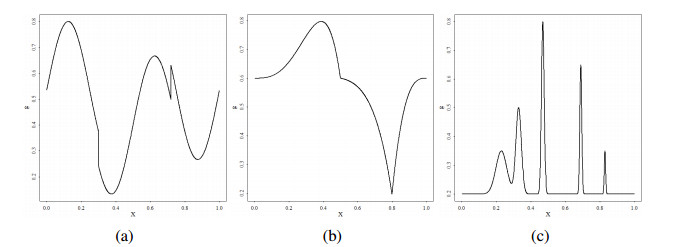
In real Hilbert spaces, for the purpose of trying to deal with the pseudo-monotone variational inequalities problem, we present a new extrapolation projection contraction algorithm based on the golden ratio in this study. Unlike ordinary inertial extrapolation, the algorithms are constructed based on a convex combined structure about the entire iterative trajectory. Extrapolation parameter ψ is selected in a more relaxed range instead of only taking the golden ratio ϕ=√5+12 as the upper bound. Second, we propose an alternating extrapolation projection contraction algorithm to better increase the convergence effects of the extrapolation projection contraction algorithm based on the golden ratio. All our algorithms employ non-constantly decreasing adaptive step-sizes. The weak convergence results of the two algorithms are established for the pseudo-monotone variational inequalities. Additionally, the R-linear convergence results are investigated for strongly pseudo-monotone variational inequalities. Finally, we show the validity and superiority of the suggested methods with several numerical experiments. The numerical results show that alternating extrapolation does have obvious acceleration effect in practical application compared with no alternating extrapolation. Thus, the obvious effect of relaxing the selection range of parameter ψ on our two algorithms is clearly demonstrated.
Citation: Cuijie Zhang, Zhaoyang Chu. New extrapolation projection contraction algorithms based on the golden ratio for pseudo-monotone variational inequalities[J]. AIMS Mathematics, 2023, 8(10): 23291-23312. doi: 10.3934/math.20231184
[1] | Yanyan Gao, Yangjiang Wei . Group codes over symmetric groups. AIMS Mathematics, 2023, 8(9): 19842-19856. doi: 10.3934/math.20231011 |
[2] | Chunqiang Cui, Jin Chen, Shixun Lin . Metric and strong metric dimension in TI-power graphs of finite groups. AIMS Mathematics, 2025, 10(1): 705-720. doi: 10.3934/math.2025032 |
[3] | Yunpeng Bai, Yuanlin Li, Jiangtao Peng . Unit groups of finite group algebras of Abelian groups of order 17 to 20. AIMS Mathematics, 2021, 6(7): 7305-7317. doi: 10.3934/math.2021428 |
[4] | Huani Li, Ruiqin Fu, Xuanlong Ma . Forbidden subgraphs in reduced power graphs of finite groups. AIMS Mathematics, 2021, 6(5): 5410-5420. doi: 10.3934/math.2021319 |
[5] | Yingyu Luo, Yu Wang . Supercommuting maps on unital algebras with idempotents. AIMS Mathematics, 2024, 9(9): 24636-24653. doi: 10.3934/math.20241200 |
[6] | Guoqing Wang . A generalization of Kruyswijk-Olson theorem on Davenport constant in commutative semigroups. AIMS Mathematics, 2020, 5(4): 2992-3001. doi: 10.3934/math.2020193 |
[7] | Yongli Zhang, Jiaxin Shen . Flag-transitive non-symmetric 2-designs with λ prime and exceptional groups of Lie type. AIMS Mathematics, 2024, 9(9): 25636-25645. doi: 10.3934/math.20241252 |
[8] | Hatice Gülsün Akay . (Co-)fibration of generalized crossed modules. AIMS Mathematics, 2024, 9(11): 32782-32796. doi: 10.3934/math.20241568 |
[9] | Xiaofei Cao, Yuyue Huang, Xue Hua, Tingyu Zhao, Sanzhang Xu . Matrix inverses along the core parts of three matrix decompositions. AIMS Mathematics, 2023, 8(12): 30194-30208. doi: 10.3934/math.20231543 |
[10] | Hui Yan, Hongxing Wang, Kezheng Zuo, Yang Chen . Further characterizations of the weak group inverse of matrices and the weak group matrix. AIMS Mathematics, 2021, 6(9): 9322-9341. doi: 10.3934/math.2021542 |
In real Hilbert spaces, for the purpose of trying to deal with the pseudo-monotone variational inequalities problem, we present a new extrapolation projection contraction algorithm based on the golden ratio in this study. Unlike ordinary inertial extrapolation, the algorithms are constructed based on a convex combined structure about the entire iterative trajectory. Extrapolation parameter ψ is selected in a more relaxed range instead of only taking the golden ratio ϕ=√5+12 as the upper bound. Second, we propose an alternating extrapolation projection contraction algorithm to better increase the convergence effects of the extrapolation projection contraction algorithm based on the golden ratio. All our algorithms employ non-constantly decreasing adaptive step-sizes. The weak convergence results of the two algorithms are established for the pseudo-monotone variational inequalities. Additionally, the R-linear convergence results are investigated for strongly pseudo-monotone variational inequalities. Finally, we show the validity and superiority of the suggested methods with several numerical experiments. The numerical results show that alternating extrapolation does have obvious acceleration effect in practical application compared with no alternating extrapolation. Thus, the obvious effect of relaxing the selection range of parameter ψ on our two algorithms is clearly demonstrated.
This paper considers the following heteroscedastic model:
Yi=f(Xi)Ui+g(Xi),i∈{1,⋯,n}. | (1.1) |
In this equation, g(x) is a known mean function, and the variance function r(x)(r(x):=f2(x)) is unknown. Both the mean function g(x) and variance function r(x) are defined on [0,1]. The random variables U1,…,Un are independent and identically distributed (i.i.d.) with E[Ui]=0 and V[Ui]=1. Furthermore, the random variable Xi is independent of Ui for any i∈{1,⋯,n}. The purpose of this paper is to estimate the mth derivative functions r(m)(x)(m∈N) from the observed data (X1,Y1),⋯,(Xn,Yn) by a wavelet method.
Heteroscedastic models are widely used in economics, engineering, biology, physical sciences and so on; see Box [1], Carroll and Ruppert [2], Härdle and Tsybakov [3], Fan and Yao [4], Quevedo and Vining [5] and Amerise [6]. For the above estimation model (1.1), the most popular method is the kernel method. Many important and interesting results of kernel estimators have been obtained by Wang et al. [7], Kulik and Wichelhaus [8] and Shen et al. [9]. However, the optimal bandwidth parameter of the kernel estimator is not easily obtained in some cases, especially when the function has some sharp spikes. Because of the good local properties in both time and frequency domains, the wavelet method has been widely used in nonparametric estimation problems; see Donoho and Johnstone [10], Cai [11], Nason et al. [12], Cai and Zhou [13], Abry and Didier [14] and Li and Zhang [15]. For the estimation problem (1.1), Kulik and Raimondo [16] studied the adaptive properties of warped wavelet nonlinear approximations over a wide range of Besov scales. Zhou et al. [17] developed wavelet estimators for detecting and estimating jumps and cusps in the mean function. Palanisamy and Ravichandran [18] proposed a data-driven estimator by applying wavelet thresholding along with the technique of sparse representation. The asymptotic normality for wavelet estimators of variance function under α−mixing condition was obtained by Ding and Chen [19].
In this paper, we focus on nonparametric estimation of the derivative function r(m)(x) of the variance function r(x). It is well known that derivative estimation plays an important and useful role in many practical applications (Woltring [20], Zhou and Wolfe, [21], Chacón and Duong [22], Wei et al.[23]). For the estimation model (1.1), a linear wavelet estimator and an adaptive nonlinear wavelet estimator for the derivative function r(m)(x) are constructed. Moreover, the convergence rates over L˜p(1≤˜p<∞) risk of two wavelet estimators are proved in Besov space Bsp,q(R) with some mild conditions. Finally, numerical experiments are carried out, where an automatic selection method is used to obtain the best parameters of two wavelet estimators. According to the simulation study, both wavelet estimators can efficiently estimate the derivative function. Furthermore, the nonlinear wavelet estimator shows better performance than the linear estimator.
This paper considers wavelet estimations of a derivative function in Besov space. Now, we first introduce some basic concepts of wavelets. Let ϕ be an orthonormal scaling function, and the corresponding wavelet function is denoted by ψ. It is well known that {ϕτ,k:=2τ/2ϕ(2τx−k),ψj,k:=2j/2ψ(2jx−k),j≥τ,k∈Z} forms an orthonormal basis of L2(R). This paper uses the Daubechies wavelet, which has a compactly support. Then, for any integer j∗, a function h(x)∈L2([0,1]) can be expanded into a wavelet series as
h(x)=∑k∈Λj∗αj∗,kϕj∗,k(x)+∞∑j=j∗∑k∈Λjβj,kψj,k(x),x∈[0,1]. | (1.2) |
In this equation, Λj={0,1,…,2j−1}, αj∗,k=⟨h,ϕj∗,k⟩[0,1] and βj,k=⟨h,ψj,k⟩[0,1].
Lemma 1.1. Let a scaling function ϕ be t-regular (i.e., ϕ∈Ct and |Dαϕ(x)|≤c(1+|x|2)−l for each l∈Z and α=0,1,…,t). If {αk}∈lp and 1≤p≤∞, there exist c2≥c1>0 such that
c12j(12−1p)‖(αk)‖p≤‖∑k∈Λjαk2j2ϕ(2jx−k)‖p≤c22j(12−1p)‖(αk)‖p. |
Besov spaces contain many classical function spaces, such as the well known Sobolev and Hölder spaces. The following lemma gives an important equivalent definition of a Besov space. More details about wavelets and Besov spaces can be found in Meyer [24] and Härdle et al. [25].
Lemma 1.2. Let ϕ be t-regular and h∈Lp([0,1]). Then, for p,q∈[1,∞) and 0<s<t, the following assertions are equivalent:
(i) h∈Bsp,q([0,1]);
(ii) {2js‖h−Pjh‖p}∈lq;
(iii) {2j(s−1p+12)‖βj,k‖p}∈lq.
The Besov norm of h can be defined by
‖h‖Bsp,q=‖(ατ,k)‖p+‖(2j(s−1p+12)‖βj,k‖p)j≥τ‖q, |
where ‖βj,k‖pp=∑k∈Λj|βj,k|p.
In this section, we will construct our wavelet estimators, and give the main theorem of this paper. The main theorem shows the convergence rates of wavelet estimators under some mild assumptions. Now, we first give the technical assumptions of the estimation model (1.1) in the following.
A1: The variance function r:[0,1]→R is bounded.
A2: For any i∈{0,…,m−1}, variance function r satisfies r(i)(0)=r(i)(1)=0.
A3: The mean function g:[0,1]→R is bounded and known.
A4: The random variable X satisfies X∼U([0,1]).
A5: The random variable U has a moment of order 2˜p(˜p≥1).
In the above assumptions, A1 and A3 are conventional conditions for nonparametric estimations. The condition A2 is used to prove the unbiasedness of the following wavelet estimators. In addition, A4 and A5 are technique assumptions, which will be used in Lemmas 4.3 and 4.5.
According to the model (1.1), our linear wavelet estimator is constructed by
ˆrlinn(x):=∑k∈Λj∗ˆαj∗,kϕj∗,k(x). | (2.1) |
In this definition, the scale parameter j∗ will be given in the following main theorem, and
ˆαj,k:=1nn∑i=1Y2i(−1)mϕ(m)j,k(Xi)−∫10g2(x)(−1)mϕ(m)j,k(x)dx. | (2.2) |
More importantly, it should be pointed out that this linear wavelet estimator is an unbiased estimator of the derivative function r(m)(x) by Lemma 4.1 and the properties of wavelets.
