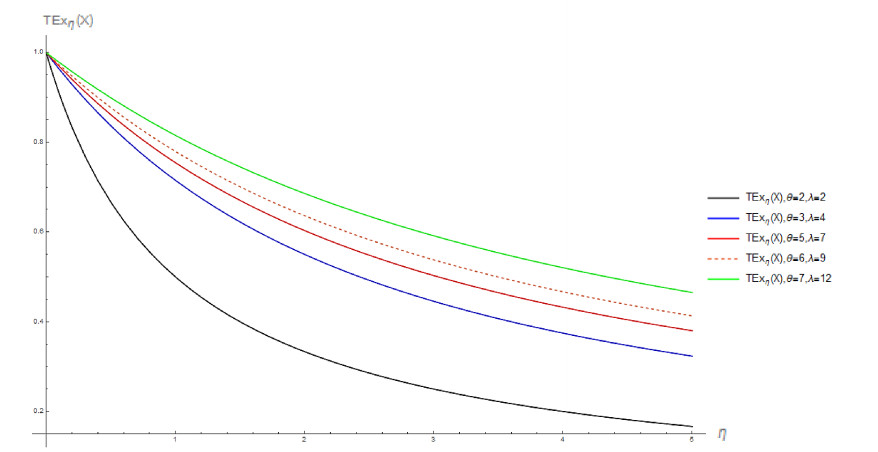
In this paper, the Tsallis and Renyi extropy is presented as a continuous measure of information under the continuous distribution. Furthermore, the features and their connection to other information measures are introduced. Some stochastic comparisons and results on the order statistics and upper records are given. Moreover, some theorems about the maximum Tsallis and Renyi extropy are discussed. On the other hand, numerical results of the non-parametric estimation of Tsallis extropy are calculated for simulated and real data with application to time series model and its forecasting.
Citation: Mohamed Said Mohamed, Najwan Alsadat, Oluwafemi Samson Balogun. Continuous Tsallis and Renyi extropy with pharmaceutical market application[J]. AIMS Mathematics, 2023, 8(10): 24176-24195. doi: 10.3934/math.20231233
[1] | Ramy Abdelhamid Aldallal, Haroon M. Barakat, Mohamed Said Mohamed . Exploring weighted Tsallis extropy: Insights and applications to human health. AIMS Mathematics, 2025, 10(2): 2191-2222. doi: 10.3934/math.2025102 |
[2] | Mohamed Said Mohamed, Haroon M. Barakat, Aned Al Mutairi, Manahil SidAhmed Mustafa . Further properties of Tsallis extropy and some of its related measures. AIMS Mathematics, 2023, 8(12): 28219-28245. doi: 10.3934/math.20231445 |
[3] | Mansour Shrahili . Some new results involving residual Renyi's information measure for k-record values. AIMS Mathematics, 2024, 9(5): 13313-13335. doi: 10.3934/math.2024649 |
[4] | Mansour Shrahili, Mohamed Kayid . Uncertainty quantification based on residual Tsallis entropy of order statistics. AIMS Mathematics, 2024, 9(7): 18712-18731. doi: 10.3934/math.2024910 |
[5] | Baria A. Helmy, Amal S. Hassan, Ahmed K. El-Kholy, Rashad A. R. Bantan, Mohammed Elgarhy . Analysis of information measures using generalized type-Ⅰ hybrid censored data. AIMS Mathematics, 2023, 8(9): 20283-20304. doi: 10.3934/math.20231034 |
[6] | M. R. Irshad, S. Aswathy, R. Maya, Amer I. Al-Omari, Ghadah Alomani . A flexible model for bounded data with bathtub shaped hazard rate function and applications. AIMS Mathematics, 2024, 9(9): 24810-24831. doi: 10.3934/math.20241208 |
[7] | Mansour Shrahili, Mohamed Kayid, Mhamed Mesfioui . Stochastic inequalities involving past extropy of order statistics and past extropy of record values. AIMS Mathematics, 2024, 9(3): 5827-5849. doi: 10.3934/math.2024283 |
[8] | I. A. Husseiny, M. Nagy, A. H. Mansi, M. A. Alawady . Some Tsallis entropy measures in concomitants of generalized order statistics under iterated FGM bivariate distribution. AIMS Mathematics, 2024, 9(9): 23268-23290. doi: 10.3934/math.20241131 |
[9] | Refah Alotaibi, Mazen Nassar, Zareen A. Khan, Wejdan Ali Alajlan, Ahmed Elshahhat . Entropy evaluation in inverse Weibull unified hybrid censored data with application to mechanical components and head-neck cancer patients. AIMS Mathematics, 2025, 10(1): 1085-1115. doi: 10.3934/math.2025052 |
[10] | Ayed. R. A. Alanzi, Muhammad Imran, M. H. Tahir, Christophe Chesneau, Farrukh Jamal, Saima Shakoor, Waqas Sami . Simulation analysis, properties and applications on a new Burr XII model based on the Bell-X functionalities. AIMS Mathematics, 2023, 8(3): 6970-7004. doi: 10.3934/math.2023352 |
In this paper, the Tsallis and Renyi extropy is presented as a continuous measure of information under the continuous distribution. Furthermore, the features and their connection to other information measures are introduced. Some stochastic comparisons and results on the order statistics and upper records are given. Moreover, some theorems about the maximum Tsallis and Renyi extropy are discussed. On the other hand, numerical results of the non-parametric estimation of Tsallis extropy are calculated for simulated and real data with application to time series model and its forecasting.
Supported by R, the continuous Shannon entropy (Shannon [14]) of the random variable (RV) X is given by
SH(X)=−E(lnh(X))=−∫Rh(x)lnh(x)dx, | (1.1) |
where h(.) is the probability density function (pdf). Lad et al. [5] produced the extropy as a dual Shannon entropy measure. The extropy of the discrete RV X supported on Q={x1,...,xN} and with corresponding probability vector p=(p1,...,pN), is
Ex(X)=−N∑i=1(1−pi)ln(1−pi). | (1.2) |
Moreover, the view of the extropy of the continuous RV X supported on R has been introduced in many pieces of literature, see for example Raqab and Qiu [11] and Qiu [9], can be shown as follows:
Ex(X)=−12∫Rh(x)2dx. | (1.3) |
The literature has offered several entropy measures and their generalizations. Through the various uncertainty generalizations, Tsallis [15] presented the Tsallis entropy. The continuous Tsallis (C-Ts) entropy of the continuous RV X supported on R, 1≠η>0, is defined as follows:
TEnη(X)=1η−1(1−∫Rhη(x)dx), | (1.4) |
when η is 1, then limη→1TEnη(X)=SH(X).
Renyi [12] suggested a model referred to as continuous Renyi (C-Re) entropy of order η of the continuous RV X with pdf h(x) as
REnη(X)=11−ηln∫∞0hη(x)dx, | (1.5) |
where 1≠η>0. It's simple to see that, when η→1, REnη(X) tends to SH(X).
The Tsallis and Renyi extropy under the discrete distribution have been presented in the literature. Xue and Deng [19] suggested the model Tsallis of extropy, the dual of Tsallis entropy function, and examined its maximum value. Besides, Balakrishnan et al. [2] study the Tsallis of extropy and apply it to pattern recognition. Liu and Xiao [6] introduced Renyi extropy and looked at the maximum value of it. Jawa et al. [4] discuss the past and residual of Tsallis and Renyi extropy via the softmax function.
