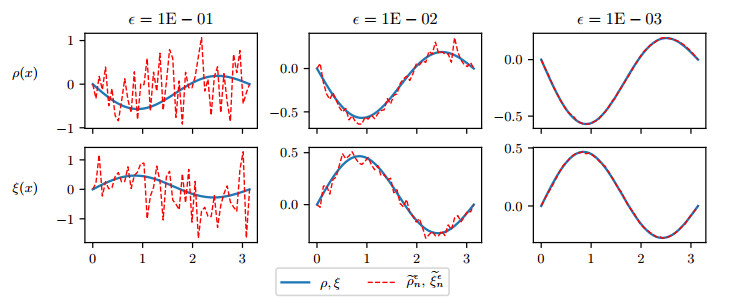
The main goal of this work is to study a regularization method to reconstruct the solution of the backward non-linear hyperbolic equation utt+αΔ2ut+βΔ2u=F(x,t,u) come with the input data are blurred by random Gaussian white noise. We first prove that the considered problem is ill-posed (in the sense of Hadamard), i.e., the solution does not depend continuously on the data. Then we propose the Fourier truncation method for stabilizing the ill-posed problem. Base on some priori assumptions for the true solution we derive the error and a convergence rate between a mild solution and its regularized solutions. Also, a numerical example is provided to confirm the efficiency of theoretical results.
Citation: Phuong Nguyen Duc, Erkan Nane, Omid Nikan, Nguyen Anh Tuan. Approximation of the initial value for damped nonlinear hyperbolic equations with random Gaussian white noise on the measurements[J]. AIMS Mathematics, 2022, 7(7): 12620-12634. doi: 10.3934/math.2022698
[1] | Zeliha Korpinar, Mustafa Inc, Ali S. Alshomrani, Dumitru Baleanu . The deterministic and stochastic solutions of the Schrodinger equation with time conformable derivative in birefrigent fibers. AIMS Mathematics, 2020, 5(3): 2326-2345. doi: 10.3934/math.2020154 |
[2] | Hatice Taskesen . Qualitative results for a relativistic wave equation with multiplicative noise and damping terms. AIMS Mathematics, 2023, 8(7): 15232-15254. doi: 10.3934/math.2023778 |
[3] | Areej A. Almoneef, Abd-Allah Hyder, Mohamed A. Barakat, Abdelrheem M. Aly . Stochastic solutions of the geophysical KdV equation: Numerical simulations and white noise impact. AIMS Mathematics, 2025, 10(3): 5859-5879. doi: 10.3934/math.2025269 |
[4] | Da Shi, Zhao Li, Dan Chen . New traveling wave solutions, phase portrait and chaotic patterns for the dispersive concatenation model with spatio-temporal dispersion having multiplicative white noise. AIMS Mathematics, 2024, 9(9): 25732-25751. doi: 10.3934/math.20241257 |
[5] | Hui Sun, Yangyang Lyu . Temporal Hölder continuity of the parabolic Anderson model driven by a class of time-independent Gaussian fields with rough initial conditions. AIMS Mathematics, 2024, 9(12): 34838-34862. doi: 10.3934/math.20241659 |
[6] | Dan Chen, Da Shi, Feng Chen . Qualitative analysis and new traveling wave solutions for the stochastic Biswas-Milovic equation. AIMS Mathematics, 2025, 10(2): 4092-4119. doi: 10.3934/math.2025190 |
[7] | Nafissa T. Trouba, Huiying Xu, Mohamed E. M. Alngar, Reham M. A. Shohib, Haitham A. Mahmoud, Xinzhong Zhu . Soliton solutions and stability analysis of the stochastic nonlinear reaction-diffusion equation with multiplicative white noise in soliton dynamics and optical physics. AIMS Mathematics, 2025, 10(1): 1859-1881. doi: 10.3934/math.2025086 |
[8] | Hamood Ur Rehman, Aziz Ullah Awan, Sayed M. Eldin, Ifrah Iqbal . Study of optical stochastic solitons of Biswas-Arshed equation with multiplicative noise. AIMS Mathematics, 2023, 8(9): 21606-21621. doi: 10.3934/math.20231101 |
[9] | Abdelkader Moumen, Khaled A. Aldwoah, Muntasir Suhail, Alwaleed Kamel, Hicham Saber, Manel Hleili, Sayed Saifullah . Investigation of more solitary waves solutions of the stochastics Benjamin-Bona-Mahony equation under beta operator. AIMS Mathematics, 2024, 9(10): 27403-27417. doi: 10.3934/math.20241331 |
[10] | Wael W. Mohammed, Farah M. Al-Askar . New stochastic solitary solutions for the modified Korteweg-de Vries equation with stochastic term/random variable coefficients. AIMS Mathematics, 2024, 9(8): 20467-20481. doi: 10.3934/math.2024995 |
The main goal of this work is to study a regularization method to reconstruct the solution of the backward non-linear hyperbolic equation utt+αΔ2ut+βΔ2u=F(x,t,u) come with the input data are blurred by random Gaussian white noise. We first prove that the considered problem is ill-posed (in the sense of Hadamard), i.e., the solution does not depend continuously on the data. Then we propose the Fourier truncation method for stabilizing the ill-posed problem. Base on some priori assumptions for the true solution we derive the error and a convergence rate between a mild solution and its regularized solutions. Also, a numerical example is provided to confirm the efficiency of theoretical results.
Given an open bounded domain Ω⊂Rd which has a smooth boundary Γ, and a positive real number T. We consider the non-linear hyperbolic partial different equation with the strong damping αΔ2ut, as follows
utt+αΔ2ut+βΔ2u=F(x,t,u),(x,t)∈Ω×(0,T), | (1.1) |
associated with the final value functions
u(x,T)=ρ(x),ut(x,T)=ξ(x),x∈Ω, | (1.2) |
and the Dirichlet boundary condition
u(x,t)=0,(x,t)∈Γ×(0,T), | (1.3) |
where α,β are positive constants, and the source F(x,t,u) is a given function of the variable u.