On the other hand, a nonlinear wavelet estimator is defined by
ˆrnonn(x):=∑k∈Λj∗ˆαj∗,kϕj∗,k(x)+j1∑j=j∗ˆβj,kI{|ˆβj,k|≥κtn}ψj,k(x). | (2.3) |
In this equation, IA denotes the indicator function over an event A, tn=2mj√lnn/n,
ˆβj,k:=1nn∑i=1(Y2i(−1)mψ(m)j,k(Xi)−wj,k)I{|Y2i(−1)mψ(m)j,k(Xi)−wj,k|≤ρn}, | (2.4) |
ρn=2mj√n/lnn, and wj,k=∫10g2(x)(−1)mψ(m)j,k(x)dx. The positive integer j∗ and j1 will also be given in our main theorem, and the constant κ will be chosen in Lemma 4.5. In addition, we adopt the following symbol: x+:=max{x,0}. A≲ denotes A\leq cB for some constant c > 0 ; A\gtrsim B means B\lesssim A ; A\thicksim B stands for both A\lesssim B and B\lesssim A .
In this position, the convergence rates of two wavelet estimators are given in the following main theorem.
Main theorem For the estimation model (1.1) with the assumptions A1-A5, r^{(m)}(x)\in B_{p, q}^s({{[0, 1]}}) (p, q \in \left[1, \infty\right) , s > 0) and 1 \le \tilde{p} < \infty , if \{p > \tilde{p} \ge 1, s > 0\} or \{1 \leq p \leq \tilde{p}, s > 1/p\} .
(a) the linear wavelet estimator \hat r_n^{lin}(x) with s' = s-({\frac{1}{p}-\frac{1}{\tilde{p}}})_+ and {2^{{j_*}}}\sim{n^{\frac{1}{{2s' + 2m+1}}}} satisfies
\begin{eqnarray} {\rm{E}}\left[\left \| \hat r_n^{lin}(x)-r^{(m)}(x) \right \|^{\tilde{p}}_{\tilde{p}}\right]\lesssim {n^{-\frac{{\tilde{p}s'}}{{2s' + 2m+1}}}}. \end{eqnarray} | (2.5) |
(b) the nonlinear wavelet estimator \hat r_n^{non}(x) with {2^{{j_*}}}\sim{n^{\frac{1}{{2t+2m+1}}}} \left(t > s\right) and {2^{{j_1}}}\sim \left(\frac{n}{{\ln n}}\right)^{\frac{1}{2m+1}} satisfies
\begin{eqnarray} {\rm{E}}\left[\left \| \hat r_n^{non}(x)-r^{(m)}(x) \right \|^{\tilde{p}}_{\tilde{p}}\right]\lesssim (\ln n)^{\tilde{p}-1} \left( \frac{\ln n}{n}\right)^{\tilde{p} \delta}, \end{eqnarray} | (2.6) |
where
\begin{eqnarray*} \delta = min\left\lbrace \frac{s}{2s+2m+1}, \frac{s-1/p+1 /\tilde{p}}{2(s-1 /p)+2m+1} \right\rbrace = \begin{cases} \frac{s}{2s+2m+1} & p > \frac{\tilde{p}(2m+1)}{2s+2m+1} \\ \frac{s-1/p+1 /\tilde{p}}{2(s-1 /p)+2m+1} & p \leq \frac{\tilde{p}(2m+1)}{2s+2m+1}. \end{cases} \end{eqnarray*} |
Remark 1. Note that n^{-\frac{s\tilde{p}}{2s+1}} \; (n^{-\frac{(s-1/p+1 /\tilde{p})\tilde{p}}{2(s-1 /p)+1}}) is the optimal convergence rate over L^{\tilde{p}} (1\leq \tilde{p} < +\infty) risk for nonparametric wavelet estimations (Donoho et al. [26]). The linear wavelet estimator can obtain the optimal convergence rate when p > \tilde{p}\ge1 and m = 0 .
Remark 2. When m = 0 , this derivative estimation problem reduces to the classical variance function estimation. Then, the convergence rates of the nonlinear wavelet estimator are same as the optimal convergence rates of nonparametric wavelet estimation up to a \ln n factor in all cases.
Remark 3. According to main theorem (a) and the definition of the linear wavelet estimator, it is easy to see that the construction of the linear wavelet estimator depends on the smooth parameter s of the unknown derivative function r^{(m)}(x) , which means that the linear estimator is not adaptive. Compared with the linear estimator, the nonlinear wavelet estimator only depends on the observed data and the sample size. Hence, the nonlinear estimator is adaptive. More importantly, the nonlinear wavelet estimator has a better convergence rate than the linear estimator in the case of p\leq\tilde{p} .
In order to illustrate the empirical performance of the proposed estimators, we produce a numerical illustration using an adaptive selection method, which is used to obtain the best parameters of the wavelet estimators. For the problem (1.1), we choose three common functions, HeaviSine , Corner and Spikes , as the mean function g(x) ; see Figure 1. Those functions are usually used in wavelet literature. On the other hand, we choose the function f(x) by f_{1}(x) = 3(4x-2)^{2} e^{-(4x-2)^{2}} , f_{2}(x) = sin(2\pi sin\pi x) and f_{3}(x) = -(2x-1)^{2}+1 , respectively. In addition, we assume that the random variable U satisfies U\sim N[0, 1] . The aim of this paper is to estimate the derivative function r^{(m)}(x) of the variance function r(x) (r = f^{2}) by the observed data (X_{1}, Y_{1}), \ldots, (X _{n}, Y_{n}) . In this section, we adopt r_{1}(x) = [f_{1}(x)]^{2} , r_{2}(x) = [f_{2}(x)]^{2} and r_{3}(x) = [f_{3}(x)]^{2} . For the sake of simplicity, our simulation study focuses on the derivative function r'(x)(m = 1) and r(x)(m = 0) by the observed data (X_{1}, Y_{1}), \ldots, (X _{n}, Y_{n}) \; (n = 4096) . Furthermore, we use the mean square error ( MSE\; (\hat r(x), r(x)) = \frac{1}{n}\sum\limits_{i = 1}^{n}(\hat r(X_{i})-r(X_{i}))^{2} ) and the average magnitude of error ( AME\; (\hat r(x), r(x)) = \frac{1}{n}\sum\limits_{i = 1}^{n}|\hat r(X_{i})-r(X_{i})| ) to evaluate the performances of the wavelet estimators separately.
For the linear and nonlinear wavelet estimators, the scale parameter j_{*} and threshold value \lambda\; (\lambda = \kappa t_{n}) play important roles in the function estimation problem. In order to obtain the optimal scale parameter and threshold value of wavelet estimators, this section uses the two-fold cross validation (2FCV) approach (Nason [27], Navarro and Saumard [28]). During the first example of simulation study, we choose HeaviSine as the mean function g(x) , and f_{1}(x) = 3(4x-2)^{2} e^{-(4x-2)^{2}} . The estimation results of two wavelet estimators are presented by Figure 2. For the optimal scale parameter j_{*} of the linear wavelet estimator, we built a collection of j_{*} and j_{*} = 1, \ldots, log2(n)-1 . The best parameter j_{*} is selected by minimizing a 2FCV criterion denoted by 2FCV (j_{*}) ; see Figure 2(a). According to Figure 2(a), it is easy to see that the 2FCV (j_{*}) and MSE both can get the minimum value when j_{*} = 4 . For the nonlinear wavelet estimator, the best threshold value \lambda is also obtained by the 2FCV (\lambda) criterion in Figure 2(b). Meanwhile, the parameter j_{*} is same as the linear estimator, and the parameter j_{1} is chosen as the maximum scale parameter log2(n)-1 . From Figure 2(c) and 2(d), the linear and nonlinear wavelet estimators both can get a good performance with the best scale parameter and threshold value. More importantly, the nonlinear wavelet estimator shows better performance than the linear estimator.
In the following simulation study, more numerical experiments are presented to sufficiently verify the performance of the wavelet method. According to Figures 3–10, the wavelet estimators both can obtain good performances in different cases. Especially, the nonlinear wavelet estimator gets better estimation results than the linear estimator. Also, the MSE and AME of the wavelet estimators in all examples are provided by Table 1. Meanwhile, it is easy to see from Table 1 that the nonlinear wavelet estimators can have better performance than the linear estimators.
HeaviSine | Corner | Spikes | |||||||
r_{1} | r_{2} | r_{3} | r_{1} | r_{2} | r_{3} | r_{1} | r_{2} | r_{3} | |
MSE(\hat r^{lin}, r) | 0.0184 | 0.0073 | 0.0071 | 0.0189 | 0.0075 | 0.0064 | 0.0189 | 0.0069 | 0.0052 |
MSE(\hat r^{non}, r) | 0.0048 | 0.0068 | 0.0064 | 0.0044 | 0.0070 | 0.0057 | 0.0042 | 0.0061 | 0.0046 |
MSE(\hat r'^{lin}, r') | 0.7755 | 0.0547 | 0.0676 | 0.7767 | 0.1155 | 0.0737 | 0.7360 | 0.2566 | 0.0655 |
MSE(\hat r'^{non}, r') | 0.2319 | 0.0573 | 0.0560 | 0.2204 | 0.0644 | 0.0616 | 0.2406 | 0.2868 | 0.0539 |
AME(\hat r^{lin}, r) | 0.0935 | 0.0653 | 0.0652 | 0.0973 | 0.0667 | 0.0615 | 0.0964 | 0.0621 | 0.0550 |
AME(\hat r^{non}, r) | 0.0506 | 0.0641 | 0.0619 | 0.0486 | 0.0649 | 0.0583 | 0.0430 | 0.0595 | 0.0518 |
AME(\hat r'^{lin}, r') | 0.6911 | 0.1876 | 0.2348 | 0.7021 | 0.2686 | 0.2451 | 0.6605 | 0.4102 | 0.2320 |
AME(\hat r'^{non}, r') | 0.3595 | 0.1862 | 0.2125 | 0.3450 | 0.2020 | 0.2229 | 0.3696 | 0.4198 | 0.2095 |
Now, we provide some lemmas for the proof of the main Theorem.