This paper introduces the C-Ts and C-Re extropy under the continuous distribution lifetime. Moreover, presenting the maximum of both models. The remainder of this article is as follows: Section 2 discusses the C-Ts extropy model with its properties and their connection to other measures. Furthermore, examples of the models for different distributions are introduced. Section 3 gives the maximum C-Ts extropy and some properties depending on it. Section 4 provides the maximum CRe extropy. Finally, Section 5 ends the article with some non-parametric estimations of C-Ts extropy applied to simulated and real data and discusses the estimation for the forecasting time series of OECD pharmaceutical market data.
In this section, we introduce the rendition of the C-Ts extropy based on the continuous distribution lifetime.
In the same manner, introduced in Lad et al. [5], we can present the extropy of the continuous RV X supported on R as follows:
Ex(X)=−∫R(1−h(x))ln(1−h(x))dx. | (2.1) |
In our work, we will deal with both Eqs (1.3) and (2.1) as a representative form of extropy.
Inspired by the idea of discrete Tsallis of extropy, and the continuous distribution lifetime, we present the C-Ts extropy by the following definition.
Definition 2.1. Let X be a continuous RV supported in [a,b], −∞<a<b<∞, having a pdf h(.). Before we introduce the concept of C-Ts extropy, we must mention that the value of the expression (1−h(x))η can be negative or non-negative according to the value of the pdf h(x)>1 or h(x)≤1, respectively. If h(x)≤1, then (1−h(x))η gives real value for all 1≠η>0. If h(x)>1, then (1−h(x))η gives real value when η∈Z+∖{1}. Otherwise, it gives a complex result when η is a non-positive integer. Then, the C-Ts extropy can be given as
TExη(X)=1η−1(∫ba(1−h(x))dx−∫ba(1−h(x))ηdx)=1η−1(b−a−1−∫ba(1−h(x))ηdx), | (2.2) |
where the conditions on η can be given in two cases:
(1) 1≠η>0 if h(x)≤1.
(2) η∈Z+∖{1} if h(x)>1.
Proposition 2.1. Assume that X is a continuous RV supported in [a,b], −∞<a<b<∞. From (2.2), where 1≠η>0, if h(x)≤1 then the C-Ts extropy is non-negative.
Proof. From (2.2), the C-Ts extropy can be rewritten as
TExη(X)=1η−1(∫ba(1−h(x))dx−∫ba(1−h(x))ηdx)=1η−1(∫ba(1−h(x))(1−(1−h(x))η−1)dx). | (2.3) |
Provided that h(x)≤1, when η>1, the function z(y)=yη−1 is increasing, y>0, therefore 1−(1−h(x))η−1≥0. While, when 0<η<1, the function z(y)=yη−1 is decreasing, y>0, therefore 1−(1−h(x))η−1≤0. Then, the C-Ts extropy is non-negative.
Example 2.1. Assume that the continuous RV X has a continuous uniform distribution over [a,b], −∞<a<b<∞ symbolize by U(a,b) with pdf h(x)=1b−a. Then, from (2.2), the C-Ts extropy is given by
TExη(X)=1η−1(b−a−1−(b−a−1)η(b−a)η−1), | (2.4) |
where 1≠η>0 if h(x)≤1 and η∈Z+∖{1} if h(x)>1. In particular, the C-Ts extropy equals zero if b−a=1.
Example 2.2. Consider that the continuous RV X has power function distribution with pdf given by
h(x)=θx(θ−1)λθ,0≤x≤λ,andθ,λ>0. |
Then, from (2.2), the C-Ts extropy is given by
TExη(X)=1η−1(λ−1−∫λ0(1−θx(θ−1)λθ)ηdx), |
where 1≠η>0 if h(x)≤1 and η∈Z+∖{1} if h(x)>1. Figure 1 shows the C-Ts extropy of power function distribution with different values of θ and λ. Furthermore, we can see that when the difference between θ and λ increases, the C-Ts extropy increases.
In view of Figure 1, we can see that all the given values of θ and λ of the power function distribution satisfy the condition h(x)≤1 in Eq (2.2) and C-Ts extropy exist where 1≠η>0. For example, Figure 2 shows the plot of h(x)≤1 when θ=5 and 0<λ≤7. In contrast, Figure 2 shows that h(x) has the values h(x)≤1 and h(x)>1, for values like θ=6 and 0<λ≤4. As a result, the value of C-Ts extropy will only exist under the conditions described in Definition 2.1.
The next proposition discuss the C-Ts extropy when η tends to 1.
Proposition 2.2. Providing that X is a continuous RV supported in [a,b], −∞<a<b<∞. Then, from (2.1) and (2.2), we have
limη→1TExη(X)=Ex(X), | (2.5) |
which is valid only for 1≠η>0 and h(x)≤1.
Proof. From (2.2), with applying L′Hˆopital′s rule, we get
limη→1TExη(X)=limη→11η−1(b−a−1−∫ba(1−h(x))ηdx)=limη→1−∫ba(1−h(x))ηln(1−h(x))dx=−∫ba(1−h(x))ln(1−h(x))dx=Ex(X). |
If h(x)≥1, then η∈Z+∖{1}={2,3,...}, which can't be tends to 1. Thus, limη→1TExη(X)=Ex(X) only when 1≠η>0 and h(x)≤1
In the next, we will obtain some significant results of C-Ts extropy when the parameter η=2 is selected.
Remark 2.1. From Definition 2.1, when the parameter η=2 is selected, then the C-Ts extropy is valid for h(x)≤1 or h(x)>1.
Proposition 2.3. Assume that X is a continuous RV supported in [a,b], −∞<a<b<∞. Then, from (1.3), (1.4), Definition 2.1 and Remark 2.1, we have
TEx2(X)=TEn2(X)=1+2Ex(X). |
Proof. From (2.2), at η=2, we have
TEx2(X)=12−1(b−a−1−∫ba(1−h(x))2dx)=b−a−1−(b−a−2+∫bah2(x)dx)=1−∫bah2(x)dx=TEn2(X)=1+2Ex(X). |
Definition 2.2. (Shaked and Shanthikumar [13]) Provided that X and Y be RV's with pdf's h and g, cdf's H and G, respectively. In the dispersive order, it is said that X is smaller than Y, symbolized by X≤DISY, if G−1(H(x))−x is increasing in x≥0.
Lemma 2.1. If X≤DISY, then TEx2(X)≤TEx2(Y).
Proof. From Definition 2.1 and Remark 2.1, at η=2, we have
TEx2(X)=1−∫bah2(x)dx=1−∫bah(x)dH(x)=1−∫10h(H−1(u))du. |
If X≤DISY, thus, by (2.2), we have h(H−1(u))≥g(G−1(u)), ∀u∈(0,1). Therefore,
TEx2(X)=1−∫10h(H−1(u))du≤1−∫10g(G−1(u))du=TEx2(Y). |
Based on the independent and identically distributed observations (iid) X1,X2,...,Xn and Y1,Y2,...,Yn. If X≤DISY, then we have
(1) Xi:n≤DISYi:n (see Theorem 3.B.26 in Shaked and Shanthikumar [13]), i=1,2,...,n.
(2) PXn≤DISPYn (see Belzunce et al. [3]).
Where Xi:n and Yi:n, i=1,2,...,n, are the ith order statistics of X1,X2,...,Xn and Y1,Y2,...,Yn, respectively, and PXn and PYn are the nth upper records of X and Y, respectively. Thus, we can conclude with the following results.
Proposition 2.4. If X≤DISY, thus
(1) TEx2(Xi:n)≤TEx2(Yi:n), i=1,2,...,n.
(2) TEx2(PXn)≤TEx2(PYn).
