^{*}(\hat{\lambda}(1-\tilde{z})))}}{\widehat{W}_{v}^{*}(\hat{\lambda})(1-\tilde{z})} \left\lbrace \frac{(1-\widehat{W}_{v}^{*}(\hat{\lambda} (1-\tilde{z})))(\tilde{z}+(1-\tilde{z})A^{*}(\hat{\lambda}))+(1-\tilde{z})\hat{A}^{*}(\hat{\lambda})\widehat{W}_{v}^{*}(\hat{\lambda})}{{(\alpha \hat{L}_{b}^{*}(\hat{\lambda}(1-\tilde{z}))\hat{\hat{R}}_{b}^{*}(\hat{\lambda}(1-\tilde{z})))+\bar{\alpha} \tilde{z} \hat{E}_{f}^{*}(\hat{\lambda}(1-\tilde{z}))\hat{S}_{f}^{*}(\hat{\lambda}(1-\tilde{z})))}\\{(\tilde{z}+(1-\tilde{z})\hat{A}^{*}(\hat{\lambda}))-\tilde{z}}}\right\rbrace. \end{align} | (2.62) |
By using the above equation, the only unknown \varUpsilon_{0} was eliminated as follows, which involves normalising condition and by adjusting \tilde{z} = 1 :
\begin{align} \varUpsilon_{0}+\varUpsilon(1)+\varPsi_{b}(1)+\Pi_{b}(1)+\mho_{v}(1)+\acute{D}(1)+\varOmega(1) = 1 \end{align} | (2.63) |
\begin{align} \varUpsilon_{0} = \frac{\widehat{W}_{v}^{*}(\hat{\lambda})\left\lbrace \hat{A}^{*}(\hat{\lambda})-(\bar{\alpha}+\alpha \hat{\lambda}(E(\hat{L}_{b})+E(\hat{\hat{R}}_{b}))+\bar{\alpha}\hat{\lambda}(E(\hat{E}_{f})+\hat{\lambda} E(\hat{S}_{f}))) \right\rbrace }{\alpha \hat{A}^{*}(\hat{\lambda}) \widehat{W}_{v}^{*}(\hat{\lambda})+\alpha\hat{\lambda} E(\widehat{W}_{v})}. \end{align} | (2.64) |
Theorem 3. In order for the model to satisfy the stability criteria, \bar{\alpha}+\alpha \hat{\lambda} (E(\hat{L}_{b})+E(\hat{\hat{R}}_{b}))+\bar{\alpha}\hat{\lambda}(E(\hat{E}_{f})+\hat{\lambda} E(\hat{S}_{f})) < \hat{A}^{*}(\hat{\lambda}) , If the server was idle, regularly busy on both the phase, lower rate service, delay and on repair, then the probability of the stationary distribution customers number in the designed system and in the orbit was determined by K(\tilde{z}) = \varUpsilon_{0}+ \varUpsilon(\tilde{z})+\tilde{z}\varPsi_{b} (\tilde{z})+\tilde{z}\Pi_{b} (\tilde{z})+\tilde{z}\mho_{v} (\tilde{z})+\acute{D}(\tilde{z})+\varOmega(\tilde{z}) and \varTheta(\tilde{z}) = \varUpsilon_{0}+ \varUpsilon(\tilde{z})+\varPsi_{b} (\tilde{z})+\Pi_{b} (\tilde{z})+\mho_{v} (\tilde{z})+\acute{D}(\tilde{z})+\varOmega(\tilde{z}).
Proof. Now, by substituting the equations from (2.57) to (2.62) in \varTheta(\tilde{z}) = \varUpsilon_{0}+\varUpsilon(\tilde{z})+\varPsi_{b} (\tilde{z})+\Pi_{b} (\tilde{z})+\mho_{v} (\tilde{z})+\acute{D}(\tilde{z})+\varOmega(\tilde{z}) and in K(\tilde{z}) = \varUpsilon_{0}+ \varUpsilon(\tilde{z})+\tilde{z}\varPsi_{b} (\tilde{z})+\tilde{z}\Pi_{b} (\tilde{z})+\tilde{z}\mho_{v} (\tilde{z})+\acute{D}(\tilde{z})+\varOmega(\tilde{z}) we find the PGF of the number of customers in the orbit and system finally the results are given by the following:
\begin{align} \varTheta(\tilde{z}) = &\varUpsilon_{0}\left\lbrace\frac{(\alpha(1-\widehat{W}_{v}^{*}(\hat{\lambda}))(\tilde{z}+(1-\tilde{z})\hat{A}^{*}(\hat{\lambda}))+\alpha \widehat{W}_{v}^{*}(\hat{\lambda}) \hat{A}^{*}(\hat{\lambda})(1-\tilde{z}))}{{\widehat{W}_{v}^{*}(\hat{\lambda})((\alpha \hat{L}_{b}^{*}(\hat{\lambda}(1-\tilde{z}))\hat{\hat{R}}_{b}^{*}(\hat{\lambda}(1-\tilde{z})))+\bar{\alpha} \tilde{z} \hat{E}_{f}^{*}(\hat{\lambda}(1-\tilde{z}))\hat{S}_{f}^{*}(\hat{\lambda}(1-\tilde{z})))}\\{(\tilde{z}+(1-\tilde{z})\hat{A}^{*}(\hat{\lambda}))-\tilde{z}}}\right\rbrace \end{align} | (2.65) |
\begin{align} K(\tilde{z}) = &\varUpsilon_{0}\left\lbrace\frac{{\tilde{z}(1-\widehat{W}_{v}^{*}(\hat{\lambda}(1-\tilde{z})))+\alpha(1-\tilde{z})\widehat{W}_{v}^{*}(\hat{\lambda})\hat{A}^{*}(\hat{\lambda})\hat{L}_{b}^{*}(\hat{\lambda}(1-\tilde{z}))\hat{\hat{R}}_{b}^{*}(\hat{\lambda}(1-\tilde{z}))}\\{-\bar{\alpha}\tilde{z}(1-\widehat{W}_{v}^{*}(\hat{\lambda}(1-\tilde{z})))(\tilde{z}+(1-\tilde{z})\hat{A}^{*}(\hat{\lambda}))\hat{E}_{f}^{*}(\hat{\lambda}(1-\tilde{z}))\hat{S}_{f}^{*}(\hat{\lambda}(1-\tilde{z}))}} {{\widehat{W}_{v}^{*}(\hat{\lambda})((\alpha \hat{L}_{b}^{*}(\hat{\lambda}(1-\tilde{z}))\hat{\hat{R}}_{b}^{*}(\hat{\lambda}(1-\tilde{z})))+\bar{\alpha} \tilde{z} \hat{E}_{f}^{*}(\hat{\lambda}(1-\tilde{z}))\hat{S}_{f}^{*}(\hat{\lambda}(1-\tilde{z})))}\\{(\tilde{z}+(1-\tilde{z})\hat{A}^{*}(\hat{\lambda}))-\tilde{z}}}\right\rbrace \end{align} | (2.66) |
where \varUpsilon_{0} is given by Eq (2.64).