Lemma 4.1. For the model (1.1) with A2 and A4,
\begin{gather} {\rm{E}}[{{{\hat\alpha}_{j, k}}}] = {\alpha_{j, k}} , \end{gather} | (4.1) |
\begin{gather} {\rm{E}}\left[ \frac{1}{n}\sum\limits_{i = 1}^n \left({Y_i^{2}{(-1)^{m}{\psi^{(m)}_{j, k}}(X_i)}}-w_{j, k}\right)\right] = \beta _{j, k} . \end{gather} | (4.2) |
Proof. According to the definition of {\hat \alpha _{j, k}} ,
\begin{align*} {\rm{E}}[{{{\hat\alpha}_{j, k}}}] & = {\rm{E}}\left[{ \frac{1}{n}\sum\limits_{i = 1}^n {Y_i^{2}{(-1)^{m}{\phi^{(m)}_{j, k}}(X_i)}}-\int_{0}^{1} {{g^2}(x){(-1)^{m}{\phi^{(m)} _{j, k}}(x)}dx} }\right]\\ & = \frac{1}{n}\sum\limits_{i = 1}^n{\rm{E}}\left[{Y_i^{2}{(-1)^{m}{\phi^{(m)}_{j, k}}(X_i)}}\right]- \int_{0}^{1} {{g^2}(x){(-1)^{m}{\phi^{(m)} _{j, k}}(x)}dx}\\ & = {\rm{E}}\left[{Y_1^{2}{(-1)^{m}{\phi^{(m)}_{j, k}}(X_1)}}\right] -\int_{0}^{1} {{g^2}(x){(-1)^{m}{\phi^{(m)} _{j, k}}(x)}dx}\\ & = {\rm{E}}\left[{r({{X_1}})U_1^{2}(-1)^{m}{\phi^{(m)}_{j, k}}({{X_1}})}\right] + 2{\rm{E}}[{f({{X_1}}){U_1}g({{X_1}}){(-1)^{m}{\phi^{(m)}_{j, k}}(X_1)}}] \\ &+ {\rm{E}}\left[ {{g^2({{X_1}})}{(-1)^{m}{\phi^{(m)}_{j, k}}(X_1)}}\right]- \int_{0}^{1} {{g^2}(x){(-1)^{m}{\phi^{(m)} _{j, k}}(x)}dx}. \end{align*} |
Then, it follows from A4 that
{\rm{E}}\left[{{g^2({{X_1}})}{(-1)^{m}{\phi^{(m)}_{j, k}}(X_1)}}\right] = \int_{0}^{1} {{g^2}(x){(-1)^{m}{\phi^{(m)} _{j, k}}(x)}dx}. |
Using the assumption of independence between {U_i} and {X_i} ,
{\rm{E}}\left[{r({{X_1}})U_1^{2}(-1)^{m}{\phi^{(m)}_{j, k}}({{X_1}})}\right] = {\rm{E}}[{U_1^{2}}]{\rm{E}}\left[{r({{X_1}})(-1)^{m}{\phi^{(m)}_{j, k}}({{X_1}})}\right], |
{\rm{E}}[{f({{X_1}}){U_1}g({{X_1}}){(-1)^{m}{\phi^{(m)}_{j, k}}(X_1)}}] = {\rm{E}}[{U_1}]{\rm{E}}[{f({{X_1}})g({{X_1}}){(-1)^{m}{\phi^{(m)}_{j, k}}(X_1)}}]. |
Meanwhile, the conditions {\rm{V}}[{U_1}] = 1 and {\rm{E}}[{U_1}] = 0 imply {\rm{E}}[{U_1^{2}}] = 1 . Hence, one gets
\begin{align*} {\rm{E}}[{{{\hat\alpha}_{j, k}}}]& = {\rm{E}}\left[{r({{X_1}})(-1)^{m}{\phi^{(m)}_{j, k}}({{X_1}})}\right]\\ & = \int_{0}^{1} {r({x})(-1)^{m}{\phi^{(m)}_{j, k}}(x)dx} = (-1)^{m} \int_{0}^{1} {r({x}){\phi^{(m)}_{j, k}}(x)dx}\\ & = \int_{0}^{1} {r^{(m)}({x}){\phi_{j, k}}(x)dx} = \alpha _{j, k} \end{align*} |
by the assumption A2.
On the other hand, one takes \psi instead of \phi , and w_{j, k} instead of \int_{0}^{1} {{g^2}(x){(-1)^{m}{\phi^{(m)} _{j, k}}(x)}dx} . The second equation will be proved by the similar mathematical arguments.
Lemma 4.2. (Rosenthal's inequality) Let X_{1}, \ldots, X_{n} be independent random variables such that {\rm{E}}[X_{i}] = 0 and {\rm{E}}[|X_{i}|^{p}] < \infty . Then,
\begin{align*} {\rm{E}}\left[{{{\left|\sum\limits_{i = 1}^n X_{i}\right|}^{p}}}\right] \lesssim \begin{cases} \sum\limits_{i = 1}^n {\rm{E}}\left[{{{\left| X_{i}\right|}^{p}}}\right]+\left( \sum\limits_{i = 1}^n {\rm{E}}\left[{{{\left| X_{i}\right|}^{2}}}\right]\right) ^{\frac{p}{2}}, &\mathit{\text{ p > 2 }}, \\ \left(\sum\limits_{i = 1}^n {\rm{E}}\left[{{{\left| X_{i}\right|}^{2}}}\right]\right)^{\frac{p}{2}}, & {{ 1\leq p\leq 2 }}. \end{cases} \end{align*} |
Lemma 4.3. For the model (1.1) with A1–A5, 2^{j}\le n and 1\le\tilde{p} < \infty ,
\begin{gather} {\rm{E}}\left[{{{\left|{{{\hat \alpha }_{j, k}} - {\alpha _{j, k}}}\right|}^{\tilde{p}}}}\right] \lesssim n^{-\frac{\tilde{p}}{2}}2^{\tilde{p} mj} , \end{gather} | (4.3) |
\begin{gather} {\rm{E}}\left[{{{\left|{{{\hat \beta }_{j, k}} - {\beta _{j, k}}}\right|}^{\tilde{p}}}}\right] \lesssim \left( \dfrac{\ln n}{n}\right) ^{-\frac{\tilde{p}}{2}}2^{\tilde{p} mj} . \end{gather} | (4.4) |
Proof. By (4.1) and the independence of random variables {X_i} and {U_i} , one has
\begin{align*} \left|{{\hat \alpha }_{j, k}} - {\alpha _{j, k}}\right|& = \left| \frac{1}{n}\sum\limits_{i = 1}^n {Y_i^{2}{(-1)^{m}{\phi^{(m)}_{j, k}}(X_i)}} - \int_{0}^{1} {{g^2}(x){(-1)^{m}{\phi^{(m)} _{j, k}}(x)}dx} -{\rm{E}}\left[\hat \alpha _{j, k}\right]\right|\\ & = \dfrac{1}{n} \left|\sum\limits_{i = 1}^n \left( {Y_i^{2}{(-1)^{m}{\phi^{(m)}_{j, k}}(X_i)}}-{\rm{E}}\left[{Y_i^{2}{(-1)^{m}{\phi^{(m)}_{j, k}}(X_i)}} \right] \right) \right| \\ & = \dfrac{1}{n} \left|\sum\limits_{i = 1}^n A_{i}\right|. \end{align*} |
In this above equation, A_{i}: = {Y_i^{2}{(-1)^{m}{\phi^{(m)}_{j, k}}(X_i)}}-{\rm{E}}\left[{Y_i^{2}{(-1)^{m}{\phi^{(m)}_{j, k}}(X_i)}} \right] .
According to the definition of A_{i} , one knows that {\rm{E}}\left[A_{i}\right] = 0 and
\begin{align*} {\rm{E}}\left[\left|A_{i}\right|^{\tilde{p}}\right] & = {\rm{E}}\left[\left|{Y_i^{2}{(-1)^{m}{\phi^{(m)}_{j, k}}(X_i)}}-{\rm{E}}\left[{Y_i^{2}{(-1)^{m}{\phi^{(m)}_{j, k}}(X_i)}} \right]\right|^{\tilde{p}}\right]\\ &\lesssim {\rm{E}}\left[\left|{Y_i^{2}{(-1)^{m}{\phi^{(m)}_{j, k}}(X_i)}}\right|^{\tilde{p}}\right]\\ &\lesssim {\rm{E}}\left[\left|(r({X_1})U_1^{2}+g^{2}(X_1)){(-1)^{m}{\phi^{(m)}_{j, k}}(X_i)}\right|^{\tilde{p}}\right]\\ &\lesssim {\rm{E}}\left[U_{1}^{2\tilde{p}}\right] {\rm{E}}\left[\left|r({X_1}){\phi^{(m)}_{j, k}}(X_i)\right|^{\tilde{p}}\right]+{\rm{E}}\left[\left|g^{2}({X_1}){\phi^{(m)}_{j, k}}(X_i)\right|^{\tilde{p}}\right]. \end{align*} |
The assumption A5 shows {\rm{E}}[{U_1^{2\tilde{p}}}]\lesssim 1 . Furthermore, it follows from A1 and A3 that
\begin{gather*} {\rm{E}}[{U_1^{2\tilde{p}}}]{\rm{E}}\left[|{r({{X_1}}){{\phi^{(m)}_{j, k}}(X_1)|^{\tilde{p}}}}\right] \lesssim {\rm{E}}\left[{|{\phi^{(m)}_{j, k}}(X_1)|^{\tilde{p}}}\right] , \\ {\rm{E}}\left[{g^{2\tilde{p}}({{X_1}}){|{\phi^{(m)}_{j, k}}(X_1)|^{\tilde{p}}}}\right] \lesssim {\rm{E}}\left[{|{\phi^{(m)}_{j, k}}(X_1)|^{\tilde{p}}}\right]. \end{gather*} |
In addition, and the properties of wavelet functions imply that
\begin{align*} {\rm{E}}\left[\left|{\phi^{(m)}_{j, k}}(X_i)\right|^{\tilde{p}}\right] = \int_{0}^{1} |{\phi^{(m)}_{j, k}}(x)|^{\tilde{p}}dx& = 2^{j(\tilde{p}/2+m \tilde{p}-1)} \int_{0}^{1} |\phi^{(m)}(2^{j}x-k)|^{\tilde{p}}d(2^{j}x-k)\\ & = 2^{j(\tilde{p}/2+m \tilde{p}-1)} ||\phi^{(m)}||_{\tilde{p}}^{\tilde{p}}\lesssim 2^{j(\tilde{p}/2+m \tilde{p}-1)}. \end{align*} |
Hence,
{\rm{E}}\left[\left|A_{i}\right|^{\tilde{p}}\right] \lesssim 2^{j(\tilde{p}/2+m \tilde{p}-1)}. |
Especially in \tilde{p} = 2 , {\rm{E}}\left[\left|A_{i}\right|^{2}\right] \lesssim 2^{2mj} .
Using Rosenthal's inequality and 2^{j}\le n ,
\begin{align*} \begin{split} {\rm{E}}\left[{{{\left|{{{\hat \alpha }_{j, k}} - {\alpha _{j, k}}}\right|}^{\tilde{p}}}}\right] & = \dfrac{1}{n^{\tilde{p}}} {\rm{E}}\left[{{{\left|\sum\limits_{i = 1}^n A_{i}\right|}^{\tilde{p}}}}\right]\\ &\lesssim \begin{cases} \dfrac{1}{n^{\tilde{p}}} \left(\sum\limits_{i = 1}^n {\rm{E}}\left[{{{\left| A_{i}\right|}^{\tilde{p}}}}\right]+(\sum\limits_{i = 1}^n {\rm{E}}\left[{{{\left| A_{i}\right|}^{2}}}\right])^{\frac{\tilde{p}}{2}} \right), & { \tilde{p} > 2, } \\ \dfrac{1}{n^{\tilde{p}}} \left(\sum\limits_{i = 1}^n {\rm{E}}\left[{{{\left| A_{i}\right|}^{2}}}\right]\right)^{\frac{\tilde{p}}{2}}, & { 1 \leq \tilde{p} \leq 2 , } \end{cases}\\ &\lesssim \begin{cases} \dfrac{1}{n^{\tilde{p}}} \left(n \cdot 2^{j(\frac{\tilde{p}}{2}+m \tilde{p}-1)} + (n \cdot 2^{2mj})^{\frac{\tilde{p}}{2}}\right), & { \tilde{p} > 2, } \\ \dfrac{1}{n^{\tilde{p}}} \left( n \cdot 2^{2mj} \right)^{\frac{\tilde{p}}{2}}, & { 1 \leq \tilde{p} \leq 2, } \end{cases}\\ &\lesssim n^{-\frac{\tilde{p}}{2}}2^{\tilde{p}mj}. \end{split} \end{align*} |
Then, the first inequality is proved.
For the second inequality, note that
\begin{align*} \beta _{j, k} & = {\rm{E}}\left[ \frac{1}{n}\sum\limits_{i = 1}^n \left({Y_i^{2}{(-1)^{m}{\psi^{(m)}_{j, k}}(X_i)}}-w_{j, k}\right)\right]\\ & = \frac{1}{n}\sum\limits_{i = 1}^n {\rm{E}}\left[ \left({Y_i^{2}{(-1)^{m}{\psi^{(m)}_{j, k}}(X_i)}}-\int_{0}^{1} {{g^2}(x){(-1)^{m}{\psi^{(m)} _{j, k}}(x)}dx}\right)\right]\\ & = \frac{1}{n}\sum\limits_{i = 1}^n {\rm{E}}\left[ K_{i}\right] \end{align*} |
with (4.2) and K_{i}: = {Y_i^{2}{(-1)^{m}{\psi^{(m)}_{j, k}}(X_i)}}-\int_{0}^{1} {{g^2}(x){(-1)^{m}{\psi^{(m)} _{j, k}}(x)}dx}.