The pdf of the jth order statistics Xj:n in a sample of size n is
hj:n(x)=Hj−1(x)¯Hn−j(x)h(x)B(j,n−j+1), | (2.6) |
where B(j,n−j+1) is the beta function, ¯H(.)=1−H(.) and H(.) is the cumulative distribution function (cdf). In the following example, based on U(a,b) distribution, we will obtain the C-Ts extropy of the jth order statistics Xj:n as follows.
Example 2.3. Provided that X is a continuous RV supported in [a,b], −∞<a<b<∞. Thus, from (2.6), Definition 2.1 and Remark 2.1, the C-Ts extropy of the jth order statistics Xj:n of the U(a,b) distribution is given by
TExη(Xj:n)=1η−1(b−a−1−∫ba(1−hj:n(x))ηdx)=1η−1(b−a−1−∫ba(1−Hj−1(x)¯Hn−j(x)h(x)B(j,n−j+1))ηdx)=1η−1(b−a−1−η∑i=0(ηi)(−1)i(B(j,n−j+1))i(b−a)in∫ba(x−a)ij−i(b−x)in−ijdx)=1η−1(b−a−1−η∑i=0(ηi)(−1)i(b−a)1−iB(ij−i+1,in−ij+1)(B(j,n−j+1))i). |
Based on the jth order statistics Xj:n, we will obtain some significant results of C-Ts extropy when the choice of η=2.
Proposition 2.5. Provided that X is a continuous RV supported in [a,b], −∞<a<b<∞. Then, from (1.3), (1.4), (2.6), Definition 2.1 and Remark 2.1, we have
TEx2(Xj:n)=TEn2(Xj:n)=1+2Ex(Xj:n). |
Proposition 2.6. Let X and Y be two continuous RV's with cdf's H and G, respectively. Moreover, X and Y supports in [a,b1] and [a,b2], respectively, where −∞<a<b1<∞ and −∞<a<b2<∞. Provided that ∫b1adx and ∫b2ady exists, then, for a fixed j (1≤j≤n), X and Y have a common distribution iff TEx2(Xj:n)=TEx2(Yj:n).
Proof. Proof of sufficiency is sufficient. Suppose that TEx2(Xj:n)=TEx2(Yj:n), then, from (2.6), we have
∫b1a(1−Hj−1(x)¯Hn−j(x)h(x)B(j,n−j+1))2dx=∫b2a(1−Gj−1(x)¯Gn−j(x)g(x)B(j,n−j+1))2dx, |
after simplification, we get
∫b1aH2j−2(x)¯H2n−2j(x)h2(x)dx=∫b2aG2j−2(x)¯G2n−2j(x)g2(x)dx, |
which is equivalent to
∫b1aH2j−2(x)¯H2n−2j(x)τX(x)d¯H2(x)=∫b2aG2j−2(x)¯G2n−2j(x)τY(x)d¯G2(x), |
where τX(x)=h(x)¯H(x) and τY(x)=g(x)¯G(x). Setting w=¯H2(x) or w=¯G2(x), thus, we have
∫10(1−√w)2j−2wn−jτX(H−1(1−√w))dw=∫10(1−√w)2j−2wn−jτY(G−1(1−√w))dw. |
Equivalently
∫10(1−√w)2j−2[τX(H−1(1−√w))−τY(G−1(1−√w))]wrdw=0,r=n−j≥0. | (2.7) |
From Stone-Weierstrass Theorem and its corollary (Aliprantis and Burkinshaw [1]): If χ is a continuous function on (0, 1) such that ∫10xnχ(x)dx=0 ∀n≥0, then χ(x)=0, x∈(0,1). Thus, from (1.5), we have τX(H−1(1−√w))=τY(G−1(1−√w)), w∈[0,1]. Put 1−√w=u, then we have H−1(u)=G−1(u), u∈(0,1), and the result follows.
In this section, we will present the maximum C-Ts extropy by the following theorem.
Theorem 3.1. Provided that X is a continuous RV supported in [a,b], −∞<a<b<∞. Thus, from (2.2), X has the maximum C-Ts extropy iff it follows the continuous uniform distribution, where 1≠η>0 if h(x)≤1 and η∈Z+∖{1} if h(x)>1.
Proof. From Definition 2.1, we have
TExη(X)=1η−1(∫ba(1−h(x))dx−∫ba(1−h(x))ηdx), |
subject to
∫bah(x)dx=1. | (3.1) |
We can obtain the maximization of TExη(X), using Lagrange multipliers method as follows:
L(X)=1η−1(∫ba(1−h(x))dx−∫ba(1−h(x))ηdx)+μ(∫bah(x)dx−1). |
Differentiating L(X) with respect to h(x) then equating to zero, we obtain
dL(X)dh(x)=0=1η−1(−1+η(1−h(x))η−1)+μ, |
therefore, we get
h(x)=1−(1η+1−ηημ)1η−1. | (3.2) |
To find the value of μ, we substitute (3.2) in the constrain (3.1), thus
μ=η1−η((1−1b−a)η−1−1η). | (3.3) |
Substituting (3.3) in (3.2), it holds h(x)=1b−a is the pdf of the continuous U(a,b) distribution.
Proposition 3.1. Provided that X is a continuous RV supported in [a,b], −∞<a<b<∞, provided that b−a≥2. Then, from (1.4) and Definition 2.1, we have
(1) TExη(X)≤TEnη(X), if 0<η<2.
(2) TExη(X)≥TEnη(X), if η>2.
Proof. From (1.4) and Definition 2.1, we have
TEnη(X)−TExη(X)=1α−1(2−(b−a)−∫bahη(x)dx+∫ba(1−h(x)ηdx). |
Therefore, the Lagrange function (L(X)) is given by
L(X)=TEnη(X)−TExη(X)+μ(∫bah(x)dx−1). |
Then, the derivative with respect to h(x) is
dL(X)dh(x)=−ηη−1(hη−1(x)+(1−h(x))η−1)+μ, |
thus, we can note the vanishing equation
hη−1(x)+(1−h(x))η−1=k,kisaconstant, |
and the rest of the proof will be in the same manner given in Balakrishnan et al. [2].
Figure 3 shows the comparison between TExη(X) and TEnη(X) according to Proposition 3.1 of uniform and power function distributions.
Theorem 3.2. Provided that X is a continuous RV supported in [a,b], −∞<a<b<∞. Then, from Definition 2.1, The C-Ts extropy is less than or equal to 1.
Proof. We can see that the C-Ts extropy of the continuous uniform distribution increases to 1 as (b−a) increases. From (2.4), assume the function
T(b−a)=T(Z)=Z−1−(Z−1)ηZη−1, |
then, its derivative is given by
T′(Z)=Zη−(Z−1)η−1(η+Z−1)Zη, |
its sign, by mean value theorem, is given by η(Z−1+ε)η−1−η(Z−1)η−1, for some ε∈(0,1). Therefore, we can see that T(Z) increases for η>1 and decreases for 0<η<1. Moreover, as Z tends to ∞, we have the limit of uniform C-Ts extropy as follows:
limZ→+∞TExη(X)=limZ→+∞Z−1η−1(1−(1−1Z)η−1)=limZ→+∞Z−1Z=1. |
From the maximum C-Ts extropy given in Theorem 3.1, C-Ts extropy is less than or equal to 1. Or, we can implement the proof simply by using Bernoulli's inequality, as follows:
TExη(X)=1η−1(b−a−1−∫ba(1−h(x))ηdx)≤1η−1(b−a−1−∫ba(1−ηh(x))dx)≤1η−1(b−a−1−(b−a−η))≤1. |
Inspired by the idea of the discrete Renyi extropy introduced by Liu and Xiao [6], we presented the C-Re extropy in this section. Let X be a continuous RV supported in [a,b], −∞<a<b<∞, having a pdf h(.). It is obvious from the logarithmic function that its domain is (o,∞). Therefore, the C-Re extropy exists only when h(x)≤1 and b−a>1. Otherwise, it will return to a complex result or vanish. Then, the C-Re extropy, 1≠η>0, is given by
RExη(X)=11−η(−(b−a−1)ln(b−a−1)+(b−a−1)ln∫ba(1−h(x))ηdx), | (4.1) |
where h(x)≤1 and b−a>1.