As we all know, the amplitude of a wave is related to the amount of energy it carries. A high amplitude wave carries a large amount of energy and vice versa. A wave propagates through a certain environment, its energy will decrease as time goes on, so wave amplitude also decreases (called damped wave). The damped wave equations are widely used in science and engineering, especially in physics. They can describe how waves propagate. It applies to all kinds of waves, from water waves [8] to sound and vibrations [13,21], and even light and radio waves [10].
Let us briefly describe some previous results related to the Problem (1.1). In recent years, much attention has been paid to the study on the properties and asymptotic behavior of the solution on Problem (1.1) subject to the initial conditions u(x,0)=ρ(x), ut(x,0)=ξ(x) (pioneering works [1,2,5,9,15]). However, to the best of our knowledge, there are not any result on backward problem (1.1)–(1.3).
In practice we usually do not have these final value functions, instead they are suggested from the experience of the researcher. A more reliable way is to use their observed values. However, we all know that observations always come with random errors, these errors are derived from the ability of the measuring device (measurement error). It is therefore natural that observations are observed usually in the presence of some noise. In this paper, we will consider the case where these perturbation are an additive stochastic white noise
ρϵ(x)=ρ(x)+ϵW(x),ξϵ(x)=ξ(x)+ϵW(x), | (1.4) |
where ϵ is the amplitude of the noise and W(x) is a Gaussian white noise process. Suppose further that even the observations (1.4) cannot be observed exactly, but they can only be observed in discretized form
⟨ρϵ,φp⟩=⟨ρ,φp⟩+ϵ⟨W,φp⟩,⟨ξϵ,φp⟩=⟨ξ,φp⟩+ϵ⟨W,φp⟩,p=1,…,N, | (1.5) |
where {φp} is a orthonormal basic of Hilbert space H; ⟨,⟩ denotes the inner product in H; Wp:=⟨W,φp⟩ are standard normal distribution; and ⟨ρϵ,φp⟩ are independent random variables for orthonormal functions φp. For more detail on the white noise model see, [3,11,12].
It is well-known that Problem (1.1)–(1.4) is ill-posed in the sense of Hadamard (if the solution exists, then it does not depend continuously on the final values), and regularization methods for it are required. The aim of this paper is to recover the unknown final value functions ρ, ξ from indirect and noisy discrete observations (1.5) and then we use them to establish a regularized solution by the Fourier truncation method. To the best of our knowledge, the present paper may be the fist study for ill-posed problem for hyperbolic equations with Gaussian white noise. We have learned more ideas from these articles[14,17,18,20], but the detailed technique is different.
The organizational structure of this paper is as follows. Section 2 introduces some preliminary materials. Section 3 uses the Fourier series to obtain the mild solution and analyse the ill-posedness of problem. Section 4 presents an example of an ill-posed problem with random noise. In Section 5, we draw into main results: first we propose a new regularized solution, and then we give the convergent estimates between a mild solution and a regularized solution under some priori assumptions on the exact solution. To end this section, we discuss a regularization parameter choice rule. Finally, Section 6 reports numerical implementations to support our theoretical results and to show the validity of the proposed reconstruction method.
Throughout this paper, let us denote the Hilbert space H:=L2(Ω), and ⟨⋅,⋅⟩ is the inner product of H. Since Ω is the bounded open set, there exists a Hilbert orthonormal basic {φp}∞p=1 in H (φp∈H10(Ω)∩C∞(Ω)) and a sequence {λp}∞p=1 of real, 0≤λ1≤λ2≤…≤limp→∞λp=+∞, such that −Δφp(x)=λpφp(x) for x∈Ω and φp(x)=0 for x∈∂Ω. We say that λp are the eigenvalues of of −Δ and φp are the associated eigenfunctions. The Sobolev class of function is defined as follows
Hμ={f∈H:∞∑p=1λμp⟨f,φp⟩2<∞}. |
It is a Hilbert space endowed with the norm ‖f‖2Hμ=∑∞p=1λμp⟨f,φp⟩2. For τ,ν>0, following [4,6], we introduce the special Gevrey classes of functions
Gσ,ν={f∈H:∞∑p=1eσλpλνp⟨f,ep⟩2<+∞}. |
We remark that Gσ,ν is also the Hilbert space endowed with the norm ‖f‖2Gσ,ν=∑∞p=1eσλpλνp⟨f,ei⟩2.
Definition 2.1 (Bochner space [22]). Given a probability measure space (˜Ω,M,μ), a Hilbert space H. The Bochner space L2(˜Ω,H)≡L2((˜Ω,M,μ);H) is defined to be the functions u:˜Ω↦H such that the corresponding norm is finite
‖u‖L2(˜Ω,H):=(∫˜Ω‖u(ω)‖2Hdμ(ω))1/2=(E‖u‖2H)1/2<+∞. | (2.1) |
Definition 2.2 (Reconstruction of the final value functions). Given ρ,ξ∈Hμ (μ>0), which have sequences of n (is known as sample size) discrete observations ⟨ρϵ,φp⟩ and ⟨ξϵ,φp⟩, p=1,…,n. Non-parametric estimation of ρ and ξ are suggested as
˜ρn(x)=n∑p=1⟨ρϵ,φp⟩φp(x),˜ξϵn(x)=n∑p=1⟨ξϵ,φp⟩φp(x). | (2.2) |
Lemma 2.1. Given ρ,ξ∈Hμ (μ>0), then the estimation errors are
E||˜ρn−ρ||2H≤ϵ2n+1λμn||ρ||2Hμ,E‖˜ξN−ξ‖2H≤ϵ2n+1λμn||ξ||2Hμ. | (2.3) |
Here n(ϵ):=n depends on ϵ and satisfies that limϵ→0+n(ϵ)=+∞.