Let B_{i}: = K_{i}\mathbb{I}_{\left\{|K_{i}| \leq \rho_{n}\right\}}-{\rm{E}}\left[K_{i}\mathbb{I}_{\left\{|K_{i}| \leq \rho_{n}\right\}}\right] . Then, by the definition of {\hat \beta }_{j, k} in (2.4),
\begin{align} |{{{\hat \beta }_{j, k}} - {\beta _{j, k}}}| = |\frac{1}{n}\sum\limits_{i = 1}^n K_{i}\mathbb{I}_{\left\{|K_{i}| \leq \rho_{n}\right\}}-{\beta _{j, k}}| \leq \frac{1}{n} \left| \sum\limits_{i = 1}^n B_{i}\right| +\frac{1}{n} \sum\limits_{i = 1}^n {\rm{E}}\left[ |K_{i}|\mathbb{I}_{\left\{|K_{i}| > \rho_{n}\right\}}\right]. \end{align} | (4.5) |
Similar to the arguments of A_{i} , it is easy to see that {\rm{E}}\left[B_{i}\right] = 0 and
{\rm{E}}\left[\left|B_{i}\right|^{\tilde{p}}\right] \lesssim {\rm{E}}\left[\left|K_{i}\mathbb{I}_{\left\{|K_{i}| \leq \rho_{n}\right\}}\right|^{\tilde{p}}\right] \lesssim {\rm{E}}\left[\left|K_{i}\right|^{\tilde{p}}\right]\lesssim 2^{j(\frac{\tilde{p}}{2}+m \tilde{p}-1)}. |
Especially in the case of \tilde{p} = 2 , one can obtain {\rm{E}}\left[\left|B_{i}\right|^{2}\right] \lesssim 2^{2mj}. On the other hand,
\begin{eqnarray} {\rm{E}}\left[ |K_{i}|\mathbb{I}_{\left\{\left|K_{i}\right| > \rho_{n}\right\}}\right] \lesssim {\rm{E}}\left[ |K_{i}|\cdot \dfrac{|K_{i}|}{\rho_{n}}\right] = \dfrac{{\rm{E}}\left[K_{1}^{2}\right]}{\rho_{n}} \lesssim \dfrac{2^{2mj}}{\rho_{n}} = t_n = 2^{mj}\sqrt{\frac{\ln n}{n}}. \end{eqnarray} | (4.6) |
According to Rosenthal's inequality and 2^{j}\le n ,
\begin{align*} \begin{split} {\rm{E}}\left[{{{|{{{\hat \beta }_{j, k}} - {\beta _{j, k}}}|}^{\tilde{p}}}}\right] &\lesssim \dfrac{1}{n^{\tilde{p}}} {\rm{E}}\left[{{{\left|\sum\limits_{i = 1}^n B_{i}\right|}^{\tilde{p}}}}\right]+(t_{n})^{\tilde{p}}\\ &\lesssim \begin{cases} \dfrac{1}{n^{\tilde{p}}} \left(\sum\limits_{i = 1}^n {\rm{E}}\left[{{{\left| B_{i}\right|}^{\tilde{p}}}}\right]+(\sum\limits_{i = 1}^n {\rm{E}}\left[{{{\left| B_{i}\right|}^{2}}}\right])^{\frac{\tilde{p}}{2}} \right)+(t_{n})^{\tilde{p}}, & { \tilde{p} > 2, } \\ \dfrac{1}{n^{\tilde{p}}} \left(\sum\limits_{i = 1}^n {\rm{E}}\left[{{{\left| B_{i}\right|}^{2}}}\right]\right)^{\frac{\tilde{p}}{2}}+(t_{n})^{\tilde{p}}, & { 1 \leq \tilde{p} \leq 2, } \end{cases}\\ &\lesssim \begin{cases} \dfrac{1}{n^{\tilde{p}}} \left(n \cdot 2^{j(\frac{\tilde{p}}{2}+m \tilde{p}-1)} + (n \cdot 2^{2mj})^{\frac{\tilde{p}}{2}}\right)+\left( \dfrac{\ln n}{n}\right) ^{-\frac{\tilde{p}}{2}}\cdot 2^{\tilde{p} mj}, & { \tilde{p} > 2 , } \\ \dfrac{1}{n^{\tilde{p}}} \left( n \cdot 2^{2mj} \right)^{\frac{\tilde{p}}{2}}+\left( \dfrac{\ln n}{n}\right) ^{-\frac{\tilde{p}}{2}}\cdot 2^{\tilde{p} mj}, & { 1 \leq \tilde{p} \leq 2, } \end{cases}\\ &\lesssim \left( \dfrac{\ln n}{n}\right) ^{-\frac{\tilde{p}}{2}}2^{\tilde{p} mj}. \end{split} \end{align*} |
Then, the second inequality is proved.
Lemma 4.4. (Bernstein's inequality) Let X_{1}, \ldots, X_{n} be independent random variables such that {\rm{E}}[X_{i}] = 0 , |{{X_i}}| < M and {\rm{E}}[|X_{i}|^{2}] : = \sigma^{2} . Then, for each \nu > 0
\begin{align*} {\rm{P}}\left({\frac{1}{n}\left|{\sum\limits_{i = 1}^n {{X_i}}}\right| \ge \nu }\right) \le 2 \exp\left\{{ - \frac{n \nu^{2}}{{2({\sigma^{2} +\nu M/{3}})}}}\right\}. \end{align*} |
Lemma 4.5. For the model (1.1) with A1–A5 and 1\leq\tilde{p} < +\infty , there exists a constant \kappa > 1 such that
\begin{eqnarray} {\rm{P}}\left({{\left|{{{\hat \beta }_{j, k}} - {\beta _{j, k}}}\right|}}\ge\kappa{t_n}\right) \lesssim n^{-\tilde{p}}. \end{eqnarray} | (4.7) |
Proof. According to (4.5), one gets K_{i} = {Y_i^{2}{(-1)^{m}{\psi^{(m)}_{j, k}}(X_i)}}-\int_{0}^{1} {{g^2}(x){(-1)^{m}{\psi^{(m)} _{j, k}}(x)}dx} , B_{i} = K_{i}\mathbb{I}_{\left\{|K_{i}| \leq \rho_{n}\right\}}-{\rm{E}}\left[K_{i}\mathbb{I}_{\left\{|K_{i}| \leq \rho_{n}\right\}}\right] and
\begin{align*} |{{{\hat \beta }_{j, k}} - {\beta _{j, k}}}| \leq \frac{1}{n} \left| \sum\limits_{i = 1}^n B_{i}\right| +\frac{1}{n} \sum\limits_{i = 1}^n {\rm{E}}\left[ |K_{i}|\mathbb{I}_{\left\{|K_{i}| > \rho_{n}\right\}}\right]. \end{align*} |
Meanwhile, (4.6) shows that there exists c > 0 such that {\rm{E}}\left[|K_{i}|\mathbb{I}_{\left\{\left|K_{i}\right| > \rho_{n}\right\}}\right] \leq c{t_n} . Furthermore, the following conclusion is true.
\begin{align*} \left\{{|{{{\hat \beta }_{j, k}}-{\beta _{j, k, u}}}| \ge \kappa {t_n}}\right\} &\subseteq \left\{\Bigg[{\frac{1}{n} \left| \sum\limits_{i = 1}^n B_{i}\right| +\frac{1}{n} \sum\limits_{i = 1}^n {\rm{E}}\left( |K_{i}|\mathbb{I}_{\left\{|K_{i}| > \rho_{n}\right\}}\right)\Bigg] \ge \kappa {t_n}}\right\}\\ &\subseteq \left\{{\frac{1}{n}\left|{\sum\limits_{i = 1}^n {{B_i}}}\right| \ge (\kappa-c ){t_n}}\right\}. \end{align*} |
Note that the definition of B_{i} implies that |{{B_i}}|\lesssim \rho_{n} and {\rm{E}}\left[B_{i} \right] = 0 . Using the arguments of Lemma 4.3, {\rm{E}}[{B_{_i}^2}] : = \sigma^{2} \lesssim 2^{2mj} . Furthermore, by Bernstein's inequality,
\begin{align*} {\rm{P}}\left({\frac{1}{n}\left|{\sum\limits_{i = 1}^n {{B_i}}}\right| \ge (\kappa-c) {t_n}}\right) &\lesssim \exp\left\{{ - \frac{n (\kappa-c )^{2} {t_n}^2}{{2({\sigma^{2} +{{(\kappa-c ){t_n} \rho_{n}}}/{3}})}}}\right\}\\ &\lesssim \exp\left\{{ - \frac{n (\kappa-c )^{2} 2^{2mj}\cdot\frac{\ln n}{n}}{{2({2^{2mj} +{{(\kappa-c )\cdot 2^{2mj}}}/{3}})}}}\right\}\\ & = \exp\left\{ { -(\ln n) \frac{{{(\kappa-c ) ^2}}}{{2({1 + {(\kappa-c )}/{3}})}}}\right\} \\ & = {n^{ - \frac{{{(\kappa-c ) ^2}}}{2({1 +(\kappa-c)/{3}})}}}. \end{align*} |
Then, one can choose large enough \kappa such that
\begin{eqnarray*} {\rm{P}}\left({{\left|{{{\hat \beta }_{j, k}} - {\beta _{j, k}}}\right|}}\ge\kappa{t_n}\right) \lesssim {n^{ - \frac{{{(\kappa-c ) ^2}}}{2({1 +{(\kappa-c)}/{3}})}}}\lesssim n^{-\tilde{p}}. \end{eqnarray*} |
Proof of (a): Note that
\begin{align*} \left \| \hat r_n^{lin}(x)-r^{(m)}(x) \right \|^{\tilde{p}}_{\tilde{p}} \lesssim \left \| \hat r_n^{lin}(x)-{P_{j_{*}}}r^{(m)}(x) \right \|^{\tilde{p}}_{\tilde{p}}+\left \| {P_{j_{*}}}r^{(m)}(x)-r^{(m)}(x) \right \|^{\tilde{p}}_{\tilde{p}} \end{align*} |
Hence,
\begin{align} {\rm{E}}\left[ \left \| \hat r_n^{lin}(x)-r^{(m)}(x) \right \|^{\tilde{p}}_{\tilde{p}}\right] &\lesssim {\rm{E}}\left[\left \| \hat r_n^{lin}(x)-{P_{j_{*}}}r^{(m)}(x) \right \|^{\tilde{p}}_{\tilde{p}}\right] +\left \| {P_{j_{*}}}r^{(m)}(x)-r^{(m)}(x) \right \|^{\tilde{p}}_{\tilde{p}}. \end{align} | (4.8) |
\blacksquare The stochastic term {\rm{E}}\left[\left \| \hat r_n^{lin}(x)-{P_{j_{*}}}r^{(m)}(x) \right \|^{\tilde{p}}_{\tilde{p}}\right] .
It follows from Lemma 1.1 that
\begin{align*} {\rm{E}}\left[\left \| \hat r_n^{lin}(x)-{P_{j_{*}}}r^{(m)}(x) \right \|^{\tilde{p}}_{\tilde{p}}\right] & = {\rm{E}}\left[\left\| \sum\limits_{k \in {\mit\Lambda _{{j_*}}}} \left( {\hat \alpha}_{{j_*}, k}-{\alpha}_{{j_*}, k}\right)\phi_{j_*, k}(x)\right\| ^{\tilde{p}}_{\tilde{p}}\right] \\ &\sim 2^{j_*(\frac{1}{2}-\frac{1}{\tilde{p}}){\tilde{p}}} \sum\limits_{k \in {\mit\Lambda _{{j_*}}}} {\rm{E}}\left[\left| {\hat \alpha}_{{j_*}, k}-{\alpha}_{{j_*}, k} \right| ^{\tilde{p}}\right]. \end{align*} |
Then, according to (4.3), |{{\mit\Lambda _{{j_*}}}}|\sim{2^{j_*}} and {2^{{j_*}}} \sim{n^{\frac{1}{{2s' + 2m+1}}}} , one gets
\begin{align} {\rm{E}}\left[\left \| \hat r_n^{lin}(x)-{P_{j_{*}}}r^{(m)}(x) \right \|^{\tilde{p}}_{\tilde{p}}\right] \sim 2^{j_* \frac{\tilde{p}}{2}(2m+1)} \cdot n^{-\frac{\tilde{p}}{2}} \sim {n^{-\frac{{\tilde{p}s'}}{{2s' + 2m+1}}}}. \end{align} | (4.9) |
\blacksquare The bias term \left \| {P_{j_{*}}}r^{(m)}(x)-r^{(m)}(x) \right \|^{\tilde{p}}_{\tilde{p}} .