Proposition 4.1. Provided that X is a continuous RV supported in [a,b], −∞<a<b<∞. Then, from (2.1) and (4.1), we have
limη→1RExη(X)=Ex(X). | (4.2) |
Proof. From (4.1), with applying L′Hˆopital′s rule, we get
limη→1RExη(X)=limη→111−η(−(b−a−1)ln(b−a−1)+(b−a−1)ln∫ba(1−h(x))ηdx)=limη→11−1((b−a−1)∫ba(1−h(x))ηln(1−h(x))dx∫ba(1−h(x))ηdx)=−∫ba(1−h(x))ln(1−h(x))dx=Ex(X). |
Example 4.1. Suppose that the continuous RV X has U(a,b) distribution, provided that b−a≠1. Then, the C-Re extropy is given by
RExη(X)=11−η(−(b−a−1)ln(b−a−1)+(b−a−1)ln∫ba(1−h(x))ηdx)=11−η(−(b−a−1)ln(b−a−1)+(b−a−1)ln∫ba(1−1b−a)ηdx)=(b−a−1)lnb−ab−a−1, | (4.3) |
where b−a≠1.
In this subsection, we will present the maximum C-Re extropy by the following theorem.
Theorem 4.1. Provided that X is a continuous RV supported in [a,b], −∞<a<b<∞. Thus, from (4.1), X has the maximum C-Re extropy iff it follows the continuous uniform distribution.
Proof. From (4.1), we have
RExη(X)=11−η(−(b−a−1)ln(b−a−1)+(b−a−1)ln∫ba(1−h(x))ηdx), |
subject to
∫bah(x)dx=1. | (4.4) |
We can obtain the maximization of RExη(X), using Lagrange multipliers method as follows:
L(X)=11−η(−(b−a−1)ln(b−a−1)+(b−a−1)ln∫ba(1−h(x))ηdx)+μ(∫bah(x)dx−1). |
Differentiating L(X) with respect to h(x) then equating to zero, we obtain
dL(X)dh(x)=0=11−η(−η(b−a−1)(1−h(x))η−1∫ba(1−h(x))ηdx)+μ, |
therefore, we get
h(x)=1−(μ(1−η)η(b−a−1)∫ba(1−h(x))ηdx)1η−1. | (4.5) |
To find the value of μ, we substitute (4.5) in the constrain (4.4), thus
μ=η(b−a−1)(1−η)∫ba(1−h(x))ηdx(1−1b−a)η−1. | (4.6) |
Substituting (4.6) in (4.5), it holds h(x)=1b−a is the pdf of the continuous U(a,b) distribution.
The non-parametric estimation is used in many works to estimate the extropy and its related measures. The non-parametric kernel density estimation is a common procedure used in many works of literature as a smoothed estimator, see, for example, Qiu and Jia [5], Noughabi and Jarrahiferiz [10] and Jahanshahi et al. [12]. In this section, we present the empirical estimator of the pdf to estimate the C-Ts extropy using the kernel non-parametric estimator. Let the sequence {Xj,1≤j≤n} be a random sample drawn from a population with pdf h(.). From Definition 2.1, the empirical Tsallis extropy is defined as
TExη(hn)=1η−1(∫ba(1−hn(x))(1−(1−hn(x))η−1)dx)=1η−1(n−1∑j=1∫Xj+1:nXj:n(1−hn(x))(1−(1−hn(x))η−1)dx)=1η−1(n−1∑j=1(Xj+1:n−Xj:n)(1−hn(Xj:n))(1−(1−hn(Xj:n))η−1)), | (5.1) |
where X1:n≤X2:n≤...≤Xn:n is the order statistic of the random sample. Furthermore, hn(.) is the density kernel estimator of h(.) defined by (see, Parzen [8])
hn(x)=1nBn∑i=1kr(x−XiB), |
where kr(x) is the kernel function (we use the Gaussian kernel) and B is the bandwidths. To choose the bandwidths, we use different methods like plug-in selectors (includes rule-of-thumb BRT and direct plug-in BDPI) and cross-validation selectors (includes unbiased cross-validation BUCV and biased cross-validation BBCV). Figure 4 shows the Gaussian kernel density estimator rule-of-thumb bandwidth (BRT−Gaussian) compared with different bandwidths selection. Tables 1 and 2 show the Tsallis extropy estimator with different values of η and sample size n=10,20,30,70,90,100,150,200, and we can conclude the following:
n | Bandwidths with η=0.1 | Bandwidths with η=0.9 | ||||||
BRT | BDPI | BUCV | BBCV | BRT | BDPI | BUCV | BBCV | |
10 | 0.01568894 | 0.01225054 | 0.0129823 | 0.012995 | 0.01529039 | 0.01200904 | 0.01271073 | 0.01272289 |
20 | 0.00648184 | 0.007448753 | 0.006082191 | 0.006076309 | 0.006403521 | 0.00734512 | 0.006013289 | 0.006007541 |
30 | 0.004392641 | 0.004579266 | 0.004121339 | 0.004128011 | 0.004352873 | 0.004536029 | 0.004086354 | 0.004092911 |
70 | 0.003419211 | 0.003454668 | 0.00345291 | 0.003452436 | 0.003409897 | 0.003445159 | 0.00344341 | 0.00344294 |
90 | 0.001635452 | 0.001550102 | 0.001539633 | 0.001540461 | 0.001629029 | 0.001544334 | 0.001533942 | 0.001534764 |
100 | 0.001509869 | 0.001433574 | 0.001422614 | 0.001420712 | 0.001504396 | 0.001428641 | 0.001417757 | 0.001415868 |
150 | 0.001472468 | 0.001406587 | 0.00141964 | 0.00141884 | 0.001469182 | 0.001403589 | 0.001416586 | 0.001415789 |
200 | 0.001071068 | 0.001004348 | 0.001027615 | 0.001027698 | 0.001069151 | 0.001002663 | 0.001025851 | 0.001025934 |
n | Bandwidths with η=3 | Bandwidths with η=6 | ||||||
BRT | BDPI | BUCV | BBCV | BRT | BDPI | BUCV | BBCV | |
10 | 0.01430656 | 0.01140461 | 0.01203303 | 0.01204388 | 0.01304385 | 0.01061012 | 0.01114672 | 0.01115594 |
20 | 0.006203853 | 0.007082083 | 0.005837306 | 0.005831896 | 0.005932838 | 0.006727799 | 0.00559768 | 0.005592718 |
30 | 0.004250744 | 0.004425095 | 0.003996382 | 0.00400265 | 0.004110336 | 0.004272832 | 0.003872394 | 0.00387827 |
70 | 0.003385607 | 0.003420364 | 0.003418641 | 0.003418176 | 0.003351307 | 0.003385354 | 0.003383666 | 0.003383211 |
90 | 0.001612328 | 0.001529326 | 0.001519136 | 0.001519943 | 0.001588865 | 0.001508223 | 0.001498314 | 0.001499099 |
100 | 0.001490156 | 0.001415801 | 0.001405111 | 0.001403256 | 0.001470123 | 0.001397723 | 0.001387306 | 0.001385498 |
150 | 0.001460602 | 0.001395758 | 0.00140861 | 0.001407822 | 0.001448461 | 0.001384673 | 0.001397319 | 0.001396544 |
200 | 0.001064142 | 0.0009982576 | 0.001021239 | 0.001021322 | 0.00105704 | 0.000992009 | 0.001014699 | 0.001014781 |
(1) For fixed η and n increases, Tsallis extropy decreases.