The mean system size and the mean orbit size:
Under the steady state condition of the system, the mean and orbit size was given as follows:
(1) The mean number of customers in the orbit (\hat{L}_{q}) was obtained by differentiating Eq (2.65) with respect to \tilde{z} , along by evaluating at \tilde{z} = 1 , we get \hat{L}_{q} :
\begin{align*} \hat{L}_{q} = \lim\limits_{\tilde{z} = 1}\left\lbrace\frac{d}{d\tilde{z}}\varTheta(\tilde{z})\right\rbrace \end{align*} |
\begin{align*} \hat{L}_{q} = &\frac{2\alpha\hat{\lambda} E(\widehat{W}_{v}) (1-\hat{A}^{*}(\hat{\lambda})) +\alpha \hat{\lambda}^{2} E(\widehat{W}_{v}^{2})} {2(\alpha\hat{\lambda} E(\widehat{W}_{v})+ \alpha \hat{A}^{*}(\hat{\lambda})\widehat{W}_{v}^{*}(\hat{\lambda}))} \\ &+\frac{{\alpha\hat{\lambda}^{2} (E(\hat{L}_{b}^{2})+2 E(\hat{L}_{b})E(\hat{\hat{R}}_{b})+ E(\hat{\hat{R}}_{b}^{2})) +\bar{\alpha}\hat{\lambda}^{2} (E(\hat{S}_{f}^{2})+E(E_{f}^{2}))}\\{+2\alpha \hat{\lambda} (E(\hat{L}_{b})+E(\hat{\hat{R}}_{b}))(1-\hat{A}^{*}(\hat{\lambda}))}} {2(\hat{A}^{*}(\hat{\lambda})-\alpha\hat{\lambda} (E(\hat{L}_{b})+E(\hat{\hat{R}}_{b})) +\bar{\alpha}(1+\hat{\lambda}( E(\hat{S}_{f})+E(\hat{E}_{f})))} \\ \\ &+\frac{{2\bar{\alpha} \hat{\lambda} ( E(\hat{S}_{f})+E(\hat{E}_{f})) + 2\bar{\alpha} (1- \hat{A}^{*}(\hat{\lambda})) + 2\bar{\alpha} \hat{\lambda} (E(\hat{S}_{f})+E(\hat{E}_{f})) (1- \hat{A}^{*}(\hat{\lambda}))}\\{+2\bar{\alpha} \hat{\lambda}^{2} ( E(\hat{S}_{f})E(\hat{E}_{f}))} } { 2(\hat{A}^{*}(\hat{\lambda})-\alpha\hat{\lambda} (E(\hat{L}_{b})+E(\hat{\hat{R}}_{b})) +\bar{\alpha}(1+\hat{\lambda} ( E(\hat{S}_{f})+E(\hat{E}_{f})))) }. \end{align*} |
(2) The mean number of customers in the system \hat{L}_{s} is given by differentiating Eq (2.66) with respect to \tilde{z} , and then by evaluating \tilde{z} = 1 , we get \hat{L}_{s} :
\begin{align*} \hat{L}_{s}& = \lim\limits_{\tilde{z} = 1}\left\lbrace\frac{d}{d\tilde{z}}K(\tilde{z})\right\rbrace \end{align*} |
\begin{align*} \hat{L}_{s} = &\frac{2\alpha\hat{\lambda} \hat{A}^{*}(\hat{\lambda}) \widehat{W}_{v}^{*}(\hat{\lambda}) (E(\hat{L}_{b})+E(\hat{\hat{R}}_{b})) +2\hat{\lambda} E(\widehat{W}_{v}) + \hat{\lambda}^{2} E(\widehat{W}_{v}^{2})} {2(\alpha \hat{\lambda} E(\widehat{W}_{v})+ \alpha \hat{A}^{*}(\hat{\lambda})\widehat{W}_{v}^{*}(\hat{\lambda}))} \\& - \frac {{ 2 (\bar{\alpha}\hat{\lambda} E(\widehat{W}_{v})+ \bar{\alpha} \hat{\lambda}^{2} E(\widehat{W}_{v}) ( E(\hat{S}_{f})+E(\hat{E}_{f})) + \bar{\alpha} \hat{\lambda} E(\widehat{W}_{v}) }\\{+ \bar{\alpha} \hat{\lambda} E(\widehat{W}_{v}) (1-\hat{A}^{*}(\hat{\lambda})))+\bar{\alpha} \hat{\lambda}^{2} E(\widehat{W}_{v}^{2}) } } {2(\alpha\hat{\lambda} E(\widehat{W}_{v})+ \alpha \hat{A}^{*}(\hat{\lambda})\widehat{W}_{v}^{*}(\hat{\lambda}))} \\& + \frac {{\alpha \hat{\lambda}^{2} (E(\hat{L}_{b}^{2})+2( E(\hat{L}_{b})E(\hat{\hat{R}}_{b})) +E(\hat{\hat{R}}_{b}^{2})) + \bar{\alpha} \hat{\lambda}^{2}( E(\hat{S}_{f}^{2}) }\\{+2( E(\hat{S}_{f})E(\hat{E}_{f}))+E(\hat{E}_{f}^{2}))+ 2\alpha\hat{\lambda} (E(L_{b})+E(\hat{\hat{R}}_{b})) (1-\hat{A}^{*}(\hat{\lambda}))}} {2(\hat{A}^{*}(\hat{\lambda})-\alpha\hat{\lambda} (E(\hat{L}_{b})+E(\hat{\hat{R}}_{b})) -\bar{\alpha}(1+\hat{\lambda} ( E(\hat{S}_{f})+E(\hat{E}_{f}))))} \\& +\frac { 2 ( \bar{\alpha} \hat{\lambda} (E(\hat{S}_{f})+E(\hat{E}_{f})) + \bar{\alpha} (1- \hat{A}^{*}(\hat{\lambda})) + \bar{\alpha} \hat{\lambda} (E(\hat{S}_{f})+E(\hat{E}_{f})) (1- \hat{A}^{*}(\hat{\lambda})))} {2 (A^{*}(\hat{\lambda})-\alpha\hat{\lambda} (E(\hat{L}_{b})+E(\hat{\hat{R}}_{b})) -\bar{\alpha}(1+\hat{\lambda} (E(\hat{S}_{f})+E(\hat{E}_{f})))))}. \end{align*} |
Some of the most important performance measures of this M/G/1 retrial queueing system are derived in this section, which was subjected to the two-phase essential service with starting failure along with working vacation under steady state; additionally, we derived the reliability analysis, mean busy period and mean cycle and also orbit size distribution during the departure epoch of this proposed model.
System state probabilities:
(1) The probability that the server is idle under steady state at the time of retrial was given by the following:
\begin{align} \varUpsilon(1) = \frac{\varUpsilon_{0}(1-\hat{A}^{*}(\hat{\lambda}))}{\widehat{W}_{v}^{*}(\hat{\lambda})}\left\lbrace \frac{\hat{\lambda} E(\widehat{W}_{v})+\widehat{W}_{v}^{*}(\hat{\lambda})(\bar{\alpha}+\bar{\alpha}\hat{\lambda} (E(\hat{S}_{f})+E(\hat{E}_{f}))+\alpha \hat{\lambda} (E(\hat{L}_{b})+E(\hat{\hat{R}}_{b})))}{A^{*}(\hat{\lambda})-(\bar{\alpha}+\alpha \hat{\lambda} (E(\hat{L}_{b})+E(\hat{\hat{R}}_{b}))+\bar{\alpha}\hat{\lambda}( E(\hat{S}_{f})+E(\hat{E}_{f}) )}\right\rbrace. \end{align} | (2.67) |
(2) The probability of the server to be busy on normal first phase service under the steady state was given by the following:
\begin{align} \varPsi_{b}(1) = \frac{\varUpsilon_{0}\alpha \hat{\lambda} E(\hat{L}_{b})}{\widehat{W}_{v}^{*}(\hat{\lambda})}\left\lbrace\frac{\hat{\lambda} E(\widehat{W}_{v})+\hat{A}^{*}(\hat{\lambda})\widehat{W}_{v}^{*}(\hat{\lambda})} {\hat{A}^{*}(\hat{\lambda})-(\bar{\alpha}+\alpha \hat{\lambda} (E(\hat{L}_{b})+E(\hat{\hat{R}}_{b}))+\bar{\alpha}\hat{\lambda}( E(\hat{S}_{f})+E(\hat{E}_{f}) )}\right\rbrace. \end{align} | (2.68) |
(3) The probability of the server to be busy on normal second phase service under the steady state was given by the following:
\begin{align} \Pi_{b}(1) = \frac{\varUpsilon_{0}\alpha \hat{\lambda} E(\hat{\hat{R}}_{b})}{\widehat{W}_{v}^{*}(\hat{\lambda})}\left\lbrace\frac{\hat{\lambda} E(\widehat{W}_{v})+\hat{A}^{*}(\hat{\lambda})\widehat{W}_{v}^{*}(\hat{\lambda})}{\hat{A}^{*}(\hat{\lambda})-(\bar{\alpha}+\alpha \hat{\lambda} (E(\hat{L}_{b})+E(\hat{\hat{R}}_{b}))+\bar{\alpha}\hat{\lambda}( E(\hat{S}_{f})+E(\hat{E}_{f}) )}\right\rbrace. \end{align} | (2.69) |
(4) The probability under steady state of the server to be busy during working vacation period was given by the following:
\begin{align} \mho_{v}(1) = \frac{\varUpsilon_{0} \hat{\lambda} E(\widehat{W}_{v})}{\widehat{W}_{v}^{*}(\hat{\lambda})}. \end{align} | (2.70) |
(5) The probability under steady state of the server is delayed to repair at the time of normal busy period was given by the following:
\begin{align} \acute{D}(1) = \frac{\varUpsilon_{0}\bar{\alpha}\hat{\lambda} E(\hat{E}_{f})}{\widehat{W}_{v}^{*}(\hat{\lambda})}\left\lbrace\frac{\hat{\lambda} E(\widehat{W}_{v})+\hat{A}^{*}(\hat{\lambda})\widehat{W}_{v}^{*}(\hat{\lambda})}{\hat{A}^{*}(\hat{\lambda})-(\bar{\alpha}+\alpha \hat{\lambda} (E(\hat{L}_{b})+E(\hat{\hat{R}}_{b}))+\bar{\alpha}\hat{\lambda}( E(\hat{S}_{f})+E(\hat{E}_{f}) )}\right\rbrace. \end{align} | (2.71) |
(6) The probability under steady state of the server is under repair at the time of normal busy period was given by the following:
\begin{align} \varOmega(1) = \frac{\varUpsilon_{0}\bar{\alpha}\hat{\lambda} E(\hat{S}_{f})}{\widehat{W}_{v}^{*}(\hat{\lambda})}\left\lbrace\frac{\hat{\lambda} E(\widehat{W}_{v})+\hat{A}^{*}(\hat{\lambda})\widehat{W}_{v}^{*}(\hat{\lambda})}{\hat{A}^{*}(\hat{\lambda})-(\bar{\alpha}+\alpha \hat{\lambda} (E(\hat{L}_{b})+E(\hat{\hat{R}}_{b}))+\bar{\alpha}\hat{\lambda}( E(S_{f})+E(\hat{E}_{f}) )}\right\rbrace. \end{align} | (2.72) |
(7) The expected time the customer spends in system (\widehat{W}_{s}) and in orbit (\widehat{W}_{q}) under the condition of steady state, taken for the Little's formula is as follows:
\begin{align} \widehat{W}_{s} = \frac{\hat{L}_{s}}{\hat{\lambda}}. \end{align} | (2.73) |
\begin{align} \widehat{W}_{q} = \frac{\hat{L}_{q}}{\hat{\lambda}}. \end{align} | (2.74) |
When a server in the trial queueing system is unreliable, reliability measures will give the data required for the system's improvement. The following availability indicators are gathered in order to validate and corroborate the model's analytical findings. Unreliable servers in queuing systems require measures of dependability to offer adequate data, which are necessary for system improvement. The failure frequency and availability measures are obtained to explain the validity of the logical findings of the framed model.