When p > \tilde{p} \ge 1 , s' = s-({\frac{1}{p}-\frac{1}{\tilde{p}}})_+ = s . Using Hölder inequality, Lemma 1.2 and r^{(m)} \in B_{p, q}^s({{[0, 1]}}) ,
\left \| {P_{j_{*}}}r^{(m)}(x)-r^{(m)}(x) \right \|^{\tilde{p}}_{\tilde{p}}\lesssim \left \| {P_{j_{*}}}r^{(m)}(x)-r^{(m)}(x) \right \|^{\tilde{p}}_{p} \lesssim 2^{-j_{*} \tilde{p} s} = 2^{-j_{*} \tilde{p} s'}\sim {n^{-\frac{\tilde{p}s'}{{2s' + 2m+1}}}}. |
When 1 \leq p\leq\tilde{p} and s > \dfrac{1}{p} , one knows that B_{p, q}^s({{[0, 1]}}) \subseteq B_{\tilde{p}, \infty}^{s'} ({{[0, 1]}}) and
\begin{align*} \left \| {P_{j_{*}}}r^{(m)}(x)-r^{(m)}(x) \right \|^{\tilde{p}}_{\tilde{p}} \lesssim 2^{-j_{*} \tilde{p} s'}\sim {n^{-\frac{\tilde{p} s'}{{2s' + 2m+1}}}}. \end{align*} |
Hence, the following inequality holds in both cases.
\begin{align} \left \| {P_{j_{*}}}r^{(m)}(x)-r^{(m)}(x) \right \|^{\tilde{p}}_{\tilde{p}} \lesssim {n^{-\frac{\tilde{p} s'}{{2s' + 2m+1}}}}. \end{align} | (4.10) |
Finally, the results (4.8)–(4.10) show
\begin{eqnarray*} {\rm{E}}\left[\left \| \hat r_n^{lin}(x)-r^{(m)}(x) \right \|^{\tilde{p}}_{\tilde{p}}\right]\lesssim {n^{-\frac{\tilde{p} s'}{{2s' + 2m+1}}}}. \end{eqnarray*} |
Proof of (b): By the definitions of \hat r_n^{lin}(x) and \hat r_n^{non}(x) , one has
\begin{align*} \left\| {\hat r_n^{non}(x) - r^{(m)}(x)}\right\| ^{\tilde{p}}_{\tilde{p}}&\lesssim \left\| \hat r_n^{lin}(x) - {P_{{j_*}}}r^{(m)}(x)\right\| ^{\tilde{p}}_{\tilde{p}} + \left\| r^{(m)}(x)-{P_{{j_1} + 1}}r^{(m)}(x)\right\| ^{\tilde{p}}_{\tilde{p}}\\ &+\left\| \sum\limits_{j = {j_*}}^{{j_1}} \sum\limits_{k \in {\mit\Lambda _j}} {\left({{{\hat\beta }_{j, k}}{\mathbb{I}_{\{{|{{{\hat \beta }_{j, k}}}| \ge \kappa {t_n}}\}}} - {\beta _{j, k}}}\right)}{\psi _{j, k}}(x)\right\| ^{\tilde{p}}_{\tilde{p}}. \end{align*} |
Furthermore,
\begin{align} {\rm{E}}\left[\left \| \hat r_n^{non}(x)-r^{(m)}(x) \right \|^{\tilde{p}}_{\tilde{p}}\right] \lesssim T_{1}+T_{2}+Q. \end{align} | (4.11) |
In this above inequality,
\begin{gather*} T_{1}: = {\rm{E}}\left[\left\| \hat r_n^{lin}(x) - {P_{{j_*}}}r^{(m)}(x)\right\| ^{\tilde{p}}_{\tilde{p}} \right], \\ T_{2}: = \left\| r^{(m)}(x)-{P_{{j_1} + 1}}r^{(m)}(x)\right\| ^{\tilde{p}}_{\tilde{p}}, \\ Q: = {\rm{E}}\left[ \left\| \sum\limits_{j = {j_*}}^{{j_1}} \sum\limits_{k \in {\mit\Lambda _j}} {\left({{{\hat\beta }_{j, k}}{\mathbb{I}_{\{{|{{{\hat \beta }_{j, k}}}| \ge \kappa {t_n}}\}}} - {\beta _{j, k}}}\right)}{\psi _{j, k}}(x)\right\| ^{\tilde{p}}_{\tilde{p}}\right]. \end{gather*} |
\blacksquare For T_{1} . According to (4.9) and {2^{{j_*}}}\sim{n^{\frac{1}{{2t + 2m+1}}}} \left(t > s\right) ,
\begin{align} T_{1} \sim 2^{j_* \frac{\tilde{p}}{2}(2m+1)} \cdot n^{-\frac{\tilde{p}}{2}} \sim n^{-\frac{\tilde{p} t}{2t+2m+1}} < n^{-\frac{\tilde{p} s}{2s+2m+1}} \leq n^{-\tilde{p} \delta}. \end{align} | (4.12) |
\blacksquare For T_{2} . Using similar mathematical arguments as (4.10), when p > \tilde{p} \ge 1 , one can obtain T_{2}: = \left\| r^{(m)}(x)-{P_{{j_1} + 1}}r^{(m)}(x)\right\| ^{\tilde{p}}_{\tilde{p}} \lesssim 2^{-j_{1}\tilde{p}s} . This with {2^{{j_1}}}\sim \left(\frac{n}{{\ln n}}\right)^{\frac{1}{2m+1}} leads to
T_{2}\lesssim 2^{-j_{1} \tilde{p}s} < \left( \frac{\ln n}{{n}}\right)^{\frac{\tilde{p}s}{2m+1}} \le \left( \frac{\ln n}{{ n}}\right)^{\frac{\tilde{p}s}{2s+2m+1}} \le \left( \frac{\ln n}{{ n}}\right)^{\tilde{p} \delta}. |
On the other hand, when 1 \leq p\leq\tilde{p} and s > \dfrac{1}{p} , one has B_{p, q}^s({{[0, 1]}}) \subseteq B_{\tilde{p}, \infty}^{s-{\frac{1}{p}+\frac{1}{\tilde{p}}}} ({{[0, 1]}}) and
\begin{align*} T_{2} \lesssim 2^{-j_1 \tilde{p} (s-1/p+1/\tilde{p})}\sim \left( \dfrac{\ln n}{n}\right) ^{\frac{\tilde{p}(s-1/p+1/\tilde{p})}{2m+1}} < \left( \dfrac{\ln n}{n}\right) ^{\frac{\tilde{p}(s-1/p+1/\tilde{p})}{2(s-1/p)+2m+1}} \le \left( \frac{\ln n}{{ n}}\right)^{\tilde{p} \delta}. \end{align*} |
Therefore, for each 1 \le \tilde{p} < \infty ,
\begin{align} T_{2}\lesssim \left( \frac{\ln n}{{ n}}\right)^{\tilde{p} \delta}. \end{align} | (4.13) |
\blacksquare For Q . According to Hölder inequality and Lemma 1.1,
\begin{align*} Q&\lesssim (j_1-j_*+1)^{\tilde{p}-1} \sum\limits_{j = {j_*}}^{{j_1}} {\rm{E}}\left[ \left\| \sum\limits_{k \in {\mit\Lambda _j}} {\left({{{\hat\beta }_{j, k}}{\mathbb{I}_{\{{|{{{\hat \beta }_{j, k}}}| \ge \kappa {t_n}}\}}} - {\beta _{j, k}}}\right)}{\psi _{j, k}}(x)\right\| ^{\tilde{p}}_{\tilde{p}}\right] \\ &\lesssim (j_1-j_*+1)^{\tilde{p}-1} \sum\limits_{j = {j_*}}^{{j_1}} 2^{j(\frac{1}{2}-\frac{1}{\tilde{p}}){\tilde{p}}} \sum\limits_{k \in {\mit\Lambda _{j}}} {\rm{E}}\left[|{{{\hat \beta }_{j, k}}{\mathbb{I}_{\{{|{{{\hat \beta }_{j, k}}}|\ge \kappa{t_n}}\}}} - {\beta _{j, k}}}|^{\tilde{p}}\right]. \end{align*} |
Note that
\begin{align*} |{{{\hat \beta }_{j, k}}{\mathbb{I}_{\{{|{{{\hat \beta }_{j, k}}}|\ge \kappa{t_n}}\}}} - {\beta _{j, k}}}|^{\tilde{p}} & = |{{{\hat \beta }_{j, k}} - {\beta _{j, k}}}|^{\tilde{p}}{{\mathbb{I}_{\{{|{{{\hat \beta }_{j, k}}}| \ge \kappa {t_n}, | {{\beta _{j, k}}}| < \frac{{\kappa {t_n}}}{2}}\}}} + |{{{\hat \beta }_{j, k}} - {\beta _{j, k}}}|^{\tilde{p}}{\mathbb{I}_{\{{|{{{\hat \beta }_{j, k}}}| \ge \kappa {t_n}, |{{\beta _{j, k}}}|\ge \frac{{\kappa {t_n}}}{2}}\}}}}\\ &+ |{{\beta _{j, k}}}|^{\tilde{p}}{{\mathbb{I}_{\{{|{{{\hat \beta }_{j, k}}}| < \kappa {t_n}, | {{\beta _{j, k}}}| > 2\kappa {t_n}}\}}} + |{{\beta _{j, k}}}|^{\tilde{p}}{\mathbb{I}_{\{{|{{{\hat \beta }_{j, k}}}| < \kappa {t_n}, | {{\beta _{j, k}}}| \le 2\kappa {t_n}}\}}}}. \end{align*} |
Meanwhile,
\begin{gather*} {{\{{|{{{\hat \beta }_{j, k}}}|\ge \kappa{t_n}}, |{{\beta _{j, k}}}| < \frac{{\kappa {t_n}}}{2}\}}} \subseteq{{\{|{{{\hat \beta }_{j, k}} - {\beta _{j, k}}}| > \frac{{\kappa{t_n}}}{2}\}}}, \\ {{\{{|{{{\hat \beta }_{j, k}}}| < \kappa{t_n}}, |{{\beta _{j, k}}}| > 2\kappa {t_n}\}}}\subseteq {{\{|{{{\hat \beta }_{j, k}} - {\beta _{j, k}}}| > \kappa{t_n}\}}}\subseteq {{\{|{{{\hat \beta }_{j, k}} - {\beta _{j, k}}}| > \frac{{\kappa{t_n}}}{2}\}}}. \end{gather*} |
Then, Q can be decomposed as
\begin{align} Q\lesssim (j_1-j_*+1)^{\tilde{p}-1}\left( Q_{1}+Q_{2}+Q_{3}\right), \end{align} | (4.14) |
where
\begin{gather*} Q_{1}: = \sum\limits_{j = {j_*}}^{{j_1}} 2^{j(\frac{1}{2}-\frac{1}{\tilde{p}}){\tilde{p}}} \sum\limits_{k \in {\mit\Lambda _{j}}} {\rm{E}}\left[|{{{\hat \beta }_{j, k}} - {\beta _{j, k}}}|^{\tilde{p}} {\mathbb{I}_{\{|{{{\hat \beta }_{j, k}} - {\beta _{j, k}}}| > \frac{{\kappa{t_n}}}{2}\}}}\right], \\ Q_{2}: = \sum\limits_{j = {j_*}}^{{j_1}} 2^{j(\frac{1}{2}-\frac{1}{\tilde{p}}){\tilde{p}}} \sum\limits_{k \in {\mit\Lambda _{j}}} {\rm{E}}\left[|{{{\hat \beta }_{j, k}} - {\beta _{j, k}}}|^{\tilde{p}} {\mathbb{I}_{\{|{{\beta _{j, k}}}|\ge \frac{{\kappa {t_n}}}{2}\}}}\right], \\ Q_{3}: = \sum\limits_{j = {j_*}}^{{j_1}} 2^{j(\frac{1}{2}-\frac{1}{\tilde{p}}){\tilde{p}}} \sum\limits_{k \in {\mit\Lambda _{j}}} | {\beta _{j, k}}|^{\tilde{p}} {\mathbb{I}_{\{|{{\beta _{j, k}}}|\le 2\kappa {t_n}\}}}. \end{gather*} |
\blacksquare For {Q_1} . It follows from the Hölder inequality that