(2) For fixed n and η increases, Tsallis extropy decreases.
(3) The Tsallis extropy under the bandwidths BRT gives a large value than the other bandwidths selections.
In this subsection, we illustrate a dataset that compares sales and consumption across several countries in the pharmaceutical business. From Figures 5 and 6, this study focuses on the OECD countries which contain 8 countries in the pharmaceutical market variables (Antidepressants; Anxiolytics; Drugs used in diabetes; Respiratory system) from 2010 to 2021 (Defined daily dosage per 1000 inhabitants per day), see [7]. Table 3 shows the Tsallis extropy estimator with different values of η and we can conclude the following:
η | Bandwidths | |||
BRT | BDPI | BUCV | BBCV | |
0.1 | 0.0004175204 | 0.0006107105 | 4.314579 ×10−6 | 0.000392944 |
0.9 | 0.0004175065 | 0.0006106806 | 4.314578 ×10−6 | 0.0003929316 |
3 | 0.0004174699 | 0.0006106023 | 4.314574 ×10−6 | 0.0003928992 |
6 | 0.0004174176 | 0.0006104904 | 4.314568 ×10−6 | 0.0003928529 |
9 | 0.000417313 | 0.0006102668 | 4.314557 ×10−6 | 0.0003927603 |
12 | 0.0004171737 | 0.0006099688 | 4.314542 ×10−6 | 0.0003926369 |
(1) When η increases, Tsallis extropy decreases.
(2) The Tsallis extropy under the bandwidths BDPI gives a large value than the other bandwidths selections.
In this part, we study the forecasting time series of Austria pharmaceutical market from 2021 to 2030 for the two variables, anxiolytics and drugs used in diabetes. Then, we obtain the Tsallis extropy estimator of the obtained results. Figures 7 and 8 show the fitted model to the anxiolytics and drugs used in diabetes variables which both fitted to ARIMA(0,1,0) with (AIC = 54.09, BIC = 54.39 and p-value 0.74) and (AIC = 14.13, BIC = 14.44 and p-value 0.505), respectively.
Figure 9 shows the time series and its forecasting of Austria pharmaceutical market from 2021 to 2030 for the two variables Anxiolytics and Drugs used in diabetes. Tables 4 and 5 show the Tsallis extropy estimator of 80 and 95 forecasting interval of anxiolytics and drugs used in diabetes of Austria pharmaceutical market, respectively, and we can conclude the following:
η | Bandwidths (80 forecasting interval) | Bandwidths (95 forecasting interval) | ||||||
BRT | BDPI | BUCV | BBCV | BRT | BDPI | BUCV | BBCV | |
0.1 | [0.01278551, 0.02367084] | [0.0118221, 0.02306017] | [0.01200616, 0.02319374] | [0.01199755, 0.02318694] | [0.01282185, 0.02371729] | [0.01184626, 0.02310411] | [0.01203778, 0.02323823] | [0.01202923, 0.02323139] |
0.9 | [0.01203026, 0.02267522] | [0.01117969, 0.02211642] | [0.01134294, 0.02223877] | [0.01133531, 0.02223254] | [0.01233652, 0.02307425] | [0.01143332, 0.02249436] | [0.01161111, 0.02262128] | [0.01160317, 0.02261481] |
3 | [0.0103122, 0.02031479] | [0.009701415, 0.0198728] | [0.009820118, 0.01996984] | [0.009814586, 0.0199649] | [0.01117408, 0.02149344] | [0.01043706, 0.02099279] | [0.01058318, 0.02110254] | [0.01057667, 0.02109695] |
6 | [0.008393795, 0.01748806] | [0.008018444, 0.01717329] | [0.008092826, 0.01724268] | [0.008089374, 0.01723915] | [0.009757514, 0.01947896] | [0.009207808, 0.0190735] | [0.009317947, 0.01916259] | [0.009313052, 0.01915805] |
9 | [0.006945648, 0.01518132] | [0.006720525, 0.01495867] | [0.00676616, 0.01500796] | [0.006764053, 0.01500546] | [0.008578555, 0.01771483] | [0.008170232, 0.01738691] | [0.008252944, 0.01745914] | [0.008249277, 0.01745546] |
12 | [0.005838708, 0.01328733] | [0.005708993, 0.01313129] | [0.005736063, 0.013166] | [0.005734821, 0.01316424] | [0.007592507, 0.01616612] | [0.007290802, 0.01590135] | [0.007352628, 0.01595982] | [0.007349894, 0.01595684] |
η | Bandwidths (80 forecasting interval) | Bandwidths (95 forecasting interval) | ||||||
BRT | BDPI | BUCV | BBCV | BRT | BDPI | BUCV | BBCV | |
0.1 | [0.01287194, 0.02378289] | [0.01188874, 0.02316626] | [0.0120817, 0.02330113] | [0.01207308, 0.02329425] | [0.01287625, 0.02378862] | [0.01189883, 0.02317169] | [0.01208585, 0.02330662] | [0.01207643, 0.02329974] |
0.9 | [0.01277373, 0.02365185] | [0.01180502, 0.02304195] | [0.01199523, 0.02317536] | [0.01198674, 0.02316856] | [0.01281218, 0.02370307] | [0.01184414, 0.02309053] | [0.01202942, 0.02322451] | [0.01202009, 0.02321768] |
3 | [0.01252066, 0.02331242] | [0.01158896, 0.02271983] | [0.01177214, 0.02284949] | [0.01176396, 0.02284288] | [0.012646, 0.02348046] | [0.01170216, 0.02287929] | [0.01188297, 0.02301081] | [0.01187386, 0.0230041] |
6 | [0.01217064, 0.02283873] | [0.01128939, 0.02227002] | [0.01146296, 0.02239451] | [0.01145521, 0.02238816] | [0.01241357, 0.02316725] | [0.01150326, 0.02258196] | [0.01167784, 0.02271004] | [0.01166905, 0.02270352] |
9 | [0.01183364, 0.02237785] | [0.01100012, 0.02183207] | [0.01116459, 0.02195159] | [0.01115725, 0.0219455] | [0.01218683, 0.02285961] | [0.01130886, 0.02228979] | [0.01147744, 0.02241452] | [0.01146895, 0.02240816] |
12 | [0.01150912, 0.0219294] | [0.01072077, 0.02140563] | [0.01087661, 0.02152038] | [0.01086966, 0.02151453] | [0.01196562, 0.02255743] | [0.01111885, 0.02200266] | [0.01128163, 0.02212413] | [0.01127343, 0.02211794] |
(1) When η increases, Tsallis extropy decreases.
(2) The Tsallis extropy under the bandwidths BRT gives a large value than the other bandwidths selections.