(1) The probability of the availability of the server under the steady state was occupied by either a positive customer or by an idle period in which the steady state accessibility of the server was considered as \wedge_v = 1-\lim_{\tilde{z}\to1}\varOmega(\tilde{\tilde{z}}) = 1-\varOmega(1) ,
\begin{align*} \wedge_v = &1-\frac{\varUpsilon_{0}\bar{\alpha}\hat{\lambda} E(\hat{S}_{f})}{\widehat{W}_{v}^{*}(\hat{\lambda})}\left\lbrace \frac{\hat{\lambda} E(\widehat{W}_{v})+\hat{ A}^{*}(\hat{\lambda})\widehat{W}_{v}^{*}(\hat{\lambda})}{\hat{A}^{*}(\hat{\lambda})-\left( \bar{\alpha}+\alpha \hat{\lambda} (E(\hat{L}_{b})+E(\hat{\hat{R}}_{b}))+\bar{\alpha}\hat{\lambda}(E(\hat{E}_{f})+ E(\hat{S}_{f}))\right) }\right\rbrace. \end{align*} |
The failure frequency during the steady state is gained as F_{\Gamma} = \bar{\alpha} \varPsi(1) ,
\begin{align*} F_{\Gamma} = &\bar{\alpha}\left\lbrace\frac{\alpha \varUpsilon_{0}(\hat{\lambda})E(\hat{L}_{b})(\hat{\lambda} E(\widehat{W}_{v})+\hat{A}^{*}(\hat{\lambda})\widehat{W}_{v}^{*}(\hat{\lambda}))}{\widehat{W}_{v}^{*}(\hat{\lambda})(\hat{A}^{*}(\hat{\lambda})-\alpha \hat{\lambda} (E(\hat{L}_{b})+E(\hat{\hat{R}}_{b}))-\bar{\alpha}(1+\hat{\lambda} E(\hat{S}_{f})))} \right\rbrace . \end{align*} |
Let's assume that, under steady state conditions, E(T_{b}) and E(T_{C}) represent the anticipated lengths of the busy period and busy cycle, respectively. The following conclusions are directly obtained by using the argument of an alternating renewal process, which results in the following:
\begin{align*} \varUpsilon_{0} = \frac{ E(T_{0})} { E(T_{b}) +E(T_{0}) }; \quad E(T_{b}) = \frac{1}{\hat{\lambda}} \left\lbrace\frac{1}{\varUpsilon_{0}} -1\right\rbrace;\quad E(T_{c}) = \frac{1}{\hat{\lambda} \varUpsilon_{0}} = E(T_{b}) +E(T_{0}); \end{align*} |
where T_{0} is the amount of time the system has spent in an empty state. Due to the fact that the interval between two customers' arrival times is exponentially distributed along the parameter \hat{\lambda} , then E(T_{0}) = \frac{1}{\hat{\lambda}} . Using the aforementioned necessary outcomes, we obtain the following anticipated steady state probability:
\begin{align*} E(T_{b}) = & \frac{1} {\hat{\lambda}} \left\lbrace \frac{ {\alpha \hat{\lambda} (E(\widehat{W}_{v}) + \widehat{W}_{v}^{*}(\hat{\lambda}) (E(\hat{L}_{b})+E(\hat{\hat{R}}_{b}))) +\bar{\alpha} \widehat{W}_{v}^{*}(\hat{\lambda}) \left(1+\hat{\lambda} ( E(\hat{S}_{f})+E(\hat{E}_{f}))\right)}\\{-\hat{A}^{*}(\hat{\lambda})\widehat{W}_{v}^{*}(\hat{\lambda})(1-\alpha)} } {\widehat{W}_{v}^{*}(\hat{\lambda}) \left( \hat{A}^{*}(\hat{\lambda}) - \alpha \hat{\lambda} (E(\hat{L}_{b})+E(\hat{\hat{R}}_{b})) -\bar{\alpha}(1+ \hat{\lambda} E(\hat{S}_{f}))\right) }\right\rbrace \\ E(T_{c}) = & \frac{1} {\hat{\lambda} \varUpsilon_{0}} = \frac{1}{\hat{\lambda}} \left\lbrace \frac{ \alpha \hat{A}^{*}(\hat{\lambda}) \widehat{W}_{v}^{*}(\hat{\lambda}) +\alpha \hat{\lambda} E(\widehat{W}_{v}) } {\widehat{W}_{v}^{*}(\hat{\lambda}) \left( \hat{A}^{*}(\hat{\lambda}) - \alpha \hat{\lambda} (E(\hat{L}_{b})+E(\hat{\hat{R}}_{b})) -\bar{\alpha}(1+ \hat{\lambda} (E(\hat{E}_{f})+E(\hat{S}_{f})))\right) }\right\rbrace . \end{align*} |
PASTA's (Poisson arrival see time average) argument states that the orbit must have n customers immediately following a disappearance if and only if the system contained n+1 customers prior to the disappearance. Assume that if you specify the likelihood that there are n customers in the orbit at the time of departure {\hat{L}_{n}^{+}; n\geq0}, n , then there will be n customers, with the normalizing constant K_{0} :
\begin{align} \hat{L}_{n}^{+} = K_{0} \int_{0}^{\infty}\mho_{n,v}(\tilde{x})\mu_{v}(\tilde{x})d\tilde{x} + K_{0} \int_{0}^{\infty}\Pi_{n,b}(\tilde{x})\mu_{sb}(\tilde{x})d\tilde{x} + K_{0} \int_{0}^{\infty}\varOmega_{n}(\tilde{x})v(\tilde{x})d\tilde{x};\quad n\geq1. \end{align} | (2.75) |
Corollary 1. The quantity of customers in orbit during the departure epoch whose probability generating function was estimated by the following:
\begin{align} \hat{L}_{n}^{+} = K_{0} \int_{0}^{\infty}\mho_{n,v}(\tilde{x})\mu_{v}(\tilde{x})d\tilde{x} + K_{0} \int_{0}^{\infty}\Pi_{n,b}(\tilde{x})\mu_{sb}(\tilde{x})d\tilde{x} + K_{0} \int_{0}^{\infty}\varOmega_{n}(\tilde{x})v(\tilde{x})d\tilde{x};\quad n\geq1 \end{align} | (2.76) |
\begin{align*} \label{77} \hat{L}_{D}(\tilde{z}) = \frac{K_{0}\hat{\lambda} P_{0}} {\widehat{W}_{v}^{*}(\hat{\lambda})} \left\lbrace \frac{\widehat{W}_{v}^{*}(\hat{\lambda}(1-\tilde{z}))\left({(\alpha \hat{L}_{b}^{*}(\hat{\lambda}(1-\tilde{z}))\hat{\hat{R}}_{b}^{*}(\hat{\lambda}(1-\tilde{z})))+\bar{\alpha} \tilde{z} E_{f}^{*}(\hat{\lambda}(1-\tilde{z})) }\\{\hat{S}_{f}^{*}(\hat{\lambda}(1-\tilde{z}))(\tilde{z}+(1-\tilde{z})\hat{A}^{*}(\hat{\lambda}))-\tilde{z}} \right) }{{(\alpha \hat{L}_{b}^{*}(\hat{\lambda}(1-\tilde{z}))\hat{\hat{R}}_{b}^{*}(\hat{\lambda}(1-\tilde{z})))+\bar{\alpha} \tilde{z} E_{f}^{*}(\hat{\lambda}(1-\tilde{z}))\hat{S}_{f}^{*}(\hat{\lambda}(1-\tilde{z})) }\\{(\tilde{z}+(1-\tilde{z})\hat{A}^{*}(\hat{\lambda}))-\tilde{z}}}\right\rbrace \end{align*} |
\begin{align} +\frac{K_{0}\hat{\lambda} P_{0}} {\widehat{W}_{v}^{*}(\hat{\lambda})} \left\lbrace \frac{{\alpha \hat{L}_{b}^{*}(\hat{\lambda}(1-\tilde{z}))\hat{\hat{R}}_{b}^{*}(\hat{\lambda}(1-\tilde{z})) (1-\widehat{W}_{v}^{*}(\hat{\lambda} (1-\tilde{z})))(\tilde{z}+(1-\tilde{z})\hat{A}^{*}(\hat{\lambda}))+}\\{\hat{A}^{*}(\hat{\lambda})\widehat{W}_{v}^{*}(\hat{\lambda})(1-\tilde{z})}} {{(\alpha \hat{L}_{b}^{*}(\hat{\lambda}(1-\tilde{z}))\hat{\hat{R}}_{b}^{*}(\hat{\lambda}(1-\tilde{z})))+\bar{\alpha} \tilde{z} E_{f}^{*}(\hat{\lambda}(1-\tilde{z}))\hat{S}_{f}^{*}(\hat{\lambda}(1-\tilde{z})) }\\{(\tilde{z}+(1-\tilde{z})\hat{A}^{*}(\hat{\lambda}))-\tilde{z}}}\right\rbrace. \end{align} | (2.77) |
Proof. Eq (2.75) was multiplied with appropriate powers for \tilde{z} ; taking summation as n = 0, 1, 2, 3, ..., the following equation was obtained:
\begin{align*} \hat{L}_{D}(\tilde{z}) = K_{0} \int_{0}^{\infty}\mho_{v}(\tilde{x},\tilde{z})\mu_{v}(\tilde{x})d\tilde{x} + K_{0} \int_{0}^{\infty}\Pi_{b}(\tilde{x},\tilde{z})\mu_{sb}(\tilde{x})d\tilde{x} + K_{0} \int_{0}^{\infty}\varOmega(\tilde{x},\tilde{z})v(\tilde{x})d\tilde{x};\quad n\geq1. \end{align*} |
Using Eqs (2.54) to (2.57) and (2.60) to (2.63) during the departure epoch number of customer in the orbit whose generating function was generated. By letting limit \tilde{z} \rightarrow 1 in (2.76), the unknown constant K_{0} was determined as follows:
\begin{align*} K_{0} = \frac{ \alpha \hat{A}^{*}(\hat{\lambda}) \widehat{W}_{v}^{*}(\hat{\lambda}) +\alpha \hat{\lambda} E(\widehat{W}_{v}) }{ \hat{\lambda} ( \hat{A}^{*}(\hat{\lambda})-\alpha\hat{\lambda} (E(\hat{L}_{b})+E(\hat{\hat{R}}_{b})) -\bar{\alpha}(1+\hat{\lambda} E(\hat{S}_{f})) + (\hat{\lambda} E(\widehat{W}_{v}) + \hat{A}^{*}(\hat{\lambda}) \widehat{W}_{v}^{*}(\hat{\lambda}) ) )}. \end{align*} |
Server vacations are included in the stochastic decomposition of unreliable retrial queueing types, which have been widely recognized. An important finding from these analyses is that, in this system, the number of customers at any one time is distributed among the sum of two independent random variables, one is the number of customers in a comparable regular queueing system at a random point in time. The second random variable is consumed by several probabilistic explanations, and the particular situations depend on the intended vacation. It has been found that several of the unreliable retrial queues exhibit stochastic decomposition. Let \chi(\tilde{z}) denote the generating function for the number of customers in both two phases or on vacation in the queueing model at random intervals of time under steady state, \phi(\tilde{z}) denote the generating function for the number of customers in the orbit during the idle, or under repair of the server at a random point of time and Z_{s} (\tilde{z}) be the generating function for the number of customers of the given system, which is to be decomposed. The stochastic decomposition law was then expressed as Z_{s} (\tilde{z}) = \phi(\tilde{z})\times \chi(\tilde{z}) . Now, the law of decomposition had been established, it was applied to the retrial model and analyses were performed. The decomposition for a two-phase queueing system was provided by the following:
\begin{align} Z_{s} (\tilde{z}) = &\chi(\tilde{z})\times\phi(\tilde{z}) \end{align} | (2.78) |
\begin{align} \phi(\tilde{z}) = &\frac{\varUpsilon_{0}+\varUpsilon(\tilde{z})+\acute{D}(\tilde{z})+\varOmega(\tilde{z})}{\varUpsilon_{0}+\varUpsilon(1)+\acute{D}(1)+\varOmega(1)} \end{align} | (2.79) |
\begin{align} \chi(\tilde{z}) = &\frac{\varPsi_{b}(\tilde{z})+\Pi_{b}(\tilde{z})+\mho_{v}(\tilde{z})} {\varPsi_{b}(1)+\Pi_{b}(1)+\mho_{v}(1)} . \end{align} | (2.80) |
Substituting the Eqs (2.64), (2.57), (2.61), (2.62) also (2.67), (2.71), (2.72) in (2.79), we obtain the following:
\begin{align*} \phi(\tilde{z}) = \left\lbrace \frac{{\widehat{W}_{v}^{*}(\hat{\lambda})(1-\tilde{z})\left(\alpha L_{b}^{*}(\hat{\lambda}(1-z))R_{b}^{*}(\hat{\lambda}(1-\tilde{z}))+\bar{\alpha}\tilde{z}E_{f}^{*}(\hat{\lambda}(1-\tilde{z}))S_{f}^{*}(\hat{\lambda}(1-\tilde{z})) \right)}\\{-\bar{\alpha}\tilde{z}(1-\widehat{W}_{v}^{*}(\hat{\lambda} (1-\tilde{z})))E_{f}^{*}(\hat{\lambda}(1-\tilde{z}))S_{f}^{*}(\hat{\lambda}(1-\tilde{z}))(\tilde{z}+(1-\tilde{z})\hat{A}^{*}(\hat{\lambda}))}}{{\widehat{W}_{v}^{*}(\hat{\lambda})(1-\tilde{z})\left( (\alpha L_{b}^{*}(\hat{\lambda}(1-\tilde{z}))R_{b}^{*}(\hat{\lambda}(1-\tilde{z}))+\bar{\alpha}\tilde{z}E_{f}^{*}(\hat{\lambda}(1-\tilde{z}))S_{f}^{*}(\hat{\lambda}(1-\tilde{z})))\right)}\\{ (\tilde{z}+(1-\tilde{z})\hat{A}^{*}(\hat{\lambda}))-\tilde{z}}}\right\rbrace \end{align*} |
\begin{align*} +\left\lbrace \frac{{-\tilde{z}(1-\tilde{z})\hat{A}^{*}(\hat{\lambda})\widehat{W}_{v}^{*}(\hat{\lambda})\left( \alpha+\bar{\alpha}E_{f}^{*}(\hat{\lambda}(1-\tilde{z}))S_{f}^{*}(\hat{\lambda}(1-\tilde{z}))\right)}\\{-\alpha \tilde{z}(1-\widehat{W}_{v}^{*}(\hat{\lambda} (1-\tilde{z})))(\tilde{z}+(1-\tilde{z})\hat{A}^{*}(\hat{\lambda}))+\tilde{z}(1-\widehat{W}_{v}^{*}(\hat{\lambda} (1-\tilde{z})))} } {{\widehat{W}_{v}^{*}(\hat{\lambda})(1-\tilde{z})\left( (\alpha L_{b}^{*}(\hat{\lambda}(1-\tilde{z}))R_{b}^{*}(\hat{\lambda}(1-\tilde{z}))+\bar{\alpha}\tilde{z}E_{f}^{*}(\hat{\lambda}(1-\tilde{z}))S_{f}^{*}(\hat{\lambda}(1-\tilde{z})))\right)}\\{ (\tilde{z}+(1-\tilde{z})\hat{A}^{*}(\hat{\lambda}))-\tilde{z}}}\right\rbrace \end{align*} |
\begin{align*} \times\left\lbrace \frac{\widehat{W}_{v}^{*}(\hat{\lambda})\left\lbrace \hat{A}^{*}(\hat{\lambda})-\left( \bar{\alpha}+\alpha\hat{\lambda} ( E(\hat{L}_{b})+E(\hat{\hat{R}}_{b})) \right)+\bar{\alpha}\hat{\lambda} \left( E(\hat{S}_{f})+E(\hat{E}_{f})\right)\right\rbrace } {{\hat{\lambda} E(\widehat{W}_{v})(1- \hat{A}^{*}(\hat{\lambda}))-\alpha\hat{\lambda}\widehat{W}_{v}^{*}(\hat{\lambda})\hat{A}^{*}(\hat{\lambda})( E(\hat{L}_{b})+E(\hat{\hat{R}}_{b}))+\bar{\alpha}\hat{\lambda}^{2} E(\widehat{W}_{v})( E(\hat{S}_{f})+E(\hat{E}_{f}))}\\{+\alpha\widehat{W}_{v}^{*}(\hat{\lambda}) \hat{A}^{*}(\hat{\lambda})}}\right\rbrace. \end{align*} |
Substituting the Eqs (2.58), (2.59), (2.60) also (2.68), (2.69), (2.70) in (2.80), we obtain the following:
\begin{align*} \chi(\tilde{z}) = \left\lbrace \frac{{\left(1-\widehat{W}_{v}^{*}(\hat{\lambda} (1-\tilde{z})) \right) (\tilde{z}+(1-\tilde{z})\hat{A}^{*}(\hat{\lambda}))(\alpha+\bar{\alpha}\tilde{z}E_{f}^{*}(\hat{\lambda}(1-\tilde{z}))S_{f}^{*}(\hat{\lambda}(1-\tilde{z})))+ }\\{\alpha \widehat{W}_{v}^{*}(\hat{\lambda})\hat{A}^{*}(\hat{\lambda})(1-\tilde{z})(1-L_{b}^{*}(\hat{\lambda}(1-\tilde{z}))R_{b}^{*}(\hat{\lambda}(1-\tilde{z})))-\tilde{z}(1-\widehat{W}_{v}^{*}(\hat{\lambda}(1-\tilde{z})))} } {{\widehat{W}_{v}^{*}(\hat{\lambda})(1-\tilde{z})\left( (\alpha L_{b}^{*}(\hat{\lambda}(1-\tilde{z}))R_{b}^{*}(\hat{\lambda}(1-\tilde{z}))+\bar{\alpha}\tilde{z}E_{f}^{*}(\hat{\lambda}(1-\tilde{z}))S_{f}^{*}(\hat{\lambda}(1-\tilde{z})))\right)}\\{ (\tilde{z}+(1-\tilde{z})\hat{A}^{*}(\hat{\lambda}))-\tilde{z}} }\right\rbrace \end{align*} |
\begin{align*} \times \left\lbrace\frac{\widehat{W}_{v}^{*}(\hat{\lambda})\left\lbrace \hat{A}^{*}(\hat{\lambda})-\left( \bar{\alpha}+\alpha\hat{\lambda}( E(\hat{L}_{b})+E(\hat{\hat{R}}_{b}))\right) +\bar{\alpha}\hat{\lambda} \left( E(\hat{S}_{f})+E(\hat{E}_{f})\right)\right\rbrace } {\alpha\hat{\lambda}\hat{A}^{*}(\hat{\lambda})\widehat{W}_{v}^{*}(\hat{\lambda})\left( E(\hat{L}_{b})+E(\hat{\hat{R}}_{b})\right) +\hat{\lambda} E(\widehat{W}_{v}^{*}(\hat{\lambda})(1-\tilde{z})){\hat{A}^{*}(\hat{\lambda})-\bar{\alpha}\left(1+\hat{\lambda}(E(\hat{S}_{f})+E(\hat{E}_{f})) \right) }}\right\rbrace. \end{align*} |
Special cases
Some of the exceptional instances of our concept are examined in this section.