Proof. Our proof starts with the observation that
E||˜ρn−ρ||H=E(n∑p=1⟨ρϵ−ρ,φp⟩2)+∞∑p=n+1⟨ρ,φp⟩2=ϵE(n∑p=1W2p)+∞∑p=n+1λ−μpλμp⟨ρ,φp⟩2≤ϵE(n∑p=1W2p)+1λμn∞∑p=n+1λμp⟨ρ,φp⟩2. |
The assumption Wp=⟨W,φp⟩iid∼N(0,1) implies that EW2p=1. We then have the desired the first result. The same conclusion can be drawn for the remaining case.
Taking the inner product on both side of (1.1) and (1.2) with φp, and set up(t)=⟨u(⋅,t),φp⟩, ρp(t)=⟨ρ,φp⟩, ξp(t)=⟨ξ,φp⟩, and Fp(u)=⟨F(⋅,t,u(⋅,t)),φp⟩, then
{u″p(t)+αλ2pu′p(t)+βλ2pup=Fp(u)(t),up(T)=ρp,up′(T)=ξp. | (3.1) |
In this work we assume that Δp:=α2λ4p−4βλ2p>0 then a quadratic equation k2−αλ2pk+βλ2p=0 has two different solutions k−p=αλ2p−√Δp2,k+p=αλ2p+√Δp2. Multiplying both sides the first equation of System (3.1) by ϕp(τ)=e(τ−t)k+p−e(τ−t)k−p√Δp, and integrating both sides from t to T,
∫Ttϕp(τ)u″p(τ)dτ+αλ2p∫Ttϕp(τ)u′p(τ)dτ+βλ2p∫Ttϕp(τ)updτ=∫Ttϕp(τ)Fp(u)(τ)dτ. | (3.2) |
The left hand side of (3.2) now becomes
[ϕp(τ)u′p(τ)−ϕ′p(τ)up(τ)+αλ2pϕp(τ)up(τ)]Tt+∫Tt[ϕ″p(τ)−αλ2pϕ′p(τ)+βλ2pϕp(τ)]up(τ)dτ. |
Since k−p, k+p satisfy the equation k2−αλ2pk+βλ2p=0, then ϕ″(τ)−αλ2pϕ′p(τ)+βλ2p=0. Hence, (3.2) becomes
[ϕp(τ)u′p(τ)−ϕ′p(τ)up(τ)+αλ2pϕp(τ)up(τ)]Tt=∫Ttϕp(τ)Fp(u)(τ)dτ. | (3.3) |
It is worth noticing that ϕp(t)=0, ϕ′p(t)=1 and −ϕ′p(T)+αλ2pϕp(T)=k−pe(T−t)k+p−k+pe(T−t)k−p√Δp. Therefore, (3.3) now becomes
up(t)=k+pe(T−t)k−p−k−pe(T−t)k+p√Δpρp−e(T−t)k+p−e(T−t)k−p√Δpξp+∫Ttk+pe(τ−t)k−p−k−pe(τ−t)k+p√ΔpFp(u)(τ)dτ. |
Lemma 3.1. Let ρ,ξ∈H. Suppose that the given problem (1.1)–(1.3) has a solution u∈C([0,T],H), then the mild solution is represented in terms of the Fourier series as follows
u(x,t)=R(T−t)ρ(x)−S(T−t)ξ(x)+∫TtS(τ−t)F(x,τ,u)dτ, | (3.4) |
where the operators R(t)f and S(t)f are
R(t)f=∞∑p=1(k+petk−p−k−petk+p√Δp⟨f,φp⟩)φp(x);S(t)f=∞∑p=1(etk+p−etk−p√Δp⟨f,φp⟩)φp(x). | (3.5) |
In this section, we present an example of Problem (1.1)–(1.3) with random noise (1.4) which is ill-posed in the sense of Hadamard (does not depend continuously on the final data). We consider the particular case as follows
{˜untt+αΔ2˜unt+βΔ2˜un=F(˜un),(x,t)∈Ω×(0,T),˜un(x,T)=0,x∈Ω,˜unt(x,T)=˜ξϵn(x),x∈Ω,˜un(x,t)=0,(x,t)∈Γ×(0,T), | (4.1) |
where F(˜un)(x,t)=∑∞p=1e−αλ2pT2T2⟨˜un(⋅,t),φp⟩φp(x). For simple computation, we assume that Ω=(0,π). It immediately follows that λp=p2. We assume further that the function ξ(x)=0 (unknown) has observations ⟨ξϵ,φp⟩=ϵ⟨W,φp⟩, p=1,…,n. Then the statistical estimate of ξ(x) is in the form.