{\rm{E}}\left[|{{{\hat \beta }_{j, k}} - {\beta _{j, k}}}|^{\tilde{p}} {\mathbb{I}_{\{|{{{\hat \beta }_{j, k}} - {\beta _{j, k}}}| > \frac{{\kappa{t_n}}}{2}\}}}\right] \le {\left( {{\rm{E}}\left[{{{|{{{\hat \beta }_{j, k}} - {\beta _{j, k}}}|}^{2\tilde{p}}}}\right]}\right)^{\frac{1}{2}}}{\left[{{\rm{P}}\left({|{{{\hat \beta }_{j, k}} - {\beta _{j, k}}}| > \frac{{\kappa {t_n}}}{2}}\right)}\right]^{\frac{1}{2}}}. |
By Lemma 4.3, one gets
{\rm{E}}\left[{{{\left|{{{\hat \beta }_{j, k}} - {\beta _{j, k}}}\right|}^{2\tilde{p}}}}\right] \lesssim \left( \dfrac{\ln n}{n}\right) ^{-\tilde{p}} \cdot 2^{2\tilde{p} mj} . |
This with Lemma 4.5, |{{\mit\Lambda _{j}}}|\sim{2^{j}} and {2^{{j_1}}}\sim \left(\frac{n}{{\ln n}}\right)^{\frac{1}{2m+1}} shows that
\begin{align} Q_{1} \lesssim \sum\limits_{j = {j_*}}^{{j_1}} 2^{j(\frac{1}{2}-\frac{1}{\tilde{p}}){\tilde{p}}}2^{j} \cdot \left( \frac{\ln n}{n}\right) ^{\frac{\tilde{p}}{2}} 2^{\tilde{p} mj} \cdot n^{-\frac{\tilde{p}}{2}} \lesssim n^{-\frac{\tilde{p}}{2}} < n^{-\tilde{p} \delta}. \end{align} | (4.15) |
\blacksquare For {Q_2} . One defines
{2^{j'}} \sim \left(\frac{n}{\ln n} \right)^{\frac{1}{{2s + 2m+1}}}. |
Clearly, {2^{{j_*}}}\sim{n^{\frac{1}{{2t+2m + 1}}}} \left(t > s\right) \le {2^{j'}}\sim \left(\frac{n}{\ln n} \right)^{\frac{1}{{2s + 2m+1}}} < {2^{{j_1}}}\sim \left(\frac{n}{\ln n} \right) ^{\frac{1}{2m+1}} . Furthermore, one rewrites
\begin{align} {Q_2} = \left({\sum\limits_{j = {j_*}}^{j'} { + \sum\limits_{j = j' + 1}^{{j_1}}}}\right) 2^{j(\frac{1}{2}-\frac{1}{\tilde{p}}){\tilde{p}}} \sum\limits_{k \in {\mit\Lambda _j}}{\rm{E}}\left[|{{{\hat \beta }_{j, k}} - {\beta _{j, k}}}|^{\tilde{p}} {\mathbb{I}_{\{|{{\beta _{j, k}}}|\ge \frac{{\kappa {t_n}}}{2}\}}}\right] : = {Q_{21}} + {Q_{22}}. \end{align} | (4.16) |
\blacksquare For {Q_{21}} . By Lemma 4.3 and {2^{j'}} \sim \left(\frac{n}{\ln n} \right)^{\frac{1}{{2s + 2m+1}}},
\begin{align} {Q_{21}}&: = \sum\limits_{j = {j_*}}^{j'} 2^{j(\frac{1}{2}-\frac{1}{\tilde{p}}){\tilde{p}}} \sum\limits_{k \in {\mit\Lambda _j}} {\rm{E}}\left[|{{{\hat \beta }_{j, k}} - {\beta _{j, k}}}|^{\tilde{p}} {\mathbb{I}_{\{|{{\beta _{j, k}}}|\ge \frac{{\kappa {t_n}}}{2}\}}}\right]\\ &\le \sum\limits_{j = {j_*}}^{j'} 2^{j(\frac{1}{2}-\frac{1}{\tilde{p}}){\tilde{p}}} \sum\limits_{k \in {\mit\Lambda _j}} {\rm{E}}\left[|{{{\hat \beta }_{j, k}} - {\beta _{j, k}}}|^{\tilde{p}} \right] \lesssim \left( \frac{\ln n}{n}\right)^{\frac{\tilde{p}}{2}} \sum\limits_{j = {j_*}}^{j'} 2^{j(2m+1) \frac{\tilde{p}}{2}}\\ &\lesssim \left( \frac{\ln n}{n}\right)^{\frac{\tilde{p}}{2}} 2^{j'(2m+1) \frac{\tilde{p}}{2}} \sim \left( \frac{\ln n}{n}\right)^{\frac{{\tilde{p}}s}{{2s + 2m+1}}} \le \left( \frac{\ln n}{n}\right)^{\tilde{p} \delta}. \end{align} | (4.17) |
\blacksquare For {Q_{22}} . Using Lemma 4.3, one has
\begin{align*} {Q_{22}}&: = \sum\limits_{j = j' + 1}^{{j_1}} 2^{j(\frac{1}{2}-\frac{1}{\tilde{p}}){\tilde{p}}} \sum\limits_{k \in {\mit\Lambda _j}} {\rm{E}}\left[|{{{\hat \beta }_{j, k}} - {\beta _{j, k}}}|^{\tilde{p}} {\mathbb{I}_{\{|{{\beta _{j, k}}}|\ge \frac{{\kappa {t_n}}}{2}\}}}\right]\\ &\lesssim \left( \frac{\ln n}{n}\right)^{\frac{\tilde{p}}{2}} \sum\limits_{j = j' + 1}^{{j_1}} 2^{j(\frac{1}{2}-\frac{1}{\tilde{p}}){\tilde{p}}+\tilde{p}mj} \sum\limits_{k \in {\mit\Lambda _j}} {\mathbb{I}_{\{|{{\beta _{j, k}}}|\ge \frac{{\kappa {t_n}}}{2}\}}}. \end{align*} |
When p > \tilde{p} \ge 1 , by the Hölder inequality, {t_n} = 2^{mj}\sqrt{{\ln n}/n} , {2^{j'}}\sim\left(\frac{n}{\ln n} \right)^{\frac{1}{{2s + 2m+1}}} and Lemma 1.2, one can obtain that
\begin{align} {Q_{22}} &\lesssim \left( \frac{\ln n}{n}\right)^{\frac{\tilde{p}}{2}} \sum\limits_{j = j' + 1}^{{j_1}} 2^{j(\frac{1}{2}-\frac{1}{\tilde{p}}){\tilde{p}}+\tilde{p}mj} \sum\limits_{k \in {\mit\Lambda _j}} \left( \dfrac{|{{\beta _{j, k}}}|}{\frac{{\kappa {t_n}}}{2}}\right) ^{\tilde{p}}\\ &\lesssim \sum\limits_{j = j' + 1}^{{j_1}} 2^{j(\frac{1}{2}-\frac{1}{\tilde{p}}){\tilde{p}}} \sum\limits_{k \in {\mit\Lambda _j}} {|\beta _{j, k}|^{\tilde{p}}} = \sum\limits_{j = j' + 1}^{{j_1}} 2^{j(\frac{1}{2}-\frac{1}{\tilde{p}}){\tilde{p}}} \left\| \beta _{j, k} \right\| ^{\tilde{p}}_{\tilde{p}}\\ &\le \sum\limits_{j = j' + 1}^{{j_1}} 2^{j(\frac{1}{2}-\frac{1}{\tilde{p}}){\tilde{p}}} \cdot 2^{j(1-\frac{\tilde{p}}{p})}\left\| \beta _{j, k} \right\| ^{\tilde{p}}_{p}\\ & \lesssim \sum\limits_{j = j' + 1}^{{j_1}} 2^{-j\tilde{p}s} \lesssim 2^{-j'\tilde{p}s} \sim \left( \frac{\ln n}{n}\right)^{\frac{{\tilde{p}}s}{{2s + 2m+1}}} \le \left( \frac{\ln n}{n}\right)^{\tilde{p} \delta}. \end{align} | (4.18) |
When 1\leq p\leq\tilde{p} , it follows from Lemma 1.2 that
\begin{align} {Q_{22}} &\lesssim \left( \frac{\ln n}{n}\right)^{\frac{\tilde{p}}{2}} \sum\limits_{j = j' + 1}^{{j_1}} 2^{j(\frac{1}{2}-\frac{1}{\tilde{p}}){\tilde{p}}+\tilde{p}mj} \sum\limits_{k \in {\mit\Lambda _j}} \left( \dfrac{|{{\beta _{j, k}}}|}{\frac{{\kappa {t_n}}}{2}}\right) ^{p}\\ &\lesssim \left( \frac{\ln n}{n}\right)^{\frac{\tilde{p}-p}{2}} \sum\limits_{j = j' + 1}^{{j_1}} 2^{j(\frac{1}{2}-\frac{1}{\tilde{p}}){\tilde{p}}+j(\tilde{p}-p)m} \left\| \beta _{j, k} \right\|^{p}_{p}\\ &\le \left( \frac{\ln n}{n}\right)^{\frac{\tilde{p}-p}{2}} \sum\limits_{j = j' + 1}^{{j_1}} 2^{-j(sp+\frac{p}{2}-\frac{\tilde{p}}{2}-(\tilde{p}-p)m)}. \end{align} | (4.19) |
Take
\epsilon : = sp-\dfrac{\tilde{p}-p}{2} (2m+1). |
Then, (4.19) can be rewritten as
\begin{align} {Q_{22}} \lesssim \left( \frac{\ln n}{n}\right)^{\frac{\tilde{p}-p}{2}} \sum\limits_{j = j' + 1}^{{j_1}} 2^{-j \epsilon}. \end{align} | (4.20) |
When \epsilon > 0 holds if and only if p > \frac{\tilde{p}(2m+1)}{2s+2m+1} , \delta = \frac{s}{2s+2m+1} and
\begin{align} {Q_{22}} \lesssim \left( \frac{\ln n}{n}\right)^{\frac{\tilde{p}-p}{2}} 2^{-j' \epsilon} \sim \left( \frac{\ln n}{n}\right)^{\frac{{\tilde{p}}s}{{2s + 2m+1}}} = \left( \frac{\ln n}{n}\right)^{\tilde{p} \delta}. \end{align} | (4.21) |
When \epsilon\le0 holds if and only if p \leq \frac{\tilde{p}(2m+1)}{2s+2m+1} , \delta = \frac{s-1/p+1 / \tilde{p}}{2(s-1 /p)+2m+1} . Define
2^{j''} \sim \left( \frac{n}{\ln n}\right) ^{\frac{\delta}{s-1/p+1/\tilde{p}}} = \left( \frac{n}{\ln n}\right) ^{\frac{1}{2(s-1/p)+2m+1}} , |
and obviously, {2^{j'}} \sim \left(\frac{n}{\ln n} \right)^{\frac{1}{{2s + 2m+1}}} < 2^{j''} \sim \left(\frac{n}{\ln n}\right) ^{\frac{\delta}{s-1/p+1/\tilde{p}}} < {2^{{j_1}}}\sim \left(\frac{n}{\ln n} \right) ^{\frac{1}{2m+1}} . Furthermore, one rewrites
\begin{align} \begin{split} {Q_{22}} & = \left({\sum\limits_{j = {j' + 1}}^{j''} + \sum\limits_{j = j'' + 1}^{{j_1}}}\right) 2^{j(\frac{1}{2}-\frac{1}{\tilde{p}}){\tilde{p}}} \sum\limits_{k \in {\mit\Lambda _j}} {\rm{E}}\left[|{{{\hat \beta }_{j, k}} - {\beta _{j, k}}}|^{\tilde{p}} {\mathbb{I}_{\{|{{\beta _{j, k}}}|\ge \frac{{\kappa {t_n}}}{2}\}}}\right]\\ & : = {Q_{221}} + {Q_{222}}. \end{split} \end{align} | (4.22) |
For {Q_{221}} . Note that \frac{\tilde{p}-p}{2}+\frac{\delta \epsilon }{s-1/p+1 /\tilde{p}} = \tilde{p} \delta in the case of \epsilon\le0 . Then, by the same arguments of (4.20), one gets