Case 1: If E(\hat{\hat{R}}_{b}) = 0 and E(\hat{E}_{f}) = 0 , our model is reduced to a single server retrial queue with a single phase service along with a working vacation and starting failure. In this case, the results are in good agreement with Gowsalya and Arivudainambi [36]. When the probability generating function of the number of jobs in the system is K(\tilde{z}) , the idle probability P_{0} , the probability generating function of the number of jobs in the orbit is H(\tilde{z}) , and the mean system size is L_{s} , and the mean orbit size is L_{q} :
\begin{align*} \varUpsilon_{0} = \frac{\widehat{W}_{v}^{*}(\hat{\lambda})\left\lbrace \hat{A}^{*}(\hat{\lambda})-(\bar{\alpha}+\alpha \hat{\lambda}(E(\hat{L}_{b}))+\bar{\alpha}\hat{\lambda}(\hat{\lambda} E(\hat{S}_{f}))) \right\rbrace }{\alpha \hat{A}^{*}(\hat{\lambda}) \widehat{W}_{v}^{*}(\hat{\lambda})+\alpha\hat{\lambda} E(\widehat{W}_{v})} \end{align*} |
\begin{align*} L_{q} = &\frac{2\alpha\hat{\lambda} E(\widehat{W}_{v}) (1-\hat{A}^{*}(\hat{\lambda})) +\alpha \hat{\lambda}^{2} E(\widehat{W}_{v}^{2})} {2(\alpha\hat{\lambda} E(\widehat{W}_{v})+ \alpha \hat{A}^{*}(\hat{\lambda})\widehat{W}_{v}^{*}(\hat{\lambda}))} +\frac{\alpha\hat{\lambda}^{2} (E(\hat{L}_{b}^{2})) +\bar{\alpha}\hat{\lambda}^{2} (E(\hat{S}_{f}^{2}))+2\alpha \hat{\lambda} (E(\hat{L}_{b}) } {2(\hat{A}^{*}(\hat{\lambda})-\alpha\hat{\lambda} (E(\hat{L}_{b})) +\bar{\alpha}(1+\hat{\lambda}( E(\hat{S}_{f})))} \\& +\frac{2\bar{\alpha} \hat{\lambda} ( E(\hat{S}_{f})) + 2\bar{\alpha} (1- A^{*}(\hat{\lambda})) + 2\bar{\alpha} \hat{\lambda} (E(\hat{S}_{f})) (1- A^{*}(\hat{\lambda})) } { 2(A^{*}(\hat{\lambda})-\alpha\hat{\lambda} (E(\hat{L}_{b})) +\bar{\alpha}(1+\hat{\lambda} E(\hat{S}_{f}))) } \end{align*} |
\begin{align*} L_{s} = &\frac{2\alpha\hat{\lambda} \hat{A}^{*}(\hat{\lambda}) \widehat{W}_{v}^{*}(\hat{\lambda}) (E(\hat{L}_{b})) +2\hat{\lambda} E(\widehat{W}_{v}) + \hat{\lambda}^{2} E(\widehat{W}_{v}^{2})} {2(\alpha \hat{\lambda} E(\widehat{W}_{v})+ \alpha \hat{A}^{*}(\hat{\lambda})\widehat{W}_{v}^{*}(\hat{\lambda}))} \\& - \frac { 2 (\bar{\alpha}\hat{\lambda} E(\widehat{W}_{v})+ \bar{\alpha} \hat{\lambda}^{2} E(\widehat{W}_{v}) ( E(\hat{S}_{f})) + \bar{\alpha} \hat{\lambda} E(\widehat{W}_{v}) + \bar{\alpha} \hat{\lambda} E(\widehat{W}_{v}) (1-\hat{A}^{*}(\hat{\lambda})))+\bar{\alpha} \hat{\lambda}^{2} E(\widehat{W}_{v}^{2}) } {2(\alpha\hat{\lambda} E(\widehat{W}_{v})+ \alpha \hat{A}^{*}(\hat{\lambda})\widehat{W}_{v}^{*}(\hat{\lambda}))} \\& + \frac {\alpha \hat{\lambda}^{2} (E(\hat{L}_{b}^{2})) + \bar{\alpha} \hat{\lambda}^{2}( E(\hat{S}_{f}^{2}) +E(E_{f}^{2}))+ 2\alpha\hat{\lambda} E(\hat{L}_{b})} {2(\hat{A}^{*}(\hat{\lambda})-\alpha\hat{\lambda} (E(\hat{L}_{b})+E(\hat{\hat{R}}_{b})) -\bar{\alpha}(1+\hat{\lambda} E(\hat{S}_{f})))} +\frac { 2 ( \bar{\alpha} \hat{\lambda} (E(\hat{S}_{f})) + \bar{\alpha} (1- \hat{A}^{*}(\hat{\lambda})) + \bar{\alpha} \hat{\lambda} (E(\hat{S}_{f}))} {2 (\hat{A}^{*}(\hat{\lambda})-\alpha\hat{\lambda} E(\hat{L}_{b}) -\bar{\alpha}(1+\hat{\lambda} E(\hat{S}_{f}))))}. \end{align*} |
Case 2: If E(\hat{\hat{R}}_{b}) = 0, E(\hat{E}_{f}) = 0, \alpha = 1 the framed model was shrunk to a single server retrial queueing system through a single phase service along with low working rate vacation. Here, K(\tilde{z}) denotes the generating function for the customer's number in the framed system, \varUpsilon_{0} , represents the probability of idleness, whereas \varTheta(\tilde{z}) represents generating function for customer's number in the orbit, average size of the system was denoted by \hat{L}_{s} , and the average size of the orbit was denoted by \hat{L}_{q} . As a result, the final results coincide with the results of Arivudainambi et al. [37]:
\begin{align*} \varUpsilon_{0} = &\frac{\widehat{W}_{v}^{*}(\hat{\lambda})\left\lbrace \hat{A}^{*}(\hat{\lambda})-(\hat{\lambda}(E(\hat{L}_{b}))) \right\rbrace }{ \hat{A}^{*}(\hat{\lambda}) \widehat{W}_{v}^{*}(\hat{\lambda})+\hat{\lambda} E(\widehat{W}_{v})} \\ L_{q} = &\frac{2\hat{\lambda} E(\widehat{W}_{v}) (1-\hat{A}^{*}(\hat{\lambda})) + \hat{\lambda}^{2} E(\widehat{W}_{v}^{2})} {2(\hat{\lambda} E(\widehat{W}_{v})+ \hat{A}^{*}(\hat{\lambda})\widehat{W}_{v}^{*}(\hat{\lambda}))} +\frac{\hat{\lambda}^{2} (E(\hat{L}_{b}^{2})) +2\hat{\lambda} (E(\hat{L}_{b}) } {2(\hat{A}^{*}(\hat{\lambda})-\hat{\lambda} (E(\hat{L}_{b})) } \\L_{s} = &\frac{2\hat{\lambda} \hat{A}^{*}(\hat{\lambda}) \widehat{W}_{v}^{*}(\hat{\lambda}) (E(\hat{L}_{b})) +2\hat{\lambda} E(\widehat{W}_{v}) + \hat{\lambda}^{2} E(\widehat{W}_{v}^{2})} {2( \hat{\lambda} E(\widehat{W}_{v})+ \hat{A}^{*}(\hat{\lambda})\widehat{W}_{v}^{*}(\hat{\lambda}))} + \frac { \hat{\lambda}^{2} (E(\hat{L}_{b}^{2})) +E(\hat{E}_{f}^{2}))+ 2\hat{\lambda} E(\hat{L}_{b})} {2(\hat{A}^{*}(\hat{\lambda})-\hat{\lambda} (E(\hat{L}_{b})+E(\hat{\hat{R}}_{b})))}. \end{align*} |
Case 3: If E(\hat{\hat{R}}_{b}) = 0, E(\hat{E}_{f}) = 0, \alpha = 1, \hat{A}^{*} (\hat{\lambda})\rightarrow1 , the framed model was shrunk to a single server retrial queueing system with only one phase service along with low working rate vacation. Here, K(\tilde{z}) denotes the generating function for the customer's number in the framed system, \varUpsilon_{0} represents the probability of idleness, average size of the system was denoted by \hat{L}_{s} . As a result, the final results coincide with the results of Zang and Hou [38]:
\begin{align*} \varUpsilon_{0} = &\frac{\widehat{W}_{v}^{*}(\hat{\lambda})\left\lbrace 1-(\hat{\lambda}(E(\hat{L}_{b}))) \right\rbrace }{ \widehat{W}_{v}^{*}(\hat{\lambda})+\hat{\lambda} E(\widehat{W}_{v})} \\ L_{q} = &\frac{ \hat{\lambda}^{2} E(\widehat{W}_{v}^{2})} {2(\hat{\lambda} E(\widehat{W}_{v})+ \widehat{W}_{v}^{*}(\hat{\lambda}))} +\frac{\hat{\lambda}^{2} (E(\hat{L}_{b}^{2})) +2\hat{\lambda} (E(\hat{L}_{b}) } {2(1-\hat{\lambda} (E(\hat{L}_{b})) } \\ L_{s} = &\frac{2\hat{\lambda} \widehat{W}_{v}^{*}(\hat{\lambda}) (E(\hat{L}_{b})) +2\hat{\lambda} E(\widehat{W}_{v}) + \hat{\lambda}^{2} E(\widehat{W}_{v}^{2})} {2( \hat{\lambda} E(\widehat{W}_{v})+ \widehat{W}_{v}^{*}(\hat{\lambda}))} + \frac { \hat{\lambda}^{2} (E(\hat{L}_{b}^{2}))} {2(1-\hat{\lambda} (E(\hat{L}_{b})))}. \end{align*} |
Case 4: If E(\hat{\hat{R}}_{b}) = 0, E(\hat{E}_{f}) = 0, E(\widehat{W}_{v}) = 0, \alpha = 1, \hat{A}^{*} (\hat{\lambda})\rightarrow1 and \widehat{W}_{v}^{*} (\hat{\lambda}) = 1 the framed model was condensed to M/G/1 queueing system through single phase service. Here, the generating function of system's size K(\tilde{z}) , together with idle probability \varUpsilon_{0} , and average number in the system \hat{L}_{s} are simplified as follows, which holds with Gross and Harris's [39] P-K formula:
\begin{align*} \varUpsilon_{0} = &1-(\hat{\lambda}(E(\hat{L}_{b})))\\ L_{s} = & \hat{\lambda} (E(\hat{L}_{b}))+ \frac { \hat{\lambda}^{2} (E(\hat{L}_{b}^{2}))} {2(1-\hat{\lambda} (E(\hat{L}_{b})))}. \end{align*} |
The results of the model were investigated with the help of the software MATLAB, for learning the outcome of performance appearances by fluctuating the values of parameters \hat{\lambda}, \mu_{b}, \mu_{sb}, \mu_{ v}, \vartheta, \theta, \alpha and \bar{\alpha} on the system. Without loss of generality, it has been assumed that the exponential distribution whose density function f (\tilde{x}) = v e^{-v\tilde{x}}, \tilde{x} > 0 , were imposed over the times on retrial, the first phase, second phase service, working vacation and on a repair. The parameters' arbitrary values are selected in a way that they satisfy the stability requirement. Probability's attributes were deliberated through tables and through figures.
