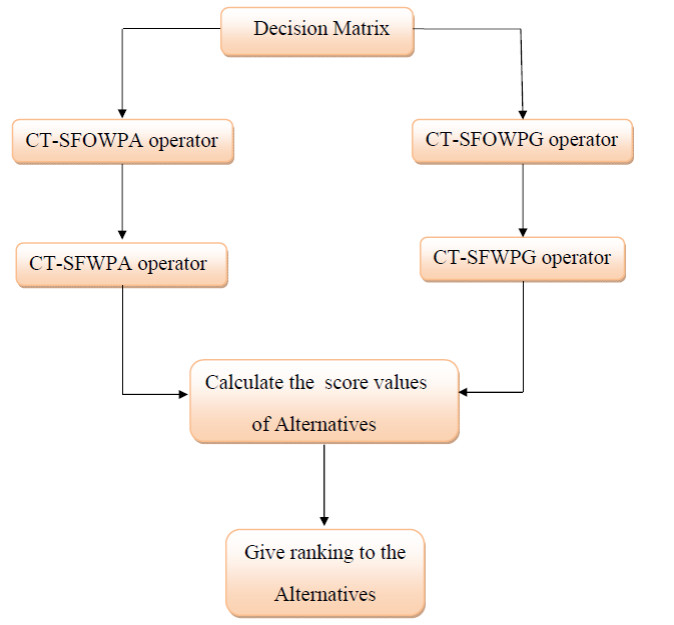
The goal of this research is to develop many aggregation operators for aggregating various complex T-Spherical fuzzy sets (CT-SFSs). Existing fuzzy set theory and its extensions, which are a subset of real numbers, handle the uncertainties in the data, but they may lose some useful information and so affect the decision results. Complex Spherical fuzzy sets handle two-dimensional information in a single set by covering uncertainty with degrees whose ranges are extended from the real subset to the complex subset with unit disk. Thus, motivated by this concept, we developed certain CT-SFS operation laws and then proposed a series of novel averaging and geometric power aggregation operators. The properties of some of these operators are investigated. A multi-criteria group decision-making approach is also developed using these operators. The method's utility is demonstrated with an example of how to choose the best choices, which is then tested by comparing the results to those of other approaches.
Citation: Muhammad Qiyas, Muhammad Naeem, Saleem Abdullah, Neelam Khan. Decision support system based on complex T-Spherical fuzzy power aggregation operators[J]. AIMS Mathematics, 2022, 7(9): 16171-16207. doi: 10.3934/math.2022884
[1] | Khawlah Alhulwah, Muhammad Azeem, Mehwish Sarfraz, Nasreen Almohanna, Ali Ahmad . Prioritized aggregation operators for Schweizer-Sklar multi-attribute decision-making for complex spherical fuzzy information in mobile e-tourism applications. AIMS Mathematics, 2024, 9(12): 34753-34784. doi: 10.3934/math.20241655 |
[2] | Muhammad Akram, Sumera Naz, Feng Feng, Ghada Ali, Aqsa Shafiq . Extended MABAC method based on 2-tuple linguistic T-spherical fuzzy sets and Heronian mean operators: An application to alternative fuel selection. AIMS Mathematics, 2023, 8(5): 10619-10653. doi: 10.3934/math.2023539 |
[3] | Hafiz Muhammad Athar Farid, Muhammad Riaz, Gustavo Santos Garcia . T-spherical fuzzy information aggregation with multi-criteria decision-making. AIMS Mathematics, 2023, 8(5): 10113-10145. doi: 10.3934/math.2023512 |
[4] | Muhammad Ali Khan, Saleem Abdullah, Alaa O. Almagrabi . Analysis of deep learning technique using a complex spherical fuzzy rough decision support model. AIMS Mathematics, 2023, 8(10): 23372-23402. doi: 10.3934/math.20231188 |
[5] | Ashraf Al-Quran . T-spherical linear Diophantine fuzzy aggregation operators for multiple attribute decision-making. AIMS Mathematics, 2023, 8(5): 12257-12286. doi: 10.3934/math.2023618 |
[6] | Muhammad Qiyas, Muhammad Naeem, Neelam Khan . Fractional orthotriple fuzzy Choquet-Frank aggregation operators and their application in optimal selection for EEG of depression patients. AIMS Mathematics, 2023, 8(3): 6323-6355. doi: 10.3934/math.2023320 |
[7] | Muhammad Naeem, Aziz Khan, Shahzaib Ashraf, Saleem Abdullah, Muhammad Ayaz, Nejib Ghanmi . A novel decision making technique based on spherical hesitant fuzzy Yager aggregation information: application to treat Parkinson's disease. AIMS Mathematics, 2022, 7(2): 1678-1706. doi: 10.3934/math.2022097 |
[8] | Muhammad Naeem, Muhammad Qiyas, Lazim Abdullah, Neelam Khan, Salman Khan . Spherical fuzzy rough Hamacher aggregation operators and their application in decision making problem. AIMS Mathematics, 2023, 8(7): 17112-17141. doi: 10.3934/math.2023874 |
[9] | Tahir Mahmood, Azam, Ubaid ur Rehman, Jabbar Ahmmad . Prioritization and selection of operating system by employing geometric aggregation operators based on Aczel-Alsina t-norm and t-conorm in the environment of bipolar complex fuzzy set. AIMS Mathematics, 2023, 8(10): 25220-25248. doi: 10.3934/math.20231286 |
[10] | Tehreem, Harish Garg, Kinza Ayaz, Walid Emam . Multi attribute decision-making algorithms using Hamacher Choquet-integral operators with complex intuitionistic fuzzy information. AIMS Mathematics, 2024, 9(12): 35860-35884. doi: 10.3934/math.20241700 |
The goal of this research is to develop many aggregation operators for aggregating various complex T-Spherical fuzzy sets (CT-SFSs). Existing fuzzy set theory and its extensions, which are a subset of real numbers, handle the uncertainties in the data, but they may lose some useful information and so affect the decision results. Complex Spherical fuzzy sets handle two-dimensional information in a single set by covering uncertainty with degrees whose ranges are extended from the real subset to the complex subset with unit disk. Thus, motivated by this concept, we developed certain CT-SFS operation laws and then proposed a series of novel averaging and geometric power aggregation operators. The properties of some of these operators are investigated. A multi-criteria group decision-making approach is also developed using these operators. The method's utility is demonstrated with an example of how to choose the best choices, which is then tested by comparing the results to those of other approaches.
Zadeh [61] defined fuzzy set (FS) theory. Fuzzy set theory is a incredible achievement with applications in a variety of fields. In every element of X (universal set) on [0,1] (closed interval), an FS is defined by σ, a membership degree (MD). After that, fuzzy set theory was extended to intuitionistic fuzzy sets by Atanassov [1] (IFS). An IFS is characterized by two functions, MD and non-membership degree (NMD), on the closed interval 0 to 1. Moreover, their sum must belong to [0,1], i.e., (membership degree (σ) + non-membership degree (υ)) ∈[0,1]. If we take σ=0.7 and υ=0.4 correspondingly, then their sum is greater than one. As a result of this constraint, Yager [57,58] developed the Pythagorean fuzzy set (PFS) concept of, expanding the domain of the IF set. A PFS is characterized by two functions, MD and NMD, on the closed interval 0 to 1, and the sum of the squares of MD and NMD is less than or equal to one, i.e., sum (σ2,υ2)∈[0,1]. As a result, Pythagorean fuzzy sets are the generalized form of IFSs, as the domain of PFSs is greater than that of IFSs. Khan et al. [21] defined Pythagorean fuzzy Dombi aggregation operators and their application in decision support system. Abdullah et al. [7] proposed Pythagorean cubic fuzzy Hamacher aggregation operators and their application in green supply selection problem.
While IFSs and PFSs can accurately define the ambiguous details, there are still challenges that IFSs and PFSs are unable to overcome. For example, when the expert selects 0.6 for MD and 0.9 for NMD, the condition of PFSs is such that 0.62+0.92=1.17>1. So, with regard to this condition, Yager [59] defined the definition of q-rung orthopair fuzzy sets (q-ROFSs), as being more effective than intuitionistic fuzzy sets and envisioning fuzzy sets to deal with challenging and ambiguous data. In addition, Liu & Wang [25] have developed q-ROF aggregation operators (AOs) to calculate the assessment details. Peng et al. [35] introduced the exponential operational laws and AOs for q-ROFSs. Qiyas et al. [48] defined similarity measures based on q-rung linear Diophantine fuzzy sets and discussed their application in multiple attribute decision making (MADM).
Ullah et al. [47] presented the concept of a T-Spherical fuzzy set (T-SPS) and defined some AOs. Ullah et al. [60] defined the Hamacher AOs for T-SFSs. Munir et al. [31] used Einstein hybrid AOs based on T-SFSs for solving MCGDM problems. Garg et al. [12] proposed several new T-SFS operators. The MADM problem was solved by Liu et al. [27] by defining the Muirhead mean operators for the T-SFS information. However, some algorithms are described in the literature (Ullah et al. [45,49]) to address pattern recognition and other problems using information measures such as similarity and correlation. Quek et al. [37] introduced some novel T-Spherical fuzzy set operational laws and obtained some of their properties. The Einstein interactive averaging AOs and the Einstein interactive geometric AOs are two types of Einstein AOs based on these new procedures. Ju et al. [19] created the T-SF interaction AOs and built the TODIM method in a T-Spherical fuzzy information using these operators. The MADM approach was used by Chen et al. [9] to investigate certain generalized T-Spherical and group-generalized fuzzy geometric aggregation operators. Guleria and Bajaj [17] defined various T-Spherical fuzzy soft set aggregation operations.
Inspired by the performance of the power operator, several forms of power aggregation operators (PAOs) have been introduced for different fuzzy settings, such as the generalized PAOs [52,56], intuitionistic fuzzy power AO [65], the interval-valued intuitionistic fuzzy power AO [23], Pythagorean fuzzy power AO [24,51] and hesitant fuzzy PAO [66]. Garg & Rani [13,41] provided weighted and powerful AOs to solve the MADM problem. Zhou, Chen & Liu suggested generalizing PAOs [63], and Zhou & Chen also introduced linguistic generalized power aggregation operators [64]. Xu [53] has defined the IF power AOs and interval-valued intuitionistic fuzzy power AOs. In addition, Xu & Cai [54] established an uncertain power ordered weighted averaging operator utilizing the PA (power averaging) operator and the uncertain ordered weighted averaging operator. Xu & Yager [52] have implemented an uncertain ordered weighted geometric operator using the power geometric operator and the UOW averaging operator. Xiong et al. [55] proposed an extended power average operator for decision-making, a case study in the emergency response plan selection of civil aviation. Chen et al. [10] defined a power-average operator based hybrid multi-attribute online product recommendation model for consumer decision-making.
Zhang et al. [62], mixed complex numbers and fuzzy sets and defined the concept of complex fuzzy sets (CFSs). Further, Ramot et al. [40] defined a new notion that is somewhat different from other studies, in which they expanded the range of MD to the unit circle in the complex plane, unlike the others that were limited, the generalized form of Zadeh fuzzy sets (FSs) [61]. A CFS is represented by a complex valued function, such as σℑ(x)=κℑ(x).e2πiΩκℑ(x) satisfying the condition: 0≤κℑ(x),Ωκℑ(x)≤1. The distinction between CFSs and FSs is that the CFSs range is not limited to [0,1] but spread in a complex plane to a unit disk. In FS theory, the CFSs information has earned more attention. Bi et al. [8] recently defined complex fuzzy geometrical AOs. Due to their merits and benefits, CFSs are extensively tested for problems in DM and other fields [32]. Since FSs and CFSs can only describe the MD and their complex-valued grade, they are unable to express the complex-valued negative degree of membership. Alkouri et al. [44] proposed the complex intuitionistic fuzzy set (CIFS) structure, which essentially consists of two complex membership functions that represent the positive and negative membership grades of an element. Ma et al. [30] defined the CFS idea for resolving the problems under multi-periodic factors. Dick et al. [11] discussed several CFSs, and Liu & Zhang [26] developed the results of Dick et al. [11]. Hu et al. [18] suggested some new distance steps for the CFS and investigated the consistency of CFS operations.
However, in some theories like fuzzy sets [61], intuitionistic fuzzy sets [1], and complex fuzzy sets [40], each element in these types of sets is represented as an ordered pair of MD and NMD with the ultimate aim of limiting their total to one [20,44]. Garg and Rani [13] suggested some CIFS aggregation operators and used these operators to solve a multi-attribute decision making (MADM) problem. The theory of power AOs for CIFSs was defined by Rani and Garg [41], which was later applied in MADM. Singh et al. [43] defined the interval-valued lattices of CFSs and their granular decomposition. Selvachandran et al. [42] suggested several similarity tests of CFSs, and their applications studied in pattern recognition. In [36], Quek and Selvachandran researched group-associated CIFS algebraic structures. Garg and Rani [14,15] developed Bonferroni mean operators and robust average and geometric AOs for CIFSs. Liu et al. [39] defined an approach for the MAGDM problem under Cq-ROFs linguistic information using the Heronian mean operators. Liu et al. [28] defined some AOs for complex q-rung orthopair fuzzy sets (Cq-ROFSs) and discussed their applications in MCGDM. Garg et al. [16] investigated the innovative approach of Cq-ROFSs, which are a combination of q-ROFSs and CFSs, for dealing with difficult and complicated information. Naeem et al. [34] defined complex Spherical fuzzy sets and applied them to a decision support system using entropy measure and a power operator. Karaaslan and Dawood [22] proposed CT-SF Dombi AOs and studied their applications in MCDM. Ali et al. [2] defined some AOs for CT-SFSs and discussed their application in the MADM problem. Akram et al. [3] presented a hybrid DM framework under complex Spherical fuzzy (CSF) prioritized weighted aggregation operators. Akram et al. [4] defined some extension of the TOPSIS model to the DM under CSF information. Akram et al. [5] proposed hybrid DM frameworks under complex Spherical fuzzy soft sets. Akram et al. [6] proposed complex Spherical Dombi fuzzy aggregation operators for decision-making.
In the CT-SFS theory, membership degree (MD), neutral membership degree (NuMD) and non-membership degree (NMD) are complex valued and are represented in polar coordinates. The amplitude term connected with the MD, NuMD and NMD gives the extent of an object's belongings in a CIFS, whereas the phase term associated with MD, NuMD and NMD gives extra information, which is generally related to periodicity. The phase terms are novel MD, NuMD and NMD parameters, and they are the parameters that distinguish the traditional T-SFS and CT-SFS theories. T-SFS theory deals with only one dimension at a time, while the CT-SFS deals with two dimensions at a time. CT-SFSs are very effective in representing the two dimensions of certain objects, as the phase term of a CT-SFS is used to capture the second dimension. In addition, T-SFSs are quite remarkable as a general model for fuzzy information, which becomes indispensable when neutral opinions occur. Thus, by introducing this second dimension, all of the information can be projected in a single set, avoiding information loss. The features of CFS theory and the T-SFS theory are combined with the CT-SFS theory.
We present the theory of PA operators among the CT-SFSs, maintaining the benefits of this collection and focusing on the value of aggregation operators. Based on our knowledge, operators cannot be used in the aforementioned studies to manage CT-SFS information. In order to do this, we first describe certain operation laws for CT-SFSs and analyze their basic properties. Next, some PA operators called CT-SFS power average, CT-SFS power geometric, CT-SFS weighted power average and geometric, as well as their respective ordered weighted operators, are proposed to aggregate the various complex T-Spherical fuzzy numbers (CT-SFNs). The basic features of these operators will be discussed in detail. In addition, we suggest a MCGDM method based on current CT-SFSs operators. Both the viability and the effectiveness of the strategy have been demonstrated by an illustrative example.
The rest of the manuscript is organized as follows: In Section 2, we summarize briefly the definitions of CFSs, PFSs, CPFSs, Cq-ROFSs and CT-SFSs. In Section 3, we propose some simple operational laws for CT-SFNs, and a series of averaging and geometric aggregation operators are developed based on the defined operational laws in Section 4. In Section 5, we describe a MCGDM approach using the developed operators with the CT-SFS information, where CT-SFNs are characterized by each element of the set. An example is described in Section 6, to show the functionality of the proposed method. Also, we compare our results with other results of current approaches, and finally this analysis is summarized in Section 7.
In this section, we give a brief literature review of existing concepts such as CFSs, CIFSs, CPFSs, Cq-ROFSs.
Definition 2.1. [40] A CFS C on X (universal set) is of the form:
C={⟨x,σC(x)⟩|x∈X}, | (2.1) |
where σC:U→{z:z∈C,|z|≤1} and σC(x)=a+ib=κC(x).e2πiΩκC(x). Here, κC(x)=√a2+b2∈R and κC(x),ΩC(x)∈[0,1], where i=√−1.
Definition 2.2. [44] A CIFS I on X is of the form:
I={⟨x,σI(x),υI(x)⟩|x∈X}, | (2.2) |
where σI:U→{z1:z1∈I,|z1|≤1}, υI:U→{z2:z2∈I,|z2|≤1}, such as σI(x)=z1=a1+ib1 and υI(x)=z2=a2+ib2 on the condition that 0≤|z1|+|z2|≤1 or σI(x)=κI(x).e2πiΩκI(x) and υi(x)=ξi(x).e2πiΩξI(x) satisfying the conditions 0≤κI(x)+ξI(x)≤1 and 0≤ΩκI(x)+ΩξI(x)≤1. The term HI(x)=R.e2πiΩR, such that R=1−(|z1|+|z2|), and ΩR(x)=1−(ΩκI(x)+ΩξI(x)) is considered a hesitancy degree of (x). Furthermore, I=(κ.e2πiΩκ,ξ.e2πiΩξ) is called the complex intuitionistic fuzzy number (CIFN).
Definition 2.3. [46] A CPFS P on X is of the form:
P={⟨x,σP(x),υP(x)⟩|x∈X}, | (2.3) |
where σP:U→{z1:z1∈P,|z1|≤1}, υP:U→{z2:z2∈P,|z2|≤1}, such as σP(x)=z1=a1+ib1 and υP(x)=z2=a2+ib2 on the condition that 0≤|z1|2+|z2|2≤1 or σP(x)=κP(x).e2πiΩκP(x) and υP(x)=ξP(x).e2πiΩξP(x) satisfy the condition 0≤κ2P(x)+ξ2P(x)≤1, and 0≤Ω2κP(x)+Ω2ξP(x)≤1. The term HP(x)=R.e2πiΩR, such that R=√1−(κ2P(x)+ξ2P(x)) and ΩR(x)=√1−(Ω2κP(x)+Ω2ξP(x)) is considered a hesitancy degree of x. Furthermore, P=(κ.e2πiΩκ,ξ.e2πiΩξ) is called the complex Pythagorean fuzzy number (CPFN). CPFS positive and negative membership grades are clearly polar or Cartesian numbers in complex form.
Definition 2.4. [28] A Cq-ROFS S on X is of the form
S={⟨x,σS(x),υS(x)⟩|x∈X}, | (2.4) |
where σS:U→{z1:z1∈S,|z1|≤1}, and υS:U→{z2:z2∈S,|z2|≤1}, such that σS(x)=z1=a1+ib1 and υS(x)=z2=a2+ib2, on the condition that 0≤|z1|q+|z2|q≤1 or σS(x)=κS(x).e2πiΩκS(x), and υS(x)=ξS(x).e2πiΩξS(x) satisfying the condition 0≤κqS(x)+ξqS(x)≤1 and 0≤ΩqκS(x)+ΩqξS(x)≤1. The term HS(x)=R.e2πiΩR, such that R=(1−κqS(x)−ξqS(x))1q and ΩR(x)=(1−(ΩqκS(x)+ΩqξS(x)))1q is considered a hesitancy degree of x. Furthermore, S=(κ.e2πiΩκ,ξ.e2πiΩξ) is called the complex q-rung orthopair fuzzy number (Cq-ROFN).