˜ξϵn(x)=n∑p=1ϵ⟨W,φp⟩φp(x). | (4.2) |
Using Lemma 3.1, System (4.1) has the mild solution
˜un(x,t)=−S(T−t)˜ξϵn+∫TtS(τ−t)F(˜un)(τ)dτ. | (4.3) |
We first show that this nonlinear integral equation has unique solution ˜un∈L∞([0,T];L2(˜Ω,H)). Indeed, let us denote
Φ(u)(x,t)=−S(T−t)˜ξϵn+∫TtS(τ−t)F(u)(τ)dτ. | (4.4) |
Let u1,u2∈L∞([0,T];L2(˜Ω,H)). Using the Hölder inequality and Parseval's identity, we obtain
E‖Φ(u1)(⋅,t)−Φ(u2)(⋅,t)‖2H=E‖∫TtS(τ−t)(F(u1)(⋅,τ)−F(u2)(⋅,τ))dτ‖2H≤TE∫Tt∞∑p=1(e(τ−t)k+p−e(τ−t)k−p√Δp⏟Πp(τ)⟨F(v1)(⋅,τ)−F(v2)(⋅,τ),φp⟩⏟ΠFp(τ))2dτ. |
Since |e−(τ−t)k−p−e−(τ−t)k+p|≤(τ−t)|k+p−k−p|≤T√Δp and (τ−t)(k+p+k−p)≤Tαλ2p, then
|Πp(τ)|=e(τ−t)(k+p+k−p)|e−(τ−t)k−p−e−(τ−t)k+p|√Δp≤Teαλ2pT. | (4.5) |
From defining the function F as above, it follows that ΠFp(τ)=e−αλ2pT2T2⟨u1(⋅,τ)−u2(⋅,τ),φp⟩. Thus
E‖Φ(u1)(⋅,t)−Φ(u2)(⋅,t)‖2H≤14TE∫Tt∞∑p=1⟨u1(⋅,τ)−u2(⋅,τ),φp⟩2dτ≤14‖u1−u2‖2L∞([0,T];L2(˜Ω,H)). |
Hence, we have that ‖Φ(u1)−Φ(u2)‖2L∞([0,T];L2(˜Ω,H))≤14‖u1−u2‖2L∞([0,T];L2(˜Ω,H)). This means that Φ is a contraction. The Banach fixed point theorem leads to a conclude that Φ(u)=u has a unique solution u∈L∞([0,T];L2(˜Ω,H)).
We then point out that System (4.1) does not depend continuously on the final data. We start by
E‖˜un(⋅,t)‖2H≥E‖S(T−t)˜ξϵn‖2H−12E‖∫TtS(τ−t)F(˜un)(τ)dτ‖2H. | (4.6) |
It is easy to verify that
E‖∫TtS(τ−t)F(˜un)(τ)dτ‖2H≤14E‖u(⋅,t)‖2H. |
This leads to
E‖˜un(⋅,t)‖2H≥89E‖S(T−t)˜ξϵn‖2H. | (4.7) |
It is worth recalling that E⟨˜ξϵn,φp⟩2=ϵ2, so
E‖S(T−t)˜ξϵn‖2H=n∑p=1[e(T−t)k+p−e(T−t)k−pk+p−k−p]2E⟨˜ξϵn,φp⟩2≥[e(T−t)k+n−e(T−t)k−nk+n−k−n]2ϵ2. | (4.8) |
We note that k+n−k−n=√Δp=√α2λ4n−βλ2n>√α2λ41−βλ21, then we have
[e(T−t)k+n−e(T−t)k−nk+n−k−n]2=e2(T−t)k+n[1−e−(T−t)(k+n−k−n)]2(k+n−k−n)2≥e2(T−t)k+n[1−e−(T−t)√α2λ41−4βλ21]2α2λ4n−4βλ2n. |
The function h(t)=e(T−t)k+n[1−e−(T−t)√α2λ41−4βλ21] is a decreasing function with respect to variable t∈[0,T], so sup0≤t≤Th(t)=h(0). This leads to
sup0≤t≤Th2(t)α2λ4n−4βλ2n=e2Tk+n[1−e−T√α2λ41−4βλ21]2α2λ4n−4βλ2n≥e2Tλn[1−e−T√α2λ41−4βλ21]2α2λ4n−4βλ2n. | (4.9) |
Combining (4.7)–(4.9) yields
E‖˜un(⋅,t)‖2H≥89e2Tλn[1−e−T√α2λ41−4βλ21]2α2λ4n−4βλ2nϵ2≥89e2Tn2[1−e−T√α2−4β]2α2n8−4βn4ϵ2. |
Let us choose n(ϵ):=n=√12Tln(1ϵ3). When ϵ→0+, we have E‖˜ξϵn‖2H=ϵ2n(ϵ)→0. However,
E‖˜un‖2C([0,T];L2(Ω))=891ϵ[1−e−T√α2−4β]2α2[12Tln(1ϵ3)]4−4β[12Tln(1ϵ3)]2→+∞. |
Thus, we can conclude that Problem (1.1)–(1.3) with random noise (1.4) which is ill-posed in the sense of Hadamard.
To come up with a regularized solution, we first denote a truncation operator 1Nf=∑Np=1⟨f,φp⟩φp(x) for all f∈H. Now, let us consider a problem as follows
{˜UNtt+αΔ2˜UNt+βΔ2˜UN=1NF(x,t,˜UN),(x,t)∈Ω×(0,T),˜UN(x,T)=1N˜ρn(x),x∈Ω,˜UNt(x,T)=1N˜ξϵn(x),x∈Ω,˜UN(x,t)=0,(x,t)∈Γ×(0,T), | (5.1) |
where ˜ρn(x), ˜ξϵn(x) as in Definition 2.2 and N, n are called the regularized parameter and the sample size respectively. Applying Lemma 3.1, Problem (5.1) has the mild solution
˜UN(x,t)=RN(T−t)˜ρϵn(x)−SN(T−t)˜ξϵn(x)+∫TtSN(τ−t)F(x,τ,˜UN)dτ, | (5.2) |
where
RN(t)f=N∑p=1k+petk−p−k−petk+p√Δp⟨f,φp⟩φp(x);SN(t)f=N∑p=1etk+p−etk−p√Δp⟨f,φp⟩φp(x). | (5.3) |
The non-linear integral equation is called the regularized solution of Problem (1.1)–(1.3) with the perturbation random model (1.4). And N serves as the regularization parameter.