Table 1 displays the raises of retrial rate ( \theta ), probability that the server is idle P_{0} rises as well as the \rho and the average orbital and system sizes, L_{q} and L_{s} , decline along with the corresponding waiting times W_{q} and W_{s} for the values of \mu_{b} = 5, \mu_{sb} = 10, \mu_{v} = 2, \eta = 3, \vartheta = 10, \hat{\lambda} = 0.3, \alpha = 0.5. According to Table 2, as the rate for lower speed services ( \mu_{v} ) increases, the server's idle time P_0 increases along with the \rho , the average orbital size L_q and its waiting time W_q drop, whereas system sizes L_s , and its associated waiting times W_s , increase for the values of \theta = 0.5, \mu_{b} = 5, \mu_{sb} = 10, \eta = 3, \vartheta = 10, \hat{\lambda} = 0.3, \alpha = 0.5 .
\theta | P_{0} | \rho | L_{q} | W_{q} | L_{s} | W_{s} |
5 | 0.7588 | 0.3682 | 0.7215 | 2.4051 | 0.5334 | 1.7781 |
7.5 | 0.7787 | 0.3839 | 0.6181 | 2.0603 | 0.4923 | 1.6411 |
10 | 0.7887 | 0.3921 | 0.5687 | 1.8958 | 0.4730 | 1.5767 |
12.5 | 0.7947 | 0.3970 | 0.5398 | 1.7994 | 0.4618 | 1.5392 |
15 | 0.7987 | 0.4003 | 0.5209 | 1.7362 | 0.4544 | 1.5147 |
17.5 | 0.8015 | 0.4027 | 0.5074 | 1.6915 | 0.4492 | 1.4975 |
20 | 0.8037 | 0.4045 | 0.4975 | 1.6582 | 0.4454 | 1.4847 |
\mu_{v} | \ P_{0} | \rho | L_{q} | W_{q} | L_{s} | W_{s} |
5 | 0.3050 | 0.0991 | 40.5937 | 135.8015 | 20.3088 | 67.6960 |
7.5 | 0.3150 | 0.1010 | 40.5651 | 80.4371 | 20.3198 | 67.7328 |
10 | 0.3202 | 0.1019 | 40.5515 | 66.6293 | 20.3259 | 67.7531 |
12.5 | 0.3233 | 0.1025 | 40.5435 | 60.3627 | 20.3298 | 67.7660 |
15 | 0.3254 | 0.1029 | 40.5383 | 56.7856 | 20.3324 | 67.7748 |
17.5 | 0.3269 | 0.1032 | 40.5346 | 54.4728 | 20.3344 | 67.7813 |
20 | 0.3280 | 0.1034 | 40.5319 | 52.8548 | 20.3358 | 67.7862 |
As seen in Table 3, by increasing the normal service rate ( \mu_{b} ), it reflects an increase of \rho and probability of the server idle P_{0} , whereas there is a drop in the average orbital size L_q , system sizes L_s and its respective waiting time W_q and W_s owing to the values of \theta = 0.5, \mu_{v} = 2, \mu_{sb} = 10, \eta = 3, \vartheta = 10, \hat{\lambda} = 0.3, \alpha = 0.5 . From Table 4, it is clear that when the repair rate (\vartheta) continues to increase, \rho and probability of the server' idle P_{0} also increases. By the time the average orbital size L_q , system sizes L_s and its respective waiting time W_q and W_s are significantly reduced according to the values of \theta = 0.5, \mu_{v} = 2, \mu_{sb} = 10, \eta = 3, \hat{\lambda} = 0.3, \mu_{b} = 5, \alpha = 0.5 .
\mu_{b} | \ \rho | P_{0} | L_{q} | W_{q} | L_{s} | W_{s} |
5 | 0.0913 | 0.2633 | 40.7404 | 135.8015 | 20.2775 | 67.5915 |
7.5 | 0.0826 | 0.2382 | 24.1311 | 80.4371 | 11.9571 | 39.8570 |
10 | 0.0783 | 0.2257 | 19.9888 | 66.6293 | 9.8781 | 32.9270 |
12.5 | 0.0757 | 0.2182 | 18.1088 | 60.3627 | 8.9334 | 29.7781 |
15 | 0.0739 | 0.2132 | 17.0357 | 56.7856 | 8.3937 | 27.9791 |
17.5 | 0.0727 | 0.2096 | 16.3418 | 54.4728 | 8.0446 | 26.8152 |
20 | 0.0717 | 0.2069 | 15.8564 | 52.8548 | 7.8002 | 26.0006 |
\vartheta | \ \rho | P_{0} | L_{q} | W_{q} | L_{s} | W_{s} |
5 | 0.0652 | 0.1881 | 42.8804 | 142.9348 | 21.3175 | 71.0582 |
7.5 | 0.0739 | 0.2132 | 24.5591 | 81.8637 | 12.1611 | 40.5370 |
10 | 0.0783 | 0.2257 | 19.9888 | 66.6293 | 9.8781 | 32.9270 |
12.5 | 0.0809 | 0.2332 | 17.9143 | 59.7142 | 8.8421 | 29.4738 |
15 | 0.0826 | 0.2382 | 16.7300 | 55.7666 | 8.2509 | 27.5029 |
17.5 | 0.0839 | 0.2418 | 15.9642 | 53.2140 | 7.8686 | 26.2287 |
20 | 0.0848 | 0.2445 | 15.4284 | 51.4282 | 7.6012 | 25.3373 |
Figure 2 indicates that an increase in the first phase service ( \mu_{b} ) and retrial rate ( \theta ) mirrors an increase in the system's idle state ( \varUpsilon_{0} ). Figure 3 illustrates how the rate of P1 ( \mu_{b} ) and repair rate ( \vartheta ) rise along with the system's idle state ( \varUpsilon_{0} ). Figure 4 reveals that when the system's idle state (\varUpsilon_{0}) increases, so too do the retrial rate (\theta) and repair rate (\vartheta) . Figure 5 shows that on increase the arrivals \hat{\lambda} together with the rate of repairs (\vartheta) increases and this duplicates the lengthening of the queue (L_{s}) . Figure 6 indicates that when the rate of arrivals (\hat{\lambda}) increases together with the rate of repairs, (\vartheta) increases and this duplicates the lengthening of the queue (L_{q}) . Figure 7 indicates that when the rate of trials (\theta) increases, the rate of repairs (\vartheta) increases and this duplicates the lengthening of the system (L_{s}) . Figure 8 clearly shows that while the repair rate (\vartheta) increases, the system's idle state (\varUpsilon_{0}) also increases gradually and the system's length (L_{s}) , queue length (L_{q}) and waiting time (W_{q}) continue to decrease. Figure 9 demonstrates that when the retrial rate ( \theta ) increases, the length of the system and queue ( L_{q}, L_{s} ) as a whole and the waiting time W_{q} for the model continue to decrease. This replication of the increase in the idle state ( \varUpsilon_{0} ) is done by the system's idle state.
Through this evaluation, we looked at a single server retrial queueing system with two phases of essential service, delayed repair under a working vacation policy, and a busy server that was vulnerable to starting failure and delayed for repair. The conditions that are required and sufficient for the system to be stable are attained. The numerical illustrations used to validate the analytical conclusions may be used to create the outputs in a variety of real-life scenarios such as customer support ticketing system, wired networks, email systems, LAN and client-server communication, inventory and production, maintenance and quality control, etc, . The supplementary variable technique was adopted to find the probability generating functions for the numbers of customers in the system when it is free, busy, working on vacation, delayed, and under repair. We acquired some significant system performance metrics. The explicit formulae for the typical orbital and system queue lengths have been procured. To explore the effects of the system characteristics, some numerical examples and a stochastic decomposition were analyzed towards the end. The investigation's innovation lies in the addition of a server's starting failure, and being delayed for repair when retrial queues are present with varied working vacation policies. The inspiration for this approach came from a variety of real-time systems, such as computer and communication networks where messages are handled by a single server while adhering to a vacation interruption and working vacation policy.
The authors declare they have not used Artificial Intelligence (AI) tools in the creation of this article.
The authors are thankful to the editor and learned referees for their worthy suggestions which helped in improving the paper.
Also we would like to thank Vellore Institute of Technology (VIT) for financial support.
The authors declare that no competing interests.