In this consideration, we have discussed the C-Ts and C-Re extropy under the continuous case, and discuss the conditions when the continuous distributions can be valid to apply in C-Ts and C-Re extropy. We have illustrated some properties of the presented models with examples of some distributions like uniform and power function distributions. Besides, our models with the other uncertainty measures and order statistics are compared. Moreover, we have discussed the condition of the maximum C-Ts and C-Re extropy, which both returned to the uniform distribution. A non-parametric estimation has been introduced of the Tsallis extropy and we see that its increases depend on the values of n, η and the selection of the bandwidth. In comparing C-Ts and C-Re extropy with the original version of entropy, we can see that no constraints are held on the pdf of the entropy measures. Moreover, we must have some restrictions on the pdf in C-Ts and C-Re extropy. Furthermore, when the Tsallis entropy parameter η approaches 1, it converges to the classical Shannon entropy. In contrast, the C-Ts extropy converges to the extropy measure when η tends to 1, only at h(x)≤1. The choice of the non-extensive parameter η can significantly impact the behavior and interpretation of the entropy measure; therefore, when η=2, the C-Ts extropy and entropy coincide, which means that the two models have the same performance in evaluating uncertain information. In future work, some relative works of entropy, e.g., Quantum X-entropy in generalized quantum evidence theory (Xiao [16]); On the maximum entropy negation of a complex-valued distribution (Xiao [17]); Evidential fuzzy multicriteria decision making based on belief entropy (Xiao [18]) can be implemented in extropy and its related measures.
The authors declare they have not used Artificial Intelligence (AI) tools in the creation of this article.
This research is supported by researchers supporting project number (RSPD2023R548), King Saud University, Riyadh, Saudi Arabia.
The authors declare no conflict of interest.
[1] | C. Aliprantis, O. Burkinshaw, Principles of real analysis, North Holland: Elsevier, 1990. http://dx.doi.org/10.1016/C2009-0-22273-2 |
[2] |
N. Balakrishnan, F. Buono, M. Longobardi, On Tsallis extropy with an application to pattern recognition, Stat. Probabil. Lett., 180 (2022), 109241. http://dx.doi.org/10.1016/j.spl.2021.109241 doi: 10.1016/j.spl.2021.109241
![]() |
[3] |
F. Belzunce, R. Lillo, J. Ruiz, M. Shaked, Stochastic comparisons of nonhomogeneous processes, Probab. Eng. Inform. Sci., 15 (2001), 199–224. http://dx.doi.org/10.1017/S0269964801152058 doi: 10.1017/S0269964801152058
![]() |
[4] |
T. Jawa, N. Fatima, N. Sayed-Ahmed, R. Aldallal, M. Mohamed, Residual and past discrete Tsallis and Renyi extropy with an application to softmax function, Entropy, 24 (2022), 1732. http://dx.doi.org/10.3390/e24121732 doi: 10.3390/e24121732
![]() |
[5] |
F. Lad, G. Sanfilippo, G. Agro, Extropy: complementary dual of entropy, Stat. Sci., 30 (2015), 40–58. http://dx.doi.org/10.1214/14-STS430 doi: 10.1214/14-STS430
![]() |
[6] | J. Liu, F. Xiao, Renyi extropy, Commun. Stat. Theor. M., 52 (2023), 5836–5847. http://dx.doi.org/10.1080/03610926.2021.2020843 |
[7] | OECD Health Statistics, Pharmaceutical market, OECD, 2023. http://dx.doi.org/10.1787/data-00545-en |
[8] |
E. Parzen, On estimation of a probability density function and mode, Ann. Math. Statist., 33 (1962), 1065–1076. http://dx.doi.org/10.1214/aoms/1177704472 doi: 10.1214/aoms/1177704472
![]() |
[9] |
G. Qiu, The extropy of order statistics and record values, Stat. Probabil. Lett., 120 (2017), 52–60. http://dx.doi.org/10.1016/j.spl.2016.09.016 doi: 10.1016/j.spl.2016.09.016
![]() |
[10] |
G. Qiu, K. Jia, Extropy estimators with applications in testing uniformity, J. Nonparametr. Stat., 30 (2018), 182–196. http://dx.doi.org/10.1080/10485252.2017.1404063 doi: 10.1080/10485252.2017.1404063
![]() |
[11] |
M. Raqab, G. Qiu, On extropy properties of ranked set sampling, Statistics, 53 (2019), 210–226. http://dx.doi.org/10.1080/02331888.2018.1533963 doi: 10.1080/02331888.2018.1533963
![]() |
[12] | A. Renyi, On measures of entropy and information, Proceedings of the Fourth Berkeley Symposium on Mathematical Statistics and Probability, 1961,547–561. |
[13] | M. Shaked, J. George Shanthikumar, Stochastic orders, New York: Springer, 2007. http://dx.doi.org/10.1007/978-0-387-34675-5 |
[14] |
C. Shannon, A mathematical theory of communication, Bell System Technical Journal, 27 (1948), 379–423. http://dx.doi.org/10.1002/j.1538-7305.1948.tb01338.x doi: 10.1002/j.1538-7305.1948.tb01338.x
![]() |
[15] |
C. Tsallis, Possible generalization of Boltzmann-Gibbs statistics, J. Stat. Phys., 52 (1988), 479–487. http://dx.doi.org/10.1007/BF01016429 doi: 10.1007/BF01016429
![]() |
[16] |
F. Xiao, Quantum X-entropy in generalized quantum evidence theory, Inform. Sciences, 643 (2023), 119177. http://dx.doi.org/10.1016/j.ins.2023.119177 doi: 10.1016/j.ins.2023.119177
![]() |
[17] |
F. Xiao, On the maximum entropy negation of a complex-valued distribution, IEEE T. Fuzzy Syst., 29 (2021), 3259–3269. http://dx.doi.org/10.1109/TFUZZ.2020.3016723 doi: 10.1109/TFUZZ.2020.3016723
![]() |
[18] |
F. Xiao, EFMCDM: evidential fuzzy multicriteria decision making based on belief entropy, IEEE T. Fuzzy Syst., 28 (2020), 1477–1491. http://dx.doi.org/10.1109/TFUZZ.2019.2936368 doi: 10.1109/TFUZZ.2019.2936368
![]() |
[19] | Y. Xue, Y. Deng, Tsallis eXtropy, Commun. Stat. Theor. M., 52 (2023), 751–762. http://dx.doi.org/10.1080/03610926.2021.1921804 |