Definition 2.5. [56] For a family of Φi(i=1,...,n), the power average operator is defined as:
PA(Φ1,...,Φn)=n∑i=1(1+T(Φi))Φin∑i=1(1+T(Φi)), | (2.5) |
where T(Φi)=∑ni=1Sup(Φi,Φs), and Sup(Φi,Φs) is the support of Φi from Φs and known as the similarity index, which satisfies the below properties:
(1) Sup(Φi,Φs)∈[0,1];
(2) Sup(Φi,Φs)= Sup(Φs,Φi);
(3) Sup(Φi,Φs)≥ Sup(Φk,Φl), if |Φi−Φs|≤|Φk−Φl|.
CT-SFSs and their basic operation laws for the collection of CT-SFNs, as well as their corresponding PA operators, are introduced in this section.
Definition 3.1. [2] A CT-SFS S on X is of the form
S={⟨x,σS(x),υS(x),χS(x)⟩|x∈X}, | (3.1) |
where σS:U→{z1:z1∈S,|z1|≤1},υS:U→{z2:z2∈S,|z2|≤1}, and χS:U→{z3:z3∈S,|z3|≤1} such that σS(x)=z1=a1+ib1, υS(x)=z2=a2+ib2, χS(x)=z3=a3+ib3, on the condition that 0≤|z1|q+|z2|q+|z3|q≤1 or σS(x)=κS(x).e2πiΩκS(x), υS(x)=ξS(x).e2πiΩξS(x) and χ(x)=ϕS(x).e2πiΩϕS(x) satisfying the conditions; 0≤κqS(x)+ξqS(x)+ϕqS(x)≤1 and 0≤ΩqκS(x)+ΩqξS(x)+ΩqϕS(x)≤1. The term HS(x)=R.e2πiΩR, such that R=(1−κqS(x)−ξqS(x)−ϕqS(x))1q, and ΩR(x)=(1−(ΩqκS(x)+ΩqξS(x)+ΩqϕS(x)))1q is considered a hesitancy degree of x. Furthermore, S=(κ.e2πiΩκ,ξ.e2πiΩξ,ϕ.e2πiΩϕ) is called the complex T-Spherical fuzzy number (CT-SFN).
Definition 3.2. For CT-SFN Υ={(κΥ,ΩκΥ),(ξΥ,ΩξΥ),(ϕΥ,ΩϕΥ)}, the score function Sc∗ of Υ is defined as
Sc∗(Υ)=12|(κqΥ−ξqΥ−ϕqΥ)+(ΩqκΥ−ΩqξΥ−ΩqϕΥ)|, | (3.2) |
and the accuracy function Hc∗ of Υ is defined as:
Hc∗(Υ)=12|(κqΥ+ξqΥ+ϕqΥ)+(ΩqκΥ+ΩqξΥ+ΩqϕΥ)|, | (3.3) |
where Sc∗(Υ)∈[0,1] and Hc∗(Υ)∈[0,1].
Definition 3.3. The following comparison rules between two CT-SFNs Υ1 and Υ2 are satisfied:
(1) If Sc∗(Υ1)>Sc∗(Υ2), then Υ1>Υ2;
(2) If Sc∗(Υ1)=Sc∗(Υ2),
(3) If Hc∗(Υ1)>Hc∗(Υ2), then Υ1>Υ2;
(4) If Hc∗(Υ1)=Hc∗(Υ2), then Υ1=Υ2.
Definition 3.4. For any two CT-SFNs Υ1={(κΥ1,ΩκΥ1),(ξΥ1,ΩξΥ1),(ϕΥ1,ΩϕΥ1)} and
Υ2={(κΥ2,ΩκΥ2),(ξΥ2,ΩξΥ2),(ϕΥ2,ΩϕΥ2)}, the distance measure is defined as:
d(Υ1,Υ2)=14[|κqΥ1−κqΥ2|+|ξqΥ1−ξqΥ2|+|ϕqΥ1−ϕqΥ2|+12π(|ΩqκΥ1−ΩqκΥ2|+|ΩqξΥ1−ΩqξΥ2|+|ΩqϕΥ1−ΩqϕΥ2|)]. | (3.4) |
Definition 3.5. For any two CT-SFNs Υ1={(κΥ1,ΩκΥ1),(ξΥ1,ΩξΥ1),(ϕΥ1,ΩϕΥ1)} and
Υ2={(κΥ2,ΩκΥ2),(ξΥ2,ΩξΥ2),(ϕΥ2,ΩϕΥ2)} and λ>0, the basic operation laws are defined as:
(1) Υ1⊕Υ2={(q√1−2∏i=1(1−κqΥi),2π(1−2∏i=1(1−ΩqκΥi2π))),(2∏i=1ξΥi,2π(2∏i=1ΩξΥi2π)),(2∏i=1ϕΥi,2π(2∏i=1ΩϕΥi2π))};
(2) Υ1⊗Υ2={(2∏i=1κΥi,2π(2∏i=1ΩκΥi2π)),(q√1−2∏i=1(1−ξqΥi),2π(1−2∏i=1(1−ΩqξΥi2π))),(q√1−2∏i=1(1−ϕqΥi),2π(1−2∏i=1(1−ΩqϕΥi2π)))};
(3) λΥ1={(q√1−(1−κqΥ1)λ,2π(1−(1−ΩqκΥ12π)λ)),(ξλΥ1,2π(ΩξΥ12π)λ),(ϕλΥ1,2π(ΩϕΥ12π)λ)};
(4) Υλ1={(κλΥ1,2π(ΩκΥ12π)λ),(q√1−(1−ξqΥ1)λ,2π(1−(1−ΩqξΥ12π)λ)),(q√1−(1−ϕqΥ1)λ,2π(1−(1−ΩqϕΥ12π)λ))}.
Theorem 3.1. Let Υ1,Υ2,Υ3 be any three CT-SFNs. Then, the following properties are satisfied.
(1) Υ1⊕Υ2=Υ2⊕Υ1;
(2) Υ1⊗Υ2=Υ2⊗Υ1;
(3) (Υ1⊕Υ2)⊕Υ3=Υ1⊕(Υ2⊕Υ3);
(4) (Υ1⊗Υ2)⊗Υ3=Υ1⊗(Υ2⊗Υ3).
Proof. Here, we prove parts (1) and (3), and the proofs of the others are similar.
(1) As Υ1={(κΥ1,ΩκΥ1),(ξΥ1,ΩξΥ1),(ϕΥ1,ΩϕΥ1)} and
Υ2={(κΥ2,ΩκΥ2),(ξΥ2,ΩξΥ2),(ϕΥ2,ΩϕΥ2)} are the two CT-SFNs, then we have
Υ1⊕Υ2={(q√1−2∏i=1(1−κqΥi),2π(1−2∏i=1(1−ΩqκΥi2π))),(2∏i=1ξΥi,2π(2∏i=1ΩξΥi2π)),(2∏i=1ϕΥi,2π(2∏i=1ΩϕΥi2π))}={(q√κqΥ1+κqΥ2−κqΥ1κqΥ2,2π(ΩqκΥ12π+ΩqκΥ22π−ΩqκΥ12πΩqκΥ22π)),(ξΥ1ξΥ2,2π(ΩξΥ12πΩξΥ22π)),(ϕΥ1ϕΥ2,2π(ΩϕΥ12πΩϕΥ22π))}={(q√κqΥ2+κqΥ1−κqΥ2κqΥ1,2π(ΩqκΥ22π+ΩqκΥ12π−ΩqκΥ22πΩqκΥ12π)),(ξΥ1ξΥ2,2π(ΩξΥ22πΩξΥ12π)),(ϕΥ1ϕΥ2,2π(ΩϕΥ22πΩϕΥ12π))}=Υ2⊕Υ1 |
(3) As Υ1={(κΥ1,ΩκΥ1),(ξΥ1,ΩξΥ1),(ϕΥ1,ΩϕΥ1)},
Υ2={(κΥ2,ΩκΥ2),(ξΥ2,ΩξΥ2),(ϕΥ2,ΩϕΥ2)} and
Υ3={(κΥ3,ΩκΥ3),(ξΥ3,ΩξΥ3),(ϕΥ3,ΩϕΥ3)} are the three CT-SFNs, we have
(Υ1⊕Υ2)⊕Υ3={(q√1−2∏i=1(1−κqΥi),2π(1−2∏i=1(1−ΩqκΥi2π))),(2∏i=1ξΥi,2π(2∏i=1ΩξΥi2π)),(2∏i=1ϕΥi,2π(2∏i=1ΩϕΥi2π))}⊕((κΥ3,ΩκΥ3),(ξΥ3,ΩξΥ3),(ϕΥ3,ΩϕΥ3))={(q√1−3∏i=1(1−κqΥi),2π(1−3∏i=1(1−ΩqκΥi2π))),(3∏i=1ξΥi,2π(3∏i=1ΩξΥi2π)),(3∏i=1ϕΥi,2π(3∏i=1ΩϕΥi2π))}={(κΥ1,ΩκΥ1),(ξΥ1,ΩξΥ1),(ϕΥ1,ΩϕΥ1)}⊕{(q√1−3∏i=2(1−κqΥi),2π(1−3∏i=2(1−ΩqκΥi2π))),(3∏i=2ξΥi,2π(3∏i=2ΩξΥi2π)),(3∏i=2ϕΥi,2π(3∏i=2ΩϕΥi2π))}=Υ1⊕(Υ2⊕Υ3). |
Theorem 3.2. For any two CT-SFNs Υ1 and Υ2 and λ,λ1,λ2 (positive real numbers), we have
(1) λ(Υ1⊕Υ2)=λΥ1⊕λΥ2;
(2) (Υ1⊗Υ2)λ=Υλ1⊕Υλ2;
(3) (λ1+λ2)Υ1=λ1Υ1⊕λ2Υ1;
(4) Υλ1+λ21=Υλ11⊗Υλ21.
Proof. Here, we have proven only parts (1) and (3), and the proofs of other parts are similar.
As given that Υ1 and Υ2 are CT-SFNs, we have
λ(Υ1⊕Υ2)=λ{(q√1−2∏i=1(1−κqΥi),2π(1−2∏i=1(1−ΩqκΥi2π))),(2∏i=1ξΥi,2π(2∏i=1ΩξΥi2π)),(2∏i=1ϕΥi,2π(2∏i=1ΩϕΥi2π))}={(q√1−2∏i=1(1−κqΥi)λ,2π(1−2∏i=1(1−ΩqκΥi2π)λ)),(2∏i=1ξλΥi,2π(2∏i=1ΩξΥi2π)λ),(2∏i=1ϕλΥi,2π(2∏i=1ΩϕΥi2π)λ)}={(q√1−(1−κqΥ1)λ,2π(1−(1−ΩqκΥ12π)λ)),(ξλΥ1,2π(ΩξΥ12π)λ),(ϕλΥ1,2π(ΩϕΥ12π)λ)⊕(q√1−(1−κqΥ2)λ,2π(1−(1−ΩqκΥ22π)λ)),(ξλΥ2,2π(ΩξΥ22π)λ),(ϕλΥ2,2π(ΩϕΥ22π)λ)}=λΥ1⊕λΥ2. |
Hence, λ(Υ1⊕Υ2)=λΥ1⊕λΥ2.
(3) From the operational laws we can write given,
(λ1+λ2)Υ1={(q√1−(1−κqΥ1)λ1+λ2,2π(1−(1−ΩqκΥ12π)λ1+λ2)),(ξλ1+λ2Υ1,2π(ΩξΥ12π)λ1+λ2),(ϕλ1+λ2Υ1,2π(ΩϕΥ12π)λ1+λ2)}={(q√1−(1−κqΥ1)λ1,2π(1−(1−ΩqκΥ12π)λ1)),(ξλ1Υ1,2π(ΩξΥ12π)λ1),(ϕλ1Υ1,2π(ΩϕΥ12π)λ1)⊕{(q√1−(1−κqΥ1)λ2,2π(1−(1−ΩqκΥ12π)λ2)),(ξλ2Υ1,2π(ΩξΥ12π)λ2),(ϕλ2Υ1,2π(ΩϕΥ12π)λ2)}}=λ1Υ1⊕λ2Υ1. |
Several power averaging (PA) operators are introduced with the CT-SF information in this section, using the defined operation laws of the CT-SFNs, in order to aggregate the CT-SFNs.
Definition 4.1. For a family of CT-SFNs Υi={(κΥi,ΩκΥi),(ξΥi,ΩξΥi),(ϕΥi,ΩϕΥi)}(i=1,...,n), the complex T-Spherical fuzzy power averaging (CT-SFPA) aggregation operator is a function CT-SFPA:Ωn→Ω defined by
CT−SFPA(Υ1,...,Υn)=ρ1Υ1⊕...⊕ρnΥn, | (4.1) |
where ρi=1+T(Υi)∑ni=1(1+T(Υi)) and T(Υi)=n∑s=is≠i(Sup(Υi,Υs))(i=1,...,n). Here, Sup(Υi,Υs) is the support of Υi from Υs satisfying the aforementioned properties, and Sup(Υi,Υs)=1−d(Υi,Υs), where d is the distance measure defined in Definition (3.1).
Theorem 4.1. Let a family of CT-SFNs be Υi={(κΥi,ΩκΥi),(ξΥi,ΩξΥi),(ϕΥi,ΩϕΥi)}(i=1,...,n). Then, the aggregated value obtained by using the CT-SFPA operator is also a CT-SFN and is given as follows.
CT−SFPA(Υ1,...,Υn)={(q√1−n∏i=1(1−κqΥi)ρi,2π(1−n∏i=1(1−ΩqκΥi2π)ρi)),(n∏i=1ξρiΥi,2π(n∏i=1(ΩξΥi2π)ρi)),(n∏i=1ϕρiΥi,2π(n∏i=1(ΩϕΥi2π)ρi))} | (4.2) |
Proof. To prove Eq (4.2), we use mathematical induction. For each i,Υi is a CT-SFN and Υi>0. Therefore, we have ρiΥi is again a CT-SFN. Then, applying the mathematical induction steps on n, we obtain the following.
(1) For n=2, we get Υ1={(κΥ1,ΩκΥ1),(ξΥ1,ΩξΥ1),(ϕΥ1,ΩϕΥ1)} and Υ2={(κΥ2,ΩκΥ2),(ξΥ2,ΩξΥ2),(ϕΥ2,ΩϕΥ2)}. As a result of the CT-SFNs operating law, we have the following.
ρ1Υ1={(q√1−(1−κqΥ1)ρ1,2π(1−(1−ΩqκΥ12π)ρ1)),(ξρ1Υ1,2π(ΩξΥ12π)ρ1),(ϕρ1Υ1,2π(ΩϕΥ12π)ρ1)}, |
and
ρ2Υ2={(q√1−(1−κqΥ2)ρ2,2π(1−(1−ΩqκΥ22π)ρ2)),(ξρ2Υ2,2π(ΩξΥ22π)ρ2),(ϕρ2Υ2,2π(ΩϕΥ22π)ρ2)} |
As a result, using the CT-SFNs addition law, we have the following.
CT−SFPA(Υ1,Υ2)={(q√1−(1−κqΥ1)ρ1,2π(1−(1−ΩqκΥ12π)ρ1)),(ξρ1Υ1,2π(ΩξΥ12π)ρ1),(ϕρ1Υ1,2π(ΩϕΥ12π)ρ1)}⊕{(q√1−(1−κqΥ2)ρ2,2π(1−(1−ΩqκΥ22π)ρ2)),(ξρ2Υ2,2π(ΩξΥ22π)ρ2),(ϕρ2Υ2,2π(ΩϕΥ22π)ρ2)}={(q√1−2∏i=1(1−κqΥi)ρi,2π(1−2∏i=1(1−ΩqκΥi2π)ρi)),(2∏i=1ξρiΥi,2π(2∏i=1(ΩξΥi2π)ρi)),(2∏i=1ϕρiΥi,2π(2∏i=1(ΩϕΥi2π)ρi))} |
Thus, the result holds for n=2.
(2) Let Eq (4.2) be true for n=k, i.e., the following.
CT−SFPA(Υ1,Υ2)={(q√1−k∏i=1(1−κqΥi)ρi,2π(1−k∏i=1(1−ΩqκΥi2π)ρi)),(k∏i=1ξρiΥi,2π(k∏i=1(ΩξΥi2π))ρi),(k∏i=1ϕρiΥi,2π(k∏i=1(ΩϕΥi2π))ρi)} |
Then, n=k+1, and we get the following.
CT−SFPA(Υ1,...,Υk+1)=CT−SFPA(Υ1,...,Υk)⊕CT−SFPA(Υk+1)={(q√1−k∏i=1(1−κqΥi)ρi,2π(1−k∏i=1(1−ΩqκΥi2π)ρi)),(k∏i=1ξρiΥi,2π(k∏i=1(ΩξΥi2π)ρi)),(k∏i=1ϕρiΥi,2π(k∏i=1(ΩϕΥi2π)ρi))}⊕{(q√1−(1−κqΥk+1)ρk+1,2π(1−(1−ΩqκΥk+12π)ρk+1)),(ξρk+1Υk+1,2π(ΩξΥk+12π)ρk+1),(ϕρk+1Υk+1,2π(ΩϕΥk+12π)ρk+1)}={(q√1−k+1∏i=1(1−κqΥi)ρi,2π(1−k+1∏i=1(1−ΩqκΥi2π)ρi)),(k+1∏i=1ξρiΥi,2π(k+1∏i=1(ΩξΥi2π)ρi)),(k+1∏i=1ϕρiΥi,2π(k+1∏i=1(ΩϕΥi2π)ρi))} |
Thus, Eq (4.2) is true for all positive natural numbers n.
The CT-SFPA operator satisfies some of the properties listed below, according to Theorem (4.1).