Lemma 5.1 ([16,19]). Given f∈H and t∈[0,T]. We have the following estimates:
‖RN(t)f‖2H≤CRe2αtk2N‖f‖2H;‖SN(t)f‖2H≤CSe2αtk2N‖f‖2H. | (5.4) |
where CR, CS are constants dependent on α, T.
Theorem 5.1. Given the functions ρ,ξ∈H. Assume that F∈C(Ω×[0,T]×R) satisfies the globally Lipschitz property with respect to the third variable i.e., there exists a constant L>0 independent of x,t,u1,u2 such that
‖F(⋅,t,u1(⋅,t))−F(⋅,t,u2(⋅,t))‖H≤L‖u1(⋅,t)−u2(⋅,t)‖H. |
Then the nonlinearintegral equation (5.2) has a unique solution ˜UN∈L∞([0,T],L2(˜Ω;H)).
Proof. Define the operator P:L∞([0,T],L2(˜Ω;H))↦L∞([0,T],L2(˜Ω;H)) as following
P(v)(x,t)=RN(T−t)˜ρϵn(x)−SN(T−t)˜ξϵn(x)+∫TtSN(τ−t)F(x,τ,v)dτ. |
For integer m≥1, we shall begin with showing that for any v1,v2∈L∞([0,T],L2(˜Ω;H))
E‖Pm(v1)(⋅,t)−Pm(v2)(⋅,t)‖2H≤[L2CSTe2αTλ2N]m(T−t)mm!‖v1−v2‖2L∞([0,T],L2(˜Ω;H)). | (5.5) |
We now proceed by induction on m. For the base case (m=1),
E‖P(v1)(⋅,t)−P(v2)(⋅,t)‖2H=E‖∫TtSN(τ−t)(F(x,τ,v1)−F(x,τ,v2))dτ‖2H≤TE∫TtCSe2αtλ2N‖F(⋅,τ,v1)−F(⋅,τ,v2)‖2Hdτ≤T(T−t)L2CSe2αTλ2N‖v1−v2‖2L∞([0,T],L2(˜Ω;H)), |
where we apply Lemma 5.1 and the Lipschitz condition of F. Thus it is correct for m=1. For the inductive hypothesis, it is true for m=m0. We show that (5.5) is true for m+1.
E‖Pm+1(v1)(⋅,t)−Pm+1(v2)(⋅,t)‖2H=E‖P(Pm(v1))(⋅,t)−P(Pm(v2))(⋅,t)‖2H=E‖∫TtSN(τ−t)(F(x,τ,Pm(v1))−F(x,τ,Pm(v2)))dτ‖2H≤TE∫TtCSe2αtλ2N‖F(⋅,τ,Pm(v1))−F(⋅,τ,Pm(v2))‖2Hdτ≤[L2CSTe2αTλ2N]m+1‖v1−v2‖2L∞([0,T],L2(˜Ω;H))∫Tt(T−τ)mm!dτ. | (5.6) |
From the inductive hypothesis, we have
E‖Pm+1(v1)(⋅,t)−Pm+1(v2)(⋅,t)‖2H≤[L2CSTe2αTλ2N]m+1‖v1−v2‖2L∞([0,T],L2(˜Ω;H))∫Tt(T−τ)mm!dτ. |
Hence, by the principle of mathematical induction, Formula (5.5) holds. We realize that,
limm→∞[L2CSTe2αTλ2N]mm!=0, |
and therefore, there will exist a positive number m=m0, such that Pm0 is a contraction. It means that Pm0(˜UN)=˜UN has a unique solution ˜UN∈L∞([0,T];L2(˜Ω,H)). This leads to P(Pm0(˜UN))=P(˜UN). Since P(Pm0(˜UN))=Pm0(P(˜UN)), it follows that Pm0(P(˜UN))=P(˜UN). Hence P(˜UN) is a fixed point of Pm0. By the uniqueness of the fixed point of Pm0, we conclude that P(˜UN)=˜UN has a unique solution ˜UN∈L∞([0,T];L2(˜Ω,H)).
Theorem 5.2. Let ρ,ξ∈Hμ, (μ>0). Assume that System (1.1)–(1.3) has the exact solution u∈C([0,T];Gσ,2), where σ>2αT. Given ε>0, the following estimate holds
E‖˜UN(⋅,t)−u(⋅,t)‖2H≤2e−2αtλ2N(2λ−2N‖u‖L∞([0,T];Gσ,2))e2CSL2T(T−t)+2e2α(T−t)λ2N[3CR(ϵ2n+1λμn||ρ||2Hμ)+3CS(ϵ2n+1λμn||ξ||2Hμ)]e3CSL2T(T−t), | (5.7) |
where the regularization parameter N(ϵ):=N and the sample size n(ϵ):=n are choosen such that
limϵ→0+N(ϵ)=+∞,limϵ→0+ϵ2n(ϵ)e2αTλ2N(ϵ)=limϵ→0+e2αTλ2N(ϵ)λμn(ϵ)=0. | (5.8) |
Remark 5.1. The order of convergence of (5.7) is
e−2αtλ2N(ϵ)max{ϵ2n(ϵ)e2αTλ2N(ϵ);e2αTλ2N(ϵ)λμn(ϵ);1λ2N(ϵ)}. | (5.9) |
There are many ways to choose the parameters n(ϵ),N(ϵ), that satisfies (5.8). Since λn(ϵ)∼(n(ϵ))2/d [7], one of the ways we can do by choosing the regularization parameter N(ϵ) such that λN(ϵ) satisfies e2αTλ2N(ϵ)=(n(ϵ))a, where 0<a<2μ/d. Then we obtain λ2N(ϵ)=a2αTln(n(ϵ)). The sample size n(ϵ) is chosen as n(ϵ)=(1/ϵ)b/(a+1), (0<b<2). In this case, the error will be of order