1. | Mohamed Said Mohamed, Haroon M. Barakat, Aned Al Mutairi, Manahil SidAhmed Mustafa, Further properties of Tsallis extropy and some of its related measures, 2023, 8, 2473-6988, 28219, 10.3934/math.20231445 | |
2. | Ramy Abdelhamid Aldallal, Haroon M. Barakat, Mohamed Said Mohamed, Exploring weighted Tsallis extropy: Insights and applications to human health, 2025, 10, 2473-6988, 2191, 10.3934/math.2025102 |
n | Bandwidths with η=0.1 | Bandwidths with η=0.9 | ||||||
BRT | BDPI | BUCV | BBCV | BRT | BDPI | BUCV | BBCV | |
10 | 0.01568894 | 0.01225054 | 0.0129823 | 0.012995 | 0.01529039 | 0.01200904 | 0.01271073 | 0.01272289 |
20 | 0.00648184 | 0.007448753 | 0.006082191 | 0.006076309 | 0.006403521 | 0.00734512 | 0.006013289 | 0.006007541 |
30 | 0.004392641 | 0.004579266 | 0.004121339 | 0.004128011 | 0.004352873 | 0.004536029 | 0.004086354 | 0.004092911 |
70 | 0.003419211 | 0.003454668 | 0.00345291 | 0.003452436 | 0.003409897 | 0.003445159 | 0.00344341 | 0.00344294 |
90 | 0.001635452 | 0.001550102 | 0.001539633 | 0.001540461 | 0.001629029 | 0.001544334 | 0.001533942 | 0.001534764 |
100 | 0.001509869 | 0.001433574 | 0.001422614 | 0.001420712 | 0.001504396 | 0.001428641 | 0.001417757 | 0.001415868 |
150 | 0.001472468 | 0.001406587 | 0.00141964 | 0.00141884 | 0.001469182 | 0.001403589 | 0.001416586 | 0.001415789 |
200 | 0.001071068 | 0.001004348 | 0.001027615 | 0.001027698 | 0.001069151 | 0.001002663 | 0.001025851 | 0.001025934 |
n | Bandwidths with η=3 | Bandwidths with η=6 | ||||||
BRT | BDPI | BUCV | BBCV | BRT | BDPI | BUCV | BBCV | |
10 | 0.01430656 | 0.01140461 | 0.01203303 | 0.01204388 | 0.01304385 | 0.01061012 | 0.01114672 | 0.01115594 |
20 | 0.006203853 | 0.007082083 | 0.005837306 | 0.005831896 | 0.005932838 | 0.006727799 | 0.00559768 | 0.005592718 |
30 | 0.004250744 | 0.004425095 | 0.003996382 | 0.00400265 | 0.004110336 | 0.004272832 | 0.003872394 | 0.00387827 |
70 | 0.003385607 | 0.003420364 | 0.003418641 | 0.003418176 | 0.003351307 | 0.003385354 | 0.003383666 | 0.003383211 |
90 | 0.001612328 | 0.001529326 | 0.001519136 | 0.001519943 | 0.001588865 | 0.001508223 | 0.001498314 | 0.001499099 |
100 | 0.001490156 | 0.001415801 | 0.001405111 | 0.001403256 | 0.001470123 | 0.001397723 | 0.001387306 | 0.001385498 |
150 | 0.001460602 | 0.001395758 | 0.00140861 | 0.001407822 | 0.001448461 | 0.001384673 | 0.001397319 | 0.001396544 |
200 | 0.001064142 | 0.0009982576 | 0.001021239 | 0.001021322 | 0.00105704 | 0.000992009 | 0.001014699 | 0.001014781 |
η | Bandwidths | |||
BRT | BDPI | BUCV | BBCV | |
0.1 | 0.0004175204 | 0.0006107105 | 4.314579 ×10−6 | 0.000392944 |
0.9 | 0.0004175065 | 0.0006106806 | 4.314578 ×10−6 | 0.0003929316 |
3 | 0.0004174699 | 0.0006106023 | 4.314574 ×10−6 | 0.0003928992 |
6 | 0.0004174176 | 0.0006104904 | 4.314568 ×10−6 | 0.0003928529 |
9 | 0.000417313 | 0.0006102668 | 4.314557 ×10−6 | 0.0003927603 |
12 | 0.0004171737 | 0.0006099688 | 4.314542 ×10−6 | 0.0003926369 |
η | Bandwidths (80 forecasting interval) | Bandwidths (95 forecasting interval) | ||||||
BRT | BDPI | BUCV | BBCV | BRT | BDPI | BUCV | BBCV | |
0.1 | [0.01278551, 0.02367084] | [0.0118221, 0.02306017] | [0.01200616, 0.02319374] | [0.01199755, 0.02318694] | [0.01282185, 0.02371729] | [0.01184626, 0.02310411] | [0.01203778, 0.02323823] | [0.01202923, 0.02323139] |
0.9 | [0.01203026, 0.02267522] | [0.01117969, 0.02211642] | [0.01134294, 0.02223877] | [0.01133531, 0.02223254] | [0.01233652, 0.02307425] | [0.01143332, 0.02249436] | [0.01161111, 0.02262128] | [0.01160317, 0.02261481] |
3 | [0.0103122, 0.02031479] | [0.009701415, 0.0198728] | [0.009820118, 0.01996984] | [0.009814586, 0.0199649] | [0.01117408, 0.02149344] | [0.01043706, 0.02099279] | [0.01058318, 0.02110254] | [0.01057667, 0.02109695] |
6 | [0.008393795, 0.01748806] | [0.008018444, 0.01717329] | [0.008092826, 0.01724268] | [0.008089374, 0.01723915] | [0.009757514, 0.01947896] | [0.009207808, 0.0190735] | [0.009317947, 0.01916259] | [0.009313052, 0.01915805] |
9 | [0.006945648, 0.01518132] | [0.006720525, 0.01495867] | [0.00676616, 0.01500796] | [0.006764053, 0.01500546] | [0.008578555, 0.01771483] | [0.008170232, 0.01738691] | [0.008252944, 0.01745914] | [0.008249277, 0.01745546] |
12 | [0.005838708, 0.01328733] | [0.005708993, 0.01313129] | [0.005736063, 0.013166] | [0.005734821, 0.01316424] | [0.007592507, 0.01616612] | [0.007290802, 0.01590135] | [0.007352628, 0.01595982] | [0.007349894, 0.01595684] |
η | Bandwidths (80 forecasting interval) | Bandwidths (95 forecasting interval) | ||||||
BRT | BDPI | BUCV | BBCV | BRT | BDPI | BUCV | BBCV | |
0.1 | [0.01287194, 0.02378289] | [0.01188874, 0.02316626] | [0.0120817, 0.02330113] | [0.01207308, 0.02329425] | [0.01287625, 0.02378862] | [0.01189883, 0.02317169] | [0.01208585, 0.02330662] | [0.01207643, 0.02329974] |
0.9 | [0.01277373, 0.02365185] | [0.01180502, 0.02304195] | [0.01199523, 0.02317536] | [0.01198674, 0.02316856] | [0.01281218, 0.02370307] | [0.01184414, 0.02309053] | [0.01202942, 0.02322451] | [0.01202009, 0.02321768] |
3 | [0.01252066, 0.02331242] | [0.01158896, 0.02271983] | [0.01177214, 0.02284949] | [0.01176396, 0.02284288] | [0.012646, 0.02348046] | [0.01170216, 0.02287929] | [0.01188297, 0.02301081] | [0.01187386, 0.0230041] |
6 | [0.01217064, 0.02283873] | [0.01128939, 0.02227002] | [0.01146296, 0.02239451] | [0.01145521, 0.02238816] | [0.01241357, 0.02316725] | [0.01150326, 0.02258196] | [0.01167784, 0.02271004] | [0.01166905, 0.02270352] |
9 | [0.01183364, 0.02237785] | [0.01100012, 0.02183207] | [0.01116459, 0.02195159] | [0.01115725, 0.0219455] | [0.01218683, 0.02285961] | [0.01130886, 0.02228979] | [0.01147744, 0.02241452] | [0.01146895, 0.02240816] |