Property 1 (Idempotency). Let Υ0 be a CT-SFN, and if Υi=Υ0 for all i=1,...,n, then
CT−SFPA(Υ1,...,Υn)=Υ0 | (4.3) |
Proof. Let Υ0={(κΥ0,ΩκΥ0),(ξΥ0,ΩξΥ0),(ϕΥ0,ΩϕΥ0)} and
Υi={(κΥi,ΩκΥi),(ξΥi,ΩξΥi),(ϕΥi,ΩϕΥi)} be the CT-SFNs, such that Υi=Υ0 for all i, where κΥi=κΥ0,ξΥi=ξΥ0,ϕΥi=ϕΥ0,ΩκΥi=ΩκΥ0,ΩξΥi=ΩξΥ0 and ΩϕΥi=ΩϕΥ0 for all i. Then, based on the ρi, we have ∑ni=1ρi=1. So, by Theorem (4.1), we get
CT−SFPA(Υ1,...,Υn)={(q√1−n∏i=1(1−κqΥ0)ρi,2π(1−n∏i=1(1−ΩqκΥ02π)ρi)),(n∏i=1ξρiΥ0,2π(n∏i=1(ΩξΥ02π)ρi)),(n∏i=1ϕρiΥ0,2π(n∏i=1(ΩϕΥ02π)ρi))}={(q√1−(1−κqΥ0)n∑i=1ρi,2π(1−(1−ΩqκΥ02π)n∑i=1ρi)),(ξρiΥ0,2π(ΩξΥ02π)n∑i=1ρi),(ϕρiΥ0,2π(ΩϕΥ02π)n∑i=1ρi)}={(κΥ0,ΩκΥ0),(ξΥ0,ΩξΥ0),(ϕΥ0,ΩϕΥ0)}=Υ0 |
Property 2 (Boundedness). Let Υi={(κΥi,ΩκΥi),(ξΥi,ΩξΥi),(ϕΥi,ΩϕΥi)}(i=1,...,n) be the family of CT-SFNs, and Υ−={mini(κΥi),mini(ΩκΥi),mini(ξΥi),mini(ΩξΥi),maxi(ϕΥi),maxi(ΩϕΥi)} and
Υ+={maxi(κΥi),maxi(ΩκΥi),mini(ξΥi),mini(ΩξΥi),mini(ϕΥi),mini(ΩϕΥi)}. Then,
Υ−≤CT−SFPA(Υ1,...,Υn)≤Υ+. | (4.4) |
Proof. Take Υ≤CT−SFPA(Υ1,...,Υn), and hence by Theorem (4.1), we get Υ={(κΥ,ΩκΥ),(ξΥ,ΩξΥ),(ϕΥ,ΩϕΥ)}. For a CT-SFN Υi, we have
mini(κΥi)≤κΥi≤maxi(κΥi)⇒1−maxi(κΥi)≤1−(κΥi)≤1−mini(κΥi)⇒(1−maxi(κΥi))ρi≤(1−Υi)ρi≤(1−mini(κΥi))ρi⇒n∏i=1(1−maxi(κΥi))ρi≤n∏i=1(1−κΥi)ρi≤n∏i=1(1−mini(κΥi))ρi⇒(1−maxi(κΥi))n∑i=1ρi≤n∏i=1(1−κΥi)ρi≤(1−mini(κΥi))n∑i=1ρi⇒1−maxi(κΥi)≤n∏i=1(1−κΥi)ρi≤1−mini(κΥi)⇒mini(κΥi)≤1−n∏i=1(1−κΥi)ρi≤maxi(κΥi)⇒mini(κΥi)≤κΥ≤maxi(κΥi), |
and
mini(ξΥi)≤ξΥi≤maxi(ξΥi)⇒(mini(ξΥi))ρi≤(ξΥi)ρi≤(maxi(ξΥi))ρi⇒n∏i=1(mini(ξΥi))ρi≤n∏i=1(ξΥi)ρi≤n∏i=1(maxi(ξΥi))ρi⇒(mini(ξΥi))n∑i=1ρi≤n∏i=1(ξΥi)ρi≤(maxi(ξΥi))n∑i=1ρimini(ϕΥi)≤ϕΥi≤maxi(ϕΥi)⇒(mini(ϕΥi))ρi≤(ϕΥi)ρi≤(maxi(ϕΥi))ρi⇒n∏i=1(mini(ϕΥi))ρi≤n∏i=1(ϕΥi)ρi≤n∏i=1(maxi(ϕΥi))ρi⇒(mini(ϕΥi))n∑i=1ρi≤n∏i=1(ϕΥi)ρi≤(maxi(ϕΥi))n∑i=1ρi |
⇒mini(κΥi)≤n∏i=1(κΥi)ρi≤maxi(κΥi),⇒mini(ξΥi)≤ξΥ≤maxi(ξΥi),⇒mini(ϕΥi)≤ϕΥ≤maxi(ϕΥi). |
Similarly, we obtain mini(ΩκΥi)≤ΩκΥ≤maxi(ΩκΥi), mini(ΩξΥi)≤ΩξΥ≤maxi(ΩξΥi) and mini(ΩϕΥi)≤ΩϕΥ≤maxi(ΩϕΥi). Now, by using Definition (3.1), we get the following.
Sc∗(Υ)=12|(κqΥ−ξqΥ−ϕqΥ)+(ΩqκΥ−ΩqξΥ−ΩqϕΥ)|≤(maxi(κΥi)−mini(ξΥi)−mini(ϕΥi))+12(maxi(ΩκΥi)−mini(ΩξΥi)−mini(ΩϕΥi))=Sc∗(Υ−) |
Sc∗(Υ)=12|(κqΥ−ξqΥ−ϕqΥ)+(ΩqκΥ−ΩqξΥ−ΩqϕΥ)|≥(mini(κΥi)−maxi(ξΥi)−maxi(ϕΥi))+12(mini(ΩκΥi)−maxi(ΩξΥi)−maxi(ΩϕΥi))=Sc∗(Υ+) |
Thus, Sc∗(Υ−)≤Sc∗(Υ)≤Sc∗(Υ+).
Hence, by the ranking order, we have
Υ_≤CT−SFPA(Υ1,...,Υn)≤Υ+. |
Property 3 (Commutivity). Let Υi={(κΥi,ΩκΥi),(ξΥi,ΩξΥi),(ϕΥi,ΩϕΥi)}(i=1,...,n) be the family of CT-SFNs, if (Υ∗1,...,Υ∗n) are the permutations of (Υ1,...,Υn). Then,
CT−SFPA(Υ1,...,Υn)=CT−SFPA(Υ∗1,...,Υ∗n). | (4.5) |
Proof. (Υ∗1,...,Υ∗n) is an arbitrary arrangement of (Υ1,...,Υn). Therefore,
CT−SFPA(Υ1,...,Υn)=n∑i=1(1+T(Υi))Υin∑i=1(1+T(Υi))=n∑i=1(1+T(Υ∗i))Υ∗in∑i=1(1+T(Υ∗i))=CT−SFPA(Υ∗1,...,Υ∗n) |
Thus, CT−SFPA(Υ1,...,Υn)=CT−SFPA(Υ∗1,...,Υ∗n).
Property 4 (Monotonicity). Let Υi={(κΥi,ΩκΥi),(ξΥi,ΩξΥi),(ϕΥi,ΩϕΥi)} and
Υ∗i={(κ∗Υi,Ω∗κΥi),(ξ∗Υi,Ω∗ξΥi),(ϕ∗Υi,Ω∗ϕΥi)}(i=1,...,n) be the families of CT-SFNs, such that Υi≤Υ∗i. Then,
CT−SFPA(Υ1,...,Υn)≤CT−SFPA(Υ∗1,...,Υ∗n) | (4.6) |
Proof. It's given that κΥi≤κ∗Υi,ΩκΥi≤Ω∗κΥi, ξΥi≤ξ∗Υi,ΩξΥi≤Ω∗ξΥi, ϕΥi≤ϕ∗Υi and ΩϕΥi≤Ω∗ϕΥi for all i. Then,
1−κ∗Υi≤1−κΥin∏i=1(1−κqΥi)ρi≤1−n∏i=1(1−κ∗qΥi)ρi⟹q√(1−n∏i=1(1−κqΥi)ρi)≤q√(1−n∏i=1(1−κ∗qΥi)ρi) |
and
1−Ω∗κΥi≤1−ΩκΥin∏i=1(1−ΩqκΥi)ρi≤1−n∏i=1(1−Ω∗qκΥi)ρi⟹q√(1−n∏i=1(1−ΩqκΥi)ρi)≤q√(1−n∏i=1(1−Ω∗qκΥi)ρi) |
Similarly, we can show that ξΥi≤ξ∗Υi,ΩξΥi≤Ω∗ξΥi, ϕΥi≤ϕ∗Υi and ΩϕΥi≤Ω∗ϕΥi. Thus, we obtain
{(q√1−n∏i=1(1−κqΥi)ρi,2π(1−n∏i=1(1−ΩqκΥi2π)ρi)),(n∏i=1ξρiΥi,2π(n∏i=1(ΩξΥi2π)ρi)),(n∏i=1ϕρiΥi,2π(n∏i=1(ΩϕΥi2π)ρi))}≤{(q√1−n∏i=1(1−κ∗qΥi)ρi,2π(1−n∏i=1(1−Ω∗qκΥi2π)ρi)),(n∏i=1ξ∗ρiΥi,2π(n∏i=1(Ω∗ξΥi2π)ρi)),(n∏i=1ϕ∗ρiΥi,2π(n∏i=1(Ω∗ϕΥi2π)ρi))}. |
Hence, from the above Equation we prove that, CT−SFPA(Υ1,...,Υn)≤CT−SFPA(Υ∗1,...,Υ∗n).
In this part, we consider the distinct weighting vector of the CT-SFNs Υi(i=1,...,n) during the aggregation process, as opposed to the above-mentioned CT-SFPA operator, and developed a modified CT-SF weighted power averaging (CT-SFWPA) aggregation operator as follows.
Definition 4.2. For a family of CT-SFNs Υi(i=1,...,n), the CT-SFWPA operator is a function CT−SFWPA:Ωn→Ω defined by:
CT−SFWPA(Υ1,...,Υn)=ϕ1Υ1⊕...⊕ϕnΥn | (4.7) |
where ϕi=ψi(1+T/(Υi))∑ni=1ψi(1+T/(Υi)),T/(Υi)=n∑s=1s≠iψs(Sup(Υi,Υs)) and ψ=(ψ1,...,ψn)T be the weight vector of CT-SFNs Υi, such that ψi>0 and ∑ni=1ψi=1.
Theorem 4.2. Let a family of CT-SFNs Υi={(κΥi,ΩκΥi),(ξΥi,ΩξΥi),(ϕΥi,ΩϕΥi)}(i=1,...,n), with their corresponding weight vector ψ=(ψ1,...,ψn)T, such that ψi>0 and ∑ni=1ψi=1. Then, the aggregated value obtained by using the CT-SFWPA operator is again a CT-SFN, and it is given as follows.
CT−SFWPA(Υ1,...,Υn)={(q√1−n∏i=1(1−κqΥi)ϕi,2π(1−n∏i=1(1−ΩqκΥi2π)ϕi)),(n∏i=1ξϕiΥi,2π(n∏i=1(ΩξΥi2π)ϕi)),(n∏i=1ϕϕiΥi,2π(n∏i=1(ΩϕΥi2π)ϕi))} | (4.8) |
Proof. The proof is the same as that of Theorem (4.1).
For a family of CT-SFNs Υi(i=1,...,n) with weight vector ψ=(ψ1,...,ψn)T, such that ψi>0 and ∑ni=1ψi=1, the CT-SFWPA operator satisfies the same properties as the CT-SFPA operator, such as the following.
Property 1 (Idempotency). Let Υ0 be a CT-SFN, and if Υi=Υ0 for all i=1,...,n, then,
CT−SFWPA(Υ1,...,Υn)=Υ0. | (4.9) |
Property 2 (Boundedness). Let Υ− and Υ+ be the lower bound and upper bound of the CT-SFNs Υi(i=1,...,n), respectively. Then, we have,
Υ−≤CT−SFWPA(Υ1,...,Υn)≤Υ+. | (4.10) |
Property 3 (Commutivity). For a permutation (Υ∗1,...,Υ∗n) of CT-SFNs (Υ1,...,Υn) and their corresponding permutation weights ψ∗=(ψ∗1,...,ψ∗n)T, ψ=(ψ1,...,ψn)T, we have
CT−SFWPA(Υ1,...,Υn)=CT−SFWPA(Υ∗1,...,Υ∗n). | (4.11) |
Property 4 (Monotonicity). Let Υi={(κΥi,ΩκΥi),(ξΥi,ΩξΥi),(ϕΥi,ΩϕΥi)} and
Υ∗i={(κ∗Υi,Ω∗κΥi),(ξ∗Υi,Ω∗ξΥi),(ϕ∗Υi,Ω∗ϕΥi)}(i=1,...,n) be the families of CT-SFNs, such that Υi≤Υ∗i. Then,
CT−SFWPA(Υ1,...,Υn)≤CT−SFWPA(Υ∗1,...,Υ∗n) | (4.12) |
The defined aggregation operator has been extended to its ordered weighted form in this subsection.
Definition 4.3. For a family of CT-SFNs Υi(i=1,...,n), a complex T-Spherical fuzzy ordered weighted power averaging (CT-SFOWPA) operator is a function CT−SFOWPA:Ωn→Ω defined as:
CT−SFOWPA(Υ1,...,Υn)=ζ1Υσ(1)⊕...⊕ζnΥσ(n), | (4.13) |
where Ω denotes the set of CT-SFNs, and σ(1),...,σ(n) are the permutations of (1,...,n) satisfies that Υσ(i−1)≥Υσ(i) for i=2,...,n. Also, ζi is defined as
ζi=g(BiTV)−g(Bi−1TV), | (4.14) |
where Bi=∑is=1Vσ(s),Vσ(i)=1+n∑s=1s≠i(Sup(Υi,Υs)),TV=∑ni=1Vσ(i) and the mapping g:[0,1]→[0,1] is a basic unit-interval monotonic function that satisfies the three properties g(0)=0,g(1)=1, and if x≤y then g(x)≤g(y).
Theorem 4.3. Let a family of CT-SFNs be Υi={(κΥi,ΩκΥi),(ξΥi,ΩξΥi),(ϕΥi,ΩϕΥi)}(i=1,...,n). Then, the aggregated value calculated by using the CT-SFOWPA operator is again a CT-SFN and given as
CT−SFOWPA(Υ1,...,Υn)={(q√1−n∏i=1(1−κqΥσ(i))ζi,2π(1−n∏i=1(1−ΩqκΥσ(i)2π)ζi)),(n∏i=1ξζiΥσ(i),2π(n∏i=1(ΩξΥσ(i)2π)ζi)),(n∏i=1ϕζiΥσ(i),2π(n∏i=1(ΩϕΥσ(i)2π)ζi))}, | (4.15) |
where ζi is defined as in Eq (4.14).
In addition, the CT-SFOWPA operator satisfies the properties of idempotency, boundedness, commutativity and monotonicity.
The AOs mentioned above are expanded in this subsection to geometric AOs with complex T-Spherical fuzzy information, such as CSF power geometric (CT-SFPG), CT-SF weighted power geometric (CT-SFWPG) and CT-SF ordered weighted power geometric (CT-SFOWPG).
Definition 4.4. For a family of CT-SFNs Υi(i=1,...,n), the complex T-Spherical fuzzy power geometric (CT-SFPG) operator is a function CT−SFPG:Ωn→Ω defined as:
CT−SFPG=(Υ1,...,Υn)=Υρ11⊗...⊗Υρnn, | (4.16) |
where ρi=1+T(Υi)∑ni=1(1+T(Υi)) and T(Υi)=n∑s=1s≠i(Sup(Υi,Υs))(i=1,...,n). Here, Sup(Υi,Υs) is the support of Υi from Υs satisfying the aforementioned properties, and Sup(Υi,Υs)=1−d(Υi,Υs), while d is the distance measure.
Theorem 4.4. Let a family of CT-SFNs Υi={(κΥi,ΩκΥi),(ξΥi,ΩξΥi),(ϕΥi,ΩϕΥi)}(i=1,...,n). Then, the aggregated value obtained by using the CT-SFPG operator is again a CT-SFN and given as
CT−SFPG(Υ1,...,Υn)={(n∏i=1κρiΥi,2π(n∏i=1(ΩκΥi2π)ρi)),(q√1−n∏i=1(1−ξqΥi)ρi,2π(1−n∏i=1(1−ΩqξΥi2π)ρi)),(q√1−n∏i=1(1−ϕqΥi)ρi,2π(1−n∏i=1(1−ΩqϕΥi2π)ρi))}. | (4.17) |
Proof. We'll show that Eq (4.17) holds by using mathematical induction. For each i,Υi is a CT-SFN, and Υi>0; therefore, we have that Υρii is still CT-SFN. Then, using the steps of mathematical induction, we have the following.
(1) For n=2, we get Υ1={(κΥ1,ΩκΥ1),(ξΥ1,ΩξΥ1),(ϕΥ1,ΩϕΥ1)} and Υ2={(κΥ2,ΩκΥ2),(ξΥ2,ΩξΥ2),(ϕΥ2,ΩϕΥ2)}. Thus, by the operational law of CT-SFNs, we have
Υρ11={(κρ1Υ1,2π(ΩκΥ12π)ρ1),(q√1−(1−ξqΥ1)ρ1,2π(1−(1−ΩqξΥ12π)ρ1)),(q√1−(1−ϕqΥ1)ρ1,2π(1−(1−ΩqϕΥ12π)ρ1))} |
and
Υρ22={(κρ2Υ2,2π(ΩκΥ22π)ρ2),(q√1−(1−ξqΥ2)ρ2,2π(1−(1−ΩqξΥ22π)ρ2)),(q√1−(1−ϕqΥ2)ρ2,2π(1−(1−ΩqϕΥ22π)ρ2))}. |
Hence, using the addition law of CT-SFNs, we obtain
CT−SFPG(Υ1,Υ2)={(κρ1Υ1,2π(ΩκΥ12π)ρ1),(q√1−(1−ξqΥ1)ρ1,2π(1−(1−ΩqξΥ12π)ρ1)),(q√1−(1−ϕqΥ1)ρ1,2π(1−(1−ΩqϕΥ12π)ρ1))}⊗{(κρ2Υ2,2π(ΩκΥ22π)ρ2),(q√1−(1−ξqΥ2)ρ2,2π(1−(1−ΩqξΥ22π)ρ2)),(q√1−(1−ϕqΥ2)ρ2,2π(1−(1−ΩqϕΥ22π)ρ2))}={(2∏i=1κρiΥi,2π(2∏i=1(ΩκΥi2π)ρi)),(q√1−2∏i=1(1−ξqΥi)ρi,2π(1−2∏i=1(1−ΩqξΥi2π)ρi)),(q√1−2∏i=1(1−ϕqΥi)ρi,2π(1−2∏i=1(1−ΩqϕΥi2π)ρi)).} |
Thus, the resuls holds for n=2.
(2) Let Eq (4.17) be true for n=k, (k is a positive natural number), i.e.,
CT−SFPG(Υ1,...,Υk)={(k∏i=1κρiΥi,2π(k∏i=1(ΩκΥi2π)ρi)),(q√1−k∏i=1(1−ξqΥi)ρi,2π(1−k∏i=1(1−ΩqξΥi2π)ρi)),(q√1−k∏i=1(1−ϕqΥi)ρi,2π(1−k∏i=1(1−ΩqϕΥi2π)ρi))}. |
Then, n=k+1, and we get
CT−SFPG(Υ1,...,Υk+1)=CT−SFPG(Υ1,...,Υk)⊗CT−SFPG(Υk+1)={(k∏i=1κρiΥi,2π(k∏i=1(ΩκΥi2π)ρi)),(q√1−k∏i=1(1−ξqΥi)ρi,2π(1−k∏i=1(1−ΩqξΥi2π)ρi)),(q√1−k∏i=1(1−ϕqΥi)ρi,2π(1−k∏i=1(1−ΩqϕΥi2π)ρi))}⊗{(κρk+1Υk+1,2π(ΩκΥk+12π)ρk+1),(q√1−(1−ξqΥk+1)ρk+1,2π(1−(1−ΩqξΥk+12π)ρk+1)),(q√1−(1−ϕqΥk+1)ρk+1,2π(1−(1−ΩqϕΥk+12π)ρk+1))}={(k+1∏i=1κρiΥi,2π(k+1∏i=1(ΩκΥi2π)ρi)),(q√1−k+1∏i=1(1−ξqΥi)ρi,2π(1−k+1∏i=1(1−ΩqξΥi2π)ρi)),(q√1−k+1∏i=1(1−ϕqΥi)ρi,2π(1−k+1∏i=1(1−ΩqϕΥi2π)ρi))}. |
Thus, Eq (4.17) is true for all positive natural numbers n.