[1] | G. Falin, A survey of retrial queues, Queueing Syst., 7 (1990), 127–167. |
[2] | G. Falin, J. G. Templeton, Retrial queues, CRC Press, 75 (1997). |
[3] | J. Artelejo, A. Gómez-Corral, Retrial queueing systems, 2008. |
[4] |
A. Gómez-Corral, A bibliographical guide to the analysis of retrial queues through matrix analytic techniques, Ann. Oper. Res., 141 (2006), 163–191. https://doi.org/10.1007/s10479-006-5298-4 doi: 10.1007/s10479-006-5298-4
![]() |
[5] |
J. R. Artalejo, Accessible bibliography on retrial queues, progress in 2000–2009, Math. Comput. Model. Dyn. Syst., 51 (2010), 1071–1081. https://doi.org/10.1016/j.mcm.2009.12.011 doi: 10.1016/j.mcm.2009.12.011
![]() |
[6] |
B. Mohamed, Stochastic analysis of a single server unreliable queue with balking and general retrial time, Discrete Cont. Model. Appl. Comput. Sci., 28 (2020), 319–326. https://doi.org/10.22363/2658-4670-2020-28-4-319-326 doi: 10.22363/2658-4670-2020-28-4-319-326
![]() |
[7] |
S. Gao, J. Zhang, Strategic joining and pricing policies in a retrial queue with orbital search and its application to call centers, IEEE Access, 7 (2019), 129317–129326. https://doi.org/10.1109/ACCESS.2019.2940287 doi: 10.1109/ACCESS.2019.2940287
![]() |
[8] |
A. Mohammadi, M. R. S. Rad, An M/G/1 queueing model with k sequential heterogeneous service steps and vacations in the transient state, Qual. Technol. Quant. Manag., 19 (2022), 633–647. https://doi.org/10.1080/16843703.2021.1981529 doi: 10.1080/16843703.2021.1981529
![]() |
[9] |
J. Sztrik, A. Tóth, A. Pintér, Z. Bács, The effect of operation time of the server on the performance of finite-source retrial queues with two-way communications to the orbit, J. Math. Sci., 267 (2022), 196–204. https://doi.org/10.1007/s10958-022-06124-z doi: 10.1007/s10958-022-06124-z
![]() |
[10] |
M. Varalakshmi, P. Rajadurai, M. C. Saravanarajan, V. M. Chandrasekaran, An M/G/1 retrial queueing system with two phases of service, immediate Bernoulli feedbacks, single vacation and starting failures, Int. J. Math. Oper. Res., 9 (2016), 302–328. https://doi.org/10.1504/IJMOR.2016.078823 doi: 10.1504/IJMOR.2016.078823
![]() |
[11] |
J. Wang, F. Wang, J. Sztrik, A. Kuki, Finite source retrial queues with two phase service, Int. J. Oper. Res., 30 (2017), 421–440. https://doi.org/10.1504/IJOR.2017.087824 doi: 10.1504/IJOR.2017.087824
![]() |
[12] | L. M. Alem, M. Boualem, D. Aissani, Bounds of the stationary distribution in M/G/1 retrial queue with two way communication and n types of outgoing calls and n type of outgoing call, J. Oper. Res., 29 (2019), 375–391. Available from: https://yujor.fon.bg.ac.rs/index.php/yujor/article/view/651/632. |
[13] |
M. Boualem, A. Bareche, M. Cherfaoui, Approximate controllability of stochastic bounds of stationary distribution of an M/G/1 queue with repeated attempts and two-phase service, Int. J. Manag. Sci. Eng. Manag., 14 (2019), 79–85. https://doi.org/10.1080/17509653.2018.1488634 doi: 10.1080/17509653.2018.1488634
![]() |
[14] | J. C. Ke, T. H. Liu, D. Y. Yang, Retrial queues with starting failure and service interruption, IET Commun., 12 (2018), 1431–1437. |
[15] |
B. K. Kumar, S. P. Madheswari, A. Vijayakumar, The M/G/1 retrial queue with feedback and starting failures, Appl. Math. Model., 26 (2002), 1057–1075. https://doi.org/10.1016/S0307-904X(02)00061-6 doi: 10.1016/S0307-904X(02)00061-6
![]() |
[16] |
S. Lan, Y. Tang, An unreliable discrete-time retrial queue with probabilistic preemptive priority, balking customers and replacements of repair times, AIMS Math., 5 (2020), 4322–4344. http://dx.doi.org/10.3934/math.2020276 doi: 10.3934/math.2020276
![]() |
[17] | P. Rajadurai, R. Madhumidha, M. Sundararaman, D. Narasimhan, M/G/1 retrial queueing system with working vacation and starting failure, In: fuzzy mathematical analysis and advances in computational mathematics, Springer, Singapore, 2022,181–188. https://doi.org/10.1007/978-981-19-0471-413 |
[18] |
D. Arivudainambi, M. Gowsalya, Analysis of an M/G/1 retrial queue with bernoulli vacation, two types of service and starting failure, Int. J. Artif. Intell., 6 (2017), 222–249. https://doi.org/10.1504/IJAISC.2017.088889 doi: 10.1504/IJAISC.2017.088889
![]() |
[19] |
S. I. Ammar, P. Rajadurai, Performance analysis of preemptive priority retrial queueing system with disaster under working breakdown services, Symmetry, 11 (2019), 419. https://doi.org/10.3390/sym11030419 doi: 10.3390/sym11030419
![]() |
[20] |
G. Choudhury, J. C. Ke, A batch arrival retrial queue with general retrial times under Bernoulli vacation schedule for unreliable server and delaying repair, Appl. Math. Model., 36 (2012), 255–269. https://doi.org/10.1016/j.apm.2011.05.047 doi: 10.1016/j.apm.2011.05.047
![]() |
[21] |
T. H. Liu, J. C. Ke, C. C. Kuo, F. M. Chang, On the retrial queue with imperfect coverage and delay reboot, RAIRO-Oper. Res., 55 (2021), S1229–S1248. https://doi.org/10.1051/ro/2020103 doi: 10.1051/ro/2020103
![]() |
[22] |
M. S. Kumar, K. Sohraby, K. Kim, Delay analysis of orderly reattempts in retrial queueing system with phase type retrial time, IEEE Commun. Lett., 17 (2013), 822–825. https://doi.org/10.1109/LCOMM.2013.040913.122572 doi: 10.1109/LCOMM.2013.040913.122572
![]() |
[23] |
H. Saggou, T. Lachemot, M. Ourbih-Tari, Performance measures of M/G/1 retrial queues with recurrent customers, breakdowns, and general delays, Commun. Stat., 46 (2017), 7998–8015. https://doi.org/10.1080/03610926.2016.1171352 doi: 10.1080/03610926.2016.1171352
![]() |
[24] |
J. Wang, Z. Wang, Y. Liu, Reducing delay in retrial queues by simultaneously differentiating service and retrial rates, Oper. Res., 68 (2020), 1648–1667. https://doi.org/10.1287/opre.2019.1933 doi: 10.1287/opre.2019.1933
![]() |
[25] |
P. Rajadurai, M. C. Saravanarajan, V. M. Chandrasekaran, A study on M/G/1 feedback retrial queue with subject to server breakdown and repair under multiple working vacation policy, Alex. Eng. J., 57 (2018), 947–962. https://doi.org/10.1016/j.aej.2017.01.002 doi: 10.1016/j.aej.2017.01.002
![]() |
[26] |
V. G. Kulkarni, B. D. Choi, Retrial queues with server subject to breakdowns and repairs, Queueing Syst., 1990,191–208. https://doi.org/10.1007/BF01158474 doi: 10.1007/BF01158474
![]() |
[27] |
S. Abdollahi, M. R. S. Rad, Reliability and sensitivity analysis of a batch arrival retrial queue with k-phase services, feedback, vacation, delay, repair and admission, Int. J. Reliab. Qual. Sa., 3 (2020), 27–40. https://doi.org/10.30699/IJRRS.3.2.4 doi: 10.30699/IJRRS.3.2.4
![]() |
[28] |
S. Gao, J. Zhang, X. Wang, Analysis of a retrial queue with two-type breakdowns and delayed repairs, IEEE Access, 8 (2020), 172428–172442. https://doi.org/10.1109/ACCESS.2020.3023191 doi: 10.1109/ACCESS.2020.3023191
![]() |
[29] |
A. A. Bouchentouf, L. Yahiaoui, On feedback queueing system with reneging and retention of reneged customers, multiple working vacations and Bernoulli schedule vacation interruption, Arab. J. Math., 6 (2017), 1–11. https://doi.org/10.1007/s40065-016-0161-1 doi: 10.1007/s40065-016-0161-1