\begin{align} {Q_{221}} \lesssim \left( \frac{\ln n}{n}\right)^{\frac{\tilde{p}-p}{2}} \sum\limits_{j = {j' + 1}}^{j''} 2^{-j\epsilon} \lesssim \left( \frac{\ln n}{n}\right)^{\frac{\tilde{p}-p}{2}} 2^{-{j''}\epsilon} \sim \left( \frac{\ln n}{n}\right)^{\tilde{p} \delta}. \end{align} | (4.23) |
For {Q_{222}} . The conditions 1\leq p\leq\tilde{p} and s > 1/p imply B_{p, q}^s({{[0, 1]}}) \subset B_{\tilde{p}, q}^{s-\frac{1}{p}+\frac{1}{\tilde{p}}}({{[0, 1]}}) . Similar to (4.18), one obtains
\begin{align} {Q_{222}} &\lesssim \left( \frac{\ln n}{n}\right)^{\frac{\tilde{p}}{2}} \sum\limits_{j = j'' + 1}^{{j_1}} 2^{j(\frac{1}{2}-\frac{1}{\tilde{p}}){\tilde{p}}+\tilde{p}mj} \sum\limits_{k \in {\mit\Lambda _j}} \left( \dfrac{|{{\beta _{j, k}}}|}{\frac{{\kappa {t_n}}}{2}}\right) ^{\tilde{p}}\\ &\lesssim \sum\limits_{j = j'' + 1}^{j_1} 2^{j(\frac{1}{2}-\frac{1}{\tilde{p}}){\tilde{p}}} \left\|{\beta _{j, k}} \right\| ^{\tilde{p}}_{\tilde{p}} \lesssim \sum\limits_{j = j'' + 1}^{j_1} 2^{j(\frac{1}{2}-\frac{1}{\tilde{p}}){\tilde{p}}} \cdot 2^{-j(s-\frac{1}{\tilde{p}}+\frac{1}{2}){\tilde{p}}}\\ &\lesssim 2^{-j'' (s-{\frac{1}{p}+\frac{1}{\tilde{p}}})\tilde{p}} \sim \left( \frac{\ln n}{n}\right)^{\tilde{p}\delta}. \end{align} | (4.24) |
Combining (4.18), (4.21), (4.23) and (4.24),
{Q_{22}}\lesssim \left( \frac{\ln n}{n}\right)^{\tilde{p}\delta}. |
This with (4.16) and (4.17) shows that
\begin{align} {Q_{2}}\lesssim \left( \frac{\ln n}{n}\right)^{\tilde{p} \delta}. \end{align} | (4.25) |
\blacksquare For {Q_3} . According to the definition of {2^{j'}} , one can write
\begin{align*} {Q_3} = \left({\sum\limits_{j = {j_*}}^{j'} + \sum\limits_{j = j'+1}^{{j_1}}}\right) 2^{j(\frac{1}{2}-\frac{1}{\tilde{p}}){\tilde{p}}} \sum\limits_{k \in {\mit\Lambda _j}} |{\beta _{j, k}}|^{\tilde{p}} {\mathbb{I}_{\{|{{\beta _{j, k}}}|\le 2\kappa {t_n}\}}}: = {Q_{31}} + {Q_{32}}. \end{align*} |
\blacksquare For {Q_{31}} . It is easy to see that
\begin{align*} \begin{split} {Q_{31}}&: = \sum\limits_{j = {j_*}}^{j'} 2^{j(\frac{1}{2}-\frac{1}{\tilde{p}}){\tilde{p}}} \sum\limits_{k \in {\mit\Lambda _j}} |{\beta _{j, k}}|^{\tilde{p}} {\mathbb{I}_{\{|{{\beta _{j, k}}}|\le 2\kappa {t_n}\}}} \le \sum\limits_{j = {j_*}}^{j'} 2^{j(\frac{1}{2}-\frac{1}{\tilde{p}}){\tilde{p}}} \sum\limits_{k \in {\mit\Lambda _j}} \left( 2\kappa {t_n}\right) ^{\tilde{p}} \\ &\lesssim \left( \frac{\ln n}{n}\right)^{\frac{\tilde{p}}{2}} \cdot 2^{(2m+1)j'\frac{\tilde{p}}{2}} \sim \left( \frac{\ln n}{n}\right)^{\frac{{\tilde{p}}s}{{2s + 2m+1}}} \le \left( \frac{\ln n}{n}\right)^{\tilde{p} \delta}. \end{split} \end{align*} |
\blacksquare For {Q_{32}} . One rewrites {Q_{32}} = \sum\limits_{j = j' + 1}^{{j_1}} 2^{j(\frac{1}{2}-\frac{1}{\tilde{p}}){\tilde{p}}} \sum\limits_{k \in {\mit\Lambda _j}} |{\beta _{j, k}}|^{\tilde{p}} {\mathbb{I}_{\{|{{\beta _{j, k}}}|\le 2\kappa {t_n}\}}} . When p > \tilde{p}\ge1 , using the Hölder inequality and Lemma 1.2,
\begin{align*} {Q_{32}} \le \sum\limits_{j = j' + 1}^{{j_1}} 2^{j(\frac{1}{2}-\frac{1}{\tilde{p}}){\tilde{p}}} \sum\limits_{k \in {\mit\Lambda _j}} |{\beta _{j, k}}|^{\tilde{p}} \lesssim 2^{-j'\tilde{p}s} \sim \left( \frac{\ln n}{n}\right)^{\frac{{\tilde{p}}s}{{2s + 2m+1}}} \le \left( \frac{\ln n}{n}\right)^{\tilde{p} \delta}. \end{align*} |
When 1\leq p\leq\tilde{p} , one has
\begin{align*} \begin{split} {Q_{32}} & \le \sum\limits_{j = j' + 1}^{{j_1}} 2^{j(\frac{1}{2}-\frac{1}{\tilde{p}}){\tilde{p}}} \sum\limits_{k \in {\mit\Lambda _j}} |{\beta _{j, k}}|^{\tilde{p}} \left( \frac{2 \kappa{t_n}}{|\beta _{j, k}|}\right) ^{\tilde{p}-p}\\ &\lesssim \left( \frac{\ln n}{n}\right)^{\frac{\tilde{p}-p}{2}} \sum\limits_{j = j' + 1}^{{j_1}} 2^{j(\frac{1}{2}-\frac{1}{\tilde{p}}){\tilde{p}}+j(\tilde{p}-p)m} \left\| \beta _{j, k} \right\|^{p}_{p}\\ &\le \left( \frac{\ln n}{n}\right)^{\frac{\tilde{p}-p}{2}} \sum\limits_{j = j' + 1}^{{j_1}} 2^{-j(sp+\frac{p}{2}-\frac{\tilde{p}}{2}-(\tilde{p}-p)m)}\\ & = \left( \frac{\ln n}{n}\right)^{\frac{\tilde{p}-p}{2}} \sum\limits_{j = j' + 1}^{{j_1}} 2^{-j\epsilon}. \end{split} \end{align*} |
For the case of \epsilon > 0 , one can easily obtain that \delta = \frac{s}{2s+2m+1} and
\begin{align*} {Q_{32}} \lesssim \left(\frac{\ln n}{n}\right)^{\frac{\tilde{p}-p}{2}} 2^{-j' \epsilon} \sim \left( \frac{\ln n}{n}\right)^{\frac{{\tilde{p}}s}{{2s + 2m+1}}} = \left( \frac{\ln n}{n}\right)^{\tilde{p} \delta}. \end{align*} |
When \epsilon \le 0 , \delta = \frac{s-1/p+1 /\tilde{p}}{2(s-1 /p)+2m+1} . Moreover, by the definition of 2^{j''} , one rewrites
\begin{align*} {Q_{32}} = \left({\sum\limits_{j = {j' + 1}}^{j''} + \sum\limits_{j = j'' + 1}^{{j_1}}}\right) 2^{j(\frac{1}{2}-\frac{1}{\tilde{p}}){\tilde{p}}} \sum\limits_{k \in {\mit\Lambda _j}} |{\beta _{j, k}}|^{\tilde{p}} {\mathbb{I}_{\{|{{\beta _{j, k}}}|\le 2\kappa {t_n}\}}} : = {Q_{321}} + {Q_{322}}. \end{align*} |
Note that
\begin{align*} {Q_{321}} &\lesssim \left(\frac{\ln n}{n}\right)^{\frac{\tilde{p}-p}{2}} \sum\limits_{j = {j' + 1}}^{j''} 2^{-j\epsilon} \lesssim \left(\frac{\ln n}{n}\right)^{\frac{\tilde{p}-p}{2}} 2^{-{j''}\epsilon} \sim \left( \frac{\ln n}{n}\right)^{\tilde{p} \delta}. \end{align*} |
On the other hand, similar to the arguments of (4.24), one has
\begin{align*} \begin{split} {Q_{322}} &\le \sum\limits_{j = j'' + 1}^{j_1} 2^{j(\frac{1}{2}-\frac{1}{\tilde{p}}){\tilde{p}}} \sum\limits_{k \in {\mit\Lambda _j}} |{\beta _{j, k}}|^{\tilde{p}} = \sum\limits_{j = j'' + 1}^{j_1} 2^{j(\frac{1}{2}-\frac{1}{\tilde{p}}){\tilde{p}}} \left\|{\beta _{j, k}} \right\| ^{\tilde{p}}_{\tilde{p}} \lesssim \left( \frac{\ln n}{n}\right)^{\tilde{p} \delta}. \end{split} \end{align*} |
Therefore, in all of the above cases,
\begin{align} {Q_{3}}\lesssim \left( \frac{\ln n}{n}\right)^{\tilde{p} \delta}. \end{align} | (4.26) |
Finally, combining the above results (4.14), (4.15), (4.25) and (4.26), one gets
\begin{eqnarray*} Q \lesssim (j_1-j_*+1)^{\tilde{p}-1} \left( \frac{\ln n}{n}\right)^{\tilde{p} \delta} \lesssim (\ln n)^{\tilde{p}-1} \left( \frac{\ln n}{n}\right)^{\tilde{p}\delta}. \end{eqnarray*} |
This with (4.11)–(4.13) shows
{\rm{E}}\left[\left \| \hat r_n^{non}(x)-r^{(m)}(x) \right \|^{\tilde{p}}_{\tilde{p}}\right]\lesssim (\ln n)^{\tilde{p}-1} \left( \frac{\ln n}{n}\right)^{\tilde{p}\delta}. |
This paper considers wavelet estimations of the derivatives r^{(m)}(x) of the variance function r(x) in a heteroscedastic model. The upper bounds over L^{\tilde{p}} (1\leq \tilde{p} < \infty) risk of the wavelet estimators are discussed under some mild assumptions. The results show that the linear wavelet estimator can obtain the optimal convergence rate in the case of p > \tilde{p}\ge1 . When p\leq\tilde{p} , the nonlinear wavelet estimator has a better convergence rate than the linear estimator. Moreover, the nonlinear wavelet estimator is adaptive. Finally, some numerical experiments are presented to verify the good performances of the wavelet estimators.