For a family of CT-SFNs Υi(i=1,...,n) with weight vector ψ=(ψ1,...,ψn)T, such that ψi>0 and ∑ni=1ψi=1, the CT-SFPG operator satisfies certain properties, such as the following.
Property 1 (Idempotency). Let Υ0 be a CT-SFN, and if Υi=Υ0 for all i=1,...,n, then
CT−SFPG(Υ1,...,Υn)=Υ0. | (4.18) |
Property 2 (Boundedness). Let Υi={(κΥi,ΩκΥi),(ξΥi,ΩξΥi),(ϕΥi,ΩϕΥi)}(i=1,...,n) be the family of CT-SFNs, and Υ−={mini(κΥi),mini(ΩκΥi),mini(ξΥi),mini(ΩξΥi),maxi(ϕΥi),maxi(ΩϕΥi)} and
Υ+={maxi(κΥi),maxi(ΩκΥi),mini(ξΥi),mini(ΩξΥi),mini(ϕΥi),mini(ΩϕΥi)}. Then,
Υ−≤CT−SFPG(Υ1,...,Υn)≤Υ+. | (4.19) |
Property 3 (Commutivity). Let Υi={(κΥi,ΩκΥi),(ξΥi,ΩξΥi),(ϕΥi,ΩϕΥi)}(i=1,...,n) be family of CT-SFNs. If (Υ∗1,...,Υ∗n) are the permutation of (Υ1,...,Υn), then
CT−SFPG(Υ1,...,Υn)=CT−SFPG(Υ∗1,...,Υ∗n). | (4.20) |
Property 4 (Monotonicity). Let Υi={(κΥi,ΩκΥi),(ξΥi,ΩξΥi),(ϕΥi,ΩϕΥi)} and
Υ∗i={(κ∗Υi,Ω∗κΥi),(ξ∗Υi,Ω∗ξΥi),(ϕ∗Υi,Ω∗ϕΥi)}(i=1,...,n) be the families of CT-SFNs, such that Υi≤Υ∗i. Then,
CT−SFPG(Υ1,...,Υn)≤CT−SFPG(Υ∗1,...,Υ∗n) | (4.21) |
In this section, we consider the distinct weighting factor of the CT-SFNs Υi(i=1,...,n) during the aggregation process, as opposed to the above CT-SFPG operator, and suggest a new CT-SF weighted power geometric (CT-SFWPG) aggregation operator.
Definition 4.5. For a family of CT-SFNs Υi(i=1,...,n), the CT-SFWPG operator is a function CT−SFWPG:Ωn→Ω defined by;
CT−SFWPG(Υ1,...,Υn)=Υϕ11⊗...⊗Υϕnn, | (4.22) |
where ϕi=ψi(1+T/(Υi))∑ni=1(1+T/(Υi)),T/(Υi)=n∑s=1s≠iψs(Sup(Υi,Υs)) and ψ=(ψ1,...,ψn)T are the weights of CT-SFNs Υi, such that ψi>0 and ∑ni=1ψi=1.
Theorem 4.5. Let a family of CT-SFNs Υi={(κΥi,ΩκΥi),(ξΥi,ΩξΥi),(ϕΥi,ΩϕΥi)}(i=1,...,n), with the respective weight vector ψ=(ψ1,...,ψn)T, such that ψi>0 and ∑ni=1ψi=1. Then, the aggregated value obtained by using the CT-SFWPG operator is again a CT-SFN, as follows.
CT−SFWPG(Υ1,...,Υn)={(n∏i=1κϕiΥi,2π(n∏i=1(ΩκΥi2π)ϕi)),(q√1−n∏i=1(1−ξqΥi)ϕi,2π(1−n∏i=1(1−ΩqξΥi2π)ϕi)),(q√1−n∏i=1(1−ϕqΥi)ϕi,2π(1−n∏i=1(1−ΩqϕΥi2π)ϕi))}. | (4.23) |
Proof. The proof of the theorem is same as that of Theorem (???).
For a family of CT-SFNs Υi(i=1,...,n) with weight vector ψ=(ψ1,...,ψn)T, such that ψi>0 and ∑ni=1ψi=1, the CT-SFWPG operator also satisfies the same properties as the CT-SFPG operator such as the following.
Property 1 (Idempotency). Let Υ0 be a CT-SFN, and if Υi=Υ0 for all i=1,...,n, then
CT−SFWPG(Υ1,...,Υn)=Υ0. | (4.24) |
Property 2 (Boundedness). Let Υ− and Υ+ be the lower bound and upper bound of the CT-SFNs Υi(i=1,...,n), respectively. Then, we have,
Υ−≤CT−SFWPG(Υ1,...,Υn)≤Υ+. | (4.25) |
Property 3 (Commutivity). For a permutation (Υ∗1,...,Υ∗n) of CT-SFNs (Υ1,...,Υn) and their corresponding permutation weights ψ∗=(ψ∗1,...,ψ∗n)T of ψ=(ψ1,...,ψn)T, we have
CT−SFWPG(Υ1,...,Υn)=CT−SFWPG(Υ∗1,...,Υ∗n). | (4.26) |
Property 4 (Monotonicity). Let Υi={(κΥi,ΩκΥi),(ξΥi,ΩξΥi),(ϕΥi,ΩϕΥi)} and
Υ∗i={(κ∗Υi,Ω∗κΥi),(ξ∗Υi,Ω∗ξΥi),(ϕ∗Υi,Ω∗ϕΥi)}(i=1,...,n) be the families of CT-SFNs, such that Υi≤Υ∗i. Then,
CT−SFWPG(Υ1,...,Υn)≤CT−SFWPG(Υ∗1,...,Υ∗n). | (4.27) |
The existing AO is expanded to an ordered weighted AO in this segment.
Definition 4.6. For a family of CT-SFNs Υi(i=1,...,n), a complex T-Spherical fuzzy ordered weighted power geometric (CT-SFOWPG) operator is a mapping CT−SFOWPG:Ωn→Ω defined by
CT−SFOWPG(Υ1,...,Υn)=Υζ1σ(1)⊗...⊗Υζnσ(n), | (4.28) |
where Ω represent the CT-SFNs, and σ(1),...,σ(n) are the permutations of (1,...,n) satisfying that Υσ(i−1)≥Υσ(i) for i=2,...,n. Also, ζi is defined as
ζi=g(BiTV)−g(Bi−1TV), | (4.29) |
where Bi=∑is=1Vσ(s),Vσ(i)=1+∑ns=1s≠i(Sup(Υi,Υs)),TV=∑ni=1Vσ(i), and the mapping g:[0,1]→[0,1] is a basic unit-interval monotonic function satisfying these three properties: g(0)=0,g(1)=1, if x≤y then g(x)≤g(y).
Theorem 4.6. Let a family of CT-SFNs Υi={(κΥi,ΩκΥi),(ξΥi,ΩξΥi),(ϕΥi,ΩϕΥi)}(i=1,...,n). Then, the aggregated value calculated by using the CT-SFOWPG operator is also a CT-SFN and given as
CT−SFOWPG(Υ1,...,Υn)={(n∏i=1κζiΥσ(i),2π(n∏i=1(ΩκΥσ(i)2π)ζi)),(q√1−n∏i=1(1−ξqΥσ(i))ζi,2π(1−n∏i=1(1−ΩqξΥσ(i)2π)ζi)),(q√1−n∏i=1(1−ϕqΥσ(i))ζi,2π(1−n∏i=1(1−ΩqϕΥσ(i)2π)ζi))}, | (4.30) |
where ζi is defined as in Eq (4.29).
Also, the CT-SFOWPA operator meets the properties of idempotency, commutativity and boundedness when applied to the set of CT-SFNs.
Using the given operators and the CT-SFS information, an MCGDM algorithm is created in this section. Suppose that a DM problem with the m alternatives ℘1,...,℘m, is evaluated with the n criteria C1,...,Cn. Let us have p experts E=(E1,...,Ep) who evaluate the different alternatives with the different criteria. Every expert evaluates every alternative with the CT-SF information and assigns its rating values to CT-SFNs Υ(k)ij={(κ(k)Υij,Ω(k)κΥij),(ξ(k)Υij,Ω(k)ξΥij),(ϕ(k)Υij,Ω(k)ϕΥij)}, where k=1,...,p;i=1,...,m; and j=1,...,n, 0≤κ(k)Υij+ξ(k)Υij+ϕ(k)Υij≤1 and 0≤Ω(k)κΥij+Ω(k)ξΥij+Ω(k)ϕΥij≤1. Further, assume that the weights of the criteria are ψ=(ψ1,...,ψn)T, such that ψi>0 and ∑ni=1ψi=1. Then, to find the most desirable alternatives, the defined operators are used to define an MCGDM approach under the CT-SF information, and we have the below steps.
Step 1. Define a CT-SFNs matrix R(k)=(Υ(k)ij)m×n with the values of every alternative assigned by expert E(k)(k=1,...,p) as follows.
R(k)=(C1C2...Cn℘1Υ(k)11Υ(k)12...Υ(k)1n℘2Υ(k)21Υ(k)22Υ(k)2n . .. . . .. . . .. .℘mΥ(k)m1Υ(k)m2 . . .Υ(k)mn) |
Step 2a. Aggregate the expert's rating values R(k)(k=1,...,p) into the total collective CT-SF decision matrix R=(Ξij) where Ξij={(κΥij,ΩκΥij),(ξΥij,ΩξΥij),(ϕΥij,ΩϕΥij)} by using the CT-SFOWPA operator as follows.
Ξij=CT−SFOWPA(Υ(1)ij,...,Υ(p)ij)={(q√1−p∏k=1(1−(κqΥij)(σ(k)))ζ(k)ij,2π(1−p∏k=1(1−(ΩqκΥij)(σ(k))2π)ζ(k)ij)),(n∏k=1(ξ(σ(k))Υij)ζ(k)ij,2π(p∏k=1(Ω(σ(k))ξΥij2π)ζ(k)ij)),(n∏k=1(ϕ(σ(k))Υij)ζ(k)ij,2π(p∏k=1(Ω(σ(k))ϕΥij2π)ζ(k)ij))} |
Step 2b. Aggregate the expert's rating values R(k)(k=1,...,p) into the total collective CT-SF decision matrix R=(Ξij) where Ξij={(κΥij,ΩκΥij),(ξΥij,ΩξΥij),(ϕΥij,ΩϕΥij)} by using the CT-SFOWPG operator as follows:
Ξij=Cq−ROFOWPG(Υ(1)ij,...,Υ(p)ij)={(p∏k=1(κ(σ(k))Υij)ζ(k)ij,2π(p∏k=1(Ω(σ(k))κΥij2π)ζ(k)ij)),(q√1−p∏k=1(1−(ξqΥij)(σ(k)))ζ(k)ij,2π(1−p∏k=1(1−(ΩqξΥij)(σ(k))2π)ζ(k)ij)),(q√1−p∏k=1(1−(ϕqΥij)(σ(k)))ζ(k)ij,2π(1−p∏k=1(1−(ΩqϕΥij)(σ(k))2π)ζ(k)ij))} |
where ζ(1)ij,...,ζ(p)ij are the weights obtained by using Eq (4.14), and σ is the permutation mapping from (1,...,p) to (1,...,p).
Step 3a. Aggregate the collected values R=(Ξij) of alternatives ℘i(i=1,...,m) into the total assessment value Ξi={(κΥi,ΩκΥi),(ξΥi,ΩξΥi),(ϕΥi,ΩϕΥi)} using the power averaging operator as defined in Eq (4.2).
For example, if we aggregate every value of the alternative using the CT-SFWPA, we will obtain the evaluation value of the alternative Ξi(i=1,...,m) as:
Ξi=CT−SFWPA(Ξi1,...,Ξin)={(q√1−n∏j=1(1−κqΥij)ρj,2π(1−n∏j=1(1−ΩqκΥij2π)ρj)),(n∏i=1ξρiΥij,2π(n∏i=1(ΩξΥij2π)ρi)),(n∏i=1ϕρiΥij,2π(n∏i=1(ΩϕΥij2π)ρi))} |
Step 3b. Aggregate the collected values R=(Ξij) of alternatives ℘i(i=1,...,m) into the total assessment value Ξi={(κΥi,ΩκΥi),(ξΥi,ΩξΥi),(ϕΥi,ΩϕΥi)} using the power geometric operator as defined in Eq (4.8).
For example, if we aggregate every value of the alternative using the CT-SFWPA, we will obtain the evaluation value of the alternative Ξi(i=1,...,m) as;
Ξi=CT−SFWPG(Ξi1,...,Ξin)={(n∏i=1κρiΥi,2π(n∏i=1(ΩκΥi2π)ρi)),(q√1−n∏i=1(1−ξqΥi)ρi,2π(1−n∏i=1(1−ΩqξΥi2π)ρi)),(q√1−n∏i=1(1−ϕqΥi)ρi,2π(1−n∏i=1(1−ΩqϕΥi2π)ρi))} |
Step 4. Find the score value from the total aggregated values of
Ξi={(κΥi,ΩκΥi),(ξΥi,ΩξΥi),(ϕΥi,ΩϕΥi)}(i=1,...,m) by using the following equation:
Sc∗(Υ)=12|(κqΥ−ξqΥ−ϕqΥ)+(ΩqκΥ−ΩqξΥ−ΩqϕΥ)|. |
Step 5. Allow ranking of the alternatives ℘i(i=1,...,m) and select the appropriate one (s).
In Figure 1, we show the proposed algorithm steps.
Emergency management is a term that refers to dealing with the possibility of major incidents and disasters, as well as involving government and other public bodies in the emergency response, prevention, disposal and recovery process, as well as developing an effective response plan to take a variety of necessary steps. The use of research, technology, planning, and management implies assuring the security of public safety, health, and property-related emergency operations, as well as facilitating society's harmonious and long-term growth. Natural disasters have been causing enormous injury and damage to human life and the global economy in recent years. The Emergency Management Center (EMC) will construct a range of emergency choices based on the types of incidents and ask specialists from other disciplines to assess alternative emergency plans in order to effectively limit the damages sustained by significant incidents and catastrophes. Alternative emergency assessment is a critical component of emergency management. The traditional DM problem is in the core, and it has attracted a lot of attention from a lot of people. As a result, we will apply the defined technique to the stated problem, to determine the best emergency solution for the EMC. Four better alternatives would be investigated further after a series of screenings. There are five alternatives set as follows; ℘={℘1,...,℘5}. Expert discussions take into account the following four requirements for proper modeling of the characteristics of alternatives: (C1) preparation ability, (C2) rescuing ability, C3 Restoration ability, (C4) Reaction capacity. ψ=(0.20,0.25,0.25,0.30)T are the weights of the criteria, such that ∑4i=1Θi=1. The information given by experts E1,E2 and E3 is shown in Tables 1–3 with the weight vector ϖ=(0.3,0.4,0.3).