ϵtbT(a+1)max{ϵ2−b;ϵba+1(2μd−a);ab2α(a+1)ln1ϵ}. |
Proof of Theorem 5.2. Let us define the integral equation
uN=RN(T−t)ρ(x)−SN(T−t)ξ(x)+∫TtSN(τ−t)F(x,τ,uN)dτ. |
Then, we have
E‖˜UN(⋅,t)−u(⋅,t)‖2H≤2E‖˜UN(⋅,t)−uN(⋅,t)‖2H+2E‖uN(⋅,t)−u(⋅,t)‖2H. | (5.10) |
For easy tracking, we divide the above estimate into two main steps:
Step 1. We have
E‖˜UN(⋅,t)−uN(⋅,t)‖2H≤3E‖RN(T−t)(˜ρϵn−ρ)‖2H+3E‖SN(T−t)(˜ξϵn−ξ)‖2H+3E‖∫TtSN(τ−t)(F(x,τ,˜UN)−F(x,τ,uN))dτ‖2H. |
By Höder's inequality and the results in Lemma 5.1, we have
E‖˜UN(⋅,t)−uN(⋅,t)‖2H≤3CRe2α(T−t)λ2NE‖˜ρϵn−ρ‖2H+3CSe2α(T−t)λ2NE‖˜ξϵn−ξ‖2H+3TE∫TtCSe2α(τ−t)λ2N‖F(⋅,τ,˜UN)−F(⋅,τ,uN))‖2Hdτ. |
Use the results of Lemma 2.1 and the Lipschitz property of F, we have
E‖˜UN(⋅,t)−uN(⋅,t)‖2H≤3CRe2α(T−t)λ2N(ϵ2n+1λμn||ρ||2Hμ)+3CSe2α(T−t)λ2N(ϵ2n+1λμn||ξ||2Hμ)+3CSL2TE∫Tte2α(τ−t)λ2N‖˜UN(⋅,τ)−uN(⋅,τ)‖2Hdτ. | (5.11) |
Multiplying both sides (5.11) to e2αtλ2N, we derive that
e2αtλ2NE‖˜UN(⋅,t)−uN(⋅,t)‖2H≤3CRe2αTλ2N(ϵ2n+1λμn||ρ||2Hμ)+3CSe2αTλ2N(ϵ2n+1λμn||ξ||2Hμ)+3CSL2TE∫Tte2ατλ2N‖˜UN(⋅,τ)−uN(⋅,τ)‖2Hdτ. |
Gronwall's inequality leads to
e2αtλ2NE‖˜UN(⋅,t)−uN(⋅,t)‖2H≤e2αTλ2N[3CR(ϵ2n+1λμn||ρ||2Hμ)+3CS(ϵ2n+1λμn||ξ||2Hμ)]e3CSL2T(T−t). | (5.12) |
Step 2. To evaluate the remining term, we define the truncation version of the solution u as following
χNu(x,t)=RN(T−t)ρ(x)−SN(T−t)ξ(x)+∫TtSN(τ−t)F(x,τ,u)dτ. |
Then, we have
‖uN(⋅,t)−u(⋅,t)‖2H≤2‖uN(⋅,t)−χNu(⋅,t)‖2H+2‖χNu(⋅,t)−u(⋅,t)‖2H. | (5.13) |
Sub-step 1.1. By Höder's inequality, Lemma 5.1 and the Lipschitz property of F, we have
‖uN(⋅,t)−χNu(⋅,t)‖2H=‖∫TtSN(τ−t)(F(x,τ,uN)−F(x,τ,u))dτ‖2H≤T∫TtCSe2α(τ−t)λ2N‖F(⋅,τ,uN)−F(⋅,τ,u)‖2Hdτ≤CSL2TE∫Tte2α(τ−t)λ2N‖uN(⋅,τ)−u(⋅,τ)‖2Hdτ. | (5.14) |
Since u∈C([0,T];Gσ,2), then
‖χNu(⋅,t)−u(⋅,t)‖2H=∞∑p=N+1⟨u(⋅,t),φp⟩2≤e−2αtλNλ−2N∞∑p=N+1e2αtλpλ2p⟨u(⋅,t),φp⟩2≤e−2αtλNλ−2N‖u(⋅,t)‖L∞([0,T];Gσ,2). | (5.15) |
Substituting (5.14) and (5.15) into (5.13), we have
‖uN(⋅,t)−u(⋅,t)‖2H≤2CSL2TE∫Tte2α(τ−t)λ2N‖uN(⋅,τ)−u(⋅,τ)‖2Hdτ+2e−2αtλNλ−2N‖u‖L∞([0,T];Gσ,2). |
Multiplying both sides above formula to e2αtλN, we have
e2αtλ2N‖uN(⋅,t)−u(⋅,t)‖2H≤2CSL2TE∫Tte2ατλ2N‖uN(⋅,τ)−u(⋅,τ)‖2Hdτ+2λ−2N‖u‖L∞([0,T];Gσ,2). |
Using Gronwall's inequality, we obtain
e2αtλ2N‖uN(⋅,t)−u(⋅,t)‖2H≤(2λ−2N‖u‖L∞([0,T];Gσ,2))e2CSL2T(T−t). | (5.16) |
The proof is completed by combining (5.10), (5.12) and (5.16).
We propose the general scheme of our numerical calculation. For simplicity, we fix T=1 and Ω=(0,π). The eigenelements of the Dirichlet problem for the Laplacian in Ω have the following form:
φp=√2πsin(px),λp=p2, for p=1,2,… |
To find a numerical solution to Eq (5.2), we first need to define a set of Nx×Nt grid points in the domain Ω×[0,T]. Let Δx=π/Nx is the time step, Δt=1/Nt is the spatial step, the coordinates of the mesh points are xj=jΔx, j=0,…,Nx, and ti=iΔt, i=0,…,Nt, and the values of the regularized solution ˜UN(x,t) at these grid points are ˜UN(xj,ti)≈˜Uij, where we denote ˜Uij by the numerical estimate of the regularized solution ˜UN(x,t) of at the point (xj,ti).