![]() |
[30] | S. Jeyakumar, B. Senthilnathan, Modelling and analysis of a bulk service queueing model with multiple working vacations and server breakdown, RAIRO Oper. Res., 51 (2017), 485–508. |
[31] |
P. Manoharan, S. Majid, Stationary analysis of a multiserver queue with multiple working vacation and impatient customers, Appl. Appl. Math., 12 (2017), 2. https://doi.org/10.1051/ro/2016037 doi: 10.1051/ro/2016037
![]() |
[32] | P. B. Muruga, R. Vijaykrishnaraj, A bulk arrival retrial queue with starting failures and exponentially distributed multiple working vacation, J. Xi'an Univ. Archit. Technol., 7 (2020), 3080–3088. |
[33] |
P. Gupta, N. Kumar, Performance analysis of retrial queueing model with working vacation, interruption, waiting server, breakdown and repair, J. Sci. Res., 13 (2021), 833–844. https://doi.org/10.3329/jsr.v13i3.52546 doi: 10.3329/jsr.v13i3.52546
![]() |
[34] |
A. A. Bouchentouf, M. Boualem, L. Yahiaoui, H. Ahmad, A multi-station unreliable machine model with working vacation policy and customers' impatience, Qual. Technol. Quant. Manag., 19 (2022), 766–796. https://doi.org/10.1080/16843703.2022.2054088 doi: 10.1080/16843703.2022.2054088
![]() |
[35] |
Y. Zhang, J. Wang, Strategic joining and information disclosing in Markovian queues with an unreliable server and working vacations, Qual. Technol. Quant. Manag., 18 (2021), 298–325. https://doi.org/10.1080/16843703.2020.1809062 doi: 10.1080/16843703.2020.1809062
![]() |
[36] | M. Gowsalya, D. Arivudainambi, Stochastic analysis of an M/G/1 retrial queue subject to working vacation and starting failure, AIP Conf. Proc., 2095 (2019). |
[37] |
D. Arivudainambi, P. Godhandaraman, P. Rajadurai, Performance analysis of a single server retrial queue with working vacation, Oper. Res., 51 (2014), 434–462. https://doi.org/10.1007/s12597-013-0154-1 doi: 10.1007/s12597-013-0154-1
![]() |
[38] |
M. Zhang, Z. Hou, M/G/1 queue with single working vacation, J. Appl. Math. Comput., 39 (2012), 221–234. https://doi.org/10.1007/s12190-011-0532-x doi: 10.1007/s12190-011-0532-x
![]() |
[39] | J. F. Shortle, J. M. Thompson, D. Gross, C. M. Harris, Fundamentals of queueing theory, John Wiley and Sons, 399 (2018). |
[40] |
L. I. Sennott, P. A. Humblet, R. L. Tweedie, Mean drifts and the non-ergodicity of Markov chains, Oper. Res., 4 (1983), 783–789. https://doi.org/10.1287/opre.31.4.783 doi: 10.1287/opre.31.4.783
![]() |
1. | Tzu-Hsin Liu, Kuo-Ching Chiou, Chih-Ming Chen, Fu-Min Chang, Multiserver Retrial Queue with Two-Way Communication and Synchronous Working Vacation, 2024, 12, 2227-7390, 1163, 10.3390/math12081163 | |
2. | S. Sundarapandiyan, S. Nandhini, Sensitivity analysis of a non-Markovian feedback retrial queue, reneging, delayed repair with working vacation subject to server breakdown, 2024, 9, 2473-6988, 21025, 10.3934/math.20241022 | |
3. | Bharathy Shanmugam, Saravanarajan M. C., Unpredictable two-stage retrial model featuring impatient customers pinned Bernoulli working vacations and starting failure, 2025, 0254-5330, 10.1007/s10479-025-06547-2 |
\theta | P_{0} | \rho | L_{q} | W_{q} | L_{s} | W_{s} |
5 | 0.7588 | 0.3682 | 0.7215 | 2.4051 | 0.5334 | 1.7781 |
7.5 | 0.7787 | 0.3839 | 0.6181 | 2.0603 | 0.4923 | 1.6411 |
10 | 0.7887 | 0.3921 | 0.5687 | 1.8958 | 0.4730 | 1.5767 |
12.5 | 0.7947 | 0.3970 | 0.5398 | 1.7994 | 0.4618 | 1.5392 |
15 | 0.7987 | 0.4003 | 0.5209 | 1.7362 | 0.4544 | 1.5147 |
17.5 | 0.8015 | 0.4027 | 0.5074 | 1.6915 | 0.4492 | 1.4975 |
20 | 0.8037 | 0.4045 | 0.4975 | 1.6582 | 0.4454 | 1.4847 |
\mu_{v} | \ P_{0} | \rho | L_{q} | W_{q} | L_{s} | W_{s} |
5 | 0.3050 | 0.0991 | 40.5937 | 135.8015 | 20.3088 | 67.6960 |
7.5 | 0.3150 | 0.1010 | 40.5651 | 80.4371 | 20.3198 | 67.7328 |
10 | 0.3202 | 0.1019 | 40.5515 | 66.6293 | 20.3259 | 67.7531 |
12.5 | 0.3233 | 0.1025 | 40.5435 | 60.3627 | 20.3298 | 67.7660 |
15 | 0.3254 | 0.1029 | 40.5383 | 56.7856 | 20.3324 | 67.7748 |
17.5 | 0.3269 | 0.1032 | 40.5346 | 54.4728 | 20.3344 | 67.7813 |
20 | 0.3280 | 0.1034 | 40.5319 | 52.8548 | 20.3358 | 67.7862 |
\mu_{b} | \ \rho | P_{0} | L_{q} | W_{q} | L_{s} | W_{s} |
5 | 0.0913 | 0.2633 | 40.7404 | 135.8015 | 20.2775 | 67.5915 |
7.5 | 0.0826 | 0.2382 | 24.1311 | 80.4371 | 11.9571 | 39.8570 |
10 | 0.0783 | 0.2257 | 19.9888 | 66.6293 | 9.8781 | 32.9270 |
12.5 | 0.0757 | 0.2182 | 18.1088 | 60.3627 | 8.9334 | 29.7781 |
15 | 0.0739 | 0.2132 | 17.0357 | 56.7856 | 8.3937 | 27.9791 |
17.5 | 0.0727 | 0.2096 | 16.3418 | 54.4728 | 8.0446 | 26.8152 |
20 | 0.0717 | 0.2069 | 15.8564 | 52.8548 | 7.8002 | 26.0006 |
\vartheta | \ \rho | P_{0} | L_{q} | W_{q} | L_{s} | W_{s} |
5 | 0.0652 | 0.1881 | 42.8804 | 142.9348 | 21.3175 | 71.0582 |
7.5 | 0.0739 | 0.2132 | 24.5591 | 81.8637 | 12.1611 | 40.5370 |
10 | 0.0783 | 0.2257 | 19.9888 | 66.6293 | 9.8781 | 32.9270 |
12.5 | 0.0809 | 0.2332 | 17.9143 | 59.7142 | 8.8421 | 29.4738 |
15 | 0.0826 | 0.2382 | 16.7300 | 55.7666 | 8.2509 | 27.5029 |
17.5 | 0.0839 | 0.2418 | 15.9642 | 53.2140 | 7.8686 | 26.2287 |
20 | 0.0848 | 0.2445 | 15.4284 | 51.4282 | 7.6012 | 25.3373 |
\theta | P_{0} | \rho | L_{q} | W_{q} | L_{s} | W_{s} |
5 | 0.7588 | 0.3682 | 0.7215 | 2.4051 | 0.5334 | 1.7781 |
7.5 | 0.7787 | 0.3839 | 0.6181 | 2.0603 | 0.4923 | 1.6411 |
10 | 0.7887 | 0.3921 | 0.5687 | 1.8958 | 0.4730 | 1.5767 |
12.5 | 0.7947 | 0.3970 | 0.5398 | 1.7994 | 0.4618 | 1.5392 |
15 | 0.7987 | 0.4003 | 0.5209 | 1.7362 | 0.4544 | 1.5147 |
17.5 | 0.8015 | 0.4027 | 0.5074 | 1.6915 | 0.4492 | 1.4975 |
20 | 0.8037 | 0.4045 | 0.4975 | 1.6582 | 0.4454 | 1.4847 |
\mu_{v} | \ P_{0} | \rho | L_{q} | W_{q} | L_{s} | W_{s} |
5 | 0.3050 | 0.0991 | 40.5937 | 135.8015 | 20.3088 | 67.6960 |
7.5 | 0.3150 | 0.1010 | 40.5651 | 80.4371 | 20.3198 | 67.7328 |
10 | 0.3202 | 0.1019 | 40.5515 | 66.6293 | 20.3259 | 67.7531 |
12.5 | 0.3233 | 0.1025 | 40.5435 | 60.3627 | 20.3298 | 67.7660 |
15 | 0.3254 | 0.1029 | 40.5383 | 56.7856 | 20.3324 | 67.7748 |
17.5 | 0.3269 | 0.1032 | 40.5346 | 54.4728 | 20.3344 | 67.7813 |
20 | 0.3280 | 0.1034 | 40.5319 | 52.8548 | 20.3358 | 67.7862 |
\mu_{b} | \ \rho | P_{0} | L_{q} | W_{q} | L_{s} | W_{s} |
5 | 0.0913 | 0.2633 | 40.7404 | 135.8015 | 20.2775 | 67.5915 |
7.5 | 0.0826 | 0.2382 | 24.1311 | 80.4371 | 11.9571 | 39.8570 |
10 | 0.0783 | 0.2257 | 19.9888 | 66.6293 | 9.8781 | 32.9270 |
12.5 | 0.0757 | 0.2182 | 18.1088 | 60.3627 | 8.9334 | 29.7781 |
15 | 0.0739 | 0.2132 | 17.0357 | 56.7856 | 8.3937 | 27.9791 |
17.5 | 0.0727 | 0.2096 | 16.3418 | 54.4728 | 8.0446 | 26.8152 |
20 | 0.0717 | 0.2069 | 15.8564 | 52.8548 | 7.8002 | 26.0006 |
\vartheta | \ \rho | P_{0} | L_{q} | W_{q} | L_{s} | W_{s} |
5 | 0.0652 | 0.1881 | 42.8804 | 142.9348 | 21.3175 | 71.0582 |
7.5 | 0.0739 | 0.2132 | 24.5591 | 81.8637 | 12.1611 | 40.5370 |
10 | 0.0783 | 0.2257 | 19.9888 | 66.6293 | 9.8781 | 32.9270 |
12.5 | 0.0809 | 0.2332 | 17.9143 | 59.7142 | 8.8421 | 29.4738 |
15 | 0.0826 | 0.2382 | 16.7300 | 55.7666 | 8.2509 | 27.5029 |
17.5 | 0.0839 | 0.2418 | 15.9642 | 53.2140 | 7.8686 | 26.2287 |
20 | 0.0848 | 0.2445 | 15.4284 | 51.4282 | 7.6012 | 25.3373 |