C1 |
℘1(⟨0.4,2π(0.3)⟩,⟨0.2,2π(0.3)⟩,⟨0.5,2π(0.6)⟩) |
℘2(⟨0.3,2π(0.2)⟩,⟨0.5,2π(0.4)⟩,⟨0.4,2π(0.3)⟩) |
℘3(⟨0.6,2π(0.4)⟩,⟨0.3,2π(0.2)⟩,⟨0.2,2π(0.5)⟩) |
℘4(⟨0.2,2π(0.2)⟩,⟨0.4,2π(0.3)⟩,⟨0.8,2π(0.7)⟩) |
℘5(⟨0.1,2π(0.4)⟩,⟨0.5,2π(0.5)⟩,⟨0.7,2π(0.3)⟩) |
C2 |
(⟨0.3,2π(0.1)⟩,⟨0.4,2π(0.4)⟩,⟨0.2,2π(0.3)⟩) |
(⟨0.2,2π(0.5)⟩,⟨0.1,2π(0.2)⟩,⟨0.7,2π(0.4)⟩) |
(⟨0.4,2π(0.3)⟩,⟨0.3,2π(0.6)⟩,⟨0.8,2π(0.5)⟩) |
(⟨0.1,2π(0.2)⟩,⟨0.2,2π(0.8)⟩,⟨0.9,2π(0.1)⟩) |
(⟨0.5,2π(0.3)⟩,⟨0.5,2π(0.3)⟩,⟨0.3,2π(0.6)⟩) |
C3 |
(⟨0.2,2π(0.2)⟩,⟨0.5,2π(0.4)⟩,⟨0.3,2π(0.6)⟩) |
(⟨0.4,2π(0.5)⟩,⟨0.3,2π(0.7)⟩,⟨0.7,2π(0.3)⟩) |
(⟨0.3,2π(0.2)⟩,⟨0.8,2π(0.3)⟩,⟨0.5,2π(0.9)⟩) |
(⟨0.5,2π(0.4)⟩,⟨0.6,2π(0.5)⟩,⟨0.2,2π(0.4)⟩) |
(⟨0.1,2π(0.3)⟩,⟨0.2,2π(0.2)⟩,⟨0.9,2π(0.7)⟩) |
C4 |
(⟨0.2,2π(0.3)⟩,⟨0.4,2π(0.6)⟩,⟨0.5,2π(0.4)⟩) |
(⟨0.4,2π(0.2)⟩,⟨0.8,2π(0.3)⟩,⟨0.2,2π(0.8)⟩) |
(⟨0.5,2π(0.1)⟩,⟨0.3,2π(0.4)⟩,⟨0.7,2π(0.6)⟩) |
(⟨0.1,2π(0.4)⟩,⟨0.4,2π(0.1)⟩,⟨0.5,2π(0.9)⟩) |
(⟨0.2,2π(0.3)⟩,⟨0.6,2π(0.5)⟩,⟨0.4,2π(0.3)⟩) |
C1 |
℘1(⟨0.2,2π(0.3)⟩,⟨0.3,2π(0.2)⟩,⟨0.5,2π(0.4)⟩) |
℘2(⟨0.6,2π(0.4)⟩,⟨0.4,2π(0.3)⟩,⟨0.4,2π(0.7)⟩) |
℘3(⟨0.1,2π(0.2)⟩,⟨0.2,2π(0.4)⟩,⟨0.9,2π(0.8)⟩) |
℘4(⟨0.5,2π(0.3)⟩,⟨0.4,2π(0.5)⟩,⟨0.3,2π(0.6)⟩) |
℘5(⟨0.3,2π(0.1)⟩,⟨0.7,2π(0.3)⟩,⟨0.4,2π(0.2)⟩) |
C2 |
(⟨0.7,2π(0.2)⟩,⟨0.5,2π(0.6)⟩,⟨0.4,2π(0.5)⟩) |
(⟨0.3,2π(0.4)⟩,⟨0.4,2π(0.8)⟩,⟨0.6,2π(0.2)⟩) |
(⟨0.5,2π(0.3)⟩,⟨0.3,2π(0.4)⟩,⟨0.5,2π(0.7)⟩) |
(⟨0.2,2π(0.4)⟩,⟨0.7,2π(0.2)⟩,⟨0.3,2π(0.9)⟩) |
(⟨0.4,2π(0.8)⟩,⟨0.6,2π(0.5)⟩,⟨0.4,2π(0.2)⟩) |
C3 |
(⟨0.2,2π(0.2)⟩,⟨0.5,2π(0.4)⟩,⟨0.4,2π(0.6)⟩) |
(⟨0.4,2π(0.5)⟩,(0.3,2π(0.8)),(0.7,2π(0.2))) |
(⟨0.3,2π(0.1)⟩,⟨0.6,2π(0.6)⟩,⟨0.3,2π(0.5)⟩) |
(⟨0.4,2π(0.8)⟩,⟨0.9,2π(0.4)⟩,⟨0.5,2π(0.3)⟩) |
(⟨0.2,2π(0.1)⟩,⟨0.7,2π(0.2)⟩,⟨0.3,2π(0.9)⟩) |
C4 |
(⟨0.7,2π(0.3)⟩,⟨0.3,2π(0.4)⟩,⟨0.4,2π(0.8)⟩) |
(⟨0.3,2π(0.4)⟩,⟨0.5,2π(0.9)⟩,⟨0.6,2π(0.2)⟩) |
(⟨0.6,2π(0.5)⟩,⟨0.4,2π(0.5)⟩,⟨0.5,2π(0.3)⟩) |
(⟨0.5,2π(0.3)⟩,⟨0.9,2π(0.4)⟩,⟨0.3,2π(0.8)⟩) |
(⟨0.2,2π(0.6)⟩,⟨0.1,2π(0.3)⟩,⟨0.9,2π(0.5)⟩) |
C1 |
℘1(⟨0.2,2π(0.6)⟩,⟨0.5,2π(0.3)⟩,⟨0.3,2π(0.4)⟩) |
℘2(⟨0.5,2π(0.2)⟩,⟨0.1,2π(0.4)⟩,⟨0.7,2π(0.5)⟩) |
℘3(⟨0.8,2π(0.3)⟩,⟨0.3,2π(0.6)⟩,⟨0.4,2π(0.8)⟩) |
℘4(⟨0.3,2π(0.5)⟩,⟨0.4,2π(0.9)⟩,⟨0.6,2π(0.2)⟩) |
℘5(⟨0.6,2π(0.4)⟩,⟨0.6,2π(0.2)⟩,⟨0.5,2π(0.7)⟩) |
C2 |
(⟨0.5,2π(0.7)⟩,⟨0.6,2π(0.4)⟩,⟨0.3,2π(0.2)⟩) |
(⟨0.4,2π(0.2)⟩,⟨0.7,2π(0.5)⟩,⟨0.5,2π(0.6)⟩) |
(⟨0.6,2π(0.5)⟩,⟨0.3,2π(0.8)⟩,⟨0.4,2π(0.5)⟩) |
(⟨0.3,2π(0.4)⟩,⟨0.8,2π(0.6)⟩,⟨0.6,2π(0.4)⟩) |
(⟨0.7,2π(0.8)⟩,⟨0.4,2π(0.3)⟩,⟨0.8,2π(0.3)⟩) |
C3 |
(⟨0.7,2π(0.5)⟩,⟨0.4,2π(0.6)⟩,⟨0.3,2π(0.4)⟩) |
(⟨0.5,2π(0.8)⟩,⟨0.6,2π(0.4)⟩,⟨0.7,2π(0.2)⟩) |
(⟨0.6,2π(0.4)⟩,⟨0.5,2π(0.7)⟩,⟨0.4,2π(0.5)⟩) |
(⟨0.8,2π(0.6)⟩,⟨0.2,2π(0.5)⟩,⟨0.6,2π(0.7)⟩) |
(⟨0.4,2π(0.7)⟩,⟨0.3,2π(0.4)⟩,⟨0.8,2π(0.4)⟩) |
C4 |
(⟨0.5,2π(0.7)⟩,⟨0.7,2π(0.4)⟩,⟨0.5,2π(0.4)⟩) |
(⟨0.8,2π(0.5)⟩,⟨0.4,2π(0.6)⟩,⟨0.6,2π(0.5)⟩) |
(⟨0.6,2π(0.8)⟩,⟨0.5,2π(0.5)⟩,⟨0.3,2π(0.2)⟩) |
(⟨0.4,2π(0.2)⟩,⟨0.8,2π(0.7)⟩,⟨0.6,2π(0.6)⟩) |
(⟨0.3,2π(0.3)⟩,⟨0.9,2π(0.8)⟩,⟨0.7,2π(0.8)⟩) |
Step 1. The CT-SFN information is used by the three experts to evaluate options, and their individual rating values are provided in Tables 1–3 in the decision matrix.
Step 2a. Different assessments of the experts Υ(k)ij(k=1,2,3) are aggregated into a collective one Ξij(i=1,...,5;j=1,...,4), taking the function g(x)=x2 and using the CT-SFOWPA operator. The obtained values are given in Table 4.
C1 |
℘1(⟨0.432,2π(0.123)⟩,⟨0.193,2π(0.345)⟩,⟨0.274,2π(0.213)⟩) |
℘2(⟨0.352,2π(0.254)⟩,⟨0.327,2π(0.351)⟩,⟨0.183,2π(0.301)⟩) |
℘3(⟨0.142,2π(0.242)⟩,⟨0.275,2π(0.221)⟩,⟨0.272,2π(0.121)⟩) |
℘4(⟨0.334,2π(0.331)⟩,(0.342,2π(0.141)),(0.319,2π(0.232))) |
℘5(⟨0.422,2π(0.224)⟩,⟨0.164,2π(0.232)⟩,⟨0.280,2π(0.326)⟩) |
C2 |
(⟨0.381,2π(0.326)⟩,⟨0.218,2π(0.127)⟩,⟨0.281,2π(0.190)⟩) |
(⟨0.255,2π(0.127)⟩,⟨0.119,2π(0.342)⟩,⟨0.188,2π(0.208)⟩) |
(⟨0.142,2π(0.469)⟩,⟨0.234,2π(0.121)⟩,⟨0.162,2π(0.165)⟩) |
(⟨0.361,2π(0.253)⟩,⟨0.311,2π(0.322)⟩,⟨0.231,2π(0.311)⟩) |
(⟨0.531,2π(0.326)⟩,⟨0.135,2π(0.231)⟩,⟨0.314,2π(0.253)⟩) |
C3 |
(⟨0.241,2π(0.114)⟩,⟨0.236,2π(0.112)⟩,⟨0.302,2π(0.291)⟩) |
(⟨0.376,2π(0.231)⟩,⟨0.212,2π(0.228)⟩,⟨0.233,2π(0.196)⟩) |
(⟨0.457,2π(0.262)⟩,⟨0.327,2π(0.241)⟩,⟨0.200,2π(0.175)⟩) |
(⟨0.332,2π(0.132)⟩,⟨0.219,2π(0.133)⟩,⟨0.173,2π(0.285)⟩) |
(⟨0.128,2π(0.313)⟩,⟨0.321,2π(0.236)⟩,⟨0.362,2π(0.321)⟩) |
C4 |
(⟨0.242,2π(0.168)⟩,⟨0.231,2π(0.211)⟩,⟨0.143,2π(0.112)⟩) |
(⟨0.364,2π(0.326)⟩,⟨0.212,2π(0.123)⟩,⟨0.231,2π(0.153)⟩) |
(⟨0.351,2π(0.235)⟩,⟨0.214,2π(0.342)⟩,⟨0.184,2π(0.207)⟩) |
(⟨0.131,2π(0.127)⟩,⟨0.281,2π(0.232)⟩,⟨0.166,2π(0.166)⟩) |
(⟨0.123,2π(0.272)⟩,⟨0.326,2π(0.131)⟩,⟨0.340,2π(0.214)⟩) |
Step 2b. We use the CT-SFOWPA operator to aggregate the different assessments of the experts Υ(k)ij(k=1,2,3), into a collective one Ξij(i=1,...,5;j=1,...,4), taking g(x)=x2. The values obtained using this operator are described in Table 5.
C1 |
℘1(⟨0.125,2π(0.231)⟩,⟨0.244,2π(0.124)⟩,⟨0.276,2π(0.291)⟩) |
℘2(⟨0.342,2π(0.132)⟩,⟨0.221,2π(0.347)⟩,⟨0.201,2π(0.182)⟩) |
℘3(⟨0.250,2π(0.237)⟩,⟨0.313,2π(0.221)⟩,⟨0.193,2π(0.102)⟩) |
℘4(⟨0.213,2π(0.321)⟩,⟨0.131,2π(0.353)⟩,⟨0.201,2π(0.198)⟩) |
℘5(⟨0.123,2π(0.212)⟩,⟨0.243,2π(0.132)⟩,⟨0.223,2π(0.271)⟩) |
C2 |
(⟨0.213,2π(0.215)⟩,⟨0.139,2π(0.144)⟩,⟨0.321,2π(0.221)⟩) |
(⟨0.142,2π(0.336)⟩,⟨0.326,2π(0.242)⟩,⟨0.281,2π(0.183)⟩) |
(⟨0.325,2π(0.223)⟩,⟨0.433,2π(0.136)⟩,⟨0.163,2π(0.172)⟩) |
(⟨0.238,2π(0.232)⟩,⟨0.189,2π(0.336)⟩,⟨0.136,2π(0.261)⟩) |
(⟨0.137,2π(0.121)⟩,⟨0.231,2π(0.211)⟩,⟨0.275,2π(0.147)⟩) |
C3 |
(⟨0.212,2π(0.231)⟩,⟨0.237,2π(0.351)⟩,⟨0.231,2π(0.286)⟩) |
(⟨0.121,2π(0.132)⟩,⟨0.362,2π(0.191)⟩,⟨0.123,2π(0.133)⟩) |
(⟨0.238,2π(0.313)⟩,⟨0.223,2π(0.401)⟩,⟨0.248,2π(0.192)⟩) |
(⟨0.183,2π(0.151)⟩,⟨0.313,2π(0.232)⟩,⟨0.210,2π(0.316)⟩) |
(⟨0.332,2π(0.172)⟩,⟨0.128,2π(0.213)⟩,⟨0.321,2π(0.242)⟩) |
C4 |
(⟨0.192,2π(0.221)⟩,⟨0.339,2π(0.321)⟩,⟨0.243,2π(0.184)⟩) |
(⟨0.320,2π(0.113)⟩,⟨0.242,2π(0.252)⟩,⟨0.199,2π(0.121)⟩) |
(⟨0.222,2π(0.434)⟩,⟨0.355,2π(0.342)⟩,⟨0.326,2π(0.398)⟩) |
(⟨0.346,2π(0.231)⟩,⟨0.127,2π(0.221)⟩,⟨0.135,2π(0.231)⟩) |
(⟨0.138,2π(0.215)⟩,⟨0.212,2π(0.131)⟩,⟨0.272,2π(0.216)⟩) |
Step 3a. Now, we use the CT-SFWPA operator to aggregate the different values Ξij(i=1,...,5;j=1,...,4), obtained from Table 4, with the corresponding weights are ψ=(0.20,0.25,0.25,0.30)T. The total values of each alternative ℘i(i=1,...,5) are,
℘1=(⟨0.385,2π(0.298)⟩,⟨0.194,2π(0.221)⟩,⟨0.242,2π(0.183)⟩),℘2=(⟨0.331,2π(0.390)⟩,⟨0.216,2π(0.234)⟩,⟨0.164,2π(0.276)⟩),℘3=(⟨0.289,2π(0.326)⟩,⟨0.266,2π(0.195)⟩,⟨0.351,2π(0.123)⟩),℘4=(⟨0.312,2π(0.240)⟩,⟨0.186,2π(0.247)⟩,⟨0.232,2π(0.285)⟩),℘5=(⟨0.273,2π(0.318)⟩,⟨0.162,2π(0.229)⟩,⟨0.187,2π(0.101)⟩). |
Step 3b. We use the CT-SFWPG operator to aggregate the different values Ξij(i=1,...,5;j=1,...,4), obtained from Table 5, with the corresponding weights ψ=(0.20,0.25,0.25,0.30)T. The total values of each alternative ℘i(i=1,...,5) are,
℘1=(⟨0.136,2π(0.125)⟩,⟨0.236,2π(0.323)⟩,⟨0.143,2π(0.198)⟩),℘2=(⟨0.237,2π(0.214)⟩,⟨0.282,2π(0.227)⟩,⟨0.265,2π(0.209)⟩),℘3=(⟨0.122,2π(0.193)⟩,⟨0.306,2π(0.325)⟩,⟨0.287,2π(0.162)⟩),℘4=(⟨0.329,2π(0.202)⟩,⟨0.197,2π(0.284)⟩,⟨0.321,2π(0.318)⟩),℘5=(⟨0.219,2π(0.267)⟩,⟨0.222,2π(0.314)⟩,⟨0.259,2π(0.190)⟩). |
Step 4. The scores of the alternatives ℘i(i=1,...,5) are computed on the basis of the overall assessment values of Ξi(i=1,...,5;j=1,...,4) as follows:
Sc∗(℘1)=0.684,Sc∗(℘2)=0.528,Sc∗(℘3)=0.603,Sc∗(℘4)=0.429,Sc∗(℘5)=0.378. |
Meanwhile, the scores of the alternatives ℘i(i=1,...,5) are based on the aggregated values of Step 3b.
Sc∗(℘1)=0.724,Sc∗(℘2)=0.542,Sc∗(℘3)=0.594,Sc∗(℘4)=0.496,Sc∗(℘5)=0.438. |
Step 5. The ranking of all feasible alternatives ℘i(i=1,...,5) is shown as follows.
℘1>℘3>℘2>℘4>℘5 |
Thus, the best alternative is ℘1, that is, ℘1 is the most optimal choice.
In Figure 2, we show graphically the ranking order of the alternatives.
Now, we take a transferred alternative Table 6, as shown as:
C1 |
E(1)(⟨0.2,2π(0.3)⟩,⟨0.5,2π(0.6)⟩,⟨0.3,2π(0.4)⟩) |
E(2)(⟨0.3,2π(0.5)⟩,⟨0.3,2π(0.1)⟩,⟨0.7,2π(0.8)⟩) |
E(3)(⟨0.5,2π(0.2)⟩,⟨0.8,2π(0.3)⟩,⟨0.5,2π(0.5)⟩) |
C2 |
(⟨0.2,2π(0.1)⟩,⟨0.6,2π(0.2)⟩,⟨0.4,2π(0.9)⟩) |
(⟨0.3,2π(0.4)⟩,⟨0.4,2π(0.6)⟩,⟨0.6,2π(0.5)⟩) |
(⟨0.4,2π(0.8)⟩,⟨0.5,2π(0.4)⟩,⟨0.7,2π(0.4)⟩) |
C3 |
(⟨0.2,2π(0.4)⟩,⟨0.6,2π(0.3)⟩,⟨0.4,2π(0.6)⟩) |
(⟨0.4,2π(0.6)⟩,⟨0.3,2π(0.5)⟩,⟨0.8,2π(0.4)⟩) |
(⟨0.5,2π(0.1)⟩,⟨0.4,2π(0.2)⟩,⟨0.6,2π(0.9)⟩) |
C4 |
(⟨0.1,2π(0.3)⟩,⟨0.6,2π(0.5)⟩,⟨0.8,2π(0.7)⟩) |
(⟨0.6,2π(0.4)⟩,⟨0.2,2π(0.2)⟩,⟨0.7,2π(0.9)⟩) |
(⟨0.3,2π(0.7)⟩,⟨0.5,2π(0.4)⟩,⟨0.4,2π(0.3)⟩) |
When various MCGDM techniques are used to solve the same DM problem, they produce different outcomes (rankings), which contributes to ambiguous results. In order to investigate the reliability and validity of the MCGDM techniques, Wang & Triantaphyllou [50] offered the following test conditions.
Test criteria on 1. The MCGDM strategy is effective if the better option remains the same and the nonoptimal alternative is converted to a worse alternative while the relative values of the decision criteria remains constant.
Test criteria on 2. A successful MCGDM strategy should follow transitive properties.
Test criteria on 3. When dividing the MCGDM problem into sub-problems and applying the proposed MCGDM approach to these sub-problems for ranking alternatives, the MCGDM approach is effective. The ranking of the alternatives is identical to the ranking of the problem.
The above criteria are used to determine the correctness of the suggested solution.
In order to evaluate the validity of the developed method using criteria on 1, the non-optimal alternative ℘5 is substituted by the worst alternative ℘/5 in the original decision matrix for each expert, and the rating values are reported in Table 4.
We now compute the scores of the alternatives using the CT-SFOWPA operator in Step 2 and the CT-SFWPA operator in Step 3 for these modified results, which are; Sc∗(℘1)=0.822, Sc∗(℘2)=0.641, Sc∗(℘3)=0.683, Sc∗(℘4)=0.435, Sc∗(℘5)=0.263. As a result, the final ranking of the alternatives indicates that ℘1 remains the best choice and that the method developed fulfills test criteria on 1.
We divided the original decision making problem into sub DM problems, which contained the options (℘1,℘2,℘3,℘4), (℘2,℘3,℘4,℘5) and (℘1,℘3,℘4,℘5), in order to test the defined MCGDM approach with criteria 2 and 3. When we apply the suggested MCGDM technique to these subproblems, we get the ratings as ℘1>℘3>℘2>℘4, ℘3>℘2>℘4>℘5 and ℘1>℘3>℘4>℘5. We get the final ranking order as ℘1>℘3>℘2>℘4>℘5 by introducing the ranking of alternatives to these smaller problems. This is a non-decomposed problem that discloses a transitive property. As a result, the defined MCGDM approach is consistent with criteria 2 and 3.
In order to demonstrate the benefits of the defined aggregation operators, we compare the proposed approach to previous methods in this section. First the priorities considered by the experts are translated into SFNs, taking the phase term relevant to the CT-SFN as zero. On the basis of this information, we tested the current methods, as follows.
(1) If we apply the T-SFS aggregation operators, proposed by Garg et al. [12], on the stated problem, then the alternatives ℘i(i=1,...,5) score values are Sc∗(℘1)=0.672, Sc∗(℘2)=0.529, Sc∗(℘3)=0.632, Sc∗(℘4)=0.347, Sc∗(℘5)=0.274. Therefore, their corresponding ranking order is ℘1>℘3>℘2>℘4>℘5, which means that ℘1 is the appropriate option.
(2) If we apply the T-SFS aggregation operators, proposed by Liu et al. [27], on the stated problem, then the alternatives ℘i(i=1,...,5) score values are Sc∗(℘1)=0.794,Sc∗(℘2)=0.653, Sc∗(℘3)=0.812, Sc∗(℘4)=0.645, Sc∗(℘5)=0.573. Therefore, their corresponding ranking order is ℘3>℘1>℘2>℘4>℘5, which means that ℘3 is the appropriate option.
(3) If we apply T-SFS power Maclaurin symmetric mean operators, proposed by Munir et al. [31], on the stated problem, then the alternatives ℘i(i=1,...,5) score values are Sc∗(℘1)=0.434, Sc∗(℘2)=0.424, Sc∗(℘3)=0.326, Sc∗(℘4)=0.223, Sc∗(℘5)=0.238. Therefore, their corresponding ranking order is ℘1>℘2>℘3>℘5>℘4, which means that ℘1 is the appropriate option.
(4) If we apply T-SFS operators, proposed by Ullah et al. [49], on the stated problem, then the alternatives ℘i(i=1,...,5) score values are Sc∗(℘1)=0.742, Sc∗(℘2)=0.463, Sc∗(℘3)=0.572, Sc∗(℘4)=0.651, Sc∗(℘5)=0.552. Therefore, their corresponding ranking order is ℘1>℘4>℘3>℘5>℘2, which means that ℘1 is the appropriate option.