Initialization step. The numerical process starts when time t=T. Since ˜UN(x,T)=RN(0)˜ρϵn, then
˜UNtj≈˜UN(x,T)=N∑p=1⟨˜ρϵn,φp⟩φp(xj)=N∑p=1⟨ρϵ,φp⟩φp(xj),j=1,…,Nx. | (6.1) |
Iteration steps. For ti<T, we want to determine
˜UN(x,ti)=RN(T−ti)˜ρϵn−SN(T−ti)˜ξϵn+∫TtiSN(τ−ti)F(˜UN)(τ)dτ⏟I(ti), | (6.2) |
where I(ti) is performed in backward time as following
I(ti)=N∑p=1[∫Ttie(τ−ti)k+p−e(τ−ti)k−p√ΔpFp(˜UN)(τ)dτ]φp(x)=N∑p=1[Nt−1∑k=i∫tk+1tke(τ−ti)k+p−e(τ−ti)k−p√ΔpFp(˜UN)(tk+1)dτ]φp(x). |
It is worth pointing out that, the Simpson's rule leads to the approximation
Fp(˜UN)(ti)=⟨F(˜UN)(⋅,ti),φp⟩≈Δ3Nx∑h=1Ch[F(˜UN(xh,ti))φp(xh)], |
where
Ch={1,if h=0 or h=Nx,2,if h≠0,h≠Nx and h is odd,4,if h≠0,h≠Nx and h is even. |
Error estimation. We use the absolute error estimation between the regularized solution and the exact solution as follows
Err(ti)=(1Nx+1Nx∑j=0|u(xj,ti)−˜UN(xj,ti)|2)1/2. | (6.3) |
In this example, we fixed α=0.3, β=0.01 and present the inputs
ρ(x)=e−2sinx+e−1sin2x;ξ(x)=−2e−2sinx−e−1sin2x, |
and source data F(x,t,u)=f(x,t)+11+u2, where
f(x,t)=(4−2α+β)(e−2tsinx+e−4tsinxsin22x+e−6tsin3x+2e−5tsin2xsin2x)1+e−2tsin22x+e−4tsin2x+2e−3tsinxsin2x+(1−16α+16β)(e−tsin2x+e−3tsin22x+e−5tsinxsin2x+2e−4tsinxsin22x)1+e−2tsin22x+e−4tsin2x+2e−3tsinxsin2x. |
It is easy to check that the exact solution of Problem (1.1)–(1.3) is given by u(x,t)=e−tsin2x+e−2tsinx.
Figure 1 compares ρ(x), ξ(x) with their estimates ˜ρϵn(x), ˜ξϵn(x), respectively. When ϵ tends to 0, the estimates are consistent with that of the exact ones. Figure 2 presents a 3D graph of the exact solution u and the regularized solution for the case ϵ=1E−03. Figure 3 displays the numerical convergence for different values of ϵ and t.
Table 1 shows the values of Err(t) from (6.3) calculated numerically. As a conclusion, our proposed regularization method works properly and the numerical solution method is also feasible in practice.
ϵ | Err(14) | Err(12) | Err(34) |
5E−01 | 1.071213E+00 | 2.464307E−01 | 1.124781E−01 |
1E−01 | 3.161161E−02 | 5.976654E−03 | 2.063242E−03 |
1E−02 | 1.143085E−04 | 1.761839E−05 | 4.912298E−05 |
1E−03 | 5.851911E−06 | 2.820096E−07 | 2.488333E−10 |
This research is supported by Industrial University of Ho Chi Minh City (IUH) under grant number 130/HD-DHCN. Nguyen Anh Tuan thanks the Van Lang University for the support.
The authors declare no conflict of interest.