12 | [0.01150912, 0.0219294] | [0.01072077, 0.02140563] | [0.01087661, 0.02152038] | [0.01086966, 0.02151453] | [0.01196562, 0.02255743] | [0.01111885, 0.02200266] | [0.01128163, 0.02212413] | [0.01127343, 0.02211794] |
n | Bandwidths with η=0.1 | Bandwidths with η=0.9 | ||||||
BRT | BDPI | BUCV | BBCV | BRT | BDPI | BUCV | BBCV | |
10 | 0.01568894 | 0.01225054 | 0.0129823 | 0.012995 | 0.01529039 | 0.01200904 | 0.01271073 | 0.01272289 |
20 | 0.00648184 | 0.007448753 | 0.006082191 | 0.006076309 | 0.006403521 | 0.00734512 | 0.006013289 | 0.006007541 |
30 | 0.004392641 | 0.004579266 | 0.004121339 | 0.004128011 | 0.004352873 | 0.004536029 | 0.004086354 | 0.004092911 |
70 | 0.003419211 | 0.003454668 | 0.00345291 | 0.003452436 | 0.003409897 | 0.003445159 | 0.00344341 | 0.00344294 |
90 | 0.001635452 | 0.001550102 | 0.001539633 | 0.001540461 | 0.001629029 | 0.001544334 | 0.001533942 | 0.001534764 |
100 | 0.001509869 | 0.001433574 | 0.001422614 | 0.001420712 | 0.001504396 | 0.001428641 | 0.001417757 | 0.001415868 |
150 | 0.001472468 | 0.001406587 | 0.00141964 | 0.00141884 | 0.001469182 | 0.001403589 | 0.001416586 | 0.001415789 |
200 | 0.001071068 | 0.001004348 | 0.001027615 | 0.001027698 | 0.001069151 | 0.001002663 | 0.001025851 | 0.001025934 |
n | Bandwidths with η=3 | Bandwidths with η=6 | ||||||
BRT | BDPI | BUCV | BBCV | BRT | BDPI | BUCV | BBCV | |
10 | 0.01430656 | 0.01140461 | 0.01203303 | 0.01204388 | 0.01304385 | 0.01061012 | 0.01114672 | 0.01115594 |
20 | 0.006203853 | 0.007082083 | 0.005837306 | 0.005831896 | 0.005932838 | 0.006727799 | 0.00559768 | 0.005592718 |
30 | 0.004250744 | 0.004425095 | 0.003996382 | 0.00400265 | 0.004110336 | 0.004272832 | 0.003872394 | 0.00387827 |
70 | 0.003385607 | 0.003420364 | 0.003418641 | 0.003418176 | 0.003351307 | 0.003385354 | 0.003383666 | 0.003383211 |
90 | 0.001612328 | 0.001529326 | 0.001519136 | 0.001519943 | 0.001588865 | 0.001508223 | 0.001498314 | 0.001499099 |
100 | 0.001490156 | 0.001415801 | 0.001405111 | 0.001403256 | 0.001470123 | 0.001397723 | 0.001387306 | 0.001385498 |
150 | 0.001460602 | 0.001395758 | 0.00140861 | 0.001407822 | 0.001448461 | 0.001384673 | 0.001397319 | 0.001396544 |
200 | 0.001064142 | 0.0009982576 | 0.001021239 | 0.001021322 | 0.00105704 | 0.000992009 | 0.001014699 | 0.001014781 |
η | Bandwidths | |||
BRT | BDPI | BUCV | BBCV | |
0.1 | 0.0004175204 | 0.0006107105 | 4.314579 ×10−6 | 0.000392944 |
0.9 | 0.0004175065 | 0.0006106806 | 4.314578 ×10−6 | 0.0003929316 |
3 | 0.0004174699 | 0.0006106023 | 4.314574 ×10−6 | 0.0003928992 |
6 | 0.0004174176 | 0.0006104904 | 4.314568 ×10−6 | 0.0003928529 |
9 | 0.000417313 | 0.0006102668 | 4.314557 ×10−6 | 0.0003927603 |
12 | 0.0004171737 | 0.0006099688 | 4.314542 ×10−6 | 0.0003926369 |
η | Bandwidths (80 forecasting interval) | Bandwidths (95 forecasting interval) | ||||||
BRT | BDPI | BUCV | BBCV | BRT | BDPI | BUCV | BBCV | |
0.1 | [0.01278551, 0.02367084] | [0.0118221, 0.02306017] | [0.01200616, 0.02319374] | [0.01199755, 0.02318694] | [0.01282185, 0.02371729] | [0.01184626, 0.02310411] | [0.01203778, 0.02323823] | [0.01202923, 0.02323139] |
0.9 | [0.01203026, 0.02267522] | [0.01117969, 0.02211642] | [0.01134294, 0.02223877] | [0.01133531, 0.02223254] | [0.01233652, 0.02307425] | [0.01143332, 0.02249436] | [0.01161111, 0.02262128] | [0.01160317, 0.02261481] |
3 | [0.0103122, 0.02031479] | [0.009701415, 0.0198728] | [0.009820118, 0.01996984] | [0.009814586, 0.0199649] | [0.01117408, 0.02149344] | [0.01043706, 0.02099279] | [0.01058318, 0.02110254] | [0.01057667, 0.02109695] |
6 | [0.008393795, 0.01748806] | [0.008018444, 0.01717329] | [0.008092826, 0.01724268] | [0.008089374, 0.01723915] | [0.009757514, 0.01947896] | [0.009207808, 0.0190735] | [0.009317947, 0.01916259] | [0.009313052, 0.01915805] |
9 | [0.006945648, 0.01518132] | [0.006720525, 0.01495867] | [0.00676616, 0.01500796] | [0.006764053, 0.01500546] | [0.008578555, 0.01771483] | [0.008170232, 0.01738691] | [0.008252944, 0.01745914] | [0.008249277, 0.01745546] |
12 | [0.005838708, 0.01328733] | [0.005708993, 0.01313129] | [0.005736063, 0.013166] | [0.005734821, 0.01316424] | [0.007592507, 0.01616612] | [0.007290802, 0.01590135] | [0.007352628, 0.01595982] | [0.007349894, 0.01595684] |
η | Bandwidths (80 forecasting interval) | Bandwidths (95 forecasting interval) | ||||||
BRT | BDPI | BUCV | BBCV | BRT | BDPI | BUCV | BBCV | |
0.1 | [0.01287194, 0.02378289] | [0.01188874, 0.02316626] | [0.0120817, 0.02330113] | [0.01207308, 0.02329425] | [0.01287625, 0.02378862] | [0.01189883, 0.02317169] | [0.01208585, 0.02330662] | [0.01207643, 0.02329974] |
0.9 | [0.01277373, 0.02365185] | [0.01180502, 0.02304195] | [0.01199523, 0.02317536] | [0.01198674, 0.02316856] | [0.01281218, 0.02370307] | [0.01184414, 0.02309053] | [0.01202942, 0.02322451] | [0.01202009, 0.02321768] |
3 | [0.01252066, 0.02331242] | [0.01158896, 0.02271983] | [0.01177214, 0.02284949] | [0.01176396, 0.02284288] | [0.012646, 0.02348046] | [0.01170216, 0.02287929] | [0.01188297, 0.02301081] | [0.01187386, 0.0230041] |
6 | [0.01217064, 0.02283873] | [0.01128939, 0.02227002] | [0.01146296, 0.02239451] | [0.01145521, 0.02238816] | [0.01241357, 0.02316725] | [0.01150326, 0.02258196] | [0.01167784, 0.02271004] | [0.01166905, 0.02270352] |
9 | [0.01183364, 0.02237785] | [0.01100012, 0.02183207] | [0.01116459, 0.02195159] | [0.01115725, 0.0219455] | [0.01218683, 0.02285961] | [0.01130886, 0.02228979] | [0.01147744, 0.02241452] | [0.01146895, 0.02240816] |
12 | [0.01150912, 0.0219294] | [0.01072077, 0.02140563] | [0.01087661, 0.02152038] | [0.01086966, 0.02151453] | [0.01196562, 0.02255743] | [0.01111885, 0.02200266] | [0.01128163, 0.02212413] | [0.01127343, 0.02211794] |