(5) If we apply CT-SFS aggregation operators, proposed by Ali et al. [2], on the stated problem, then the alternatives ℘i(i=1,...,5) score values are Sc∗(℘1)=0.532, Sc∗(℘2)=0.413, Sc∗(℘3)=0.628, Sc∗(℘4)=0.611, Sc∗(℘5)=0.537. Therefore, their corresponding ranking order is ℘3>℘4>℘5>℘1>℘2, which means that ℘3 is the appropriate option.
(6) If we apply CT-SF Dombi aggregation operators, proposed by Karaaslan and Dawood [22], on the stated problem, then the alternatives ℘i(i=1,...,5) score values are Sc∗(℘1)=0.574, Sc∗(℘2)=0.462, Sc∗(℘3)=0.382, Sc∗(℘4)=0.283, Sc∗(℘5)=0.299. Therefore, their corresponding ranking order is ℘1>℘2>℘3>℘5>℘4, which means that ℘1 is the appropriate option.
(7) If we apply CT-SF 2-tuple linguistic Muirhead mean AOs, proposed by Liu et al. [29], on the stated problem, then the alternatives ℘i(i=1,...,5) score values are Sc∗(℘1)=0.630, Sc∗(℘2)=0.625, Sc∗(℘3)=0.651, Sc∗(℘4)=0.563,Sc∗(℘5)=0.517. Therefore, their corresponding ranking order is ℘3>℘1>℘2>℘4>℘5, which means that ℘3 is the appropriate option.
In Figure 3, we show graphically the ranking of alternatives using different approaches.
Figure 3 shows the ranking order of alternatives. The example of the operators studied in this paper shows that the best alternative is ℘1 whereas the best alternatives are ℘1 and ℘3 when we apply the existing operators. It's worth noting that the existing operators can only work with one dimension at a time. As a result, the operators provided here are more generic, as they may capture two dimensions and convey more full data. Hence, the CT-SFS model is an extension of current theories that simultaneously deal with imprecision and periodicity.
The CT-SFS theory combines the features of both the CFS and T-SFS theories. CT-SFSs have three degrees (MD, NuMD and NMD) in polar coordinates, in such a way that the range of the degrees is extended from [0,1] to the unit disk in a complex plane. The concept that prioritized AOs for CT-SFNs would enable us to tackle a new class of decision-making problems motivated us to write this research paper.
The purpose of this article is to provide information in the form of CT-SFSs and using PA operators involved in the DM process. First, we've established some fundamental operational laws as well as a new score function for ranking the CT-SFNs. We offer a variety of power averaging and geometric AOs, such as CT-SFPA, CT-SFWPA, CT-SFOWPA, CT-SFPG, CT-SFWPG and CT-SFOWPG; and we investigate the basic properties of these operators, keeping these points in mind with the CT-SFS details. We have provided the mathematical description of the MCGDM problem, and then an algorithm we initiated for the MCGDM problem. To demonstrate the validity of the approach, a practical example we used to describe the stated method, and the results we compared to some of the other current methods. A validity test was also used to assess the effectiveness and validity of our proposed technique. Using the proposed operators, we conducted a comparison with existing operators in the literature to demonstrate the superiority of the proposed operators. It should also be mentioned that under the CT-SFSs information, existing operators can be considered a special case of the developed method.
Other aggregation operators such as Hamacher, Bonferroni mean, similarity measures, Dombi, Maclaurin's symmetric mean, Banzhaf-Choquet copula, Heronian mean and fractional orthotriple fuzzy operators will be studied in the future for this structure.
The authors would like to thank the Deanship of Scientific Research at Umm Al-Qura University for supporting this work by grant (Code: 22UQU4310396DSR15).
The authors declares that they have no conflict of interest.
[1] |
K. T. Atanassov, More on intuitionistic fuzzy sets, Fuzzy Set. Syst., 33 (1989), 37–45. https://doi.org/10.1016/0165-0114(89)90215-7 doi: 10.1016/0165-0114(89)90215-7
![]() |
[2] |
Z. Ali, T. Mahmood, M. S. Yang, Complex T-spherical fuzzy aggregation operators with application to multi-attribute decision making, Symmetry, 12 (2020), 1311. https://doi.org/10.3390/sym12081311 doi: 10.3390/sym12081311
![]() |
[3] |
M. Akram, A. Khan, J. C. R. Alcantud, G. Santos-García, A hybrid decision-making framework under complex spherical fuzzy prioritized weighted aggregation operators, Expert Syst., 38 (2021), 12712. https://doi.org/10.1111/exsy.12712 doi: 10.1111/exsy.12712
![]() |
[4] |
M. Akram, C. Kahraman, K. Zahid, Extension of TOPSIS model to the decision-making under complex spherical fuzzy information, Soft Comput., 25 (2021), 10771–10795. https://doi.org/10.1007/s00500-021-05945-5 doi: 10.1007/s00500-021-05945-5
![]() |
[5] |
M. Akram, M. Shabir, A. N. Al-Kenani, J. C. R. Alcantud, Hybrid decision-making frameworks under complex spherical fuzzy-soft sets, J. Math., 2021. https://doi.org/10.1155/2021/5563215 doi: 10.1155/2021/5563215
![]() |
[6] | M. Akram, A. Khan, F. Karaaslan, Complex spherical dombi fuzzy aggregation operators for decision-making, Soft Comput., 37 (2021). |
[7] |
S. Abdullah, M. Qiyas, M. Naeem, Y. Liu, Pythagorean Cubic fuzzy Hamacher aggregation operators and their application in green supply selection problem, AIMS Math., 7 (2022), 4735–4766. https://doi.org/10.3934/math.2022263 doi: 10.3934/math.2022263
![]() |
[8] |
L. Bi, S. Dai, B. Hu, Complex fuzzy geometric aggregation operators, Symmetry, 10 (2018), 251. https://doi.org/10.3390/sym10070251 doi: 10.3390/sym10070251
![]() |
[9] |
Y. Chen, M. Munir, T. Mahmood, A. Hussain, S. Zeng, Some generalized T-spherical and group-generalized fuzzy geometric aggregation operators with application in MADM problems, J. Math., 2021. https://doi.org/10.1155/2021/5578797 doi: 10.1155/2021/5578797
![]() |
[10] |
Z. S. Chen, L. L. Yang, R. M. Rodríguez, S. H. Xiong, K. S. Chin, L. Martínez, Power-average-operator-based hybrid multiattribute online product recommendation model for consumer decision-making, Int. J. Intell. Syst., 36 (2021), 2572–2617. https://doi.org/10.1002/int.22394 doi: 10.1002/int.22394
![]() |
[11] |
S. Dick, R. R. Yager, O. Yazdanbakhsh, On Pythagorean and complex fuzzy set operations, IEEE T. Fuzzy Syst., 24 (2015), 1009–1021. https://doi.org/10.1109/TFUZZ.2015.2500273 doi: 10.1109/TFUZZ.2015.2500273
![]() |
[12] |
H. Garg, M. Munir, K. Ullah, T. Mahmood, N. Jan, Algorithm for T-spherical fuzzy multi-attribute decision making based on improved interactive aggregation operators, Symmetry, 10 (2018), 670. https://doi.org/10.3390/sym10120670 doi: 10.3390/sym10120670
![]() |
[13] |
H. Garg, D. Rani, Some generalized complex intuitionistic fuzzy aggregation operators and their application to multicriteria decision-making process, Arab. J. Sci. Eng., 44 (2019), 2679–2698. https://doi.org/10.1007/s13369-018-3413-x doi: 10.1007/s13369-018-3413-x
![]() |
[14] |
H. Garg, D. Rani, New generalised Bonferroni mean aggregation operators of complex intuitionistic fuzzy information based on Archimedean t-norm and t-conorm, J. Exp. Theor. Artif. In., 32 (2020), 81–109. https://doi.org/10.1080/0952813X.2019.1620871 doi: 10.1080/0952813X.2019.1620871
![]() |
[15] |
H. Garg, D. Rani, Robust averaging-geometric aggregation operators for complex intuitionistic fuzzy sets and their applications to MCDM process, Arab. J. Sci. Eng., 45 (2020), 2017–2033. https://doi.org/10.1007/S13369-019-03925-4 doi: 10.1007/S13369-019-03925-4
![]() |
[16] |
H. Garg, J. Gwak, T. Mahmood, Z. Ali, Power aggregation operators and VIKOR methods for complex q-rung orthopair fuzzy sets and their applications, Mathematics, 8 (2020), 538. https://doi.org/10.3390/math8040538 doi: 10.3390/math8040538
![]() |
[17] |
A. Guleria, R. K. Bajaj, T-spherical fuzzy soft sets and its aggregation operators with application in decision-making, Sci. Iran., 28 (2021), 1014–1029. https://doi.org/10.24200/SCI.2019.53027.3018 doi: 10.24200/SCI.2019.53027.3018
![]() |
[18] |
B. Hu, L. Bi, S. Dai, S. Li, Distances of complex fuzzy sets and continuity of complex fuzzy operations, J. Intell. Fuzzy Syst., 35 (2018), 2247–2255. https://doi.org/10.3233/JIFS-172264 doi: 10.3233/JIFS-172264
![]() |
[19] |
Y. Ju, Y. Liang, C. Luo, P. Dong, E. D. S. Gonzalez, A. Wang, T-spherical fuzzy TODIM method for multi-criteria group decision-making problem with incomplete weight information, Soft Comput., 25 (2021), 2981–3001. https://doi.org/10.1007/s00500-020-05357-x doi: 10.1007/s00500-020-05357-x
![]() |
[20] | G. J. Klir, B. Yuan, Fuzzy sets and fuzzy logic: Theory and applications, prentice hall of India Private Limited, New Delhi, 2005. https://doi.org/10.5860/choice.33-2786 |
[21] |
A. A. Khan, S. Ashraf, S. Abdullah, M. Qiyas, J. Luo, S. U. Khan, Pythagorean fuzzy Dombi aggregation operators and their application in decision support system, Symmetry, 11 (2019), 383. https://doi.org/10.3390/sym11030383 doi: 10.3390/sym11030383
![]() |
[22] |
F. Karaaslan, M. A. D. Dawood, Complex T-spherical fuzzy Dombi aggregation operators and their applications in multiple-criteria decision-making, Complex Intell. Syst., 2021, 1–24. https://doi.org/10.1007/s40747-021-00446-2 doi: 10.1007/s40747-021-00446-2
![]() |
[23] |
P. Liu, Multiple attribute group decision making method based on interval-valued intuitionistic fuzzy power Heronian aggregation operators, Comput. Ind. Eng., 108 (2017), 199–212. https://doi.org/10.1016/j.cie.2017.04.033 doi: 10.1016/j.cie.2017.04.033
![]() |
[24] |
P. Liu, H. Li, Interval-valued intuitionistic fuzzy power Bonferroni aggregation operators and their application to group decision making, Cogn. Comput., 9 (2017), 494–512. https://doi.org/10.1007/s12559-017-9453-9 doi: 10.1007/s12559-017-9453-9
![]() |
[25] |
P. Liu, P. Wang, Some q-rung orthopair fuzzy aggregation operators and their applications to multiple-attribute decision making, Int. J. Intell. Syst., 33 (2018), 259–280. https://doi.org/10.1002/int.21927 doi: 10.1002/int.21927
![]() |
[26] |
L. Liu, X. Zhang, Comment on Pythagorean and complex fuzzy set operations, IEEE T. Fuzzy Syst., 26 (2018), 3902–3904. https://doi.org/10.1109/TFUZZ.2018.2853749 doi: 10.1109/TFUZZ.2018.2853749
![]() |
[27] |
P. Liu, Q. Khan, T. Mahmood, N. Hassan, T-spherical fuzzy power Muirhead mean operator based on novel operational laws and their application in multi-attribute group decision making, IEEE Access, 7 (2019), 22613–22632. https://doi.org/10.1109/ACCESS.2019.2896107 doi: 10.1109/ACCESS.2019.2896107
![]() |
[28] |
P. Liu, T. Mahmood, Z. Ali, Complex q-rung orthopair fuzzy aggregation operators and their applications in multi-attribute group decision making, Information, 11 (2020), 5. https://doi.org/10.3390/info11010005 doi: 10.3390/info11010005
![]() |
[29] |
P. Liu, Z. Ali, T. Mahmood, Novel complex T-spherical fuzzy 2-tuple linguistic muirhead mean aggregation operators and their application to multi-attribute decision-making, Int. J. Comput. Intell. Syst., 14 (2020), 295–331. https://doi.org/10.2991/ijcis.d.201207.003 doi: 10.2991/ijcis.d.201207.003
![]() |
[30] |
J. Ma, G. Zhang, J. Lu, A method for multiple periodic factor prediction problems using complex fuzzy sets, IEEE T. Fuzzy Syst., 20 (2011), 32–45. https://doi.org/10.1109/TFUZZ.2011.2164084 doi: 10.1109/TFUZZ.2011.2164084