[1] |
M. Aassila, A. Guesmia, Energy decay for a damped nonlinear hyperbolic equation, Appl. Math. Lett., 12 (1999), 49–52. https://doi.org/10.1016/S0893-9659(98)00171-2 doi: 10.1016/S0893-9659(98)00171-2
![]() |
[2] |
G. Chen, F. Da, Blow-up of solution of Cauchy problem for three-dimensional damped nonlinear hyperbolic equation, Nonlinear Anal. Theor., 71 (2009), 358–372. https://doi.org/10.1016/j.na.2008.10.132 doi: 10.1016/j.na.2008.10.132
![]() |
[3] |
P.-L. Chow, I. A. Ibragimov, R. Z. Khasminskii, Statistical approach to some ill-posed problems for linear partial differential equations, Probab. Theory Relat. Fields, 113 (1999), 421–441. https://doi.org/10.1007/s004400050212 doi: 10.1007/s004400050212
![]() |
[4] |
C. Cao, M. A. Rammaha, E. S. Titi, Gevrey regularity for nonlinear analytic parabolic equations on the sphere, J. Dyn. Differ. Equ., 12 (2000), 411–433. https://doi.org/10.1023/A:1009072526324 doi: 10.1023/A:1009072526324
![]() |
[5] |
G. Chen, Y. Wang, Z. Zhao, Blow-up of solution of an initial boundary value problem for a damped nonlinear hyperbolic equation, Appl. Math. Lett., 17 (2004), 491–497. https://doi.org/10.1016/S0893-9659(04)90116-4 doi: 10.1016/S0893-9659(04)90116-4
![]() |
[6] |
C. Foias, R. Temam, Gevrey class regularity for the solutions of the Navier-Stokes equations, J. Funct. Anal., 87 (1989), 359–369. https://doi.org/10.1016/0022-1236(89)90015-3 doi: 10.1016/0022-1236(89)90015-3
![]() |
[7] | L. Hörmander, Linear partial differential operators, Berlin, Heidelberg: Springer, 1963. https://doi.org/10.1007/978-3-642-46175-0 |
[8] |
A. Imani, D. Ganji, H. B. Rokni, H. Latifizadeh, E. Hesameddini, M. H. Rafiee, Approximate traveling wave solution for shallow water wave equation, Appl. Math. Model., 36 (2012), 1550–1557. https://doi.org/10.1016/j.apm.2011.09.030 doi: 10.1016/j.apm.2011.09.030
![]() |
[9] |
W. Liu, K. Chen, Existence and general decay for nondissipative hyperbolic differential inclusions with acoustic/memory boundary conditions, Math. Nachr., 289 (2016), 300–320. https://doi.org/10.1002/mana.201400343 doi: 10.1002/mana.201400343
![]() |
[10] |
J. C. Maxwell, Ⅷ. A dynamical theory of the electromagnetic field, Phil. Trans. R. Soc., 155 (1865), 459–512. https://doi.org/10.1098/rstl.1865.0008 doi: 10.1098/rstl.1865.0008
![]() |
[11] |
P. Mathé, S. V. Pereverzev, Optimal discretization of inverse problems in hilbert scales. Regularization and self-regularization of projection methods, SIAM J. Numer. Anal., 38 (2001), 1999–2021. https://doi.org/10.1137/S003614299936175X doi: 10.1137/S003614299936175X
![]() |
[12] | D. Picard, G. Kerkyacharian, Estimation in inverse problems and second-generation wavelets, In: Proceedings of the international congress of mathematicians, European Mathematical Society Publishing House, Madrid, August 22–30, 2006,713–739. https://doi.org/10.4171/022-3/37 |
[13] | A. D. Pierce, Acoustics: an introduction to its physical principles and applications, Cham: Springer, 2019. https://doi.org/10.1007/978-3-030-11214-1 |
[14] |
N. D. Phuong, N. H. Tuan, D. Baleanu, T. B. Ngoc, On Cauchy problem for nonlinear fractional differential equation with random discrete data, Appl. Math. Comput., 362 (2019), 124458. https://doi.org/10.1016/j.amc.2019.05.029 doi: 10.1016/j.amc.2019.05.029
![]() |
[15] |
C. Song, Z. Yang, Existence and nonexistence of global solutions to the cauchy problem for a nonlinear beam equation, Math. Method. Appl. Sci., 33 (2010), 563–575. https://doi.org/10.1002/mma.1175 doi: 10.1002/mma.1175
![]() |
[16] |
N. H. Tuan, V. V. Au, N. H. Can, Regularization of initial inverse problem for strongly damped wave equation, Appl. Anal., 97 (2018), 69–88. https://doi.org/10.1080/00036811.2017.1359560 doi: 10.1080/00036811.2017.1359560
![]() |
[17] |
N. H. Tuan, D. Baleanu, T. N. Thach, D. O'Regan, N. H. Can, Final value problem for nonlinear time fractional reaction-diffusion equation with discrete data, J. Comput. Appl. Math., 376 (2020), 112883. https://doi.org/10.1016/j.cam.2020.112883 doi: 10.1016/j.cam.2020.112883
![]() |
[18] |
N. A. Triet, T. T. Binh, N. D. Phuong, D. Baleanu, N. H. Can, Recovering the initial value for a system of nonlocal diffusion equations with random noise on the measurements, Math. Method. Appl. Sci., 44 (2021), 5188–5209. https://doi.org/10.1002/mma.7102 doi: 10.1002/mma.7102
![]() |
[19] |
N. H. Tuan, D. V. Nguyen, V. V. Au, D. Lesnic, Recovering the initial distribution for strongly damped wave equation, Appl. Math. Lett., 73 (2017), 69–77. https://doi.org/10.1016/j.aml.2017.04.014 doi: 10.1016/j.aml.2017.04.014
![]() |
[20] |
N. H. Tuan, E. Nane, D. O'Regan, N. D. Phuong, Approximation of mild solutions of a semilinear fractional differential equation with random noise, Proc. Amer. Math. Soc., 148 (2020), 3339–3357. https://doi.org/10.1090/proc/15029 doi: 10.1090/proc/15029
![]() |
[21] |
G. F. Wheeler, W. P. Crummett, The vibrating string controversy, Am. J. Phys., 55 (1987), 33–37. https://doi.org/10.1119/1.15311 doi: 10.1119/1.15311
![]() |
[22] |
G. A. Zou, B. Wang, Stochastic Burgers' equation with fractional derivative driven by multiplicative noise, Comput. Math. Appl., 74 (2017), 3195–3208. https://doi.org/10.1016/j.camwa.2017.08.023 doi: 10.1016/j.camwa.2017.08.023
![]() |
1. | Khalid K. Ali, K. R. Raslan, Amira Abd-Elall Ibrahim, Mohamed S. Mohamed, On study the fractional Caputo-Fabrizio integro differential equation including the fractional q-integral of the Riemann-Liouville type, 2023, 8, 2473-6988, 18206, 10.3934/math.2023925 |
ϵ | Err(14) | Err(12) | Err(34) |
5E−01 | 1.071213E+00 | 2.464307E−01 | 1.124781E−01 |
1E−01 | 3.161161E−02 | 5.976654E−03 | 2.063242E−03 |
1E−02 | 1.143085E−04 | 1.761839E−05 | 4.912298E−05 |
1E−03 | 5.851911E−06 | 2.820096E−07 | 2.488333E−10 |