We would like to thank the reviewers for their valuable comments and suggestions, which helped us to improve the quality of the manuscript. This paper is supported by the Guangxi Natural Science Foundation (No. 2022JJA110008), National Natural Science Foundation of China (No. 12001133), Center for Applied Mathematics of Guangxi (GUET), and Guangxi Colleges and Universities Key Laboratory of Data Analysis and Computation.
All authors declare that they have no conflicts of interest.
[1] |
D. V. Thong, D. V. Hieu, Inertial extragradient algorithms for strongly pseudomonotone variational inequalities, J. Comput. Appl. Math., 341 (2018), 80–98. https://doi.org/10.1016/j.cam.2018.03.019 doi: 10.1016/j.cam.2018.03.019
![]() |
[2] |
P. K. Anh, D. V. Thong, N. T. Vinh, Improved inertial extragradient methods for solving pseudo-monotone variational inequalities, Optimization, 71 (2020), 505–528. https://doi.org/10.1080/02331934.2020.1808644 doi: 10.1080/02331934.2020.1808644
![]() |
[3] |
P. Q. Khanh, D. V. Thong, N. T. Vinh, Versions of the subgradient extragradient method for pseudomonotone variational inequalities, Acta Appl. Math., 170 (2020), 319–345. https://doi.org/10.1007/s10440-020-00335-9 doi: 10.1007/s10440-020-00335-9
![]() |
[4] |
S. Reich, D. V. Thong, Q. L. Dong, X. H. Li, V. T. Dung, New algorithms and convergence theorems for solving variational inequalities with non-Lipschitz mappings, Numer. Algorithms, 87 (2021), 527–549. https://doi.org/10.1007/s11075-020-00977-8 doi: 10.1007/s11075-020-00977-8
![]() |
[5] | F. Facchinei, J. S. Pang, Finite-Dimensional Variational Inequalities and Complementarity Problems, In: Springer Series in Operations Research, New York: Springer, 2003. |
[6] | I. Konnov, Combined relaxation methods for variational inequalities, Berlin: Springer-Verlag, 2001. |
[7] | G. M. Korpelevich, The extragradient method for finding saddle points and other problems, Matecon, 12 (1976), 747–756. |
[8] |
Y. Censor, A. Gibali, S. Reich, The subgradient extragradient method for solving variational inequalities in Hilbert space, J. Optim. Theory Appl., 148 (2011), 318–335. https://doi.org/10.1007/s10957-010-9757-3 doi: 10.1007/s10957-010-9757-3
![]() |
[9] |
Y. Censor, A. Gibali, S. Reich, Strong convergence of subgradient extragradient methods for the variational inequality problem in Hilbert space, Optim. Method. Softw., 26 (2011), 827–845. https://doi.org/10.1080/10556788.2010.551536 doi: 10.1080/10556788.2010.551536
![]() |
[10] |
Y. Censor, A. Gibali, S. Reich, Extensions of Korpelevich's extragrsient method for the variational inequality problem in Euclidean space, Optimization, 61 (2012), 1119–1132. https://doi.org/10.1080/02331934.2010.539689 doi: 10.1080/02331934.2010.539689
![]() |
[11] | B. S. He, A class of projection and contraction methods for monotone variational inequalities, Appl. Math. Optim., 35 (1997), 69–76. |
[12] |
X. J. Cai, G. Y. Gu, B. S. He, On the O(1/t) convergence rate of the projection and contraction methods for variational inequalities with Lipschitz continuous monotone operators, Comput. Optim. Appl., 57 (2014), 339–363. https://doi.org/10.1007/s10589-013-9599-7 doi: 10.1007/s10589-013-9599-7
![]() |
[13] |
Q. L. Dong, Y. J. Cho, L. L. Zhong, T. M. Rassias, Inertial projection and contraction algorithms for variational inequalities, J. Global Optim., 70 (2018), 687–704. https://doi.org/10.1007/s10898-017-0506-0 doi: 10.1007/s10898-017-0506-0
![]() |
[14] |
Q. L. Dong, Y. J. Cho, T. M. Rassias, The projection and contraction methods for finding common solutions to variational inequality problems, Optim. Lett., 12 (2018), 1871–1896. https://doi.org/10.1007/s11590-017-1210-1 doi: 10.1007/s11590-017-1210-1
![]() |
[15] |
Y. Shehu, O. S. Iyiola, Projection methods with alternating inertial steps for variational inequalities: Weak and linear convergence, Appl. Numer. Math., 157 (2020), 315–337. https://doi.org/10.1016/j.apnum.2020.06.009 doi: 10.1016/j.apnum.2020.06.009
![]() |
[16] |
Y. Malitsky, Golden ratio algorithms for variational inequalities, Math. Program., 184 (2018), 383–410. https://doi.org/10.1007/s10107-019-01416-w doi: 10.1007/s10107-019-01416-w
![]() |
[17] |
D. V. Thong, N. T. Vinh, Y. J. Cho, New strong convergence theorem of the inertial projection and contraction method for variational inequality problems, Numer. Algorithms, 84 (2019), 285–305. https://doi.org/10.1007/s11075-019-00755-1 doi: 10.1007/s11075-019-00755-1
![]() |
[18] |
P. Cholamjiak, D. V. Thong, Y. J. Cho, A novel inertial projection and contraction method for solving pseudomonotone variational inequality problems, Acta Appl. Math., 169 (2019), 217–245. https://doi.org/10.1007/s10440-019-00297-7 doi: 10.1007/s10440-019-00297-7
![]() |
[19] |
X. K. Chang, J. F. Yang, A golden ratio primal-dual algorithm for structured convex optimization, J. Sci. Comput., 87 (2021), 47. https://doi.org/10.1007/s10915-021-01452-9 doi: 10.1007/s10915-021-01452-9
![]() |
[20] |
X. K. Chang, J. F. Yang, H. C. Zhang, Golden ratio primal-dual algorithm with linesearch, SIAM J. Optim., 32 (2022), 1584–1613. https://doi.org/10.1137/21M1420319 doi: 10.1137/21M1420319
![]() |
[21] | H. H. Bauschke, P. L. Combettes, Convex analysis and monotone operator theory in Hilbert spaces, New York: Springer, 2011. |
[22] | K. Goebel, S. Reich, Uniform Convexity, Hyperbolic Geometry, and Nonexpansive Mappings, New York: Marcel Dekker, 1984. |
[23] | J. M. Ortega, W. C. Rheinboldt, Iterative solution of nonlinear equations in several variables, New York: Academic Press, 1970. |
[24] |
J. Mashreghi, M. Nasri, Forcing strong convergence of Korpelevich's method in Banach spaces with its applications in game theory, Nonlinear Anal., 72 (2010), 2086–2099. https://doi.org/10.1016/j.na.2009.10.009 doi: 10.1016/j.na.2009.10.009
![]() |
[25] |
G. L. Acedo, H. K. Xu, Iterative methods for strict pseudo-contractions in Hilbert spaces, Nonlinear Anal., 67 (2007), 2258–2271. https://doi.org/10.1016/j.na.2006.08.036 doi: 10.1016/j.na.2006.08.036
![]() |
[26] |
M. O. Osilike, S. C. Aniagbosor, Weak and strong convergence theorems for fixed points of asymptotically nonexpansive mappings, Math. Comput. Modell., 32 (2000), 1181–1191. https://doi.org/10.1016/S0895-7177(00)00199-0 doi: 10.1016/S0895-7177(00)00199-0
![]() |
[27] |
Y. Shehu, Q. L. Dong, L. L. Liu, Fast alternated inertial projection algorithms for pseudo-monotone variational inequalities, J. Comput. Appl. Math., 415 (2022), 114517. https://doi.org/10.1016/j.cam.2022.114517 doi: 10.1016/j.cam.2022.114517
![]() |
[28] | P. T. Harker, J.-S. Pang, A damped-Newton method for the linear complementarity problem, in: Computational Solution of Nonlinear Systems of Equations (Fort Collins, CO, 1988), In: Lectures in Applied Mathematics, 1990. |
1. | Junke Kou, Hao Zhang, Partial Derivatives Estimation of Multivariate Variance Function in Heteroscedastic Model via Wavelet Method, 2024, 13, 2075-1680, 69, 10.3390/axioms13010069 |
HeaviSine | Corner | Spikes | |||||||
r_{1} | r_{2} | r_{3} | r_{1} | r_{2} | r_{3} | r_{1} | r_{2} | r_{3} | |
MSE(\hat r^{lin}, r) | 0.0184 | 0.0073 | 0.0071 | 0.0189 | 0.0075 | 0.0064 | 0.0189 | 0.0069 | 0.0052 |
MSE(\hat r^{non}, r) | 0.0048 | 0.0068 | 0.0064 | 0.0044 | 0.0070 | 0.0057 | 0.0042 | 0.0061 | 0.0046 |
MSE(\hat r'^{lin}, r') | 0.7755 | 0.0547 | 0.0676 | 0.7767 | 0.1155 | 0.0737 | 0.7360 | 0.2566 | 0.0655 |
MSE(\hat r'^{non}, r') | 0.2319 | 0.0573 | 0.0560 | 0.2204 | 0.0644 | 0.0616 | 0.2406 | 0.2868 | 0.0539 |
AME(\hat r^{lin}, r) | 0.0935 | 0.0653 | 0.0652 | 0.0973 | 0.0667 | 0.0615 | 0.0964 | 0.0621 | 0.0550 |
AME(\hat r^{non}, r) | 0.0506 | 0.0641 | 0.0619 | 0.0486 | 0.0649 | 0.0583 | 0.0430 | 0.0595 | 0.0518 |
AME(\hat r'^{lin}, r') | 0.6911 | 0.1876 | 0.2348 | 0.7021 | 0.2686 | 0.2451 | 0.6605 | 0.4102 | 0.2320 |
AME(\hat r'^{non}, r') | 0.3595 | 0.1862 | 0.2125 | 0.3450 | 0.2020 | 0.2229 | 0.3696 | 0.4198 | 0.2095 |
HeaviSine | Corner | Spikes | |||||||
r_{1} | r_{2} | r_{3} | r_{1} | r_{2} | r_{3} | r_{1} | r_{2} | r_{3} | |
MSE(\hat r^{lin}, r) | 0.0184 | 0.0073 | 0.0071 | 0.0189 | 0.0075 | 0.0064 | 0.0189 | 0.0069 | 0.0052 |
MSE(\hat r^{non}, r) | 0.0048 | 0.0068 | 0.0064 | 0.0044 | 0.0070 | 0.0057 | 0.0042 | 0.0061 | 0.0046 |
MSE(\hat r'^{lin}, r') | 0.7755 | 0.0547 | 0.0676 | 0.7767 | 0.1155 | 0.0737 | 0.7360 | 0.2566 | 0.0655 |
MSE(\hat r'^{non}, r') | 0.2319 | 0.0573 | 0.0560 | 0.2204 | 0.0644 | 0.0616 | 0.2406 | 0.2868 | 0.0539 |
AME(\hat r^{lin}, r) | 0.0935 | 0.0653 | 0.0652 | 0.0973 | 0.0667 | 0.0615 | 0.0964 | 0.0621 | 0.0550 |
AME(\hat r^{non}, r) | 0.0506 | 0.0641 | 0.0619 | 0.0486 | 0.0649 | 0.0583 | 0.0430 | 0.0595 | 0.0518 |
AME(\hat r'^{lin}, r') | 0.6911 | 0.1876 | 0.2348 | 0.7021 | 0.2686 | 0.2451 | 0.6605 | 0.4102 | 0.2320 |
AME(\hat r'^{non}, r') | 0.3595 | 0.1862 | 0.2125 | 0.3450 | 0.2020 | 0.2229 | 0.3696 | 0.4198 | 0.2095 |