![]() |
[31] |
M. Munir, H. Kalsoom, K. Ullah, T. Mahmood, Y. M. Chu, T-spherical fuzzy Einstein hybrid aggregation operators and their applications in multi-attribute decision making problems, Symmetry, 12 (2020), 365. https://doi.org/10.3390/sym12030365 doi: 10.3390/sym12030365
![]() |
[32] | H. T. Nguyen, A. Kandel, V. Kreinovich, Complex fuzzy sets: Towards new foundations, Ninth IEEE Int. Conf. Fuzzy Syst. FUZZ-IEEE 2000. https://doi.org/10.1109/FUZZY.2000.839195 |
[33] |
M. Naeem, M. Qiyas, M. M. Al-Shomrani, S. Abdullah, Similarity measures for fractional orthotriple fuzzy sets using cosine and cotangent functions and their application in accident emergency response, Mathematics, 8 (2020), 1653. https://doi.org/10.3390/math8101653 doi: 10.3390/math8101653
![]() |
[34] |
M. Naeem, M. Qiyas, T. Botmart, S. Abdullah, N. Khan, Complex spherical fuzzy decision support system based on entropy measure and power operator, J. Funct. Space., 2022. https://doi.org/10.1155/2022/8315733 doi: 10.1155/2022/8315733
![]() |
[35] |
X. Peng, J. Dai, H. Garg, Exponential operation and aggregation operator for q-rung orthopair fuzzy set and their decision-making method with a new score function, Int. J. Intell. Syst., 33 (2018), 2255–2282. https://doi.org/10.1002/int.22028 doi: 10.1002/int.22028
![]() |
[36] |
S. G. Quek, G. Selvachandran, B. Davvaz, M. Pal, The algebraic structures of complex intuitionistic fuzzy soft sets associated with groups and subgroups, Sci. Iran., 26 (2019), 1898–1912. https://doi.org/10.24200/SCI.2018.50050.1485 doi: 10.24200/SCI.2018.50050.1485
![]() |
[37] |
S. G. Quek, G. Selvachandran, M. Munir, T. Mahmood, K. Ullah, L. H. Son, et al., Multi-attribute multi-perception decision-making based on generalized T-spherical fuzzy weighted aggregation operators on neutrosophic sets, Mathematics, 7 (2019), 780. https://doi.org/10.3390/math7090780 doi: 10.3390/math7090780
![]() |
[38] |
M. Qiyas, S. Abdullah, F. Khan, M. Naeem, Banzhaf-Choquet-Copula-based aggregation operators for managing fractional orthotriple fuzzy information, Alex. Eng. J., 61 (2022), 4659–4677. https://doi.org/10.1016/j.aej.2021.10.029 doi: 10.1016/j.aej.2021.10.029
![]() |
[39] |
M. Qiyas, M. Naeem, S. Abdullah, F. Khan, N. Khan, H. Garg, Fractional orthotriple fuzzy rough Hamacher aggregation operators and their application on service quality of wireless network selection, Alex. Eng. J., 61 (2022), 10433–10452. https://doi.org/10.1016/j.aej.2022.03.002 doi: 10.1016/j.aej.2022.03.002
![]() |
[40] |
D. Ramot, R. Milo, M. Friedman, A. Kandel, Complex fuzzy sets, IEEE T. Fuzzy Syst., 10 (2002), 171–186. https://doi.org/10.1109/91.995119 doi: 10.1109/91.995119
![]() |
[41] |
D. Rani, H. Garg, Complex intuitionistic fuzzy power aggregation operators and their applications in multicriteria decision-making, Expert Syst., 35 (2018), 12325. https://doi.org/10.1111/exsy.12325 doi: 10.1111/exsy.12325
![]() |
[42] |
G. Selvachandran, H. Garg, M. H. Alaroud, A. R. Salleh, Similarity measure of complex vague soft sets and its application to pattern recognition, Int. J. Fuzzy Syst., 20 (2018), 1901–1914. https://doi.org/10.1007/s40815-018-0492-5 doi: 10.1007/s40815-018-0492-5
![]() |
[43] |
P. K. Singh, G. Selvachandran, C. A. Kumar, Interval-valued complex fuzzy concept lattice and its granular decomposition, Recent Dev. Mach. Learn. Data Anal., 2019,275–283. https://doi.org/10.1007/978-981-13-1280-9_26 doi: 10.1007/978-981-13-1280-9_26
![]() |
[44] |
Z. Tao, B. Han, H. Chen, On intuitionistic fuzzy copula aggregation operators in multiple-attribute decision making, Cogn. Comput., 10 (2018), 610–624. https://doi.org/10.1007/s12559-018-9545-1 doi: 10.1007/s12559-018-9545-1
![]() |
[45] |
K. Ullah, T. Mahmood, N. Jan, Similarity measures for T-spherical fuzzy sets with applications in pattern recognition, Symmetry, 10 (2018), 193. https://doi.org/10.3390/sym10060193 doi: 10.3390/sym10060193
![]() |
[46] |
K. Ullah, T. Mahmood, Z. Ali, N. Jan, On some distance measures of complex Pythagorean fuzzy sets and their applications in pattern recognition, Complex Intell. Syst., 2019, 1–13. https://doi.org/10.1007/s40747-019-0103-6 doi: 10.1007/s40747-019-0103-6
![]() |
[47] |
K. Ullah, N. Hassan, T. Mahmood, N. Jan, M. Hassan, Evaluation of investment policy based on multi-attribute decision-making using interval valued T-spherical fuzzy aggregation operators, Symmetry, 11 (2019), 357. https://doi.org/10.3390/sym11030357 doi: 10.3390/sym11030357
![]() |
[48] |
K. Ullah, T. Mahmood, Z. Ali, N. Jan, On some distance measures of complex Pythagorean fuzzy sets and their applications in pattern recognition, Complex Intell. Syst., 6 (2020), 15–27. https://doi.org/10.1007/s40747-019-0103-6 doi: 10.1007/s40747-019-0103-6
![]() |
[49] |
K. Ullah, H. Garg, T. Mahmood, N. Jan, Z. Ali, Correlation coefficients for T-spherical fuzzy sets and their applications in clustering and multi-attribute decision making, Soft Comput., 24 (2020), 1647–1659. https://doi.org/10.1007/s00500-019-03993-6 doi: 10.1007/s00500-019-03993-6
![]() |
[50] |
X. Wang, E. Triantaphyllou, Ranking irregularities when evaluating alternatives by using some ELECTRE methods, Omega, 36 (2008), 45–63. https://doi.org/10.1016/j.omega.2005.12.003 doi: 10.1016/j.omega.2005.12.003
![]() |
[51] |
G. Wei, M. Lu, Pythagorean fuzzy power aggregation operators in multiple attribute decision making, Int. J. Intell. Syst., 33 (2018), 169–186. https://doi.org/10.1002/int.21946 doi: 10.1002/int.21946
![]() |
[52] |
Z. Xu, R. R. Yager, Power-geometric operators and their use in group decision making, IEEE T. Fuzzy Syst., 18 (2009), 94–105. https://doi.org/10.1109/TFUZZ.2009.2036907 doi: 10.1109/TFUZZ.2009.2036907
![]() |
[53] |
Z. Xu, Approaches to multiple attribute group decision making based on intuitionistic fuzzy power aggregation operators, Knowl.-Based Syst., 24 (2011), 749–760. https://doi.org/10.1016/j.knosys.2011.01.011 doi: 10.1016/j.knosys.2011.01.011
![]() |
[54] |
Z. Xu, X. Cai, Uncertain power average operators for aggregating interval fuzzy preference relations, Group Decis. Negot., 21 (2012), 381–397. https://doi.org/10.1007/s10726-010-9213-7 doi: 10.1007/s10726-010-9213-7
![]() |
[55] |
S. H. Xiong, Z. S. Chen, J. P.Chang, K. S. Chin, On extended power average operators for decision-making: A case study in emergency response plan selection of civil aviation, Comput. Ind. Eng., 130 (2019), 258–271. https://doi.org/10.1016/j.cie.2019.02.027 doi: 10.1016/j.cie.2019.02.027
![]() |
[56] |
R. R. Yager, The power average operator, IEEE T. Syst., 31 (2013), 724–731. https://doi.org/10.1109/3468.983429 doi: 10.1109/3468.983429
![]() |
[57] |
R. R. Yager, A. M. Abbasov, Pythagorean membership grades, complex numbers, and decision making, Int. J. Intell. Syst., 28 (2013), 436–452. https://doi.org/10.1002/int.21584 doi: 10.1002/int.21584
![]() |
[58] | R. R. Yager, Pythagorean fuzzy subsets, 2013 joint IFSA world congress and NAFIPS annual meeting (IFSA/NAFIPS), 2013, 57–61. https://doi.org/10.1109/IFSA-NAFIPS.2013.6608375 |
[59] |
R. R. Yager, Generalized orthopair fuzzy sets, IEEE T. Fuzzy Syst., 25 (2016), 1222–1230. https://doi.org/10.1109/TFUZZ.2016.2604005 doi: 10.1109/TFUZZ.2016.2604005
![]() |
[60] |
Z. Yang, X. Li, H. Garg, M. Qi, Decision support algorithm for selecting an antivirus mask over COVID-19 pandemic under spherical normal fuzzy environment, Int. J. Environ. Res. Pub. Heal., 17 (2020), 3407. https://doi.org/10.3390/ijerph17103407 doi: 10.3390/ijerph17103407
![]() |
[61] |
L. A. Zadeh, Fuzzy sets, Inf. Control, 8 (2020), 338–353. https://doi.org/10.2307/2272014 doi: 10.2307/2272014
![]() |
[62] |
G. Zhang, T. S. Dillon, K. Y. Cai, J. Ma, J. Lu, Operation properties and δ-equalities of complex fuzzy sets, Int. J. Approx. Reason., 50 (2009), 1227–1249. https://doi.org/10.1016/j.ijar.2009.05.010 doi: 10.1016/j.ijar.2009.05.010
![]() |
[63] |
L. Zhou, H. Chen, J. Liu, Generalized power aggregation operators and their applications in group decision making, Comput. Ind. Eng., 62 (2012), 989–999. https://doi.org/10.1016/j.cie.2011.12.025 doi: 10.1016/j.cie.2011.12.025
![]() |
[64] |
L. Zhou, H. Chen, A generalization of the power aggregation operators for linguistic environment and its application in group decision making, Knowl.-Based Syst., 26 (2012), 216–224. https://doi.org/10.1016/j.knosys.2011.08.004 doi: 10.1016/j.knosys.2011.08.004
![]() |
[65] |
L. Zhou, H. Chen, J. Liu, Generalized power aggregation operators and their applications in group decision making, Comput. Ind. Eng., 62 (2012), 989–999. https://doi.org/10.1016/j.cie.2011.12.025 doi: 10.1016/j.cie.2011.12.025
![]() |
[66] |
Z. Zhang, Hesitant fuzzy power aggregation operators and their application to multiple attribute group decision making, Inf. Sci., 234 (2013), 150–181. https://doi.org/10.1016/j.ins.2013.01.002 doi: 10.1016/j.ins.2013.01.002
![]() |
1. | Ashraf Al-Quran, T-spherical linear Diophantine fuzzy aggregation operators for multiple attribute decision-making, 2023, 8, 2473-6988, 12257, 10.3934/math.2023618 | |
2. | Esmail Hassan Abdullatif Al-Sabri, Muhammad Rahim, Fazli Amin, Rashad Ismail, Salma Khan, Agaeb Mahal Alanzi, Hamiden Abd El-Wahed Khalifa, Multi-criteria decision-making based on Pythagorean cubic fuzzy Einstein aggregation operators for investment management, 2023, 8, 2473-6988, 16961, 10.3934/math.2023866 | |
3. | Lijun Ma, Kinza Javed, Zeeshan Ali, Shi Yin, Stepping Up: Education Department Installs Biometric System to Ensure Based on Complex T-Spherical Hesitant Fuzzy Aczel-Alsina Aggregation Operators, 2024, 12, 2169-3536, 22624, 10.1109/ACCESS.2024.3359904 | |
4. | Yujie Gu, Menghao Xue, Yunwen Miao, Mingxuan Zhao, Qing Ma, Efficient fuzzy simulations for estimating the failure credibility of engineering structures under fuzzy environment, 2024, 155, 12709638, 109688, 10.1016/j.ast.2024.109688 | |
5. | Yuqi Zang, Jiamei Zhao, Wenchao Jiang, Tong Zhao, Advanced Linguistic Complex T-Spherical Fuzzy Dombi-Weighted Power-Partitioned Heronian Mean Operator and Its Application for Emergency Information Quality Assessment, 2024, 16, 2071-1050, 3069, 10.3390/su16073069 | |
6. | Weijia Ren, Yuhong Du, Ronglu Sun, Yuqin Du, Mubo Lü, A novel decision-making method based on complex cubic q-rung orthopair fuzzy information, 2023, 45, 10641246, 3213, 10.3233/JIFS-230402 | |
7. | Jiayou Shen, Nan Yang, Hejun Liang, A Hesitation-Associated Multi-Attribute Decision-Making Method Based on Generalized Interval-Valued Hesitation Fuzzy Weighted Heronian Averaging Operator, 2024, 12, 2227-7390, 3857, 10.3390/math12233857 |
C1 |
℘1(⟨0.4,2π(0.3)⟩,⟨0.2,2π(0.3)⟩,⟨0.5,2π(0.6)⟩) |
℘2(⟨0.3,2π(0.2)⟩,⟨0.5,2π(0.4)⟩,⟨0.4,2π(0.3)⟩) |
℘3(⟨0.6,2π(0.4)⟩,⟨0.3,2π(0.2)⟩,⟨0.2,2π(0.5)⟩) |
℘4(⟨0.2,2π(0.2)⟩,⟨0.4,2π(0.3)⟩,⟨0.8,2π(0.7)⟩) |
℘5(⟨0.1,2π(0.4)⟩,⟨0.5,2π(0.5)⟩,⟨0.7,2π(0.3)⟩) |
C2 |
(⟨0.3,2π(0.1)⟩,⟨0.4,2π(0.4)⟩,⟨0.2,2π(0.3)⟩) |
(⟨0.2,2π(0.5)⟩,⟨0.1,2π(0.2)⟩,⟨0.7,2π(0.4)⟩) |
(⟨0.4,2π(0.3)⟩,⟨0.3,2π(0.6)⟩,⟨0.8,2π(0.5)⟩) |
(⟨0.1,2π(0.2)⟩,⟨0.2,2π(0.8)⟩,⟨0.9,2π(0.1)⟩) |
(⟨0.5,2π(0.3)⟩,⟨0.5,2π(0.3)⟩,⟨0.3,2π(0.6)⟩) |
C3 |
(⟨0.2,2π(0.2)⟩,⟨0.5,2π(0.4)⟩,⟨0.3,2π(0.6)⟩) |
(⟨0.4,2π(0.5)⟩,⟨0.3,2π(0.7)⟩,⟨0.7,2π(0.3)⟩) |
(⟨0.3,2π(0.2)⟩,⟨0.8,2π(0.3)⟩,⟨0.5,2π(0.9)⟩) |
(⟨0.5,2π(0.4)⟩,⟨0.6,2π(0.5)⟩,⟨0.2,2π(0.4)⟩) |
(⟨0.1,2π(0.3)⟩,⟨0.2,2π(0.2)⟩,⟨0.9,2π(0.7)⟩) |
C4 |
(⟨0.2,2π(0.3)⟩,⟨0.4,2π(0.6)⟩,⟨0.5,2π(0.4)⟩) |
(⟨0.4,2π(0.2)⟩,⟨0.8,2π(0.3)⟩,⟨0.2,2π(0.8)⟩) |
(⟨0.5,2π(0.1)⟩,⟨0.3,2π(0.4)⟩,⟨0.7,2π(0.6)⟩) |
(⟨0.1,2π(0.4)⟩,⟨0.4,2π(0.1)⟩,⟨0.5,2π(0.9)⟩) |
(⟨0.2,2π(0.3)⟩,⟨0.6,2π(0.5)⟩,⟨0.4,2π(0.3)⟩) |
C1 |
℘1(⟨0.2,2π(0.3)⟩,⟨0.3,2π(0.2)⟩,⟨0.5,2π(0.4)⟩) |
℘2(⟨0.6,2π(0.4)⟩,⟨0.4,2π(0.3)⟩,⟨0.4,2π(0.7)⟩) |
℘3(⟨0.1,2π(0.2)⟩,⟨0.2,2π(0.4)⟩,⟨0.9,2π(0.8)⟩) |
℘4(⟨0.5,2π(0.3)⟩,⟨0.4,2π(0.5)⟩,⟨0.3,2π(0.6)⟩) |
℘5(⟨0.3,2π(0.1)⟩,⟨0.7,2π(0.3)⟩,⟨0.4,2π(0.2)⟩) |
C2 |
(⟨0.7,2π(0.2)⟩,⟨0.5,2π(0.6)⟩,⟨0.4,2π(0.5)⟩) |
(⟨0.3,2π(0.4)⟩,⟨0.4,2π(0.8)⟩,⟨0.6,2π(0.2)⟩) |
(⟨0.5,2π(0.3)⟩,⟨0.3,2π(0.4)⟩,⟨0.5,2π(0.7)⟩) |
(⟨0.2,2π(0.4)⟩,⟨0.7,2π(0.2)⟩,⟨0.3,2π(0.9)⟩) |
(⟨0.4,2π(0.8)⟩,⟨0.6,2π(0.5)⟩,⟨0.4,2π(0.2)⟩) |
C3 |
(⟨0.2,2π(0.2)⟩,⟨0.5,2π(0.4)⟩,⟨0.4,2π(0.6)⟩) |
(⟨0.4,2π(0.5)⟩,(0.3,2π(0.8)),(0.7,2π(0.2))) |
(⟨0.3,2π(0.1)⟩,⟨0.6,2π(0.6)⟩,⟨0.3,2π(0.5)⟩) |
(⟨0.4,2π(0.8)⟩,⟨0.9,2π(0.4)⟩,⟨0.5,2π(0.3)⟩) |
(⟨0.2,2π(0.1)⟩,⟨0.7,2π(0.2)⟩,⟨0.3,2π(0.9)⟩) |
C4 |
(⟨0.7,2π(0.3)⟩,⟨0.3,2π(0.4)⟩,⟨0.4,2π(0.8)⟩) |
(⟨0.3,2π(0.4)⟩,⟨0.5,2π(0.9)⟩,⟨0.6,2π(0.2)⟩) |
(⟨0.6,2π(0.5)⟩,⟨0.4,2π(0.5)⟩,⟨0.5,2π(0.3)⟩) |
(⟨0.5,2π(0.3)⟩,⟨0.9,2π(0.4)⟩,⟨0.3,2π(0.8)⟩) |
(⟨0.2,2π(0.6)⟩,⟨0.1,2π(0.3)⟩,⟨0.9,2π(0.5)⟩) |
C1 |
℘1(⟨0.2,2π(0.6)⟩,⟨0.5,2π(0.3)⟩,⟨0.3,2π(0.4)⟩) |
℘2(⟨0.5,2π(0.2)⟩,⟨0.1,2π(0.4)⟩,⟨0.7,2π(0.5)⟩) |
℘3(⟨0.8,2π(0.3)⟩,⟨0.3,2π(0.6)⟩,⟨0.4,2π(0.8)⟩) |
℘4(⟨0.3,2π(0.5)⟩,⟨0.4,2π(0.9)⟩,⟨0.6,2π(0.2)⟩) |
℘5(⟨0.6,2π(0.4)⟩,⟨0.6,2π(0.2)⟩,⟨0.5,2π(0.7)⟩) |
C2 |
(⟨0.5,2π(0.7)⟩,⟨0.6,2π(0.4)⟩,⟨0.3,2π(0.2)⟩) |
(⟨0.4,2π(0.2)⟩,⟨0.7,2π(0.5)⟩,⟨0.5,2π(0.6)⟩) |
(⟨0.6,2π(0.5)⟩,⟨0.3,2π(0.8)⟩,⟨0.4,2π(0.5)⟩) |
(⟨0.3,2π(0.4)⟩,⟨0.8,2π(0.6)⟩,⟨0.6,2π(0.4)⟩) |
(⟨0.7,2π(0.8)⟩,⟨0.4,2π(0.3)⟩,⟨0.8,2π(0.3)⟩) |
C3 |
(⟨0.7,2π(0.5)⟩,⟨0.4,2π(0.6)⟩,⟨0.3,2π(0.4)⟩) |
(⟨0.5,2π(0.8)⟩,⟨0.6,2π(0.4)⟩,⟨0.7,2π(0.2)⟩) |
(⟨0.6,2π(0.4)⟩,⟨0.5,2π(0.7)⟩,⟨0.4,2π(0.5)⟩) |
(⟨0.8,2π(0.6)⟩,⟨0.2,2π(0.5)⟩,⟨0.6,2π(0.7)⟩) |
(⟨0.4,2π(0.7)⟩,⟨0.3,2π(0.4)⟩,⟨0.8,2π(0.4)⟩) |
C4 |
(⟨0.5,2π(0.7)⟩,⟨0.7,2π(0.4)⟩,⟨0.5,2π(0.4)⟩) |
(⟨0.8,2π(0.5)⟩,⟨0.4,2π(0.6)⟩,⟨0.6,2π(0.5)⟩) |
(⟨0.6,2π(0.8)⟩,⟨0.5,2π(0.5)⟩,⟨0.3,2π(0.2)⟩) |
(⟨0.4,2π(0.2)⟩,⟨0.8,2π(0.7)⟩,⟨0.6,2π(0.6)⟩) |
(⟨0.3,2π(0.3)⟩,⟨0.9,2π(0.8)⟩,⟨0.7,2π(0.8)⟩) |
C1 |
℘1(⟨0.432,2π(0.123)⟩,⟨0.193,2π(0.345)⟩,⟨0.274,2π(0.213)⟩) |
℘2(⟨0.352,2π(0.254)⟩,⟨0.327,2π(0.351)⟩,⟨0.183,2π(0.301)⟩) |
℘3(⟨0.142,2π(0.242)⟩,⟨0.275,2π(0.221)⟩,⟨0.272,2π(0.121)⟩) |
℘4(⟨0.334,2π(0.331)⟩,(0.342,2π(0.141)),(0.319,2π(0.232))) |
℘5(⟨0.422,2π(0.224)⟩,⟨0.164,2π(0.232)⟩,⟨0.280,2π(0.326)⟩) |
C2 |
(⟨0.381,2π(0.326)⟩,⟨0.218,2π(0.127)⟩,⟨0.281,2π(0.190)⟩) |
(⟨0.255,2π(0.127)⟩,⟨0.119,2π(0.342)⟩,⟨0.188,2π(0.208)⟩) |
(⟨0.142,2π(0.469)⟩,⟨0.234,2π(0.121)⟩,⟨0.162,2π(0.165)⟩) |
(⟨0.361,2π(0.253)⟩,⟨0.311,2π(0.322)⟩,⟨0.231,2π(0.311)⟩) |
(⟨0.531,2π(0.326)⟩,⟨0.135,2π(0.231)⟩,⟨0.314,2π(0.253)⟩) |
C3 |
(⟨0.241,2π(0.114)⟩,⟨0.236,2π(0.112)⟩,⟨0.302,2π(0.291)⟩) |
(⟨0.376,2π(0.231)⟩,⟨0.212,2π(0.228)⟩,⟨0.233,2π(0.196)⟩) |
(⟨0.457,2π(0.262)⟩,⟨0.327,2π(0.241)⟩,⟨0.200,2π(0.175)⟩) |
(⟨0.332,2π(0.132)⟩,⟨0.219,2π(0.133)⟩,⟨0.173,2π(0.285)⟩) |
(⟨0.128,2π(0.313)⟩,⟨0.321,2π(0.236)⟩,⟨0.362,2π(0.321)⟩) |
C4 |
(⟨0.242,2π(0.168)⟩,⟨0.231,2π(0.211)⟩,⟨0.143,2π(0.112)⟩) |
(⟨0.364,2π(0.326)⟩,⟨0.212,2π(0.123)⟩,⟨0.231,2π(0.153)⟩) |
(⟨0.351,2π(0.235)⟩,⟨0.214,2π(0.342)⟩,⟨0.184,2π(0.207)⟩) |
(⟨0.131,2π(0.127)⟩,⟨0.281,2π(0.232)⟩,⟨0.166,2π(0.166)⟩) |
(⟨0.123,2π(0.272)⟩,⟨0.326,2π(0.131)⟩,⟨0.340,2π(0.214)⟩) |
C1 |
℘1(⟨0.125,2π(0.231)⟩,⟨0.244,2π(0.124)⟩,⟨0.276,2π(0.291)⟩) |
℘2(⟨0.342,2π(0.132)⟩,⟨0.221,2π(0.347)⟩,⟨0.201,2π(0.182)⟩) |
℘3(⟨0.250,2π(0.237)⟩,⟨0.313,2π(0.221)⟩,⟨0.193,2π(0.102)⟩) |
℘4(⟨0.213,2π(0.321)⟩,⟨0.131,2π(0.353)⟩,⟨0.201,2π(0.198)⟩) |
℘5(⟨0.123,2π(0.212)⟩,⟨0.243,2π(0.132)⟩,⟨0.223,2π(0.271)⟩) |
C2 |
(⟨0.213,2π(0.215)⟩,⟨0.139,2π(0.144)⟩,⟨0.321,2π(0.221)⟩) |
(⟨0.142,2π(0.336)⟩,⟨0.326,2π(0.242)⟩,⟨0.281,2π(0.183)⟩) |
(⟨0.325,2π(0.223)⟩,⟨0.433,2π(0.136)⟩,⟨0.163,2π(0.172)⟩) |
(⟨0.238,2π(0.232)⟩,⟨0.189,2π(0.336)⟩,⟨0.136,2π(0.261)⟩) |
(⟨0.137,2π(0.121)⟩,⟨0.231,2π(0.211)⟩,⟨0.275,2π(0.147)⟩) |
C3 |
(⟨0.212,2π(0.231)⟩,⟨0.237,2π(0.351)⟩,⟨0.231,2π(0.286)⟩) |
(⟨0.121,2π(0.132)⟩,⟨0.362,2π(0.191)⟩,⟨0.123,2π(0.133)⟩) |
(⟨0.238,2π(0.313)⟩,⟨0.223,2π(0.401)⟩,⟨0.248,2π(0.192)⟩) |
(⟨0.183,2π(0.151)⟩,⟨0.313,2π(0.232)⟩,⟨0.210,2π(0.316)⟩) |
(⟨0.332,2π(0.172)⟩,⟨0.128,2π(0.213)⟩,⟨0.321,2π(0.242)⟩) |
C4 |
(⟨0.192,2π(0.221)⟩,⟨0.339,2π(0.321)⟩,⟨0.243,2π(0.184)⟩) |
(⟨0.320,2π(0.113)⟩,⟨0.242,2π(0.252)⟩,⟨0.199,2π(0.121)⟩) |
(⟨0.222,2π(0.434)⟩,⟨0.355,2π(0.342)⟩,⟨0.326,2π(0.398)⟩) |
(⟨0.346,2π(0.231)⟩,⟨0.127,2π(0.221)⟩,⟨0.135,2π(0.231)⟩) |
(⟨0.138,2π(0.215)⟩,⟨0.212,2π(0.131)⟩,⟨0.272,2π(0.216)⟩) |
C1 |
E(1)(⟨0.2,2π(0.3)⟩,⟨0.5,2π(0.6)⟩,⟨0.3,2π(0.4)⟩) |
E(2)(⟨0.3,2π(0.5)⟩,⟨0.3,2π(0.1)⟩,⟨0.7,2π(0.8)⟩) |
E(3)(⟨0.5,2π(0.2)⟩,⟨0.8,2π(0.3)⟩,⟨0.5,2π(0.5)⟩) |
C2 |
(⟨0.2,2π(0.1)⟩,⟨0.6,2π(0.2)⟩,⟨0.4,2π(0.9)⟩) |
(⟨0.3,2π(0.4)⟩,⟨0.4,2π(0.6)⟩,⟨0.6,2π(0.5)⟩) |
(⟨0.4,2π(0.8)⟩,⟨0.5,2π(0.4)⟩,⟨0.7,2π(0.4)⟩) |
C3 |
(⟨0.2,2π(0.4)⟩,⟨0.6,2π(0.3)⟩,⟨0.4,2π(0.6)⟩) |
(⟨0.4,2π(0.6)⟩,⟨0.3,2π(0.5)⟩,⟨0.8,2π(0.4)⟩) |
(⟨0.5,2π(0.1)⟩,⟨0.4,2π(0.2)⟩,⟨0.6,2π(0.9)⟩) |
C4 |
(⟨0.1,2π(0.3)⟩,⟨0.6,2π(0.5)⟩,⟨0.8,2π(0.7)⟩) |
(⟨0.6,2π(0.4)⟩,⟨0.2,2π(0.2)⟩,⟨0.7,2π(0.9)⟩) |
(⟨0.3,2π(0.7)⟩,⟨0.5,2π(0.4)⟩,⟨0.4,2π(0.3)⟩) |
C1 |
℘1(⟨0.4,2π(0.3)⟩,⟨0.2,2π(0.3)⟩,⟨0.5,2π(0.6)⟩) |
℘2(⟨0.3,2π(0.2)⟩,⟨0.5,2π(0.4)⟩,⟨0.4,2π(0.3)⟩) |
℘3(⟨0.6,2π(0.4)⟩,⟨0.3,2π(0.2)⟩,⟨0.2,2π(0.5)⟩) |
℘4(⟨0.2,2π(0.2)⟩,⟨0.4,2π(0.3)⟩,⟨0.8,2π(0.7)⟩) |
℘5(⟨0.1,2π(0.4)⟩,⟨0.5,2π(0.5)⟩,⟨0.7,2π(0.3)⟩) |
C2 |
(⟨0.3,2π(0.1)⟩,⟨0.4,2π(0.4)⟩,⟨0.2,2π(0.3)⟩) |
(⟨0.2,2π(0.5)⟩,⟨0.1,2π(0.2)⟩,⟨0.7,2π(0.4)⟩) |
(⟨0.4,2π(0.3)⟩,⟨0.3,2π(0.6)⟩,⟨0.8,2π(0.5)⟩) |
(⟨0.1,2π(0.2)⟩,⟨0.2,2π(0.8)⟩,⟨0.9,2π(0.1)⟩) |
(⟨0.5,2π(0.3)⟩,⟨0.5,2π(0.3)⟩,⟨0.3,2π(0.6)⟩) |
C3 |
(⟨0.2,2π(0.2)⟩,⟨0.5,2π(0.4)⟩,⟨0.3,2π(0.6)⟩) |
(⟨0.4,2π(0.5)⟩,⟨0.3,2π(0.7)⟩,⟨0.7,2π(0.3)⟩) |
(⟨0.3,2π(0.2)⟩,⟨0.8,2π(0.3)⟩,⟨0.5,2π(0.9)⟩) |
(⟨0.5,2π(0.4)⟩,⟨0.6,2π(0.5)⟩,⟨0.2,2π(0.4)⟩) |
(⟨0.1,2π(0.3)⟩,⟨0.2,2π(0.2)⟩,⟨0.9,2π(0.7)⟩) |
C4 |
(⟨0.2,2π(0.3)⟩,⟨0.4,2π(0.6)⟩,⟨0.5,2π(0.4)⟩) |
(⟨0.4,2π(0.2)⟩,⟨0.8,2π(0.3)⟩,⟨0.2,2π(0.8)⟩) |
(⟨0.5,2π(0.1)⟩,⟨0.3,2π(0.4)⟩,⟨0.7,2π(0.6)⟩) |
(⟨0.1,2π(0.4)⟩,⟨0.4,2π(0.1)⟩,⟨0.5,2π(0.9)⟩) |
(⟨0.2,2π(0.3)⟩,⟨0.6,2π(0.5)⟩,⟨0.4,2π(0.3)⟩) |
C1 |
℘1(⟨0.2,2π(0.3)⟩,⟨0.3,2π(0.2)⟩,⟨0.5,2π(0.4)⟩) |
℘2(⟨0.6,2π(0.4)⟩,⟨0.4,2π(0.3)⟩,⟨0.4,2π(0.7)⟩) |
℘3(⟨0.1,2π(0.2)⟩,⟨0.2,2π(0.4)⟩,⟨0.9,2π(0.8)⟩) |
℘4(⟨0.5,2π(0.3)⟩,⟨0.4,2π(0.5)⟩,⟨0.3,2π(0.6)⟩) |
℘5(⟨0.3,2π(0.1)⟩,⟨0.7,2π(0.3)⟩,⟨0.4,2π(0.2)⟩) |
C2 |
(⟨0.7,2π(0.2)⟩,⟨0.5,2π(0.6)⟩,⟨0.4,2π(0.5)⟩) |
(⟨0.3,2π(0.4)⟩,⟨0.4,2π(0.8)⟩,⟨0.6,2π(0.2)⟩) |
(⟨0.5,2π(0.3)⟩,⟨0.3,2π(0.4)⟩,⟨0.5,2π(0.7)⟩) |
(⟨0.2,2π(0.4)⟩,⟨0.7,2π(0.2)⟩,⟨0.3,2π(0.9)⟩) |
(⟨0.4,2π(0.8)⟩,⟨0.6,2π(0.5)⟩,⟨0.4,2π(0.2)⟩) |
C3 |
(⟨0.2,2π(0.2)⟩,⟨0.5,2π(0.4)⟩,⟨0.4,2π(0.6)⟩) |
(⟨0.4,2π(0.5)⟩,(0.3,2π(0.8)),(0.7,2π(0.2))) |
(⟨0.3,2π(0.1)⟩,⟨0.6,2π(0.6)⟩,⟨0.3,2π(0.5)⟩) |
(⟨0.4,2π(0.8)⟩,⟨0.9,2π(0.4)⟩,⟨0.5,2π(0.3)⟩) |
(⟨0.2,2π(0.1)⟩,⟨0.7,2π(0.2)⟩,⟨0.3,2π(0.9)⟩) |
C4 |
(⟨0.7,2π(0.3)⟩,⟨0.3,2π(0.4)⟩,⟨0.4,2π(0.8)⟩) |
(⟨0.3,2π(0.4)⟩,⟨0.5,2π(0.9)⟩,⟨0.6,2π(0.2)⟩) |
(⟨0.6,2π(0.5)⟩,⟨0.4,2π(0.5)⟩,⟨0.5,2π(0.3)⟩) |
(⟨0.5,2π(0.3)⟩,⟨0.9,2π(0.4)⟩,⟨0.3,2π(0.8)⟩) |
(⟨0.2,2π(0.6)⟩,⟨0.1,2π(0.3)⟩,⟨0.9,2π(0.5)⟩) |
C1 |
℘1(⟨0.2,2π(0.6)⟩,⟨0.5,2π(0.3)⟩,⟨0.3,2π(0.4)⟩) |
℘2(⟨0.5,2π(0.2)⟩,⟨0.1,2π(0.4)⟩,⟨0.7,2π(0.5)⟩) |
℘3(⟨0.8,2π(0.3)⟩,⟨0.3,2π(0.6)⟩,⟨0.4,2π(0.8)⟩) |
℘4(⟨0.3,2π(0.5)⟩,⟨0.4,2π(0.9)⟩,⟨0.6,2π(0.2)⟩) |
℘5(⟨0.6,2π(0.4)⟩,⟨0.6,2π(0.2)⟩,⟨0.5,2π(0.7)⟩) |
C2 |
(⟨0.5,2π(0.7)⟩,⟨0.6,2π(0.4)⟩,⟨0.3,2π(0.2)⟩) |
(⟨0.4,2π(0.2)⟩,⟨0.7,2π(0.5)⟩,⟨0.5,2π(0.6)⟩) |
(⟨0.6,2π(0.5)⟩,⟨0.3,2π(0.8)⟩,⟨0.4,2π(0.5)⟩) |
(⟨0.3,2π(0.4)⟩,⟨0.8,2π(0.6)⟩,⟨0.6,2π(0.4)⟩) |
(⟨0.7,2π(0.8)⟩,⟨0.4,2π(0.3)⟩,⟨0.8,2π(0.3)⟩) |
C3 |
(⟨0.7,2π(0.5)⟩,⟨0.4,2π(0.6)⟩,⟨0.3,2π(0.4)⟩) |
(⟨0.5,2π(0.8)⟩,⟨0.6,2π(0.4)⟩,⟨0.7,2π(0.2)⟩) |
(⟨0.6,2π(0.4)⟩,⟨0.5,2π(0.7)⟩,⟨0.4,2π(0.5)⟩) |
(⟨0.8,2π(0.6)⟩,⟨0.2,2π(0.5)⟩,⟨0.6,2π(0.7)⟩) |
(⟨0.4,2π(0.7)⟩,⟨0.3,2π(0.4)⟩,⟨0.8,2π(0.4)⟩) |
C4 |
(⟨0.5,2π(0.7)⟩,⟨0.7,2π(0.4)⟩,⟨0.5,2π(0.4)⟩) |
(⟨0.8,2π(0.5)⟩,⟨0.4,2π(0.6)⟩,⟨0.6,2π(0.5)⟩) |
(⟨0.6,2π(0.8)⟩,⟨0.5,2π(0.5)⟩,⟨0.3,2π(0.2)⟩) |
(⟨0.4,2π(0.2)⟩,⟨0.8,2π(0.7)⟩,⟨0.6,2π(0.6)⟩) |
(⟨0.3,2π(0.3)⟩,⟨0.9,2π(0.8)⟩,⟨0.7,2π(0.8)⟩) |
C1 |
℘1(⟨0.432,2π(0.123)⟩,⟨0.193,2π(0.345)⟩,⟨0.274,2π(0.213)⟩) |
℘2(⟨0.352,2π(0.254)⟩,⟨0.327,2π(0.351)⟩,⟨0.183,2π(0.301)⟩) |
℘3(⟨0.142,2π(0.242)⟩,⟨0.275,2π(0.221)⟩,⟨0.272,2π(0.121)⟩) |
℘4(⟨0.334,2π(0.331)⟩,(0.342,2π(0.141)),(0.319,2π(0.232))) |
℘5(⟨0.422,2π(0.224)⟩,⟨0.164,2π(0.232)⟩,⟨0.280,2π(0.326)⟩) |
C2 |
(⟨0.381,2π(0.326)⟩,⟨0.218,2π(0.127)⟩,⟨0.281,2π(0.190)⟩) |
(⟨0.255,2π(0.127)⟩,⟨0.119,2π(0.342)⟩,⟨0.188,2π(0.208)⟩) |
(⟨0.142,2π(0.469)⟩,⟨0.234,2π(0.121)⟩,⟨0.162,2π(0.165)⟩) |
(⟨0.361,2π(0.253)⟩,⟨0.311,2π(0.322)⟩,⟨0.231,2π(0.311)⟩) |
(⟨0.531,2π(0.326)⟩,⟨0.135,2π(0.231)⟩,⟨0.314,2π(0.253)⟩) |
C3 |
(⟨0.241,2π(0.114)⟩,⟨0.236,2π(0.112)⟩,⟨0.302,2π(0.291)⟩) |
(⟨0.376,2π(0.231)⟩,⟨0.212,2π(0.228)⟩,⟨0.233,2π(0.196)⟩) |
(⟨0.457,2π(0.262)⟩,⟨0.327,2π(0.241)⟩,⟨0.200,2π(0.175)⟩) |
(⟨0.332,2π(0.132)⟩,⟨0.219,2π(0.133)⟩,⟨0.173,2π(0.285)⟩) |
(⟨0.128,2π(0.313)⟩,⟨0.321,2π(0.236)⟩,⟨0.362,2π(0.321)⟩) |
C4 |
(⟨0.242,2π(0.168)⟩,⟨0.231,2π(0.211)⟩,⟨0.143,2π(0.112)⟩) |
(⟨0.364,2π(0.326)⟩,⟨0.212,2π(0.123)⟩,⟨0.231,2π(0.153)⟩) |
(⟨0.351,2π(0.235)⟩,⟨0.214,2π(0.342)⟩,⟨0.184,2π(0.207)⟩) |
(⟨0.131,2π(0.127)⟩,⟨0.281,2π(0.232)⟩,⟨0.166,2π(0.166)⟩) |
(⟨0.123,2π(0.272)⟩,⟨0.326,2π(0.131)⟩,⟨0.340,2π(0.214)⟩) |
C1 |
℘1(⟨0.125,2π(0.231)⟩,⟨0.244,2π(0.124)⟩,⟨0.276,2π(0.291)⟩) |
℘2(⟨0.342,2π(0.132)⟩,⟨0.221,2π(0.347)⟩,⟨0.201,2π(0.182)⟩) |
℘3(⟨0.250,2π(0.237)⟩,⟨0.313,2π(0.221)⟩,⟨0.193,2π(0.102)⟩) |
℘4(⟨0.213,2π(0.321)⟩,⟨0.131,2π(0.353)⟩,⟨0.201,2π(0.198)⟩) |
℘5(⟨0.123,2π(0.212)⟩,⟨0.243,2π(0.132)⟩,⟨0.223,2π(0.271)⟩) |
C2 |
(⟨0.213,2π(0.215)⟩,⟨0.139,2π(0.144)⟩,⟨0.321,2π(0.221)⟩) |
(⟨0.142,2π(0.336)⟩,⟨0.326,2π(0.242)⟩,⟨0.281,2π(0.183)⟩) |
(⟨0.325,2π(0.223)⟩,⟨0.433,2π(0.136)⟩,⟨0.163,2π(0.172)⟩) |
(⟨0.238,2π(0.232)⟩,⟨0.189,2π(0.336)⟩,⟨0.136,2π(0.261)⟩) |
(⟨0.137,2π(0.121)⟩,⟨0.231,2π(0.211)⟩,⟨0.275,2π(0.147)⟩) |
C3 |
(⟨0.212,2π(0.231)⟩,⟨0.237,2π(0.351)⟩,⟨0.231,2π(0.286)⟩) |
(⟨0.121,2π(0.132)⟩,⟨0.362,2π(0.191)⟩,⟨0.123,2π(0.133)⟩) |
(⟨0.238,2π(0.313)⟩,⟨0.223,2π(0.401)⟩,⟨0.248,2π(0.192)⟩) |
(⟨0.183,2π(0.151)⟩,⟨0.313,2π(0.232)⟩,⟨0.210,2π(0.316)⟩) |
(⟨0.332,2π(0.172)⟩,⟨0.128,2π(0.213)⟩,⟨0.321,2π(0.242)⟩) |
C4 |
(⟨0.192,2π(0.221)⟩,⟨0.339,2π(0.321)⟩,⟨0.243,2π(0.184)⟩) |
(⟨0.320,2π(0.113)⟩,⟨0.242,2π(0.252)⟩,⟨0.199,2π(0.121)⟩) |
(⟨0.222,2π(0.434)⟩,⟨0.355,2π(0.342)⟩,⟨0.326,2π(0.398)⟩) |
(⟨0.346,2π(0.231)⟩,⟨0.127,2π(0.221)⟩,⟨0.135,2π(0.231)⟩) |
(⟨0.138,2π(0.215)⟩,⟨0.212,2π(0.131)⟩,⟨0.272,2π(0.216)⟩) |
C1 |
E(1)(⟨0.2,2π(0.3)⟩,⟨0.5,2π(0.6)⟩,⟨0.3,2π(0.4)⟩) |
E(2)(⟨0.3,2π(0.5)⟩,⟨0.3,2π(0.1)⟩,⟨0.7,2π(0.8)⟩) |
E(3)(⟨0.5,2π(0.2)⟩,⟨0.8,2π(0.3)⟩,⟨0.5,2π(0.5)⟩) |
C2 |
(⟨0.2,2π(0.1)⟩,⟨0.6,2π(0.2)⟩,⟨0.4,2π(0.9)⟩) |
(⟨0.3,2π(0.4)⟩,⟨0.4,2π(0.6)⟩,⟨0.6,2π(0.5)⟩) |
(⟨0.4,2π(0.8)⟩,⟨0.5,2π(0.4)⟩,⟨0.7,2π(0.4)⟩) |
C3 |
(⟨0.2,2π(0.4)⟩,⟨0.6,2π(0.3)⟩,⟨0.4,2π(0.6)⟩) |
(⟨0.4,2π(0.6)⟩,⟨0.3,2π(0.5)⟩,⟨0.8,2π(0.4)⟩) |
(⟨0.5,2π(0.1)⟩,⟨0.4,2π(0.2)⟩,⟨0.6,2π(0.9)⟩) |
C4 |
(⟨0.1,2π(0.3)⟩,⟨0.6,2π(0.5)⟩,⟨0.8,2π(0.7)⟩) |
(⟨0.6,2π(0.4)⟩,⟨0.2,2π(0.2)⟩,⟨0.7,2π(0.9)⟩) |
(⟨0.3,2π(0.7)⟩,⟨0.5,2π(0.4)⟩,⟨0.4,2π(0.3)⟩) |